the Creative Commons Attribution 4.0 License.
the Creative Commons Attribution 4.0 License.
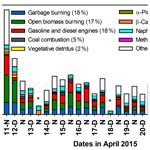
Ambient air quality in the Kathmandu Valley, Nepal, during the pre-monsoon: concentrations and sources of particulate matter and trace gases
Md. Robiul Islam
Thilina Jayarathne
Isobel J. Simpson
Benjamin Werden
John Maben
Ashley Gilbert
Puppala S. Praveen
Sagar Adhikari
Arnico K. Panday
Maheswar Rupakheti
Donald R. Blake
Robert J. Yokelson
Peter F. DeCarlo
William C. Keene
Elizabeth A. Stone
The Kathmandu Valley in Nepal is a bowl-shaped urban basin that experiences severe air pollution that poses health risks to its 3.5 million inhabitants. As part of the Nepal Ambient Monitoring and Source Testing Experiment (NAMaSTE), ambient air quality in the Kathmandu Valley was investigated from 11 to 24 April 2015, during the pre-monsoon season. Ambient concentrations of fine and coarse particulate matter (PM2.5 and PM10, respectively), online PM1, inorganic trace gases (NH3, HNO3, SO2, and HCl), and carbon-containing gases (CO2, CO, CH4, and 93 non-methane volatile organic compounds; NMVOCs) were quantified at a semi-urban location near the center of the valley. Concentrations and ratios of NMVOC indicated origins primarily from poorly maintained vehicle emissions, biomass burning, and solvent/gasoline evaporation. During those 2 weeks, daily average PM2.5 concentrations ranged from 30 to 207 µg m−3, which exceeded the World Health Organization 24 h guideline by factors of 1.2 to 8.3. On average, the non-water mass of PM2.5 was composed of organic matter (48 %), elemental carbon (13 %), sulfate (16 %), nitrate (4 %), ammonium (9 %), chloride (2 %), calcium (1 %), magnesium (0.05 %), and potassium (1 %). Large diurnal variability in temperature and relative humidity drove corresponding variability in aerosol liquid water content, the gas–aerosol phase partitioning of NH3, HNO3, and HCl, and aerosol solution pH. The observed levels of gas-phase halogens suggest that multiphase halogen-radical chemistry involving both Cl and Br impacted regional air quality. To gain insight into the origins of organic carbon (OC), molecular markers for primary and secondary sources were quantified. Levoglucosan (averaging 1230±1154 ng m−3), 1,3,5-triphenylbenzene (0.8±0.6 ng m−3), cholesterol (2.9±6.6 ng m−3), stigmastanol (1.0 ±0.8 ng m−3), and cis-pinonic acid (4.5±1.9 ng m−3) indicate contributions from biomass burning, garbage burning, food cooking, cow dung burning, and monoterpene secondary organic aerosol, respectively. Drawing on source profiles developed in NAMaSTE, chemical mass balance (CMB) source apportionment modeling was used to estimate contributions to OC from major primary sources including garbage burning (18±5 %), biomass burning (17±10 %) inclusive of open burning and biomass-fueled cooking stoves, and internal-combustion (gasoline and diesel) engines (18±9 %). Model sensitivity tests with newly developed source profiles indicated contributions from biomass burning within a factor of 2 of previous estimates but greater contributions from garbage burning (up to three times), indicating large potential impacts of garbage burning on regional air quality and the need for further evaluation of this source. Contributions of secondary organic carbon (SOC) to PM2.5 OC included those originating from anthropogenic precursors such as naphthalene (10±4 %) and methylnaphthalene (0.3±0.1 %) and biogenic precursors for monoterpenes (0.13±0.07 %) and sesquiterpenes (5±2 %). An average of 25 % of the PM2.5 OC was unapportioned, indicating the presence of additional sources (e.g., evaporative and/or industrial emissions such as brick kilns, food cooking, and other types of SOC) and/or underestimation of the contributions from the identified source types. The source apportionment results indicate that anthropogenic combustion sources (including biomass burning, garbage burning, and fossil fuel combustion) were the greatest contributors to PM2.5 and, as such, should be considered primary targets for controlling ambient PM pollution.
- Article
(2691 KB) - Full-text XML
-
Supplement
(7114 KB) - BibTeX
- EndNote
According to the World Health Organization (WHO, 2016), 4.2 million (or 7.6 % of all) premature deaths globally during 2016 were linked to ambient air pollution. The majority of these premature deaths occurred in low- to middle-income countries in the South Asia, East Asia, and western Pacific regions. The Kathmandu Valley in Nepal is home to more than 3.5 million residents who suffer from high levels of air pollutants, including particulate matter (PM), ozone (O3), carbon monoxide (CO), and volatile organic compounds (VOCs) (Bhardwaj et al., 2018; Kiros et al., 2016; Mahata et al., 2018; Putero et al., 2015; Sarkar et al., 2016; Wan et al., 2019) that are expected to have severe health impacts (Gurung and Bell, 2013).
Effective mitigation of air pollution requires understanding the major contributing sources. PM emissions contain molecular and elemental fingerprints that reflect the material from which the PM was generated and the process(es) by which it formed. For organic aerosol sources, these chemical fingerprints include molecular markers that are defined as chemical species unique to a PM source category (Schauer et al., 1996). Well-established molecular markers for primary (direct emissions) and secondary (produced in the atmosphere from reactive precursors) sources are summarized in Table 1. These species can be used to identify sources of PM in ambient air both directly and through source apportionment modeling.
The Nepal Ambient Monitoring and Source Testing Experiment (NAMaSTE) was initiated in 2015 to characterize widespread and under-sampled combustion sources in Nepal. Source characterization measurements included trace gases (Stockwell et al., 2016) and particulate matter (Goetz et al., 2018; Jayarathne et al., 2018), as well as optical properties of aerosols (Stockwell et al., 2016). The characterized sources included brick kilns, garbage burning, power generators, diesel groundwater pumps, idling motorcycles, cooking stoves, crop residue burning, and open burning of biofuels. As part of NAMaSTE, a regional monitoring station was also installed to probe the relative contribution of these sources to ambient air quality. In addition, new emissions data are being incorporated into regional air quality models for the region (Zhong et al., 2019).
High daily average concentrations of PM2.5 (up to 160 µg m−3) (Shakya et al., 2017a) and PM10 (up to 579 µg m−3) have been documented in the Kathmandu Valley (Giri et al., 2006). A satellite-derived aerosol optical depth study indicates substantial increases in particulate loading in the Kathmandu Valley and nearby background sites over the past 15 years (Mahapatra et al., 2019). Measured components of regional PM have included black carbon (BC; 17 %), sulfate (17 %), and ammonium (11 %) in PM2.5 (Shakya et al., 2017a) and organic carbon (OC; 23 %) and nitrate (2.5 %) in PM10 (Kim et al., 2015). Carbon isotope analysis of bulk aerosol sampled during the winter of 2007–2008 in the Kathmandu Valley suggested that a major fraction of particulate OC originated from primary sources (69 %), particularly local fossil fuel emissions (39 %) (Shakya et al., 2010). A recent carbon isotope study observed that fossil fuel contributed 67 % of the black carbon during April 2013 in the Kathmandu Valley (Li et al., 2016).
An earlier chemical mass balance (CMB) source apportionment study at Godawari, at the southeast edge of the Kathmandu Valley identified sources of PM2.5 OC as biomass burning (21 %), fossil fuel combustion (7 %), and secondary organic aerosol (SOA) from biogenic precursors (3 %) (Stone et al., 2010). However, the relative contributions of biomass and fossil fuel to elemental carbon (EC) were highly uncertain due to large variability in EC emissions with respect to combustion efficiency and air-to-fuel ratios. A significant fraction of PM2.5 OC (54 %–84 %) in that study was unapportioned suggesting significant contributions from other primary and secondary sources. Assessments of PM sources in this region were challenging due in part to poorly characterized emissions from brick kilns, garbage burning, and local industries (Stone et al., 2010). Anthropogenic SOA was also identified as a likely source of PM2.5 that was not previously apportioned (Stone et al., 2012).
The primary goal of the study reported herein is to characterize the composition of ambient gases and PM in the Kathmandu Valley, Nepal, and apportion major sources based on new knowledge of source-specific emissions within the region. Our specific objectives are to (1) quantify atmospheric loadings of volatile carbon-containing compounds (CO2, CO, CH4, and 93 non-methane volatile organic compounds; NMVOCs), inorganic trace gases (NH3, SO2, HNO3, HCl, total volatile inorganic Br), and PM mass in the Kathmandu Valley during the pre-monsoon season; (2) chemically characterize the major carbonaceous and ionic constituents of PM; and (3) apportion organic carbon (OC) to its sources using CMB modeling with region-specific source profiles when available. This work is designed to contribute to advancing the understanding of the role of combustion and other major pollution sources in South Asia and their effects on air quality.
2.1 Site description
Ambient air was sampled at Bode (27.689∘ N, 85.395∘ E; 1345 m a.s.l.), which is a semi-urban location close to the geographic center of the Kathmandu Valley (Fig. S1 in the Supplement). Bode was the measurement supersite during the Sustainable Atmosphere for the Kathmandu Valley-Atmospheric Brown Clouds (SusKat-ABC) international air pollution measurement campaign (Mahata et al., 2017, 2018; Sarkar et al., 2016). Bode is located in Madhyapur-Thimi Municipality and three major cities are located nearby: Kathmandu Metropolitan City to the west, Lalitpur Metropolitan City to the southwest, and Bhaktapur Municipality to the southeast. The Bode supersite was located in a newly developing suburban area that started with a grid of streets placed across what were agricultural fields, with a gradual filling in of houses on individual plots, while a lot of fields and empty plots still remain. Nearby the Bode site are agricultural fields, the Bhaktapur Industrial Estate with several small pharmaceutical, plastic, and metal industries, and about 19 brick kilns located within 5 km to the east and southeast of the site. Meteorological conditions (temperature, relative humidity (RH), barometric pressure, global radiation and precipitation) were measured at Bode for this study with data averaged every 5 min. From 23 to 26 April, on-site meteorological measurements were not available so meteorological conditions recorded at the Tribhuvan International Airport in Kathmandu, ca. 4 km to the west of Bode, were used instead.
2.2 PM and reactive trace gas sample collection
A medium volume sampler (URG-3000 ABC) was placed on the rooftop of a five-story building at Bode, approximately 15 m (50 ft) above the ground. PM samples were collected from 11 to 24 April 2015, during daytime (08:00 to 17:30) and nighttime (18:00 to 07:30) intervals. PM2.5 was sampled downstream of two 2.5 µm sharp-cut cyclones and PM10 was sampled downstream of a 10 µm sharp-cut impaction plate. Both air streams were split to collect four discrete PM samples in each size bin (total of eight samples per time interval), at nominal flow rates of 7.4 L min−1 each. The flow rate through each channel was measured before and after the sample collection with a calibrated rotameter (Gilmont Inst., Barrington, IL). PM in each size range was sampled on three 47 mm quartz fiber filters (QFFs; Tissuquartz, Pall Life Sciences, East Hills, New York) and one 47 mm Teflon filter (Teflo Membrane, 2.0 µm pore size, Pall Life Sciences). QFFs were pre-cleaned by baking at 550 ∘C for 18 h to remove organic species (Stone et al., 2007). Following collection, exposed PM samples were transferred to polystyrene petri dishes lined with pre-cleaned aluminum foil, capped, sealed with Teflon tape, stored frozen in sealed polyethylene bags, and shipped to the University of Iowa for analysis.
Soluble reactive trace gases were sampled downstream of two of the PM2.5 QFFs during daytime and nighttime periods using a filter pack technique (Bardwell et al., 1990; Keene et al., 2009; Pszenny et al., 2004). Total volatile inorganic NO3 and Cl (dominated by and hereafter referred to as HNO3 and HCl, respectively), SO2, and total volatile inorganic Br− (Brt) were sampled using a three-stage, 47 mm, Teflon filter pack housing configured with a QFF for PM collection (as described above) followed by tandem rayon filters (Schleicher and Schuell, 8S) impregnated with a solution of 10 % potassium carbonate (K2CO3) and 10 % glycerol. NH3 was sampled in parallel using an otherwise identical filter pack configured with tandem rayon filters impregnated with a solution of 10 % citric acid (C6H8O7) and 10 % glycerol.
In total, 27 sets of ambient PM and reactive gas samples were collected. Field blanks were prepared every fifth sampling period by loading, mounting, recovering, unloading, and processing filter housings using otherwise identical procedures as those for samples but without pulling air through them. All filter housings and samples were loaded and unloaded using clean-handling procedures. Impregnated filters were stored in polystyrene petri dishes that were capped, sealed with Teflon tape, stored frozen in polyethylene bags, and shipped to the University of Virginia for analysis.
Submicron PM (PM1) was characterized in parallel for non-refractory constituents using a high-resolution aerosol mass spectrometer (HR-AMS) (DeCarlo et al., 2006). The inlet for the AMS was located within 5 m of the URG sampler. Samples were size selected through a PM2.5 cyclone, and dried to below 30 % RH by a counterflow Nafion dryer. The AMS measures mass and composition of non-refractory PM1 at 1 min time resolution. Calibrations were undertaken for alignment, mass (ionization efficiency), and particle sizing. Frequent, intermittent power outages at Bode interrupted AMS operations necessitating long restart times and significant losses of sampling. Due to associated data losses, only 10 of the filter samples aligned with concurrent AMS data across the entire periods: 16 April nighttime to 17 April daytime, 18 April daytime to 21 April daytime, and 22 April daytime. An in-depth analysis of the HR-AMS data will be the subject of a forthcoming paper, but general observations will be used here to provide a higher time resolution context for the filter measurements (see Sect. 3.2.1 for a discussion of PM mass and Sect. 3.2.3 for sulfate concentrations).
2.3 Whole-air samples
Whole-air samples (WASs) were collected from 16 to 24 April 2015 before 08:25 or after 18:00 and analyzed for CO2, CO, CH4, and 93 NMVOCs using multi-column gas chromatography (Simpson et al., 2011; Stockwell et al., 2016). The WAS analytical details, including calibration procedures, are described in detail in Simpson et al. (2011). While the WAS sampling was cut short by the Gorkha earthquake on 25 April 2015 and only nine samples were collected, the limited WAS sampling still provides useful context for VOC levels and sources in the area.
2.4 PM2.5 mass measurement
PM mass was measured on Teflon filters as the difference between post- and pre-sampling filter masses. Prior to mass measurements, filters were conditioned for 48 h in a temperature- (22±0.5 ∘C) and humidity- (34±12 %) controlled environment. Masses were measured in triplicate using an analytical microbalance (Mettler Toledo XP26). PM mass per filter was converted to mass concentration (µg m−3) using sampled air volume after field blank subtraction. The analytical uncertainties in the mass measurements were calculated following Jayarathne et al. (2018). The PM2.5 data for the nighttime periods of 12 and 13 April were excluded due to sampling errors and filter damage, respectively.
2.5 Organic and elemental carbon measurement
Organic carbon (OC) and elemental carbon (EC) were measured following the National Institute for Occupational Safety and Health (NIOSH) 5040 method (NIOSH, 2003) using 1.0 cm2 filter punches from sampled QFF (Sunset OC-EC Aerosol Analyzer, Sunset Laboratories, Tigard, OR) (Birch and Cary, 1996). OC data were field blank subtracted, while EC was not detected on field blanks. The uncertainties in OC and EC measurements were calculated following Jayarathne et al. (2018).
2.6 Analysis of particulate inorganic ions
Water-soluble inorganic ions in PM were extracted in 5 mL deionized water and analyzed by ion exchange chromatography (IC) with conductivity detection (Dionex-ICS 5000), with details of the analytical method, uncertainties, and detection limit calculations provided by Jayarathne et al. (2014). Unusually high concentrations of Na+, Mg2+, Ca2+, and F− were observed in the field blanks collected on 15 April and PM samples collected from 15 to 17 April, indicating likely contamination of these samples. Thus, concentrations of these ions during this time period were not reported and were excluded in the calculation of average concentrations.
2.7 Analysis of reactive trace gases
Exposed rayon filters were extracted under sonication in 5 mL of deionized water and analyzed by IC (Dionex-ICS 3000, dual channel). The anion channel was configured with Dionex guard (AG-4A 4×50 mm) and analytical (AS-4A 4×250 mm) columns and a Dionex Anion Micro Membrane Suppressor (AMMS). The cation channel was configured with Dionex guard (IonPac CG16: 5×50 mm) and analytical (IonPac CG16: 5×250 mm) columns and a Thermo Scientific Dionex Cation Electrolytically Regenerated Suppressor (CERS 500: 4 mm). Standard solutions were matrix matched with sample extracts. Analytical results for samples were blank corrected based on median concentrations of analytes measured in extracts of field blanks. Independent analyses of tandem rayon filters indicate that all analytes were sampled by the upstream filters at average efficiencies of greater than 98 %. Average detection limits estimated following Keene et al. (1989) were 0.66 ppbv for NH3, 0.065 ppbv for HNO3, 0.035 ppbv for HCl, 0.18 ppbv for SO2, and 0.014 ppbv for Brt. Estimated precisions based on replicate analyses were approximately ±5 % of measured mixing ratios or ±0.5 times estimated detection limits (DL), whichever were the greater absolute values. Due to suspected contamination of filter samples collected during 15 to 17 April 2015 (described in Sect. 2.6), results for these samples were excluded from the reported data set.
2.8 Thermodynamic calculations
Aerosol liquid water contents (LWCs), activity coefficients, and the partitioning between ionized and solid aerosol constituents were calculated using E-AIM (Extended Aerosol Inorganics Model) IV, which considers particles comprised of , Na+, , , Cl−, and H2O (Friese and Ebel, 2010) (http://www.aim.env.uea.ac.uk/aim/aim.php, last access: 21 January 2020). E-AIM requires that the input data for ionic composition be balanced on an equivalent basis (i.e., Σ cations = Σ anions). Unmeasured ionic constituents (e.g., carboxylic anions such as oxalate), ionic constituents that were measured but are not considered by E-AIM (e.g., K+, Mg2+, and Ca2+), and random analytical errors introduce minor ion imbalances into the subsets of input data. To balance an anion deficit for a given sample, the input concentrations of , , and Cl− were increased in proportion to their measured concentrations on an equivalent basis. Similarly, to balance a cation deficit, concentrations of and Na+ were increased in proportion to their measured concentrations. Because the ionic compositions of aerosol sampled were dominated by , , , and Cl−, these adjustments in measured concentrations were relatively minor (typically < 15 % for a given analyte). Sensitivity studies indicate that alternative approaches to charge balance input data for E-AIM yield similar results (e.g., Young et al., 2013).
For each sample, the input data included the measured (or adjusted as described above) concentrations of , Na+, , , and Cl− and the corresponding temperature and RH averaged over the sampling interval. Model output used for subsequent calculations included aerosol LWC; activity coefficients for , , and Cl−; and, for mixed-phase particles, the partitioning of , , and Cl− between dissolved and solid phases. E-AIM simulated three distinct regimes: (1) at RH greater than about 75 %, the aerosol was completely deliquesced (i.e., virtually all , , and Cl− were ionized); (2) at RH less than about 60 %, particles existed entirely as solids with only tightly bound water molecules and negligible LWC; (3) at RH between about 60 % and 75 %, constituents partitioned between dissolved and solid (primarily (NH4)2SO4) phases. The extraction of aerosol samples into dilute aqueous solutions prior to analysis would have dissolved any solid phases that were originally present in particles at ambient LWCs. Consequently, the measured concentrations of ions in dilute aerosol extracts correspond to the total concentrations (ionized + solid) that existed in ambient aerosol prior to extraction. In these cases, the ratios of ionized to total (ionized + solid) , , and Cl− predicted by the model were used to calculate the fractions of the measured concentrations that were ionized in aerosol solutions at ambient RH.
Equilibrium hydrogen ion activities for PM2.5 and PM10 during each sampling interval were calculated based on the measured phase partitioning and associated thermodynamic properties of compounds with pH-dependent solubilities (HNO3, NH3, and HCl) following the approach of Keene and Savoie (1998). Briefly, using HNO3 as an example, the equilibrium
was evaluated on the basis of simultaneous measurements of gas-phase HNO3 mixing ratios and particulate concentrations in air; temperature-adjusted Henry's Law (KH) and acidity (Ka) constants for HNO3 (Young et al., 2013); and aerosol LWCs, activity coefficients, and the fractions of measured particulate concentrations that were ionized as predicted by E-AIM (described above).
Although all concentrations of particulate Cl− were greater than estimated detection limits, most mixing ratios (75 %) for volatile inorganic Cl were less than the detection limit and the balance of measurements was near the detection limit. Consequently, the phase partitioning of HCl and associated data interpretations were poorly constrained. However, NH3, HNO3, particulate , and particulate were present at concentrations well above the corresponding detection limits and, as described in Sect. 3.2.4 below, the measured gas–aerosol phase partitioning of NH3 and HNO3 yielded paired estimates of aerosol solution pHs that agreed well (generally within ±0.1 to ±0.3 pH units). In the absence of direct reliable measurements of HCl, the equilibrium mixing ratio for HCl during each sampling interval (hereafter referred to as HClcalc) was estimated using the same thermodynamic approach described above based on the mean H+ activity inferred from the measured phase partitioning of NH3 and of HNO3, the Cl− concentration for PM2.5, the thermodynamic properties of HCl, meteorological conditions, the aerosol LWC, Cl− activity coefficient, and fraction of measured particulate Cl− that was ionized as predicted by E-AIM.
Results based on the above approach are subject to several inherent limitations. (1) As indicated above, E-AIM IV evaluates only a subset of major inorganic constituents. Because potential influences of organic matter on aerosol hygroscopic properties are not considered, the modeled estimates of water contents may diverge to some extent from those in ambient air. However, as mentioned above, paired independent estimates of aerosol solution pH based on the phase partitioning of HNO3 and of NH3 and corresponding meteorological conditions measured simultaneously yielded similar results. These two compounds have distinct thermodynamic properties and associated pH-dependent solubilities; the solubility of HNO3 decreases, whereas that of NH3 increases with decreasing solution pH. The good agreement between the paired results suggests that estimates of aerosol pH during the campaign were relatively insensitive to potential influences of organic matter on water contents. (2) PM2.5 and PM10 were collected in bulk over relatively long (nominally 12 h) sampling intervals, which could have driven artifact phase changes of compounds with pH-dependent solubilities and associated bias in the measured gas and particle phases species concentrations. For example, based on their thermodynamic properties, NH3 partitions preferentially with the more highly acidic, typically smaller longer-lived aerosol size fractions, whereas HNO3 and HCl partition preferentially with less acidic, typically larger shorter-lived aerosol size fractions (Keene et al., 2004; Young et al., 2013). When chemically distinct particles are mixed together in a bulk PM2.5 or PM10 sample, the pH of the bulk mixture typically differs from that of the aerosol size fractions with which these gases partitioned preferentially in ambient air, which drives artifact volatilization of NH3 as well as HNO3 and HCl (Keene et al., 1990; Young et al., 2013). (3) Similarly, mixing chemically distinct particles sampled at different times over sampling intervals could drive artifact volatilization or condensation of gases. Exposing time-integrated aerosol samples to gas-phase mixing ratios that vary over sampling intervals can also drive artifact phase changes. Because of their large surface-to-volume ratios, sub-micrometer-diameter particles rapidly equilibrate (in seconds to minutes) with interstitial gases and, consequently, are typically at or near thermodynamic equilibrium with the gas phase (Meng and Seinfeld, 1996). In contrast, larger particles equilibrate more slowly and may exhibit finite phase disequilibria (e.g., Keene and Savoie, 1998). The assumption of thermodynamic equilibrium on which this analysis is based may not be entirely valid for constituents associated primarily with larger aerosol size fractions. (4) In addition, the use of average values to characterize meteorological conditions over sampling intervals does not capture the full range of variability of the multiphase system. On most days, RH fell to minima less than 60 % during daytime and increased to maxima greater than 75 % at night. Consequently, based on E-AIM, the actual hydration state of particles varied from virtually dehydrated to virtually completely deliquesced conditions over most diel cycles. Presumably, between collection and recovery, the compositions of aerosol deposits on sample filters exposed to ambient air also evolved in response to changing RH and temperature. If so, meteorological conditions at recovery times rather than those averaged over sampling intervals may be more appropriate metrics for evaluating phase partitioning and pH. (5) Finally, the thermodynamic properties of gases considered herein (particularly HCl) are associated with non-trivial uncertainty that contributes to variability in results as discussed by Young et al. (2013). Despite these limitations, the results provide useful insight regarding major processes that modulate gas–aerosol phase partitioning of major atmospheric constituents, associated aerosol acidities, and pH-dependent chemical transformations in the Kathmandu Valley.
2.9 Extraction and analysis of organic species in PM2.5 by gas chromatography mass spectrometry
All glassware used in solvent extraction was prewashed with ultrapure water and baked at 500 ∘C for 5.5 h. Based on the OC loading on the filters, one or more QFF for each time period was extracted following the procedure described in Al-Naiema et al. (2015). Organic species were analyzed using gas chromatography coupled to mass spectrometry (GC-MS, Agilent Technologies GC-MS 7890A) equipped with an Agilent DB-5 column (30 m × 0.25 mm × 0.25 µm) and electron ionization (EI) source with a temperature program described in Stone et al. (2012). All the measured species were field blank subtracted, and analytical uncertainties of the measurements were propagated from the standard deviation of the field blanks and 20 % of the measured concentration to conservatively account for compound recovery from QFF. Details of the extraction process and GC temperature program are provided in the Supplement (S1).
2.10 Quality control in chromatographic measurements of PM
For every five ambient samples, one lab blank, one field blank, and one spike recovery sample were analyzed for both organic species and inorganic ions. Spike samples were prepared from blank filters spiked with known concentrations of analytes. These quality control samples were extracted simultaneously with ambient samples. Spike recoveries, reported as percent, were calculated as the quotient of the lab blank-corrected measured concentration and spiked concentration. Spike recoveries of all the reported chemical species were within ±20 % for the organic species and ±10 % for the inorganic ions. Reproducibility and method detection limits for all the organic species are presented in Table S1 in the Supplement.
2.11 Chemical mass balance modeling
PM2.5 OC was apportioned to its contributing sources using the Environmental Protection Agency's chemical mass balance (EPA-CMB) model (version 8.2) using molecular marker concentrations in ambient PM2.5 and source profiles as model inputs. Source profiles for garbage burning (fire nos. 14A and 14B), open biomass burning (fire no. 39), biomass- and dung-powered traditional cooking stoves (fire nos. 37, 38, 40, and 41) were drawn from NAMaSTE in 2015 (Jayarathne et al., 2018). Other primary and secondary source profiles were drawn from the literature: vegetative detritus (Hildemann et al., 1991; Rogge et al., 1993), non-catalyzed gasoline engines (Lough et al., 2007; Schauer et al., 2002), diesel engines (Lough et al., 2007), small-scale coal combustion (Zhang et al., 2008), isoprene, monoterpene, and sesquiterpene-derived SOA (Kleindienst et al., 2007), and aromatic SOA from naphthalene and methyl-naphthalene (Kleindienst et al., 2012). The model sensitivity to the input source profiles were evaluated by systematically varying the biomass burning and garbage burning profiles developed in NAMaSTE-2015 (Jayarathne et al., 2018), which examined the following source profiles: open biomass burning, a mud stove fueled by wood and cow dung, a mud stove fueled by cow dung, a mud stove fueled by twigs, a mud stove fueled by wood, and mixed garbage burning (samples A and B, discussed further in Sect. 3.6).
2.12 Statistical analysis
The detectability of organic and inorganic species was 100 %, except for 17α(H)-22,29,30-trisnorhopane (96 %), 17β(H)-21α(H)-30-norhopane (96 %), cholesterol (93 %), stigmasterol (89 %), 1-methylchrysene (93 %), stigmastanol (67 %), retene (56 %), and coprostanol (30 %). Prior to statistical analysis, data points with values below detection limits were replaced with the limit of detection (LOD) (Hewett and Ganser, 2007). All the concentrations were tested for normality and lognormality using the Anderson–Darling test. Concentrations of all the species were either normally or lognormally distributed; thus Pearson's correlation (r) was employed for correlation analysis. Two sample t tests were used to compare the means of daytime and nighttime concentrations. All statistical tests were performed in Minitab (version 17) and significance was assessed at the 95 % confidence interval (p≤0.05).
3.1 Abundance of VOC, CO2, and CO
3.1.1 VOC abundance
Excluding oxygenated compounds, the 10 most abundant NMVOCs in descending order based on median values were ethene, ethyne, ethane, propene, propane, i-pentane, i-butane, n-butane, toluene, and m∕p-xylene. These and other selected NMVOC measurements in WAS are summarized in Table 2, with the corresponding precisions, accuracies, and detection limits. Ethene and propene are major biomass burning products and major components of vehicle exhaust (Akagi et al., 2011; Guo et al., 2011), so their high abundance is expected given the prevalence of these sources. Ethyne is a general combustion tracer that is expected to reflect vehicular, biomass, and biofuel combustion (Abad et al., 2011). Ethane also has multiple major sources including fossil fuel evaporation and combustion, biomass burning, and biofuel combustion (Guo et al., 2011; Xiao et al., 2008). Ethane correlated most strongly with the combustion tracer ethene (r=0.81) and with CH4 (r=0.66), which has many anthropogenic sources, suggesting that multiple ethane sources contribute. CH4 is likely then to derive at least partially from combustion and is not expected to derive from natural gas production, processing, or transmission, due to a lack of natural gas infrastructure in the Kathmandu Valley in 2015, aside from a small number of household-scale biogas plant systems. Additionally, the presence of numerous outliers in the data set suggests that individual grab samples were occasionally impacted disproportionately by local rather than regional sources (Table S2). The C3-C5 alkanes are not major biomass burning products but are associated with liquefied petroleum gas (LPG) and gasoline (Guo et al., 2011). Their abundance here suggests a traffic or fossil fuel source (as discussed in Sect. 3.1.2). In urban centers toluene often reflects traffic, gasoline evaporation, and/or solvents (Ou et al., 2015; Tsai et al., 2006). Four-stroke engines are abundant in the Kathmandu Valley, and their emissions are rich in aromatic VOCs (Shrestha et al., 2013). Here toluene correlated best with C4-C5 alkanes and ethylbenzene (r=0.86 to 0.94) though surprisingly poorly with the vehicle exhaust tracer ethene (r=0.01). While recognizing the limited sample size, this could suggest gasoline evaporation due to toluene's correlation with i-pentane (Tsai et al., 2006). Further study would help to clarify toluene's sources.
Table 2Means, standard deviations, medians, and ranges of concentrations of methane, CO, CO2, carbonyl sulfide (COS), and select non-methane volatile organic compound (NMVOC) mixing ratios measured at Bode in April 2015 (n=9). Species reported here include the 20 most abundant species, with all NMVOC measurements provided in Table S1. Units are in parts per billion by volume, unless noted.
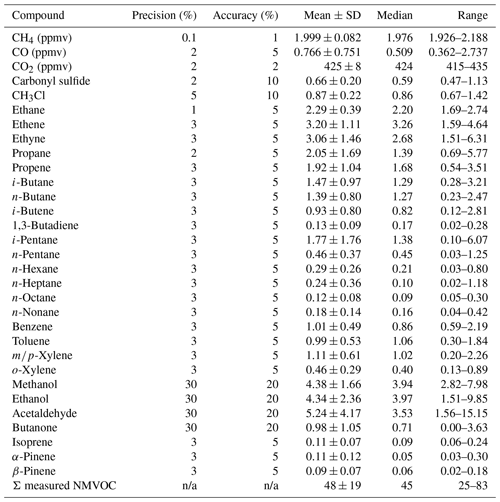
n/a: not applicable.
Relative to previous measurements of NMVOC with a PTR-TOF-MS (proton transfer reaction time-of-flight mass spectrometer) at Bode from December 2012 to January 2013 during the SusKat-ABC campaign (Sarkar et al., 2016), concentrations of the NMVOCs listed in Table 3 were generally lower during NAMaSTE in April 2015. Seasonal variability in meteorology likely contributes to these differences. Mixing layer depths and associated dilution of regional emissions peak during the pre-monsoon season (March–May, including this study), whereas mixing layer depths are shallower during winter (Mues et al., 2017). Several rain events occurred during April 2015 (specifically on 12 to 13, 15, 17 to 18, and 21 April) with a total of 24.2 mm of precipitation. Associated scavenging would also have contributed to the lower pollution levels during this study relative to the dry winter season characterized during the SusKat-ABC campaign. Notably, isoprene levels were nearly 10 times lower during April 2015 compared to the winter of 2012–2013. Sample collection in the early morning and late afternoon contributed to low isoprene concentrations in this study as peak isoprene concentration is typically observed during the midday (Karl et al., 2007). Previous studies report two primary reasons for low isoprene emissions: (i) immaturity of leaves, until reaching an age of 23 d (Kuzma and Fall, 1993) and (ii) temperatures lower than 35 ∘C (Monson et al., 1992). By April, nearly all deciduous trees in the Kathmandu Valley have leaves, although spring 2015 was unusually cold and the low temperatures leading up to and during the measurement period did not favor isoprene emissions as further discussed in Sect. 3.2.6. The whole-air sampling in this study provides additional chemical detail to the high time resolution measurements by PTR-TOF-MS during the SusKat-ABC intensive campaign (Sarkar et al., 2016), including the resolution of alkane and aromatic VOC isomers, chlorofluorocarbons, and alkyl nitrates (Table S2).
Table 3Comparison of mean concentrations of select volatile compounds measured during this study with those measured during prior studies in the Kathmandu Valley and cities in South and Southeast Asia. Units are in parts per billion by volume.
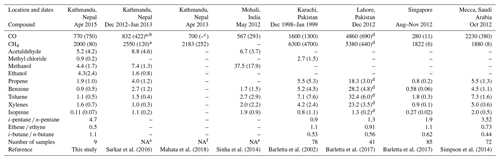
a Data from Bhardwaj et al. (2018). b Monthly average for January 2013. c Dash denotes data not available. d Standard error. e NMVOC were measured continuously by PTR-MS. f CO and CH4 were measured continuously.
In comparison to other cities in South Asia (Table 3), the NMVOC levels observed in Kathmandu were 1.3 to 8.5 times lower than in Mohali, India (Sinha et al., 2014), 2.6 to 6.7 times lower than in Karachi, Pakistan (Barletta et al., 2002), 9.5 to 33 times lower than in heavily polluted Lahore, Pakistan (Barletta et al., 2017), and about a factor of 2 higher than in Singapore (Barletta et al., 2017). Bearing in mind the small sample size, the observed NMVOC levels in Kathmandu indicate that in April 2015, it was moderately polluted with respect to other South and Southeast Asian cities, with relatively low biogenic VOC influences.
CO2 concentrations in Kathmandu are elevated above the global background (Table 2). CO (like the NMVOCs and PM) is an excellent indicator of air pollution levels and is derived from combustion rather than solvents or secondary sources. As shown in Table 3, the pattern of CO enhancements relative to other studies is similar to the pattern for most NMVOCs. The three studies in Kathmandu during the dry season have similar values to each other. The importance of combustion as a source of air pollutants, particularly PM, is consistent with the carbon mass balance source apportionment results discussed in Sect. 3.3.
3.1.2 VOC sources
Because the NMVOC data set is too small (n=9) to be used in source apportionment techniques like positive matrix factorization (PMF), we use VOC ratios to further probe source influences. All NMVOC ratios cited herein have been reported previously by Simpson et al. (2014) and references therein, Akagi et al. (2011), and Stockwell et al. (2016). The ratio of i-pentane ∕ n-pentane increases from ∼1 for natural gas to ∼4 for gasoline evaporation. Here, the ratio was 4.7±0.4 (r=0.97), consistent with gasoline evaporation as has been seen in other cities such as Mecca, Saudi Arabia (Simpson et al., 2014). The diurnal ambient temperature during this study ranged from 12 to 29 ∘C, which is conducive to evaporation. As in Mecca, fuel pump hoses in the Kathmandu Valley are not equipped with vapor recovery technology and vehicles are not equipped with catalytic converters, which may partially explain the abundance of the gasoline evaporation tracer i-pentane. Ethene/ethyne can be used to differentiate petrochemical sources (10–30) from biomass burning (2–5) and vehicle exhaust (1–3), with ratios below 1 reflecting older emissions control technology due to higher ethyne emissions. Here the ratio was 0.5, similar to Saudi Arabia (0.73), suggesting a very large impact of older or poorly maintained vehicles (Zhang et al., 1995). The i-butane ∕ n-butane ratio can sometimes help to distinguish influence from vehicles (∼0.2 to 0.3), LPG combustion (∼0.42 to 0.46), and natural gas leaks (∼0.6 to 1.0). Here the butane ratio was relatively high (∼1, r2=0.90) as compared to cities in Saudi Arabia and Pakistan (0.4 to 0.6; Barletta et al., 2017; Simpson et al., 2014). The cause of the relatively abundant i-butane could be a mix of sources, such as non-evaporative liquid petroleum gas emissions (1.39), aged gasoline generator (1.17), diesel generator (0.87), agricultural fires (0.93), or zigzag kilns (0.84) (Stockwell et al., 2016), which requires further investigation. Acetaldehyde was a major NMVOC consistent with past work (Table 3), and it has a variety of poorly constrained primary and secondary sources (Akagi et al., 2011; Stockwell et al., 2016). These observations are consistent with the PMF analysis of NMVOC measurements during the SusKat-ABC intensive campaign, which indicated that traffic and industrial emissions were the largest sectors contributing to NMVOC mass loadings, at 17 % and 18 %, respectively (Sarkar et al., 2017). The large diversity of combustion emissions in Kathmandu, the apparent influence of point NMVOC sources, and chemical signatures not previously observed in South Asia (discussed above) indicate that additional research with a larger sampling size is needed to better understand NMVOC sources in the Kathmandu Valley. Such research is ongoing as part of our second Nepal Ambient Monitoring and Source Testing Experiment (NAMaSTE2).
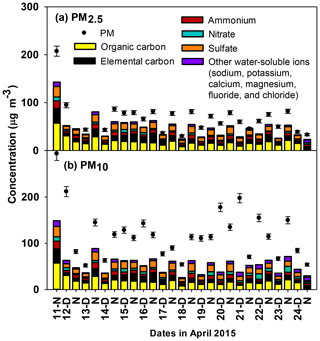
Figure 1Non-water mass concentrations of (a) PM2.5 and (b) PM10 during daytime and nighttime periods and mass contributions from OC, EC, and inorganic ions. OC and EC were not measured in PM10 samples and were assumed to be the same as PM2.5 for mass balance purposes. The remaining mass of PM2.5 includes elements associated with OC (hydrogen, oxygen, nitrogen, etc.), metals, and other unmeasured species. Error bars represent propagated analytical uncertainties. The PM2.5 mass was not quantified for nighttime samples collected on 12 April nor 13 April as described in Sect. 2.2.
3.2 Particulate matter and inorganic trace gases
3.2.1 PM2.5 and PM10 concentrations
PM2.5 mass concentrations averaged over 11 h at the Bode supersite from 11 to 24 April 2015 ranged from 30.0 to 207.4 µg m−3 (Fig. 1) and averaged (± standard deviation) 68.2±34.7 µg m−3. PM10 mass concentrations ranged from 51.9 to 294.0 µg m−3 and averaged 119.7±55.2 µg m−3. All of the 11 h PM2.5 and PM10 concentrations exceed the World Health Organization (WHO) 24 h guidelines of 25 and 50 µg m−3, respectively. The maximum concentrations of PM2.5 and PM10 occurred during the night of 11 April (Fig. 1), concurrent with the Bisket Jatra festival; see Sect. 3.4 for a detailed description of this pollution event and its source characteristics.
The average PM2.5 concentration observed in this study is about half the mean 24 h average PM2.5 concentration near six major road intersections in the Kathmandu Valley (which averaged 125±56 µg m−3) during the relatively drier period February–April 2014 (Shakya et al., 2017b). The average PM2.5 concentration in the Kathmandu Valley was about a factor of 2 higher than at a more rural and cleaner foothill site at Godawari (34 µg m−3) during April 2006 (Stone et al., 2010) and about a factor of 13 higher than the PM1 concentration (5.4 µg m−3) at the Nepal Climate Observatory-Pyramid (NCO-P) site (near the base camp for Mt. Everest) in the southern Himalaya (27.95∘ N, 86.82∘ E) during March–April 2006 (Bonasoni et al., 2008). The average PM10 concentration in the Kathmandu Valley observed in this study period is similar to the average concentration (155±124 µg m−3) of total suspended particles at Bode between April 2013 and March 2014 (Chen et al., 2015).
PM concentrations at Bode were consistently higher during nighttime (83 µg m−3 for PM2.5 and 121 µg m−3 for PM10) compared to daytime (54 µg m−3 for PM2.5 and 117 µg m−3 for PM10). The high time resolution data for the AMS total signal (Fig. 2) indicate that PM mass increases overnight, peaks around 08:00 local time, and thereafter decreases to minima around 17:00. Diurnal variability in PM loadings is attributed to four interrelated factors. (1) Boundary layers and the corresponding volumes of air into which pollutants are emitted are relatively shallower at colder nocturnal temperatures (Mues et al., 2017). Although vertical temperature profiles were not measured, the Kathmandu Valley frequently experiences shallow nocturnal inversion as evident in ceilometer measurements during the SusKat-ABC campaign (Mues et al., 2017). (2) Wind speeds during the pre-monsoon season and the corresponding dilution of PM emitted into or produced within that air flow are typically lower at night (< 1 m s−1) relative to daytime (1 to 5 m s−1) (Fig. 2). The afternoon increase in wind speed corresponds to minimum PM values, while lower wind speeds in early evening coincide with higher concentrations. (3) The diurnal wind dynamics in the Kathmandu Valley have been previously described (Mahata et al., 2017; Panday and Prinn, 2009; Panday et al., 2009; Sarkar et al., 2016). From midday to dusk, strong westerly flows carry pollutants from Kathmandu and Lalitpur towards the east and south passes of the valley, and the mixing layer height reaches its maximum. During evening, relatively stagnant cooler air causes pollutants from the Bhaktapur Industrial Estate (which includes ∼19 biomass- and coal-fired brick kilns) located within 1 to 5 km of Bode to accumulate near the surface, with slight elevation due to mild downslope flows. In the early morning, elevated pollutants briefly recirculate back to the surface. Later in the morning, upslope flows loft polluted air prior to the emergence of the strong westerly winds. (4) As discussed in more detail below, diel variability in temperature and RH drove corresponding diel variability in aerosol liquid water content, aerosol solution pH, and the gas–aerosol phase partitioning of compounds with pH-dependent solubilities. The higher RHs and aerosol liquid water contents at night shifted partitioning towards the particulate phase, thereby contributing to relatively higher PM mass concentrations at night. All of the above factors contribute to the relatively higher PM concentrations in near-surface air at Bode during nighttime.
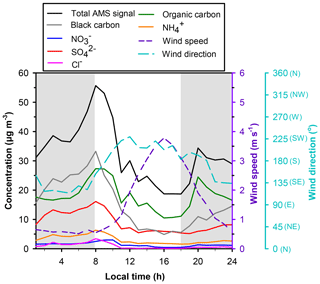
Figure 2Diurnal trends in average total PM1 mass and concentrations of non-refractory inorganic species measured with the AMS, average BC measured with the aethalometer, and average wind speed and direction at Bode on 13 and 16 to 24 April 2015. The shaded region represents the duration for nighttime filter collection and the unshaded region represents the duration for daytime filter collection.
3.2.2 PM2.5 organic and elemental carbon
OC concentrations ranged from 7.9 to 57.3 µg C m−3 (averaging 17.6±9.6 µg C m−3) and accounted for 26±5 % of total PM2.5 mass. The corresponding mass concentrations of organic matter (OM) were estimated by multiplying OC mass concentrations by a factor of 1.7 to account for the associated elements (primarily oxygen, hydrogen, and nitrogen). The OM : OC conversion factor of 1.7 was obtained from the AMS measurement and falls towards the urban end of the range (1.6–2.1) recommended by Turpin and Lim (2001). OM accounted for an average of 48±9 % of PM2.5 mass. EC concentrations ranged from 2.3 to 30.8 µg m−3 (averaging 9.0±6.4 µg m−3) and accounted for 13±6 % of PM2.5 mass. Major sources for OC and EC are discussed in Sect. 3.3.
3.2.3 Inorganic ions in PM, trace gases, and gas–aerosol phase partitioning
The major ionic components of PM2.5 were sulfate, ammonium, and nitrate accounting for 16±4 %, 9±3 %, and 4±2 % of PM2.5 and 11±3 %, 6±2 %, and 3±2 % of PM10, respectively (Table S3). Ratios of these ions indicate that secondary inorganic compounds including ammonium sulfate and ammonium nitrate were important components of PM (Carrico et al., 2003). The relative abundances of these species in the Kathmandu Valley are within the ranges of those reported previously (Shakya et al., 2008, 2010, 2017a).
Large diel variability in temperature and RH drove corresponding variability in aerosol LWC, which contributed to diel variability in the phase partitioning of , , and and aerosol solution pH (Fig. 3). In contrast, under acidic conditions (as existed during this campaign; see below) and in the presence of high aerosol surface area, SO2 and H2SO4 are relatively insensitive to the variability of aerosol LWC and solution pH. Under these conditions, virtually all SO2 partitions into the gas phase and virtually all H2SO4 partitions into the particulate phase. Consequently, the phase partitioning of oxidized S (Fig. 3f) can be interpreted without complications introduced by corresponding phase changes in response to variable LWC and pH.
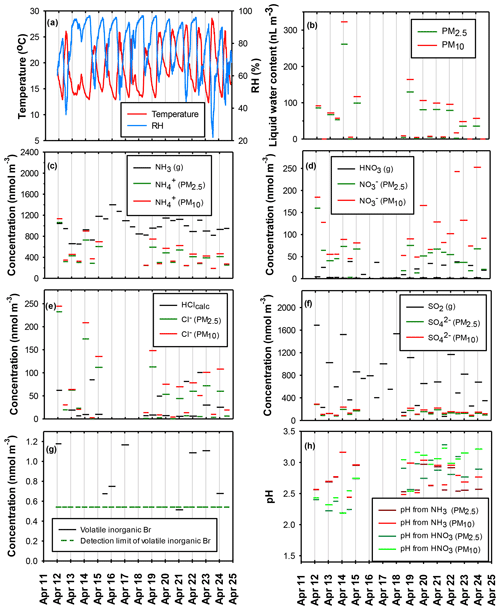
Figure 3(a) Temperature and RH; (b) liquid water content (LWC) of PM2.5 and PM10; (c) gas-phase NH3 and particulate associated with PM2.5 and PM10; (d) gas-phase HNO3 and particulate associated with PM2.5 and PM10; (e) gas-phase HClcalc and particulate Cl− associated with PM2.5 and PM10; (f) gas-phase SO2 and particulate associated with PM2.5 and PM10; (g) detectable concentrations of volatile inorganic Br; (h) equilibrium aerosol solution pH calculated from the measured gas–aerosol phase partitioning of NH3 with PM2.5 and PM10 and HNO3 with PM2.5 and PM10. Vertical grid lines denote local midnight.
Both SO2 and particulate were systematically higher at night and lower during the daytime (Table 4, Fig. 3f). The average concentration of particulate measured with the AMS followed a similar day–night trend, with peak concentrations occurring around 08:00 local time (Fig. 2). The corresponding total oxidized S (SO2 + particulate ) during daytime versus nighttime (Table 4) typically differed by factors of 2 to 5. If the photochemical oxidation of SO2 to H2SO4 had contributed significantly to the diel variability in SO2, SO2 and particulate would have been anticorrelated, which was not the case. These results imply that diel variability in atmospheric dynamics (wind velocity, boundary layer depth, and transport of chemically distinct air masses within the valley, such as air masses with brick kiln influence at night) were major drivers of the observed variability in both species as discussed in Sect. 3.2.1.
Table 4Mean (± standard deviation) concentrations of reactive gases, particulate phase inorganic ions, and percent of these species in gas phase. Total concentrations correspond to the sum of gas phase and PM10.

* Bromine concentrations below detection limit were replaced with the limit of detection (LOD) ∕ √2 in calculating average and standard deviation.
Total NH3 (NH3 + particulate ) exhibited a diel pattern similar to that of oxidized S (Table 4, Fig. 3c) although relative day–night differences were proportionally smaller (typically less than a factor of 2). Day–night differences in total NO3 (HNO3 + particulate ) and total Cl (HClcalc + particulate Cl−) (Table 4, Fig. 3d and e, respectively) were somewhat more variable but also tended to be higher at night than during the day. Taken together, the above results support the hypothesis that the transport of chemically distinct air masses from different source regions during daytime versus nighttime was a major factor that drove diel variability in the composition of the multiphase gas–aerosol system at Bode. Concentrations of Na+ (in nmol m−3) associated with PM2.5 (median – 4.0, range – undetectable to 19.0) and PM10 (median – 8.7, range – undetectable to 40.9) were typically much lower than those of particulate Cl− and total Cl (Fig. 3e). These relationships indicate that, in contrast to some other continental regions (e.g., Young et al., 2013; Jordan et al., 2016), refractory NaCl emitted from crustal and/or marine sources was not the primary source for particulate and volatile Cl at Bode. Instead, total Cl (HClcalc + particulate Cl−) showed high correlation with potassium (r=0.91, p<0.001) and total NH3 (NH3 + particulate ) (r=0.78, p<0.001), suggesting their co-emission from biomass burning (Jayarathne et al., 2018; Keene et al., 2006; Sheesley et al., 2003), brick kilns located within the Kathmandu Valley that impact air masses arriving at Bode at night (Stockwell et al., 2016), and/or garbage burning (Jayarathne et al., 2018).
Concentrations of volatile inorganic Br (including HBr, Br2, HOBr, BrCl, and BrO) ranged from < 0.54 to 1.18 nmol m−3 and were greater than the detection limit in 8 of 27 samples (Fig. 3g). Seven of the eight detectable mixing ratios were during nighttime sampling intervals, which suggests a possible diel cycle in multiphase chemical processing of volatile Br and/or systematic variability as a function of transport from different source regions. Br− was not measured in aerosol samples, so the corresponding variability of particulate and total (volatile + particulate) Br is not known. Possible sources for reactive Br in the region include biomass burning and fossil fuel combustion (Sander et al., 2003).
Concentrations of volatile and particulate inorganic Cl measured at Bode fell within the ranges of those measured in polluted continental air (Young et al., 2013). In addition, concentrations of volatile inorganic Br and Cl at Bode fell within the ranges of those measured in marine air (Keene et al., 2009; Sander et al., 2003). The lack of relevant ancillary measurements during the period of the campaign precluded a quantitative assessment of the potential impacts of reactive halogens on regional air quality in the Kathmandu Valley. However, drawing on related measurements and model calculations elsewhere (Keene et al., 1999, 2009; Long et al., 2014; Sander et al., 2003; Young et al., 2013), our results in conjunction with the presence of acidic, deliquesced aerosol support the hypothesis that multiphase halogen-radical chemistry involving both Br and Cl impacted air quality via two pathways. (1) At high NOx mixing ratios in polluted continental regions, the nocturnal reaction of N2O5 with particulate Cl− produces significant ClNO2, which photolyzes following sunrise yielding a burst of Cl atoms (e.g., Brown et al., 2013). ClNO2 is also a nocturnal reservoir for NOx and thereby slows NOx destruction at night. (2) The scavenging of volatile HOCl and HOBr into acidic aerosol solution and their subsequent reaction with Cl− and Br− produces Cl2, BrCl, and Br2, which subsequently volatilize to the gas phase and photolyze during daytime yielding atomic Br and additional atomic Cl (e.g., Keene et al., 2009). These autocatalytic reactions proceed in both the light and dark and would enhance halogen activation at night and sustain halogen-radical chemistry during daytime relative to predictions based on ClNO2 activation alone. The associated production and scavenging of halogen nitrates also accelerates the destruction of NOx. Cl and Br radicals contribute to the oxidation of hydrocarbons and, together with related reactions that impact NOx cycling, perturb HOx–NOx photochemistry relative to that predicted in the absence of reactions involving halogens.
Particulate calcium, magnesium, and fluoride in the Himalayan region originate primarily from the deflation of surface soils (Carrico et al., 2003). Contributions of these ions to PM10 mass were statistically greater (p=0.03, 0.01, and < 0.001, respectively) relative to those to PM2.5 (Table S3) consistent with results reported previously by Hinds (2012). Heavy vehicular traffic on the many unpaved roads in the valley and to some extent windblown soil dust from the open agriculture fields (by relatively stronger winds in the pre-monsoon season) likely contributed to regional dust emissions. This speculation is supported by the significantly higher concentrations of the calcium and magnesium associated with PM10 during daytime (p=0.049 and 0.005, respectively) when vehicular traffic is higher relative to night. The long-distance transport of dust from arid regions upwind may also contribute to that produced locally.
3.2.4 Aerosol pH
Aerosol solution pH, inferred from the phase partitioning of NH3 and HNO3, ranged from 2.2 to 3.3, and most paired estimates for the same sampling interval agreed within ±0.1 to ±0.3 pH units (Fig. 3h). Solution pH estimated from the partitioning of a given gas with PM10 was greater than those for PM2.5 by < 0.01 to about 0.3 pH units. These results indicate that particles larger than 2.5 µm diameter were typically less acidic than smaller particles, which is consistent with size-resolved acidities characterized in other continental regions (e.g., Young et al., 2013). These results are also consistent with the proportionately greater divergence between concentrations of (Fig. 3d) and Cl− (Fig. 3e) associated with PM10 versus PM2.5 relative to those for (Fig. 3c). Based on their thermodynamic properties, HNO3 and HCl partition preferentially with less acidic particles, whereas NH3 partitions preferentially with the more acidic particles.
3.2.5 PM2.5 molecular markers and secondary organic aerosol tracers
Organic species, particularly molecular markers and SOA tracers (Table 1), were measured to identify sources of OC (Table 5). The identified sources include biomass burning, food cooking, dung burning, garbage burning, coal combustion, fossil fuel use, and secondary aerosol from both biogenic and anthropogenic sources.
Table 5PM2.5 mass concentrations, OC, EC, inorganic ions, and organic species measured at Bode in the Kathmandu Valley.
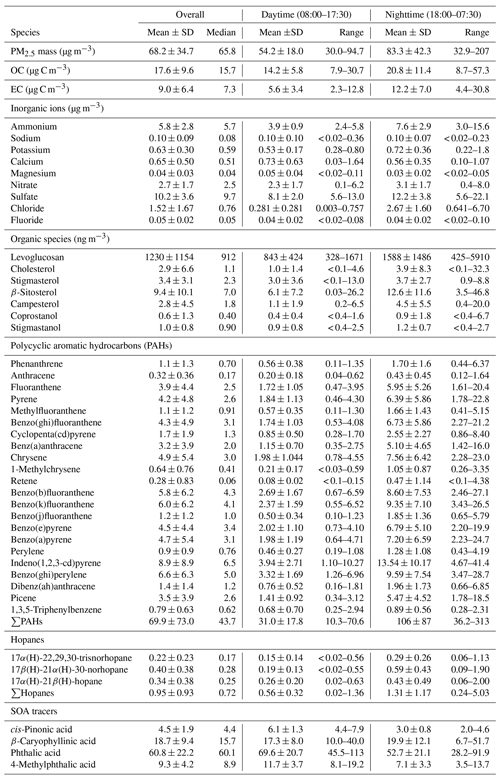
SD: standard deviation.
Herein, we present the first measurements of 1,3,5-triphenylbenzene (TPB) in Nepal, which is a unique molecular marker of plastic burning. This tracer is associated with the combustion of garbage (Jayarathne et al., 2018; Simoneit et al., 2005), which occurs widely in Nepal (Wiedinmyer et al., 2014). Open garbage or trash burning is recognized as a common method to dispose of waste materials in Nepal and other South Asian countries (Wiedinmyer et al., 2014). Garbage contains plastic items (e.g., bags, packaging, food containers) in addition to food waste, paper, cardboard, foil packaging, and other items (Stockwell et al., 2016). TPB was detected in every sample (Fig. 4a). Ratios of TPB to OC concentrations during daytime and nighttime (0.04±0.02 ng µgOC−1) suggest that garbage burning contributed generally consistent fractions of PM2.5 OC. In addition, TPB was significantly correlated with the biomass burning marker levoglucosan (r=0.66, p<0.001), which suggests that both tracers may have been emitted during the co-combustion of plastic materials and biomass including cellulose-containing food waste, paper, and cardboard in garbage.
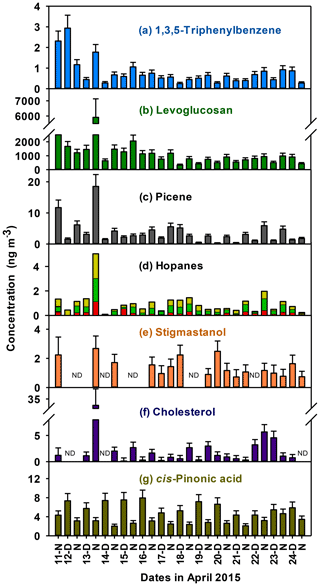
Figure 4Daytime and nighttime concentrations of (a) 1,3,5-triphenylbenzene, (b) levoglucosan, (c) picene, (d) 17α(H)-22,29,30-trisnorhopane in red, 17β(H)-21α(H)-30-norhopane in light green, and 17α(H)-21β(H)-hopane in yellow, (e) stigmastanol, (f) cholesterol, and (g) cis-pinonic acid in PM2.5 in the Kathmandu Valley, Nepal. Error bars represent analytical uncertainties propagated from measurements. Measurements below the instrumental detection limits are marked as ND.
Additional molecular markers indicated the presence of biomass burning, coal combustion, fossil fuel use, dung burning, and food cooking. Levoglucosan, a biomass burning marker, was observed throughout the sampling period (Fig. 4b). Biomass burning emissions that impact the Kathmandu Valley during April include local biomass combustion in brick kilns and food cooking (Pariyar et al., 2013), as well as garden waste burning, agro-residue burning, and regional wild fires (Khanal, 2015; Stone et al., 2010). Picene, a marker of coal combustion (Oros and Simoneit, 2000) and tire burning (Downard et al., 2015), was consistently detected (Fig. 4c). Picene varied diurnally, with higher concentrations at nighttime (0.25±0.13 ng µgOC−1) compared to daytime (0.14±0.16 ng µgOC−1), consistent with southeasterly winds at night that transported emissions from coal-fired brick kilns to Bode. Nine hopanes were identified, indicating fossil fuel influences on PM2.5 in the form of coal burning and/or vehicle emissions (Fig. 4d). Stigmastanol, a unique molecular marker of cow dung burning (Sheesley et al., 2003), was detected in about 67 % of the samples (Fig. 4e, Table 5). Cholesterol, which is emitted from both dung burning (Sheesley et al., 2003) as well as food cooking (Rogge et al., 1991), was detected in 93 % samples (Fig. 4f). Cholesterol and stigmastanol exhibited distinct temporal variabilities, the former having higher OC-normalized concentrations during nighttime and the latter having higher OC-normalized concentrations during daytime. This suggests that these two were emitted from different sources, i.e., cholesterol from food cooking.
Concentrations of molecular markers in Bode measured in this study are compared to a more rural site at Godawari, on the outskirts of the Kathmandu Valley during April 2006 (Stone et al., 2010). Godawari is located ∼11 km south of Bode at the base of the western face of a mountain (Phulchoki) that rises ∼1200 m above the valley floor, with very low population density nearby. While Bode experiences afternoon westerlies that cross the valley from the western to the eastern passes, the flow in Godawari is dominated by the up- and downslope flows generated by Mt. Phulchoki. Higher concentrations of most markers at Bode, including levoglucosan (by a factor of 5), picene (by a factor of 23), and 17β(H)-21α(H)-30-norhopane (by a factor of 13) indicate the larger impact of biomass burning and fossil fuel combustion at this site, compared to Godawari, likely due to the higher population density and industrial activities. The average concentration for stigmastanol at Bode (1.02 ng m−3) was similar to Godawari (0.9 ng m−3) (Stone et al., 2010). Although dung burning is not common in the Kathmandu Valley and its outskirts, dung is a more widely used fuel in rural areas of southern Nepal and India. Thus, it is expected that most of the dung burning tracers observed at Bode were primarily transported from other regions.
SOA produced from the oxidation of the biogenic precursors (monoterpenes and sesquiterpenes) were indicated by cis-pinonic acid and β-caryophyllinic acid, respectively (Fig. 4g, Table 5). OC normalized concentrations of cis-pinonic acid (ratio of cis-pinonic acid to total OC associated with PM2.5) were significantly higher during daytime compared to nighttime (p<0.001), which is consistent with the photochemical production of cis-pinonic acid during daytime (Claeys et al., 2007; Kleindienst et al., 2007) but may also reflect relative differences in emissions of precursors for cis-pinonic acid in air mass source regions upwind of the site during daytime versus nighttime. In contrast, isoprene concentrations during the study period were low (Table 2) and corresponding concentrations of methyltetrols (tracers of SOA produced from the oxidation of isoprene) were below detection limits. These results are consistent with the expectation of low isoprene emissions from recently emergent leaves coupled with relatively low temperatures as discussed above (Kuzma and Fall, 1993; Lewandowski et al., 2008; Monson et al., 1992; Shen et al., 2015). The chromatographic data suggested the presence of 2-methylglyceric acid, an isoprene SOA tracer generated under high-NOx conditions; however, this species could not be semiquantified due to the low recovery (< 10 %) of structurally similar hydroxy acids from the solvent extraction. Nonetheless, these results suggest that isoprene-derived SOA in the Kathmandu Valley has a larger relative contribution from high-NOx reactions compared to low NOx. The relative distribution of high- and low-NOx isoprene SOA tracers should be evaluated in future studies.
Phthalic acid and 4-methylphthalic acid (Table 5) are photooxidation products of naphthalene and methyl-naphthalene, respectively (Kleindienst et al., 2012). These species were also reported to be observed from vehicle emission (Kawamura and Kaplan, 1987) but may be used as anthropogenic SOA tracers in an absence of correlation with primary source tracers (e.g., hopane) (Al-Naiema and Stone, 2017). Phthalic acid and 4-methylphthalic acid did not correlate significantly with hopanes (r=0.29 and 0.25, respectively) and EC (r=0.16 and 0.12, respectively), suggesting that primary combustion was not their major source. Both species were present in significantly higher OC normalized concentrations during the daytime (p<0.001). The daytime maxima may be due to photochemical production (Kleindienst et al., 2012) and/or the transport of air masses that passed over Kathmandu during the daytime.
3.3 Chemical mass balance source apportionment modeling of PM2.5 OC
3.3.1 Base case model result
CMB modeling was used to apportion PM2.5 OC to five primary sources (garbage burning, biomass burning inclusive of open burning and biomass-fueled cooking stoves, gasoline and diesel engines, coal combustion, and vegetative detritus) and four secondary sources (monoterpene SOA, sesquiterpene SOA, naphthalene SOA, and methylnaphthalene SOA). The “base case” results represent the best estimate of the source contributions to OC in this study and utilize the most representative source profiles available (see Sect. 2.11). Of those that were resolvable, primary sources contributed an average of 60±16 % of OC and secondary sources accounted for 15±5 % of OC, while the remaining 25±16 % of OC was not apportioned and is referred to as “other sources” (Fig. 5, Table 6). Other sources may include contributions from cooking with non-biomass fuels (e.g., LPG), mixed industrial emissions, dust emissions, and other uncharacterized primary and secondary sources that could not be apportioned because marker species were not measured (e.g., Si, Al in the case of dust), source profiles are not available (e.g., for local industry), or available profiles are considered unsuitable (e.g., for food cooking, given the inherent variability of this source). Other sources may also include OC from apportioned sources, in the case that they were underestimated. The CMB model did not converge for samples collected on the night of 13 April (13-N) and day of 18 April (18-D), and thus primary source contributions are not reported for these samples.
Table 6Relative primary and secondary source contributions to PM2.5 OC during day and night periods in the Kathmandu Valley. Source contributions during day and night periods were compared and p values < 0.05 (marked by *) indicate significant differences at the 95 % confidence interval.
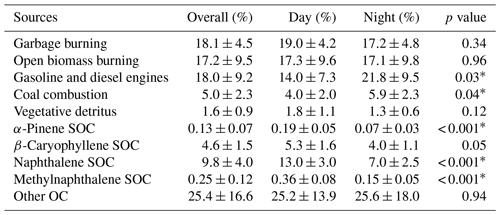
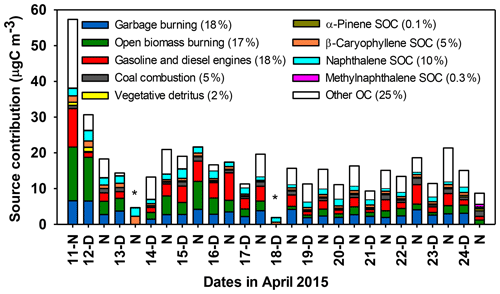
Figure 5Apportionment of primary and secondary sources for PM2.5 OC based on CMB modeling. Values in parentheses are average percent contributions by the corresponding sources. Missing primary sources on 13-N and 18-D of April are marked with stars (see Sect. 3.5).
The garbage burning contribution to PM2.5 OC ranged from 11 % to 27 % and averaged 18±4 %. These results indicate that garbage burning OC is a major source of PM2.5 in the Kathmandu Valley. To our knowledge, this is the first study to apportion PM2.5 OC to garbage burning source based on a unique molecular marker (TPB) in CMB. A tracer based estimation of garbage burning contributions to PM2.5 in the Mexico City Metropolitan Area using antimony (Sb) as a tracer indicated that garbage burning contributed ∼28 % of PM2.5 (Christian et al., 2010). Hodzic et al. (2012) estimated that organic aerosols in the Mexico City valley could be reduced by 2 %–40 % by complete mitigation of garbage burning. The large estimates of garbage burning contributions to PM2.5 OC and PM2.5 in Kathmandu and Mexico City demonstrate the importance of this source to local air quality in heavily polluted urban air. Further, this source should be considered in source apportionment in regions where open garbage burning is a common practice.
Biomass burning contributed 5 % to 43 % of PM2.5 OC, averaging 17±10 %. This estimate is expected to encompass a wide range of biomass burning sources, including biofuel and open burning of biomass. Biomass is widely used as a fuel for household cooking and heating (Pattanayak et al., 2005; Pokhrel et al., 2015; Yevich and Logan, 2003) and in brick kilns (Maithel et al., 2012; Stockwell et al., 2016). In addition, the burning of agricultural residue and wild fires commonly occur in April in Nepal (Khanal, 2015). The source profile used for biomass burning in Nepal was based on the characterization of emissions from an open biomass fire of twigs and dung (Jayarathne et al., 2018) to reflect that molecular markers for wood burning (levoglucosan) and dung burning (stigmastanol) were present. Vegetative detritus, which is waxy material produced from the abrasion of plant leaves, contributed an average of 1.6±0.9 % of PM2.5 OC and is sometimes associated with biomass burning emissions, due to lofted vegetative matter during combustion. A small contribution from this source is consistent with prior studies in the region (Stone et al., 2010).
Contributions from fossil fuel combustion to PM2.5 OC included emissions from gasoline and diesel engines and coal combustion. More than 1 million vehicles (Mahata et al., 2017) and approximately 0.25 million power generators fueled by gasoline and diesel operate within the valley (Mahata et al., 2017). The combined OC contributions to PM2.5 OC from these sources ranged from 5 % to 48 % and averaged 18±9 % (Table 6). These two sources are reported together because they both contribute to evaporative emissions of motor oil. Coal combustion contributed 1 % to 10 % and averaged 5.0±2.3 % of PM2.5 OC. Coal combustion contributions to PM2.5 OC at Bode were significantly greater during nighttime periods (5.9±2.3 %) compared to daytime (3.9±2.0 %, p=0.04). As discussed above, these diel differences reflect the proximity of coal-fired brick kilns located to the south and east of the sampling site coupled with the transport of emissions via the southeasterly winds at night. Relative contributions of coal combustion to PM2.5 (0.8±0.4 µgC m−3) in the Kathmandu Valley are about 4 times greater than those at a more rural location in Nepal (Stone et al., 2010). Brick kilns operate only in the dry season in Nepal and not during the summer monsoon (rainy) season, making this a seasonal PM2.5 source.
Naphthalene-derived secondary organic carbon (SOC) was the largest identified SOA source, contributing 10±4 % of OC, while methylnaphthalene-derived SOC contributed 0.3±0.1 %. Average contributions of biogenic SOC to PM2.5 OC ranged from 0.03 % to 0.29 % for monoterpenes and 1.5 % to 8.3 % for sesquiterpenes. As noted above, isoprene SOA tracers were not quantified. Relatively lower contributions from biogenic SOC during winter and post-winter months were reported in previous studies in Nepal (Stone et al., 2010), the southeastern US (Kleindienst et al., 2007), and the midwestern US (Lewandowski et al., 2008). Thus, the SOA in the Kathmandu Valley during the pre-monsoon season was dominated by anthropogenic influences with a ∼70 % contribution to total SOC. Naphthalene and methylnaphthalene were reported to mainly come from diesel exhaust (Schauer et al., 1999) and industrial emissions and biomass burning (Jia and Batterman, 2010), indicating that reduction in emissions from these sources would reduce anthropogenic SOA production. It is likely that other SOA sources (e.g., those associated with monoaromatic VOCs, biomass burning, and other SOA precursors) that were not characterized in this study also contributed to PM2.5 OC. Contributions from all four SOA sources to PM2.5 OC were significantly greater (p<0.001) during daytime (18±4 %) relative to nighttime (11±3 %) (Table 6). These results are consistent with those reported in other studies (Kleindienst et al., 2007; Plewka et al., 2006; Xu et al., 2015) and are attributed to photochemical production from precursors during daytime and/or regional variability in emissions of precursors in upwind source regions for air transport to Bode during daytime versus nighttime. The low source contribution of biogenic relative to anthropogenic SOC is consistent with the low biogenic VOC levels (Table 2). Based on results from the SusKat-ABC campaign, oxidation products of aromatic VOCs (most importantly benzene) probably also contributed substantially to SOC (Sarkar et al., 2017). In this study, the uncharacterized contributions from aromatics would be classified as other or unapportioned OC.
3.3.2 Sensitivity to garbage and biomass burning profiles
The sensitivity of the CMB source apportionment results to the input source profiles was examined by systematically varying either the garbage burning or biomass burning source profiles while keeping other profiles constant, following prior studies (Sheesley et al., 2003; Stone et al., 2010). The sensitivity test results are summarized in Fig. 6, and the model performance metrics are summarized in Fig. S2.
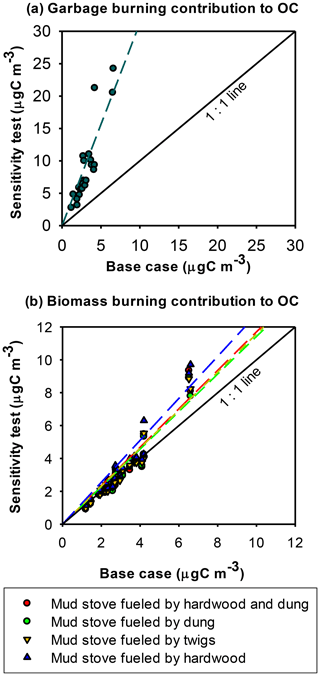
Figure 6Sensitivity of CMB model results to the input source profiles: (a) sensitivity of garbage burning contributions to PM2.5 OC to the garbage burning profile and (b) sensitivity of biomass burning contributions to PM2.5 OC to biomass burning profiles.
Two garbage burning profiles were examined from a single fire of mixed-waste burning (NAMaSTE fire no. 14; Jayarathne et al., 2018). Profile A (the base case profile) corresponds to more smoldering conditions with a modified combustion efficiency (MCE) of 0.89, and profile B corresponding to a mixture of flaming and smoldering combustion with an MCE of 0.93 (Jayarathne et al., 2018). The mixed waste included food waste, paper, plastic bags, cloth, diapers, and rubber shoes. These garbage materials were damp with the previous night's rainfall and were rekindled with newspaper on occasions (Stockwell et al., 2016). Switching from profile A to B increased the amount of PM2.5 OC apportioned to garbage burning by a factor of 3.0 (Fig. 6a) to 46±13 % of OC, indicating that the model was highly sensitive to the garbage burning profile. The model result from profile B, on some days, caused the CMB apportioned OC to exceed the observed OC. Garbage tends to be burned inefficiently, and it is possible that this source contributes to more OC estimated in the base case scenario and may account for much of the unapportioned OC. Given its potential contributions to PM2.5, additional sampling and the characterization of this source are warranted.
Burning of wood, dung, and crop residue is a major energy source of households in South Asia, while crop residue is also burned in fields (Saud et al., 2011; Yevich and Logan, 2003). An open biomass fire of twigs and dung was used as the base case biomass profile (NAMaSTE fire no. 39) (Jayarathne et al., 2018). Four other biomass burning profiles, consisting of a one-pot traditional mud cooking stove fueled with hardwood (fire no. 37), twigs (fire no. 38), dung (fire no. 40), hardwood and dung (fire no. 41), were examined, and the OC apportioned to biomass burning changed by factors of 0.4–1.9 (Fig. 6b). The lowest estimate corresponded to wood- and dung-fueled mud cooking stoves and the highest estimate corresponded to a wood-fueled mud cooking stove, with 15±8 % and 39±16 % OC apportioned to biomass burning (primarily cooking), respectively. The agreement of the sensitivity tests with the base case results being within a factor of 2 indicates a relatively stable CMB apportionment of biomass burning to OC. Because some of the sensitivity tests predict a higher biomass burning contribution to OC than the base case result, this source may contribute to some of the unapportioned OC in the base case result.
The base case CMB model apportioned PM2.5 EC to five primary sources (garbage burning, biomass burning, gasoline and diesel engines, coal combustion, and vegetative detritus). The largest contributor of EC was gasoline and diesel engines (89±7 %), with smaller contributions from biomass burning (7±7 %), coal combustion (3±2 %), garbage burning (1±1 %), and vegetative detritus (0.1±0.1 %) (Fig. S3). EC apportionment was highly sensitive to different biomass and garbage profiles (Fig. S4) with the EC apportioned to garbage burning varying by a factor of 10 and EC apportioned to biomass burning varying by a factor of 0.2–3.4. Due to the large model sensitivity to the selected profile, the EC apportionment is not considered to be as robust as the OC apportionment and is subject to larger uncertainties. To better constrain EC source contributions, additional measurements (i.e., radiocarbon) would be needed.
3.4 PM composition and sources during Bisket Jatra, the local New Year festival
Bisket Jatra, a 9 d festival from 10 to 18 April, celebrated the start of the local New Year (14 April) in the Vikram Samvat calendar. Festivities were held in Bhaktapur, located in the southeast corner of the Kathmandu Valley. Two main events occurred on the afternoons and evenings of 11 and 13 April that attracted a large number of spectators, increased vehicle traffic in the surrounding area, and involved cooking food both indoors and outdoors. The prevailing southeasterly winds during nighttime would have transported air masses from Bhaktapur to Bode. The maximum concentrations of PM2.5 and PM10 measured over the campaign (207 and 294 µg m−3, respectively) were during night of 11 April (Fig. 1). The PM10 concentration on the night of 13 April (145 µg m−3) was also relatively high compared to other sampling periods; the corresponding PM2.5 concentration was not available due to filter damage. OC and EC concentrations were about 3 times higher on 11 April and 2 times higher on 13 April compared to the average values over the study period. Compared to more typical concentrations based on average values during the study period, on 11 April, levoglucosan was 3 times greater and on 13 April, levoglucosan, cholesterol, and hopanes were 5, 11, and 5 to 6 times greater, respectively. Similarly, inorganic gases and other PM2.5 species were elevated on 11 and 13 April by factors of 2 to 4. The increased emissions from cooking and vehicle traffic associated with the festival were the most likely sources for the relatively higher concentrations of these species.
The base case CMB source apportionment on the night of 11 April indicated that sources contributing to PM2.5 OC were biomass burning (26±8 %), gasoline and diesel engines (19±3 %), garbage burning (12±4 %), coal combustion (1.6±0.5 %), vegetative detritus (1.5±0.8 %), monoterpene SOC (0.03±0.02 %), sesquiterpene SOC (3.1±4.2 %), naphthalene SOC (3.7±0.8 %), methylnaphthalene SOC (0.09±0.12 %), and other sources (34±15 %). The biomass burning contribution to PM2.5 OC on the night of 11 April was approximately 1.5 times higher than the average biomass burning contribution during the study period. The magnitude of biomass burning and gasoline and diesel engine contributions to PM2.5 OC on that night were the highest (15±4 µgC m−3 and 11±1 µgC m−3, respectively) among the study period, which were approximately 5 times and 4 times higher than the average contributions of these sources over the study period. The relatively higher contributions from these two sources on the night of 11 April indicated the influences of the Bisket Jatra festival on the air quality in the Kathmandu Valley. In contrast, contributions of other primary sources (garbage burning, coal combustion, and vegetative detritus) and SOA to PM2.5 OC were approximately 2 to 3 times lower on the night of 11 April compared to their study averages. The CMB model did not converge for the sample collected on the night of 13 April, likely because the local pollution sources on that night (e.g., meat cooking indicated by cholesterol) were not well represented by the source profiles in the model.
The major sources of PM2.5 OC during 19–24 April after the 9 d festival were garbage burning (18±4 %), biomass burning (11±3 %), and gasoline and diesel engines (15±6 %). Contributions from biomass burning (23±10 %) and gasoline and diesel burning (21±11 %) were relatively higher during the 9 d festival while the contribution from garbage burning (18±5 %) remained the same. Meanwhile, the percent contributions from other primary and secondary sources remained very consistent throughout the whole sampling period.
Filter sampling and off-line analyses showed that primary combustion sources were the major contributors to volatile and reactive gases, PM2.5, OC, and EC in Kathmandu in mid-April 2015 (pre-monsoon). Using regionally specific source profiles when available, major primary OC sources were estimated to be garbage burning (18±5 %), biomass burning (17±10 %), and gasoline and diesel engines (18±9 %). This study provides the first apportionment of PM to garbage burning in South Asia, and indicates that it is among the major OC sources. However, the model sensitivity tests indicate that the garbage burning source contribution can vary widely depending on the input source profile (and increase by up to a factor of 3), indicating that this source may have an even larger impact on PM. Garbage burning contributions to PM may be further constrained with other elemental tracers (e.g., Sb), and garbage burning should be further characterized in terms of its variability with respect to garbage composition and combustion conditions. The importance of brick kilns to gases and PM in Kathmandu is demonstrated by the elevated concentrations of SO2, , NH3, , K+, and Cl− as well as increased coal burning contributions to PM2.5 OC at night. Gasoline evaporation and poorly maintained vehicles as well as some unidentified mixed sources were recognized as major contributors of VOCs. Since garbage burning, biomass burning, vehicle emissions, and coal combustion are, at least in part, controllable, they are potential targets for emission reductions to reduce ambient PM2.5 in the Kathmandu Valley. Mitigation strategies could include improvements to waste management; higher efficiency of biomass use to reduce PM emissions from cooking, heating, or brick kilns; and reductions in emissions from vehicles. Controlling these combustion sources would also reduce emissions of VOCs, SO2, NOx, and reactive halogen species that impact air quality through interrelated gas-phase and multiphase chemical pathways that produce SOA and contribute to aerosol acidity.
This study characterized air quality in the Kathmandu Valley for 13 d but was halted prematurely by the Gorkha earthquake that struck Nepal on 25 April 2015. A longer period of study is required to better understand the seasonal variation in pollution sources and the role of SOA during periods of higher biogenic VOC levels. Approximately 30 % of the PM2.5 OC was unapportioned to the sources evaluated in this model. Likely additional sources include evaporative emissions from vehicles, local industries, agricultural burning, and unquantified SOA from monoaromatic species (like benzene, toluene, etc.) that were measured in ambient air in this study.
Data reports for reactive trace gases, PM2.5, and PM10 measurements, and CMB model results are freely available for download at https://doi.org/10.17605/OSF.IO/5HNFK (Islam et al., 2019). Other gas-phase measurements are reported in Table S2.
The supplement related to this article is available online at: https://doi.org/10.5194/acp-20-2927-2020-supplement.
EAS, WCK, PFD, RJY, MR, and AKP designed and directed the study; TJ, BW, PSP, SA, AKP, MR, and PFD conducted field operations, collected field samples, and/or collected field data; MRI, TJ, IJS, JM, AG, DRB, and WCK conducted laboratory analyses of samples; MRI, TJ, IJS, BW, JM, AG, DRB, RJY, PFD, WCK, and EAS analyzed data; MRI and EAS conducted CMB modeling. All authors contributed to writing and/or reviewing the paper.
The authors declare that they have no conflict of interest.
We thank Pratik Man Singdan, Bhogendra Kathayat, and Shyam Kumar Newar from Nepal for their help in sample collection.
This project was funded by the National Science Foundation through the grant entitled “Collaborative Research: Measurements of Selected Combustion Emissions in Nepal and Bhutan Integrated with Source Apportionment and Chemical Transport Modeling for South Asia” via award numbers AGS-1351616 to the University of Iowa, AGS-0003865 to the University of Virginia (UVA), and AGS-1349976 to the University of Montana and with the grant entitled “Ambient and Source Characterization of Aerosol Size and Composition in Nepal and Bhutan using High-Resolution Aerosol Mass Spectrometry” via award number AGS-1461458 to Drexel University. Maheswar Rupakheti was supported by the Institute for Advanced Sustainability Studies (IASS) which is funded by the German Ministry of Education and Research (BMBF) and the Brandenburg State Ministry of Science, Research and Culture (MWFK). NAMaSTE was partially supported by core funds of ICIMOD contributed by the governments of Afghanistan, Australia, Austria, Bangladesh, Bhutan, China, India, Myanmar, Nepal, Norway, Pakistan, Switzerland, and the United Kingdom, as well as by funds from the Government of Sweden to ICIMOD's Atmosphere Initiative.
This paper was edited by Steven Brown and reviewed by two anonymous referees.
Abad, G. G., Allen, N. D. C., Bernath, P. F., Boone, C. D., McLeod, S. D., Manney, G. L., Toon, G. C., Carouge, C., Wang, Y., Wu, S., Barkley, M. P., Palmer, P. I., Xiao, Y., and Fu, T. M.: Ethane, ethyne and carbon monoxide concentrations in the upper troposphere and lower stratosphere from ACE and GEOS-Chem: a comparison study, Atmos. Chem. Phys., 11, 9927–9941, https://doi.org/10.5194/acp-11-9927-2011, 2011.
Akagi, S., Yokelson, R. J., Wiedinmyer, C., Alvarado, M., Reid, J., Karl, T., Crounse, J., and Wennberg, P.: Emission factors for open and domestic biomass burning for use in atmospheric models, Atmos. Chem. Phys., 11, 9, 4039–4072, https://doi.org/10.5194/acp-11-4039-2011, 2011.
Al-Naiema, I., Estillore, A. D., Mudunkotuwa, I. A., Grassian, V. H., and Stone, E. A.: Impacts of co-firing biomass on emissions of particulate matter to the atmosphere, Fuel, 162, 111–120, https://doi.org/10.1016/j.fuel.2015.08.054, 2015.
Al-Naiema, I. M. and Stone, E. A.: Evaluation of anthropogenic secondary organic aerosol tracers from aromatic hydrocarbons, Atmos. Chem. Phys., 17, 3, 2053–2065, https://doi.org/10.5194/acp-17-2053-2017, 2017.
Bardwell, C., Maben, J., Hurt, J., Keene, W., Galloway, J., Boatman, J., and Wellman, D. J. G. B. C.: A technique using high-flow, dichotomous filter packs for measuring major atmospheric chemical constituents, Global Biogeochem. Cy., 4, 151–163, https://doi.org/10.1029/GB004i002p00151, 1990.
Barletta, B., Meinardi, S., Simpson, I. J., Khwaja, H. A., Blake, D. R., and Rowland, F. S. J. A. E.: Mixing ratios of volatile organic compounds (VOCs) in the atmosphere of Karachi, Pakistan, Atmos. Environ., 36, 3429–3443, https://doi.org/10.1016/S1352-2310(02)00302-3, 2002.
Barletta, B., Simpson, I. J., Blake, N. J., Meinardi, S., Emmons, L. K., Aburizaiza, O. S., Siddique, A., Zeb, J., Liya, E. Y., and Khwaja, H. A. J. J. o. A. C.: Characterization of carbon monoxide, methane and nonmethane hydrocarbons in emerging cities of Saudi Arabia and Pakistan and in Singapore, J. Atmos. Chem., 74, 87–113, https://doi.org/10.1007/s10874-016-9343-7, 2017.
Bhardwaj, P., Naja, M., Rupakheti, M., Lupascu, A., Mues, A., Panday, A. K., Kumar, R., Mahata, K. S., Lal, S., Chandola, H. C., and Lawrence, M. G.: Variations in surface ozone and carbon monoxide in the Kathmandu Valley and surrounding broader regions during SusKat-ABC field campaign: role of local and regional sources, Atmos. Chem. Phys., 18, 11949–11971, https://doi.org/10.5194/acp-18-11949-2018, 2018.
Birch, M. E. and Cary, R. A.: Elemental carbon-based method for monitoring occupational exposures to particulate diesel exhaust, Aerosol Sci. Technol., 25, 221–241, https://doi.org/10.1080/02786829608965393, 1996.
Bonasoni, P., Laj, P., Angelini, F., Arduini, J., Bonafe, U., Calzolari, F., Cristofanelli, P., Decesari, S., Facchini, M., and Fuzzi, S.: The ABC-Pyramid Atmospheric Research Observatory in Himalaya for aerosol, ozone and halocarbon measurements, Sci. Total Environ., 391, 252–261, https://doi.org/10.1016/j.scitotenv.2007.10.024, 2008.
Brown, S. S., Thornton, J. A., Keene, W. C., Pszenny, A. A. P., Sive, B. C., Dube, W. P., Wagner, N. L., Young, C. J., Riedel, T. P., Roberts, J. M., VandenBoer, T. C., Bahreini, R., Ozturk, F., Middlebrook, A. M., Kim, S., Hubler, G., and Wolfe, D. E.: Nitrogen, Aerosol Composition, and Halogens on a Tall Tower (NACHTT): Overview of a wintertime air chemistry field study in the front range urban corridor of Colorado, J. Geophys. Res.-Atmos., 118, 8067–8085, https://doi.org/10.1002/jgrd.50537, 2013.
Carrico, C. M., Bergin, M. H., Shrestha, A. B., Dibb, J. E., Gomes, L., and Harris, J. M. J. A. E.: The importance of carbon and mineral dust to seasonal aerosol properties in the Nepal Himalaya, Atmos. Environ., 37, 2811–2824, https://doi.org/10.1016/S1352-2310(03)00197-3, 2003.
Chen, P., Kang, S., Li, C., Rupakheti, M., Yan, F., Li, Q., Ji, Z., Zhang, Q., Luo, W., and Sillanpää, M.: Characteristics and sources of polycyclic aromatic hydrocarbons in atmospheric aerosols in the Kathmandu Valley, Nepal, Sci. Total Environ., 538, 86–92, https://doi.org/10.1016/j.scitotenv.2015.08.006, 2015.
Christian, T. J., Yokelson, R., Cárdenas, B., Molina, L., Engling, G., and Hsu, S.-C.: Trace gas and particle emissions from domestic and industrial biofuel use and garbage burning in central Mexico, Atmos. Chem. Phys., 10, 565–584, https://doi.org/10.5194/acp-10-565-2010, 2010.
Claeys, M., Szmigielski, R., Kourtchev, I., Van der Veken, P., Vermeylen, R., Maenhaut, W., Jaoui, M., Kleindienst, T. E., Lewandowski, M., and Offenberg, J. H.: Hydroxydicarboxylic acids: markers for secondary organic aerosol from the photooxidation of α-pinene, Environ. Sci. Technol., 41, 1628–1634, https://doi.org/10.1021/es0620181, 2007.
DeCarlo, P. F., Kimmel, J. R., Trimborn, A., Northway, M. J., Jayne, J. T., Aiken, A. C., Gonin, M., Fuhrer, K., Horvath, T., Docherty, K. S., Worsnop, D. R., and Jimenez, J. L.: Field-deployable, high-resolution, time-of-flight aerosol mass spectrometer, Anal. Chem., 78, 8281–8289, https://doi.org/10.1021/ac061249n, 2006.
Downard, J., Singh, A., Bullard, R., Jayarathne, T., Rathnayake, C. M., Simmons, D. L., Wels, B. R., Spak, S. N., Peters, T., and Beardsley, D.: Uncontrolled combustion of shredded tires in a landfill – Part 1: Characterization of gaseous and particulate emissions, Atmos. Environ., 104, 195–204, https://doi.org/10.1016/j.atmosenv.2014.12.059, 2015.
Friese, E. and Ebel, A.: Temperature dependent thermodynamic model of the system H+--Na+---Cl−-H2O, J. Phys. Chem. A, 114, 11595–11631, https://doi.org/10.1021/jp101041j, 2010.
Giri, D., Murthy, K., Adhikary, P., Khanal, S. J. I. J. o. E. S., and Technology: Ambient air quality of Kathmandu Valley as reflected by atmospheric particulate matter concentrations (PM10), Int. J. Environ. Sci. Technol., 3, 403–410, https://doi.org/10.1007/BF03325949, 2006.
Goetz, J. D., Giordano, M. R., Stockwell, C. E., Christian, T. J., Maharjan, R., Adhikari, S., Bhave, P. V., Praveen, P. S., Panday, A. K., Jayarathne, T., Stone, E. A., Yokelson, R. J., and DeCarlo, P. F.: Speciated online PM1 from South Asian combustion sources – Part 1: Fuel-based emission factors and size distributions, Atmos. Chem. Phys., 18, 14653–14679, https://doi.org/10.5194/acp-18-14653-2018, 2018.
Guo, H., Zou, S. C., Tsai, W. Y., Chan, L. Y., and Blake, D. R.: Emission characteristics of nonmethane hydrocarbons from private cars and taxis at different driving speeds in Hong Kong, Atmos. Environ., 45, 2711–2721, https://doi.org/10.1016/j.atmosenv.2011.02.053, 2011.
Gurung, A. and Bell, M. L.: The state of scientific evidence on air pollution and human health in Nepal, Environ. Res., 124, 54–64, https://doi.org/10.1016/j.envres.2013.03.007, 2013.
Hewett, P. and Ganser, G. H.: A comparison of several methods for analyzing censored data, Ann. Occup. Hyg., 51, 611–632, https://doi.org/10.1093/annhyg/mem045, 2007.
Hildemann, L. M., Markowski, G. R., and Cass, G. R.: Chemical-composition of emissions from urban sources of fine organic aerosol, Environ. Sci. Technol., 25, 744–759, https://doi.org/10.1021/es00016a021, 1991.
Hinds, W. C.: Aerosol technology: properties, behavior, and measurement of airborne particles, John Wiley & Sons, Inc., New York, 1–13, 2012.
Hodzic, A., Wiedinmyer, C., Salcedo, D., and Jimenez, J. L.: Impact of Trash Burning on Air Quality in Mexico City, Environ. Sci. Technol., 46, 4950–4957, https://doi.org/10.1021/es203954r, 2012.
Islam, M. R., Jayarathne, T. J., Gilbert, A., Rupakheti, M., Maben, J., Keene, W. C., and Stone, E. A.: Field Campaign Data from NAMaSTE 2015: PM2.5 and PM10 chemical composition, reactive trace gases, and chemical mass balance model results, https://doi.org/10.17605/OSF.IO/5HNFK, 2019.
Jaoui, M., Lewandowski, M., Kleindienst, T. E., Offenberg, J. H., and Edney, E. O.: β-caryophyllinic acid: An atmospheric tracer for β-caryophyllene secondary organic aerosol, Geophys. Res. Lett., 34, L05816, https://doi.org/10.1029/2006GL028827, 2007.
Jayarathne, T., Stockwell, C. E., Yokelson, R. J., Nakao, S., and Stone, E. A.: Emissions of fine particle fluoride from biomass burning, Environ. Sci. Technol., 48, 12636–12644, https://doi.org/10.1021/es502933j, 2014.
Jayarathne, T., Stockwell, C. E., Bhave, P. V., Praveen, P. S., Rathnayake, C. M., Islam, M. R., Panday, A. K., Adhikari, S., Maharjan, R., Goetz, J. D., DeCarlo, P. F., Saikawa, E., Yokelson, R. J., and Stone, E. A.: Nepal Ambient Monitoring and Source Testing Experiment (NAMaSTE): emissions of particulate matter from wood- and dung-fueled cooking fires, garbage and crop residue burning, brick kilns, and other sources, Atmos. Chem. Phys., 18, 2259–2286, https://doi.org/10.5194/acp-18-2259-2018, 2018.
Jia, C. R. and Batterman, S.: A Critical Review of Naphthalene Sources and Exposures Relevant to Indoor and Outdoor Air, Int. J. Environ. Res. Publ. Health, 7, 2903–2939, https://doi.org/10.3390/ijerph7072903, 2010.
Karl, T., Guenther, A., Yokelson, R. J., Greenberg, J., Potosnak, M., Blake, D. R., and Artaxo, P.: The tropical forest and fire emissions experiment: Emission, chemistry, and transport of biogenic volatile organic compounds in the lower atmosphere over Amazonia, J. Geophys. Res.-Atmos., 112, 302, https://doi.org/10.1029/2007jd008539, 2007.
Kawamura, K. and Kaplan, I. R.: Motor exhaust emissions as a primary source for dicarboxylic acids in Los Angeles ambient air, Environ. Sci. Technol., 21, 105–110, https://doi.org/10.1021/es00155a014, 1987.
Keene, W., Khalil, M. A. K., Erickson, D., McCulloch, A., Graedel, T. E., Lobert, J. M., Aucott, M. L., Gong, S. L., Harper, D. B., and Kleiman, G.: Composite global emissions of reactive chlorine from anthropogenic and natural sources: Reactive Chlorine Emissions Inventory, J. Geophys. Res., 104, 8429–8440, 1999.
Keene, W. C. and Savoie, D. L.: The pH of deliquesced sea-salt aerosol in polluted marine air, Geophys. Res. Lett., 25, 2181–2184, https://doi.org/10.1029/98GL01591, 1998.
Keene, W. C., Talbot, R. W., Andreae, M. O., Beecher, K., Berresheim, H., Castro, M., Farmer, J. C., Galloway, J. N., Hoffmann, M. R., and Li, S. M.: An intercomparison of measurement systems for vapor and particulate phase concentrations of formic and acetic acids, J. Geophys. Res., 94, 6457–6471, https://doi.org/10.1029/JD094iD05p06457, 1989.
Keene, W. C., Pszenny, A. A. P., Jacob, D. J., Duce, R. A., Galloway, J. N., Schultz-Tokos, J. J., Sievering, H., and Boatman, J. F.: The geochemical cycling of reactive chlorine through the marine troposphere, Global Biogeochem. Cy., 4, 407–430, https://doi.org/10.1029/GB004i004p00407, 1990.
Keene, W. C., Pszenny, A. A. P., Maben, J. R., Stevenson, E., and Wall, A.: Closure evaluation of size-resolved aerosol pH in the New England coastal atmosphere during summer, J. Geophys. Res., 109, D23307, https://doi.org/10.1029/2004jd004801, 2004.
Keene, W. C., Lobert, J. M., Crutzen, P. J., Maben, J. R., Scharffe, D. H., Landmann, T., Hély, C., and Brain, C.: Emissions of major gaseous and particulate species during experimental burns of southern African biomass, J. Geophys. Res., 111, D04301, https://doi.org/10.1029/2005JD006319, 2006.
Keene, W. C., Long, M. S., Pszenny, A. A. P., Sander, R., Maben, J. R., Wall, A. J., O'Halloran, T. L., Kerkweg, A., Fischer, E. V., and Schrems, O.: Latitudinal variation in the multiphase chemical processing of inorganic halogens and related species over the eastern North and South Atlantic Oceans, Atmos. Chem. Phys., 9, 7361–7385, https://doi.org/10.5194/acp-9-7361-2009, 2009.
Khanal, S.: Wildfire trends in Nepal based on MODIS burnt-area data, Banko Janakari, 25, 76–79, 2015.
Kim, B. M., Park, J.-S., Kim, S.-W., Kim, H., Jeon, H., Cho, C., Kim, J.-H., Hong, S., Rupakheti, M., Panday, A. K., Park, R. J., Hong, J., and Yoon, S.-C.: Source apportionment of PM10 mass and particulate carbon in the Kathmandu Valley, Nepal, Atmos. Environ. Pt. A, 123, 190–199, https://doi.org/10.1016/j.atmosenv.2015.10.082, 2015.
Kiros, F., Shakya, K. M., Rupakheti, M., Regmi, R. P., Maharjan, R., Byanju, R. M., Naja, M., Mahata, K., Kathayat, B., and Peltier, R. E.: Variability of anthropogenic gases: Nitrogen oxides, sulfur dioxide, ozone and ammonia in Kathmandu Valley, Nepal, Aer. Air Qual. Res., 16, 3088–3101, https://doi.org/10.4209/aaqr.2015.07.0445, 2016.
Kleindienst, T., Jaoui, M., Lewandowski, M., Offenberg, J., and Docherty, K.: The formation of SOA and chemical tracer compounds from the photooxidation of naphthalene and its methyl analogs in the presence and absence of nitrogen oxides, Atmos. Chem. Phys., 12, 8711–8726, https://doi.org/10.5194/acp-12-8711-2012, 2012.
Kleindienst, T. E., Jaoui, M., Lewandowski, M., Offenberg, J. H., Lewis, C. W., Bhave, P. V., and Edney, E. O.: Estimates of the contributions of biogenic and anthropogenic hydrocarbons to secondary organic aerosol at a southeastern US location, Atmos. Environ., 41, 8288–8300, https://doi.org/10.1016/j.atmosenv.2007.06.045, 2007.
Kuzma, J. and Fall, R.: Leaf isoprene emission rate is dependent on leaf development and the level of isoprene synthase, Plant Physiol., 101, 435–440, https://doi.org/10.1104/pp.101.2.435, 1993.
Lewandowski, M., Jaoui, M., Offenberg, J. H., Kleindienst, T. E., Edney, E. O., Sheesley, R. J., and Schauer, J. J.: Primary and secondary contributions to ambient PM in the midwestern United States, Environ. Sci. Technol., 42, 9, 3303–3309, https://doi.org/10.1021/es0720412, 2008.
Li, C. L., Bosch, C., Kang, S. C., Andersson, A., Chen, P. F., Zhang, Q. G., Cong, Z. Y., Chen, B., Qin, D. H., and Gustafsson, O.: Sources of black carbon to the Himalayan-Tibetan Plateau glaciers, Nat. Commun., 7, 12574, https://doi.org/10.1038/ncomms12574, 2016.
Long, M., Keene, W., Easter, R. C., Sander, R., Liu, X., Kerkweg, A., and Erickson, D.: Sensitivity of tropospheric chemical composition to halogen-radical chemistry using a fully coupled size-resolved multiphase chemistry–global climate system: halogen distributions, aerosol composition, and sensitivity of climate-relevant gases, Atmos. Chem. Phys., 14, 3397–3425, https://doi.org/10.5194/acp-14-3397-2014, 2014.
Lough, G. C., Christensen, C. G., Schauer, J. J., Tortorelli, J., Mani, E., Lawson, D. R., Clark, N. N., and Gabele, P. A.: Development of molecular marker source profiles for emissions from on-road gasoline and diesel vehicle fleets, J. Air Waste Manage., 57, 1190–1199, https://doi.org/10.3155/1047-3289.57.10.1190, 2007.
Mahapatra, P. S., Puppala, S. P., Adhikary, B., Shrestha, K. L., Dawadi, D. P., Paudel, S. P., and Panday, A. K.: Air quality trends of the Kathmandu Valley: A satellite, observation and modeling perspective, Atmos. Environ., 201, 334–347, https://doi.org/10.1016/j.atmosenv.2018.12.043, 2019.
Mahata, K. S., Panday, A. K., Rupakheti, M., Singh, A., Naja, M., and Lawrence, M. G.: Seasonal and diurnal variations in methane and carbon dioxide in the Kathmandu Valley in the foothills of the central Himalayas, Atmos. Chem. Phys., 17, 12573–12596, https://doi.org/10.5194/acp-17-12573-2017, 2017.
Mahata, K. S., Rupakheti, M., Panday, A. K., Bhardwaj, P., Naja, M., Singh, A., Mues, A., Cristofanelli, P., Pudasainee, D., Bonasoni, P., and Lawrence, M. G.: Observation and analysis of spatio-temporal characteristics of surface ozone and carbon monoxide at multiple sites in the Kathmandu Valley, Nepal, Atmos. Chem. Phys., 18, 14113–14132, https://doi.org/10.5194/acp-18-14113-2018, 2018.
Maithel, S., Lalchandani, D., Malhotra, G., Bhanware, P., Uma, R., Ragavan, S., and Athalye, V.: Brick Kilns Performance Assessment: A Roadmap for Cleaner Brick Production in India, New Delhi: Shakti sustainable energy foundation climate works Foundation supported initiative, Report, available at: http://www.indiaenvironmentportal.org.in/files/file/Brick_Kilns_Performance_Assessment.pdf (last access: 24 January 2020), 2012.
Meng, Z. and Seinfeld, J. H.: Time scales to achieve atmospheric gas-aerosol equilibrium for volatile species, Atmos. Environ., 30, 2889–2900, https://doi.org/10.1016/1352-2310(95)00493-9, 1996.
Monson, R. K., Jaeger, C. H., Adams, W. W., Driggers, E. M., Silver, G. M., and Fall, R.: Relationships among isoprene emission rate, photosynthesis, and isoprene synthase activity as influenced by temperature, Plant Physiol., 98, 1175–1180, https://doi.org/10.1104/pp.98.3.1175, 1992.
Mues, A., Rupakheti, M., Münkel, C., Lauer, A., Bozem, H., Hoor, P., Butler, T., and Lawrence, M. G.: Investigation of the mixing layer height derived from ceilometer measurements in the Kathmandu Valley and implications for local air quality, Atmos. Chem. Phys., 17, 8157–8176, https://doi.org/10.5194/acp-17-8157-2017, 2017.
NIOSH Manual of Analytical Methods: Method 5040, available at: https://www.cdc.gov/niosh/docs/2003-154/pdfs/5040.pdf (last access: 21 January 2020), 2003.
Oros, D. and Simoneit, B.: Identification and emission rates of molecular tracers in coal smoke particulate matter, Fuel, 79, 515–536, https://doi.org/10.1016/S0016-2361(99)00153-2, 2000.
Ou, J. M., Guo, H., Zheng, J. Y., Cheung, K., Louie, P. K. K., Ling, Z. H., and Wang, D. W.: Concentrations and sources of non-methane hydrocarbons (NMHCs) from 2005 to 2013 in Hong Kong: A multi-year real-time data analysis, Atmos. Environ., 103, 196–206, https://doi.org/10.1016/j.atmosenv.2014.12.048, 2015.
Panday, A. K. and Prinn, R. G.: Diurnal cycle of air pollution in the Kathmandu Valley, Nepal: Observations, J. Geophys. Res.-Atmos., 114, D09305, https://doi.org/10.1029/2008JD009777, 2009.
Panday, A. K., Prinn, R. G., and Schar, C.: Diurnal cycle of air pollution in the Kathmandu Valley, Nepal: 2. Modeling results, J. Geophys. Res.-Atmos., 114, D21308, https://doi.org/10.1029/2008jd009808, 2009.
Pariyar, S. K., Das, T., and Ferdous, T.: Environment and health impact for brick kilns in Kathmandu valley, Int. J. Sci. Technol. Res., 2, 184–187, 2013.
Pattanayak, S. K., Yang, J. C., Whittington, D., and Bal Kumar, K.: Coping with unreliable public water supplies: averting expenditures by households in Kathmandu, Nepal, Water Resour. Res., 41, W02012, https://doi.org/10.1029/2003WR002443, 2005.
Plewka, A., Gnauk, T., Brüggemann, E., and Herrmann, H.: Biogenic contributions to the chemical composition of airborne particles in a coniferous forest in Germany, Atmos. Environ., 40, 103–115, https://doi.org/10.1016/j.atmosenv.2005.09.090, 2006.
Pokhrel, A. K., Bates, M. N., Acharya, J., Valentiner-Branth, P., Chandyo, R. K., Shrestha, P. S., Raut, A. K., and Smith, K. R. J. A. E.: PM2.5 in household kitchens of Bhaktapur, Nepal, using four different cooking fuels, Atmos. Environ., 113, 159–168, https://doi.org/10.1016/j.atmosenv.2015.04.060, 2015.
Pszenny, A., Moldanová, J., Keene, W., Sander, R., Maben, J., Martinez, M., Crutzen, P., Perner, D., and Prinn, R.: Halogen cycling and aerosol pH in the Hawaiian marine boundary layer, Atmos. Chem. Phys., 4, 147–168, https://doi.org/10.5194/acp-4-147-2004, 2004.
Putero, D., Cristofanelli, P., Marinoni, A., Adhikary, B., Duchi, R., Shrestha, S., Verza, G., Landi, T., Calzolari, F., and Busetto, M.: Seasonal variation of ozone and black carbon observed at Paknajol, an urban site in the Kathmandu Valley, Nepal, Atmos. Chem. Phys., 15, 13957–13971, https://doi.org/10.5194/acp-15-13957-2015, 2015.
Rogge, W. F., Hildemann, L. M., Mazurek, M. A., Cass, G. R., and Simoneit, B. R.: Sources of fine organic aerosol, 1. Charbroilers and meat cooking operations, Environ. Sci. Technol., 25, 6, 1112–1125, 1991.
Rogge, W. F., Hildemann, L. M., Mazurek, M. A., Cass, G. R., and Simoneit, B. R.: Sources of fine organic aerosol, 4. Particulate abrasion products from leaf surfaces of urban plants, Environ. Sci. Technol., 27, 2700–2711, 1993.
Sander, R., Keene, W. C., Pszenny, A. A. P., Arimoto, R., Ayers, G. P., Baboukas, E., Cainey, J. M., Crutzen, P. J., Duce, R. A., Hönninger, G., Huebert, B. J., Maenhaut, W., Mihalopoulos, N., Turekian, V. C., and Van Dingenen, R.: Inorganic bromine in the marine boundary layer: a critical review, Atmos. Chem. Phys., 3, 1301–1336, https://doi.org/10.5194/acp-3-1301-2003, 2003.
Sarkar, C., Sinha, V., Kumar, V., Rupakheti, M., Panday, A., Mahata, K. S., Rupakheti, D., Kathayat, B., and Lawrence, M. G.: Overview of VOC emissions and chemistry from PTR-TOF-MS measurements during the SusKat-ABC campaign: high acetaldehyde, isoprene and isocyanic acid in wintertime air of the Kathmandu Valley, Atmos. Chem. Phys., 16, 3979–4003, https://doi.org/10.5194/acp-16-3979-2016, 2016.
Sarkar, C., Sinha, V., Sinha, B., Panday, A. K., Rupakheti, M., and Lawrence, M. G.: Source apportionment of NMVOCs in the Kathmandu Valley during the SusKat-ABC international field campaign using positive matrix factorization, Atmos. Chem. Phys., 17, 8129–8156, https://doi.org/10.5194/acp-17-8129-2017, 2017.
Saud, T., Singh, D., Mandal, T., Gadi, R., Pathak, H., Saxena, M., Sharma, S., Gautam, R., Mukherjee, A., and Bhatnagar, R.: Spatial distribution of biomass consumption as energy in rural areas of the Indo-Gangetic plain, Biomass Bioenerg., 35, 932–941, https://doi.org/10.1016/j.biombioe.2010.11.001, 2011.
Schauer, J. J., Rogge, W. F., Hildemann, L. M., Mazurek, M. A., Cass, G. R., and Simoneit, B. R.: Source apportionment of airborne particulate matter using organic compounds as tracers, Atmos. Environ., 30, 3837–3855, https://doi.org/10.1016/1352-2310(96)00085-4, 1996.
Schauer, J. J., Kleeman, M. J., Cass, G. R., and Simoneit, B. R. T.: Measurement of emissions from air pollution sources, 2. C-1 through C-30 organic compounds from medium duty diesel trucks, Environ. Sci. Technol., 33, 1578–1587, https://doi.org/10.1021/es980081n, 1999.
Schauer, J. J., Kleeman, M. J., Cass, G. R., and Simoneit, B. R.: Measurement of emissions from air pollution sources, 5. C1-C32 organic compounds from gasoline-powered motor vehicles, Environ. Sci. Technol., 36, 1169–1180, https://doi.org/10.1021/es0108077, 2002.
Shakya, K. M., Ziemba, L. D., and Griffin, R. J.: Characteristics and sources of carbonaceous, ionic, and isotopic species of wintertime atmospheric aerosols in Kathmandu Valley, Nepal, Aer. Air Qual. Res., 10, 219–230, https://doi.org/10.4209/aaqr.2009.10.0068, 2010.
Shakya, K. M., Peltier, R. E., Shrestha, H., and Byanju, R. M.: Measurements of TSP, PM10, PM2.5, BC, and PM chemical composition from an urban residential location in Nepal, Atmos. Pollut. Res., 8, 1123–1131, https://doi.org/10.1016/j.apr.2017.05.002, 2017a.
Shakya, K. M., Rupakheti, M., Shahi, A., Maskey, R., Pradhan, B., Panday, A., Puppala, S. P., Lawrence, M., and Peltier, R. E.: Near-road sampling of PM2.5, BC, and fine-particle chemical components in Kathmandu Valley, Nepal, Atmos. Chem. Phys., 17, 6503–6516, https://doi.org/10.5194/acp-17-6503-2017, 2017b.
Shakya, P. R., Shrestha, P., Tamrakar, C. S., and Bhattarai, P. K.: Studies on potential emission of hazardous gases due to uncontrolled open-air burning of waste vehicle tyres and their possible impacts on the environment, Atmos. Environ., 42, 6555–6559, https://doi.org/10.1016/j.atmosenv.2008.04.013, 2008.
Sheesley, R. J., Schauer, J. J., Chowdhury, Z., Cass, G. R., and Simoneit, B. R.: Characterization of organic aerosols emitted from the combustion of biomass indigenous to South Asia, J. Geophys. Res., 108, 4285, https://doi.org/10.1029/2002JD002981, 2003.
Shen, R.-Q., Ding, X., He, Q.-F., Cong, Z.-Y., and Wang, X.-M.: Seasonal variation of secondary organic aerosol tracers in Central Tibetan Plateau, Atmos. Chem. Phys., 15, 8781–8793, https://doi.org/10.5194/acp-15-8781-2015, 2015.
Shrestha, S. R., Oanh, N. T. K., Xu, Q. S., Rupakheti, M., and Lawrence, M. G.: Analysis of the vehicle fleet in the Kathmandu Valley for estimation of environment and climate co-benefits of technology intrusions, Atmos. Environ., 81, 579–590, https://doi.org/10.1016/j.atmosenv.2013.09.050, 2013.
Simoneit, B. R. T., Schauer, J. J., Nolte, C. G., Oros, D. R., Elias, V. O., Fraser, M. P., Rogge, W. F., and Cass, G. R.: Levoglucosan, a tracer for cellulose in biomass burning and atmospheric particles, Atmos. Environ., 33, 173–182, 1999.
Simoneit, B. R. T., Medeiros, P. M., and Didyk, B. M.: Combustion products of plastics as indicators for refuse burning in the atmosphere, Environ. Sci. Technol., 39, 6961–6970, https://doi.org/10.1021/es050767x, 2005.
Simpson, I. J., Akagi, S., Barletta, B., Blake, N., Choi, Y., Diskin, G., Fried, A., Fuelberg, H., Meinardi, S., and Rowland, F.: Boreal forest fire emissions in fresh Canadian smoke plumes: C1-C10 volatile organic compounds (VOCs), CO2, CO, NO2, NO, HCN and CH3CN, Atmos. Chem. Phys., 11, 6445–6463, https://doi.org/10.5194/acp-11-6445-2011, 2011.
Simpson, I. J., Aburizaiza, O. S., Siddique, A., Barletta, B., Blake, N. J., Gartner, A., Khwaja, H., Meinardi, S., Zeb, J., and Blake, D. R.: Air Quality in Mecca and Surrounding Holy Places in Saudi Arabia During Hajj: Initial Survey, Environ. Sci. Technol., 48, 8529–8537, https://doi.org/10.1021/es5017476, 2014.
Sinha, V., Kumar, V., and Sarkar, C.: Chemical composition of pre-monsoon air in the Indo-Gangetic Plain measured using a new air quality facility and PTR-MS: high surface ozone and strong influence of biomass burning, Atmos. Chem. Phys., 14, 5921–5941, https://doi.org/10.5194/acp-14-5921-2014, 2014.
Stockwell, C. E., Christian, T. J., Goetz, J. D., Jayarathne, T., Bhave, P. V., Praveen, P. S., Adhikari, S., Maharjan, R., DeCarlo, P. F., Stone, E. A., Saikawa, E., Blake, D. R., Simpson, I., Yokelson, R. J., and Panday, A. K.: Nepal Ambient Monitoring and Source Testing Experiment (NAMaSTE): Emissions of trace gases and light-absorbing carbon from wood and dung cooking fires, garbage and crop residue burning, brick kilns, and other sources, Atmos. Chem. Phys., 16, 11043–11081, https://doi.org/10.5194/acp-16-11043-2016, 2016.
Stone, E. A., Lough, G. C., Schauer, J. J., Praveen, P. S., Corrigan, C. E., and Ramanathan, V.: Understanding the origin of black carbon in the atmospheric brown cloud over the Indian Ocean, J. Geophys. Res., 112, D22S23, https://doi.org/10.1029/2006jd008118, 2007.
Stone, E. A., Schauer, J. J., Pradhan, B. B., Dangol, P. M., Habib, G., Venkataraman, C., and Ramanathan, V.: Characterization of emissions from South Asian biofuels and application to source apportionment of carbonaceous aerosol in the Himalayas, J. Geophys. Res., 115, D06301, https://doi.org/10.1029/2009JD011881, 2010.
Stone, E. A., Nguyen, T. T., Pradhan, B. B., and Dangol, P. M.: Assessment of biogenic secondary organic aerosol in the Himalayas, Environ. Chem., 9, 263–272, https://doi.org/10.1071/en12002, 2012.
Tsai, W. Y., Chan, L. Y., Blake, D. R., and Chu, K. W.: Vehicular fuel composition and atmospheric emissions in South China: Hong Kong, Macau, Guangzhou, and Zhuhai, Atmos. Chem. Phys., 6, 3281–3288, https://doi.org/10.5194/acp-6-3281-2006, 2006.
Turpin, B. J. and Lim, H.-J.: Species contributions to PM2.5 mass concentrations: Revisiting common assumptions for estimating organic mass, Aerosol Sci. Tech., 35, 602–610, https://doi.org/10.1080/02786820119445, 2001.
Wan, X., Kang, S. C., Rupakheti, M., Zhang, Q. G., Tripathee, L., Guo, J. M., Chen, P. F., Rupakheti, D., Panday, A. K., Lawrence, M. G., Kawamura, K., and Cong, Z. Y.: Molecular characterization of organic aerosols in the Kathmandu Valley, Nepal: insights into primary and secondary sources, Atmos. Chem. Phys., 19, 2725–2747, https://doi.org/10.5194/acp-19-2725-2019, 2019.
WHO: Mortality and burden of disease from ambient air pollution, World Health Organization, retrieved from: https://www.who.int/airpollution/ambient/en/ (last access: 2 April 2019), 2016.
Wiedinmyer, C., Yokelson, R. J., and Gullett, B. K.: Global emissions of trace gases, particulate matter, and hazardous air pollutants from open burning of domestic waste, Environ. Sci. Technol., 48, 9523–9530, https://doi.org/10.1021/es502250z, 2014.
Xiao, Y. P., Logan, J. A., Jacob, D. J., Hudman, R. C., Yantosca, R., and Blake, D. R.: Global budget of ethane and regional constraints on US sources, J. Geophys. Res., 113, D21306, https://doi.org/10.1029/2007jd009415, 2008.
Xu, L., Guo, H., Boyd, C. M., Klein, M., Bougiatioti, A., Cerully, K. M., Hite, J. R., Isaacman-VanWertz, G., Kreisberg, N. M., and Knote, C.: Effects of anthropogenic emissions on aerosol formation from isoprene and monoterpenes in the southeastern United States, P. Natl. Acad. Sci. USA, 112, 37–42, https://doi.org/10.1073/pnas.1417609112, 2015.
Yevich, R. and Logan, J. A.: An assessment of biofuel use and burning of agricultural waste in the developing world, Global Biogeochem. Cy., 17, 1095, https://doi.org/10.1029/2002GB001952, 2003.
Young, A. H., Keene, W. C., Pszenny, A. A., Sander, R., Thornton, J. A., Riedel, T. P., and Maben, J. R.: Phase partitioning of soluble trace gases with size-resolved aerosols in near-surface continental air over northern Colorado, USA, during winter, J. Geophys. Res., 118, 9414–9427, https://doi.org/10.1002/jgrd.50655, 2013.
Zhang, Y., Stedman, D. H., Bishop, G. A., Guenther, P. L., and Beaton, S. P.: Worldwide on-road vehicle exhaust emissions study by remote sensing, Environ. Sci. Technol., 29, 2286–2294, https://doi.org/10.1021/es00009a020, 1995.
Zhang, Y., Schauer, J. J., Zhang, Y., Zeng, L., Wei, Y., Liu, Y., and Shao, M.: Characteristics of particulate carbon emissions from real-world Chinese coal combustion, Environ. Sci. Technol., 42, 5068–5073, https://doi.org/10.1021/es7022576, 2008.
Zhong, M., Saikawa, E., Avramov, A., Chen, C., Sun, B., Ye, W., Keene, W. C., Yokelson, R. J., Jayarathne, T., Stone, E. A., Rupakheti, M., and Panday, A. K.: Nepal Ambient Monitoring and Source Testing Experiment (NAMaSTE): emissions of particulate matter and sulfur dioxide from vehicles and brick kilns and their impacts on air quality in the Kathmandu Valley, Nepal, Atmos. Chem. Phys., 19, 8209–8228, https://doi.org/10.5194/acp-19-8209-2019, 2019.