the Creative Commons Attribution 4.0 License.
the Creative Commons Attribution 4.0 License.
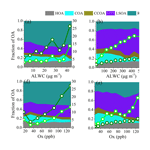
Distinctions in source regions and formation mechanisms of secondary aerosol in Beijing from summer to winter
Jing Duan
Ru-Jin Huang
Chunshui Lin
Wenting Dai
Meng Wang
Yifang Gu
Ying Wang
Haobin Zhong
Yan Zheng
Haiyan Ni
Uli Dusek
Yang Chen
Yongjie Li
Douglas R. Worsnop
Colin D. O'Dowd
Junji Cao
To investigate the sources and evolution of haze pollution in different seasons, long-term (from 15 August to 4 December 2015) variations in chemical composition of PM1 were characterized in Beijing, China. Positive matrix factorization (PMF) analysis with a multi-linear engine (ME-2) resolved three primary and two secondary organic aerosol (OA) sources, including hydrocarbon-like OA (HOA), cooking OA (COA), coal combustion OA (CCOA), local secondary OA (LSOA) and regional SOA (RSOA). The sulfate source region analysis implies that sulfate was mainly transported at a large regional scale in late summer, while local and/or nearby sulfate formation may be more important in winter. Meanwhile, distinctly different correlations between sulfate and RSOA or LSOA (i.e., better correlation with RSOA in late summer, similar correlations with RSOA and LSOA in autumn, and close correlation with LSOA in early winter) confirmed the regional characteristic of RSOA and local property of LSOA. Secondary aerosol species including secondary inorganic aerosol (SIA – sulfate, nitrate, and ammonium) and SOA (LSOA and RSOA) dominated PM1 during all three seasons. In particular, SOA contributed 46 % to total PM1 (with 31 % as RSOA) in late summer, whereas SIA contributed 41 % and 45 % to total PM1 in autumn and early winter, respectively. Enhanced contributions of secondary species (66 %–76 % of PM1) were also observed in pollution episodes during all three seasons, further emphasizing the importance of secondary formation processes in haze pollution in Beijing. Combining chemical composition and meteorological data, our analyses suggest that both photochemical oxidation and aqueous-phase processing played important roles in SOA formation during all three seasons, while for sulfate formation, gas-phase photochemical oxidation was the major pathway in late summer, aqueous-phase reactions were more responsible during early winter and both processes had contributions during autumn.
- Article
(5600 KB) - Full-text XML
-
Supplement
(666 KB) - BibTeX
- EndNote
Atmospheric particulate matter (PM) has broad impacts on the environment, including air quality (Molina et al., 2007; Sun et al., 2010, 2013; Huang et al., 2014), regional and global climate (Kaufman et al., 2002; IPCC, 2013; Molina et al., 2015), and human health (Pope et al., 2002; Lelieveld et al., 2015). Over the past decades, PM pollution in China has become one of the most serious environmental problems (Li et al., 2017; An et al., 2019). Beijing, the capital of China, has been suffering from severe haze events, with annual concentrations of PM2.5 frequently exceeding the Chinese National Ambient Air Quality Standard (35 µg m−3 as an annual average) (He et al., 2001; Streets et al., 2007; Huang et al., 2014; Wang et al., 2015). Effective mitigation of PM pollution requires a better understanding of the emission sources and atmospheric evolution processes (Cao et al., 2012a, b; Huang et al., 2014; Guo et al., 2014; Sun et al., 2014).
The Aerodyne aerosol mass spectrometers (AMSs) have been widely used to obtain real-time measurements of the chemical composition of the non-refractory PM (NR-PM), including organic aerosol (OA), sulfate, nitrate, ammonium and chloride. Real-time techniques such as that employed by an AMS overcome some limitations of offline techniques, for instance, measurement artifacts or limited time resolution (DeCarlo et al., 2006; Canagaratna et al., 2007; Ng et al., 2011b). The aerosol chemical speciation monitor (ACSM), which is a simplified version of AMS, was designed for long-term measurements of NR-PM1. In Beijing, a number of online and offline studies have been conducted in recent years to investigate the chemical composition, emission sources and formation mechanisms of PM (Chan and Yao, 2008; Zhao et al., 2013; Huang et al., 2014; Tian et al., 2014; Ho et al., 2015; Wang et al., 2015; Xu et al., 2015; Yang et al., 2015; Elser et al., 2016). It has been found that OA is the most dominant contributor to fine PM and that the secondary aerosol plays an important role in haze formation (Huang et al., 2014; Elser et al., 2016).
Atmospheric receptor models, e.g., positive matrix factorization (PMF; Paatero and Tapper, 1994), have been successfully used to perform OA source apportionment based on the OA mass spectral data (Lanz et al., 2007; Ulbrich et al., 2009; Thornhill et al., 2010; Sun et al., 2012, 2013; Elser et al., 2016; Wang et al., 2017). Primary OA (POA) sources such as hydrocarbon-like OA (HOA), cooking OA (COA), and biomass burning OA (BBOA) or coal combustion OA (CCOA) have been identified, while secondary OA (SOA) factors could be resolved either based on oxidation state (i.e., less-oxidized oxygenated OA (LO-OOA) and more-oxidized oxygenated OA (MO-OOA)) or based on volatility (i.e., semi-volatility oxygenated OA (SV-OOA) and low-volatility oxygenated OA (LV-OOA)) (Huang et al., 2012; Crippa et al., 2013; Hu et al., 2013; Wang et al., 2017). PMF analyses have been used in a number of studies in Beijing (Huang et al., 2010; Sun et al., 2013, 2014, 2016, 2018; Huang et al., 2014; Elser et al., 2016; Hu et al., 2016).
Despite the large number of aforementioned studies, the major sources and mechanisms responsible for the PM pollution during haze events are not well constrained, mainly due to complex interplay among local emission, regional transport, secondary reaction and meteorological influence (Volkamer et al., 2006; Ma et al., 2010; Tao et al., 2012; Sun et al., 2014; Zhang et al., 2017). For example, Hu et al. (2016) reported a stable ∼80 % contribution of secondary species to PM1 in summertime Beijing, while PM1 mass concentration in winter changed dramatically due to different meteorological conditions and enhanced primary emissions. However, Huang et al. (2014) and Elser et al. (2016) found that secondary aerosol formation also plays a crucial role in wintertime haze events in Beijing. The formation mechanisms of secondary aerosol during haze events are not well constrained. Besides photochemical reactions, aqueous-phase reactions have been suggested to contribute to SOA formation. For example, PMF studies show that an aqueous OOA factor contributed 12 % of total OA in wintertime Beijing and that the oxidation degree of OA increased at high RH levels (> 50 %) (Sun et al., 2016). In combination with the back-trajectory analysis, it is found that high PM1 concentrations in Beijing were associated with air masses from the south and southwest and characterized by high fractions of MO-OOA and secondary inorganic aerosol, whereas direct emissions from local sources were the main contributor during clean events (Sun et al., 2015). These results show the inhomogeneity in the contribution to PM pollution depending on different sampling locations and seasons, highlighting the need for more studies on chemical composition, sources and atmospheric evolution of PM.
In this study, we discuss the seasonal characteristics of the chemical nature, sources and atmospheric evolution of PM1 in urban Beijing. Specifically, the formation mechanisms of secondary species and the impacts of meteorological conditions on the haze pollution are elucidated.
2.1 Measurement site
Measurements were conducted at an urban site in the National Center for Nanoscience (39.99∘ N, 116.32∘ E) in Beijing, which is close to the fourth ring of Beijing and surrounded by residential, commercial and traffic areas. All instruments were deployed on the roof of a five-story building (∼20 m above the ground) and the measurements were performed from 15 August to 4 December 2015.
2.2 Instrumentation
NR-PM1 species including organics, sulfate, nitrate, ammonium and chloride were continuously measured by an Aerodyne quadrupole ACSM (Q-ACSM) with a time resolution of ∼30 min. Detailed descriptions of ACSM operation can be found elsewhere (Ng et al., 2011a; Wang et al., 2017). Briefly, the ambient aerosol was sampled at a flow rate of ∼3 L min−1 through a 3∕8 in. stainless steel tube and a University Research Glass ware (URG) cyclone (Model: URG-2000-30ED); a size cut of 2.5 µm in front of the sampling inlet was used to remove coarse particles. A Nafion dryer (MD-110-48S; Perma Pure, Inc., Lakewood, NJ, USA) was applied to dry aerosol particles before they entered the ACSM, and the submicron aerosol was subsampled into the ACSM with a flow rate of 85 cc min−1 fixed by a 100 µm diameter critical aperture. The submicron particles were focused into a narrow beam by an aerodynamic lens and impacted a hot vaporizer (). The resulting vapor was ionized with electron impact and chemically characterized with a quadrupole mass spectrometer. Monodispersed 300 nm ammonium nitrate particles, generated by an atomizer (Model 9302, TSI Inc., Shoreview, MN, USA) and selected by a differential mobility analyzer (DMA; TSI model 3080), were used to determine the response factor (RF) and calibrate the ionization efficiency (IE) (Ng et al., 2011a).
An aethalometer (Model AE-33, Magee Scientific) was used for the determination of black carbon (BC) concentration with a time resolution of 1 min. SO2 was measured by an Ecotech EC 9850 sulfur dioxide analyzer, CO by a Thermo Scientific Model 48i carbon monoxide analyzer, NOx by a Thermo Scientific Model 42i NO-NO2-NOx analyzer and O3 by a Thermo Scientific Model 49i ozone analyzer. Meteorological parameters, including wind speed, wind direction, relative humidity (RH) and temperature, were measured by an automatic weather station (MAWS201, Vaisala, Vantaa, Finland) and a wind sensor (Vaisala Model QMW101-M2).
2.3 Data analysis
2.3.1 ACSM data analysis
The standard ACSM data analysis software in Igor Pro (WaveMetrics, Inc., Lake Oswego, Oregon USA) was used to analyze the ACSM dataset. IE was determined by comparing the response factors of ACSM to the mass calculated with the known particle size and the number concentration from a condensation particle counter (CPC; TSI model 3772). Standard relative ionization efficiencies (RIEs) were used for organics, nitrate and chloride (i.e., 1.4 for organics, 1.1 for nitrate and 1.3 for chloride) and RIEs for ammonium (6.4) and sulfate (1.2) were estimated from the IE calibrations using NH4NO3 and NH4SO4. The collection efficiency (CE) was introduced to correct for the particle loss due to particle bounce, which is influenced by aerosol acidity, composition and the aerosol water content. As aerosol was dried before entering the ACSM and particles are overall neutralized, the influences of particle phase water and acidity are expected to be negligible. Therefore, CE was determined as CEdry= max ( ANMF), where ANMF represents the mass fraction of ammonium nitrate in NR-PM1 (Middlebrook et al., 2012).
2.3.2 Source apportionment
PMF was used to perform the source apportionment on the organic spectral data as implemented by the multilinear engine (ME-2; Paatero, 1997) via the interface SoFi (Source Finder) coded in Igor Wavemetrics (Canonaco et al., 2013). First, a range of solutions with two to eight factors from unconstrained runs were examined. The POA factors mixed seriously with the SOA factors in the three-factor solution, and there was no new interpretable factor when increasing the factor numbers above 4 in the PMF analysis. Therefore, the four-factor solution (HOA + CCOA, COA, OOA1 and OOA2) was adopted (Fig. S1 in the Supplement). In the four-factor solution, the COA factor was well-defined through the much higher contribution of m∕z 55 than m∕z 57 in its profile and the symbolic diurnal cycle of three peaks corresponding to the time of breakfast, lunch and dinner, supporting the assignment of the COA factor. Although the COA profile was well-defined, HOA and CCOA were totally mixed in the four-factor PMF solution, and the mixed factor had hydrocarbon-like fragments of CnH2n−1 and CnH2n+1 as in HOA but substantial amounts of polycyclic aromatic hydrocarbon (PAH)-related ions as in CCOA. This mixed HOA + CCOA factor could not be further separated when increasing the number of factors, likely due to the low mass resolution in ACSM data and limited capacity of PMF in separating similar factors. The mixture of HOA and CCOA factors was also observed in Sun et al. (2018), suggesting the difficulty in separating HOA and CCOA with PMF for the ACSM dataset. Compared to PMF, the ME-2 approach can direct the apportionment towards an environmentally meaningful solution by introducing a priori information (profiles) for certain factors (Canonaco et al., 2013; Crippa et al., 2014; Frohlich et al., 2015). The ME-2 runs of five factors were performed to separate HOA from CCOA and further optimize the apportionment solutions. We first constrained the HOA using the HOA profile from Ng et al. (2011b), which is the average over 15 sites all over the world (including China, Japan, Europe and the United States). Previous studies have suggested that the HOA spectra from Europe and China are similar (Ng et al., 2011b; Elser et al., 2016) despite the different vehicle fuel patterns in China and Europe. When HOA was constrained, a new CCOA factor could be resolved. However, this CCOA factor was seriously mixed with OOA as indicated by a relatively higher intensity at m∕z 44 in the CCOA profile (Fig. S2). We thus further constrained the CCOA profile to decrease the influence of OOA on the CCOA factor. A CCOA profile from our previous study (Wang et al., 2017) was used to constrain CCOA. To minimize the effect from nonlocal input profiles (for both HOA and CCOA), the a value approach was used to adjust the input profiles to a certain extent. In addition, we also constrained the COA profile from the four-factor PMF solution with an a value of 0, which is a well-defined local profile as discussed above.
We tested a values for HOA and CCOA profiles between 0 and 1 with an interval of 0.1 and obtained 121 possible results, among which six solutions were reasonable based on the verification of the rationality of unconstrained factors, distinct mass spectra and time series, interpretable diurnal cycles, and good correlations with external tracers for all factors. The final profiles and time series of individual factor were averaged from these six solutions, and the standard deviations of intensities at each m∕z were shown as error bars.
2.4 Liquid water content
Aerosol liquid water content (ALWC) was predicted using the ISORROPIA-II model (Fountoukis and Nenes, 2007) with ACSM aerosol composition and meteorological parameters (temperature and relative humidity) as input. The ISORROPIA-II model then calculated the composition and phase state of a –––Cl−–H2O system in thermodynamic equilibrium, and the concentration of H+ and ALWC could be resolved.
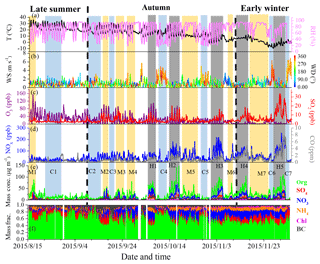
Figure 1Time series of (a) temperature (T) and relative humidity (RH), (b) wind speed (WS) and wind direction (WD), (c) O3 and SO2, (d) CO and NOx, (e) PM1 species, and (f) mass fractions of PM1 species during the entire study. Seven clean episodes (C1–C7), seven moderate-pollution episodes (M1–M7) and five high-pollution episodes (H1–H5) are marked for further discussion. The dates in this and other figures are given as year/month/day.
3.1 Overview of mass concentration and chemical composition
Figure 1 shows the time series of meteorological parameters, trace gases and PM1 composition during the entire measurement period. The relatively clean events and polluted episodes occurred alternatively during the entire campaign. As shown in Fig. 1, the variations in PM1 species are strongly associated with meteorological conditions. For example, clean periods were generally associated with northerly and northwesterly winds with high wind speeds. However, serious pollution episodes were related to southerly winds with low wind speeds (< 1 m s−1), indicating the important role of stagnant meteorological conditions in haze pollution (Takegawa et al., 2009; Huang et al., 2010; Sun et al., 2014). The mass concentration of PM1 varied from 0.4 to 260.7 µg m−3. Considering that the long-term measurements in our study have different meteorological conditions, we separated the entire study into three periods as late summer (15 August to 10 September), autumn (11 September to 10 November) and early winter (11 November to 4 December) in order to discuss the seasonal variations in PM1 mass concentration and chemical composition.
Table 1Summary of PM1 mass concentrations and composition as well as OA composition in Beijing during different seasons.
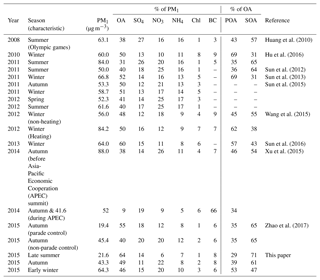
The average mass concentration of PM1 was 21.6 µg m−3 in late summer (Fig. S3), which was much lower than that measured in July–August 2011 (50.0 µg m−3; Sun et al., 2012) and in August–September 2011 (84.0 µg m−3; Hu et al., 2016) (see Table 1). This lower PM1 concentration was likely associated with the 2015 China Victory Day parade control from 23 August to 3 September, which significantly improved air quality in Beijing (Zhao et al., 2017). OA constituted a major fraction of PM1 mass (64 %), followed by sulfate (14 %), BC (8 %), ammonium (7 %), nitrate (6 %) and chloride (1 %). During autumn, the mean concentration of PM1 increased to 43.3 µg m−3, which was 2 times higher than that in late summer. OA contributed a mass fraction of 49 %, followed by nitrate, sulfate, ammonium, BC and chloride with the mass fractions of 22 %, 11 %, 8 %, 8 % and 2 %, respectively. Compared to late summer, the mass fraction of OA decreased to 49 % (but the OA mass increased from 13.8 to 21.2 µg m−3), and the mass fraction of inorganic species increased correspondingly. The increase in inorganics was particularly noticeable for nitrate, which increased from 6 % to 22 % (or from 1.3 to 9.5 µg m−3). The mean concentration of PM1 was 64.3 µg m−3 in early winter, even higher than those in late summer and autumn. This PM1 average concentration in wintertime Beijing is similar to other studies, such as Hu et al. (2016) (60.0 µg m−3), Sun et al. (2013) (66.8 µg m−3) and Sun et al. (2016) (64.0 µg m−3). OA accounted for 46 % of PM1 mass in early winter, followed by 20 % of nitrate, 15 % of sulfate, 10 % of ammonium, 6 % of BC and 3 % of chloride (Fig S3).
As shown in Figs. 1f and S3, OA dominated PM1 mass in late summer. In autumn and early winter, however, the contribution of OA decreased and secondary inorganic aerosol increased to be equally important. It should also be noted that nitrate had a more important contribution than sulfate to PM1 during autumn and early winter, with nitrate∕sulfate mass ratios of 2.0 and 1.3 in autumn and early winter, respectively. This phenomenon is likely due to the efficient emission reduction of SO2 and the continuous increase in NOx because of dramatic growth of the vehicle fleets and large emissions from industries (Xu et al., 2015). Therefore, nitrate is expected to play a more important role in PM pollution in the near future and controlling NOx emission would greatly help mitigate air pollution in Beijing.
The diurnal cycles of PM1 species during different seasons are shown in Fig. S4. OA was characterized by three peaks occurring in the morning (06:00–09:00 LT), at noon (12:00–14:00 LT) and in the evening (19:00–22:00 LT) during all three seasons. Such diurnal patterns were partially influenced by the emission behavior of pollution sources, i.e., traffic, cooking and/or coal burning emissions (Huang et al., 2012; Sun et al., 2012; Crippa et al., 2013). Due to the relatively flat planetary boundary layer (PBL) height related to stagnant meteorological conditions in early winter compared to that in autumn and late summer, the noon peak of OA was more evident in early winter. The morning peak of OA was even more pronounced than the noon peak in late summer. Such a diurnal cycle was likely related to the efficient photochemical oxidation in the morning and efficient dilution effect resulted from PBL height increase at noon.
The diurnal cycle of nitrate varied significantly during different seasons due to the seasonal difference in photochemical production and gas-particle partitioning (Sun et al., 2015). Compared to nitrate, sulfate showed a relatively flat diurnal cycle in all seasons. A clear increase in sulfate in the afternoon was observed during late summer and autumn due to enhanced photochemical processes (Takegawa et al., 2009). In the winter, however, sulfate showed a decreasing trend in the afternoon, suggesting low photochemical production as discussed below. Chloride presented a morning peak and then rapidly decreased to a low concentration level at ∼ 18:00 LT during late summer, while in both autumn and winter, chloride displayed a diurnal cycle with higher concentrations at nighttime, which may be related to the local emission from coal combustion. BC also showed a similar diurnal cycle with higher concentrations at nighttime and lower concentrations in the daytime during all three seasons.
3.2 Primary OA factors
Three POA factors were resolved in this study: HOA, COA and CCOA. As shown in Fig. 2a, the HOA mass spectrum is characterized by prominent hydrocarbon ion series of CnH2n−1 and CnH particularly m∕z 27, 29, 41, 43, 55, 57, 67 and 71. The HOA spectrum is similar to previously reported HOA spectra at various urban sites (He et al., 2011; Ng et al., 2011; Sun et al., 2012). The time series of HOA is also correlated well with that of BC, which is an external tracer of incomplete combustion (R2=0.56). The mass fractions of HOA (10 %–13 %) and diurnal cycles in different seasons are rather consistent. There are two peaks from rush hours, i.e., 07:00–09:00 LT in the morning and around 20:00 LT in the evening. The nighttime concentrations are generally high (Fig. S4), likely due to increased diesel fleets, which are allowed in urban Beijing only at night, and the decrease in PBL during nighttime.
The COA profile is characterized by prominent ion peaks at m∕z 55 and m∕z 57 (Fig. 2b) and a higher ratio of intensity at m∕z 55 over that at m∕z 57 (=2.3) compared to the other two primary OA components (∼1), which have been shown to be robust markers for COA (He et al., 2010; Mohr et al., 2012; Crippa et al., 2013; Elser et al., 2016). This COA mass spectrum is highly correlated with other COA profiles reported in previous studies (Crippa et al., 2013; Elser et al., 2016; Wang et al., 2017), and the time series correlated well with that of m∕z 55 with R2=0.81. The COA diurnal cycle showed two obvious peaks at lunch (12:00 LT) and dinner (20:00 LT) time and a smaller peak at breakfast time (07:00 LT) (Fig. S4). Similar diurnal behaviors of COA have been observed in Beijing and at other urban sites (Allan et al., 2010; Sun et al., 2010, 2013). COA had a lower mass fraction of 11 % during late summer compared to autumn (20 %) and early winter (16 %).
The mass spectrum of CCOA is dominated by unsaturated hydrocarbons, particularly PAH-related ion peaks (e.g., 77, 91 and 115) (Dall'Osto et al., 2013; Hu et al., 2013). It shows a similar spectral pattern with the ambient CCOA mass spectra in Beijing and Xi'an (Elser et al., 2016). The presence of CCOA can be further validated by the good correlation with external combustion tracer chloride (R2=0.77) (Zhang et al., 2012). The time series of CCOA shows that the mass concentration of CCOA was much lower in August and September but increased dramatically after November, indicating the large emissions from residential coal combustion for domestic heating. Also, the nighttime CCOA concentrations were much higher than the daytime concentrations, further confirming the enhanced coal combustion emissions from domestic heating in wintertime nights. Specifically, on average, the mass fraction of CCOA increased from 5 % (0.7 µg m−3) in late summer to 9 % (2.0 µg m−3) in autumn and then to 26 % (7.7 µg m−3) in early winter (Fig. S3).
3.3 Secondary OA factors and sulfate sources: regional transport vs. local formation
In order to analyze sources of sulfate in our study period, the bivariate polar plots of sulfate during different seasons are displayed in Fig. 3. During late summer, the high mass concentration of sulfate was mainly located in the south and southwest regions from the sampling site, suggesting regional transport was the major source of sulfate in late summer. However, high sulfate was located both at the sampling site and in the south and southeast regions from the sampling site in autumn, which indicates that both local formation and regional transport contributed to the sulfate concentration. When it comes to the early winter, high mass concentration of sulfate was mainly located at the sampling site coming from local formation and there was almost no contribution from regional transport. These results indicate that transported sulfate at a large regional scale was more important during late summer, while local formation was the major source of sulfate in early winter due to residential heating.
Two oxygenated OA factors with very different time series were identified in our study, which we defined as local SOA (LSOA) and regional SOA (RSOA) as characterized below in detail. As shown in Fig. 3, different correlations between sulfate and RSOA or LSOA were found during different seasons. The time series of RSOA correlated well with that of sulfate during late summer with R2=0.71. This correlation coefficient decreased to 0.62 during autumn, and there was almost no correlation between RSOA and sulfate (R2=0.02) in early winter. By contrast, the correlations between LSOA and sulfate displayed the opposite variation with the correlation coefficient (R2) increasing from 0.40 in late summer to 0.66 in autumn and 0.86 in early winter (Fig. 3a). As we have discussed that sulfate mainly came from regional transport during late summer, while the contribution of local formation increased during autumn and further became the dominant source of sulfate, these correlation variations (i.e., better correlation with RSOA in late summer, similar correlations with RSOA and LSOA in autumn and close correlation with LSOA in early winter) suggested that RSOA is related to the regional source of OOA and LSOA indicates a local source and subsequent local formation. These two SOA factors show similar mass spectra with high ratios of intensity at m∕z 44 over that at m∕z 43 (f44∕43), and the f44∕43 of RSOA (4.8) is higher than that of LSOA (2.9), suggesting that RSOA from regional transport is more oxygenated (more aged) than locally formed SOA (Sun et al., 2014, 2015). The attribution of LSOA and RSOA is further supported by the bivariate polar plots (Fig. S5), which show clearly that LSOA is mainly located at the sampling site while RSOA is mainly from the south of the sampling site. The average mass concentration of LSOA increased from 3.2 in late summer to 9.2 µg m−3 in autumn and to 12.1 µg m−3 in early winter with an increase in mass fraction from 23 in late summer to 43 % in autumn and 41 % in early winter. By contrast, the average mass concentration of RSOA decreased from 6.6 in late summer to 3.8 µg m−3 in autumn and to 1.8 µg m−3 in early winter, with a dramatic decrease in mass fraction from 48 % in late summer to 18 % in autumn and to 6 % in early winter (Fig. S3). These seasonal variations in LSOA and RSOA indicate that RSOA related to regional transport was more important during late summer, while locally formed LSOA played a dominant role in autumn and early winter.
3.4 Contribution of secondary species to PM pollution
The average PM1 concentration increased from late summer (21.6 µg m−3) to early winter (64.3 µg m−3) (Fig. S3) and the chemical composition showed a seasonal difference. The mass concentrations of secondary species increased from 15.7 µg m−3 in late summer to 30.8 µg m−3 in autumn and to 42.8 µg m−3 in early winter, but the mass fraction in PM1 decreased from 72 % in late summer to 66 % in early winter. In particular, SOA had a dominant contribution in late summer (9.8 µg m−3, 46 % of PM1), while secondary inorganic aerosol (SIA) played a key role during autumn (17.8 µg m−3, 41 % of PM1) and early winter (28.9 µg m−3, 45 % of PM1) (Fig. S3). The high SOA fraction in summer is likely associated with active photochemical oxidation, while the increased SIA fraction in autumn and early winter is likely due to enhanced gas-particle partitioning of nitrate and aqueous-phase formation of sulfate.
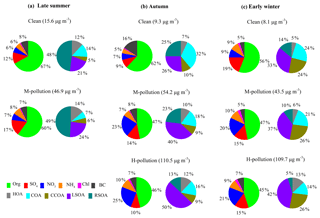
Figure 4Relative contributions of PM1 species and OA sources on clean days, M-pollution days and H-pollution days during late summer (a), autumn (b) and early winter (c).
Figure 4 shows the PM1 composition and OA sources on clean days (daily average PM1 < 20 µg m−3), medium-pollution days (M-pollution; 40 µg m−3 < daily average PM1 < 80 µg m−3) and high-pollution days (H-pollution; daily average PM1 > 80 µg m−3) during different seasons. The mass concentrations of PM1 species and OA factors, gaseous pollutants, and meteorological parameters during different periods are summarized in Table S1 in the Supplement. The average concentration of PM1 was 46.9 µg m−3 during M-pollution days, about 3 times higher than that during clean days (15.6 µg m−3) in late summer. In autumn and early winter, the average PM1 concentrations during H-pollution days (110.5 and 109.7 µg m−3, respectively) were 2 times higher than those on M-pollution days (54.2 and 43.5 µg m−3, respectively) and 10 times higher than those on clean days (9.3 and 8.1 µg m−3, respectively). As shown in Fig. 4, the mass fraction of secondary aerosol species (SIA and SOA) increased from clean days (52 %–70 %) to M-pollution days (67 %–76 %) and H-pollution days (66 %–74 %) during all three seasons, emphasizing the significant enhancements of secondary aerosol formation in haze pollution events (Huang et al., 2014; Jiang et al., 2015; Zheng et al., 2015). In late summer, the mass concentration of LSOA increased from 2.2 µg m−3 (21 % of OA) during clean days to 6.7 µg m−3 (24 % of OA) during M-pollution days, and the mass concentration of RSOA increased from 5.0 µg m−3 (48 % of OA) during clean days to 13.8 µg m−3 (49 % of OA) during M-pollution days, suggesting that regional transport played a more important role than local formation in both clean and haze pollution events during late summer. The mass concentration of LSOA increased from 1.5 on clean days to 10.2 µg m−3 on M-pollution days and to 25.4 µg m−3 on H-pollution days during autumn and increased from 1.5 µg m−3 on clean days to 7.5 µg m−3 on M-pollution days and to 20.7 µg m−3 on H-pollution days during early winter. In comparison, the mass concentration of RSOA increased from 1.5 and 0.6 µg m−3 on clean days to 5.9 and 2.0 µg m−3 on M-pollution days and to 6.6 and 2.5 µg m−3 on H-pollution days during autumn and early winter, respectively. The increased rates of LSOA were much higher than that of RSOA; thus, the mass fraction of LSOA increased dramatically from clean days to M-pollution and H-pollution days in autumn and early winter (i.e., 26 % to 40 % and 50 % during autumn and 33 % to 37 % and 42 % during early winter), whereas the mass fraction of RSOA decreased from clean days to M-pollution and H-pollution days (i.e., 25 % to 23 % and 13 % during autumn and 14 % to 10 % and 5 % during early winter). These observations suggest that locally formed SOA had more important contributions than regional sources in haze pollution during autumn and early winter, implying a different contribution of secondary aerosol in different seasons.
3.5 Episodic analysis and meteorological effects
The clean and pollution episodes occurred in “sawtooth cycles”, in which meteorological conditions, regional transport, local emissions and secondary formation intertwine and play different roles in the evolution of PM pollution. To get a better insight into aerosol sources and atmospheric processes, seven clean episodes (average PM1 concentration < 20 µg m−3), seven M-pollution episodes (40 µg m−3 < average PM1 concentration < 80 µg m−3) and five H-pollution episodes (average PM1 concentration > 100 µg m−3) were selected for further analysis. As shown in Fig. 5, the pollution episodes were generally associated with higher RH and lower wind speeds (< 1 m s−1) than in clean episodes in autumn and early winter, with RH usually higher than 60 % in pollution episodes (both M-pollution and H-pollution) and lower than 45 % in clean episodes. Specifically, an M-pollution (M1; 47.6 µg m−3) episode in late summer had similar RH and wind speed to the adjacent clean period (C1; 14.1 µg m−3). However, the contribution of organic species decreased from 68 % in C1 to 61 % in M1, but the mass fraction of secondary inorganic species (particularly sulfate) increased from 23 % in C1 to 33 % in M1. This phenomenon may result from enhanced photochemical formation of secondary species in M1 due to higher oxidation capacity as M1 had higher O3 concentration (54.1 ppb) than C1 (31.0 ppb). In autumn, the mass concentrations of organics increased from 4.8 to 6.3 during C2–C5 to 21.2–27.8 µg m−3 during M2–M6, while the contributions decreased from 56 %–71 % to 39 %–55 %, and the corresponding contributions of secondary inorganic species increased from 17 %–29 % during C2–C5 to 36 %–52 % during M2–M6 with mass concentrations increasing from 1.6–2.9 to 16.7–33.1 µg m−3. The contributions of secondary organic species to OA also increased from 50 %–61 % to 55 %–73 % with mass concentrations increasing from 2.7–3.6 to 14.1–19.4 µg m−3. This indicates a notable production and accumulation of secondary aerosol during pollution events. Compared to M-pollution episodes, there was no further increase in the contribution of secondary inorganic species during H1–H3 (42 %–47 %) although the mass concentrations increased to 45.3–56.6 µg m−3 due to the systematic concentration growths of all species from M-pollution to H-pollution. Secondary organic species also had similar contributions to OA during H1–H3 (52 %–75 %) to that during M2–M6 (55 %–73 %) although the mass concentrations increased from 14.1–19.4 to 25.6–38.5 µg m−3. Further analysis shows that the RH during H1–H3 (71.7 %–81.6 %) is lower than that during M2–M6 (74.1 %–91.8 %), which indicates that the stronger aqueous-phase chemistry during M2–M6 may lead to the efficient formation of secondary species, and the mass concentration growths of secondary species were faster than that of other species in PM1; thus, the mass fraction of secondary species in M2–M6 was higher or similar to that in H1–H3. A similar phenomenon was also found in early winter. The contributions of secondary species increased from clean episodes (C6 and C7) to pollution episodes (M7, H4 and H5), while the contributions of secondary species were similar in M7, H4 and H5 because of similar RH. These PM evolution characteristics observed here highlight the importance of meteorological conditions for driving particulate pollution (Li et al., 2017) and imply different formation mechanisms of PM pollution during different seasons.
3.6 Photochemical oxidation and aqueous-phase chemistry
To further elucidate the formation mechanisms of secondary aerosol, the sulfur oxidation ratio () (Sun et al., 2006) was calculated according to Eq. (1):
where n[SO4] and n[SO2] are the molar concentrations of sulfate and SO2, respectively. Figure 6a–c plots versus Ox (= O3 + NO2) concentration which is a tracer to indicate photochemical processing during late summer, autumn and early winter, respectively. During late summer, positive correlations between and Ox with similar slopes and correlation coefficients in RH < 65 % and RH > 65 % were observed, suggesting the important role of photochemical oxidation during late summer irrespective of the RH range. During autumn and early winter, at RH < 65 % sulfate was also formed by photochemical oxidation because of the positive correlations between and Ox, while there was no correlation between and Ox at RH > 65 %, indicating that other processes (e.g., aqueous-phase reactions) may contribute to the sulfate formation in high-RH conditions. This is supported by the relationships between and ALWC at RH > 65 % and low atmospheric oxidative capacities of Ox < 60 ppb (Fig. 6d–f). There were positive correlations between and ALWC during all three seasons in high-RH conditions, indicating the contribution of aqueous-phase processing to the sulfate formation. Meanwhile, we found that was up to ∼0.6 with Ox while it was only up to ∼0.3 with ALWC during late summer, suggesting the more important role of photochemical oxidation for the sulfate formation during late summer. By contrast, during early winter the increase in with ALWC (from ∼0.05 to ∼0.5) was more efficient than that with Ox (from ∼0.05 to ∼0.2), indicating that aqueous-phase reactions were more responsible during early winter. During autumn, was up to about 0.4–0.5 both with Ox and ALWC, suggesting that for sulfate formation during autumn both photochemical oxidation and aqueous-phase reaction had important contributions. It should be noted that at the typical atmospheric level of OH radicals, the lifetime of SO2 from the reaction with OH is about 1 week (Seinfeld and Pandis, 2016; Zhang et al., 2015), and the bivariate polar plots of Ox in late summer also showed a regional source (Fig. S6). Thus, SO2 oxidation into sulfate may proceed during long-range transport in late summer (Rodhe et al., 1981), consistent with our results in Fig. 3.
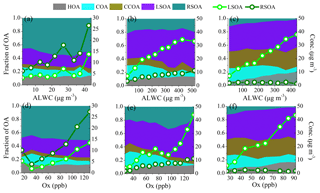
Figure 7Variations in the mass fractions and mass concentrations of LSOA and RSOA as functions of ALWC or Ox in (a, d) late summer, (b, e) autumn and (c, f) early winter. The data were binned according to the ALWC concentration (5 µg m−3 increment in late summer, 50 µg m−3 increment in autumn and early winter) and Ox concentration (20 ppb increment in late summer, 10 ppb increment in autumn and early winter).
We further investigated the formation mechanisms of SOA during different seasons. Figure 7 shows the effects of ALWC and Ox on the mass concentrations and mass fractions of LSOA and RSOA during different seasons. During late summer, the ALWC ranged from 2.1 to 53.6 µg m−3; both the mass concentrations of LSOA and RSOA increased as ALWC increased when ALWC was higher than ∼25–35 µg m−3. In comparison, the ALWC concentrations during autumn and early winter were much higher than that during late summer, and the increasing trends of SOA were much obvious than that during late summer. The mass concentrations of LSOA and RSOA increased from 7.3 to 33.3 and 3.5 to 11.5 µg m−3, respectively, when ALWC increased from 12.3 to 519.6 µg m−3, and the mass fraction of SOA increased from 30 % to 38 % during autumn. In comparison, during winter, the mass concentration of LSOA increased from 5.6 to 37.9 µg m−3 when ALWC increased from 9.7 to 436.6 µg m−3 with the mass fraction of LSOA increasing from 37 % to 42 %. RSOA displayed no clear increase trend with ALWC as it played a minor contribution during early winter. These variations indicated the influence of aqueous-phase processes on the formation of SOA especially during autumn and early winter with higher ALWC. Variations in the mass concentrations and fractions of LSOA and RSOA as functions of Ox during different seasons are also shown in Fig. 7. The mass concentrations of SOA increased clearly with the increase in Ox concentration during all three seasons, and the mass fraction of SOA also increased from 64 % to 76 % during late summer and increased from 59 % to 80 % during autumn as Ox increased from 30 to 120 ppb. Similar to that of ALWC, the increasing rates of LSOA and RSOA as functions of Ox were substantially different among different seasons. In late summer, both LSOA and RSOA presented linear increases with the increase in Ox. As a comparison, LSOA showed higher increase rates with Ox than that of RSOA during autumn and early winter as LSOA played a dominant role in the haze formation during autumn and early winter. These results clearly indicate that both photochemical processing and aqueous-phase reactions played important roles in the formation of SOA during all three seasons.
In this study, an ACSM combined with an aethalometer were applied for real-time measurements of PM1 species (organics, sulfate, nitrate, ammonium, chloride and BC) from 15 August to 4 December 2015 in Beijing. The average mass concentration of PM1 varied from 21.6 in late summer to 64.3 µg m−3 in early winter, indicating that PM pollution was very serious in wintertime due to enhanced emissions, low temperatures and stagnant meteorological conditions. OA contributed the major fraction (46 %–64 %) to PM1 mass during all three seasons, followed by nitrate (6 %–22 %) or sulfate (11 %–15 %). Regarding the OA factors, three primary OAs (HOA, COA and CCOA) and two secondary OAs (LSOA and RSOA) were resolved. Seasonal variations suggested that SOA dominated OA during late summer and autumn, whereas POA played a more important role in early winter due to the dramatically increased fraction of CCOA in the heating season (from 5 % in late summer to 26 % in early winter). A higher RSOA fraction (48 % of OA) in late summer and higher LSOA fractions in autumn (43 % of OA) and early winter (41 % of OA) and different correlations between RSOA and sulfate were found in our study, suggesting that regional transport played a more important role in SOA and sulfate sources in late summer, while local formation was important in winter due to heating.
Haze evolution and formation mechanisms of PM1 were also discussed. Results suggested that secondary aerosol species including SIA (sulfate, nitrate and ammonium) and SOA (LSOA and RSOA) dominated PM1 species during all three seasons with fractions of 72 %, 71 % and 66 % during late summer, autumn and early winter, respectively. SOA had a dominant contribution to PM1 in late summer, while SIA played a key role during autumn and early winter. Higher contributions of secondary species (SIA and SOA) further observed in pollution episodes emphasized the importance of the secondary formation processes in haze pollution in Beijing. We explored the formation mechanisms of secondary aerosol during different seasons and found that both photochemical processing and aqueous-phase processing played important roles in SOA formation during all three seasons. In comparison, for sulfate formation, both photochemical oxidation and aqueous-phase reaction had contributions during autumn, while photooxidation played a more important role during late summer and aqueous-phase reactions were more responsible during early winter.
Raw data used in this study are archived at the Institute of Earth Environment, Chinese Academy of Sciences, and are available on request by contacting the corresponding author.
The supplement related to this article is available online at: https://doi.org/10.5194/acp-19-10319-2019-supplement.
RJH and JC designed the study. JD, YG, YW and HZ performed the online measurements. Data analysis and source apportionment were done by JD, RJH and CL. JD and RJH wrote the paper. JD and RJH interpreted data and prepared display items. All authors commented on and discussed the paper.
Douglas R. Worsnop is an employee of Aerodyne Research, Inc. (ARI), and an ACSM produced by Aerodyne was used in this study.
This article is part of the special issue “Regional transport and transformation of air pollution in eastern China”. It is not associated with a conference.
This work was supported by the National Natural Science Foundation of China (NSFC) under grant no. 91644219 and no. 41877408 and the National Key Research and Development Program of China (no. 2017YFC0212701). The authors acknowledge financial support from the Cross Innovative Team fund from the State Key Laboratory of Loess and Quaternary Geology (SKLLQG) (no. SKLLQGTD1801)
This research has been supported by the National Natural Science Foundation of China (NSFC) (grant nos. 91644219 and 41877408) and the National Key Research and Development Program of China (grant no. 2017YFC0212701) and the Cross Innovative Team fund from SKLLQG (grant no. SKLLQGTD1801).
This paper was edited by Luisa Molina and reviewed by two anonymous referees.
Allan, J. D., Williams, P. I., Morgan, W. T., Martin, C. L., Flynn, M. J., Lee, J., Nemitz, E., Phillips, G. J., Gallagher, M. W., and Coe, H.: Contributions from transport, solid fuel burning and cooking to primary organic aerosols in two UK cities, Atmos. Chem. Phys., 10, 647–668, https://doi.org/10.5194/acp-10-647-2010, 2010.
An, Z., Huang, R.-J., Zhang, R., Tie, X., Li, G., Cao, J., Zhou, W., Shi, Z., Han, Y., Gu, Z., and Ji, Y.: Severe haze in northern China: A synergy of anthropogenic emissions and atmospheric processes, P. Natl. Acad. Sci. USA, 116, 8657–8666, https://doi.org/10.1073/pnas.1900125116, 2019.
Canagaratna, M. R., Jayne, J. T., Jimenez, J. L., Allan, J. D., Alfarra, M. R., Zhang, Q., Onasch, T. B., Drewnick, F., Coe, H., Middlebrook, A., Delia, A., Williams, L. R., Trimborn, A. M., Northway, M. J., DeCarlo, P. F., Kolb, C. E., Davidovits, P., and Worsnop, D. R.: Chemical and microphysical characterization of ambient aerosols with the Aerodyne aerosol mass spectrometer, Mass Spectrom. Rev., 26, 185–222, https://doi.org/10.1002/mas.20115, 2007.
Canonaco, F., Crippa, M., Slowik, J. G., Baltensperger, U., and Prévôt, A. S. H.: SoFi, an IGOR-based interface for the efficient use of the generalized multilinear engine (ME-2) for the source apportionment: ME-2 application to aerosol mass spectrometer data, Atmos. Meas. Tech., 6, 3649–3661, https://doi.org/10.5194/amt-6-3649-2013, 2013.
Cao, J. J., Shen, Z. X., Chow, J. C., Watson, J. G., Lee, S. C., Tie, X. X., Ho, K. F., Wang, G. H., and Han, Y. M.: Winter and summer PM2.5 chemical compositions in fourteen Chinese cities, J. Air Waste Manage., 62, 1214–1226, https://doi.org/10.1080/10962247.2012.701193, 2012a.
Cao, J. J., Wang, Q., Chow, J. C., Watson, J. G., Tie, X., Shen, Z., Wang, P., and An, Z.: Impacts of aerosol compositions on visibility impairment in Xi'an, China, Atmos. Environ., 59, 559–566, https://doi.org/10.1016/j.atmosenv.2012.05.036, 2012b.
Chan, C. K. and Yao, X.: Air pollution in mega cities in China, Atmos. Environ., 42, 1–42, https://doi.org/10.1016/j.atmosenv.2007.09.003, 2008.
Crippa, M., DeCarlo, P. F., Slowik, J. G., Mohr, C., Heringa, M. F., Chirico, R., Poulain, L., Freutel, F., Sciare, J., Cozic, J., Di Marco, C. F., Elsasser, M., Nicolas, J. B., Marchand, N., Abidi, E., Wiedensohler, A., Drewnick, F., Schneider, J., Borrmann, S., Nemitz, E., Zimmermann, R., Jaffrezo, J.-L., Prévôt, A. S. H., and Baltensperger, U.: Wintertime aerosol chemical composition and source apportionment of the organic fraction in the metropolitan area of Paris, Atmos. Chem. Phys., 13, 961–981, https://doi.org/10.5194/acp-13-961-2013, 2013.
Crippa, M., Canonaco, F., Lanz, V. A., Äijälä, M., Allan, J. D., Carbone, S., Capes, G., Ceburnis, D., Dall'Osto, M., Day, D. A., DeCarlo, P. F., Ehn, M., Eriksson, A., Freney, E., Hildebrandt Ruiz, L., Hillamo, R., Jimenez, J. L., Junninen, H., Kiendler-Scharr, A., Kortelainen, A.-M., Kulmala, M., Laaksonen, A., Mensah, A. A., Mohr, C., Nemitz, E., O'Dowd, C., Ovadnevaite, J., Pandis, S. N., Petäjä, T., Poulain, L., Saarikoski, S., Sellegri, K., Swietlicki, E., Tiitta, P., Worsnop, D. R., Baltensperger, U., and Prévôt, A. S. H.: Organic aerosol components derived from 25 AMS data sets across Europe using a consistent ME-2 based source apportionment approach, Atmos. Chem. Phys., 14, 6159–6176, https://doi.org/10.5194/acp-14-6159-2014, 2014.
Dall'Osto, M., Ovadnevaite, J., Ceburnis, D., Martin, D., Healy, R. M., O'Connor, I. P., Kourtchev, I., Sodeau, J. R., Wenger, J. C., and O'Dowd, C.: Characterization of urban aerosol in Cork city (Ireland) using aerosol mass spectrometry, Atmos. Chem. Phys., 13, 4997–5015, https://doi.org/10.5194/acp-13-4997-2013, 2013.
DeCarlo, P. F., Kimmel, J. R., Trimborn, A., Northway, M. J., Jayne, J. T., Aiken, A. C., Gonin, M., Fuhrer, K., Horvath, T., Docherty, K. S., Worsnop, D. R., and Jimenez, J. L.: Field-deployable, high-resolution, time-of-flight aerosol mass spectrometer, Anal. Chem., 78, 8281–8289, https://doi.org/10.1021/ac061249n, 2006.
Elser, M., Huang, R.-J., Wolf, R., Slowik, J. G., Wang, Q., Canonaco, F., Li, G., Bozzetti, C., Daellenbach, K. R., Huang, Y., Zhang, R., Li, Z., Cao, J., Baltensperger, U., El-Haddad, I., and Prévôt, A. S. H.: New insights into PM2.5 chemical composition and sources in two major cities in China during extreme haze events using aerosol mass spectrometry, Atmos. Chem. Phys., 16, 3207–3225, https://doi.org/10.5194/acp-16-3207-2016, 2016.
Fountoukis, C. and Nenes, A.: ISORROPIA II: a computationally efficient thermodynamic equilibrium model for K+––––Na+–––Cl–H2O aerosols, Atmos. Chem. Phys., 7, 4639–4659, https://doi.org/10.5194/acp-7-4639-2007, 2007.
Fröhlich, R., Crenn, V., Setyan, A., Belis, C. A., Canonaco, F., Favez, O., Riffault, V., Slowik, J. G., Aas, W., Aijälä, M., Alastuey, A., Artiñano, B., Bonnaire, N., Bozzetti, C., Bressi, M., Carbone, C., Coz, E., Croteau, P. L., Cubison, M. J., Esser-Gietl, J. K., Green, D. C., Gros, V., Heikkinen, L., Herrmann, H., Jayne, J. T., Lunder, C. R., Minguillón, M. C., Močnik, G., O'Dowd, C. D., Ovadnevaite, J., Petralia, E., Poulain, L., Priestman, M., Ripoll, A., Sarda-Estève, R., Wiedensohler, A., Baltensperger, U., Sciare, J., and Prévôt, A. S. H.: ACTRIS ACSM intercomparison – Part 2: Intercomparison of ME-2 organic source apportionment results from 15 individual, co-located aerosol mass spectrometers, Atmos. Meas. Tech., 8, 2555–2576, https://doi.org/10.5194/amt-8-2555-2015, 2015.
Guo, S., Hu, M., Zamora, M. L., Peng, J., Shang, D., Zheng, J., Du, Z., Wu, Z., Shao, M., Zeng, L., Molina, M. J., and Zhang, R.: Elucidating severe urban haze formation in China, P. Natl. Acad. Sci. USA, 111, 17373–17378, https://doi.org/10.1073/pnas.1419604111, 2014.
He, K., Yang, F., Ma, Y., Zhang, Q., Yao, X., Chan, C. K., Cadle, S., Chan, T., and Mulawa, P.: The characteristics of PM2.5 in Beijing, China, Atmos. Environ., 35, 4959–4970, https://doi.org/10.1016/S1352-2310(01)00301-6, 2001.
He, L.-Y., Lin, Y., Huang, X.-F., Guo, S., Xue, L., Su, Q., Hu, M., Luan, S.-J., and Zhang, Y.-H.: Characterization of high-resolution aerosol mass spectra of primary organic aerosol emissions from Chinese cooking and biomass burning, Atmos. Chem. Phys., 10, 11535–11543, https://doi.org/10.5194/acp-10-11535-2010, 2010.
He, L.-Y., Huang, X.-F., Xue, L., Hu, M., Lin, Y., Zheng, J., Zhang, R., and Zhang, Y.-H.: Submicron aerosol analysis and organic source apportionment in an urban atmosphere in Pearl River Delta of China using high-resolution aerosol mass spectrometry, J. Geophys. Res.-Atmos., 116, D12304, https://doi.org/10.1029/2010JD014566, 2011.
Ho, K. F., Huang, R.-J., Kawamura, K., Tachibana, E., Lee, S. C., Ho, S. S. H., Zhu, T., and Tian, L.: Dicarboxylic acids, ketocarboxylic acids, α-dicarbonyls, fatty acids and benzoic acid in PM2.5 aerosol collected during CAREBeijing-2007: an effect of traffic restriction on air quality, Atmos. Chem. Phys., 15, 3111–3123, https://doi.org/10.5194/acp-15-3111-2015, 2015.
Hu, W., Hu, M., Hu, W., Jimenez, J. L., Yuan, B., Chen, W., Wang, M., Wu, Y., Chen, C., Wang, Z., Peng, J., Zeng, L., and Shao, M.: Chemical composition, sources, and aging process of submicron aerosols in Beijing: Contrast between summer and winter, J. Geophys. Res.-Atmos., 121, 1955–1977, https://doi.org/10.1002/2015JD024020, 2016.
Hu, W. W., Hu, M., Yuan, B., Jimenez, J. L., Tang, Q., Peng, J. F., Hu, W., Shao, M., Wang, M., Zeng, L. M., Wu, Y. S., Gong, Z. H., Huang, X. F., and He, L. Y.: Insights on organic aerosol aging and the influence of coal combustion at a regional receptor site of central eastern China, Atmos. Chem. Phys., 13, 10095–10112, https://doi.org/10.5194/acp-13-10095-2013, 2013.
Huang, R. J., Zhang, Y. L., Bozzetti, C., Ho, K. F., Cao, J. J., Han, Y. M., Daellenbach, K. R., Slowik, J. G., Platt, S. M., Canonaco, F., Zotter, P., Wolf, R., Pieber, S. M., Bruns, E. A., Crippa, M., Ciarelli, G., Piazzalunga, A., Schwikowski, M., Abbaszade, G., Schnelle-Kreis, J., Zimmermann, R., An, Z., Szidat, S., Baltensperger, U., Haddad, I. E., and Prevot, A. S. H.: High secondary aerosol contribution to particulate pollution during haze events in China, Nature, 514, 218–222, 2014.
Huang, X.-F., He, L.-Y., Hu, M., Canagaratna, M. R., Sun, Y., Zhang, Q., Zhu, T., Xue, L., Zeng, L.-W., Liu, X.-G., Zhang, Y.-H., Jayne, J. T., Ng, N. L., and Worsnop, D. R.: Highly time-resolved chemical characterization of atmospheric submicron particles during 2008 Beijing Olympic Games using an Aerodyne High-Resolution Aerosol Mass Spectrometer, Atmos. Chem. Phys., 10, 8933–8945, https://doi.org/10.5194/acp-10-8933-2010, 2010.
Huang, X.-F., He, L.-Y., Xue, L., Sun, T.-L., Zeng, L.-W., Gong, Z.-H., Hu, M., and Zhu, T.: Highly time-resolved chemical characterization of atmospheric fine particles during 2010 Shanghai World Expo, Atmos. Chem. Phys., 12, 4897–4907, https://doi.org/10.5194/acp-12-4897-2012, 2012.
IPCC: Climate Change 2013: The Physical Science Basis, Contribution of Working Group I to the Fifth Assessment Report of the Intergovernmental Panel on Climate Change, Cambridge University Press, Cambridge, UK and New York, NY, USA, 2013.
Jiang, Q., Sun, Y. L., Wang, Z., and Yin, Y.: Aerosol composition and sources during the Chinese Spring Festival: fireworks, secondary aerosol, and holiday effects, Atmos. Chem. Phys., 15, 6023–6034, https://doi.org/10.5194/acp-15-6023-2015, 2015.
Kaufman, Y. J., Tanre, D., and Boucher, O.: A satellite view of aerosols in the climate system, Nature, 419, 215–223, https://doi.org/10.1038/nature01091, 2002.
Lanz, V. A., Alfarra, M. R., Baltensperger, U., Buchmann, B., Hueglin, C., and Prévôt, A. S. H.: Source apportionment of submicron organic aerosols at an urban site by factor analytical modelling of aerosol mass spectra, Atmos. Chem. Phys., 7, 1503–1522, https://doi.org/10.5194/acp-7-1503-2007, 2007.
Lelieveld, J., Evans, J. S., Fnais, M., Giannadaki, D., and Pozzer, A.: The contribution of outdoor air pollution sources to premature mortality on a global scale, Nature, 525, 367–371, 2015.
Li, H., Zhang, Q., Zhang, Q., Chen, C., Wang, L., Wei, Z., Zhou, S., Parworth, C., Zheng, B., Canonaco, F., Prévôt, A. S. H., Chen, P., Zhang, H., Wallington, T. J., and He, K.: Wintertime aerosol chemistry and haze evolution in an extremely polluted city of the North China Plain: significant contribution from coal and biomass combustion, Atmos. Chem. Phys., 17, 4751–4768, https://doi.org/10.5194/acp-17-4751-2017, 2017.
Li, Y. J., Sun, Y., Zhang, Q., Li, X., Li, M., Zhou, Z., and Chan, C. K.: Real-time chemical characterization of atmospheric particulate matter in China: A review, Atmos. Environ., 158, 270–304, https://doi.org/10.1016/j.atmosenv.2017.02.027, 2017.
Ma, J., Chen, Y., Wang, W., Yan, P., Liu, H., Yang, S., Hu, Z., and Lelieveld, J.: Strong air pollution causes widespread haze-clouds over China, J. Geophys. Res., 115, D18204, https://doi.org/10.1029/2009JD013065, 2010.
Middlebrook, A. M., Bahreini, R., Jimenez, J. L., and Canagaratna, M. R.: Evaluation of composition-dependent collection efficiencies for the Aerodyne aerosol mass spectrometer using field data, Aerosol Sci. Tech., 46, 258–271, https://doi.org/10.1080/02786826.2011.620041, 2012.
Mohr, C., DeCarlo, P. F., Heringa, M. F., Chirico, R., Slowik, J. G., Richter, R., Reche, C., Alastuey, A., Querol, X., Seco, R., Peñuelas, J., Jiménez, J. L., Crippa, M., Zimmermann, R., Baltensperger, U., and Prévôt, A. S. H.: Identification and quantification of organic aerosol from cooking and other sources in Barcelona using aerosol mass spectrometer data, Atmos. Chem. Phys., 12, 1649–1665, https://doi.org/10.5194/acp-12-1649-2012, 2012.
Molina, L. T., Kolb, C. E., de Foy, B., Lamb, B. K., Brune, W. H., Jimenez, J. L., Ramos-Villegas, R., Sarmiento, J., Paramo-Figueroa, V. H., Cardenas, B., Gutierrez-Avedoy, V., and Molina, M. J.: Air quality in North America's most populous city – overview of the MCMA-2003 campaign, Atmos. Chem. Phys., 7, 2447–2473, https://doi.org/10.5194/acp-7-2447-2007, 2007.
Molina, L. T., Gallardo, L., Andrade, M., Baumgardner, D., Borbor-Cordova, M., Borquez, R., Casassa, G., Cereceda-Balic, F., Dawidowski, L., Garreaud, R., Huneeus, N., Lambert, F., McCarty, J. L., Mc Phee, J., Mena-Carrasco, M., Raga, G. B., Schmitt, C., and Schwarz, J. P.: Pollution and its impacts on the South American cryosphere, Earth's Future, 3, 345–369, https://doi.org/10.1002/2015EF000311, 2015.
Ng, N. L., Herndon, S. C., Trimborn, A., Canagaratna, M. R., Croteau, P. L., Onasch, T. B., Sueoer, D., Worsnop, D. R., Zhang, Q., Sun, Y. L., and Jayne, J. T.: An Aerosol Chemical Speciation Monitor (ACSM) for routine monitoring of the composition and mass concentrations of ambient aerosol, Aerosol Sci. Tech., 45, 770–784, https://doi.org/10.1080/02786826.2011.560211, 2011a.
Ng, N. L., Canagaratna, M. R., Jimenez, J. L., Zhang, Q., Ulbrich, M., and Worsnop, D. R.: Real-time methods for estimating organic component mass concentrations from aerosol mass spectrometer data, Environ. Sci. Technol., 45, 910–916, https://doi.org/10.1021/es102951k, 2011b.
Paatero, P.: Least squares formulation of robust non-negative factor analysis, Chemometr. Intell. Lab., 37, 23–35, https://doi.org/10.1016/S0169-7439(96)00044-5, 1997.
Paatero, P. and Tapper, U.: Positive Matrix Factorization: A Non-Negative Factor Model with Optimal Utilization of Error Estimates of Data Values, Environmetrics, 5, 111–126, https://doi.org/10.1002/env.3170050203, 1994.
Pope, C. A., Burnett, R. T., Thun, M. J., Calle, E. E., Krewski, D., Ito, K., and Thurston, G. D.: Lung cancer, cardiopulmonary mortality, and long-term exposure to fine particulate air pollution, J. Am. Med. Assoc., 287, 1132–1141, 2002.
Rodhe, H., Crutzen, P., and Vanderpol, A.: Formation of Sulfuric and Nitric-Acid in the Atmosphere during Long-Range Transport, Tellus, 33, 132–141, 1981.
Seinfeld, J. H. and Pandis, S. N.: Atmospheric chemistry and physics: from air pollution to climate change, John Wiley & Sons, 2016.
Streets, D. G., Fu, J. S., Jang, C. J., Hao, J., He, K., Tang, X., Zhang, Y., Wang, Z., Li, Z., Zhang, Q., Wang, L., Wang, B., and Yu, C.: Air quality during the 2008 Beijing Olympic games, Atmos. Environ., 41, 480–492, https://doi.org/10.1016/j.atmosenv.2006.08.046, 2007.
Sun, J., Zhang, Q., Canagaratna, M. R., Zhang, Y., Ng, N. L., Sun, Y., Jayne, J. T., Zhang, X., Zhang, X., and Worsnop, D. R.: Highly time- and size-resolved characterization of submicron aerosol particles in Beijing using an Aerodyne Aerosol Mass Spectrometer, Atmos. Environ., 44, 131–140, https://doi.org/10.1016/j.atmosenv.2009.03.020, 2010.
Sun, Y., Zhuang, G., Tang, A., Wang, Y., and An, Z.: Chemical characteristics of PM2.5 and PM10 in haze-fog episodes in Beijing, Environ. Sci. Technol., 40, 3148–3155, https://doi.org/10.1021/es051533g, 2006.
Sun, Y., Jiang, Q., Wang, Z., Fu, P., Li, J., Yang, T., and Yin, Y.: Investigation of the sources and evolution processes of severe haze pollution in Beijing in January 2013, J. Geophys. Res.-Atmos., 119, 4380–4398, https://doi.org/10.1002/2014JD021641, 2014.
Sun, Y., Du, W., Fu, P., Wang, Q., Li, J., Ge, X., Zhang, Q., Zhu, C., Ren, L., Xu, W., Zhao, J., Han, T., Worsnop, D. R., and Wang, Z.: Primary and secondary aerosols in Beijing in winter: sources, variations and processes, Atmos. Chem. Phys., 16, 8309–8329, https://doi.org/10.5194/acp-16-8309-2016, 2016.
Sun, Y., Xu, W., Zhang, Q., Jiang, Q., Canonaco, F., Prévôt, A. S. H., Fu, P., Li, J., Jayne, J., Worsnop, D. R., and Wang, Z.: Source apportionment of organic aerosol from 2-year highly time-resolved measurements by an aerosol chemical speciation monitor in Beijing, China, Atmos. Chem. Phys., 18, 8469–8489, https://doi.org/10.5194/acp-18-8469-2018, 2018.
Sun, Y. L., Wang, Z., Dong, H., Yang, T., Li, J., Pan, X., Chen, P., and Jayne, J. T.: Characterization of summer organic and inorganic aerosols in Beijing, China with an Aerosol Chemical Speciation Monitor, Atmos. Environ., 51, 250–259, https://doi.org/10.1016/j.atmosenv.2012.01.013, 2012.
Sun, Y. L., Wang, Z. F., Fu, P. Q., Yang, T., Jiang, Q., Dong, H. B., Li, J., and Jia, J. J.: Aerosol composition, sources and processes during wintertime in Beijing, China, Atmos. Chem. Phys., 13, 4577–4592, https://doi.org/10.5194/acp-13-4577-2013, 2013.
Sun, Y. L., Wang, Z. F., Du, W., Zhang, Q., Wang, Q. Q., Fu, P. Q., Pan, X. L., Li, J., Jayne, J., and Worsnop, D. R.: Long-term real-time measurements of aerosol particle composition in Beijing, China: seasonal variations, meteorological effects, and source analysis, Atmos. Chem. Phys., 15, 10149–10165, https://doi.org/10.5194/acp-15-10149-2015, 2015.
Takegawa, N., Miyakawa, T., Kuwata, M., Kondo, Y., Zhao, Y., Han, S., Kita, K., Miyazaki, Y., Deng, Z., Xiao, R., Hu, M., van Pinxteren, D., Herrmann, H., Hofzumahaus, A., Holland, F., Wahner, A., Blake, D. R., Sugimoto, N., and Zhu, T.: Variability of submicron aerosol observed at a rural site in Beijing in the summer of 2006, J. Geophys. Res., 114, D00G05, https://doi.org/10.1029/2008jd010857, 2009.
Tao, M., Chen, L., Su, L., and Tao, J.: Satellite observation of regional haze pollution over the North China Plain, J. Geophys. Res., 117, D12203, https://doi.org/10.1029/2012JD017915, 2012.
Thornhill, D. A., Williams, A. E., Onasch, T. B., Wood, E., Herndon, S. C., Kolb, C. E., Knighton, W. B., Zavala, M., Molina, L. T., and Marr, L. C.: Application of positive matrix factorization to on-road measurements for source apportionment of diesel- and gasoline-powered vehicle emissions in Mexico City, Atmos. Chem. Phys., 10, 3629–3644, https://doi.org/10.5194/acp-10-3629-2010, 2010.
Tian, S., Pan, Y., Liu, Z., Wen, T., and Wang, Y.: Size-resolved aerosol chemical analysis of extreme haze pollution events during early 2013 in urban Beijing, China, J. Hazard. Mater., 279, 452–460, https://doi.org/10.1016/j.jhazmat.2014.07.023, 2014.
Ulbrich, I. M., Canagaratna, M. R., Zhang, Q., Worsnop, D. R., and Jimenez, J. L.: Interpretation of organic components from Positive Matrix Factorization of aerosol mass spectrometric data, Atmos. Chem. Phys., 9, 2891–2918, https://doi.org/10.5194/acp-9-2891-2009, 2009.
Volkamer, R., Jimenez, J. L., Martini, F. S., Dzepina, K., Zhang, Q., Salcedo, D., Molina, L. T., Worsnop, D. R., and Molina,M. J.: Secondary organic aerosol formation from anthropogenic air pollution: Rapid and higher than expected, Geophys. Res. Lett., 33, L17811, https://doi.org/10.1029/2006GL026899, 2006.
Wang, L., Liu, Z., Sun, Y., Ji, D., and Wang, Y.: Long-range transport and regional sources of PM2.5 in Beijing based on long-term observations from 2005 to 2010, Atmos. Res., 157, 37–48, https://doi.org/10.1016/j.atmosres.2014.12.003, 2015.
Wang, P., Cao, J. J., Shen, Z. X., Han, Y. M., Lee, S. C., Huang, Y., Zhu, C. S., Wang, Q. Y., Xu, H. M., and Huang, R.-J.: Spatial and seasonal variations of PM2.5: mass and species during 2010 in Xi'an, China, Sci. Total Environ., 508, 477–487, https://doi.org/10.1016/j.scitotenv.2014.11.007, 2015.
Wang, Q., Sun, Y., Jiang, Q., Du, W., Sun, C., Fu, P., and Wang, Z.: Chemical composition of aerosol particles and light extinction apportionment before and during the heating season in Beijing, China, J. Geophys. Res.-Atmos., 120, 12708–12722, https://doi.org/10.1002/2015JD023871, 2015.
Wang, Y. C., Huang, R. J., Ni, H. Y., Chen, Y., Wang, Q. Y., Li, G. H., Tie, X. X., Shen, Z. X., Huang, Y., Liu, S. X., Dong, W. M., Xue, P., Fröhlich, R., Canonaco, F., Elser, M., Daellenbach, K.R., Bozzetti, C., Haddad, EI., and Cao, J. J.: Chemical composition, sources and secondary processes of aerosols in Baoji city of northwest China, Atmos. Environ., 158, 128–137, https://doi.org/10.1016/j.atmosenv.2017.03.026, 2017.
Xu, W. Q., Sun, Y. L., Chen, C., Du, W., Han, T. T., Wang, Q. Q., Fu, P. Q., Wang, Z. F., Zhao, X. J., Zhou, L. B., Ji, D. S., Wang, P. C., and Worsnop, D. R.: Aerosol composition, oxidation properties, and sources in Beijing: results from the 2014 Asia-Pacific Economic Cooperation summit study, Atmos. Chem. Phys., 15, 13681–13698, https://doi.org/10.5194/acp-15-13681-2015, 2015.
Xu, Z. J., Wen, T. X., Li, X. R., Wang, J. G., and Wang, Y. S.: Characteristics of carbonaceous aerosols in Beijing based on two-year observation, Atmos. Pollut. Res., 6, 202–208, https://doi.org/10.5094/APR.2015.024, 2015.
Yang, Y., Liu, X., Qu., Y., Wang, J., An, J., Zhang, Y., and Zhang, F.: Formation mechanism of continuous extreme haze episodes in the megacity Beijing, China, in January 2013, Atmos. Res., 155, 192–203, https://doi.org/10.1016/j.atmosres.2014.11.023, 2015.
Zhang, H., Wang, S., Hao, J., Wan, L., Jiang, J., Zhang, M., Mestl, H. E. S., Alnes, L. W. H., Aunan, K., and Mellouki, A. W.: Chemical and size characterization of particles emitted from the burning of coal and wood in rural households in Guizhou, China, Atmos. Environ., 51, 94–99, https://doi.org/10.1016/j.atmosenv.2012.01.042, 2012.
Zhang, R. Y., Wang, G. H., Guo, S., Zamora, M. L., Ying, Q., Lin, Y., Wang, W. G., Hu, M., and Wang, Y.: Formation of urban fine particulate matter. Chem. Rev., 115, 3803–3855, https://doi.org/10.1021/acs.chemrev.5b00067, 2015.
Zhang, Y., Tang, L., Croteau, P. L., Favez, O., Sun, Y., Canagaratna, M. R., Wang, Z., Couvidat, F., Albinet, A., Zhang, H., Sciare, J., Prévôt, A. S. H., Jayne, J. T., and Worsnop, D. R.: Field characterization of the PM2.5 Aerosol Chemical Speciation Monitor: insights into the composition, sources, and processes of fine particles in eastern China, Atmos. Chem. Phys., 17, 14501–14517, https://doi.org/10.5194/acp-17-14501-2017, 2017.
Zheng, G. J., Duan, F. K., Su, H., Ma, Y. L., Cheng, Y., Zheng, B., Zhang, Q., Huang, T., Kimoto, T., Chang, D., Pöschl, U., Cheng, Y. F., and He, K. B.: Exploring the severe winter haze in Beijing: the impact of synoptic weather, regional transport and heterogeneous reactions, Atmos. Chem. Phys., 15, 2969–2983, https://doi.org/10.5194/acp-15-2969-2015, 2015.
Zhao, J., Du, W., Zhang, Y., Wang, Q., Chen, C., Xu, W., Han, T., Wang, Y., Fu, P., Wang, Z., Li, Z., and Sun, Y.: Insights into aerosol chemistry during the 2015 China Victory Day parade: results from simultaneous measurements at ground level and 260 m in Beijing, Atmos. Chem. Phys., 17, 3215–3232, https://doi.org/10.5194/acp-17-3215-2017, 2017.
Zhao, X. J., Zhao, P. S., Xu, J., Meng,, W., Pu, W. W., Dong, F., He, D., and Shi, Q. F.: Analysis of a winter regional haze event and its formation mechanism in the North China Plain, Atmos. Chem. Phys., 13, 5685–5696, https://doi.org/10.5194/acp-13-5685-2013, 2013.