the Creative Commons Attribution 4.0 License.
the Creative Commons Attribution 4.0 License.
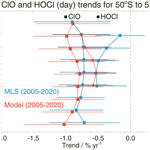
Upper stratospheric ClO and HOCl trends (2005–2020): Aura Microwave Limb Sounder and model results
Lucien Froidevaux
Douglas E. Kinnison
Michelle L. Santee
Luis F. Millán
Nathaniel J. Livesey
William G. Read
Charles G. Bardeen
John J. Orlando
Ryan A. Fuller
We analyze Aura Microwave Limb Sounder (MLS) monthly zonal mean time series of ClO and HOCl between 50∘ S and 50∘ N to estimate upper stratospheric trends in these chlorine species from 2005 through 2020. We compare these observations to those from the Whole Atmosphere Community Climate Model version 6 (WACCM6), run under the specified dynamics configuration. The model sampling follows the MLS coverage in space and local time. We use version 5 MLS ClO zonal mean daytime profiles and similarly binned daytime ClO model profiles from 32 to 1.5 hPa. For MLS HOCl, we use the version 5 offline product derived from daily zonal mean radiances rather than averaged level-2 profiles; MLS HOCl is scientifically useful between 10 and 2 hPa, and the HOCl monthly zonal means are separated into day and night for comparison to WACCM6. We find good agreement (mostly within ∼ 10 %) between the climatological MLS ClO daytime distributions and the model ClO climatology for 2005–2020. The model HOCl climatology, however, underestimates the MLS HOCl climatology by about 30 %. This could well be caused by a combination of fairly large systematic uncertainties in both the model-assumed rate constant for the formation of HOCl and the MLS HOCl retrievals themselves.
The model daytime ClO trends versus latitude and pressure agree quite well with those from MLS. MLS-derived near-global upper stratospheric daytime trends between 7 and 2 hPa are −0.73 ± 0.40 % yr−1 for ClO and −0.39 ± 0.35 % yr−1 for HOCl, with 2σ uncertainty estimates used here. The corresponding model decreases are somewhat faster than observed (although the difference is not statistically significant), with trend values of −0.85 ± 0.45 % yr−1 for ClO and −0.64 ± 0.37 % yr−1 for HOCl. Both data and model results point to a faster trend in ClO than in HOCl. The MLS ClO trends are consistent with past estimates of upper stratospheric ClO trends from satellite and ground-based microwave data. As discussed in the past, trends in other species (in particular, positive trends in CH4 and H2O) can lead to a ClO decrease that is faster than the decrease in total inorganic chlorine. Regarding trends in HOCl, positive trends in HO2 can lead to a faster rate of formation for HOCl as a function of time, which partially offsets the decreasing trend in active chlorine.
The decreasing trends in upper stratospheric ClO and HOCl provide additional confirmation of the effectiveness of the Montreal Protocol and its amendments, which have led to the early stages of an expected long-term ozone recovery from the effects of ozone-depleting substances.
- Article
(8221 KB) - Full-text XML
-
Supplement
(417 KB) - BibTeX
- EndNote
Changes in the gaseous chlorine content of the atmosphere have been scrutinized since the late 1970s, when prescient warnings (Molina and Rowland, 1974) were made regarding likely threats to the Earth's stratospheric ozone (O3) layer from the decomposition of various chlorofluorocarbons (CFCs) emitted at the surface by human industrial activities. These threats carried human health implications as a result of increased ultraviolet (UV) radiation at the surface, which would follow from reductions in UV absorption by stratospheric ozone. Various measurements of the abundances of different chlorine species in the stratosphere followed these early years of concern regarding expected declines in global ozone. Early balloon-borne observations of chlorine monoxide (ClO) radicals in the upper stratosphere (Anderson et al., 1977; Waters et al., 1981) confirmed the predicted importance of gas-phase reactions (involving ClO, Cl, O3, and O) on upper stratospheric ozone abundances. Since the 1987 Montreal Protocol and its subsequent amendments, established to strongly reduce worldwide surface emissions of halogenated compounds harmful to the ozone layer, both the tropospheric and stratospheric chlorine budgets have been carefully studied and monitored by the atmospheric science community. This was motivated by enhanced concerns regarding ozone decreases in the lower stratosphere, after the discovery of the seasonal appearance of an ozone hole over Antarctica (Farman et al., 1985).
Studies of interannual and longer-term changes in stratospheric chlorine species were carried out by ground-based (column) measurements of HCl and ClONO2 at infrared wavelengths (Rinsland et al., 2003; Kohlhepp et al., 2011; Mahieu et al., 2014). Near-global stratospheric chlorine changes have also been tracked by satellite measurements of HCl. Indeed, this chlorine reservoir species at high altitude (near 50 km) accounts for the vast majority of Cly (total inorganic chlorine), based on past measurements of the stratospheric chlorine budget by Zander et al. (1992) and Nassar et al. (2006). Froidevaux et al. (2006) also discussed model results regarding the contribution of upper stratospheric HCl to Cly and described measurable decreases in HCl (and by inference, in Cly) from mid-2004 to early 2006, based on changes in Aura Microwave Limb Sounder (MLS) profiles. The rather fast rise in chlorine from the 1980s to the late 1990s (with increases of more than 55 %) was followed by a slower rate of decrease, as expected from model calculations. Stratospheric chlorine follows the overall tropospheric trends with about a 5-year delay, which accounts for photolysis, transport, and mixing of tropospheric compounds into the stratosphere (as discussed by Anderson et al., 2000; Waugh et al., 2001; and others).
Changes in chlorine source gases at the surface, as well as changes in stratospheric chlorine species, have been updated and documented regularly in quadrennial reports (see WMO, 2018). Based on such analyses, stratospheric HCl has been decreasing over the past two decades by about 0.5 % yr−1–1 % yr−1. This includes results from ground-based infrared measurements, as well as from near-global upper stratospheric HCl measurements by the Atmospheric Chemistry Experiment Fourier Transform Spectrometer (ACE-FTS) (see Bernath and Fernando, 2018). These results are consistent with surface total chlorine trends, based on in situ sampling of a large number of source species by ground-based networks (Engel and Rigby et al., 2018), so there is a good corroboration of the effectiveness of the Montreal Protocol and its amendments, except for some recent departures from expectations for the evolution of CFC-11 (Montzka et al., 2018). Ground-based microwave measurements of stratospheric ClO profiles over the past two decades have also made valuable contributions to these long-term chlorine composition records. This includes trend results for upper stratospheric ClO over Hawaii (Solomon et al., 2006; Connor et al., 2013) as well as for the more variable lower stratosphere over Antarctica (Nedoluha et al., 2016). These findings corroborate the longer-term decreasing trends in HCl (and Cly), although dynamical variability on timescales of 5–7 years complicates trend detection (e.g., for HCl) in the lower stratosphere (Mahieu et al., 2014; Strahan et al., 2020); this variability and its causes are still under investigation in the community.
Here, we provide an analysis of upper stratospheric trends in near-global ClO and hypochlorous acid (HOCl). These two chlorine species have been measured by the Aura Microwave Limb Sounder (MLS) globally on a near-daily basis since its launch in 2004. An analysis of their trends falls within the general theme of confirming that the Montreal Protocol has been able to significantly reduce the threat of stratospheric chlorine to global ozone. The MLS measurements of upper stratospheric ClO and HOCl have taken on a larger role, in light of the fact that MLS lost the capability of obtaining trend-quality data on upper stratospheric HCl after a hardware issue in early 2006 (see Livesey et al., 2020). The lower stratospheric HCl measurements have continued through the use of radiances from an adjacent MLS measurement band (see also the lower stratospheric MLS HCl comparisons to model results by Froidevaux et al., 2019). In Sect. 2, we describe the observations, model simulations, and methods of analysis for this work. Section 3 focuses on the trend results for ClO and HOCl, while Sect. 4 provides a discussion in the context of broader trends in upper stratospheric species. Our conclusions are summarized in Sect. 5.
In this work, we analyze temporal changes in upper stratospheric ClO and HOCl abundances, based on continuous MLS observations of both species from 2005 through 2020. We compare these observational results to those from a state-of-the-art chemistry–climate model for the same time period.
2.1 Observations
The primary data sets used in this analysis come from 16 full years (2005 through 2020) of global measurements performed by Aura MLS. The MLS antenna scans the atmospheric limb as the Aura satellite orbits the Earth in a near-polar Sun-synchronous orbit; the instrument measures thermal emission (day and night), using microwave radiometers operating at frequencies near 118, 190, 240, and 640 GHz, as well as a 2.5 THz module to measure OH (during the early part of the mission only). MLS has been providing a variety of daily vertical stratospheric temperature and composition profiles (∼ 3500 profiles per day per product), with some measurements extending down to the upper tropospheric region and some into the upper mesosphere or higher. We rely here mainly on the upper stratospheric MLS measurements of ClO and HOCl, obtained from 640 GHz radiometer data. Specifically, ClO and HOCl emissions are obtained from lines centered at 649.5 and 635.9 GHz, respectively; Waters at al. (2006) have provided an overview of the MLS instrument and its measurements, along with some sample spectra, and Read et al. (2006) have described the simulated forward model and related spectra. The MLS retrievals use an optimal estimation approach (Rodgers, 2000), with MLS-specific details provided by Livesey et al. (2006); there is no assumption of atmospheric homogeneity along the line of sight (see Livesey and Read, 2000), and the MLS retrievals make use of the instrument's views (which are all along the line of sight) during multiple consecutive MLS antenna scans of the Earth's limb. Data users interested in MLS data quality and characterization, estimated errors, and related information should consult Livesey et al. (2020), the latest update to the MLS data quality document.
In this work, we use the latest data version from MLS, namely, version 5.0 (or v5). The single-profile precision (1σ random uncertainty) is ∼ 0.1 ppbv for the ClO retrievals in the region between 32 and 1.5 hPa that we focus on here; the vertical resolution of the ClO measurements is about 3–4 km. For our analyses of daytime MLS ClO monthly zonal means in 5∘ latitude bins, the more relevant precision for averaged upper stratospheric values drops to about 0.5 %–5 %. In addition, the methodology used by the MLS team to assess the aggregate effects of simulated errors in various input parameters on the measurement retrievals (see Livesey et al., 2020) leads to systematic uncertainties on the order of 0.02–0.1 ppbv for upper stratospheric ClO, which translates to about 5 %–100 % for ClO, depending on whether one considers the peaks of the distributions (for the smaller uncertainty values) or regions away from these peaks. The standard MLS data quality screening methodology (see the above reference) has been applied to all level-2 ClO profiles, prior to averaging into monthly zonal means.
For the MLS HOCl data, we have used an offline retrieval product that shows similar results as the averaged level-2 profiles but with somewhat smaller variability. This product is created offline (i.e., after the daily processing of incoming MLS data) by averaging daily level-1 spectra before performing the retrievals of mean daily profiles, which are then averaged for this work into either day or night monthly zonal means. The offline retrieval technique follows the overall MLS retrieval methodology described by Livesey et al. (2006), except it is a one-dimensional type of retrieval (as it is not used for line-of-sight “chunks” of profiles like the level-2 “tomographic” approach). Moreover, the radiances that are used as part of the averages correspond to profiles for which the temperature and ozone retrievals in level 2 have passed the standard retrieval criteria for good quality data. This methodology is the same as that used for the MLS offline retrievals of BrO and HO2, which are also considered to be MLS “noisy products”, based on their single-profile precision values (see Millán et al., 2012, 2015, for BrO and HO2, respectively). These averaged offline products can be more stable and scientifically useful over a wider vertical range than averages of the MLS level-2 standard products (although the wider vertical range only holds for HO2). Also, the latitude grid spacing for the MLS offline HOCl product (as for the other offline products mentioned above) is 10∘ rather than the 5∘ used for ClO and other standard MLS retrieval products. We have used the precision and accuracy of HOCl estimates from the standard level-2 MLS product, as we expect similar uncertainties (or possibly better) for the offline HOCl product. The MLS HOCl precision for (day or night) 10∘ monthly zonal means is typically less than 5–10 pptv (or roughly 5 %–20 %). Systematic uncertainties are estimated to be 40–80 pptv for HOCl or about 25 %–100 %. The more limited useful vertical range for MLS HOCl is 10 to 2 hPa, and the HOCl profiles have a vertical resolution of 5–6 km. The reader is referred to Livesey et al. (2020) for more detailed information regarding the MLS HOCl standard product.
We also make use of upper stratospheric data from ACE-FTS, which was launched in 2003 as part of the Canadian SCISAT mission. The instrument uses the solar occultation technique and gathers measurements in the infrared region (at 750–4400 cm−1, with a spectral resolution of 0.02 cm−1). The ACE-FTS sampling is skewed towards middle to high latitudes, with many fewer profiles per day (per species) than obtained from MLS (30 from ACE-FTS versus ∼ 3500 from MLS). ACE-FTS has provided a wealth of constituent profile measurements over basically the same period as Aura MLS (see the overview by Bernath, 2017); we use some ACE-FTS trend results to obtain a broader description and understanding of chlorine species trends in the upper stratosphere. We have used ACE-FTS data version 4.1 in the analyses presented here; see Boone et al. (2020) and references therein for detailed information on the ACE-FTS retrievals. We have removed the largest outliers in the ACE-FTS data by using the prescription regarding data flags from Sheese et al. (2015), although this data screening makes essentially no difference to the near-global upper stratospheric data averages and related trend results in this work.
2.2 Model simulations
The model used here is the Whole Atmosphere Community Climate Model version 6 (WACCM6), a component of the Community Earth System Model 2 (CESM2), configured to use specified dynamics as described by Gettelman et al. (2019). These authors showed that this chemistry–climate model reproduces many modes of variability, as well as trends, in the middle atmosphere. WACCM6 is the “high-top” version of the Community Atmosphere Model, version 6 (CAM6; Danabasoglu et al., 2019). CAM6 includes updated representations of boundary layer processes, shallow convection, liquid cloud macrophysics, and two-moment cloud microphysics with prognostic cloud mass and concentration. This version of CAM6 uses a finite volume dynamical core (Lin, 2004). The horizontal resolution is 0.95∘ latitude × 1.25∘ longitude. The model has 88 levels with a vertical range from the surface to the lower thermosphere. The vertical resolution in the lower stratosphere ranges from 1.2 km near the tropopause to ∼ 2 km near the stratopause.
The WACCM6 model represents chemical processes from the troposphere into the lower thermosphere. The chemical scheme includes the Ox, NOx, HOx, ClOx, and BrOx chemical families, along with CH4 and its degradation products. This scheme also includes primary non-methane hydrocarbons and related oxygenated organic compounds. The chemical processes have evolved from previous versions and are summarized in detail by Emmons et al. (2020). Reaction rates follow the JPL 2015 recommendations (Burkholder et al., 2015). The chemical scheme also includes a new detailed representation of secondary organic aerosols (SOAs), based on the “simple Volatility Basis Set” approach (Tilmes et al., 2019). WACCM includes a total of 231 species and 583 chemical reactions broken down into 150 photolysis reactions, 403 gas-phase reactions, 13 tropospheric, and 17 stratospheric heterogeneous reactions. The photolytic reactions are based on both inline chemical modules and a lookup table approach (Kinnison et al., 2007).
The model scenario used here is based on historical forcings (and recent updates) from the Climate Model Intercomparison Project – Phase 6 (Meinshausen et al., 2017); any reference to “model” in this work refers to this WACCM6 scenario (unless otherwise noted, in particular, for a sensitivity study). The forcings include greenhouse gases (CH4, N2O, and CO2) and organic halogens (CH3Cl, CH3CCl3, CCl4, CFC-11, CFC-12, CFC-113, CFC-114, CFC-115, HCFC-22, HCFC-141b, HCFC-142b, CH3Br, halon-1211, halon-1301, halon-2402, CHBr3, and CH2Br2). CMIP6 specification of NOx emissions from medium-energy electrons (MEEs), solar proton events (SPEs), and galactic cosmic rays (GCRs) is also included. The 11-year solar cycle variability is taken from the Naval Research Laboratory's (NRL) solar variability model, referred to as the NRL Solar Spectral Irradiance version 2 (NRLSSI2; Coddington et al., 2016). The volcanic SO2 emissions (used in the sulfate aerosol density calculation) are derived for each volcanic eruption using the Neely and Schmidt (2016) database updated through the year 2020. This work uses the specified dynamics (SD) option (Lamarque et al., 2012), where reanalysis temperature, zonal and meridional winds, surface stress, surface pressure, and surface latent and sensible heat are used to nudge the model state, thus affecting parameterizations controlling boundary layer exchanges, advective and convective transport, and the hydrological cycle. This model's dynamical constraints, including the Quasi-Biennial Oscillation (QBO), arise from meteorological fields provided by the Modern-Era Retrospective analysis for Research and Applications Version 2 (MERRA-2; Gelaro et al., 2017), and the nudging approach is described by Kunz et al. (2011). The model meteorological fields are nudged from the surface to 50 km; above 60 km, these fields are fully interactive, with a linear transition in between. The model nudging time constant is 50 h. Model results are obtained from a simulation that, originally, started in 1980 and ended in 2014 (Gettelman et al., 2019); it was later augmented with runs through 2020. After 2014, the greenhouse gas and organic halogen inputs follow the CMIP6 SSP2-45 scenario that projects inputs beyond 2014 (O'Neill et al., 2016; Riahi et al., 2017; Meinshausen et al., 2020), the SPEs are derived from the Geostationary Observational Environmental Satellites (GOES) proton fluxes (Jackman et al., 2008), and the MEEs and GCRs are based on the CMIP6 pre-industrial control.
In terms of sampling, the flexibility of WACCM allows for a choice of profiles for local time and spatial coincidences as close as possible to each MLS profile, using the roughly 1∘ × 1∘ model bin that includes a given data location for a model local time that falls within 15 min of the MLS local time, and binned according to day or night criteria. The model's daily zonal mean profiles (sampled following the MLS locations and local times) are interpolated (as a function of log(p), where p is pressure) to the MLS retrieval grid points; for ClO and HOCl, this grid is defined by a stratospheric subset of , in units of hectopascals (hPa), where n is the pressure level index.
2.3 Analysis methods
We have used solar zenith angles less than 90∘ or larger than 100∘ to separate daytime from nighttime values, respectively, for both MLS and model profiles; after this selection, monthly zonal means were created.
In terms of trend analyses, we follow the approach for MLS data and model trends discussed by Froidevaux et al. (2019), namely, a multivariate linear regression (MLR) method, in order to fit the monthly zonal mean time series from both MLS and the model. We refer the reader to Appendix A3 of the above reference for more details regarding the regression model, which includes commonly used functional terms, namely, a linear trend and a constant term, cosine and sine functions with annual and semi-annual periodicities, and functions describing variations arising from the QBO and the El Niño–Southern Oscillation (ENSO); ENSO plays a large role (in comparison to the QBO) only in the lower stratosphere (e.g., Randel and Thompson, 2011). Here, we also include a fitted component that follows variations in solar radio flux (at 10.7 cm), F10.7, based on the Canadian solar measurements described by Tapping (2013). For the trend uncertainty estimates, as mentioned also by Froidevaux et al. (2019), we use a block bootstrap resampling method (Efron and Tibshirani, 1993), as done by Bourassa et al. (2014), Mahieu et al. (2014), and others, in trend analyses of atmospheric composition. Basically, for every fitted time series from MLS and the model, we analyze many (thousands of) resampling data of the fit residuals, with year-long blocks of values replaced by values from randomly chosen years; (twice the) standard deviations in these random distributions provide (2σ) uncertainty values. Such results are typically very similar to the 95 % confidence level (which would be arrived at by using the 2.5 and 97.5 percentile limits of the distributions). We have found that such trend uncertainty calculations generally lead to significantly larger error bars than methods that neglect the autocorrelation of the residuals, and even than some methods that include simple correction factors for this autocorrelation (see more details in a later section).
3.1 ClO
We first provide in Fig. 1 an overview of daytime ClO climatological values for January and July (averages for 2005 through 2020) in the 50∘ S–50∘ N latitude region and a comparison to the model results. As a consequence of the photochemical balance between Cl and ClO radicals in the upper stratosphere, the largest ClO abundances occur at pressure levels near 2 to 3 hPa; in the mid to lower stratosphere, the availability of reactive chlorine is limited by the conversion of ClO and NO2 to ClONO2. The observed ClO daytime distributions during January and July are well reproduced by the model results (top and middle panels in Fig. 1, respectively), with ratios between model and data between 0.9 and 1.1 for most latitudes at pressures less than 10 hPa (bottom panels in Fig. 1); in this region, the systematic uncertainty estimates for MLS ClO are about 0.02 to 0.03 ppbv (see Livesey et al., 2020) or on the order of 5 %–10 %. Near 20–30 hPa, the model ClO values in the winter hemisphere mid to high latitudes are lower than observed by ∼ 30 %, although there is not much available ClO (in a climatological average sense) in this region, and the systematic uncertainty estimates for MLS ClO are on the order of 0.1 ppbv, which can be as much as 50 %–100 %. Besides these features (and equally good model/data agreement during other months of the year, not shown), we note that the model reproduces the seasonal changes in the peak ClO abundance patterns, which are tied to other seasonal changes. Indeed, it has been shown in the past that seasonal and longer-term variations in the CH4 and H2O distributions play a primary role in the chlorine partitioning between upper stratospheric HCl and ClO (see Solomon and Garcia, 1984; Siskind et al., 1998; Froidevaux et al., 2000).
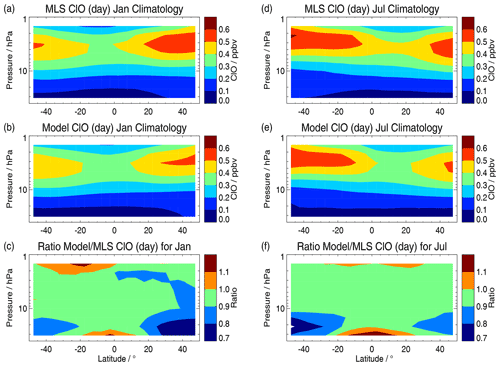
Figure 1Climatological mean fields (over 2005 through 2020) for ClO (daytime data) from MLS and model results between 50∘ S and 50∘ N and 32 to 1.5 hPa. Daytime averages (observed and simulated values) are based on values with solar zenith angles less than 90∘ only. Panels (a) and (b) show the January MLS and model climatologies, respectively, while panel (c) gives the ratio (model values divided by MLS values) for that month; panels (d), (e), and (f) are the same as (a), (b), and (c), respectively, but for July instead of January. The model daily values (throughout this work) were sampled to provide the closest match in space and time to the MLS daily level-2 data; model results were then binned in latitude and averaged over each month (and interpolated to the MLS pressure grid), in order to best match the averaging process of MLS monthly zonal mean data.
Sample time series for the MLS ClO daytime data are shown in Fig. 2, along with the model series, and regression fits (see Sect. 2) to both data and model series. Residual series are shown in the bottom panel of Fig. 2, for the fits to MLS and to the model, and also for the model minus MLS series, after taking out the average model bias versus the data. In this latitude/pressure bin (35–40∘ N/2.2 hPa), there is a slight model underestimate of the observed time series, but the modeled temporal decrease (reflected in the relevant fitted line) follows the slope of the observed tendency fairly closely. The root-mean-square (rms) residual values for this panel, and in general, are close to 5 %–7 %, although the WACCM time series actually fit the MLS data better than the regression fits do, as the rms residuals for (debiased) WACCM versus MLS data are typically between 3 % and 5 %. These ClO results are further quantified in Fig. 3, where we show excellent agreement between the modeled and observed trends versus latitude at different pressures, in terms of the magnitude and morphology. These results demonstrate statistically significant decreasing ClO trends of about −0.5 % yr−1 to −1 % yr−1 in the region between about 30 and 1 hPa from 2005–2020, with very good agreement between the measurements and the WACCM6 simulations. Fig. 3 also shows that there is no significant difference between modeled and measured ClO trends, given the size of the uncertainties (displayed in these plots as 2σ error bars), as obtained from the statistics of block bootstrap resampling of the fitted residuals (see Sect. 2.3). This good agreement between modeled and measured ClO trends can also be viewed in the pressure/latitude contour plots of Fig. 4; the trend differences (model minus data trends) shown in the bottom panel are usually less than 0.1 % yr−1 to 0.2 % yr−1. We note (from Figs. 3 and 4) that there is some asymmetry in the stratospheric ClO trends between the two hemispheres, with stronger decreases at northern than at southern midlatitudes, and with a somewhat more pronounced effect in the lower stratosphere. However, these asymmetries do not carry much statistical significance. These tendencies are opposite to what has been observed in HCl column trends (see Strahan et al., 2020), which show stronger declines in the south than in the north. Lower stratospheric ClO trends are likely to also be related to trends in CH4 and H2O, although we do not pursue this quantitatively here, other than through the WACCM results, which show a similar but slightly stronger interhemispheric asymmetry in lower stratospheric ClO trend than in the MLS data. At 32 hPa, we note that there is evidence for low-frequency (multi-year) MLS and model ClO variations with poorer regression fits to both data and model (although not shown here and not the focus of this work); this complexity is a likely reason for the larger trend discrepancies (WACCM versus data) in this region. Further investigations of interhemispheric asymmetries in lower stratospheric trends (and related age of air issues) are probably best pursued through detailed studies of longer-lived species than ClO.
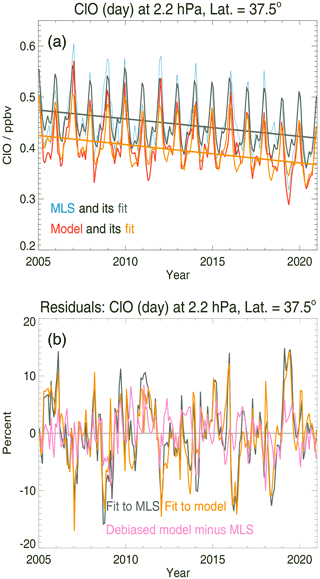
Figure 2(a) Examples of MLS and model ClO (day) monthly zonal mean time series (2005 through 2020) for the 35–40∘ N latitude bin at 2.2 hPa. The MLS data (blue) are fitted by a regression model (gray), and the model series (red) is fitted by the same type of regression model (orange). The dark gray and orange lines are the linear components of the corresponding fits to the MLS and model curves, respectively. (b) Percent residuals, for the fit to MLS (fit minus MLS) in gray, for the fit to the model (fit minus model) in orange, and for the debiased model minus MLS time series in pink.
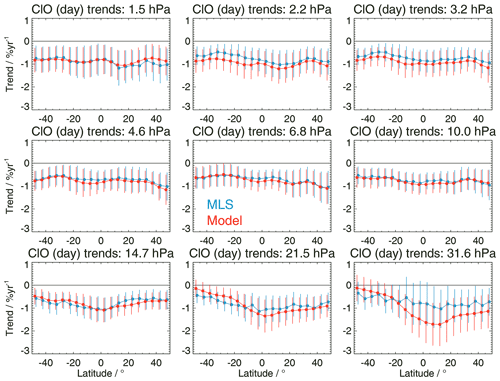
Figure 3Linear trends in upper stratospheric ClO (2005 through 2020) at different pressure levels versus latitude, as obtained from multiple regression analyses applied to monthly zonal mean daytime series from MLS (blue) and the model (red). Error bars depict the uncertainties (2σ) for these trend results, based on block bootstrap analyses of the monthly residual series from the fits to the MLS and model series.
In Fig. 5, we give the near-global (50∘ S to 50∘ N) ClO profile trend results, based on our analyses of monthly zonal mean daytime profile time series for this region as a whole. We obtain very similar trend values if we average results from separate latitude bins, or if we deseasonalize time series from different (narrower) latitude bins prior to the regression. However, we feel it is appropriate to apply the regression analysis to the whole 50∘ S to 50∘ N region to describe the resulting uncertainties in these near-global trends in a consistent way, and (particularly) to compare overall ClO trends to those in other species, as we do in a subsequent section. We see from Fig. 5 that measured near-global ClO trends are on the order of −0.7 % yr−1 to −0.8 % yr−1 in the 15–1.5 hPa range, with values closer to −1 % yr−1 near 20 to 30 hPa. Model ClO trends are typically slightly more negative than observed trends, with an average upper stratospheric value closer to −0.9 % yr−1 (for pressures less than about 15 hPa). In summary, we find very good agreement in the derived ClO trends between the model and the MLS data for 2005–2020, and the differences are not statistically significant.
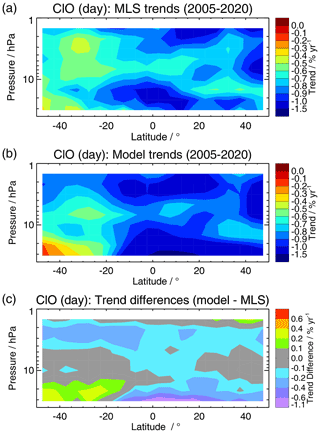
Figure 4Contour plots of ClO (day) trends (% yr−1) for the period 2005 through 2020 from (a) MLS and (b) model, with panel (c) showing the differences (% yr−1) in these trends (model – MLS).
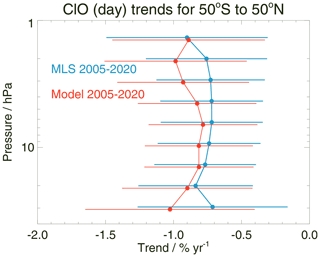
Figure 5Trends in ClO (daytime values) over 2005 through 2020 from MLS (blue) and model (red) for the 50∘ S to 50∘ N latitude range. Error bars depict the uncertainties (2σ) for these trend results, based on block bootstrap analyses of the monthly residual series from the fits to the MLS and model time series.
3.2 HOCl
We now show results for HOCl, using the same approach as for ClO. The MLS HOCl offline product (see Sect. 2.1) yields climatological fields, displayed in Fig. 6 for January and July, over the 10 to 2 hPa region, where the MLS HOCl data are deemed to be scientifically useful (see Livesey et al., 2020); this vertical range also holds for the offline product. We observe peak HOCl January (daytime) values of about 160 pptv near 5 hPa at mid to high latitudes in the Southern Hemisphere, with slightly larger July peak values in the Northern Hemisphere (near 45∘ N). These patterns are also seen in the model HOCl (daytime) distributions, albeit with a shift to smaller abundances; as seen from the model/MLS ratios in the bottom panels of Fig. 6, model HOCl values are typically about 30 % smaller than the mean measurements from MLS. This model-measurement difference is also seen in the nighttime HOCl climatology, as shown in the Supplement (Fig. S1). A small upward shift in the altitude of peak nighttime HOCl abundances is seen in the MLS data, in comparison to the daytime case (Fig. 6), as well as in the model values. Such a diurnal shift in the distribution of HOCl was also noted in the global satellite measurements of HOCl made by the Michelson Interferometer for Passive Atmospheric Sounding (MIPAS) aboard Envisat (von Clarmann et al., 2006, 2012). We note here that the MLS HOCl measurements have fairly large systematic uncertainties (2σ estimated systematic errors of 30 %–100 %; see Livesey et al., 2020), which could thus largely explain the model/data differences. We also note that slightly smoother profiles would be obtained by applying the MLS averaging kernels to the model profiles, since the MLS HOCl vertical resolution is 5–6 km; doing so would lead to an even larger model underestimate of the MLS HOCl profiles.
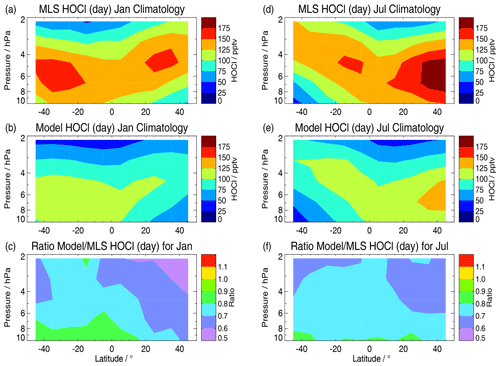
Figure 6Same as Fig. 1 except for climatological (2005–2020) HOCl daytime values from MLS and the model (see text for more details); the vertical range for useful MLS HOCl data (and for related trend analyses) is 10 to 2.2 hPa.
Another consideration to factor into the model uncertainties for HOCl has to do with the uncertainties in the rate constant for HOCl formation (). While the model used here conforms to the JPL Evaluation 18 (Burkholder et al., 2015) rate constant for this reaction, a more recent rate constant determination by Ward and Rowley (2016) leads to significantly faster HOCl formation. Model simulations were performed to compare annual mean HOCl abundances (50∘ S–50∘ N) based on these different choices of , as shown in Fig. 7a; the percent differences (in panel b) indicate that 25 %–45 % larger HOCl abundances are obtained with the faster rate constant, depending on altitude. The issue of a fairly poorly determined HOCl formation rate constant has persisted for a number of years, affecting comparisons of balloon-borne HOCl profiles and model results (Kovalenko et al., 2007), as well as analyses of MIPAS HOCl observations (von Clarmann et al., 2009, 2012). Kovalenko et al. (2007) pointed out the need for a faster rate constant to improve agreement between modeled and measured HOCl, such as the rate constant measured by Stimpfle et al. (1979), in comparison to the current (at the time) value from the JPL Evaluation of Chemical Kinetics and Photochemical Data (Sander et al., 2006); this position was supported by the MIPAS measurements of HOCl and other species over Antarctica (von Clarmann et al., 2009). Using a temperature of 240 K, appropriate for the region of interest here, in previous temperature-dependent laboratory studies leads to five different rate constant values that have oscillated over time. Specifically, the values from Stimpfle et al. (1979), Nickolaisen et al. (2000), Knight et al. (2000), Hickson et al. (2007), and Ward and Rowley (2016), respectively, yield 11.3 × 10−12, 10.3 × 10−12, 6.6 × 10−12, 8.6 × 10−12, and 12.5 × 10−12 cm3 molec.−1 s−1, leading to an average of 9.7 with a (1σ) scatter of 2.1 or a range of about 3 if all five estimates are included. For comparison, the latest evaluation (Burkholder et al., 2019) gives an HOCl formation rate constant of 8.7 × 10−12 cm3 molec.−1 s−1, although that particular report did not take into account the work from Ward and Rowley (2016). However, making use of the Superconducting Submillimeter-Wave Limb-Emission Sounder (SMILES) HOCl, ClO, and HO2 data versus time of day, Kuribayashi et al. (2014) obtained a seemingly well-constrained estimate of for a limited temperature and pressure range (7.75 ± cm3 molec.−1 s−1 at 245 K in the upper stratosphere). This leads to a value of ∼ at 240 K (as inferred using an average temperature dependence), consistent with but slightly smaller than the latest evaluation's recommendation mentioned above. To summarize, we find that the differences between MLS and model values could well stem from a combination of uncertainties in both the MLS data and the model, and it is not possible to definitively attribute the discrepancy to one or the other data set. This discussion does not include other uncertainty sources (e.g., the photochemical loss rate of HOCl), as we believe that they are smaller in magnitude.
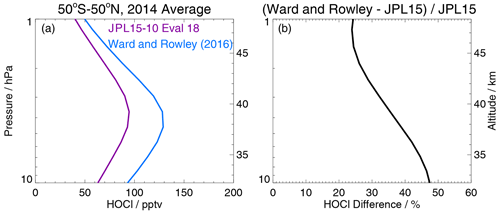
Figure 7(a) Sensitivity of average (for 2014, 50∘ S to 50∘ N) model upper stratospheric HOCl profile (pptv) to the choice of rate constant for the HOCl formation reaction between HO2 and ClO. The JPL 15-10 Evaluation 18 rate constant choice gives the purple average profile, whereas the larger rate constant derived by Ward and Rowley (2016) leads to the blue average profile. (b) The percent difference (increase) between the two curves in panel (a) (blue minus purple).
The MIPAS HOCl measurements were taken at about 10:00 and 22:00 local time during 2002–2004; the SMILES HOCl data cover the full diurnal cycle but only for part of 2009–2010. The ACE-FTS solar occultation (i.e., sunrise and sunset) measurements have recently included retrievals of stratospheric HOCl profiles (up to about 38 km), as discussed by Bernath et al. (2021). The various satellite measurements of near-global HOCl distributions are not easily compared, given their different local times and the non-negligible diurnal changes in HOCl (see SPARC, 2017). Upper stratospheric peak HOCl values from ACE-FTS, MIPAS, Aura MLS, and SMILES range from about 150 to 200 pptv, with MIPAS providing the largest values, as summarized by Bernath et al. (2021). Khosravi et al. (2013) provided a more detailed intercomparison of HOCl measurements from MIPAS, SMILES, and MLS in the upper stratosphere, with the help of model simulations of the diurnal cycle (and ClO intercomparisons were also discussed). Good agreement was obtained, overall, versus the expected HOCl diurnal variations, despite the noise in some of the data sets (with SMILES HOCl producing the least noisy data). In SPARC (2017), HOCl monthly zonal mean distributions from MIPAS, SMILES, and MLS were intercompared, albeit not for the same range of years (see also the recent update by Hegglin et al., 2021). Nighttime values were used, as this time period exhibits somewhat smaller changes versus local time than the daytime data. The MLS HOCl data were shown to be on the low side (by 20 % to 30 %) of both the MIPAS and SMILES results, with the SMILES values lying between the MLS and MIPAS values; a low bias in MLS HOCl was also seen in the comparisons presented by Khosravi et al. (2013). However, those studies used v3 HOCl data from the standard MLS product. Mean differences between v3 HOCl and v5 HOCl are on the order of 5 %–10 %, with the v5 data on the low side of v3. More to the point, the offline HOCl retrievals yield larger values, by about 25 %, than the monthly zonal means from the standard v5 product, as can be seen from a comparison of Fig. 6 for the offline MLS HOCl climatology versus Fig. S2 for the standard MLS HOCl product. The HOCl offline data values are thus about 20 % larger than the v3 MLS standard product values, so that much of the MLS low bias versus MIPAS and SMILES is mitigated by using the offline MLS HOCl product. It follows from the above comments that the WACCM6 values will also significantly underestimate the HOCl abundances from MIPAS and SMILES. Based on the above references discussing past satellite data intercomparisons for HOCl, the (2σ) systematic uncertainties for non-MLS HOCl data sets are likely larger than 10 %–15 %. The MLS v5 HOCl uncertainties are in the 40–80 pptv range (see Livesey et al., 2020), or at least ∼ 25 % (and significantly more in the lower part of the upper stratosphere); it is reasonable to expect that the offline MLS HOCl product will be affected by very similar systematic uncertainties as the MLS standard product. In summary, we cannot expect much better agreement between the various HOCl data sets than the (roughly) 20 % level of agreement implied here.
Turning to the derived trends in HOCl, these will not be affected much (in units of % yr−1) by mean differences between measured and modeled climatological values. As was done earlier for the ClO time series, we show sample daytime HOCl time series, fits, and residuals in Fig. 8. We observe from such time series that, apart from the absolute value difference between MLS and model HOCl, the measured seasonal cycle is well reproduced by the model; less photochemical destruction of upper stratospheric HOCl during the winter months accounts for the wintertime high values in the region shown (top panel). The residuals in this example (and in general) are larger, by at least a factor of 2, than those for ClO, and the correlation coefficients for the fits and for model versus data are poorer, especially when comparing regression fits to the data and (debiased) model fits to the data; the poorer fits arise because the MLS HOCl data set is noisier (even for monthly zonal means) than is ClO. Thus, in the case of HOCl, the regression fits to the model give the best results, in terms of correlation coefficients between the regression fits to the MLS or model series, as well as for the debiased model curves in comparison to the data and regarding root-mean-square residuals (as derived from data such as the curves in the bottom panel of Fig. 8). The derived trends for HOCl are shown in Fig. S3 as a function of latitude from 2.2 to 10 hPa. Many of the MLS-derived trends at specific pressures and latitudes are not statistically different from a zero-trend value, while the model-derived trends are typically negative (with values that are more negative than the measured trends) and statistically different from zero. Figure 9 provides a summary of the results for MLS and model HOCl trends, with day and night data shown separately, after multiple regression is applied to the averaged 50∘ S–50∘ N time series. For MLS data between 3 and 7 hPa, we obtain statistically significant decreasing near-global HOCl trends, both day and night. These results provide an unambiguous indication of decreasing upper stratospheric trends in HOCl, given that negative trend center values occur at all retrieval levels. There is no statistically significant difference between the nighttime and daytime results for either the MLS data or the model. The average model HOCl trend (−0.6 % yr−1) is more negative than the average MLS result (−0.4 % yr−1), although this is not a statistically significant difference, given the (2σ) error bars shown in Fig. 9 and the fact that the MLS HOCl vertical resolution is about 6 km, so there are really only about three independent retrieval levels in the pressure range displayed in Fig. 9 (and any error reduction for averaged results over all pressures would be by a factor of or 1.7, at best). However, the nighttime model and data trends at 2 hPa agree better than the daytime results, with the nighttime MLS trends exhibiting a more homogeneous behavior versus pressure than the daytime MLS trends. This is likely caused by the larger MLS signal for nighttime HOCl (see the climatological values in Fig. S1 versus the daytime values in Fig. 6); the nighttime MLS trend errors are also smaller than the corresponding daytime errors.
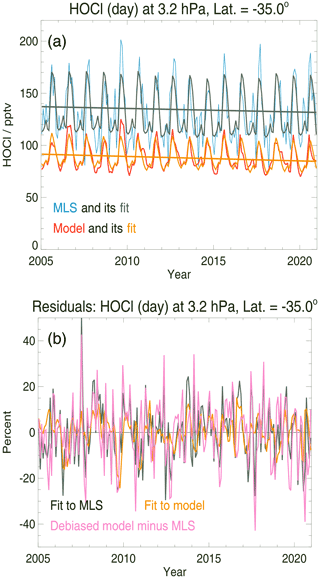
Figure 8Same as Fig. 2 except for an example at 3.2 hPa for 30 to 40∘ S (a) HOCl time series and regression fits and (b) percent residuals.
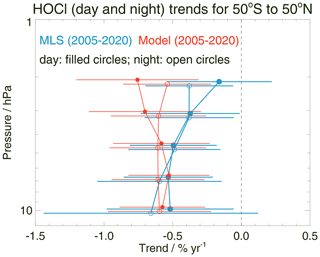
Figure 9Same as Fig. 5 except for trend results for HOCl from both day (filled circles) and night (open circles) time series analyses between 10 and 2.2 hPa.
We show in Fig. 10 a summary of the trend profiles for ClO and HOCl, both based on daytime results. We mentioned above that the nighttime HOCl results agree well with those from daytime HOCl and display better agreement versus the model nighttime results at 2 hPa. For ClO, we have also checked that nighttime trends over a limited pressure range (from 1.5 to 3.2 hPa) agree with the daytime trends (not shown), but nighttime ClO values are typically much smaller than those during the day at pressures larger than 4 hPa, where we found that no robust nighttime ClO trends can be obtained from the MLS data. Figure 10 demonstrates that both of these chlorine species have decreased over much of the globe during the past 16 years, with the ClO trends being more negative (by ∼ 0.35 % yr−1) than the trends in HOCl, both in the model and the observational results. Limiting results to an average over the uppermost stratosphere (between 2.2 and 6.8 hPa for both species), the (daytime) MLS-derived near-global upper stratospheric trends are −0.73 ± 0.40 % yr−1 for ClO and −0.39 ± 0.35 % yr−1 for HOCl. The (2σ) error bars here are the root-mean-square value applicable to this vertical range, with no reduction in error bars for the broader region; we would rather use a somewhat more conservative uncertainty than one that is too “optimistic” (such as an error reduction by a factor of 2 for ClO, which assumes uncorrelated errors between pressure levels). The corresponding model trends for this vertical range are −0.85 ± 0.45 % yr−1 for ClO and −0.64 ± 0.37 % yr−1 for HOCl. Even if the HOCl trends are not significantly different from the ClO trends at any given level, when averaged, these differences do become more significant.
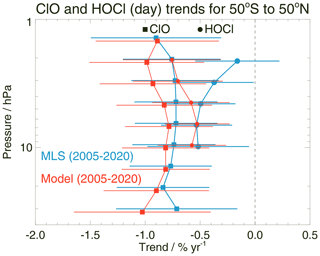
Figure 10Derived upper stratospheric trends in ClO (filled squares) and HOCl (filled circles) based on regression fits to daytime monthly zonal mean time series for both species, for 50∘ S to 50∘ N averages from 2005 through 2020; MLS results are in blue and model results in red.
In terms of the time series variability and the regression fits, the largest components are, by far, the annual and semi-annual terms (with their relative impacts somewhat dependent on latitude and pressure). For both the observed and modeled near-global cases shown in Fig. 10, about 70 %–80 % of the explained variance arises from these two terms. The ENSO and solar terms typically account for less than a few percent of the explained variance, and the same is true for short-period (less than 6-month) terms. The QBO signal is generally the largest component that remains, if one considers near-global deseasonalized percent anomaly time series, as seen in Fig. 11 for ClO and HOCl at two upper stratospheric pressure levels. The data and model fits generally behave in similar ways, although there can be some small differences between the two. The correlation coefficients between observed HOCl and ClO anomaly time series are on the order of 0.6–0.7 in the upper stratosphere (with values close to 0.8 if one smoothes out some of the short-term variability in the time series first). The model ClO anomalies track the observed anomalies quite well (with correlation coefficients close to 0.8). We provide the percent residuals associated with Fig. 11 in Fig. S4; these tend to be about twice as large for HOCl (on the order of ± 10 %) as those for ClO (on the order of ± 5 %).
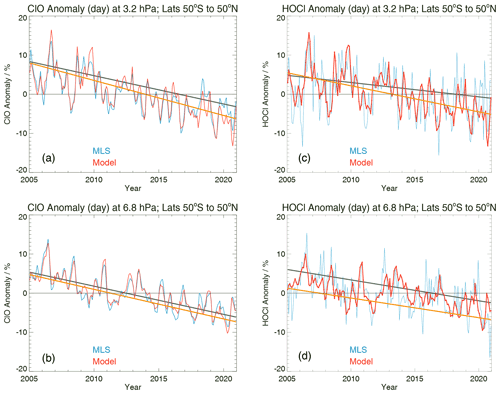
Figure 11Deseasonalized anomaly time series (percent) of MLS (blue) and WACCM (red) 50∘ S–50∘ N averages over the period 2005 through 2020 for (a) ClO at 3.2 hPa, (b) ClO at 6.8 hPa, (c) HOCl at 3.2 hPa, and (d) HOCl at 6.8 hPa. The linear components of the multivariate linear regression fits are given by dark gray and orange lines for MLS and WACCM, respectively. The associated percent residuals are provided in Fig. S4.
We now review our estimated trends in the context of past results, and we discuss potential reasons for different trends in various chlorine species in the upper stratosphere, including the slower decrease in upper stratospheric HOCl in comparison to the ClO decrease. As a reminder of the relative importance of the main inorganic chlorine species in the upper stratosphere, we display in Fig. 12 the percent contribution to total inorganic chlorine (Cly) over the 10 to 1 hPa range, based on the climatological (daytime) model results over 50∘ S–50∘ N for the time period analyzed here. The Cly abundance includes all species contributions from HCl, ClO, HOCl, and ClONO2, which are shown in the plot, as well as very minor contributions from Cl, Cl2, Cl2O2, OClO, and BrCl. The “Sum” curve shown on the right side of this figure is just the sum from the four main species whose contributions are plotted; this does not quite equal 100 % because of the very small (daytime) relative contributions from the latter five species. HCl is clearly the dominant reservoir in the upper stratosphere, as it makes up about 80 %–95 % of total inorganic chlorine in this region (see also Froidevaux et al., 2006), while ClO makes up about 5 %–15 % of the total, with minor contributions from ClONO2 and HOCl, both at the few percent level for most of this region.
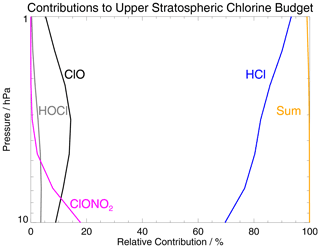
Figure 12Percent contributions of various species (daytime HCl, ClO, HOCl, and ClONO2) to the upper stratospheric chlorine budget between 10 and 1 hPa, based on climatological (16 years) daytime model results in the 50∘ S to 50∘ N latitude range. The sum of these contributions is shown in orange; there are also very small contributions in this pressure range from other species (Cl, Cl2, Cl2O2, OClO, BrCl, which are not represented here).
While published trends in chlorine species can be compared, there will always be some differences in the results, given the different measurement locations, coverage, and time periods being considered. We note that the surface maximum in total chlorine was reached in 1992–1993; following the fast initial decrease in methyl chloroform (CH3CCl3), tropospheric chlorine declined at a slower rate (O'Doherty et al., 2004). There is also evidence for slightly slower decreases in the ACE-FTS upper stratospheric HCl time series after about 2010 (Bernath and Fernando, 2018; Bernath et al., 2020a), in comparison to the rate of decline over the 2004–2010 period. In terms of the MLS ClO results discussed here, the upper stratospheric trend (for 2005–2020) of −0.73 ± 0.40 % yr−1 can be compared to other estimated trends in upper stratospheric ClO. Jones et al. (2011) reported upper stratospheric ClO trends of −0.7 ± 0.8 % yr−1 for 2001 through 2008, based on a combination of Odin Sub-Millimetre Radiometer (SMR) and Aura MLS data over the tropics; the estimated uncertainty in this satellite-based ClO trend is quite large, but the trend estimate is consistent with our result covering a longer time period. Solomon et al. (2006) displayed the rise and decline of upper stratospheric ClO abundances in the 1982–2004 time period, based on microwave ground-based profile data from Hawaii. However, the fairly large ClO trend (−1.5 % yr−1) initially obtained by these authors for 1995–2004 was superseded by analyses of an improved data set over a longer time period using a new methodology (Connor et al., 2013), which led to a ClO trend estimate (at about 4 hPa) of −0.65 ± 0.15 (2σ) % yr−1 over the 1995–2012 period. Thus, we find good consistency between our MLS results and previous trend estimates for ClO, especially given the differences in measurement coverage and time periods considered.
For the HOCl trends, we are aware of only one prior result, a recent trend estimate based on ACE-FTS HOCl data by Bernath et al. (2021), who quote a marginally significant trend of −0.23 ± 0.22 (2σ) pptv yr−1, which we translate to about −0.19 ± 0.18 % yr−1, given mean HOCl abundances (of 124 pptv) from their analysis of ACE-FTS data at 30–39 km and 60∘ S–60∘ N from 2004–2020. This can be compared to our near-global MLS HOCl trend estimate of −0.39 ± 0.35 % yr−1 for a very similar time period; while these two estimates agree within the fairly large uncertainty estimates, the MLS mean trend value represents twice as rapid a decrease as the mean ACE-FTS trend result. At this time, the cause of these differences is not known, although these measurements are among the more difficult for both instruments, and the two sampling patterns are quite different. We note that the model upper stratospheric HOCl trend is faster (at −0.64 ± 0.37 % yr−1) than the MLS-derived trend and even faster in comparison to the ACE-FTS result.
We now turn to some additional model results, as well as other relevant measurements from MLS and ACE-FTS, to discuss upper stratospheric trends in chlorine and related species in a broader context. Figure 13 shows the derived average trends in various upper stratospheric chlorine species based on our regression analyses of measured and modeled time series for monthly zonal means from 50∘ S to 50∘ N. The near-global upper stratospheric trend values in Fig. 13 are obtained from trends like those in Fig. 10 for MLS ClO and HOCl but averaged from 6.8 to 2.2 hPa. Error bars represent typical 2σ estimates, calculated from the root mean square of the 2σ estimates for pressures in the 6.8 to 2.2 hPa range; we prefer to use this more conservative error rather than the standard error in the mean, which will typically be an underestimate, since errors from different pressure levels are not completely uncorrelated. As mentioned earlier, no useful MLS-based estimate of HCl trends in the upper stratosphere could be obtained after the related MLS hardware degradation in early 2006. MLS HCl measurements are still scientifically useful in the lower stratosphere, even for trends (see the related model/data analysis by Froidevaux et al., 2019), and certainly they accurately capture the larger seasonal, interannual, and winter polar vortex HCl variations. To derive the trends based on ACE-FTS data shown in Fig. 13, we have used seasonally averaged time series of v4.1 measurements, a methodology used in previous investigations of ACE-FTS trends to lessen the impacts of that instrument's sampling patterns (see Bernath and Fernando, 2018). We have applied a simple linear fit to the deseasonalized anomalies from ACE-FTS seasonal means (from 50∘ S to 50∘ N), thus using the same type of analysis as in the latter reference. In this approach, the auto-correlation of the residuals is taken into account by following the methodology described by Tiao et al. (1990) and Weatherhead et al. (1998); the auto-correlation is assumed to follow a first-order autoregressive model, and the trend error bars are multiplied by a factor that depends on the autoregressive coefficient. We also point out that it would be more complicated to apply the MLR approach used for the MLS and model time series to the ACE-FTS seasonal data, as the MLR method we have used is based on monthly proxy values. A careful intercomparison of different approaches to estimate error bars in various trends analyses is beyond the scope of this paper, although such an intercomparison would be helpful.
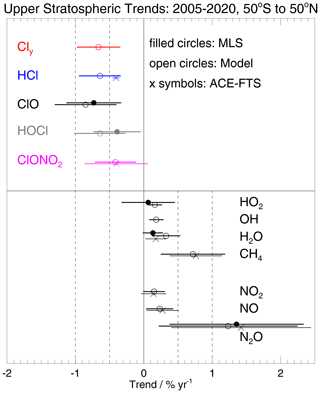
Figure 13Upper stratospheric trends in various species from 6.8 to 2.2 hPa for 50∘ S to 50∘ N, based on linear trends obtained from the regression fits to daytime time series of MLS data (filled circles) and/or model series (open circles); x symbols are from our analysis of (50∘ S to 50∘ N) ACE-FTS version 4.1 data over the 33 to 43 km range (see text). Error bars represent uncertainties (2σ), derived as described in the text.
We see in Fig. 13 (as was shown in Fig. 10) that the MLS ClO trend is more negative than the MLS HOCl trend; this is also true for the model results in Fig. 13, and the model ClO trend is also more negative than the model Cly and HCl trends (with respective values of −0.66 ± 0.30 % yr−1 and −0.64 % ± 0.30 % yr−1 (2σ)). The faster ClO decrease (versus Cly or HCl) seen in Fig. 13 is tied to the dependence of ClO on other species. More specifically, the ClO abundance ([ClO]) is roughly proportional to [HCl] [H2O] [CH4] (see Froidevaux et al., 2000). The model and observed trends in both H2O and CH4 agree well (see the bottom portion of Fig. 13). Here, we have averaged all ACE-FTS (50∘ S to 50∘ N) trends between 33 and 43 km, based on all sunrise and sunset profiles combined. The MLS v5 H2O trend of 0.13 ± 0.15 (2σ) % yr−1 is close to the trend we obtain from ACE-FTS data, at 0.18 ± 0.15 % yr−1 (which is in reasonable agreement with the near-global mid-stratospheric H2O trend of 0.24 % yr−1 provided in the broad overview of ACE-FTS trends by Bernath et al., 2020a). Although the MLS v4 H2O data suffered from a drift that led to trends that were too large, this drift has been largely mitigated in the v5 H2O data used here (Livesey et al., 2021). The measured trend in CH4, also obtained from ACE-FTS data, and the model CH4 trend (in very good agreement with the ACE-FTS trend) are significantly larger than the trends in H2O; more CH4 will thus lead, in time, to less chlorine in the form of ClO, which means a faster rate of decrease for ClO. The photochemical balance for HOCl, on the other hand, leads to [HOCl] being roughly proportional to [ClO] [HO2] ( [OH] + kHOCl+O [O]), where JHOCl is the photodissociation rate constant for HOCl, and the rate constants indicate which HOCl production or destruction reaction we are referring to. In the mid to upper stratosphere, the J term clearly dominates (see Chance et al., 1989, and also based on our diagnostics for the WACCM run used here), and we would thus expect the trend in HOCl to be less negative than the trend in ClO, given that the HO2 trend is (slightly) positive (per Fig. 13). The MLS-derived trend for HO2 comes from our analysis of the offline MLS HO2 product (see Millán et al., 2015). As recommended for this product, we performed our trend analysis using day minus night differences; that is, we constructed such monthly zonal means from the set of day and night daily zonal means; the model and data HO2 trends agree within the error bars, although the MLS error bar is quite large. The model OH trend also points to a slight positive trend, which likely stems from the increasing trends in H2O. Algebraically, a percent change in HOCl will be driven by the percent change in ClO added to the percent change in HO2, so the decreasing trend in HOCl is slowed, relative to the ClO trend, by the increasing trend in HO2. Using the modeled HO2 trend in Fig. 13 (∼ 0.2 % yr−1), which is consistent with the observed HO2 trend, one could expect the HOCl trend to lie ∼ 0.2 % closer to zero than the ClO trend; this is consistent (within the error bars) with both the modeled and measured trend differences between HOCl and ClO (these differences are ∼ 0.2 % and 0.3 %, respectively, for the model and for the measurements).
The ClONO2 trends shown in Fig. 13 are less negative than the ClO trends; this likely stems from the slightly positive trends in NO2, which can mitigate the extent of the decrease in ClONO2 (formed from ClO and NO2). We also note that the differences between the model and ACE-FTS HCl trends are somewhat larger than those between the model and MLS ClO, although the error bars in Fig. 13 indicate that none of these differences are statistically significant. It has been shown that the better sampling from emission-type measurements can provide more reliable trend estimates than in the case of sparser sampling (e.g., from occultation-type data; see Millán et al., 2016). We expect that sampling differences between ACE-FTS and MLS (or the model) contribute part of the trend differences versus MLS (or the model). In this regard, error bars in the ACE-FTS trends are likely to be smaller than the errors that would be obtained from a more fully sampled data set with less data averaging (and thus with more spatiotemporal variability).
While this is less pertinent to the chlorine species trends, we find it interesting that the N2O trends in Fig. 13 appear to be much larger than the trends in NO and NO2, which are two radicals that are the products of N2O destruction in the upper stratosphere; MLS, ACE-FTS, and the model results all point to upper stratospheric trends slightly larger than 1 % yr−1, albeit with comparable 2σ uncertainties. Some of this difference might be caused by the strong latitude dependence of the N2O trends, coupled with large trend uncertainties in a region with rapidly decreasing abundances with height; the N2O trends from ACE-FTS at lower altitudes yield small positive values that are more consistent with the NOx trends shown here, and also with tropospheric N2O trends (see also Bernath et al., 2020a). We note also that the MLS N2O trends likely constitute lower limits, given that there are some unmitigated negative drifts in the version 5 MLS N2O time series in the lower stratosphere, even after the improvements versus the v4 data (Livesey et al., 2021). Finally, there are also temperature-related effects that could potentially modify the partitioning of chlorine species over the long-term. However, since the average upper stratospheric temperature decrease over the past 16 years is less than 1K (e.g., Steiner et al., 2020), the temperature dependence issue for this time period should not lead to a significant perturbation of chlorine species trends and chlorine partitioning in this region. For the ClO or HOCl photochemical balance in particular, the strongest temperature dependence (by far) is from the Cl + CH4 reaction, but even this would lead to a fairly small (15 %–30 %) perturbation (for the cooling rate implied above) in comparison to the impact of the CH4 trend or versus the trends in the chlorine species themselves.
We have provided above a few arguments that can help explain some of the differences in upper stratospheric chlorine species trends summarized in Fig. 13. The full chemistry–climate model takes all the (modeled) factors into account, both regarding photochemical balance issues and any underlying dynamical factors, such as variations and trends in long-lived tracers that can also impact shorter-lived species.
We have analyzed Aura MLS monthly zonal mean time series of ClO and HOCl between 50∘ S and 50∘ N to estimate upper stratospheric trends in these chlorine species from 2005 through 2020. We compare these observations to those from a state-of-the-art chemistry–climate model, WACCM6, run under the specified dynamics configuration, with MERRA-2 meteorological constraints and sampled for the same time period; in addition, the model sampling follows the MLS coverage in space and local time. We use version 5 MLS ClO zonal mean (level 3) daytime profiles (associated with solar zenith angles less than 90∘) and, for comparison, similarly binned daytime ClO model profiles. For MLS HOCl, we use the version 5 offline product derived from daily zonal mean radiances (in 10∘ latitude bins) rather than averaged level-2 profiles; MLS HOCl is scientifically useful between 10 and 2 hPa, and HOCl monthly zonal means are separated into day and night averages (solar zenith angles greater than 100∘ for night conditions), for comparison, to similarly binned WACCM6 HOCl profiles.
We find good agreement (mostly within about 10 %) between the climatological MLS daytime ClO distributions and the corresponding model ClO climatology for 2005–2020. The model HOCl climatology, however, underestimates the MLS HOCl climatology by about 30 % (for both daytime and nighttime). This discrepancy could well be caused by a combination of fairly large systematic uncertainties in both the model-assumed rate constant for the formation of HOCl and the MLS HOCl retrievals themselves, although we note that these model results would likely also underestimate other satellite measurements of HOCl. The model daytime ClO trends versus latitude and pressure agree well with those from MLS ClO. Between 15 and 32 hPa, there are indications of some interhemispheric asymmetry in the MLS ClO trends, with faster decreases at northern than at southern midlatitudes, although this is not statistically significant; there is also evidence for low-frequency (multi-year) variability, especially at 32 hPa. Further investigations of interhemispheric asymmetries in lower stratospheric trends (and related age of air issues) are probably best pursued through detailed studies of longer-lived species than ClO.
MLS-derived near-global upper stratospheric daytime trends between 7 and 2 hPa are −0.73 ± 0.40 % yr−1 for ClO and −0.39 ± 0.35 % yr−1 for HOCl, with 2σ uncertainty estimates used here. The corresponding near-global upper stratospheric model trends are −0.85 ± 0.45 % yr−1 for ClO and −0.64 ± 0.37 % yr−1 for HOCl. Both data and model results point to a slower trend for HOCl than for ClO. The MLS trends for ClO are generally consistent with past estimates of upper stratospheric ClO trends, based on a combination of Odin/SMR and MLS data from 2001–2008 (Jones et al., 2011) and based on ground-based microwave results from Hawaii for 1995–2012 (Connor et al., 2013). The MLS HOCl trend represents a faster rate of change (by about a factor of 2) than the marginally significant trend (−0.19 ± 0.18 (2σ) % yr−1) that Bernath et al. (2021) obtained from a recent analysis of ACE-FTS HOCl measurements from 2004–2020.
Our general overview (Fig. 13) shows decreasing near-global trends for all the measured upper stratospheric chlorine species. Differences can arise as a result of the impact of trends in other gases that can affect the slowly varying photochemical equilibrium for different species in this region. Notably, observed and modeled positive trends in CH4 will tend to steepen the decrease of active chlorine (ClO values) in comparison to trends in HCl or Cly. Regarding trends in HOCl, positive trends in HO2 can lead to a faster rate of formation for HOCl as a function of time, which partially offsets the impact of decreases in ClO (also involved in HOCl production).
Lastly, the decreasing trends in upper stratospheric ClO and HOCl that are arrived at in this work provide additional confirmation of the effectiveness of the Montreal Protocol and its amendments, which have led to the early stages of an expected long-term ozone recovery from the effects of ozone-depleting substances (see WMO, 2018). Indeed, the known decreases in surface chlorine since the early 1990s, which are faithfully included in the model results, have played a major role in the decreasing trends of ClO and HOCl over the 2005–2020 time period.
The Aura MLS data files used here are available from NASA GSFC DISC (http://disc.sci.gsfc.nasa.gov/Aura/data-holdings/MLS; Livesey et al., 2020) and downloaded to the Jet Propulsion Laboratory computers with small delays (from a few days to a few months at most). ACE-FTS observations are available, following registration, from https://doi.org/10.20383/101.0291 (Bernath et al., 2020b), with the data quality information available at https://doi.org/10.5683/SP2/BC4ATC (Sheese and Walker, 2020). The public site ftp://ftp.seismo.nrcan.gc.ca/spaceweather/solar_flux/monthly_averages/solflux_monthly_average.txt was used to download monthly mean values of the Canadian F10.7 solar flux measurements included in our regression fits. The solar flux data set has been described by Tapping (2013); we thank Space Weather Canada for the public access to this data set. We thank NASA’s Global Monitoring and Assimilation Office (GMAO) for providing data for Modern-Era Retrospective analysis for Research and Applications, Version 2 (MERRA-2). This NASA data set is public and can be downloaded from https://gmao.gsfc.nasa.gov/reanalysis/MERRA-2/data_access/ (last access: 1 September 2021) (Gelaro et al., 2017) to constrain the WACCM6 model runs used here. The WACCM6 model results shown in this paper are available online at https://acomstaff.acom.ucar.edu/dkin/ACP_Froidevaux_2021/ (last access: 1 September 2021) (Gettelman et al., 2019).
The supplement related to this article is available online at: https://doi.org/10.5194/acp-22-4779-2022-supplement.
LF prepared this article with contributions from all co-authors. DEK provided inputs for running the necessary model runs, as well as properly averaged and formatted outputs from the model, along with various contributions to the main text and figures. MLS provided assistance in the validation and generation of the MLS ClO data, along with comments on the article. LFM provided the MLS HOCl offline products and related expertise, along with comments on the article. NJL provided leadership for MLS overall, along with comments regarding the article. WGR provided leadership for the MLS forward model and data retrievals, along with measurement science expertise and related article comments. CGB provided assistance towards obtaining the model runs, as well as comments regarding the model description. JJO provided assistance regarding the available laboratory data on the HOCl formation rate constant and its related uncertainties, along with article comments. RAF provided properly formatted and averaged MLS data sets for the various species analyzed in this work.
The contact author has declared that neither they nor their co-authors have any competing interests.
Publisher’s note: Copernicus Publications remains neutral with regard to jurisdictional claims in published maps and institutional affiliations.
We are thankful to the whole MLS team (past and present) for their contributions over the years to the MLS instrument, data, processing, and database management; all this has contributed to making this research work possible. We also gratefully acknowledge the work of the whole ACE-FTS team in producing and sharing their updated data sets, as these were used here as part of the discussion and comparisons. The Atmospheric Chemistry Experiment (ACE), also known as SCISAT, is a Canadian-led mission mainly supported by the Canadian Space Agency. F10.7 data collection and dissemination are supported by the National Research Council of Canada, with the participation of Natural Resources Canada and support by the Canadian Space Agency. Douglas E. Kinnison was funded in part by NASA. WACCM is a component of the CESM, supported by the National Science Foundation (NSF). We would like to acknowledge high-performance computing support from Cheyenne (https://doi.org/10.5065/D6RX99HX) provided by NCAR's Computational and Information Systems Laboratory, sponsored by the NSF. Work at the Jet Propulsion Laboratory, California Institute of Technology, was performed under contract with the National Aeronautics and Space Administration (80NM0018D0004). We also gratefully acknowledge the reviewers of this work for their comments and suggestions.
This research has been supported by the National Aeronautics and Space Administration (grant nos. 80NM0018D0004 and 105357-444491.02.03.02.91). Douglas E. Kinnison was funded for this work under NASA (grant no. 80NSSC20K0926).
This paper was edited by Thomas von Clarmann and reviewed by two anonymous referees.
Anderson, J., Margitan, J. J., and Stedman, D. H.: Atomic Chlorine and the Chlorine Monoxide Radical in the Stratosphere: Three in situ Observations, Science, 198, 4316, https://doi.org/10.1126/science.198.4316.501, 1977.
Anderson, J., Russell III, J. M., Solomon, S., and Deaver, L. E.: Halogen Occultation Experiment confirmation of stratospheric chlorine decreases in accordance with the Montreal Protocol, J. Geophys. Res., 105, 4483–4490, 2000.
Bernath, P. F.: The Atmospheric Chemistry Experiment (ACE), J. Quant. Spectrosc. Ra., 186, 3–16, https://doi.org/10.1016/j.jqsrt.2016.04.006, 2017.
Bernath, P. and Fernando, A. M.: Trends in stratospheric HCl from the ACE satellite mission, J. Quant. Spectrosc. Ra., 217, 126–129, https://doi.org/10.1016/j.jqsrt.2018.05.027, 2018.
Bernath P. F., Steffen J., Crouse J., and Boone C. D.: Sixteen-year trends in atmospheric trace gases from orbit, J. Quant. Spectrosc. Ra. 253, 107178, https://doi.org/10.1016/j.jqsrt.2020.107178, 2020a.
Bernath, P., Steffen, J., Crouse, J., and Boone, C.: Atmospheric Chemistry Experiment SciSat Level 2 Processed Data, v4, Federated Research Data Repository [data set], https://doi.org/10.20383/101.0291, 2020b.
Bernath, P. F., Crouse J., Hughes, R. C., and Boone C. D.: The Atmospheric Chemistry Experiment Fourier Transform Experiment (ACE-FTS) version 4.1 retrievals: Trends and seasonal distributions, J. Quant. Spectrosc. Ra., 259, 107409, https://doi.org/10.1016/j.jqsrt.2020.107409, 2021.
Boone, C. D., Bernath, P. F., Cok, D., Jones, S. C., and Steffen, J.: Version 4 retrievals for the Atmospheric Chemistry Experiment Fourier Transform Spectrometer (ACE-FTS) and imagers, J. Quant. Spectrosc. Ra., 247, 106939, https://doi.org/10.1016/j.jqsrt.2020.106939, 2020.
Bourassa, A. E., Degenstein, D. A., Randel, W. J., Zawodny, J. M., Kyrölä, E., McLinden, C. A., Sioris, C. E., and Roth, C. Z.: Trends in stratospheric ozone derived from merged SAGE II and Odin-OSIRIS satellite observations, Atmos. Chem. Phys., 14, 6983-6994, https://doi.org/10.5194/acp-14-6983-2014, 2014.
Burkholder, J. B., Sander, S. P., Abbatt, J. P. D., Barker, J. R., Huie, R. E., Kolb, C. E., Kurylo, M. J., Orkin, V. L., Wilmouth, D. M., and Wine, P. H.: Chemical kinetics and photochemical data for use in atmospheric studies: Evaluation No. 18, JPL Publication 15-10, Jet Propulsion Laboratory, California Institute of Technology, Pasadena, http://jpldataeval.jpl.nasa.gov (last access: 1 March 2021), 2015.
Burkholder, J. B., Sander, S. P., Abbatt, J. P. D., Barker, J. R., Cappa, C., Crounse, J. D., Dibble, T. S., Huie, R. E., Kolb, C. E., Kurylo, M. J., Orkin, V. L., Percival, C. J., Wilmouth, D. M., and Wine, P. H.: Chemical kinetics and photochemical data for use in atmospheric studies, Evaluation No. 19, JPL Publication 19-5, Jet Propulsion Laboratory, California Institute of Technology, Pasadena, http://jpldataeval.jpl.nasa.gov (last access: 1 March 2021), 2019.
Chance, K. V., Johnson, D. G., and Traub, W. A.: Measurement of stratospheric HOCl concentration profiles including diurnal variation, J. Geophys. Res., 94, 11059–11069, 1989.
Coddington, O., Lean, J., Pilewskie, P., Snow, M., and Lindholm, D.: A solar irradiance climate data record, B. Am. Meteorol. Soc., 97, 1265–1282, https://doi.org/10.1175/BAMS-D-14-00265.1, 2016.
Connor, B. J., Mooney, T., Nedoluha, G. E., Barrett, J. W., Par- rish, A., Koda, J., Santee, M. L., and Gomez, R. M.: Re-analysis of ground-based microwave ClO measurements from Mauna Kea, 1992 to early 2012, Atmos. Chem. Phys., 13, 8643–8650, https://doi.org/10.5194/acp-13-8643-2013, 2013.
Danabasoglu, G., Lamarque, J.-F., Bacmeister, J., Bailey, D. A., DuVivier, A. K., Edwards, J., Emmons, L. K., Fasullo, J., Garcia, R., Gettelman, A., Hannay, C., Holland, M. M., Large, W. G., Lauritzen, P. H., Lawrence, D. M., Lenaerts, J. T. M., Lindsay, K., Lipscomb, W. H., Mills, M. J., Neale, R., Oleson, K. W., Otto-Bliesner, B., Phillips, A. S., Sacks, W., Tilmes, S., van Kampenhout, L., Vertenstein, M., Bertini, A., Dennis, J., Deser, C., Fischer, C., Fox-Kemper, B., Kay, J. E., Kinnison, D. E., Kushner, P. J., Larson, V. E., Long, M. C., Mickelson, S., Moore, J. K., Nienhouse, E., Polvani, L., Rasch, P. J., and Strand, W. G.: The Community Earth System Model Version 2 (CESM2), J. Adv. Model. Earth Syst., 12, e2019MS001916, https://doi.org/10.1029/2019MS001916, 2020.
Efron, B. and Tibshirani, R.: An Introduction to the Bootstrap, Monographs on Statistics and Applied Probability 57, Chapman and Hall, CRC Press, 456 p., ISBN: 0412042312, 1993.
Emmons, L. K., Schwantes, R. H., Orlando, J. J., Tyndall, G., Kinnison, D., Lamarque, J.-F., Marsh, D., Mills, M. J., Tilmes, S., Bardeen, C., Buchholz, R. R., Conley, A., Gettelman, A., Garcia, R., Simpson, I., Blake, D. R., Meinardi, S., and Pétron, G.: The Chemistry Mechanism in the Community Earth System Model version 2 (CESM2), J. Adv. Model. Earth Syst., 12, e2019MS001882, https://doi.org/10.1029/2019MS001882, 2020.
Engel, A., and Rigby, M. (Lead Authors), Burkholder, J. B., Fernandez, R. P., Froidevaux, L., Hall, B. D., Hossaini, R., Saito, T., Vollmer, M. K., and Yao, B.: Update on Ozone-Depleting Substances (ODSs) and Other Gases of Interest to the Montreal Protocol, Chapter 1 in Scientific Assessment of Ozone Depletion: 2018, Global Ozone Research and Monitoring Project – Report No. 58, World Meteorological Organization, Geneva, Switzerland, 588 p., ISBN: 978-1-7329317-1-8, https://ozone.unep.org/science/assessment/sap (last access: 1 March 2021), 2018.
Farman, J., Gardiner, B., and Shanklin, J.: Large losses of total ozone in Antarctica reveal seasonal ClOx NOx interaction, Nature, 315, 207–210, https://doi.org/10.1038/315207a0, 1985.
Froidevaux, L., Waters, J. W., Read, W. G., Connell, P. S., Kinnison, D. E., and Russell, J. M.: Variations in the free chlorine content of the stratosphere (1991–1997): Anthropogenic, volcanic, and methane influences, J. Geophys. Res., 105, 4471–4481, 2000.
Froidevaux, L., Livesey, N. J., Read, W. G., Salawitch, R. J., Waters, J. W., Drouin, B., MacKenzie, I. A., Pumphrey, H. C., Bernath, P., Boone, C., Nassar, R., Montzka, S., Elkins, J., Cunnold, D., and Waugh, D.: Temporal decrease in upper atmospheric chlorine, Geophys. Res. Lett., 33, L23813, https://doi.org/10.1029/2006GL027600, 2006.
Froidevaux, L., Kinnison, D. E., Wang, R., Anderson, J., and Fuller, R. A.: Evaluation of CESM1 (WACCM) free-running and specified dynamics atmospheric composition simulations using global multispecies satellite data records, Atmos. Chem. Phys., 19, 4783–4821, https://doi.org/10.5194/acp-19-4783-2019, 2019.
Gelaro, R., McCarty, W., Suarez, M. J., Todling, R., Molod, A., Takacs, L., Randles, C. A., Darmenov, A., Bosilovich, M. G., Reichle, R., Wargan, K., Coy, L., Cullather, R., Draper, C., Akella, S., Buchard, V., Conaty, A., da Silva, A. M., Gu, W., Kim, G.-K., Koster, R., Lucchesi, R., Merkova, D., Nielsen, J. E., Partyka, G., Pawson, S., Putman, W., Rienecker, M., Schubert, S. D., Sienkiewicz, M., and Zhao, B.: The Modern-Era Retrospective Analysis for Research and Applications, Version 2 (MERRA2), J. Clim., 30, 5419–5454, https://doi.org/10.1175/JCLI-D-16-0758.1, 2017 (data available at: https://gmao.gsfc.nasa.gov/reanalysis/MERRA-2/data_access/).
Gettelman, A., Mills, M. J., Kinnison, D. E., Garcia, R. R., Smith, A. K., Marsh, D. R., Tilmes, S., Vitt, F., Bardeen, C. G., McInerny, J., Liu, H.-L., Solomon, S. C., Polvani, L. M., Emmons, L. K., Lamarque, J.-F., Richter, J. H., Glanville, A. S., Bacmeister, J. T., Phillips, A. S., Neale, R. B., Simpson, I. R., DuVivier, A. K., Hodzic, A., and Randel, W. J.: The Whole Atmosphere Community Climate Model version 6 (WACCM6), J. Geophys. Res., 124, 12,380–12,403, https://doi.org/10.1029/2019JD030943, 2019 (data available at: https://acomstaff.acom.ucar.edu/dkin/ACP_Froidevaux_2021/).
Hegglin, M. I., Tegtmeier, S., Anderson, J., Bourassa, A. E., Brohede, S., Degenstein, D., Froidevaux, L., Funke, B., Gille, J., Kasai, Y., Kyrölä, E. T., Lumpe, J., Murtagh, D., Neu, J. L., Pérot, K., Remsberg, E. E., Rozanov, A., Toohey, M., Urban, J., von Clarmann, T., Walker, K. A., Wang, H.-J., Arosio, C., Damadeo, R., Fuller, R. A., Lingenfelser, G., McLinden, C., Pendlebury, D., Roth, C., Ryan, N. J., Sioris, C., Smith, L., and Weigel, K.: Overview and update of the SPARC Data Initiative: comparison of stratospheric composition measurements from satellite limb sounders, Earth Syst. Sci. Data, 13, 1855–1903, https://doi.org/10.5194/essd-13-1855-2021, 2021.
Hickson, K. M., Keyser, L. F., and Sander, S. P.: Temperature Dependence of the HO2 + ClO Reaction, 2. Reaction Kinetics Using the Discharge-Flow Resonance-Fluorescence Technique, J. Phys. Chem. A, 111, 8126–8138, 2007.
Jackman, C. H., Marsh, D. R., Vitt, F. M., Garcia, R. R., Fleming, E. L., Labow, G. J., Randall, C. E., López-Puertas, M., Funke, B., von Clarmann, T., and Stiller, G. P.: Short- and medium-term atmospheric constituent effects of very large solar proton events, Atmos. Chem. Phys., 8, 765–785, https://doi.org/10.5194/acp-8-765-2008, 2008.
Jones, A., Urban, J., Murtagh, D. P., Sanchez, C., Walker, K. A., Livesey, N. J., Froidevaux, L., and Santee, M. L.: Analysis of HCl and ClO time series in the upper stratosphere using satellite data sets, Atmos. Chem. Phys., 11, 5321–5333, https://doi.org/10.5194/acp-11-5321-2011, 2011.
Khosravi, M., Baron, P., Urban, J., Froidevaux, L., Jonsson, A. I., Kasai, Y., Kuribayashi, K., Mitsuda, C., Murtagh, D. P., Sagawa, H., Santee, M. L., Sato, T. O., Shiotani, M., Suzuki, M., von Clarmann, T.,Walker, K. A., and Wang, S.: Diurnal variation of stratospheric and lower mesospheric HOCl, ClO and HO2 at the equator: comparison of 1-D model calculations with measurements by satellite instruments, Atmos. Chem. Phys., 13, 7587–7606, https://doi.org/10.5194/acp-13-7587-2013, 2013.
Kinnison, D. E., Brasseur, G. P., Walters, S., Garcia, R. R., Sassi, F., Boville, B. A., Marsh, D. Harvey, L., Randall, C., Randel, W., Lamarque, J. F., Emmons, L. K., Hess, Orlando, J., Tyndall, G., and Pan, L.: Sensitivity of chemical tracers to meteorological parameters in the MOZART-3 chemical transport model, J. Geophys. Res., 112, D20302, https://doi.org/10.1029/2006JD007879, 2007.
Knight, G. P., Beiderhase, T., Helleis, F., Moortgat, G. K., and Crowley, J. N.: Reaction of HO2 with ClO: Flow Tube Studies of Kinetics and Product Formation between 215 and 298 K, J. Phys. Chem. A, 104, 1674–1685, 2000.
Kohlhepp, R., Barthlott, S., Blumenstock, T., Hase, F., Kaiser, I., Raffalski, U., and Ruhnke, R.: Trends of HCl, ClONO2, and HF column abundances from ground-based FTIR measurements in Kiruna (Sweden) in comparison with KASIMA model calculations, Atmos. Chem. Phys., 11, 4669–4677, https://doi.org/10.5194/acp-11-4669-2011, 2011.
Kovalenko, L. J., Jucks, K. W., Salawitch, R. J., Toon, G. C., Blavier, J. F., Johnson, D. G., Kleinböhl, A., Livesey, N. J., Margitan, J. J., Pickett, H. M., Santee, M. L., Sen, B., Stachnik, R. A., and Waters, J. W.: Observed and modeled HOCl profiles in the midlatitude stratosphere, Implication for ozone loss, Geophys. Res. Lett., 34, L19801, https://doi.org/10.1029/2007GL031100, 2007.
Kunz, A., Pan, L., Konopka, P., Kinnison, D., and Tilmes, S.: Chemical and dynamical discontinuity at the extratropical tropopause based on START08 and WACCM analysis, J. Geophys. Res., 116, D24302, https://doi.org/10.1029/2011JD016686, 2011.
Kuribayashi, K., Sagawa, H., Lehmann, R., Sato, T. O., and Kasai, Y.: Direct estimation of the rate constant of the reaction ClO + HO2 → HOCl + O2 from SMILES atmospheric observations, Atmos. Chem. Phys., 14, 255–266, https://doi.org/10.5194/acp-14-255-2014, 2014.
Lamarque, J.-F., Emmons, L. K., Hess, P. G., Kinnison, D. E., Tilmes, S., Vitt, F., Heald, C. L., Holland, E. A., Lauritzen, P. H., Neu, J., Orlando, J. J., Rasch, P. J., and Tyndall, G. K.: CAM-chem: description and evaluation of interactive atmospheric chemistry in the Community Earth System Model, Geosci. Model Dev., 5, 369–411, https://doi.org/10.5194/gmd-5-369-2012, 2012.
Lin, S.-J.: A “vertically-Lagrangian” finite-volume dynamical core for global atmospheric models, Mon. Weather Rev., 132, 2293– 2307, 2004.
Livesey, N. J. and Read, W. G.: Direct retrieval of line-of-sight atmospheric structure from limb sounding observations, Geophys. Res. Lett., 27, 891–894, https://doi.org/10.1029/1999GL010964, 2000.
Livesey, N. J., Van Snyder, W., Read, W. G., and Wagner, P. A.: Retrieval algorithms for the EOS Microwave Limb Sounder (MLS), IEEE Trans. Geosci. Remote Sens., 44, 1144–1155, https://doi.org/10.1109/TGRS.2006.872327, 2006.
Livesey, N. J., Read, W. G., Wagner, P. A., Froidevaux, L., Santee, M. L., Schwartz, M. J., Lambert, A., Manney, G. L., Valle, L. F. M., Pumphrey, H. C., Fuller, R. A., Jarnot, R. F., Knosp, B. W., and Lay, R. R.: EOS MLS Version 5.0× Level 2 and 3 data quality and description document, Tech. rep., Jet Propulsion Laboratory D-105336 Rev. B [data set], http://disc.sci.gsfc.nasa.gov/Aura/data-holdings/MLS (last access: 10 January 2022), 2020.
Livesey, N. J., Read, W. G., Froidevaux, L., Lambert, A., Santee, M. L., Schwartz, M. J., Millán, L. F., Jarnot, R. F., Wagner, P. A., Hurst, D. F., Walker, K. A., Sheese, P. E., and Nedoluha, G. E.: Investigation and amelioration of long-term instrumental drifts in water vapor and nitrous oxide measurements from the Aura Microwave Limb Sounder (MLS) and their implications for studies of variability and trends, Atmos. Chem. Phys., 21, 15409–15430, https://doi.org/10.5194/acp-21-15409-2021, 2021.
Mahieu, E., Chipperfield, M. P., Notholt, J., Reddmann, T., Anderson, J., Bernath, P. F., Blumenstock, T., Coffey, M. T., Dhomse, S. S., Feng, W., Franco, B., Froidevaux, L., Griffith, D. W. T., Hannigan, J. W., Hase, F., Hossaini, R., Jones, N. B., Morino, I., Murata, I., Nakajima, H., Palm, M., Paton-Walsh, C., Russell III, J. M., Schneider, M., Servais, C., Smale, D., and Walker, K. A.: Recent Northern Hemisphere stratospheric HCl increase due to atmospheric circulation changes, Nature, 515, 104–107, https://doi.org/10.1038/nature13857, 2014.
Meinshausen, M., Vogel, E., Nauels, A., Lorbacher, K., Meinshausen, N., Etheridge, D. M., Fraser, P. J., Montzka, S. A., Rayner, P. J., Trudinger, C. M., Krummel, P. B., Beyerle, U., Canadell, J. G., Daniel, J. S., Enting, I. G., Law, R. M., Lunder, C. R., O'Doherty, S., Prinn, R. G., Reimann, S., Rubino, M., Velders, G. J. M., Vollmer, M. K., Wang, R. H. J., and Weiss, R.: Historical greenhouse gas concentrations for climate modelling (CMIP6), Geosci. Model Dev., 10, 2057–2116, https://doi.org/10.5194/gmd-10-2057-2017, 2017.
Meinshausen, M., Nicholls, Z. R. J., Lewis, J., Gidden, M. J., Vogel, E., Freund, M., Beyerle, U., Gessner, C., Nauels, A., Bauer, N., Canadell, J. G., Daniel, J. S., John, A., Krummel, P. B., Luderer, G., Meinshausen, N., Montzka, S. A., Rayner, P. J., Reimann, S., Smith, S. J., van den Berg, M., Velders, G. J. M., Vollmer, M. K., and Wang, R. H. J.: The shared socio-economic pathway (SSP) greenhouse gas concentrations and their extensions to 2500, Geosci. Model Dev., 13, 3571–3605, https://doi.org/10.5194/gmd-13-3571-2020, 2020.
Millán, L., Livesey, N., Read, W., Froidevaux, L., Kinnison, D., Harwood, R., MacKenzie, I. A., and Chipperfield, M. P.: New Aura Microwave Limb Sounder observations of BrO and implications for Bry, Atmos. Meas. Tech., 5, 1741–1751, https://doi.org/10.5194/amt-5-1741-2012, 2012.
Millán, L. F., Wang, S., Livesey, N., Kinnison, D., Sagawa, H., and Kasai, Y.: Stratospheric and mesospheric HO2 observations from the Aura Microwave Limb Sounder, Atmos. Chem. Phys., 15, 2889–2902, https://doi.org/10.5194/acp-15-2889-2015, 2015.
Millán, L. F., Livesey, N. J., Santee, M. L., Neu, J. L., Manney, G. L., and Fuller, R. A.: Case studies of the impact of orbital sampling on stratospheric trend detection and derivation of tropical vertical velocities: solar occultation vs. limb emission sounding, Atmos. Chem. Phys., 16, 11521–11534, https://doi.org/10.5194/acp-16-11521-2016, 2016.
Molina, M. J., and Rowland, F. S.: Stratospheric sink for chlorofluoro-methanes: Chlorine atom catalysed destruction of ozone, Nature, 249, 810–812, 1974.
Montzka, S. A., Dutton, G. S., Yu, P., Ray, E., Portmann, R. W., Daniel, J. S., Kuijpers, L., Hall, B. D., Mondeel, D., Siso, C., Nance, J. D., Rigby, M., Manning, A. J., Hu, L., Moore, F., Miller, B. R., and Elkins, J. W. : An unexpected and persistent increase in global emissions of ozone-depleting CFC-11, Nature, 557, 413–417, https://doi.org/10.1038/s41586-018-0106-2, 2018.
Nassar, R., Bernath, P. F., Boone, C. D., Clerbaux, C., Coheur, P. F., Dufour, G., Froidevaux, L., Mahieu, E., McConnell, J. C., McLeod, S. D., Murtagh, D. P., Rinsland, C. P., Semeniuk, K., Skelton, R., Walker, K. A., and Zander, R.: A global inventory of stratospheric chlorine in 2004, J. Geophys. Res., 111, D22312, https://doi.org/10.1029/ 2006JD007073, 2006.
Neely, R. R. and Schmidt, A.: “VolcanEESM: Global volcanic sulphur dioxide (SO2) emissions database from 1850 to present – Version 1.0.”, Centre for Environmental Data Analysis, CEDA Archive [data set], https://doi.org/10.5285/76ebdc0b-0eed-4f70-b89e-55e606bcd568, 2016.
Nedoluha, G., Connor, B. J., Mooney, T., Barrett, J. W., Parrish, A., Gomez, R. M., Boyd, I., Allen, D. R., Kotkamp, M., Kremser, S., Deshler, T., Newman, P., and Santee, M. L.: 20 years of ClO measurements in the Antarctic lower stratosphere, Atmos. Chem. Phys., 16, 10725–10734, https://doi.org/10.5194/acp-16-10725-2016, 2016.
Nickolaisen, S. L., Roehl, C. M., Blakeley, L. K., Friedl, R. R., Francisco, J. S., Liu, R., and Sander, S. P.: Temperature Dependence of the HO2 + ClO Reaction, 1. Reaction Kinetics by Pulsed Photolysis-Ultraviolet Absorption and ab Initio Studies of the Potential Surface, J. Phys. Chem. A, 104, 308–319, 2000.
O'Doherty, S., Cunnold, D. M., Manning, A., Miller, B. R., Wang, R. H.-J., Krummel, P. B., Fraser, P. J., Simmonds, P. G., McCulloch, A., Weiss, R. F., Salameh, P., Porter, L. W., Prinn, R. G., Huang, J., Sturrock, G., Ryall, D., Derwent, R. G., and Montzka, S. A.: Rapid growth of hydrofluorocarbon 134a and hydrochlorofluorocarbons 141b, 142b, and 22 from Advanced Global Atmospheric Gases Experiment (AGAGE) observations at Cape Grim, Tasmania, and Mace Head, Ireland, J. Geophys. Res., 109, D06310, https://doi.org/10.1029/2003JD004277, 2004.
O'Neill, B. C., Tebaldi, C., van Vuuren, D. P., Eyring, V., Friedlingstein, P., Hurtt, G., Knutti, R., Kriegler, E., Lamarque, J.-F., Lowe, J., Meehl, G. A., Moss, R., Riahi, K., and Sanderson, B. M.: The Scenario Model Intercomparison Project (ScenarioMIP) for CMIP6, Geosci. Model Dev., 9, 3461–3482, https://doi.org/10.5194/gmd-9-3461-2016, 2016.
Randel, W. J. and Thompson, A. M.: Interannual variability and trends in tropical ozone derived from SAGE II satellite data and SHADOZ ozonesondes, J. Geophys. Res., 116, D07303, https://doi.org/10.1029/2010JD015195, 2011.
Read, W. G., Shippony, Z., and Snyder, W. V.: The clear-sky unpolarized forward model for the EOS Aura microwave limb sounder (MLS), IEEE Trans. Geosci. Remote Sens., 44, 1367–1379, https://doi.org/10.1109/TGRS.2006.873233, 2006.
Riahi, K., van Vuuren, D. P., Kriegler, E., Edmonds, J., O'Neill, B. C., Fujimori, S., Bauer, N., Calvin, K., Dellink, R., Fricko, O., Lutz, W., Popp, A., Crespo Cuaresma, J., Samir, K. C., Leimbach, M., Jiang, L., Kram, T., Rao, S., Emmerling, J., Ebi, K., Hasegawa, T., Havlik, P., Humpenöder, F., Aleluia Da Silva, L., Smith, S., Stehfest, E., Bosetti, V., Eom, J., Gernaat, D., Masui, T., Rogelj, J., Strefler, J., Drouet, L., Krey, V., Luderer, G., Harmsen, M., Takahashi, K., Baumstark, L., Doelman, J. C., Kainuma, M., Klimont, Z., Marangoni, G., Lotze-Campen, H., Obersteiner, M., Tabeau, A., and Tavoni, M.: The Shared Socioeconomic Pathways and their energy, land use, and greenhouse gas emissions implications: An overview, Glob. Environ. Change, 42, 1045 153–168, https://doi.org/10.1016/j.gloenvcha.2016.05.009, 2017.
Rinsland, C. P., Mahieu, E., Zander, R., Jones, N. B., Chipperfield, M. P., Goldman, A., Anderson, J., Russell III, J. M., Demoulin, P., Notholt, J., Toon, G. C., Blavier, J.-F., Sen, B., Sussmann, R., Wood, S. W., Meier, A., Griffith, D. W. T., Chiou, L. S., Murcray, F. J., Stephen, T. M., Hase, F., Mikuteit, S., Schulz, A., and Blumenstock, T.: Long-term trends of inorganic chlorine from ground-based infrared solar spectra: Past increases and evidence for stabilization, J. Geophys. Res. 108, D8, 4252, https://doi.org/10.1029/2002JD003001, 2003
Rodgers, C.: Inverse Methods for Atmospheric Sounding: Theory and Practice, Vol. 2 of Series on Atmospheric, Oceanic and Planetary Physics, World Scientific Publishing Co, Singapore, pp. 256, ISBN: 978-981-02-2741-1, https://doi.org/10.1142/3171, 2000.
Sander, S. P., Finlayson-Pitts, B. J., Friedl, R. R., Golden, D. M., Huie, R. E., Keller-Rudek, H., Kolb, C. E., Kurylo, M. J., Molina, M. J., Moortgat, G. K., Orkin, V. L., Ravishankara, A. R., and Wine, P. H.: Chemical Kinetics and Photochemical Data for Use in Atmospheric Studies, Evaluation Number 15, JPL Publication 06-2, Jet Propulsion Laboratory, California Institute of Technology, Pasadena, JPL Publication, https://jpldataeval.jpl.nasa.gov/ (last access: 1 March 2021), 2006.
Sheese, P. and Walker, K.: Data Quality Flags for ACE-FTS Level 2 Version 4.1/4.2 Data Set, V10, Scholars Portal Dataverse [data set], https://doi.org/10.5683/SP2/BC4ATC, 2020.
Sheese, P. E., Boone, C. D., and Walker, K. A.: Detecting physically unrealistic outliers in ACE-FTS atmospheric measurements, Atmos. Meas. Tech., 8, 741–750, https://doi.org/10.5194/amt-8-741-2015, 2015.
Siskind, D. E., Froidevaux, L., Russell, J. M., and Lean, J.: Implications of upper stratospheric trace constituent changes observed by HALOE for O3 and ClO from 1992 to 1995, Geophys. Res. Lett., 25, 18, 3513–3516, https://doi.org/10.1029/98GL02664, 1998.
Solomon, P., Barrett, J., Mooney, T., Connor, B., Parrish, A., and Siskind, D. E.: Rise and decline of active chlorine in the stratosphere, Geophys. Res. Lett., 33, L18807, https://doi.org/10.1029/2006GL027029, 2006.
Solomon, S. and Garcia, R. R.: On the distribution of long-lived tracers and chlorine species in the middle atmosphere, J. Geophys. Res., 89, 11633–11644, 1984.
SPARC: The SPARC Data Initiative: Assessment of stratospheric trace gas and aerosol climatologies from satellite limb sounders, edited by: Hegglin, M. I. and Tegtmeier, S., SPARC Report No. 8, WCRP-5/2017, available at https://www.sparc-climate.org/publications/sparc-reports/ (last access: 1 March 2021), 2017.
Steiner, A. K., Ladstädter, F., Randel, W. J., Maycock, A. C., Fu, Q., Claud, C., Gleisner, H., Haimberger, L., Ho, S.-P., Keckhut, P., Leblanc, T., Mears, C., Polvani, L. M., Santer, B. D., Schmidt, T., Sofieva, V., Wing, R., and Zou, C.-Z.: Observed Temperature Changes in the Troposphere and Stratosphere from 1979 to 2018, J. Climate, 33, 8165–8194, https://doi.org/10.1175/JCLI-D-19-0998.1, 2021.
Stimpfle, R., Perry, R., and Howard, C. J.: Temperature dependence of the reaction of ClO and HO2 radicals, J. Chem. Phys., 71, 5183–5190, https://doi.org/10.1063/1.438293, 1979.
Strahan, S. E., Smale, D., Douglass, A.R., Blumenstock, T., Hannigan, J. W., Hase, F., Jones, N. B., Mahieu, E., Notholt, J., Oman, L. D., Ortega, I., Palm, M., Prignon, M., Robinson, J., Schneider, M., Sussmann, R., and Velazco, V. A.: Observed hemispheric asymmetry in stratospheric transport trends from 1994 to 2018, Geophys. Res. Lett., 47, e2020GL088567, https://doi.org/10.1029/2020GL088567, 2020.
Tapping, K. F.: The 10.7 cm solar radio flux (F10.7), Space Weather, 11, 394–406, https://doi.org/10.1002/swe.20064, 2013 (data available at: ftp://ftp.seismo.nrcan.gc.ca/spaceweather/solar_flux/monthly_averages/solflux_monthly_average.txt, last access: 1 June 2021).
Tiao, G. C, Reinsel, G. C., Xu, D., Pedrick, J. H., Zhu, X., Miller, A. J., DeLuisi, J. J., Mateer, C. L., and Wuebbles, D. J.: Effects of autocorrelation and temporal sampling schemes on estimates of trend and spatial correlation, J. Geophys. Res., 95, 20507–20517, https://doi.org/10.1029/JD095iD12p20507, 1990.
Tilmes, S., Hodzic, A., Emmons, L. K., Mills, M. J., Gettelman, A., Kinnison, D. E., Park, M., Lamarque, J.-F., Vitt, F., Shrivastava, M., Campuzano-Jost, P., Jimenez, J. L., and Liu, X.: Climate forcing and trends of organic aerosols in the Community Earth System Model (CESM2), J. Adv. Model. Earth Syst., 11, 4323–4351, https://doi.org/10.1029/2019MS001827, 2019.
von Clarmann, T., Glatthor, N., Grabowski, U., Höpfner, M., Kellmann, S., Linden, A., Mengistu Tsidu, G., Milz, M., Steck, T., Stiller, G. P., Fischer, H., and Funke, B.: Global stratospheric HOCl distributions retrieved from infrared limb emission spectra recorded by the Michelson Interferometer for Passive Atmospheric Sounding (MIPAS), J. Geophys. Res., 111, D05311, https://doi.org/10.1029/2005JD005939, 2006.
von Clarmann, T., Glatthor, N., Ruhnke, R., Stiller, G. P., Kirner, O., Reddmann, T., Höpfner, M., Kellmann, S., Kouker, W., Linden, A., and Funke, B.: HOCl chemistry in the Antarctic Stratospheric Vortex 2002, as observed with the Michelson Interferometer for Passive Atmospheric Sounding (MIPAS), Atmos. Chem. Phys., 9, 1817–1829, https://doi.org/10.5194/acp-9-1817-2009, 2009.
von Clarmann, T., Funke, B., Glatthor, N., Kellmann, S., Kiefer, M., Kirner, O., Sinnhuber, B.-M., and Stiller, G. P.: The MIPAS HOCl climatology, Atmos. Chem. Phys., 12, 1965–1977, https://doi.org/10.5194/acp-12-1965-2012, 2012.
Ward, M. K. M. and Rowley, D. M.: Kinetics of the ClO + CH3O2 reaction over the temperature range T=250–298 K, Phys. Chem. Chem. Phys., 18, 13646–13656, https://doi.org/10.1039/c6cp00724d, 2016.
Waters, J. W., Hardy, J. C., Jarnot, R. F., and H. M. Pickett, H. M.: Chlorine monoxide radical, ozone, and hydrogen peroxide: Stratospheric measurements by microwave limb sounding, Science, 214, 61–64, https://doi.org/10.1126/science.214.4516.61, 1981.
Waters, J., Froidevaux, L., Harwood, R., Jarnot, R., Pickett, H., Read, W., Siegel, P., Cofield, R., Filipiak, M., Flower, D., Holden, J., Lau, G., Livesey, N., Manney, G., Pumphrey, H., Santee, M., Wu, D., Cuddy, D., Lay, R., Loo, M., Perun, V., Schwartz, M., Stek, P., Thurstans, R., Boyles, M., Chandra, S., Chavez, M., Chen, G.-S., Chudasama, B., Dodge, R., Fuller, R., Girard, M., Jiang, J., Jiang, Y., Knosp, B., LaBelle, R., Lam, J., Lee, K., Miller, D., Oswald, J., Patel, N., Pukala, D., Quintero, O., Scaff, D., Snyder, V., Tope, M., Wagner, P., and Walch, M.: The Earth Observing System Microwave Limb Sounder (EOS MLS) on the Aura satellite, IEEE Trans. Geosci. Remote Sens., 44, 5, 1075–1092, https://doi.org/10.1109/TGRS.2006.873771, 2006.
Waugh, D. W., Considine, D. B., and Fleming, E. L.: Is upper stratospheric chlorine decreasing as expected?, Geophys. Res. Lett., 28, 1187–1190, https://doi.org/10.1029/2000GL011745, 2001.
Weatherhead, E. C., Reinsel, G. C., Tiao, G. C., Meng, X. L., Choi, D., Cheang, W. K., Keller, T., DeLuisi, J., Wuebbles, D. J., Kerr, J. B., Miller, A. J., Oltmans, S. J., and Frederick J. E.: Factors affecting the detection of trends: Statistical considerations and applications to environmental data, J. Geophys. Res., 103, 17149–17161, https://doi.org/10.1029/98JD00995, 1998.
WMO: Scientific assessment of ozone depletion: 2018, Global Ozone Research and Monitoring Project–Report No. 58, WMO, Geneva, Switzerland, 588 pp., ISBN: 978-1-7329317-1-8, https://ozone.unep.org/science/assessment/sap (last access: 1 March 2021), 2018.
Zander, R., Gunson, M. R., Farmer, C. B., Rinsland, C. P., Irion, F. W., and Mahieu, E.: The 1985 chlorine and fluorine inventories in the stratosphere based on ATMOS observations at 30∘ north latitude, J. Atmos. Chem., 15, 171–186, https://doi.org/10.1007/BF00053758, 1992.