the Creative Commons Attribution 4.0 License.
the Creative Commons Attribution 4.0 License.
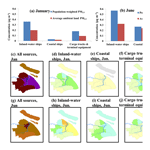
The influence of spatiality on shipping emissions, air quality and potential human exposure in the Yangtze River Delta/Shanghai, China
Junlan Feng
Yan Zhang
Shanshan Li
Jingbo Mao
Allison P. Patton
Yuyan Zhou
Weichun Ma
Cong Liu
Haidong Kan
Cheng Huang
Jingyu An
Yin Shen
Qingyan Fu
Xinning Wang
Juan Liu
Shuxiao Wang
Dian Ding
Jie Cheng
Wangqi Ge
Hong Zhu
Katherine Walker
The Yangtze River Delta (YRD) and the megacity of Shanghai are host to one of the busiest port clusters in the world; the region also suffers from high levels of air pollution. The goal of this study was to estimate the contributions of shipping to regional emissions, air quality, and population exposure and to characterize the importance of the geographic spatiality of shipping lanes and different types of ship-related sources for the baseline year of 2015, which was prior to the implementation of China's Domestic Emission Control Areas (DECAs) in 2016. The WRF-CMAQ model, which combines the Weather Research and Forecasting model (WRF) and the Community Multi-scale Air Quality (CMAQ) model, was used to simulate the influence of coastal and inland-water shipping, port emissions and ship-related cargo transport on air quality and on the population-weighted concentrations (which is a measure of human exposure). Our results showed that the impact of shipping on air quality in the YRD was primarily attributable to shipping emissions within 12 NM (nautical miles) of shore, but emissions coming from the coastal area between 24 and 96 NM still contributed substantially to ship-related PM2.5 concentrations in the YRD. The overall contribution of ships to the PM2.5 concentration in the YRD could reach 4.62 µg m−3 in summer when monsoon winds transport shipping emissions onshore. In Shanghai city, inland-water going ships were major contributors (40 %–80 %) to the shipping impact on urban air quality. Given the proximity of inland-water ships to the urban populations of Shanghai, the emissions of inland-water ships contributed more to population-weighted concentrations. These research results provide scientific evidence to inform policies for controlling future shipping emissions; in particular, in the YRD region, expanding the boundary of 12 NM from shore in China's current DECA policy to around 100 NM from shore would include most of shipping emissions affecting air pollutant exposure, and stricter fuel standards could be considered for the ships on inland rivers and other waterways close to residential regions.
- Article
(7071 KB) - Full-text XML
-
Supplement
(1361 KB) - BibTeX
- EndNote
With the increase in international maritime trade, shipping emissions and their impacts have attracted increased attention globally over the past decades (Capaldo et al., 1999; Cooper, 2003; Eyring et al., 2010; Sofiev et al., 2018). Shipping emits air pollutants that contribute to adverse impacts on climate, on air quality and on the health of people living near ports (Li et al., 2018; H. Liu et al., 2016). Globally, about 50 000 deaths in 2010 and about 90 000 deaths in 2012 due to cardiopulmonary diseases and lung cancer were attributed to exposure to particulate matter emitted from shipping (Corbett et al., 2007; Partanen et al., 2013; Winebrake et al., 2009), and 403 300 premature mortalities per year due to shipping are predicted in 2020 under business-as-usual assumptions (Sofiev et al., 2018). In Europe, ozone pollution caused by international ships led to around 3.6 % of the total estimated years of life lost and 2.6 % of premature deaths in 2005 (Campling et al., 2013). In East Asia, around 14 500 to 37 500 premature deaths per year have been primarily attributed to PM2.5 from shipping; about one-third of those deaths were in the area surrounding the East China Sea, with the largest impacts seen in mainland China (H. Liu et al., 2016).
As of 2016, China was home to 7 of the top 10 container ports, and the size of these ports has been rapidly growing to serve the increased trade via international shipping (UNCTAD, 2017). The Yangtze River Delta (YRD) is one of the economic centers and is also home to the busiest port cluster, which is comprised of more than 15 ports, including Shanghai Port, Ningbo–Zhoushan Port, Zhenjiang Port, Nantong Port, Lianyungang Port, Taizhou Port and Wenzhou Port. In 2016, the YRD generated a gross domestic product (GDP) of RMB 17.72 trillion (USD 2.76 trillion) – about 20 % of China's national GDP (Preen, 2018). Shanghai megacity itself is an important economic center, accounting for about 22 % of the total GDP in the YRD. Shanghai Port lies at the intersection of the East China Sea and the Yangtze River and has been the largest container port in the world since 2010 (Z. Liu et al., 2016).
Shanghai and the YRD are also among the most densely populated regions of China. The YRD is home to 239.1 million people; Shanghai is one of the largest cities and houses about 12.1 % of the total population of the YRD (Bright et al., 2016).
This region has suffered from severe air pollution over the past decade due to anthropogenic emissions from multiple sources. In December 2013, for example, the YRD experienced a haze episode, during which the maximum observed PM2.5 concentration in the region exceeded 590 µg m−3 (Sun et al., 2016). As severe air pollution episodes have continued and ports have grown, the shipping sector, a subset of the transportation pollution sources, has received more attention.
The high ship traffic density in Shanghai and the YRD has led to high emissions of ship-related air pollutants in this region (Fan et al., 2016). Ship-related sources of air pollution in Shanghai comprise coastal ships, inland-water ships, cargo trucks and port terminal equipment. Because some of these emissions sources are also close to densely populated areas, in particular those from ships traveling in inland waterways and from container trucks transporting cargo in and around the city, there is greater potential for higher population exposure to ship-related air pollution.
The International Maritime Organization (IMO) regulates emissions of marine pollution on a global scale. Current rules limit fuel sulfur content (FSC) to 3.5 % globally and this limit will be reduced to 0.5 % in 2020. The IMO has also designated several regional Emission Control Areas (ECAs) to benefit the atmospheric environment and human health in port and coastal communities that establish more stringent emission limits up to 200 NM from the coast in the Baltic Sea (SOX), the North Sea (SOX), North America (SOX, NOx and PM), and the US Caribbean Sea area (SOX, NOx and PM) (Viana et al., 2015). Fuel sulfur content is limited to 0.1 % in the ECAs.
China does not have an ECA designated by the IMO, but in December 2015 it designated three Domestic Emission Control Areas (DECAs) that operate in a similar manner. These DECAs limited fuel sulfur content to 0.5 % for ocean-going vessels (OGV) in three regions: the YRD, the Pearl River Delta (PRD) and the Bohai Sea. The DECA implementation timeline encouraged qualified ports to be in compliance from 1 April 2016, and specified that all ships at berth in 11 core ports within these regions would be in compliance by 1 January 2017 and that all ocean-going vessels (OGV) or coastal vessels within 12 NM of the shoreline would be in compliance by 1 January 2019. These areas would also be in compliance with the IMO requirements for fuel sulfur content. A study reported that the average reduction of PM2.5 and SO2 mass concentrations over land in the PRD due to the DECA policy were 2.7 % and 9.54 %, respectively (Liu et al., 2018a). China is currently considering additional DECA restrictions for the period beyond 2019. On 1 October 2018, 3 months earlier than originally planned, the Shanghai Maritime Safety Administration (MSA) enforced the DECA policy limiting the fuel sulfur content to 0.5 % for ocean-going vessels and domestic coastal vessels in Shanghai Port. However, the DECA policies for fuel sulfur content currently make no distinction between coastal ships that enter inland waterways and other ships. Ships such as those in Shanghai and the YRD that enter inland waterways bring emissions sources closer to population centers, resulting in a greater potential for exposure and health impacts.
Shipping emission inventories for the YRD, the PRD, and the Bohai Rim area and their major ports indicate that shipping is an important pollution source surrounding port regions (Chen et al., 2016; Fan et al., 2016; Li et al., 2016; Yau et al., 2012). Several studies have investigated the contribution of shipping emissions to the ambient air quality using different methods. Zhao et al. (2013) analyzed aerosol samples in Shanghai Port and reported that ship traffic contributed 0.63 to 3.58 µg m−3 (or 4.2 % to 12.8 %) of the total PM2.5 in the port. Primary ship-emitted particles measured by an aerosol time-of-flight mass spectrometer were typically 1.0 % to 10.0 % of the measured particle number concentration, with the contribution rising to as high as 50.0 % in spring and summer (Z. Liu et al., 2016). In Guangzhou and Zhuhai, shipping emissions were among the top contributors to PM2.5 and accounted for more than 17 % of PM2.5 mass concentrations (Tao et al., 2016). Using WRF-CMAQ, which combines the Weather Research and Forecasting model (WRF) and the Community Multi-scale Air Quality (CMAQ) model, Chen et al. (2017b) found that the contribution of shipping emissions to the PM2.5 mass concentrations in Qingdao was highest in summer (13.1 %) and lowest in winter (1.5 %). Chen et al. (2019) reported that ship traffic sources could contribute 4.0 % of the annual PM2.5 mass concentrations over land in YRD and that the maximum could reach up to 35.0 % in port regions in 2014.
In China, few studies have reported the contribution from shipping in different offshore coastal areas or different types of ship-related sources to air pollution. For example, Mao et al. (2017) estimated primary emissions from OGVs at different boundaries in the PRD region, and concluded that further expansion of the emission control area to 100 NM from shore would provide even greater benefits. However, the impacts of shipping emissions at varying distances from shore on air quality and potential human exposure, which are important when considering ECA policy, have not been rigorously studied. Mao and Rutherford (2018) studied NOx emissions from three categories of merchant vessels – OGVs, coastal vessels (CVs) and river vessels (RVs) in China's coastal region. However, less attention was paid to the impacts of inland waterway traffic and port-related sources, such as cargo trucks and terminal port equipment, on air quality and potential human exposure.
To fill this gap, the overall goal of this study was to characterize the spatial distribution of ship-related emissions and their impacts on air quality and human exposure in the YRD and Shanghai for the baseline year of 2015, which was prior to the implementation of China's DECAs in 2016. We modeled shipping emissions in different offshore areas in the YRD region and emissions from different types of ship-related sources in Shanghai city for each month of the year. To identify which offshore areas in the YRD region and which ship-related sources in Shanghai contributed the most to ambient air pollution, and human population exposure, we modeled the impacts of shipping emissions in different offshore areas (within 12 NM of the coast including inland waters and within 12–24, 24–48, 48–96 and 96–200 NM of the coast, respectively) in the YRD region as well as coastal ships, inland-water ships, and cargo trucks and port terminal equipment in and near the port areas under the jurisdiction of Shanghai MSA in 2 representative months (January and June). The results of this study could be informative regarding the consideration of the distance of regulated emissions in the design of future emissions control areas for shipping in the YRD, or regulations on the sulfur content of fuels for different types of ship-related sources in Shanghai.
In this study, we first established a shipping emission inventory based on highly resolved automatic identification system (AIS) data from 2015. We then used the WRF-CMAQ model to evaluate the impacts of shipping emissions in different offshore coastal areas (within 12 NM of the coast including inland waters and within 12–24, 24–48, 48–96 and 96–200 NM of the coast, respectively) on air quality in the YRD region. We referred to the ICCT's (International Council on Clean Transportation) working paper (Mao et al., 2017) to choose the distance bins between 12 NM (the boundary of current China's DECA) and 200 NM (the boundary of ECA designated by IMO) in this study. The model domains are shown in Fig. S1 in the Supplement. Simulations were also conducted to estimate the influence of different types of ship-related sources (coastal ships, inland-water ships, cargo trucks and port terminal equipment) on air quality in Shanghai. Finally, population-weighted PM2.5 concentrations attributable to shipping sources were calculated.
2.1 Study area and study period
Figure 1 shows the geographical area and population density for the YRD and Shanghai, the location of 16 core cities in the YRD region and 16 administrative districts within Shanghai city. The coastal cities in the YRD are Nantong, Shanghai, Jiaxing, Ningbo, Taichou and Zhoushan.
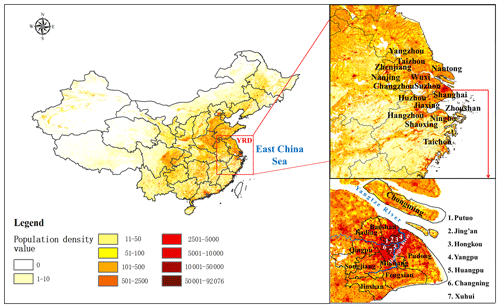
Figure 1Geographical location of the study area YRD/Shanghai including the population density in 2015. A total of 16 core cities in the YRD and 16 administrative districts in Shanghai are noted on the map. The smaller administrative districts are labeled using numbers: Putuo (1), Jing'an (2), Hongkou (3), Yangpu (4), Huangpu (5), Changning (6) and Xuhui (7).
The simulation network was developed for four domains at resolutions of 81 km × 81 km, 27 km × 27 km, 9 km × 9 km and 1 km × 1 km, respectively (Fig. S1). Domain 1 covers the whole of China. Nested domains 2, 3 and 4 cover a large part of eastern China (2), the YRD region (3; including Jiangsu, Zhejiang and Shanghai) and Shanghai at a finer resolution (4), respectively. The geographical scope of the YRD study area extended from 116.5 to 127∘ E and from 27 to 35∘ N and included an offshore distance of approximately 200 NM. The Shanghai study area ranged from 120.5 to 122.3∘ E and from 30.5 to 32∘ N, where the water is within the jurisdiction of the Shanghai MSA.
Two representative months in the year 2015, January and June, were selected to compare the seasonal effects. Higher shipping impacts were expected in summer, taking June as a representative month, because prevailing winds from the summer monsoon are directed from the ocean to the shore. January was chosen as a contrasting period with prevailing winds moving away from shore.
2.2 Emission inventories
2.2.1 Ship-related emission inventories
In this study, emission inventories were constructed based primarily on automatic identification system (AIS) data for shipping traffic activity in China, the YRD and Shanghai geographic domains. Due to the limitation of the national-scale data source, the AIS data in this study only covered the representative months of January and June 2015, whereas the YRD-scale AIS data covered the whole of 2015. AIS data include international ships, coastal ships and inland-water ships, although some river ships could be not covered by AIS data. Emissions from ships entering the geographic domains of the YRD or Shanghai were calculated using the AIS-based model developed by Fan et al. (2016), and monthly shipping emissions for January and June were used in the air quality model to capture the seasonal variation; this was expected to be more accurate than annual shipping emissions with no monthly variations. For Shanghai, estimates of emissions from ships without AIS devices were supplemented by using 2015 vessel call data provided by the Shanghai MSA and the Shanghai Municipal MSA. The detailed method, assumptions and sources are provided in Sect. S1 in the Supplement. The actual speeds and operation times of the ships involved in the calculation can be obtained from AIS data with high accuracy, whereas the installed power of the main engine (ME), auxiliary engine (AE), and auxiliary boiler (AB) and the maximum speed of ships necessary to complete the estimates were obtained from Lloyd's register (now IHS-Fairplay) (Lloyds, 2015) and the China Classification Society (CCS) database. Assumptions regarding the fuel types, sulfur contents, engine types, sources of emission factors, low-load adjustment multipliers and control factors are provided in Sect. S2 in the Supplement.
Within the Shanghai Port domain, separate emissions inventories were developed to estimate the relative air quality impacts of coastal and inland-water ships and of ship-related cargo trucks transport and port terminal equipment (cranes, forklifts and trucks used for internal transport). Many coastal ships operate in both the outer port and the inner river region of Shanghai Port, which includes the Yangtze River, the Huangpu River and other rivers in Shanghai. Consequently, a geographic boundary was used to divide the shipping emission inventory into coastal and inland sources based on AIS data (see Fig. 3c, where the black line denotes a division between coastal and inland shipping contributions to emissions).
Emissions from cargo trucks were estimated using International Vehicle Emission (IVE) model (Wang et al., 2008). The vehicular activity data were provided by the Shanghai Traffic Department. The emissions from port terminal equipment including the trucks in port were calculated based on the fuel consumption for each part of the port. Given their smaller emissions relative to shipping and other non-port sources, emissions from cargo trucks and terminal equipment were combined and gridded at a resolution of 1 km × 1 km.
2.2.2 Non-shipping emission inventories
National and local YRD emission inventories were used for emissions from all other sources (non-shipping). For the national-scale domain, we used a 2015 national emission database at a 27 km × 27 km resolution that included five pollutants (PM10, PM2.5, SO2, NOx and VOCs) and 14 source types (see Table S1 in the Supplement for details) (Zhao et al., 2018). As the national emission inventory database lacked data on CO and NH3 emissions, which are compulsory inputs for the CMAQ model, supplemental emission data on these pollutants in 2015 were obtained from the International Institute for Applied Systems Analysis (IIASA) database (at a resolution) (Stohl et al., 2015). In case of large uncertainty caused by merging data from two datasets, the ratio of CO to VOCs was checked in this study. CO and VOC emissions both result from the incomplete combustion of fuel and are likely to be related (von Schneidemesser et al., 2010; Wang et al., 2014). The ratio of CO to VOCs was 7.7 in the IIASA inventory and 7.5 in the final combined inventory. Thus, the CO/VOC shares in these two inventories were very close, and the use of the final combined inventory is acceptable. The local YRD land-based emission inventory was generated at a 4 km × 4 km resolution; it included eight source types and seven pollutants (PM10, PM2.5, SO2, NOx, CO, VOCs and NH3). Details are provided in Table S2. National and local emission data were allocated to simulation grids by spatial interpolation in ArcGIS 10.2 (ESRI, 2013).
2.3 WRF-CMAQ model setup
The models used in this study were the Weather Research and Forecasting Model (WRF) version 3.3 and the Community Multiscale Air Quality (CMAQ) model version 4.6. The selected simulation periods were 1 to 31 January and 1 to 28 June, with 72 h of spin-up time for each run. The initial and boundary conditions for meteorology were generated from the National Centers for Environmental Prediction (NCEP) Final Analysis (FNL) (NCEP, 2000) with a resolution of at 6-hour time intervals. Vertically, 27 sigma layers were set for the WRF simulation, and the results were then converted to the 24 layers required by CMAQ (version 4.6) using the MICP (Meteorology–Chemistry Interface Processor). CMAQ was configured to use the Carbon Bond mechanism (CB05) for gas-phase chemistry and the AERO4 aerosol module (Z. Liu et al., 2016).
2.4 Simulations of source contributions to air quality
Individual source contributions to gridded ambient concentrations of air pollution were estimated as the difference between the concentrations simulated with all sources included and those with the individual source excluded. For the YRD region (domain 3), the simulation was conducted for ships within different boundaries from shore (12, 12–24, 24–48, 48–96 and 96–200 NM, respectively). For the city of Shanghai, simulations were conducted for all ship-related sources in the water area under the jurisdiction of the Shanghai MSA (within approximately 12 NM of shore), coastal and inland-water shipping (as defined geographically above), and cargo transport and port terminal equipment (combined). Details of each simulation can be found in Table S3.
2.5 Model evaluation
Performance of the models was spatially evaluated by comparison with monthly average observations at monitoring stations (Fig. S2). Generally, the simulated results showed trends consistent with the observations, with increased concentrations of SO2 and PM2.5 along the Yangtze River and in the urban areas. Furthermore, daily average observations from 53 monitoring stations in 16 core YRD cities were compared with daily average simulated ambient SO2 and PM2.5 concentrations. Normalized mean bias (NMB), normalized mean error (NME), root mean-square error (RMSE) and the Pearson correlation coefficient (r) were used to qualify the degree of deviation between the observed data and the modeling results (Eder and Yu, 2007). Detail equations of the above statistical metrics are shown in Sect. S3. For each of the cities, the statistical metrics were calculated based on the average observed data and the simulated results of the monitoring stations in the city, as shown in Table 1. For most cities, SO2 and PM2.5 concentrations were underestimated to varying degrees, and the NMB was in the range of −36 % to −18 % and −34 % to 8 %, respectively. The deviations between the simulation results and the monitoring data were mainly due to the uncertainties of emission inventories and some deficiencies of meteorological and air quality models. However, there were also uncertainties associated with the measurements themselves and the comparison of grid-based predictions to measurements at point locations. The daily variability of simulated and observed SO2 and PM2.5 concentrations in four representative cities (two coastal cities and two inland cites) is displayed in Fig. S3, which indicates that the temporal variability of the simulated data was consistent with the observed data, and the air quality model could capture the pollution peak most of the time.
Table 1Statistical metrics of the model evaluation. Observed data (Obs.) and simulated data (Sim.) for each city are the average of monthly values of January and June case. NMB (normalized mean bias), NME (normalized mean error), RMSE (root mean-square error) and r (the Pearson correlation coefficient) were calculated based on the daily average observed and simulated data.
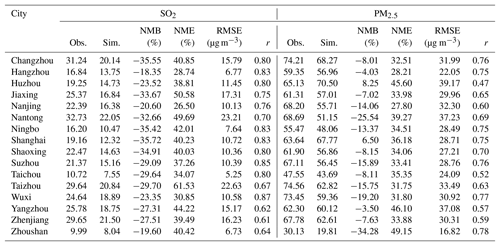
2.6 Population-weighted PM2.5 concentration
We estimated population-weighted PM2.5 concentrations for the 16 cities in the YRD region and the 16 districts within Shanghai city. The population-weighted PM2.5 concentration of the given grid cell i was calculated based on Eq. (1) (Prasannavenkatesh et al., 2015):
where PMi is defined as the PM2.5 concentration in the ith grid cell, Pi is the population in the ith grid value of P and n is the number of grid cells in the selected geographical area, for example, a city or region.
Soares et al. (2014) built a refined model for evaluating population exposure to ambient air pollution in different microenvironments. In this study, in the absence of detailed individual exposure estimates, population-weighted PM2.5 concentrations are a better approximation of potential human exposure because they give proportionately greater weight to concentrations in areas where more people live. Population-weighted exposures have been adopted as the basis for estimating the burden of disease from air pollution in the Global Burden of Disease project run by the Institute for Health Metrics and Evaluation (IHME; Cohen et al., 2017). IHME's exposure methodology is also now used by the World Health Organization.
3.1 Characteristics of shipping emissions
We estimated 7.2×105 t of annual SO2 emissions from ships in China in 2015 taking January and June as the 2 reference months (see Sect. 2.2.1 for a description of the data and Fig. 2a for the spatial pattern). Below, we discuss the quantity and other characteristics of primary emissions from ships in different offshore coastal areas in YRD regions (Sect. 3.1.1) and from different ship-related sources in Shanghai (Sect. 3.1.2).
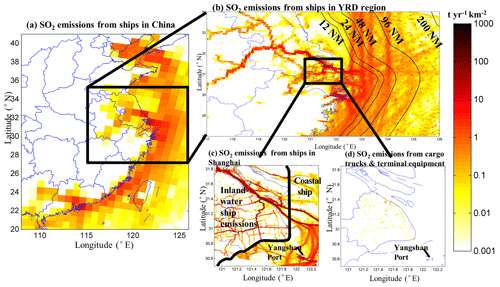
Figure 2SO2 emissions in 2015 from (a) shipping traffic in China (the average value of January and June) at a resolution of 81 km × 81 km; (b) ships in different offshore coastal areas (inland-water and within 12, 12–24, 24–48, 48–96 and 96–200 NM of the coast) in the YRD region, at a resolution of 9 km × 9 km; (c) inland-water ships and coastal ships in Shanghai, at a resolution of 1 km × 1 km; and (d) cargo trucks and port terminal equipment in Shanghai, at a resolution of 1 km × 1 km. The black line in (c) refers to the division between the inland water and coastal water for the megacity of Shanghai defined in this study.
3.1.1 Shipping emissions in the YRD region
Based on the 2015 AIS data (the whole year), the annual emissions of SO2, NOx, PM2.5 and VOCs from shipping sectors in YRD region were estimated at 2.2×105 t, 4.7×105 t, 2.7×104 t and 1.2×104 t, respectively, which accounted for 7.4 %, 11.7 %, 1.3 % and 0.3 % of the total emissions from all sources in the YRD in 2015, respectively. The emission estimates of SO2 and NOx were close to the estimates of Fu et al. (2017) for 2013, but estimates of SO2, NOx and PM2.5 were slightly lower than those of Chen et al. (2019) for 2014 due to the different temporal or spatial statistical scope. However, the proportion of ship SO2 emissions in the YRD region with respect to the total Chinese shipping emissions in this study is consistent with the 33 % to 37 % reported in the other studies (Chen et al., 2017a, 2019; Liu et al., 2018b; Lv et al., 2018).
Table 2Primary emissions (t yr−1), emission share of total shipping emissions (%) and emission density (t yr km−2) from shipping at different boundaries in the YRD region (domain 3) in 2015.
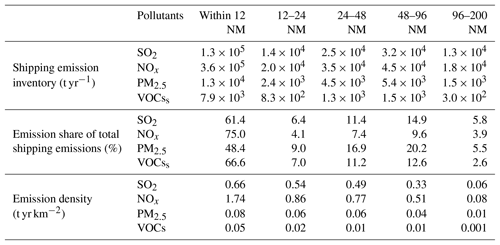
More than 60 % of the annual emissions of SO2 from ships in the YRD occurred inland or within 12 NM of shore, where 75.0 % of the NOx emissions and 48.4 % of the PM2.5 emissions from ships also occurred (Table 2). Similar results regarding the offshore distribution of shipping emissions were obtained in other studies (C. Li et al., 2018; Liu et al., 2018a). Compared with the estimates in the PRD reported by Liu et al. (2018a), our estimate of 1.3×105 t of annual SO2 emitted by ships on inland waters and within 12 NM of shore was 47 % higher than the result in the PRD (8.83×104 t), whereas our estimate of the average emission intensity of SO2 within 12 NM of shore in the YRD was 0.66 t yr km−2, which was much lower than that of the PRD (4.04 t yr km−2). One explanation for these different results may be that the YRD has a longer coastline than the PRD which leads to larger total emissions but to lower intensity. Emissions occurring within 24–48 and 48–96 NM from shore were not negligible; annual SO2 emissions in these two areas accounted for 11.4 % and 14.9 % of the total shipping emissions in the YRD, respectively. The spatial pattern of annual SO2 emissions from ships varied in different offshore coastal areas in the YRD (Fig. 2b). SO2 emissions were also high at the intersection of the Yangtze River and the Huangpu River, between 24 and 48 NM from shore and in the north–south shipping lanes between 48 and 96 NM from shore.
3.1.2 Emissions from different ship-related sources in Shanghai
The annual emissions of SO2, NOx, PM2.5 and VOCs from all ship-related sources within the administrative water area of Shanghai in 2015 were 4.9×104, 1.4×105, 6.5×103 and 4.7×103 t, respectively. The breakdown of emissions from ship-related sources in Shanghai are shown in Table 3. The emissions of SO2, NOx, PM2.5 and VOCs from inland-water ships and coastal ships accounted for the majority of primary emissions from all ship-related sources in Shanghai Port, ranging from 72 % for VOCs to about 99 % for SO2. They comprised about 17.4 % of SO2, 24.5 % of NOx, 5.2 % of PM2.5 and 0.6 % of VOC emissions from all pollution sources in Shanghai. The shipping emissions in Shanghai Port were estimated to account for 23 % of SO2, 26 % of NOx, 23 % of PM2.5 and 28 % of VOCs from total shipping emissions in the YRD.
Table 3Primary emissions (t yr−1) and the emission share of all pollution sources (%) for different types of ship-related sources in Shanghaia in 2015.
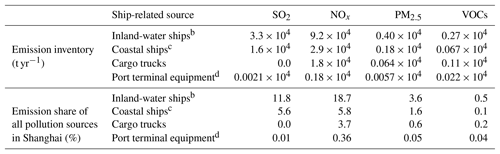
a Domain 4. b Defined as ships operating in both the outer port and in the inner river region of Shanghai Port, which includes the Yangtze River, the Huangpu River and other waterways in Shanghai. c Includes Chinese coastal and international ships. d Includes cranes and forklifts used for internal transport.
Emission estimates from this study fall within the range of estimates from other studies (e.g., Fu et al., 2012, 2017). On the basis of shipping visa data, Fu et al. (2012) determined that the total amounts of SO2, NOx and PM2.5 in the vicinity of Shanghai Port in 2010 were 3.5×104, 4.7×104 and 3.7×103 t yr−1, respectively, which is much lower than estimates in this study. Using AIS data, Fu et al. (2017) reported 5×104 t of SO2 and 7×104 t of NOx from shipping in Shanghai Port in 2013, which is close to or a bit lower than the results in this study.
Within Shanghai, following the geographical division, inland-water ships were the most important ship-related source of emissions, accounting for 67 % of SO2, 66 % of NOx, 62 % of PM2.5 and 57 % of VOC emissions from all ship-related sources in Shanghai (Table 2). Emissions of SO2, NOx, PM2.5 and VOCs from cargo trucks and port terminal equipment comprised a smaller percentage of emissions from all ship-related sources and particularly from all pollution sources; therefore, they were combined into one category in model simulation.
The spatial patterns of annual emissions from ship-related sources in Shanghai are shown using SO2 as an example in Fig. 2c and d. SO2 emissions from coastal ships were more prominent in the east–west shipping lanes and in the vicinity of Yangshan Port (Fig. 2c), whereas SO2 emissions from inland water-going ships were significantly concentrated along the Yangtze River and the Huangpu River, which run through the center of Shanghai.
3.2 The impact of shipping emissions on air quality
3.2.1 Contribution to ambient concentrations of SO2 and PM2.5 from all ships in the YRD
On average, ships contributed 0.55 µg m−3 in January (Fig. 3a) and 0.73 µg m−3 in June (Fig. 3c) to the land ambient SO2. The contribution of shipping emissions to the ambient monthly average SO2 concentration was higher in June 2015 than in January 2015 in the YRD region. The contribution from ships to the land ambient SO2 concentration peaked at 6.0 µg m−3 (24.3 % of ambient SO2) in January and 8.84 µg m−3 (69.7 % of ambient SO2 from all pollution sources) in June.
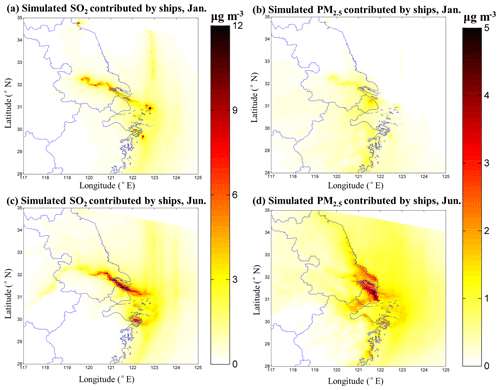
Figure 3Simulated SO2 (a, c) and PM2.5 (b, d) concentrations contributed by shipping traffic sources in the YRD region, in January 2015 (a, b) and June 2015 (c, d).
On average, ships contributed 0.36 µg m−3 in January (Fig. 3b) and 0.75 µg m−3 in June (Fig. 3d) to the ambient PM2.5 concentrations across the YRD. Similarly, the contribution of shipping emissions to ambient monthly average PM2.5 concentrations was higher in June 2015 than in January 2015 in the YRD region. The contribution from ships to the ambient PM2.5 concentration peaked at 1.84 µg m−3 (2.2 % of the total ambient PM2.5 concentration from all pollution sources) in January and 4.62 µg m−3 (18.9 % of total ambient PM2.5) in June. The highest shipping contributions to PM2.5 were located near the Shanghai Port.
The differences between the January and June contributions of shipping to air quality mainly reflect differences in meteorology. The summer monsoon winds flow from the sea toward land in June, transporting shipping emissions inland during this time, whereas the winter monsoon winds in January transport shipping emissions out to sea. Differences in shipping emissions did not explain the different results for January and June. Monthly shipping emissions in the YRD were 1.9×104 t of SO2 and 2.3×103 t of PM2.5 in January and 1.8×104 t of SO2 and 2.3×103 t of PM2.5 in June.
3.2.2 The influence of different offshore coastal areas in the YRD on air quality
Shipping emissions from inland waters and within 12 NM of shore accounted for 30 %–85 % of the total air quality impacts of ships within 200 NM of shore in January and June 2015 (Fig. 4). These results are similar to those of Lv et al. (2018), who reported that shipping emissions within 12 NM of shore contributed 30 % to 90 % of the PM2.5 induced by emissions within 200 NM. On average, ships contributed 0.24 µg m−3 to the ambient PM2.5 in January (Fig. 4a) and 0.56 µg m−3 to ambient PM2.5 concentrations in June (Fig. 4f). Peak contributions were 1.62 µg m−3 PM2.5 in January and 4.02 µg m−3 PM2.5 in June, respectively.
The average and peak contributions from the shipping emissions in specific offshore coastal areas to the ambient SO2 and PM2.5 concentrations on shore for the 2 months are listed in Table S4. Shipping emissions beyond 12 NM had a much smaller impact on the ambient SO2, with average contributions below 0.01 µg m−3 and peak contributions below 0.06 µg m−3 (Table S4).
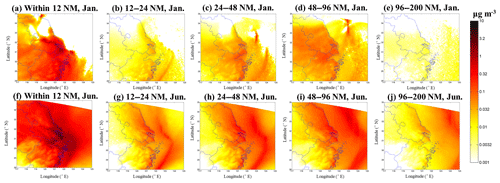
Figure 4Contributions to PM2.5 concentrations from shipping emissions at distances within 12 NM of shore (including inland-waters) (a, f), 12 to 24 NM from shore (b, g), 24 to 48 NM from shore (c, h), 48 to 96 NM from shore (d, i) and 96 to 200 NM from shore in January 2015 (a–e) and in June of 2015 (f–j).
Shipping emissions at distances of 12–24, 24–48 and 48–96 NM from shore contributed 0.01–0.07 µg m−3 to the ambient PM2.5 concentrations on average. Peak contributions of shipping emissions from areas beyond 12 NM ranged from 0.05 µg m−3 (12–24 NM) to 0.14 µg m−3 (48–96 NM) in January (Fig. 4b–d); the peak influence was higher in June and ranged from 0.2 µg m−3 (12–24 NM) to 0.34 µg m−3 (24–48 NM) (Fig. 4g–i). In the YRD region, shipping emissions on inland waters or within 12 NM of shore had larger contributions to ambient PM2.5 than more distant ships did, but the busy north–south shipping lanes far from shore also impacted ambient PM2.5 concentrations. Shipping emissions from 96 to 200 NM from shore had little impact on the air quality over land and contributed less than 0.05 µg m−3 (or 3 % of the ship-related contribution) to the ambient land PM2.5 (Fig. 4e, i).
The cumulative contributions to the ambient SO2 concentrations in the 16 core YRD cities from ships at different distances from shore in January and June 2015 differed from the PM2.5 results (Fig. 5). In both January (Fig. 5a) and June (Fig. 5c), shipping emissions within 12 NM of shore accounted for at least 78 % of the ship-related contribution to the ambient SO2 concentrations in these cities. Shipping emissions beyond 12 NM from shore had a limited contribution to theSO2 concentrations in 16 core YRD cities, implying that the boundary of 12 NM from the coast might be suitable for regulating SO2 emissions. This is also supported by the results of Schembari et al. (2012), who reported that statistically significant reductions in SO2 levels (66 % to 75 %) were found in three out of the four European harbors 5 months after the implementation of the EU directive 2005/33/EC that required all ships at berth or anchorage in European harbors to use fuels with a sulfur content of less than 0.1 % (implemented in January 2010). The quicker chemical reaction and shorter lifetime of SO2 may explain why ships further out to sea than 12 NM had a much smaller impact on land ambient SO2 concentrations (Collins et al., 2009; Krotkov et al., 2016). SO2 reacts under tropospheric conditions via both gas-phase processes (with OH) and aqueous-phase processes (with O3 or H2O2) to form sulfate aerosols, and is also physically removed via dry and wet deposition (Seinfeld and Pandis, 2006). The sulfur deposition due to shipping emissions is mainly contributed by the dry depositions (Chen et al., 2019). In the planetary boundary layer (PBL), SO2 has a short lifetime (less than 1 d during the warm season) and is concentrated near its emission sources (Krotkov et al., 2016).
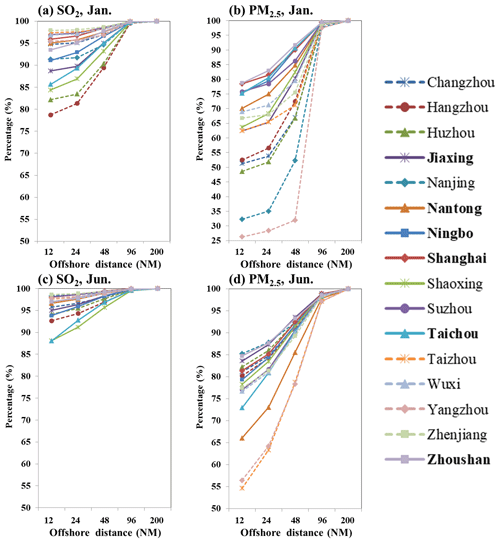
Figure 5Cumulative contributions of shipping emissions in the YRD at distances within 12 NM of shore (including inland-waters), 24 NM from shore, 48 NM from shore, 96 NM from shore, and 200 NM from shore to PM2.5 concentrations (a, c) and SO2 concentrations (b, d) in January 2015 (a, b) and in June 2015 (c, d). The names of coastal cities are in bold in the legend.
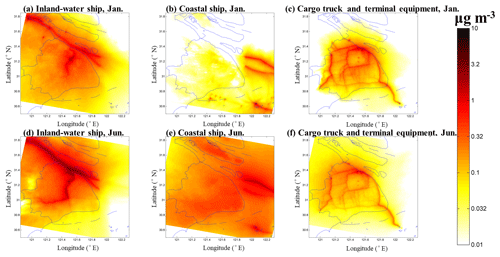
Figure 6Contributions to PM2.5 concentrations from inland-water ships (a, d), coastal ships (b, e), and cargo trucks and port terminal equipment (c, f) in January 2015 (a–c) and June 2015 (d–f).
In contrast to SO2, the cumulative contributions to PM2.5 in the 16 core YRD cities from ships at different distances from shore showed greater differences in January and June 2015. In January, the relative contributions of ships inland or within 12 NM of shore to ship-related PM2.5 concentrations ranged from 78.7 % in Zhoushan, which was mostly influenced by the closest shipping emissions, to 26.3 % in Yangzhou (Fig. 5b). In June, the relative contributions of ships inland or within 12 NM of shore to all PM2.5 emissions from ships ranged from 85.2 % in Nanjing to 54.6 % in Taizhou (Fig. 5d). Therefore, in both months, shipping emissions within 12 NM of shore were a major contributor to ship-related PM2.5 concentrations in most of the core YRD cities. Although busy north–south shipping lanes 24–96 NM from shore contributed little to SO2 concentrations to YRD cities, shipping emissions from this area contributed 12 % to 39 % of ship-related PM2.5 concentrations in YRD cities. Of PM2.5 in YRD cities contributed by ships within 200 NM of shore, 97 % is accounted for by shipping emissions within 96 NM of shore. The results of these YRD analyses suggest that although ambient ship-related SO2 concentrations were mainly affected by inland shipping or shipping within 12 NM of shore, expanding China's current DECA to around 100 NM or more from the coast would reduce the majority of the impacts of shipping on regional PM2.5 pollution. It also implies that future ECA policy should consider multiple air pollutants including the primary and secondary pollutants synchronically.
3.2.3 The influence of different ship-related sources in Shanghai Port on air quality
The impact of port-scale ship-related sources on the air quality in Shanghai was significant, and the dominant sources of ship-related emissions (i.e., coastal ships, inland-water ships and other ship-related sources) varied depending on the season and their location relative to cities (Fig. 6). Inland-water ships had a larger influence on areas within Shanghai near the Yangtze River and the Huangpu River. Inland-water ships contributed 0.24 µg m−3 in January (Fig. 6a) and 0.37 µg m−3 in June (Fig. 6d) to ambient PM2.5 on average, and accounted for 40 % to 80 % of all PM2.5 from ship-related sources. The inland-water ships had a large influence in areas near the cross section of the Yangtze River and the Huangpu River, where their contributions to ambient PM2.5 peaked at 1.87 µg m−3 in January and 2.67 µg m−3 in June (Fig. 6a, d). Coastal ships contributed 0.02 µg m−3 in January and 0.30 µg m−3 in June to ambient land PM2.5 concentrations on average. Peak contributions of coastal ships to ambient PM2.5 were 0.1 µg m−3 in January (Fig. 6b) and 0.71 µg m−3 in June (Fig. 6e). The impact of coastal ships was much smaller in January than in June due to the previously described meteorological reasons. Cargo trucks and port terminal equipment contributed 0.15 µg m−3 in January (Fig. 6c) and 0.12 µg m−3 in June (Fig. 6f) to ambient PM2.5 concentrations on average, and accounted for 10 to 45 % of PM2.5 from ship-related sources. Peak contributions of cargo trucks and port terminal equipment were 2.14 µg m−3 in January and 1.40 µg m−3 in June. The slightly larger contribution of cargo trucks and terminal equipment to PM2.5 concentrations was mainly due to the lower wind speed in winter which hindered the dispersion of pollutants. Although the contributions of cargo trucks and port terminal equipment to ambient PM2.5 were generally lower than the contributions of ships, these other ship-related sources were still important in both winter and summer due to their impact on air quality near the Shanghai city center.
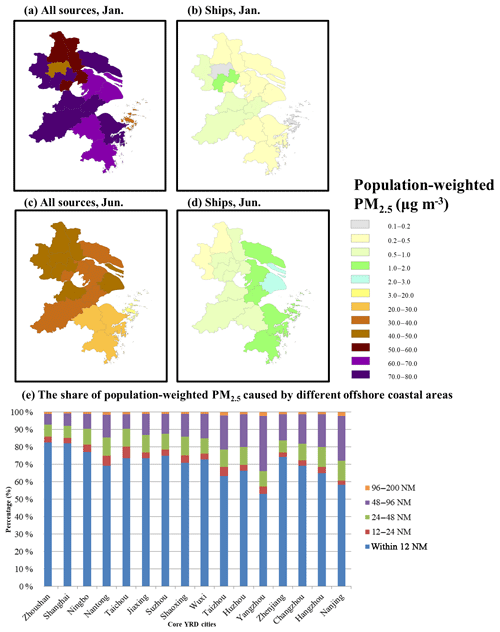
Figure 7The spatial distribution of population-weighted PM2.5 in 16 YRD cities caused by all pollution sources (a, c) and by all ships (b, d) in January 2015 (a, b) and June 2015 (c, d); the average share of population-weighted PM2.5 in 16 YRD cities caused by different offshore coastal areas in all ships (e). The cities' names are ordered by their distance from the coast.
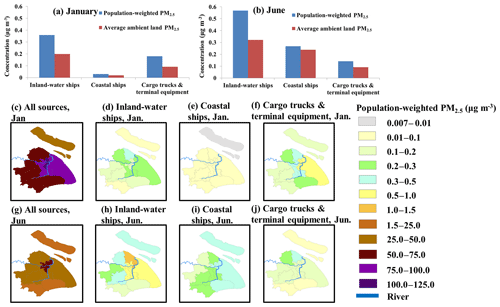
Figure 8Population-weighted PM2.5 and average PM2.5 caused by different ship-related sources in Shanghai, in January (a) and in June (b); population-weighted PM2.5 caused by all pollution sources (c, g), inland-water ships (d, h), coastal ships (e, i), and cargo trucks and port terminal equipment (f, j) in 16 districts in Shanghai, in January 2015 (c–f) and June 2015 (g–j).
3.3 Population-weighted PM2.5 concentrations
3.3.1 Influence of different offshore coastal areas in YRD on population-weighted PM2.5
Population-weighted PM2.5 concentrations in the YRD from shipping sources were larger in June (0.4 to 2.6 µg m−3 in June; Fig. 7d) than in January (0.1 to 1.2 µg m−3; Fig. 7b). This is in contrast to population-weighted PM2.5 concentrations from all pollution sources, which were higher in January (33.1 to 80.2 µg m−3; Fig. 7a) than in June (9.5 to 48.4 µg m−3; Fig. 7c). Thus, population-weighted PM2.5 concentrations from shipping sources accounted for 0.9 % to 15.5 % of the population-weighted PM2.5 concentrations from all pollution sources in June, which was larger than the contributions of 0.2 % to 1.6 % in January; this was attributed to higher ship-related population-weighted PM2.5 concentrations in June and higher population-weighted PM2.5 concentrations from all pollution sources in January. Of the 16 core YRD cities, the highest ship-related population-weighted PM2.5 concentrations were found for Shanghai in June (2.6 µg m−3), and were 1.5 times higher than the second-highest city of Nantong (1.7 µg m−3). The six cities in the YRD with the largest contributions of PM2.5 from shipping sources were all coastal cities, which suggests, as expected, that people living in coastal regions suffer higher exposure to air pollution from ship-related sources than people living in farther inland, especially during the summer monsoon period.
Taking the population-weighted PM2.5 concentrations from all shipping sources within 200 NM of the coast as the base, shipping (both in inland waters and within 12 NM of shore) was a major contributor to population-weighted PM2.5 concentrations in 16 YRD cities; it accounted for 52.9 % to 82.7 % of the population-weighted PM2.5 concentrations (Fig. 7e). The population-weighted PM2.5 concentrations from shipping within 12–24 NM from shore were much smaller, accounting for 2.5 % to 6.6 %. However, shipping emissions in the area from 24 to 48 NM from shore accounted for 6.8 % to 11.5 %, and ships from 48 to 96 NM from shore accounted for 6.3 % to 31.6 %. These shipping contributions from greater distances from the coast were larger than the contribution from ships 12–24 NM from shore; this was probably due to the fact that the busier shipping lanes are located in more remote areas, further from shore, such as 24–48 NM from the coast. Therefore, although shipping inland and within 12 NM of the shore was the dominant contributor to potential population exposure to PM2.5, ships as far as 24–96 NM from the coast could also be important.
3.3.2 The influence of different ship-related sources in Shanghai Port on potential exposure
Of the ship-related sources in Shanghai, inland-water ships were the largest contributors to both PM2.5 and population-weighted PM2.5 (Fig. 8b). The population-weighted PM2.5 in January was 0.38 µg m−3 from inland-water ships (Fig. 8a). In June, the population-weighted PM2.5 contribution from inland-water ships reached 0.57 µg m−3, as the region near the Huangpu River and the Yangtze River had a high population where inland-water ships contributed high levels of PM2.5 (Fig. 8b). In contrast, coastal ships contributed 0.27 µg m−3 and cargo trucks and port terminal equipment contributed only 0.14 µg m−3 to population-weighted PM2.5 in June. Population-weighted PM2.5 from shipping sectors in January were lower than those in June, whereas the population-weighted PM2.5 from cargo trucks and port terminal equipment was slightly higher. In both June and January, population-weighted PM2.5 concentrations from ship-related sources were larger than the average PM2.5 concentrations from ship-related sources because the population was denser in the areas most highly influenced by ship-related sources (Fig. 8a, b). The difference between the average PM2.5 concentration and the population-weighted PM2.5 concentration was largest for inland-water ships, which contributed 2 times more to the population-weighted PM2.5 concentration than to the average PM2.5 concentration.
Population-weighted PM2.5 concentrations were not evenly distributed among the 16 administrative districts in Shanghai. The population-weighted PM2.5 from all pollution sources ranged from 44.8 to 124.5 µg m−3 in January (Fig. 8c) and 23.4 to 67.2 µg m−3 in June (Fig. 8g). Heavy motor vehicle traffic probably contributed to higher population-weighted PM2.5 in the city center (Huangpu, Jing'an and Hongkou).
Areas in the city center had high population-weighted PM2.5 from inland-water ships because of the combination of dense population and location – close to Huangpu River (Fig. 8d, h). Among them, Baoshan and Yangpu had the highest population-weighted PM2.5 concentrations from inland-water ships (both around 1.31 µg m−3) in June. Moreover, in June, the population-weighted PM2.5 from coastal ships ranged from 0.17 to 0.40 µg m−3, and the coastal district (Fengxian) suffered the largest impacts. Transport of emissions by the summer monsoon caused impacts on the population-weighted PM2.5 not only in coastal districts but also in the highly populated city center. As for population-weighted PM2.5 caused by cargo trucks and port terminal equipment, Baoshan had the highest population-weighted PM2.5 in both January (0.4 µg m−3) and June (0.45 µg m−3) due to its high population and location close to the source (Fig. 8f, j). The results of the analyses of different types of ship-related sources indicated that ship-related sources close to densely populated areas substantially contribute to population exposure to air pollution.
3.4 Limitations and uncertainties
Limitations in the study were mainly related to missing some information in the database and assumptions made during the estimation of shipping emissions. When estimating the shipping emission inventory, underestimations of actual emissions may be introduced by missing information. For example, AIS data have a high coverage of coastal vessels, but many inland vessels are not equipped with AIS. Therefore, emissions from inland vessels without AIS devices were supplemented using 2015 vessel call data provided by the Shanghai MSA and the Shanghai Municipal MSA; this could introduce some uncertainties for inland river vessels. Furthermore, emissions from fishing boats were probably underestimated because AIS devices on some fishing boats may not be in use. Similarly, limited information exists on auxiliary boilers in the Lloyd's register and the CCS databases, so we calculated the main engine and auxiliary engine emissions but did not consider auxiliary boiler emissions in this study, which may cause an underestimation of shipping emissions.
In addition, we did not consider the external effects of water flow, wind and waves when calculating engine power for ships traveling through the region. This would introduce some uncertainties (Aulinger et al., 2016). According to previous studies undertaken in other areas, these factors may increase the fuel consumption of individual vessels by as much as 10 % to 20 %, whereas the effects of waves on emission estimations over extensive geographical regions are negligible (Jalkanen et al., 2009, 2012). The downstream area of the Yangtze River is located in a plateau region, where the river flow is below 0.5 m s−1 (Song and Tian, 1997; Xue et al., 2004). For Shanghai, which located at the end of the mouth of the Yangtze River to the East China Sea, an area with flat terrain, the river flow is very slow. Given that ships traveling the Yangtze River near Shanghai have speeds over ground (SOG) of about 5–10 knots (3–5 m s−1), the relative ratio of the water flow to the SOG is within 20 %. In our future work, we will fill the gap in the basic ship data and consider the external effects when building the shipping emission inventory. Finally, this work only extends from emissions to air quality and population exposures. The health impacts of ship-related air pollution in Shanghai and the YRD region will be explored in future work.
As the major economic and shipping center in China, the YRD, and in particular Shanghai, experiences high emissions of ship-related pollutants that result in significant contributions to ambient and population-weighted air pollutant concentrations. Our results showed that on average ships contributed 0.75 µg m−3 to the ambient land PM2.5 in YRD in 2015, with a peak of 4.62 µg m−3 (18.9 % of the total ambient PM2.5 concentration from all pollution sources) near Shanghai Port. The shipping emissions affecting the air quality in the YRD were mainly within 12 NM of shore (over 75 % for ship-related SO2 and 50 % for ship-related PM2.5 concentrations), but emissions from 24 to 96 nm offshore also contributed substantially to PM2.5 concentrations in the YRD under the transport of the summer monsoon. The megacities of Shanghai and Nantong had the highest ship-related population-weighted PM2.5 concentrations from the combination of high population density and high shipping emissions. In Shanghai, the inland-water ships contributed the majority (40 %–80 %) of the PM2.5 from ship-related sources; inland-water ships also contributed prominently to population-weighted PM2.5 in several districts in Shanghai. These study results on the contributions of ships at different distances from shore in the YRD and ship-related sources in and near Shanghai to ambient air quality and population-weighted PM2.5 could help to inform future ECA policies. For example, policymakers could consider whether to expand China's current DECA boundary of 12 NM from shore to around 100 NM or more to reduce the majority of the shipping impacts on air pollution concentrations and exposure. Developing more stringent regulations on the fuel quality for ships entering inland rivers or other waterways close to residential regions would be helpful to improve the local air quality and reduce human exposure in densely populated areas.
Data are available upon request from the corresponding author Yan Zhang (yan_zhang@fudan.edu.cn).
The supplement related to this article is available online at: https://doi.org/10.5194/acp-19-6167-2019-supplement.
YZ and KW conceived the study and made a roadmap for this paper. JF undertook the air quality simulation and wrote the paper. SL ran the shipping emission inventory model. JM ran the WRF model. YZ and CH provided the port-related emission inventory. CL and HK provided the roadmap for human exposure analysis. AP and WM provided constructive comments regarding data analysis. JA and LL provided the local-scale land-based emission inventory. YS and JL provided the river shipping emission data. XW and QF provided the monitoring data. SW and DD provided the national land-based emission inventory. JC, WG and HZ provided the container cargo–car traffic emission inventory.
The authors declare that they have no conflict of interest.
This article is part of the special issue “Shipping and the Environment – From Regional to Global Perspectives (ACP/OS inter-journal SI)”. It is not associated with a conference.
This research work was partly supported by the National Key Research and Development Program of China (grant no. 2016YFA060130X) and the National Natural Science Foundation of China (grant no. 21677038). Katherine Walker and Allison Patton give their thanks to the Bloomberg Foundation. The authors wish to thank Noelle Selin and Freda Fung for providing valuable advice. The opinions expressed in this article are the author's own and do not reflect the view of Fudan University, Shanghai Academy of Environmental Science, Shanghai Environmental Monitoring Center, Tsinghua University, Shanghai Urban-rural Construction and Transportation Development Research Institute, or the Health Effects Institute or its sponsors, nor do they necessarily reflect the views and policies of the EPA or motor vehicle and engine manufacturers.
This paper was edited by Andreas Petzold and reviewed by two anonymous referees.
Aulinger, A., Matthias, V., Zeretzke, M., Bieser, J., Quante, M., and Backes, A.: The impact of shipping emissions on air pollution in the greater North Sea region – Part 1: Current emissions and concentrations, Atmos. Chem. Phys., 16, 739–758, https://doi.org/10.5194/acp-16-739-2016, 2016.
Bright, E., Rose, A., and Urban, M.: LandScan 2015 High-Resolution Global Population Data Set, Oak Ridge National Laboratory, 2016.
Campling, P., Janssen, L., Vanherle, K., Cofala, J., Heyes, C., and Sander, R.: Specific evaluation of emissions from shipping including assessment for the establishment of possible new emission control areas in European Seas, Flemish Institute for Technological Research (VITO), Mol, BE, 2013.
Capaldo, K., Corbett, J. J., Kasibhatla, P., Fischbeck, P., and Pandis, S. N.: Effects of ship emissions on sulphur cycling and radiative climate forcing over the ocean, Nature, 400, 743, https://doi.org/10.1038/23438, 1999.
Chen, D., Zhao, Y., Nelson, P., Li, Y., Wang, X., Zhou, Y., Lang, J., and Guo, X.: Estimating ship emissions based on AIS data for port of Tianjin, China, Atmos. Environ., 145, 10–18, https://doi.org/10.1016/j.atmosenv.2016.08.086, 2016.
Chen, D., Wang, X., Li, Y., Lang, J., Zhou, Y., Guo, X., and Zhao, Y.: High-spatiotemporal-resolution ship emission inventory of China based on AIS data in 2014, Sci. Total Environ., 609, 776–787, 10.1016/j.scitotenv.2017.07.051, 2017a.
Chen, D., Wang, X., Nelson, P., Li, Y., Zhao, N., Zhao, Y., Lang, J., Zhou, Y., and Guo, X.: Ship emission inventory and its impact on the PM2.5 air pollution in Qingdao Port, North China, Atmos. Environ., 166, 351–361, https://doi.org/10.1016/j.atmosenv.2017.07.021, 2017b.
Chen, D., Tian, X., Lang, J., Zhou, Y., Li, Y., Guo, X., Wang, W., and Liu, B.: The impact of ship emissions on PM2.5 and the deposition of nitrogen and sulfur in Yangtze River Delta, China, Sci. Total Environ., 649, 1609–1619, https://doi.org/10.1016/j.scitotenv.2018.08.313, 2019.
Cohen, A. J., Brauer, M., Burnett, R., Anderson, H. R., Frostad, J., Estep, K., Balakrishnan, K., Brunekreef, B., Dandona, L., and Dandona, R.: Estimates and 25-year trends of the global burden of disease attributable to ambient air pollution: an analysis of data from the Global Burden of Diseases Study 2015, Lancet, 389, 1907–1918, 2017.
Collins, B., Sanderson, M. G., and Johnson, C. E.: Impact of increasing ship emissions on air quality and deposition over Europe by 2030, Meteorol. Z., 18, 25–39, 2009.
Cooper, D.: Exhaust emissions from ships at berth, Atmos. Environ., 37, 3817–3830, https://doi.org/10.1016/S1352-2310(03)00446-1, 2003.
Corbett, J. J., Winebrake, J. J., Green, E. H., Kasibhatla, P., Eyring, V., and Lauer, A.: Mortality from ship emissions: a global assessment, Environ. Sci. Technol., 41, 8512, https://doi.org/10.1021/es071686z, 2007.
Eder, B. K. and Yu, S.: A Performance Evaluation of the 2004 Release of Models-3 CMAQ, Springer US, Boston, MA, 534–542, 2007.
ESRI: ArcGIS 10.2., Environmental Systems Research Institute Inc, Redlands, 2013.
Eyring, V., Isaksen, I. S., Berntsen, T., Collins, W. J., Corbett, J. J., Endresen, O., Grainger, R. G., Moldanova, J., Schlager, H., and Stevenson, D. S.: Transport impacts on atmosphere and climate: Shipping, Atmos. Environ., 44, 4735–4771, https://doi.org/10.1016/j.atmosenv.2009.04.059, 2010.
Fan, Q., Zhang, Y., Ma, W., Ma, H., Feng, J., Yu, Q., Yang, X., Ng, S. K., Fu, Q., and Chen, L.: Spatial and Seasonal Dynamics of Ship Emissions over the Yangtze River Delta and East China Sea and Their Potential Environmental Influence, Environ. Sci. Technol., 50, 1322–1329, https://doi.org/10.1021/acs.est.5b03965, 2016.
Fu, M., Liu, H., Jin, X., and He, K.: National- to port-level inventories of shipping emissions in China, Environ. Res. Lett., 12, 114024, https://doi.org/10.1088/1748-9326/aa897a, 2017.
Fu, Q., Shen, Y., and Zhang, J.: On the ship pollutant emission inventory in Shanghai port, J. Safty Environ., 12, 57–64, 2012. (In Chinese)
Jalkanen, J.-P., Brink, A., Kalli, J., Pettersson, H., Kukkonen, J., and Stipa, T.: A modelling system for the exhaust emissions of marine traffic and its application in the Baltic Sea area, Atmos. Chem. Phys., 9, 9209–9223, https://doi.org/10.5194/acp-9-9209-2009, 2009.
Jalkanen, J.-P., Johansson, L., Kukkonen, J., Brink, A., Kalli, J., and Stipa, T.: Extension of an assessment model of ship traffic exhaust emissions for particulate matter and carbon monoxide, Atmos. Chem. Phys., 12, 2641–2659, https://doi.org/10.5194/acp-12-2641-2012, 2012.
Krotkov, N. A., McLinden, C. A., Li, C., Lamsal, L. N., Celarier, E. A., Marchenko, S. V., Swartz, W. H., Bucsela, E. J., Joiner, J., Duncan, B. N., Boersma, K. F., Veefkind, J. P., Levelt, P. F., Fioletov, V. E., Dickerson, R. R., He, H., Lu, Z., and Streets, D. G.: Aura OMI observations of regional SO2 and NO2 pollution changes from 2005 to 2015, Atmos. Chem. Phys., 16, 4605–4629, https://doi.org/10.5194/acp-16-4605-2016, 2016.
Li, C., Yuan, Z., Ou, J., Fan, X., Ye, S., Xiao, T., Shi, Y., Huang, Z., Ng, S. K., and Zhong, Z.: An AIS-based high-resolution ship emission inventory and its uncertainty in Pearl River Delta region, China, Sci. Total Environ., 573, 1–10, https://doi.org/10.1016/j.scitotenv.2016.07.219, 2016.
Li, C., Borken-Kleefeld, J., Zheng, J., Yuan, Z., Ou, J., Li, Y., Wang, Y., and Xu, Y.: Decadal evolution of ship emissions in China from 2004 to 2013 by using an integrated AIS-based approach and projection to 2040, Atmos. Chem. Phys., 18, 6075–6093, https://doi.org/10.5194/acp-18-6075-2018, 2018.
Li, M., Zhang, D., Li, C.-T., Mulvaney, K. M., Selin, N. E., and Karplus, V. J.: Air quality co-benefits of carbon pricing in China, Nat. Clim. Change, 8, 398, https://doi.org/10.1038/s41558-018-0139-4, 2018.
Liu, H., Fu, M., Jin, X., Shang, Y., Shindell, D., Faluvegi, G., Shindell, C., and He, K.: Health and climate impacts of ocean-going vessels in East Asia, Nat. Clim. Change, 6, 1037–1041, https://doi.org/10.1038/nclimate3083, 2016.
Liu, H., Jin, X., Wu, L., Wang, X., Fu, M., Lv, Z., Morawska, L., Huang, F., and He, K.: The impact of marine shipping and its DECA control on air quality in the Pearl River Delta, China, Sci. Total Environ., 625, 1476–1485, https://doi.org/10.1016/j.scitotenv.2018.01.033, 2018a.
Liu, H., Meng, Z. H., Shang, Y., Lv, Z. F., Jin, X. X., Fu, M. L., and He, K. B.: Shipping emission forecasts and cost-benefit analysis of China ports and key regions' control, Environ. Pollut., 236, 49–59, https://doi.org/10.1016/j.envpol.2018.01.018, 2018b.
Liu, Z., Lu, X., Feng, J., Fan, Q., Zhang, Y., and Yang, X.: Influence of Ship Emissions on Urban Air Quality: A Comprehensive Study Using Highly Time-Resolved Online Measurements and Numerical Simulation in Shanghai, Environ. Sci. Technol., 51, 202–211, https://doi.org/10.1021/acs.est.6b03834, 2016.
Lloyd 's register: (IHS Fairplay), 2015.
Lv, Z., Liu, H., Ying, Q., Fu, M., Meng, Z., Wang, Y., Wei, W., Gong, H., and He, K.: Impacts of shipping emissions on PM2.5 pollution in China, Atmos. Chem. Phys., 18, 15811–15824, https://doi.org/10.5194/acp-18-15811-2018, 2018.
Mao, X. and Rutherford, D.: NOX emissions from merchant vessels in coastal China: 2015 and 2030, available at: https://www.theicct.org/sites/default/files/publications/Merchant_Vessel_Emissions_China_20181229.pdf (last access: 2 March 2019), The International Council on Clean Transportation, 2018.
Mao, X., Cui, H., Roy, B., Olmer, N., Rutherford, D., and Comer, B: Distribution of air pollution from oceangoing vessels in the Greater Pearl River Delta, 2015, available at: https://www.theicct.org/sites/default/files/publications/China-GPRD-Baseline-Emissions-Inventory_ICCT-WorkingPaper_23082017_vF.pdf (last access: 15 April 2019), The International Council on Clean Transportation, 2017.
NCEP (National Centers for Environmental Prediction): NCEP FNL Operational Model Global Tropospheric Analyses, continuing from July 1999, Research Data Archive at the National Center for Atmospheric Research, Computational and Information Systems Laboratory, https://doi.org/10.5065/d6m043c6, 2000.
Partanen, A. I., Laakso, A., Schmidt, A., Kokkola, H., Kuokkanen, T., Pietikäinen, J.-P., Kerminen, V.-M., Lehtinen, K. E. J., Laakso, L., and Korhonen, H.: Climate and air quality trade-offs in altering ship fuel sulfur content, Atmos. Chem. Phys., 13, 12059–12071, https://doi.org/10.5194/acp-13-12059-2013, 2013.
Prasannavenkatesh, R., Andimuthu, R., Kandasamy, P., Rajadurai, G., Kumar, D. S., Radhapriya, P., and Ponnusamy, M.: Assessment of Population Exposure to Coarse and Fine Particulate Matter in the Urban Areas of Chennai, India, Sci. World J., 2015, 643714, https://doi.org/10.1155/2015/643714, 2015.
Preen, M.: The Yangtze River Delta Integration Plan: available at: http://www.china-briefing.com/news/2018/06/06/yangtze-river-delta-integration-plan.html (last access: 6 June 2018), 2018.
Schembari, C., Cavalli, F., Cuccia, E., Hjorth, J., Calzolai, G., Pérez, N., Pey, J., Prati, P., and Raes, F.: Impact of a European directive on ship emissions on air quality in Mediterranean harbours, Atmos. Environ., 61, 661–669, 2012.
Seinfeld, J. H. and Pandis, S. N.: Atmospheric Chemistry and Physics: From Air Pollution to Climate Change, 2nd edn. 2006, John Wiley & Sons, Hoboken, New Jersey, 2006.
Soares, J., Kousa, A., Kukkonen, J., Matilainen, L., Kangas, L., Kauhaniemi, M., Riikonen, K., Jalkanen, J.-P., Rasila, T., Hänninen, O., Koskentalo, T., Aarnio, M., Hendriks, C., and Karppinen, A.: Refinement of a model for evaluating the population exposure in an urban area, Geosci. Model Dev., 7, 1855–1872, https://doi.org/10.5194/gmd-7-1855-2014, 2014.
Sofiev, M., Winebrake, J. J., Johansson, L., Carr, E. W., Prank, M., Soares, J., Vira, J., Kouznetsov, R., Jalkanen, J. P., and Corbett, J. J.: Cleaner fuels for ships provide public health benefits with climate tradeoffs, Nat. Commun., 9, 406, https://doi.org/10.1038/s41467-017-02774-9, 2018.
Song, Z. and Tian, C.: Application of the Acoustic Doppler Current Profiler in the Yangtze River Estuary, Hydrology, 6, 31–34, 1997. (In Chinese)
Stohl, A., Aamaas, B., Amann, M., Baker, L. H., Bellouin, N., Berntsen, T. K., Boucher, O., Cherian, R., Collins, W., Daskalakis, N., Dusinska, M., Eckhardt, S., Fuglestvedt, J. S., Harju, M., Heyes, C., Hodnebrog, Ø., Hao, J., Im, U., Kanakidou, M., Klimont, Z., Kupiainen, K., Law, K. S., Lund, M. T., Maas, R., MacIntosh, C. R., Myhre, G., Myriokefalitakis, S., Olivié, D., Quaas, J., Quennehen, B., Raut, J.-C., Rumbold, S. T., Samset, B. H., Schulz, M., Seland, Ø., Shine, K. P., Skeie, R. B., Wang, S., Yttri, K. E., and Zhu, T.: Evaluating the climate and air quality impacts of short-lived pollutants, Atmos. Chem. Phys., 15, 10529–10566, https://doi.org/10.5194/acp-15-10529-2015, 2015.
Sun, K., Liu, H.-N., Ding, A.-J., and Wang, X.-Y.: WRF-Chem simulation of a severe haze episode in the Yangtze River Delta, China, Aerosol Air Qual. Res., 16, 1268–1283, https://doi.org/10.4209/aaqr.2015.04.0248, 2016.
Tao, J., Zhang, L., Cao, J., Zhong, L., Chen, D., Yang, Y., Chen, D., Chen, L., Zhang, Z., and Wu, Y.: Source apportionment of PM2.5 at urban and suburban areas of the Pearl River Delta region, south China – With emphasis on ship emissions, Sci. Total Environ., 574, 1559–1570, 2016.
UNCTAD: Review of maritime transport 2017, available at: https://unctad.org/en/PublicationsLibrary/rmt2017_en.pdf (last access: 11 October 2018), UNITED NATIONS PUBLICATION, USA, 2017.
Viana, M., Fann, N., Tobías, A., Querol, X., Rojas-Rueda, D., Plaza, A., Aynos, G., Conde, J., Fernaìndez, L., and Fernández, C.: Environmental and health benefits from designating the marmara sea and the Turkish straits as an emission control area (ECA), Environ. Sci. Technol., 49, 3304–3313, https://doi.org/10.1021/es5049946, 2015.
von Schneidemesser, E., Monks, P. S., and Plass-Duelmer, C.: Global comparison of VOC and CO observations in urban areas, Atmos. Environ., 44, 5053–5064, https://doi.org/10.1016/j.atmosenv.2010.09.010, 2010.
Wang, H., Chen, C., Huang, C., and Fu, L.: On-road vehicle emission inventory and its uncertainty analysis for Shanghai, China, Sci. Total Environ., 398, 60–67, https://doi.org/10.1016/j.scitotenv.2008.01.038, 2008.
Wang, M., Shao, M., Chen, W., Yuan, B., Lu, S., Zhang, Q., Zeng, L., and Wang, Q.: A temporally and spatially resolved validation of emission inventories by measurements of ambient volatile organic compounds in Beijing, China, Atmos. Chem. Phys., 14, 5871–5891, https://doi.org/10.5194/acp-14-5871-2014, 2014.
Winebrake, J. J., Corbett, J. J., Green, E. H., Lauer, A., and Eyring, V.: Mitigating the health impacts of pollution from oceangoing shipping: an assessment of low-sulfur fuel mandates, Environ. Sci. Technol., 43, 4776–4782, https://doi.org/10.1021/es803224q, 2009.
Xue Y., Gu J., and Wei T.: The working principle of the Acoustic Doppler Profiler and its applications in the middle and lower reaches of the Yangtze River, Mar. Sci., 28, 24–28, 2004. (In Chinese)
Yau, P. S., Lee, S. C., Corbett, J. J., Wang, C., Cheng, Y., and Ho, K. F.: Estimation of exhaust emission from ocean-going vessels in Hong Kong, Sci. Total Environ., 431, 299–306, https://doi.org/10.1016/j.scitotenv.2012.03.092, 2012.
Zhao, B., Zheng, H., Wang, S., Smith, K. R., Lu, X., Aunan, K., Gu, Y., Wang, Y., Ding, D., Xing, J., Fu, X., Yang, X., Liou, K. N., and Hao, J.: Change in household fuels dominates the decrease in PM2.5 exposure and premature mortality in China in 2005–2015, P. Natl. Acad. Sci. USA, 115, 12401–12406, https://doi.org/10.1073/pnas.1812955115, 2018
Zhao, M., Zhang, Y., Ma, W., Fu, Q., Yang, X., Li, C., Zhou, B., Yu, Q., and Chen, L.: Characteristics and ship traffic source identification of air pollutants in China's largest port, Atmos. Environ., 64, 277–286, https://doi.org/10.1016/j.atmosenv.2012.10.007, 2013.