the Creative Commons Attribution 4.0 License.
the Creative Commons Attribution 4.0 License.
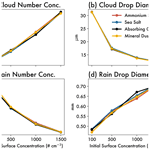
Aerosol–cloud impacts on aerosol detrainment and rainout in shallow maritime tropical clouds
Gabrielle R. Leung
Stephen M. Saleeby
G. Alexander Sokolowsky
Sean W. Freeman
Susan C. van den Heever
This study investigates how aerosol-induced changes to cloud properties subsequently influence the overall aerosol budget through changes to detrainment and rainout. We simulated an idealized field of shallow maritime tropical clouds using the Regional Atmospheric Modeling System (RAMS) and varied the aerosol loading and type between 16 simulations. The full aerosol budget was tracked over the course of the 48 h simulation, showing that increasing the aerosol loading leads to an increase in aerosol regeneration and detrainment aloft at the expense of aerosol removal via rainout. Under increased aerosol loadings, cloud droplets are smaller and more likely to evaporate before they form precipitation-sized hydrometeors. As a result, the aerosol particles contained inside these droplets are released into the environment rather than being removed to the surface via rainout. However, the few raindrops which do happen to form under increased aerosol loadings tend to be larger, since the cloud water available for collection is divided among fewer raindrops, and thus raindrops experience less evaporation. Thus, in contrast to previous work, we find that increases in aerosol loading lead to decreases in aerosol rainout efficiency, even without a decrease in the overall precipitation efficiency. We further used tobac, a package for tracking and identifying cloud objects, to identify shifts in the overall cloud population as a function of aerosol loading and type, and we found contrasting aerosol effects in shallow cumulus and congestus clouds. Shallow cumulus clouds are more sensitive to the increase in cloud edge and/or top evaporation with increased aerosol loading and thereby tend to rain less and remove fewer aerosols via rainout. On the other hand, larger congestus clouds are more protected from evaporation and are thereby able to benefit from warm-phase invigoration. This leads to an increase in rain rates but not in domain-wide aerosol rainout, as the domain total rainfall becomes concentrated over a smaller horizontal area. Trends as a function of aerosol loading were remarkably consistent between the different aerosol types tested. These results represent a pathway by which a polluted environment not only has higher aerosol loadings than a pristine one but is also less able to regulate those loadings by removal processes, instead transporting aerosols to the free troposphere where they remain available for reactivation and further aerosol–cloud interactions.
-
Please read the editorial note first before accessing the article.
-
Article
(5983 KB)
-
Supplement
(1865 KB)
-
Please read the editorial note first before accessing the article.
- Article
(5983 KB) - Full-text XML
-
Supplement
(1865 KB) - BibTeX
- EndNote
Clouds play an important role in governing the atmospheric aerosol budget through a number of simultaneous processes that transport, modify, and remove aerosols. One example of such a process occurs when aerosols are transported between the boundary layer and the free troposphere in cloud updrafts and downdrafts (Cotton et al., 1995; Engström et al., 2008; Chen et al., 2012; Twohy et al., 2017; Savre, 2021; Bardakov et al., 2022). Another such process involves aerosol particles being removed from the free atmosphere and undergoing heterogeneous chemistry when they are activated as cloud condensation nuclei (CCN) or are otherwise intercepted by hydrometeors (i.e. impaction; Feingold and Kreidenweis, 2000; Hegg et al., 2004; Ervens et al., 2018). Cloud processes near cloud tops and cloud edges may further impact the aerosol budget through the evaporation of hydrometeors, which detrains aerosol particles and impacts aerosol number concentrations and vertical distributions (Herbener et al., 2016; Corr et al., 2016; Leung and van den Heever, 2022). Alternatively, in regions where different microphysical processes are dominant, hydrometeors may grow to become precipitation-sized and fall to the surface, thus removing aerosol particles from the atmosphere via wet deposition (i.e. rainout or washout; Radke et al., 1980; Kipling et al., 2016).
While clouds transport and remove aerosols, aerosols can in turn influence cloud properties both directly and indirectly. Aerosol particles scatter and/or absorb radiation (direct effect), which can alter cloud development through changes to surface fluxes and atmospheric stability (McCormick and Ludwig, 1967; Atwater, 1970; Kim et al., 2014; Grant and van den Heever, 2014; Lee et al., 2014; Park and van den Heever, 2022; Sokolowsky et al., 2022). Aerosol particles have also been found to influence the microphysical properties of clouds (indirect effect), with impacts on cloud lifetimes, cloud types, and the overall cloud fraction (Twomey, 1977; Albrecht, 1989; Tao et al., 2012), as well as precipitation efficiency (Jiang et al., 2010; Dagan et al., 2015). Perturbations to the aerosol environment can also drive changes in the atmospheric circulation, with local, regional, and global impacts on cloud regimes (van den Heever et al., 2011; Haywood et al., 2013; Grant and van den Heever, 2014; Kim et al., 2016; Herbert et al., 2021; Williams et al., 2022; Dagan, 2022; Park and van den Heever, 2022; Leung and van den Heever, 2023a).
Assessing the overall change to the aerosol budget for a given perturbation to aerosol–cloud interactions is complex. Aerosols may induce changes to clouds and precipitation; however, these changes to clouds and precipitation may, in turn, influence the aerosol field, although findings in the literature appear to be mixed. For example, Cui and Carslaw (2006) found that increases in aerosol loading led to decreases in the efficiency of both precipitation and wet scavenging by deep convective clouds. On the other hand, while Lee and Feingold (2010) found similar trends for stratiform clouds, they determined that aerosol loading had only a minor impact on convective precipitation and scavenging efficiencies. Discrepancies such as these are difficult to resolve given the numerous cloud and aerosol processes involved. Furthermore, differences in simulated environment, aerosol type, and cloud types may also influence aerosol–cloud interactions (Khain et al., 2008; Fan et al., 2009; van den Heever et al., 2011; Altaratz et al., 2014; Grant and van den Heever, 2014; Gryspeerdt et al., 2014; Glassmeier and Lohmann, 2016; Jiang et al., 2018; Dagan and Stier, 2020). Despite these uncertainties, understanding how aerosol–cloud interactions impact the many processes controlling the aerosol budget – as well as which of those impacts are most relevant for a given cloud scene – is essential for representing realistic aerosol distributions and thus for assessing the ultimate aerosol impacts on weather and climate (Haywood and Boucher, 2000; Samset and Myhre, 2011; Boucher et al., 2013).
In this work, our goal is to examine how aerosol impacts on shallow maritime tropical clouds feed back to the aerosol budget via changes to aerosol rainout and aerosol detrainment. More specifically, we aim to answer the following two questions: (1) how does the proportion of aerosol particles that are rained-out versus those that are detrained aloft change as aerosol loading increases? And (2) how do the changes to the aerosol budget arising from rainout and detrainment vary as a function of aerosol type? We address these questions using a large set of high-resolution simulations of an idealized cloud field under a range of aerosol loadings and types, as described in Sect. 2. Trends in domain-wide aerosol budget, cloud properties, and microphysical process rates are presented in Sect. 3, while a state-of-the-art cloud-tracking package is used to separate trends among different cloud types in Sect. 4. Finally, the role of aerosol type is discussed in greater detail in Sect. 5.
2.1 Model description and configuration
The Regional Atmospheric Modelling System (RAMS version 6.3.03) is a three-dimensional, non-hydrostatic, cloud-resolving model with a two-moment bin-emulating microphysics scheme (Pielke et al., 1992; Cotton et al., 2003; Saleeby and van den Heever, 2013). Details of the model grid configuration, initial conditions, and parameter settings are described in Table 1. The RAMS model configuration used here was identical to that in Leung and van den Heever (2022), though the vertical grid is extended to reach from the surface to ∼23 km in altitude. The high horizontal, vertical, and temporal resolution (Δx=100 m, Δz= 50–300 m Δt=0.75 s) allowed the model to resolve large turbulent eddies and to represent a wide range of convection over the two diurnal cycles (48 h) that were simulated. The domain was located entirely over the ocean, and the simulation was initialized using a combination of dropsonde observations from the Cloud, Aerosol, and Monsoon Processes Philippines Experiment (CAMP2Ex; Reid et al., 2023) and ECMWF Reanalysis-5 (ERA-5) data as described in Leung and van den Heever (2022). After initialization, the model was allowed to evolve freely without additional large-scale forcing, though the solar insolation varied according to the diurnal cycle. As such, these simulations serve as an idealized representation of the microphysical, dynamical, and radiative processes driving maritime tropical convection.
The aerosol field was initialized homogenously in the horizontal direction at the first model time step but decays exponentially in the vertical direction with a scale height of 7 km. In all simulations, ice-nucleating particles (INPs) were also initialized with concentrations starting at 0.01 particles cm−3 at the surface and with the same vertical structure as the aerosol field. Both aerosol–radiation and microphysics–radiation interactions (liquid and ice phase) were included in the simulation. The aerosol budget capabilities in RAMS allowed for the tracking of aerosol number and mass in the following categories: (1) unactivated, (2) in-hydrometeor, (3) regenerated, and (4) wet-deposited or rained-out aerosol (Saleeby and van den Heever, 2013). A schematic depicting the processes governing the exchange between these categories is shown in Fig. 1. Upon initialization, all aerosol particles were initially categorized as unactivated aerosol, i.e. aerosol particles which have not yet been activated in cloud droplets. Over time, the aerosol number and mass concentration fields changed freely as particles were advected around the domain. If the aerosol particles were entrained into an updraft and encountered sufficient supersaturations to activate and serve as CCN and/or INPs, they were transferred to the in-cloud aerosol category. As water mass was transferred between hydrometeor species (i.e. cloud, drizzle, rain, ice, snow, aggregates, hail, and graupel), a corresponding fraction of aerosol was also transferred. Under subsaturated conditions, the hydrometeors evaporated, and the aerosol particles acting as CCN and/or INPs were returned to the environment as regenerated aerosol. Finally, if aerosol particles were contained within raindrops which fell to the ground (either because they were activated as CCN or because they were intercepted by a hydrometeor), they were transferred to a category tracking the accumulated aerosol mass reaching the surface via wet deposition or rainout.
2.2 Experiment set-up
The full set of simulations presented here consists of 16 simulations in which four aerosol loadings and four aerosol types were varied (Table 2). The four aerosol loadings tested span the observed range of aerosol loadings during the CAMP2Ex field campaign, from clean to highly polluted environments (Reid et al., 2023). Throughout the rest of this paper, the different aerosol loading runs are denoted by the initial aerosol number concentration at the surface (which was also the maximum aerosol number concentration initialized in each column), namely 100, 500, 1000, and 1500 cm−3. The four aerosol types tested were ammonium sulfate, sea salt, mineral dust, and absorbing carbon. These aerosol types have varying median particle sizes, solubility and hygroscopicity, and radiative properties. The aerosol size distribution was represented as a single log-normal mode with a shape factor of 1.8 (Reid et al., 2023), with the median particle size depending on the aerosol type, as specified in Table 2. Other key properties of the different aerosol types are also listed in Table 2, and corresponding aerosol optical depths (AODs) are provided in Fig. S1 in the Supplement.
2.3 Cloud identification and tracking
Individual cloud updrafts in each simulation were identified and tracked using the Tracking and Object-Based Analysis of Clouds (tobac version 1.5.0 release candidate 1) package (Heikenfeld et al., 2019; Sokolowsky et al., 2022). We provide a basic description of how tobac works (feature identification, tracking, and segmentation), but for a more comprehensive description, we direct readers to the two papers cited above. We use the term “feature” to refer to an updraft region at a given time step and “cell” to refer to a given cloud feature tracked across time. First, updraft features were identified at each analysis time step (at a frequency of 5 min) based on the three-dimensional vertical velocity field. Regions of local maximum vertical velocity were identified as features at three threshold values (1, 3, and 5 m s−1), and a centroid position was assigned to each feature. Secondly, features in subsequent time steps were linked based on their inferred motion to create a cell with a trajectory over time. Any cells which had a lifetime of less than 5 min (i.e. cells which were tracked for only a single analysis time step) were excluded from the analysis as part of the quality control (QC). Finally, a contiguous three-dimensional cloudy region (where cloud condensate was above 0.01 g kg−1) was identified around each updraft feature using the tobac watershed segmentation technique. We excluded any updraft features that were not associated with a cloudy region as part of the QC. Cloud top and base altitudes, as well as cloud areas and volumes, were calculated based on the size of this cloudy region. Similar segmentation was performed on the two-dimensional surface precipitation and aerosol rainout rates in order to identify the size of the raining and/or rainout area associated with each cloud. Based on these identified features and cells, the mean and maximum values for variables such as rain rate, aerosol rainout rate, and updraft velocity were calculated for each cloud over a given time step and over its entire lifetime. Other studies have used previous versions of tobac to effectively track cloud objects in a similar manner (e.g. Marinescu et al., 2021), but recent improvements to tobac (Sokolowsky et al., 2022) have specifically allowed for cloud objects to be identified and tracked in three dimensions and across periodic boundaries, as was necessary for this study.
It should be noted that, in this work, we are tracking on updrafts, meaning that clouds at the very beginning or end of their life cycles with updrafts weaker than 1 m s−1 are necessarily excluded from the analysis. This is a limitation of tracking packages that use a physically informed threshold to detect features; however, since tobac allows for setting multiple thresholds, we have set a fairly low minimum threshold in order to capture the majority of the cloud lifetime. Although there are numerous updrafts with maximum vertical velocities below 1 m s−1 present in the simulation, we found that such weak updrafts do not contribute significantly to the precipitation or aerosol budget, accounting for less than 2 % of precipitation and aerosol rainout. Furthermore, after applying all our QC thresholds, we found that a vast majority of the falling-rain and rained-out aerosol (75 %–80%) could be attributed to the remaining features and that this was consistent across all these simulations.
In this section, we examine the differences in domain-wide properties as a function of aerosol loading and type. Qualitatively similar cloud fields develop in all 16 simulations (Fig. S1), consisting primarily of shallow cumulus (with cloud tops ∼ 2–4 km a.g.l.) and congestus (with cloud tops ∼ 4–7 km a.g.l.). Clouds begin to form in the simulations after 6–7 h, and the cloud field develops a variety of cloud morphology and degrees of organization over the next 48 h (e.g. linear groups, scattered and/or isolated clouds, and arc clouds associated with cold pools). Deep convection (with cloud tops >7 km a.g.l.) occurs only sporadically in a handful of the simulations and does not persist in any of them. An example of the cloud scenes typically simulated is shown in Fig. 2.
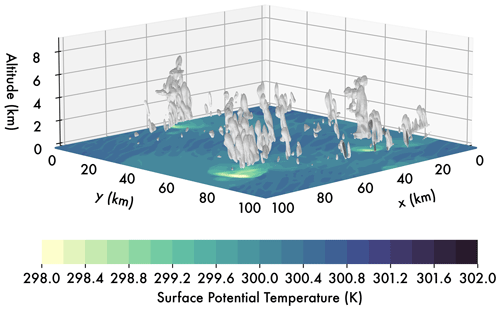
Figure 2Three-dimensional rendering of simulated cloud field at 23:00 UTC (07:00 LT) for the control sea salt simulation (initial number concentration of 100 cm−3 at the surface). Grey isosurfaces are 0.01 g kg−1 of cloud condensate. Surface colours are the potential temperature (K) at the lowest model level above the surface, with lighter colours highlighting the development of cold pools associated with the clouds.
3.1 Domain-wide aerosol budget
The domain-wide trends in the aerosol mass budget are presented in Fig. 3. For each simulation, we integrate the amount of aerosol mass in each of the four budget categories (namely unactivated, in-hydrometeor, regenerated, and rained-out) after 48 h of simulation time, and then we normalize this mass by the total initial mass at the beginning of the model run. In this manner, we quantify the percentage of aerosol mass that is apportioned to each budget category, thus providing a fairer comparison between simulations of different initial aerosol loading. If changes to the aerosol loading have no impacts on the cloud field and cloud processes impacting aerosol particles, then we would expect the same distribution of aerosol mass across the budget categories, irrespective of aerosol loading. We have chosen here to present an aerosol mass budget rather than a number budget, since aerosol mass is conserved after activation and subsequent regeneration of aerosol particles, whereas all aerosol number is not conserved when multiple aerosol particles are collected by a single hydrometeor (i.e. it is assumed that interstitial aerosol particles that are collected by a droplet cohere with the original activated particle). Considering aerosol mass therefore allows us to specifically account for all the transfers between aerosol budget categories. Over the course of the simulation, we found that less than 5 % of the aerosol mass is not tracked and is treated as a residual that is lost due to dry deposition (which is represented in RAMS but not tracked in the RAMS aerosol budget) and/or numerical diffusion; this residual proportion is very similar across all our simulations (Fig. S2).
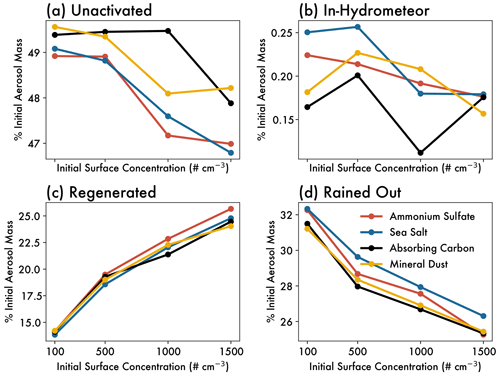
Figure 3Domain aerosol mass budget after 48 h of simulation time represented as a function of the initial aerosol surface concentration (particles cm−3) and aerosol type. Each aerosol budget term is the domain-integrated aerosol mass in a given category normalized by the total aerosol mass at initialization time, shown for (a) unactivated, (b) in-hydrometeor, (c) regenerated, and (d) rained-out aerosol. See the text for explanations of each aerosol category.
Across all the simulations, about half of the initial aerosol mass serves as CCN at some point in time, while the other half remains unactivated (Fig. 3a) for the duration of the simulation. As aerosol loading increases, the fraction of unactivated aerosol generally decreases (the fraction of activated aerosol increases), though changes are fairly small compared to the trends in the other aerosol budget categories. At first glance, this appears to contradict classic cloud parcel theory, wherein the activated fraction decreases (unactivated fraction increases) as aerosol number concentration goes up for a given updraft speed (Reutter et al., 2009). In this scenario, the increased CCN number concentrations lead to increases in cloud droplets competing to consume the available supersaturation. Thus, that parcel's maximum supersaturation is lower compared to a parcel with fewer aerosol particles and an identical updraft speed, and it is therefore unable to activate the smallest aerosol particles, which subsequently drives down the activated aerosol fraction. However, it is important to note that the results we present in Fig. 3 are not from a single cloud parcel but rather are integrated over the whole cloud field and therefore incorporate any aerosol effects that lead to changes in the updraft speed, ambient relative humidity, and cloud types. We discuss these changes to the broader cloud population in greater detail in Sect. 4. There is also a slight decrease in the aerosol mass found inside cloud droplets or embedded in drizzle or raindrops (Fig. 3b), though the trend is non-monotonic and inconsistent between aerosol types. However, the in-hydrometeor category comprises a relatively small percent of the overall aerosol budget at any given time step, and the trends in this category vary temporally (Fig. S2), since aerosol particles are only apportioned to it temporarily before being transferred to the regenerated or rained-out category.
The clearest and most temporally persistent trends in the domain-wide aerosol budget as a function of aerosol loading are seen in the proportion of aerosol mass that is regenerated (Fig. 3c) or rained-out (Fig. 3d). It is clear from these figures that the proportion of regenerated aerosol mass is enhanced, while the proportion of rained-out aerosol mass is decreased with increasing aerosol loading. While Fig. 3 shows a snapshot in time, these trends are largely consistent throughout the course of the simulation (full time series shown in Fig. S2). The opposing trends between the increases in regenerated aerosol and decreases in rained-out aerosol with increasing aerosol loading have similar magnitudes and are on the order of 7 %–10 % of the initial aerosol mass. These trends are remarkably consistent with aerosol type, as will be discussed further in Sect. 5. We emphasize here that the aerosol mass budgets we present are normalized by the initial aerosol loading – in the highest-aerosol-loading case, there is not only a greater absolute mass of aerosol that is regenerated and detrained back into the environment but also actually a larger percentage of mass involved. The changes to rainout, which is the only major aerosol sink in the budget examined here, suggest a positive feedback mechanism by which increases in aerosol loading may actually inhibit the removal of aerosol particles by clouds.
3.2 Cloud and rain microphysics
To investigate the mechanisms by which increased aerosol loading leads to aerosol regeneration being favoured over rainout, we examine trends in the cloud and rain droplet size distributions (Fig. 4). For all aerosol types, increasing the aerosol loading produces more numerous and smaller cloud droplets (Fig. 4a–b), which is consistent with the first indirect effect that has been demonstrated in many observational and modelling studies (Twomey, 1977; Tao et al., 2012). On the other hand, increasing aerosol loading produces fewer and larger raindrops (Fig. 4c–d). This effect has been demonstrated in multiple modelling studies (Berg et al., 2008; Li et al., 2013; Altaratz et al., 2014; Sheffield et al., 2015) with more limited observational support (Berg et al., 2008; May et al., 2011).
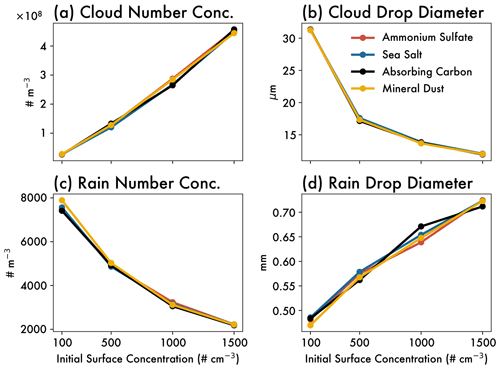
Figure 4In-cloud mean microphysical size distribution properties as a function of initial aerosol surface concentration (particles cm−3) and aerosol type: (a) cloud droplet number concentration (particles m−3), (b) cloud droplet diameter (µm), (c) rain drop number concentration (particles m−3), and (d) rain drop diameter (mm). Values are spatially and temporally averaged over cloudy (cloud condensate >0.01 g kg−1) updraft (vertical velocity >1 m s−1) grid points.
These trends in the droplet size distributions can be more easily connected to the aerosol budget by examining trends in the microphysical process budget, as shown in Fig. 5. Each term in this figure represents a different sink for cloud water and is given as an efficiency relative to the mass of water vapour transferred to liquid in cloud droplets. The value given is the percent of condensed water vapour that ends up in a particular cloud water sink. As aerosol loading increases, the cloud droplets are smaller and thus evaporate more readily (Fig. 5a) and are also less likely to be collected into rainwater (Fig. 5b). This explains the trends in the aerosol budget under increased aerosol loadings to first order; although a similar proportional mass of aerosol particles is activated and enters cloud droplets, those cloud droplets are smaller and tend to evaporate more quickly, which favours regeneration. Simultaneously, those smaller cloud droplets are less likely to be collected as rainwater, and thus the CCN contained within them are less likely to be washed out within precipitation.
In the polluted aerosol environment, although cloud water is less likely to be collected by rain, the cloud water which is collected is distributed among fewer raindrops. These raindrops are thus larger in size (Fig. 4d) and have a lower surface-area-to-volume ratio compared to raindrops that form in pristine aerosol environments, in keeping with past model results (Storer and van den Heever, 2013; Altaratz et al., 2014; Saleeby et al., 2015). As a result, even though increasing aerosol loading leads to less-efficient collection of cloud water into rainwater, it also leads to decreases in rainwater evaporation (Fig. 5c). These two trends partially offset one another, leading to mixed trends in the percentage of cloud water that falls to the surface as rain (Fig. 5d), sometimes defined as the precipitation efficiency (Cui and Carslaw, 2006; Jiang et al., 2010; Lee and Feingold, 2010; Lutsko et al., 2021; Li et al., 2022). These decreases with increasing aerosol loading are not monotonic for all aerosol types, and it is a smaller relative trend compared to those of cloud evaporation and collection. Although increasing the aerosol loading in the domain causes clouds to become less efficient at removing aerosol via rainout (Fig. 3d), it does so without necessarily impacting the precipitation efficiency itself (Fig. 5d). Earlier results by Cui and Carslaw (2006) and Lee and Feingold (2010) showed similar decreases in the aerosol rainout efficiency (or aerosol precipitation efficiency or scavenging efficiency, as they respectively referred to it), but these decreases were closely coupled with decreases in precipitation efficiency. That is to say, they showed that increased aerosol loading caused less-efficient rain formation and therefore less-efficient aerosol removal through rainout. Our results add to this and show that the reduced efficiency in aerosol removal through rainout can occur even without changes to the domain-wide precipitation efficiency.
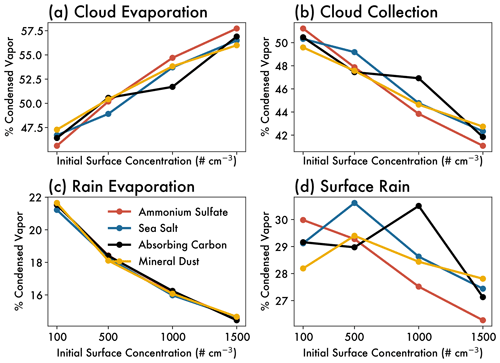
Figure 5Domain total microphysical process rates as a function of initial aerosol surface concentration (particles cm−3) and aerosol type. Each panel shows the process efficiency (in %), defined as the domain- and time-integrated process rate normalized by the domain- and time-integrated condensation rate (water vapour to liquid water), shown for (a) cloud water evaporation, (b) cloud water collection into rain, (c) rainwater evaporation, and (d) surface-accumulated rain (i.e. precipitation efficiency).
4.1 Trends in cloud numbers and median cloud properties
Given the complexity surrounding the number of processes impacting rain formation, which often have opposing trends as a function of aerosol loading, we find that increasing aerosol loading does not necessarily lead to decreases in precipitation efficiency (Fig. 5d) or the domain-wide accumulated precipitation (Fig. 6a). For some aerosol types (e.g. mineral dust), the increase in aerosol loading actually leads to an increase in the accumulated precipitation between the lowest and highest aerosol loadings tested in our set of simulations. The trends in the total number of tracked clouds are similarly non-monotonic and mixed, as shown in Fig. 6b, and suggest that aerosol loading does not have a clear impact on domain cloudiness for these simulations of maritime tropical clouds. That being said, tracking the clouds over their full lifetime with the use of tobac allows us to further subdivide clouds into those that precipitate and those which do not (Fig. 6c–d). To differentiate raining and non-raining clouds, we find the area-mean rain rate associated with each cloud feature at each point in its lifetime, and then we take the lifetime maximum of those rain rates such that non-raining clouds are those which never reach an area-mean rain rate of 0.0001 mm h−1. Clearly, the mixed trend in the total number of clouds as a result of increasing aerosol loading arises as a result of the following two opposing trends: increases in the number of non-precipitating clouds and decreases in the number of precipitating ones. This is, furthermore, consistent with the general picture of aerosol impacts on the cloud field from the process rates, as described above: although clouds still do form in environments with higher aerosol loadings, the cloud droplets evaporate more readily before the cloud is able to produce precipitation-sized particles, and thus a greater fraction of clouds never rain throughout their whole lifetime. Non-raining clouds still activate aerosol particles and regenerate them aloft as the cloud dissipates, but they do not remove them from the domain via rainout, which contributes to aerosol regeneration at the expense of rainout.
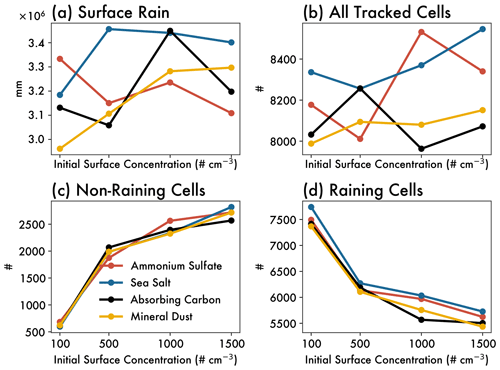
Figure 6(a) Total accumulated rain and (b–d) number of updraft cells tracked over 48 h of simulation using tobac as a function of initial aerosol surface concentration (particles cm−3) and aerosol type. Total numbers of tracked cloud cells are shown in panel (b), while panel (c) shows only non-raining updrafts, and panel (d) shows only raining updrafts. Raining updrafts are defined as those which have a mean rain rate of at least 0.0001 mm h−1 for any time step during their lifetime.
We can use tobac to aggregate the raining clouds (Fig. 7) to see how the median properties of these clouds evolve under different aerosol environments. Although increasing aerosol loading leads to fewer raining clouds, those which do rain are invigorated with higher rain rates (Fig. 7a). This is further compounded by the decrease in the median area covered by each raining cloud (Fig. 7b). Overall, we find that, under higher aerosol loadings, surface rainfall becomes more concentrated amongst fewer clouds with a higher median rain rate. However, this is contrasted by the trends in aerosol rainout rate (Fig. 7c). The aerosol impact is non-monotonic, but there is a decrease in the strength of aerosol rainout between the lowest and highest aerosol loadings for all aerosol types. We conclude that the median cloud rains more under higher aerosol loadings but rains out less aerosol. Shifts in the behaviour of the overall cloud population, as well as potential explanations for this behaviour, are discussed in the following subsection.
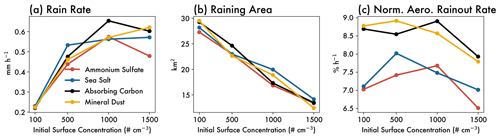
Figure 7Median updraft properties for raining updrafts as a function of initial aerosol surface concentration (particles cm−3) and aerosol type. Panels show (a) rain rate (mm h−1), (b) raining area (km2), and (c) normalized wet deposition rate (% h−1). The normalized wet deposition rate is the percent of initial aerosol mass integrated over a given column that is lost to rainout over a given time period.
4.2 Shifts in cloud population distributions
An advantage of cell tracking over prior analysis methods is the ability to examine trends and characteristics of evolving cloud population distributions as opposed to merely aggregating properties. We construct two-dimensional histograms according to cloud top height (CTH) and either rain rate (Fig. 8) or normalized aerosol rainout rate (Fig. 9), such that the value in each bin is the number of raining clouds with a given CTH and rain rate or rainout rate. The CTH, rain rate, and normalized aerosol rainout rate are defined by taking the lifetime maximum value for each tracked cloud cell, such that they represent the peak maturity for a given cloud. This approach has the benefit of being able to separately identify cloud modes according to different CTHs; in this case, the shallow cumulus mode is clearly visible as a hotspot of clouds with CTHs of ∼2 km, as is the congestus mode with CTHs of ∼4 km (e.g. Fig. 8a). This is particularly important due to our focus on tropical convection, which is known to consist of three separate cloud modes (Johnson et al., 1999; Posselt et al., 2008).
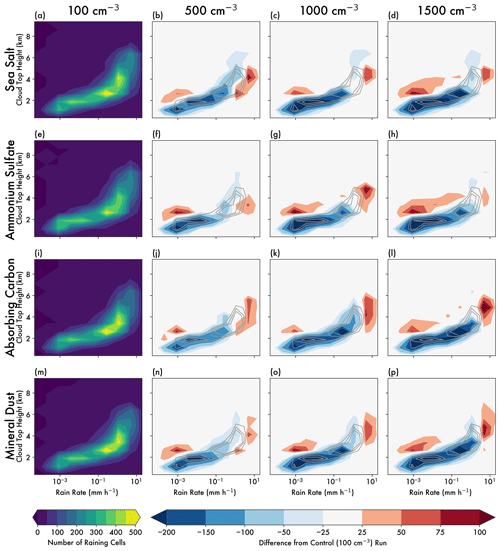
Figure 8Two-dimensional histogram of cloud top height (km) and rain rate (mm h−1) for all tracked raining clouds. Each row is a different aerosol type: (a–d) sea salt, (e–h) ammonium sulfate, (i–l) absorbing carbon, and (m–p) mineral dust. The leftmost column (a, e, i, m) is the control run with the lowest aerosol loading, and coloured contours indicate the number of raining cloud cells in each joint probability bin. The other three columns are given as a difference in the number of cloud cells relative to the control run of the same aerosol type. The overlaid grey contours correspond to n= 250, 300, and 350 in the respective control runs and are drawn to facilitate comparison between the different simulations.
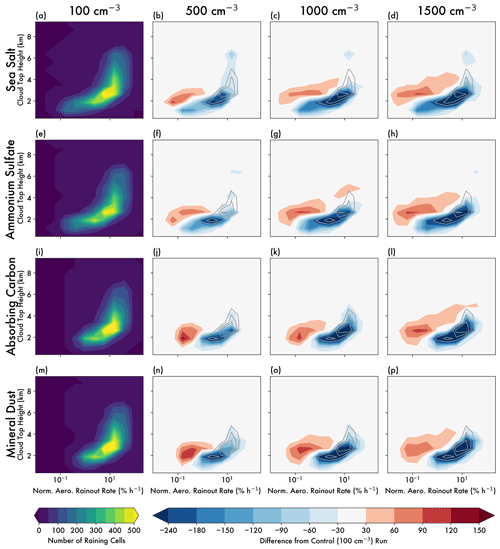
Figure 9As in Fig. 8 but for normalized aerosol rainout rate. The normalized aerosol rainout rate is the percent of initial aerosol mass integrated over a given column that is lost to rainout over a given time period. The overlaid grey contours in the three rightmost columns are n= 300, 400, and 500 in the respective control runs.
Generally, there is a positive correlation between CTH and precipitation rate, with taller clouds tending to have stronger rain rates than shallower clouds do (Adler and Mack, 1984; Smalley and Rapp, 2020). Moving from left to right in each row in Fig. 8 allows one to see the impact of increasing aerosol loadings on the CTH–rain-rate distribution, such that negative values (blue regions) are portions of the cloud population that become less frequent with increasing aerosol loading and vice-versa for positive values (red regions). Notably, increased aerosol loadings impact shallow cumulus and congestus clouds in opposite ways, emphasizing that median cloud properties over all types of clouds are insufficient for quantifying the magnitude of the aerosol effect. Shallow cumulus clouds tend to grow taller, with the modal CTH being closer to 3 km in the highest aerosol simulations, but they do so with reduced rain rates (e.g. Fig. 8d) in a manner that is similar to the precipitation suppression effect described in many past studies (e.g. Xue et al., 2008; Spill et al., 2019). On the other hand, congestus clouds also grow slightly taller but have much stronger modal rain rates, which is consistent with previous findings on the warm-phase invigoration of this tropical-cloud mode (Li et al., 2013; Sheffield et al., 2015). These results suggest that the smaller shallow cumulus clouds are more sensitive to the increase in evaporation (Fig. 5a), whereas congestus clouds with larger areas are more able to protect the interior of the cloud core from evaporation, thereby benefitting from the increase in latent heating or warm-phase invigoration associated with increased cloud droplet formation (Fig. 4a). Furthermore, this shows that the impact of increasing aerosol on precipitation efficiency is dependent on cloud type. This may explain differences between this study and recent studies such as that of Dagan (2022), which saw monotonic increases in precipitation efficiency with increasing aerosol. The latter study used a coarser grid spacing that did not resolve the shallow cumulus cloud field which we find in this work to have decreasing precipitation efficiency with increasing aerosol.
The CTH–aerosol-rainout-rate distribution in Fig. 9 clearly shows deeper clouds being associated with more aerosol rainout compared to shallower clouds. This relationship is consistent with trends in rain rate with cloud top height, as discussed previously. For shallow cumulus, increased aerosol loading leads to a decrease in their ability to remove aerosol via rainout, which follows closely with the decrease in their rain rates. However, although congestus clouds tend to produce stronger rainfall in higher-aerosol environments, they do not see a corresponding increase in their ability to rainout aerosol, which has no change or even decreases slightly for the highest aerosol loadings. These results suggest that, unlike rain rates, which can be enhanced by warm-phase invigoration, there is something of a saturation effect for rainout. At a certain point, stronger rain rates can no longer increase the amount of aerosol being rained-out, since the aerosol available to rainout in those areas has already been removed to the surface. Because the surface rainfall from these clouds becomes concentrated over smaller horizontal areas where the cloud droplets are sufficiently protected from the environment such that they can form precipitation-sized hydrometeors, there are increasing areas of clear-sky or very light precipitation that is not sufficient to remove aerosol particles to the surface. Thus, the overall aerosol impact on rainout is dominated by the decrease in rainout from shallow clouds which either have weaker rain rates or stop raining altogether. These findings provide strong process-level evidence for a potential mechanism to explain recent results from GCMs showing that frequent, light precipitation is more important than strong precipitation in regulating the amount of wet-deposited aerosols (Wang et al., 2021a, b).
We have shown throughout this paper that the influence of aerosol type on the overall aerosol budget and cloud populations is relatively small. Regardless of aerosol type, increasing aerosol loading leads to similar trends in cloud microphysics, precipitation rates, and the domain-wide aerosol budget, varying only in terms of magnitude. The different aerosol median sizes and hygroscopicities (Table 2) do influence the magnitude of the aerosol rainout, although only minimally, and they do not affect the overall trends. For example, the cloud field overall tends to be more efficient at raining out ammonium sulfate and sea salt (Fig. 3d), both of which have larger particle sizes, which is consistent with these particles having a higher activation fraction, all else being equal (Reutter et al., 2009). Note that comparing among the median cloud in each simulation (Fig. 7c) shows lower normalized aerosol rainout rates for ammonium sulfate and sea salt. This is driven by the tail of the distribution shown in Fig. 9 – since the clouds are more efficient at raining out ammonium sulfate and sea salt, cumulus clouds with low rainout rates are still able to show appreciable and trackable values. However, the integrated impact of all clouds in the field is more accurately shown in Fig. 3d or by looking at the full distribution in Fig. 9, which emphasizes the importance of evaluating changes across the whole cloud distribution and not merely in medians or means across different cloud modes.
We found that cloud properties depend more strongly on the magnitude of aerosol loading rather than on the aerosol type. The lack of variation in the cloud population distribution as a function of aerosol type (as can be observed by comparing across each column in Figs. 8 and 9) was observed despite the strong differences in clear-sky radiative-heating rates as a function of aerosol type (Fig. 10). The differences in heating rates are driven by differences in the absorbing and scattering properties of each aerosol type, with more scattering aerosol, like ammonium sulfate or sea salt, driving cooling trends with increased aerosol and more absorbing aerosol, like absorbing carbon and mineral dust, driving warming and stronger stratification of the stable layers in the domain. However, these differences in radiative heating do not appear to feed back on cloud properties within the domain – at least not on the timescales of our simulation (48 h) – as there is not sufficient time for these differences in direct aerosol effects to influence the overall cloud field. These differences may eventually lead to divergence between aerosol types as the system moves towards radiative–convective equilibrium (RCE), though there is some debate about whether the relatively short lifetimes of these shallow cumulus and congestus clouds allow for such equilibration, and longer-term and realistically forced simulations would be necessary to test this (Dagan et al., 2018). Some past research also suggests that this may be sensitive to the update timescale used for the radiation parameterization (Matsui et al., 2020). We would also expect that the radiative differences between aerosol types would be amplified over a land surface where aerosol-induced differences in the feedbacks to the surface fluxes could play a role; this is in contrast to the ocean surface (here, we have fixed the SST, though we would not expect large aerosol-induced changes in SST over the 48 h of simulation time, even if a fully interactive ocean surface were utilized).
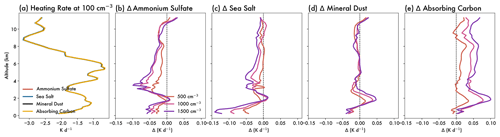
Figure 10(a) Vertical profile of domain-mean clear-sky radiative-heating rates for the lowest-aerosol-loading simulation (initial concentration of 100 particles cm−3 at the surface). Differences relative to the lowest-aerosol-loading simulation are given for each aerosol type: (b) ammonium sulfate, (c) sea salt, (d) mineral dust, and (e) absorbing carbon. Coloured lines indicate different initial aerosol concentrations.
Aerosol–cloud interactions are a key uncertainty in terms of both the overall climate forcing and weather. They are especially challenging to unravel given the large number of processes and feedbacks involved. Here, we have aimed to examine how aerosol impacts on shallow maritime tropical clouds (cumulus and congestus) subsequently modify the aerosol budget, specifically through the removal of aerosol via rainout versus the regeneration of aerosol particles via detrainment aloft. By combining a suite of high-resolution simulations with a comprehensive apportionment of an aerosol budget facilitated by the tobac tracking package, we were able to track both domain-wide trends and the processes driving those trends in different modes of the tropical-cloud population as a function of aerosol loading and aerosol type.
First, we examined trends in the domain-wide aerosol budget and cloud microphysical processes. We found that, regardless of aerosol type, increasing the aerosol loading enhances aerosol regeneration at the expense of rainout. This effectively hinders the cloud field's ability to remove aerosol and thus represents a positive feedback by which increased aerosol loadings may strengthen with time. The increased aerosol regeneration is driven by a decrease in the size of cloud droplets and thus an increase in evaporation. Cloud droplets are therefore increasingly likely to evaporate before the cloud has the chance to form rain and hence remove aerosol through surface deposition. Although there are fewer raindrops that form, each droplet that does form through stochastic collisions with other droplets then has more cloud water available for collection, since a similar amount of cloud water is being divided among fewer raindrops. As a result, these raindrops can be larger and thus experience less evaporation. Previous work has shown increasing aerosol loadings to be associated with decreases in precipitation efficiency and aerosol rainout efficiency (Cui and Carslaw, 2006; Lee and Feingold, 2010) – here, we show that the decrease in the cloud field's efficiency at removing aerosol via rainout can occur even without a corresponding decrease in the overall precipitation efficiency. In other words, even when clouds produce a similar amount of precipitation under higher aerosol loadings due to compensating changes to cloud and rain microphysics, the ability of clouds to remove aerosol via rainout is still hampered because it depends primarily on changes to cloud water collection. Instead, the aerosol particles are regenerated aloft, where they form an aerosol detrainment layer (or potentially, in the case of multilayer clouds, detrainment layers) that can serve as an aerosol source for future midlevel and multilayer clouds (Leung and van den Heever, 2022).
Additionally, we used tobac to track clouds over the course of their lifetime and to generate statistics of cloud properties. We found that increases in aerosol loading lead to more non-raining clouds and fewer raining clouds; this, again, is in keeping with the idea that precipitation is suppressed and that clouds tend to dissipate before they can form rain. Those clouds which do rain tend to have stronger rain rates over a smaller horizontal area, such that precipitation is increasingly concentrated among fewer clouds that are warm-phase invigorated under high-aerosol conditions. Although these clouds then have stronger rain rates, this does not lead to more aerosol rainout overall due to the smaller horizontal area covered by rain leaving a larger clear-sky region where aerosol particles can remain in the atmosphere. This further validates our initial domain-wide analysis showing decreases in aerosol rainout efficiency without changes to precipitation efficiency.
Finally, we examined changes caused by varying aerosol environments as a function of different cloud types and found that a mixed domain-wide trend in rain amounts is driven by contrasting aerosol effects in shallow cumulus and congestus clouds. With increasing aerosol loading, shallow cumulus clouds tend to grow taller and rain less or not at all, whereas congestus clouds only grow slightly taller and tend to have higher rain rates. These changes in rain intensity lead to shallow cumulus clouds being less able to remove aerosol via rainout. Despite congestus having higher rain rates with increased aerosol, there is a saturation effect, such that the more-intense rain no longer increases the efficiency with which aerosol is rained-out, since the rainfall depletes most of the available aerosol even before the rain rates intensify. As a result, the decreases in shallow cumulus precipitation dominate the aerosol effect on the overall amount of aerosol which is removed by rainout. Furthermore, these results underscore that aerosol–cloud interactions can be highly dependent on cloud type, given that the balance between susceptibility to evaporation and warm-phase invigoration depends on the cloud size and dominant microphysical processes and ultimately determines the sign of the precipitation response.
In general, we found that the magnitude of aerosol loading tested in our simulations had a stronger impact on aerosol–cloud impacts than did the aerosol type, despite the clear differences in the radiative-heating rates brought about by the latter. We suggest that the differences in the aerosol direct effect between different aerosol types may pose a stronger impact over longer timescales and/or over land surfaces that have more rapid surface flux feedbacks, and we recommend that future work be undertaken to investigate these scenarios. We also caution that, while these results are robust over this set of simulations with varied aerosol environments, the simulated meteorology only captures a particular maritime tropical environment, and the strength of the aerosol budget response may depend on other factors, including the large-scale meteorology. However, the consistent trends in aerosol impacts on the microphysical processes, the whole cloud population distribution, and the domain aerosol budget suggest that the aerosol–cloud interactions described here may be significant. These interactions represent a pathway by which a polluted environment not only has higher aerosol loadings than a pristine one but is actually less able to regulate those loadings by removing aerosol – instead, the aerosol is convectively transported from the boundary layer to the free troposphere, where aerosol particles remain available for reactivation and further aerosol–cloud interactions.
The source code for RAMS, namelist files, and other information and analysis scripts necessary to reproduce the simulations will be made available at https://doi.org/10.5281/zenodo.7876434 (Leung and van den Heever, 2023b). Source code for the tobac package is available at https://doi.org/10.5281/zenodo.7662376 (tobac Community, 2023).
The supplement related to this article is available online at: https://doi.org/10.5194/acp-23-5263-2023-supplement.
GRL and SCvdH designed the experiments. GRL conducted the RAMS simulations. SMS made developments to RAMS to facilitate the analysis presented here. SWF and GAS made developments to and assisted in the implementation of tobac for the cloud identification and tracking used in the analysis. GRL and SCvdH performed the data analysis and prepared the paper, with contributions and edits from all the co-authors.
The contact author has declared that none of the authors has any competing interests.
Publisher's note: Copernicus Publications remains neutral with regard to jurisdictional claims in published maps and institutional affiliations.
This article is part of the special issue “Cloud, Aerosol and Monsoon Processes Philippines Experiment (CAMP2Ex) (ACP/AMT inter-journal SI)”. It is not associated with a conference.
This research was conducted as part of the CAMP2Ex field campaign. The authors also thank the two anonymous reviewers for their insights and feedback, which greatly improved this quality of this paper.
This research has been supported by the National Aeronautics and Space Administration (NASA) CAMP2Ex project (grant no. 80NSSC18K0149).
This paper was edited by Thijs Heus and reviewed by two anonymous referees.
Adler, R. F. and Mack, R. A.: Thunderstorm Cloud Height–Rainfall Rate Relations for Use with Satellite Rainfall Estimation Techniques, J. Appl. Meteorol. Clim., 23, 280–296, 1984.
Albrecht, B. A.: Aerosols, Cloud Microphysics, and Fractional Cloudiness, Science, 245, 1227–1230, https://doi.org/10.1126/science.245.4923.1227, 1989.
Altaratz, O., Koren, I., Remer, L. A., and Hirsch, E.: Review: Cloud invigoration by aerosols – Coupling between microphysics and dynamics, Atmos. Res., 140–141, 38–60, https://doi.org/10.1016/j.atmosres.2014.01.009, 2014.
Atwater, M. A.: Planetary Albedo Changes Due to Aerosols, Science, 170, 64–66, 1970.
Bardakov, R., Krejci, R., Riipinen, I., and Ekman, A. M. L.: The Role of Convective Up- and Downdrafts in the Transport of Trace Gases in the Amazon, J. Geophys. Res.-Atmos. 127, e2022JD037265, https://doi.org/10.1029/2022JD037265, 2022.
Berg, W., L'Ecuyer, T., and van den Heever, S.: Evidence for the impact of aerosols on the onset and microphysical properties of rainfall from a combination of satellite observations and cloud-resolving model simulations, J. Geophys. Res.-Atmos., 113, D14S23, https://doi.org/10.1029/2007JD009649, 2008.
Boucher, O., Randall, D. A., Artaxo, P., Bretherton, C. S., Feingold, G., Forster, P., Kerminen, V.-M., Kondo, Y., Liao, H.-T., Lohmann, U., Rasch, P. J., Satheesh, S. K., Sherwood, S. C., Stevens, B., and Zhang, X. Y.: Clouds and Aerosols, in: Climate Change 2013: The Physical Science Basis. Contribution of Working Group I to the Fifth Assessment Report of the Intergovernmental Panel on Climate Change, Cambridge University Press, Cambridge, 571–658, https://doi.org/10.1017/CBO9781107415324.016, 2013.
Chen, G., Xue, H., Feingold, G., and Zhou, X.: Vertical transport of pollutants by shallow cumuli from large eddy simulations, Atmos. Chem. Phys., 12, 11319–11327, https://doi.org/10.5194/acp-12-11319-2012, 2012.
Corr, C. A., Ziemba, L. D., Scheuer, E., Anderson, B. E., Beyersdorf, A. J., Chen, G., Crosbie, E., Moore, R. H., Shook, M., Thornhill, K. L., Winstead, E., Lawson, R. P., Barth, M. C., Schroeder, J. R., Blake, D. R., and Dibb, J. E.: Observational evidence for the convective transport of dust over the Central United States, J. Geophys. Res.-Atmos., 121, 1306–1319, https://doi.org/10.1002/2015JD023789, 2016.
Cotton, W. R., Alexander, G. D., Hertenstein, R., Walko, R. L., McAnelly, R. L., and Nicholls, M.: Cloud venting – A review and some new global annual estimates, Earth-Sci. Rev., 39, 169–206, https://doi.org/10.1016/0012-8252(95)00007-0, 1995.
Cotton, W. R., Pielke Sr., R. A., Walko, R. L., Liston, G. E., Tremback, C. J., Jiang, H., McAnelly, R. L., Harrington, J. Y., Nicholls, M. E., Carrio, G. G., and McFadden, J. P.: RAMS 2001: Current status and future directions, Meteorol. Atmos. Phys., 82, 5–29, https://doi.org/10.1007/s00703-001-0584-9, 2003.
Cui, Z. and Carslaw, K. S.: Enhanced vertical transport efficiency of aerosol in convective clouds due to increases in tropospheric aerosol abundance, J. Geophys. Res.-Atmos., 111, D15212, https://doi.org/10.1029/2005JD006781, 2006.
Dagan, G.: Equilibrium climate sensitivity increases with aerosol concentration due to changes in precipitation efficiency, Atmos. Chem. Phys., 22, 15767–15775, https://doi.org/10.5194/acp-22-15767-2022, 2022.
Dagan, G. and Stier, P.: Ensemble daily simulations for elucidating cloud–aerosol interactions under a large spread of realistic environmental conditions, Atmos. Chem. Phys., 20, 6291–6303, https://doi.org/10.5194/acp-20-6291-2020, 2020.
Dagan, G., Koren, I., and Altaratz, O.: Aerosol effects on the timing of warm rain processes, Geophys. Res. Lett., 42, 4590–4598, https://doi.org/10.1002/2015GL063839, 2015.
Dagan, G., Koren, I., Altaratz, O., and Lehahn, Y.: Shallow Convective Cloud Field Lifetime as a Key Factor for Evaluating Aerosol Effects, iScience, 10, 192–202, https://doi.org/10.1016/j.isci.2018.11.032, 2018.
DeMott, P. J., Prenni, A. J., Liu, X., Kreidenweis, S. M., Petters, M. D., Twohy, C. H., Richardson, M. S., Eidhammer, T., and Rogers, D. C.: Predicting global atmospheric ice nuclei distributions and their impacts on climate, P. Natl. Acad. Sci. USA, 107, 11217–11222, https://doi.org/10.1073/pnas.0910818107, 2010.
Engström, A., Ekman, A. M. L., Krejci, R., Ström, J., de Reus, M., and Wang, C.: Observational and modelling evidence of tropical deep convective clouds as a source of mid-tropospheric accumulation mode aerosols, Geophys. Res. Lett., 35, L23813, https://doi.org/10.1029/2008GL035817, 2008.
Ervens, B., Sorooshian, A., Aldhaif, A. M., Shingler, T., Crosbie, E., Ziemba, L., Campuzano-Jost, P., Jimenez, J. L., and Wisthaler, A.: Is there an aerosol signature of chemical cloud processing?, Atmos. Chem. Phys., 18, 16099–16119, https://doi.org/10.5194/acp-18-16099-2018, 2018.
Fan, J., Yuan, T., Comstock, J. M., Ghan, S., Khain, A., Leung, L. R., Li, Z., Martins, V. J., and Ovchinnikov, M.: Dominant role by vertical wind shear in regulating aerosol effects on deep convective clouds, J. Geophys. Res.-Atmos., 114, D22206, https://doi.org/10.1029/2009JD012352, 2009.
Feingold, G. and Kreidenweis, S.: Does cloud processing of aerosol enhance droplet concentrations?, J. Geophys. Res.-Atmos., 105, 24351–24361, https://doi.org/10.1029/2000JD900369, 2000.
Glassmeier, F. and Lohmann, U.: Constraining Precipitation Susceptibility of Warm-, Ice-, and Mixed-Phase Clouds with Microphysical Equations, J. Atmos. Sci., 73, 5003–5023, https://doi.org/10.1175/JAS-D-16-0008.1, 2016.
Grant, L. D. and van den Heever, S. C.: Aerosol-cloud-land surface interactions within tropical sea breeze convection, J. Geophys. Res.-Atmos. 119, 8340–8361, https://doi.org/10.1002/2014JD021912, 2014.
Gryspeerdt, E., Stier, P., and Partridge, D. G.: Satellite observations of cloud regime development: the role of aerosol processes, Atmos. Chem. Phys., 14, 1141–1158, https://doi.org/10.5194/acp-14-1141-2014, 2014.
Harrington, J. Y.: Effects of radiative and microphysical processes on simulated warm and transition season Arctic stratus, PhD dissertation, Colorado State University, 289 pp., 1997.
Haywood, J. M. and Boucher, O.: Estimates of the direct and indirect radiative forcing due to tropospheric aerosols: A review, Rev. Geophys., 38, 513–543, https://doi.org/10.1029/1999RG000078, 2000.
Haywood, J. M., Jones, A., Bellouin, N., and Stephenson, D.: Asymmetric forcing from stratospheric aerosols impacts Sahelian rainfall, Nat. Clim. Change, 3, 660–665, https://doi.org/10.1038/nclimate1857, 2013.
Hegg, D. A., Covert, D. S., Jonsson, H., Khelif, D., and Friehe, C. A.: Observations of the impact of cloud processing on aerosol light-scattering efficiency, Tellus B, 56, 285–293, https://doi.org/10.1111/j.1600-0889.2004.00099.x, 2004.
Heikenfeld, M., Marinescu, P. J., Christensen, M., Watson-Parris, D., Senf, F., van den Heever, S. C., and Stier, P.: tobac 1.2: towards a flexible framework for tracking and analysis of clouds in diverse datasets, Geosci. Model Dev., 12, 4551–4570, https://doi.org/10.5194/gmd-12-4551-2019, 2019.
Herbener, S. R., Saleeby, S. M., van den Heever, S. C., and Twohy, C. H.: Tropical storm redistribution of Saharan dust to the upper troposphere and ocean surface, Geophys. Res. Lett., 43, 10463–10471, https://doi.org/10.1002/2016GL070262, 2016.
Herbert, R., Stier, P., and Dagan, G.: Isolating Large-Scale Smoke Impacts on Cloud and Precipitation Processes Over the Amazon With Convection Permitting Resolution, J. Geophys. Res.-Atmos. 126, e2021JD034615, https://doi.org/10.1029/2021JD034615, 2021.
Jiang, H., Feingold, G., and Sorooshian, A.: Effect of Aerosol on the Susceptibility and Efficiency of Precipitation in Warm Trade Cumulus Clouds, J. Atmos. Sci., 67, 3525–3540, https://doi.org/10.1175/2010JAS3484.1, 2010.
Jiang, J. H., Su, H., Huang, L., Wang, Y., Massie, S., Zhao, B., Omar, A., and Wang, Z.: Contrasting effects on deep convective clouds by different types of aerosols, Nat. Commun., 9, 3874, https://doi.org/10.1038/s41467-018-06280-4, 2018.
Johnson, R. H., Rickenbach, T. M., Rutledge, S. A., Ciesielski, P. E., and Schubert, W. H.: Trimodal Characteristics of Tropical Convection, J. Climate, 12, 2397–2418, 1999.
Khain, A. P., BenMoshe, N., and Pokrovsky, A.: Factors Determining the Impact of Aerosols on Surface Precipitation from Clouds: An Attempt at Classification, J. Atmos. Sci., 65, 1721–1748, https://doi.org/10.1175/2007JAS2515.1, 2008.
Kim, D., Wang, C., Ekman, A. M. L., Barth, M. C., and Lee, D.-I.: The responses of cloudiness to the direct radiative effect of sulfate and carbonaceous aerosols, J. Geophys. Res.-Atmos., 119, 1172–1185, https://doi.org/10.1002/2013JD020529, 2014.
Kim, M. J., Yeh, S.-W., and Park, R. J.: Effects of sulfate aerosol forcing on East Asian summer monsoon for 1985–2010, Geophys. Res. Lett., 43, 1364–1372, https://doi.org/10.1002/2015GL067124, 2016.
Kipling, Z., Stier, P., Johnson, C. E., Mann, G. W., Bellouin, N., Bauer, S. E., Bergman, T., Chin, M., Diehl, T., Ghan, S. J., Iversen, T., Kirkevåg, A., Kokkola, H., Liu, X., Luo, G., van Noije, T., Pringle, K. J., von Salzen, K., Schulz, M., Seland, Ø., Skeie, R. B., Takemura, T., Tsigaridis, K., and Zhang, K.: What controls the vertical distribution of aerosol? Relationships between process sensitivity in HadGEM3–UKCA and inter-model variation from AeroCom Phase II, Atmos. Chem. Phys., 16, 2221–2241, https://doi.org/10.5194/acp-16-2221-2016, 2016.
Lee, S.-S. and Feingold, G.: Precipitating cloud-system response to aerosol perturbations, Geophys. Res. Lett., 37, L23806, https://doi.org/10.1029/2010GL045596, 2010.
Lee, S. S., Feingold, G., McComiskey, A., Yamaguchi, T., Koren, I., Martins, J. V., and Yu, H.: Effect of gradients in biomass burning aerosol on shallow cumulus convective circulations, J. Geophys. Res.-Atmos., 119, 9948–9964, https://doi.org/10.1002/2014JD021819, 2014.
Leung, G. R. and van den Heever, S. C.: Controls on the Development and Circulation of Terminal versus Transient Congestus Clouds and Implications for Midlevel Aerosol Transport, J. Atmos. Sci., 79, 3083–3101, https://doi.org/10.1175/JAS-D-21-0314.1, 2022.
Leung, G. R. and van den Heever, S. C.: Aerosol breezes drive cloud and precipitation increases, Nat. Commun., 14, 2508, https://doi.org/10.1038/s41467-023-37722-3, 2023a.
Leung, G. R. and van den Heever, S. C.: Code associated with “Aerosol–cloud impacts on aerosol detrainment and rainout in shallow maritime tropical clouds” (Leung et al. 2023), Version v1.0-acp, Zenodo [code], https://doi.org/10.5281/zenodo.7876434, 2023b.
Li, R. L., Studholme, J. H. P., Fedorov, A. V., and Storelvmo, T.: Precipitation efficiency constraint on climate change, Nat. Clim. Change, 12, 642–648, https://doi.org/10.1038/s41558-022-01400-x, 2022.
Li, X., Tao, W.-K., Masunaga, H., Gu, G., and Zeng, X.: Aerosol Effects on Cumulus Congestus Population over the Tropical Pacific: A Cloud-Resolving Modeling Study, J. Meteorol. Soc. Jpn., 91, 817–833, https://doi.org/10.2151/jmsj.2013-607, 2013.
Lutsko, N., Sherwood, S. C., and Zhao, M.: Precipitation efficiency and climate sensitivity (invited chapter for the AGU geophysical monograph series “clouds and climate”), ESS Open Archive, https://doi.org/10.1002/essoar.10507822.1, 2021.
Marinescu, P. J., van den Heever, S. C., Heikenfeld, M., Barrett, A. I., Barthlott, C., Hoose, C., Fan, J., Fridlind, A. M., Matsui, T., Miltenberger, A. K., Stier, P., Vie, B., White, B. A., and Zhang, Y.: Impacts of Varying Concentrations of Cloud Condensation Nuclei on Deep Convective Cloud Updrafts – A Multimodel Assessment, J. Atmos. Sci., 78, 1147–1172, https://doi.org/10.1175/JAS-D-20-0200.1, 2021.
Matsui, T., Zhang, S. Q., Lang, S. E., Tao, W.-K., Ichoku, C., and Peters-Lidard, C. D.: Impact of radiation frequency, precipitation radiative forcing, and radiation column aggregation on convection-permitting West African monsoon simulations, Clim. Dynam., 55, 193–213, https://doi.org/10.1007/s00382-018-4187-2, 2020.
May, P. T., Bringi, V. N., and Thurai, M.: Do We Observe Aerosol Impacts on DSDs in Strongly Forced Tropical Thunderstorms?, J. Atmos. Sci., 68, 1902–1910, https://doi.org/10.1175/2011JAS3617.1, 2011.
McCormick, R. A. and Ludwig, J. H.: Climate Modification by Atmospheric Aerosols, Science, 156, 1358–1359, https://doi.org/10.1126/science.156.3780.1358, 1967.
Meyers, M. P., Walko, R. L., Harrington, J. Y., and Cotton, W. R.: New RAMS cloud microphysics parameterization. Part II: The two-moment scheme, Atmos. Res., 45, 3–39, https://doi.org/10.1016/S0169-8095(97)00018-5, 1997.
Park, J. M. and van den Heever, S. C.: Weakening of tropical sea breeze convective systems through interactions of aerosol, radiation, and soil moisture, Atmos. Chem. Phys., 22, 10527–10549, https://doi.org/10.5194/acp-22-10527-2022, 2022.
Pielke, R. A., Cotton, W. R., Walko, R. L., Tremback, C. J., Lyons, W. A., Grasso, L. D., Nicholls, M. E., Moran, M. D., Wesley, D. A., Lee, T. J., and Copeland, J. H.: A comprehensive meteorological modeling system – RAMS, Meteorol. Atmos. Phys., 49, 69–91, https://doi.org/10.1007/BF01025401, 1992.
Posselt, D. J., van den Heever, S. C., and Stephens, G. L.: Trimodal cloudiness and tropical stable layers in simulations of radiative convective equilibrium, Geophys. Res. Lett., 35, L08802, https://doi.org/10.1029/2007GL033029, 2008.
Radke, L. F., Hobbs, P. V., and Eltgroth, M. W.: Scavenging of Aerosol Particles by Precipitation, Journal of Applied Meteorology (1962-1982), 19, 715–722, 1980.
Reid, J. S., Maring, H. B., Narisma, G. T., Heever, S. van den, Girolamo, L. D., Ferrare, R., Lawson, P., Mace, G. G., Simpas, J. B., Tanelli, S., Ziemba, L., Diedenhoven, B. van, Bruintjes, R., Bucholtz, A., Cairns, B., Cambaliza, M. O., Chen, G., Diskin, G. S., Flynn, J. H., Hostetler, C. A., Holz, R. E., Lang, T. J., Schmidt, K. S., Smith, G., Sorooshian, A., Thompson, E. J., Thornhill, K. L., Trepte, C., Wang, J., Woods, S., Yoon, S., Alexandrov, M., Alvarez, S., Amiot, C. G., Bennett, J. R., M., B., Burton, S. P., Cayanan, E., Chen, H., Collow, A., Crosbie, E., DaSilva, A., DiGangi, J. P., Flagg, D. D., Freeman, S. W., Fu, D., Fukada, E., Hilario, M. R. A., Hong, Y., Hristova-Veleva, S. M., Kuehn, R., Kowch, R. S., Leung, G. R., Loveridge, J., Meyer, K., Miller, R. M., Montes, M. J., Moum, J. N., Nenes, T., Nesbitt, S. W., Norgren, M., Nowottnick, E. P., Rauber, R. M., Reid, E. A., Rutledge, S., Schlosser, J. S., Sekiyama, T. T., Shook, M. A., Sokolowsky, G. A., Stamnes, S. A., Tanaka, T. Y., Wasilewski, A., Xian, P., Xiao, Q., Xu, Z., and Zavaleta, J.: The coupling between tropical meteorology, aerosol lifecycle, convection, and radiation, during the Cloud, Aerosol and Monsoon Processes Philippines Experiment (CAMP2Ex), B. Am. Meteorol. Soc., 1, https://doi.org/10.1175/BAMS-D-21-0285.1, online first, 2023.
Reutter, P., Su, H., Trentmann, J., Simmel, M., Rose, D., Gunthe, S. S., Wernli, H., Andreae, M. O., and Pöschl, U.: Aerosol- and updraft-limited regimes of cloud droplet formation: influence of particle number, size and hygroscopicity on the activation of cloud condensation nuclei (CCN), Atmos. Chem. Phys., 9, 7067–7080, https://doi.org/10.5194/acp-9-7067-2009, 2009.
Saleeby, S. M. and Cotton, W. R.: A Large-Droplet Mode and Prognostic Number Concentration of Cloud Droplets in the Colorado State University Regional Atmospheric Modeling System (RAMS). Part I: Module Descriptions and Supercell Test Simulations, J. Appl. Meteorol. Clim., 43, 182–195, https://doi.org/10.1175/1520-0450(2004)043<0182:ALMAPN> 2.0.CO;2, 2004.
Saleeby, S. M. and van den Heever, S. C.: Developments in the CSU-RAMS Aerosol Model: Emissions, Nucleation, Regeneration, Deposition, and Radiation, J. Appl. Meteorol. Clim., 52, 2601–2622, https://doi.org/10.1175/JAMC-D-12-0312.1, 2013.
Saleeby, S. M., Herbener, S. R., van den Heever, S. C., and L'Ecuyer, T.: Impacts of Cloud Droplet–Nucleating Aerosols on Shallow Tropical Convection, J. Atmos. Sci., 72, 1369–1385, https://doi.org/10.1175/JAS-D-14-0153.1, 2015.
Samset, B. H. and Myhre, G.: Vertical dependence of black carbon, sulphate and biomass burning aerosol radiative forcing, Geophys. Res. Lett., 38, L24802, https://doi.org/10.1029/2011GL049697, 2011.
Savre, J.: Formation and maintenance of subsiding shells around non-precipitating and precipitating cumulus clouds, Q. J. Roy. Meteor. Soc., 147, 728–745, https://doi.org/10.1002/qj.3942, 2021.
Sheffield, A. M., Saleeby, S. M., and van den Heever, S. C.: Aerosol-induced mechanisms for cumulus congestus growth, J. Geophys. Res.-Atmos., 120, 8941–8952, https://doi.org/10.1002/2015JD023743, 2015.
Smalley, K. M. and Rapp, A. D.: The Role of Cloud Size and Environmental Moisture in Shallow Cumulus Precipitation, J. Appl. Meteorol. Clim., 59, 535–550, https://doi.org/10.1175/JAMC-D-19-0145.1, 2020.
Sokolowsky, G. A., Freeman, S. W., and van den Heever, S. C.: Sensitivities of Maritime Tropical Trimodal Convection to Aerosols and Boundary Layer Static Stability, J. Atmos. Sci., 79, 2549–2570, https://doi.org/10.1175/JAS-D-21-0260.1, 2022.
Spill, G., Stier, P., Field, P. R., and Dagan, G.: Effects of aerosol in simulations of realistic shallow cumulus cloud fields in a large domain, Atmos. Chem. Phys., 19, 13507–13517, https://doi.org/10.5194/acp-19-13507-2019, 2019.
Storer, R. L. and van den Heever, S. C.: Microphysical Processes Evident in Aerosol Forcing of Tropical Deep Convective Clouds, J. Atmos. Sci., 70, 430–446, https://doi.org/10.1175/JAS-D-12-076.1, 2013.
Tao, W.-K., Chen, J.-P., Li, Z., Wang, C., and Zhang, C.: Impact of aerosols on convective clouds and precipitation, Rev. Geophys., 50, RG2001, https://doi.org/10.1029/2011RG000369, 2012.
tobac Community: tobac – Tracking and Object-based Analysis of Clouds, Version v1.4.2, Zenodo [code], https://doi.org/10.5281/zenodo.7662376, 2023.
Twohy, C. H., Anderson, B. E., Ferrare, R. A., Sauter, K. E., L'Ecuyer, T. S., van den Heever, S. C., Heymsfield, A. J., Ismail, S., and Diskin, G. S.: Saharan dust, convective lofting, aerosol enhancement zones, and potential impacts on ice nucleation in the tropical upper troposphere, J. Geophys. Res.-Atmos. 122, 8833–8851, https://doi.org/10.1002/2017JD026933, 2017.
Twomey, S.: The Influence of Pollution on the Shortwave Albedo of Clouds, J. Atmos. Sci., 34, 1149–1152, https://doi.org/10.1175/1520-0469(1977)034<1149:TIOPOT>2.0.CO;2, 1977.
van den Heever, S. C., Stephens, G. L., and Wood, N. B.: Aerosol Indirect Effects on Tropical Convection Characteristics under Conditions of Radiative–Convective Equilibrium, J. Atmos. Sci., 68, 699–718, https://doi.org/10.1175/2010JAS3603.1, 2011.
Walko, R. L., Band, L. E., Baron, J., Kittel, T. G. F., Lammers, R., Lee, T. J., Ojima, D., Pielke, R. A., Taylor, C., Tague, C., Tremback, C. J., and Vidale, P. L.: Coupled Atmosphere–Biophysics–Hydrology Models for Environmental Modeling, J. Appl. Meteorol. Clim., 39, 931–944, https://doi.org/10.1175/1520-0450(2000)039<0931:CABHMF>2.0.CO;2, 2000.
Wang, Y., Xia, W., Liu, X., Xie, S., Lin, W., Tang, Q., Ma, H.-Y., Jiang, Y., Wang, B., and Zhang, G. J.: Disproportionate control on aerosol burden by light rain, Nat. Geosci., 14, 72–76, https://doi.org/10.1038/s41561-020-00675-z, 2021a.
Wang, Y., Xia, W., and Zhang, G. J.: What rainfall rates are most important to wet removal of different aerosol types?, Atmos. Chem. Phys., 21, 16797–16816, https://doi.org/10.5194/acp-21-16797-2021, 2021b.
Williams, A. I. L., Stier, P., Dagan, G., and Watson-Parris, D.: Strong control of effective radiative forcing by the spatial pattern of absorbing aerosol, Nat. Clim. Change, 12, 735–742, https://doi.org/10.1038/s41558-022-01415-4, 2022.
Xue, H., Feingold, G., and Stevens, B.: Aerosol Effects on Clouds, Precipitation, and the Organization of Shallow Cumulus Convection, J. Atmos. Sci., 65, 392–406, https://doi.org/10.1175/2007JAS2428.1, 2008.
- Abstract
- Introduction
- Model description and analysis approach
- Aerosol impacts on domain properties
- Aerosol impacts on cloud population distributions
- Influence of aerosol type
- Summary and discussion
- Code and data availability
- Author contributions
- Competing interests
- Disclaimer
- Special issue statement
- Acknowledgements
- Financial support
- Review statement
- References
- Supplement
Please read the editorial note first before accessing the article.
- Article
(5983 KB) - Full-text XML
-
Supplement
(1865 KB) - BibTeX
- EndNote
- Abstract
- Introduction
- Model description and analysis approach
- Aerosol impacts on domain properties
- Aerosol impacts on cloud population distributions
- Influence of aerosol type
- Summary and discussion
- Code and data availability
- Author contributions
- Competing interests
- Disclaimer
- Special issue statement
- Acknowledgements
- Financial support
- Review statement
- References
- Supplement