the Creative Commons Attribution 4.0 License.
the Creative Commons Attribution 4.0 License.
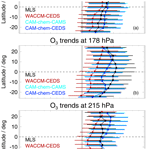
Tropical upper-tropospheric trends in ozone and carbon monoxide (2005–2020): observational and model results
Lucien Froidevaux
Douglas E. Kinnison
Benjamin Gaubert
Michael J. Schwartz
Nathaniel J. Livesey
William G. Read
Charles G. Bardeen
Jerry R. Ziemke
Ryan A. Fuller
We analyze tropical ozone (O3) and carbon monoxide (CO) distributions in the upper troposphere (UT) for 2005–2020 using Aura Microwave Limb Sounder (MLS) observations and simulations from the Whole Atmosphere Community Climate Model (WACCM) and two variants of the Community Atmosphere Model with Chemistry (CAM-chem), with each variant using different anthropogenic CO emissions. Trends and variability diagnostics are obtained from multiple linear regression. The MLS zonal mean O3 UT trend for 20° S–20° N is +0.39 ± 0.28 % yr−1; the WACCM and CAM-chem simulations yield similar trends, although the WACCM result is somewhat smaller. Our analyses of gridded MLS data yield positive O3 trends (up to 1.4 % yr−1) over Indonesia and east of that region, as well as over Africa and the Atlantic. These positive mapped O3 trends are generally captured by the simulations but in a more muted way. We find broad similarities (and some differences) between mapped MLS UT O3 trends and corresponding mapped trends of tropospheric column ozone. The MLS zonal mean CO UT trend for 20° S–20° N is −0.25 ± 0.30 % yr−1, while the corresponding CAM-chem trend is 0.0 ± 0.14 % yr−1 when anthropogenic emissions are taken from the Community Emissions Data System (CEDS) version 2. The CAM-chem simulation driven by CAMS-GLOB-ANTv5 emissions yields a tropical mean CO UT trend of 0.22 ± 0.19 % yr−1, in contrast to the slightly negative MLS CO trend. Previously published analyses of total column CO data have shown negative trends.
Our tropical composition trend results contribute to continuing international assessments of tropospheric evolution.
- Article
(16258 KB) - Full-text XML
-
Supplement
(2330 KB) - BibTeX
- EndNote
Tropospheric ozone (O3) can be influenced by downward transport from the stratospheric ozone layer, but the main O3 source in the troposphere is in situ photochemical formation through the oxidation of carbon compounds in the presence of (catalyzing) nitrogen oxides (NOx = NO + NO2) (Crutzen, 1973; Logan, 1985); tropospheric ozone loss is dominated by in situ photochemistry and by deposition at the Earth's surface (Monks et al., 2015). Past studies have also shown that the main sources of tropospheric NOx are fossil fuel combustion, biomass burning, soil microbial activity, and lightning. Global anthropogenic emissions dominate the natural NOx sources, and biomass burning plays quite a significant role in the tropics. There is evidence from in situ measurements from ozonesondes and commercial aircraft for slow increases in tropospheric and upper-tropospheric O3 abundances (e.g., Cooper et al., 2014; Gaudel et al., 2020; Thompson et al., 2021; Wang et al., 2022). At the surface, regional differences have been noted, for example, a leveling off in ozone increases over western Europe and parts of the United States after the 1990s, including some decreases, depending on the season. Changes in tropospheric ozone precursor emissions (e.g., from NOx; carbon monoxide, CO; and volatile organic compounds) have been implicated as causes for global tropospheric ozone change over the past few decades (Zhang et al., 2016; Zheng et al., 2018; Liu et al., 2022; Wang et al., 2022). Souri et al. (2017) and Zhang et al. (2016), for example, discussed the existence of decreases in NOx emissions over developed countries following emission regulations after the turn of the century. In the North Atlantic region, both surface O3 and CO have decreased; Kumar et al. (2013) showed this for 2001–2011. Such decreases have been attributed to a decline in anthropogenic emissions from North America that more than compensate for emission increases over parts of Asia. Furthermore, after the dramatic reduction in global economic activity following the coronavirus disease 2019 pandemic, significant reductions in Northern Hemisphere (NH) tropospheric ozone values were observed in 2020 and 2021, although the tropical decreases are much smaller (Ziemke et al., 2022; Steinbrecht et al., 2021; Bouarar et al., 2021; Miyazaki et al., 2021).
CO is another important pollutant in the troposphere. Its primary tropospheric sources are incomplete combustion (biomass burning emissions and pollution from industrial and traffic-related emissions) and the oxidation of methane and other hydrocarbons (Logan et al., 1981; Crutzen and Andreae, 1990; Khalil and Rasmussen, 1990); its main tropospheric loss pathway is oxidation by the hydroxyl radical (OH). Lower-tropospheric CO anomalies are propagated upward by convection and general ascent to produce a tropical “CO tape recorder” (Schoeberl et al., 2006), primarily as a result of biomass burning episodes near the equinoxes (Duncan et al., 2003, 2007; Logan et al., 2008; Nassar et al., 2009; Livesey et al., 2013; Huang et al., 2016). Further insights into the transport of CO pollution into the upper troposphere and lower stratosphere (UTLS) have been provided by Park et al. (2013), who examined CO and other species from the Atmospheric Chemistry Experiment Fourier Transform Spectrometer (ACE-FTS) and MLS. In the tropics, the clear signature of semiannual maxima centered around April and October was observed, primarily over Africa, Indonesia, and South America, with connections to biomass burning and convection patterns. Park et al. (2021) examined CO pollution transport to the UTLS during and long after the highly enhanced 2015 Indonesian fire season, using a combination of CO satellite data and model simulations (with the CAM-chem model). This model produced underestimates in CO comparisons versus tropical upper-tropospheric CO from MOPITT, as well as versus MLS and ACE-FTS CO data in this region; those retrievals compared well with MOPITT CO.
In terms of tropospheric CO trends, Worden et al. (2013a) found significant CO column decreases for the 2000–2011 period at a rate of −1.5 % yr−1 over Europe, East Asia, and the United States; this work was based mainly on data from the Measurements of Pollution in the Troposphere (MOPITT) and the Atmospheric Infrared Sounder (AIRS) (see also Warner et al., 2013). Using MOPITT data, Laken and Shahbaz (2014) obtained a significant global CO trend of −0.6 % yr−1 from 2000–2012; they also pointed to significant increasing trends over parts of Asia, South America, and Africa. Buchholz et al. (2021) found a similar result using 2002–2018 gridded time series from MOPITT CO, AIRS, and other satellite instruments; the global trend for this period was found to be −0.5 ± 0.3 % yr−1, with a slower decreasing trend during 2010–2018. Hedelius et al. (2021) also discussed MOPITT-inferred decreasing trends in column CO for 2002–2017 and pointed out that decreases in CO emissions, obtained from the Emissions Database for Global Atmospheric Research (EDGAR) version 4.3.2, do not always match column CO trends. Analyses of ground-based in situ surface CO data also point to a slowdown in the rate of decrease of CO after 2010, in comparison to the 2001–2010 decade (Patel et al., 2024). There is also a north–south interhemispheric difference in the CO abundances (and total columns), along with faster rates of decrease in the Northern Hemisphere. Decreasing CO emissions from anthropogenic and biomass burning sources appear to be the main cause of global tropospheric CO decreases (Jiang et al., 2017; Andela et al., 2017), while secondary CO resulting from methane oxidation is increasing (Gaubert et al., 2017). Some steeper CO decreases have been observed in local extra-tropical near-surface data (Li and Liu, 2011; He et al., 2013; Yoon and Pozzer, 2014; Gratz et al., 2015), apparently because of tighter air quality standards and reduced pollution from industrial and traffic-related emissions.
The upper troposphere is a complex region where production of NOx by lightning (Schumann and Huntrieser, 2007; Murray et al., 2014), aircraft NOx emissions (Hoor et al., 2009; Brasseur et al., 2016; Lee et al., 2021; Wang et al., 2022), and stratosphere–troposphere exchange (STE) (Sudo et al., 2003; Collins et al., 2003; Hegglin and Shepherd, 2009; Hess and Zbinden, 2013; Neu et al., 2014) can significantly impact ozone concentrations; STE plays a larger role in the extra-tropics than in the tropics (Hsu and Prather, 2014). Upper-tropospheric trend analyses of in situ CO data from commercial aircraft participating in the In-service Aircraft for a Global Observing System (IAGOS; see Petzold et al., 2015) measurements have indicated decreasing trends from 1995 to 2013 in northern midlatitude UT CO, with some larger (and statistically robust) trends as high as −2 % yr−1 to −3 % yr−1 over eastern Asia (Cohen et al., 2018). The UT ozone trends from the latter analyses were found to range between 0.25 and 0.45 ppbv yr−1; this reflects changes of order 0.4 % yr−1–0.8 % yr−1. In terms of variability, there are interannual composition changes in the troposphere and in the UTLS associated with ENSO (Chandra et al., 1998; Ziemke and Chandra, 2003; Nassar et al., 2009; Oman et al., 2011, 2013) and related sea surface temperature and pressure changes. It has long been known that this important mode of climate variability that originates in the Pacific Ocean, with alternating warm (El Niño) and cold (La Niña) phases, leads to disruptions in global circulation patterns and has impacts on fire and wetland emissions that affect tropospheric composition (Feely et al., 1987; Jones et al., 2001; Sudo and Takahashi, 2001; Duncan et al., 2003; Doherty et al., 2006; Calvo et al., 2010; Voulgarakis et al., 2015; Rowlinson et al., 2019).
How do changes in the upper troposphere relate to changes in the lower troposphere, such as changes in emissions? There have not been many such studies in the past, in large part because of the lack of well-sampled long-term data in the upper reaches of the troposphere, where ozone is of radiative significance. While this region is not directly connected to surface pollution, fast convection episodes in the tropics imply that there might well be some correlations between lower-tropospheric and upper-tropospheric abundances, and even for long-term trends. Long-range transport of pollution can, however, extend into the UT and also back downward with cross-continental impacts on surface pollution levels. Constraints on chemistry climate models are one important goal for studies of long-term measurements of upper-tropospheric composition. Such studies are also expected to contribute to continuing assessments of pollutant trends in the troposphere, such as the Tropospheric Ozone Assessment Report Phase II (TOAR-II), while related model simulations are of interest to continuing assessments of chemistry climate models (e.g., CMIP7).
Tropical upper-tropospheric profiles of O3 and CO have been measured on a continuous daily basis by the Microwave Limb Sounder on the Aura satellite, from a near-polar sun-synchronous orbit since late 2004. Here, we present results of trends and variability analyses of these data sets (from 2005–2020), along with a similar treatment of UT O3 and CO time series from two chemistry climate models, “specified dynamics” versions of the Whole Atmosphere Community Climate Model version 6 (WACCM6) and the Community Atmosphere Model with Chemistry (CAM-chem), both of which are configurations of the Community Earth System Model version 2.2 (CESM2.2). When using regression fits, as done here, to analyze broad-scale atmospheric time series, one should pay attention to likely drivers (e.g., ENSO) of variability in that region, since a better fitting of such variability can reduce the resulting trend uncertainties. Altogether, we use one WACCM simulation as well as two separate CAM-chem simulations (the latter two having different anthropogenic emission inputs for CO), as described in Sect. 2, where we provide more details about the MLS data and these model simulations. Section 3 focuses on the trend analysis methodology. In Sect. 4, we discuss the analysis results for O3 and then for CO; we review the UT climatologies for these species and some differences versus model simulations, and we discuss results from zonal mean and mapped trend analyses. We also place our results in the context of past analyses. We then finish with some brief conclusions in Sect. 5.
For both MLS and the chemistry climate models, we analyze monthly averaged zonal mean time series as well as monthly averaged longitude–latitude binned time series. The models have been designed to capture key dynamical and chemical processes well enough to be usefully compared to the observations. We focus on a region that is somewhat below the tropopause to minimize potential effects from stratosphere–troposphere exchange and to avoid results that might depend more on lower-stratospheric rather than tropospheric change.
2.1 Observations
The Aura MLS observational data set considered here is taken from 16 full years (2005 through 2020) of global composition measurements, with about 3500 vertical profiles per day per measured species. The MLS antenna performs scans of the atmospheric limb ahead of the Aura satellite in its near-polar sun-synchronous orbit. MLS measures daytime and nighttime thermal emission using microwave radiometers operating at frequencies near 118, 190, 240, and 640 GHz; a 2.5 THz module measured OH during the early part of the mission. The 240 GHz radiometer provides the standard O3 and CO measurements. For an overview of the MLS measurement technique, the reader is referred to Waters et al. (2006). Read et al. (2006) gave a description of the simulated MLS forward model and related spectra. The MLS retrievals (Livesey et al., 2006) use the optimal estimation approach (Rodgers, 2000); there is no assumption of atmospheric homogeneity along the line of sight (see Livesey and Read, 2000), and the retrievals make use of the MLS antenna's views along overlapping tangent rays during consecutive scans of the Earth's limb. The specifics of MLS data characterization and data quality, along with estimated errors and related information, can be found in the documentation by Livesey et al. (2022).
Here, we have used the latest data version from MLS, labeled version 5.0 or v5. More specifically, we use the binned MLS Level 3 data sets, with a latitude grid that includes the equatorial bin (−2 to +2°) and the 44 other adjacent 4° wide bins. In this work, we use monthly mean time series based on zonal averages as well as latitude bands divided into 12 longitude bins.
The typical number of MLS profiles in a monthly zonal mean 4° bin is of order 2400, and it is about 200 for each of the 12 mapped (monthly) longitude–latitude bins. Prior to averaging the MLS data, the standard MLS data quality screening criteria (Livesey et al., 2022) have been applied to all the O3 and CO Level 2 profiles; this screening removes only a very small fraction (typically 1 %–3 %) of the retrieved profiles. In the troposphere and stratosphere, the MLS O3 retrieval grid is defined by a subset of the pressure levels given by hPa, where n is the pressure level index number; for CO, the grid is twice as coarse, meaning that is used as an exponent in the above equation rather than . The bottom recommended levels for the O3 and CO retrievals are at 261 and 215 hPa, respectively. Our tropical analyses will focus on results between 215 and 147 hPa, in order to largely obtain upper-tropospheric results, as more influence from the stratosphere occurs as one gets closer to 100 hPa in the tropics. In the upper troposphere, the vertical resolution of the O3 and CO products is about 3 km and 5 km, respectively (Livesey et al., 2022). In this region, the single-profile precision (1σ random uncertainty) is 20–30 ppbv (meaning ∼ 35 %–50 %) for O3 and 15–20 ppbv (∼ 20 %–30 %) for CO. For our analyses of monthly MLS averages, the relevant precision for O3 and CO reduces to ∼ 0.5 ppbv (∼ 1 %) for 4° zonal means and ∼ 2 ppbv (∼ 4 %) for the gridded data using 30° longitude by 4° latitude bins. In addition, the methodology used by the MLS team to assess the aggregate effects of estimated errors in various input parameters, coupled with validation results (see Livesey et al., 2022), leads to systematic uncertainty estimates (1σ) of 5–12 ppbv (∼ 10 %–20 %) and 15–25 ppbv (∼ 20 %–35 %) for tropical upper-tropospheric O3 and CO, respectively.
Following validation work on UT MLS O3 and CO in the early few years since the Aura launch (Livesey et al., 2008), studies of UT MLS O3 by Livesey et al. (2013) focused on seasonal and interannual variability and comparisons versus ozonesonde data. Despite sampling differences between these measurement systems, the temporal patterns evident in the MLS UT O3 data were found to be generally well correlated with the in situ data over different low-latitude regions. Distinct seasonality was evident in O3 and CO (as well as MLS-derived ice water content) over South America and South Africa. Other patterns such as the “wave-one” pattern in tropical O3 (see Thompson et al., 2000, 2003; Wang et al., 2006) and double peaks in O3 variability over eastern equatorial Africa (with enhancements around May/June and September to November) were discussed; for MLS UT CO, distinct seasonal behavior was found, for example, in the Northern Hemisphere tropics, over eastern Asia, and across the Pacific (see also Huang et al., 2012). Livesey et al. (2013) and Huang et al. (2014) discussed the connection between emissions from intense fires over Indonesia in 2006 (following the El Niño-related drought) and dramatic concomitant enhancements in UT CO (from MLS data) over this region. This work has been expanded upon in analyses by Park et al. (2013, 2021) of the significant and long-lasting impacts of more recent El Niño-related droughts and wildfires on tropospheric and lower-stratospheric CO abundances.
Regarding MLS ozone, previous work has shown vertical oscillations in zonal mean MLS UTLS O3 profiles (e.g., see Livesey et al., 2022). There are also some biases in MLS tropical UT ozone values, which tend to be on the high side (by 10 %–20 %) with respect to ozonesonde data (see Hubert et al., 2016, Fig. 6), but the above issues are systematic in nature. While we think that neither these biases nor the small vertical oscillations (a few percent in magnitude in the region of interest here) would play a major role in changing our MLS UT trend results, given the trend uncertainties (discussed later), any time-dependent effect, if it exists, would be quite difficult to characterize or provide a fix for.
We also compare the CO simulations to CO data from Terra/MOPITT, obtained from multispectral retrievals (V9J) Level 3 dry air total column data, or XCO in ppbv (Deeter et al., 2022). The simulated CO values are smoothed by using the MOPITT a priori columns as well as the 10 layers a priori and averaging kernel profiles, as recommended for a quantitative comparison of modeled and MOPITT XCO.
2.2 Model simulations
We use the Whole Atmosphere Community Climate Model version 6 (WACCM6) and the Community Atmosphere Model with Chemistry (CAM-chem), both of which are components of the CESM2.2 (Danabasoglu et al., 2020). WACCM6 uses the high-top set of 70 model levels between the surface and the lower thermosphere (∼ 140 km), while CAM-chem uses 32 layers (low-top) that stop in the middle of the stratosphere (∼ 40 km). Both configurations run on a horizontal resolution that is 0.95° latitude × 1.25° longitude and share the same vertical grid in the troposphere, with a vertical resolution in the upper troposphere of about 1.2 km. Both CAM-chem and WACCM6 include the same representations of boundary layer processes, shallow convection, liquid cloud macrophysics, and cloud microphysics (Gettelman et al., 2019). Each model employs the same chemical mechanism processes (labeled TS1). The chemical scheme includes the Ox, NOx, HOx, ClOx, and BrOx families, along with CH4 and its degradation products, as well as primary non-methane hydrocarbons and related oxygenated organic compounds (Emmons et al., 2020). Reaction rates follow the JPL publication 19-5 recommendation (Burkholder et al., 2019). TS1 includes a total of 231 species and 583 chemical reactions broken down into 150 photolysis reactions, 403 gas-phase reactions, and 13 tropospheric and 17 stratospheric heterogeneous reactions. The photolytic reactions are based on both inline chemical modules and a lookup table approach (Kinnison et al., 2007). Secondary organic aerosols are represented through the volatility basis set approach (Tilmes et al., 2019). Comparisons of oxidants during the Korea–United States Air Quality (KORUS-AQ) experiment in South Korea led to a revision of the heterogeneous aerosol uptake of hydroperoxyl radicals (HO2) to produce H2O instead of H2O2 and a reduction of the coefficient (γ) from 0.2 to 0.1 (Gaubert et al., 2020).
To accurately represent weather conditions as well as the Quasi-Biennial Oscillation (QBO) and to reproduce various modes of middle atmospheric variability, both simulations are run in the specified dynamics (SD) mode. The model dynamical constraints are taken from meteorological fields provided by the Modern-Era Retrospective Analysis for Research and Applications version 2 or MERRA-2 (Gelaro et al., 2017). Contrary to the previous SD approach, the MERRA-2 fields, here the zonal and meridional winds and temperature, are first regridded to the model horizontal and vertical grids. The model nudging (Davis et al., 2022) is updated at every (30 min) time step using the closest 3-hourly MERRA-2 fields; nudging timescales are set at 6 h for the CAM-chem simulations and at 12 h for WACCM6. The 11-year solar cycle variability is taken from the Naval Research Laboratory's (NRL) solar model, namely the NRL Solar Spectral Irradiance version 2 (NRLSSI2; Coddington et al., 2016). Volcanic SO2 emissions (used in sulfate aerosol density calculations) are derived for significant volcanic eruptions using the Neely and Schmidt (2016) database updated through the year 2020. The model scenario used here is based on historical forcings (and recent updates) from the Coupled Model Intercomparison Project Phase 6 (Meinshausen et al., 2017). The forcings include greenhouse gases (CH4, N2O, and CO2) and organic halogens. After 2014, the greenhouse gas and organic halogen inputs follow the CMIP6 SSP5-8.5 scenario that projects inputs beyond 2014 (O'Neill et al., 2016; Riahi et al., 2017; Meinshausen et al., 2020).
The emissions used here are taken from CAMS-GLOB-ANT v5.1 in the simulation we refer to as CAM-chem-CAMS (CAMS is the Copernicus Atmosphere Monitoring Service) and CAMS-GLOB-ANT v5.3 in the WACCM simulation (labeled WACCM-CEDS) for all surface anthropogenic emissions (Soulie et al., 2024). CO anthropogenic emissions were found to be too low in South Asia and China (Gaubert et al., 2023), so these emissions were replaced by the Community Emissions Data System (CEDS) v2, presented in McDuffie et al. (2020), for the CAM-chem-CEDS simulation also analyzed here and for the only WACCM simulation used here. The CO anthropogenic emissions from the aforementioned versions 5.3 and 5.1 are almost identical; version 5.3 only includes updates to shipping emissions for years after 2017. As there are no differences in the NOx or volatile organic compound (VOC) emissions in all three simulations, we can exclude a change in CO trends between these simulations as a result of differences in CO chemical formation or sink. Daily biomass burning emissions are obtained from the Quick Fire Emissions Dataset (QFED) 2.5 (Darmenov and da Silva, 2014) in all three simulations.
The lightning NOx production and its role in ozone formation is reviewed by Verma et al. (2021). This study showed that most lightning activity occurs within deep convective clouds in the tropical and subtropical region. In our study, the emission of NO from lightning is based on the Price parameterization (Price and Rind, 1992; Price et al., 1997). This parameterization is dependent on cloud height, which includes a stronger dependence over land versus ocean (Emmons et al., 2010). The CAM-Chem and WACCM models used here derive tropical (and global) lightning NOx values of 2.34 (3.23) and 2.78 (4.11) Tg (N) yr−1, respectively (Table 1), with no significant trends over the course of these simulations. These global values are within the generally accepted global range of 3–8 Tg N yr−1 for lightning NO emission (Schumann and Huntrieser, 2007).
Table 1Some characteristics of the three chemistry climate model simulations used in this work.
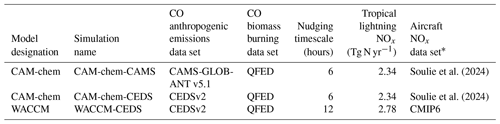
∗ For 2005–2014, the aircraft NOx emissions for WACCM-CEDS and both CAM-chem model simulations are identical. From 2015 onward, the WACCM-CEDS emissions are kept constant.
Aircraft emissions from CMIP6 were employed in WACCM6 (see Hoesly et al., 2018). Both CAM-chem simulations use the version 2.1 of CAMS-GLOB-AIR for aircraft emissions described by Soulie et al. (2024). Gaubert et al. (2020, 2023) found that this version of CAM-chem tends to overestimate tropospheric oxidants, such as ozone, hydrogen peroxide, nitric acid, and hydroxyl radical, resulting in a shorter lifetime of tropospheric methane and CO, mainly in the Northern Hemisphere extra-tropics. Some of the main model characteristics (with a focus on the differences) are summarized in Table 1.
In terms of the model run analyses, we follow the same basic approach as for the MLS data. The daily model profiles are first interpolated (as a function of log(pressure)) onto the MLS pressure grid and then binned and averaged to produce the monthly zonal means (on a 4° latitude grid) and gridded data on the same latitude–longitude grid as is described in Sect. 2.1 for MLS. We note also that we do not find much impact on the MLS versus model comparisons if we use a vertically smoothed version of the model profiles, which more properly takes into account the vertical resolution of the MLS observations, as the differences between smoothed and unsmoothed zonal mean values are much smaller than the model biases. For general simplicity, and for the above reasons, we use unsmoothed model values in this work. A more detailed example of smoothed model profile analyses is provided further below, in connection with observed seasonal CO differences between the models and the MLS measurements.
2.3 Trend analysis methods
For both MLS and model time series trend analyses in the upper troposphere, we use the multivariate linear regression (MLR) method discussed as part of similar studies performed by Froidevaux et al. (2019) for the stratosphere. We refer the reader to Appendix A3 of the above reference for more details on the regression fit model, which includes commonly used functional terms, namely a linear trend, and cosine and sine functions with annual and semi-annual periodicities, to account for these known variabilities in atmospheric composition, with 3- and 4-month periodic components to better fit shorter-term (intra-seasonal) variations, which also helps to reduce the trend error bars. In addition, we include functions describing multi-year variations caused by the QBO (which mostly affects the stratosphere) and by ENSO, which has been tied, for example, to regional droughts and biomass burning events, with related increases in convection and transport of surface pollution into the upper troposphere. The QBO-related equatorial wind data set is obtained from the publicly available data sets at the Free University of Berlin. ENSO-related data are in the form of a multivariate index, following the initial work of Wolter and Timlin (2011), as updated by Zhang et al. (2019). We have also included a fitted component that follows variations in solar radio flux (at 10.7 cm), based on Canadian solar measurements (Tapping, 2013); this component typically plays a negligible role in our results. For trend uncertainty estimates, as discussed also by Froidevaux et al. (2019, 2022), we use the block bootstrap resampling method (Efron and Tibshirani, 1993), as done by Bourassa et al. (2014) and others in such atmospheric composition analyses. For every fitted time series, we analyze thousands of resamplings of the fit residuals, with year-long blocks of residual values replaced by residual series from randomly chosen years; twice the standard deviations in these random distributions' trends provide the (2σ) trend uncertainty values that we use as trend error bars throughout this work.
3.1 Tropical UT O3
3.1.1 O3 climatologies
Although this work focuses on variability and underlying trends, we start in Fig. 1 by showing annually averaged climatological ozone comparisons between MLS, the WACCM-CEDS simulation, and the CAM-chem-CEDS simulation for 2005–2020 at 147 and 215 hPa for low latitudes (4° bin centers between 24° S and 24° N); mapped fields and zonal mean line plots are compared in this figure. At 215 hPa near 20° N and 20° S, the zonal mean O3 values from both models are ∼ 5 %–15 % lower than the MLS fields; differences of this order are also observed in the mapped fields. The differences reach about −20 % in the deep tropics, as the MLS latitudinal gradients are flat in this region, in contrast to the models' more curved behavior, with a minimum at the Equator (see panel (k)). The differences observed here are within the MLS systematic uncertainties mentioned in Sect. 2.1 (up to 24 ppbv, 2σ). These two models agree quite well in the UT region as a whole (typically within about 5 ppbv); such a good level of agreement is not too surprising, given that these models are based on a very similar framework, with nearly identical inputs (see Sect. 2.2). At smaller pressures (147 hPa and also for 100 hPa, which is not shown here), the models follow the MLS latitudinal gradients better (see panel (d) for the comparison at 147 hPa), as well as the longitudinal features (including the well-known wave-one ozone pattern discussed by Thompson et al., 2000, 2003; Wang et al., 2006; and others). However, the models exhibit a positive average bias versus MLS at these two pressure levels (see panel (e), where the model bias for 147 hPa is about +20 %). However, MLS UT O3 profiles have been found to be biased positively (by about 10 %–20 %) versus averaged tropical ozonesonde profiles (Sect. 2.1). Thus, positive model biases versus MLS ozone in the tropical UT are not likely caused by a significant underestimate by MLS. We note that the positive model biases (at 147 and 100 hPa) occur for all months of the year (not shown here), so this is not caused by a very large bias in some months, which could be partially compensated for by negative model biases in other months.
As mentioned previously, we focus on the upper-tropospheric region, somewhat removed from the tropopause, with 147 to 215 hPa being the main levels of interest in the analyses below; while the UT average differences between model and MLS are worth noting, this is not a primary concern in terms of the trend comparisons that we focus on here.
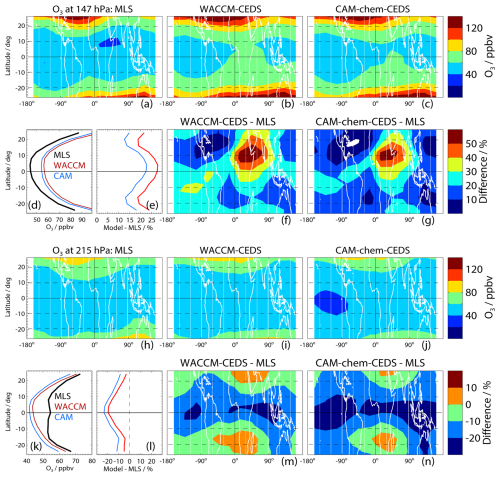
Figure 1Annually averaged climatological comparisons between MLS and model ozone fields for 2005-2020 at low latitudes (full range shown from 26° S to 26° N) at 147 hPa (a–g) and at 215 hPa (h–n). For 147 hPa: (a) climatological O3 maps from MLS, (b) from WACCM-CEDS, and (c) from CAM-chem-CEDS; panel (d) shows the zonal mean climatology from the MLS data and both model simulations, with panel (e) giving the differences in zonal means for both model simulations minus MLS (color-coded as shown in the panel (d) legend), while panel (f) provides a difference map of the climatologies from WACCM-CEDS minus MLS, and panel (g) gives the difference map for CAM-chem-CEDS minus MLS. Panels (h) through (n) provide the same information as panels (a) through (g) but for 215 hPa. We note that in panels (d) and (k), CAM is an abbreviation for the CAM-chem-CEDS simulation, and WACCM is an abbreviation for the WACCM-CEDS simulation.
3.1.2 O3 zonal mean trends
Figure S1 in the Supplement gives some time series examples for ozone at 12° N and 12° S at 147 and 215 hPa, with the MLS and modeled (WACCM-CEDS) series and their respective regression fits, along with the fitted trend lines. The linear correlation coefficients listed above each panel provide a measure of how well the chemistry climate model can fit the MLS series variability. The UT O3 WACCM-CEDS trends roughly follow the trends that are obtained from the MLS regression fits.
Regarding the ozone trends, we now switch to results from our analyses of the monthly zonal mean MLS and model time series. Figure 2 displays ozone trend results for MLS and the three simulations for 147, 178, and 215 hPa, based on a multiple linear regression analysis of the respective time series from 2005 through 2020. Figure 2 shows that the tropical upper-tropospheric MLS ozone trends are generally positive and significant (meaning that a zero trend lies outside the 2σ estimate of trend uncertainty). The observed average ozone trends at all three pressure levels lie within about 0.3 % yr−1 to 0.5 % yr−1; the peak average trends occur at 178 hPa. There are fairly small latitudinal differences at 178 and 215 hPa. At 147 hPa, the MLS results indicate ∼ 50 % larger trends in the NH tropics than in the SH tropics, although this difference is not significant. The zonal mean MLS ozone trend (averaging the three pressure levels at 147, 178, and 215 hPa) for 2005–2020 in the 20° S–20° N UT region is 0.39 ± 0.28 % yr−1. The error bars here indicate the 2σ trend uncertainty (calculated here as the root mean square of the 2σ trend uncertainties at all three pressure levels in Fig. 2). This tropical UT O3 trend is equivalent to 0.21 ± 0.15 ppbv yr−1 (based on the annual average tropical UT values of 56 ppbv measured by MLS). The corresponding model O3 zonal mean trend results obtained here for 2005–2020 have a positive trend, with excellent agreement with MLS from CAM-chem-CEDS (0.38 ± 0.28 % yr−1) and nearly identical results from CAM-chem-CAMS (0.38 ± 0.29 % yr−1). This agreement is also apparent in the latitudinal pattern, with larger trends in the NH than in the SH, even if the error bars are large enough that there is no statistically significant difference between the hemispheres. There is also good statistical agreement between the MLS zonal mean ozone trends and the slightly smaller WACCM-CEDS trends (0.21 ± 0.23 % yr−1).
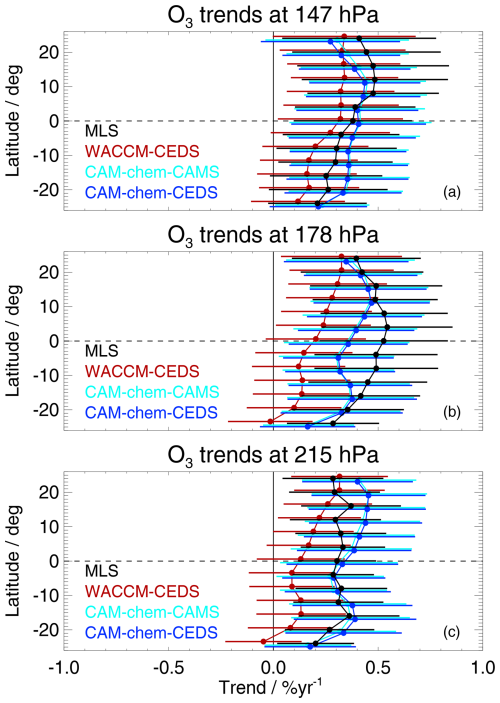
Figure 2Ozone zonal mean trends versus latitude in the tropical upper troposphere, for 2005–2020, based on MLR analyses of time series from MLS (black), WACCM-CEDS (red), CAM-chem-CAMS (cyan), and CAM-chem-CEDS (blue). Each row corresponds to a different pressure level: (a) for 147 hPa, (b) for 178 hPa, and (c) for 215 hPa, as labeled above each panel. Error bars give the uncertainties (2σ) in the estimated linear trends (see text for more details).
We note that statisticians have been working to guide common practices regarding statements of significance, and one should be sensitive to some of the broad differences that occur even within formal criteria (such as 2σ or a p level of 0.05), which could sometimes be interpreted too strictly (Wasserstein et al., 2019), as pointed out also by Y. Cohen (Yann Cohen, private communication, 17 May 2024). We keep this in mind here, but we also wish to comment on the use of broader latitude bins. Indeed, if broader latitude regions were analyzed for trends, the corresponding trend uncertainties would be reduced, which could make some of the compared trends differ by more than their 2σ error bar variability. However, the trend error reduction in our testing with a 20° wide latitude bin instead of 4° bins is only 5 %–10 %, so the uncertainties get divided by much less than the square root of the number of bins used. Thus, we do not readily obtain more significant differences in our comparisons by just averaging over broader regions. In Fig. 3, the MLS and CAM-chem-CEDS UT O3 trend sensitivity analysis is repeated for 2005–2018, 2005–2019, 2006–2020, and 2007–2020, showing the relative insensitivity of the MLS results to the choice of time period. This is also true for the CAM-chem-CEDS trends in the NH tropics, although there is more ozone trend sensitivity to the time period choice in this model's results over the SH tropics. The WACCM-CEDS tropical UT ozone trend results versus time period (not shown here) lead to a spread in the SH tropical trends that is about halfway between the small MLS trend spread and the larger CAM-chem-CEDS trend sensitivity shown in Fig. 3.
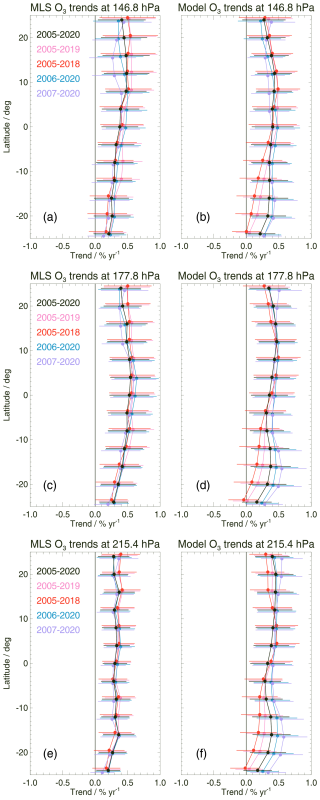
Figure 3Ozone zonal mean trends versus latitude in the tropical upper troposphere, with results from MLS data analyses shown in panels (a), (c), and (e) and model results from CAM-chem-CEDS shown in panels (b), (d), and (f). Each row corresponds to a different pressure level, as labeled. All panels show the trend sensitivity to the time period used in the regression fits. For example, black is used to show the period from 2005 through 2020; results from four other time periods are also shown, with the start or end year shifted by 1 or 2 years (see legend for the meaning of the various colors). The error bars given here represent the (2σ) uncertainties in the estimated linear trends.
3.1.3 O3 mapped trends and variability
We now turn to the mapped tropical UT trends by analyzing subsets of the O3 fields from MLS and the models, based on monthly mean time series for 2005–2020 in latitude–longitude bins rather than on zonal means. As mentioned previously, these bins are also 4° wide in latitude, and the longitude bins are 30° wide. The same regression methodology as described previously here is used for each of the binned time series; we focus on the WACCM-CEDS and CAM-chem-CEDS ozone trends, as we have found that the CAM-chem-CAMS and CAM-chem-CEDS results are quite similar, in the case of ozone at least. Figure 4 shows the resulting mapped O3 trends from MLS and the two models for 147 and 215 hPa (top and bottom rows, respectively), with the maps spanning 26° S to 26° N. Hatched bins indicate trends for which the 2σ uncertainty range encompasses the zero trend value which is often interpreted as a low level of statistical significance, although one should be cautious (see the previous section) regarding the strict application of such a criterion or wording. The largest MLS trends are observed over the Indonesian region and (mostly) to the east of that region, as well as over the northern Atlantic. The mapped trends confirm the overall zonal mean result of slightly larger O3 trends in MLS than in WACCM-CEDS. Broad regions with positive tendencies are observed in both model trend results; these regions include Southeast Asia, Indonesia, northern Australia, the Atlantic, and northern Africa, with some, but not exact, agreement with the regions mentioned above for the larger MLS trends. At 215 hPa, the slightly larger positive trends in CAM-chem-CEDS than in WACCM-CEDS over the Australian region (bottom-right quadrant, south of the Equator) contribute to the better correspondence between the zonal mean O3 trend results (Fig. 2c) between CAM-chem-CEDS and MLS over the southern tropics. The mapped trend discrepancies between the simulations and MLS are rarely outside the 2σ error bar ranges. Nevertheless, some of the discrepancies are worth noting, especially when they cover multiple adjacent bins; in particular, the easternmost longitude band shows MLS trends with (significant) positive values, in contrast to the simulation results, with binned trends that are often small and/or negative.
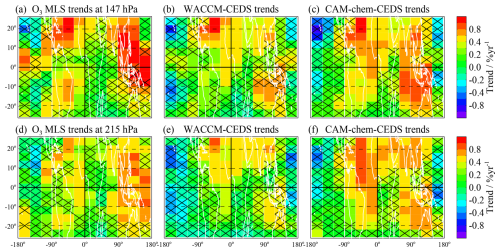
Figure 4Maps of upper-tropospheric O3 trends (% yr−1) in the tropics for 147 hPa (a–c) and 215 hPa (d–f); the latitude range is from 26° S to 26° N, with maps all centered on the Greenwich meridian. MLS trends (left column) are compared to trends from WACCM-CEDS (middle column) and CAM-chem-CEDS (right column). Black crosses show grid boxes for which the trend estimate is not significantly different from zero (based on our 2σ error estimates).
We have compared these mapped ozone trend results to those for tropospheric column ozone (TCO) obtained by Ziemke et al. (2019), using a combination of total O3 columns from the Aura Ozone Monitoring Instrument (OMI) and MLS-based stratospheric O3 columns. In Fig. 5, we show in the top two rows the trends from MLS ozone at 178 hPa (top map) versus the bottom map which provides the mapped TCO trends for the same time period, arrived at from appropriate horizontal smoothing of the results obtained following the above reference, to make the MLS and TCO horizontal resolutions comparable; this smoothing comes from an interpolation versus latitude and a weighted averaging in longitude, since the TCO results have finer longitudinal resolution (5° wide bins) than the MLS longitudinal grid used here (30° wide bins). Similarities are observed in the longitudinal pattern of UT MLS O3 and TCO trends, as shown also for three different latitude bins in panel (c) of Fig. 5; variations of a factor of 2 to 3 are observed, mostly in the northern half, between the Western Hemisphere and Eastern Hemisphere for both sets of trends, which tend to lie between roughly 0.3 % yr−1 and 1.2 % yr−1. However, the agreement between MLS UT O3 and TCO trends is often worse for other MLS pressure level choices; this can be deduced from panel (d), where R (correlation coefficient) values relating to the longitudinal variations obtained from MLS at different pressures versus the longitudinal variations in TCO are displayed as a function of latitude (y axis). In fact, one might not expect the MLS ozone UT trends to track the TCO trends very well, given that TCO measures the entire column, whereas MLS measures trends in a vertical region about 5 km wide in the upper troposphere, but this was worth looking into. Regional variability and horizontal sampling differences between MLS and OMI will also play a role (see Thompson et al., 2021, for variability aspects of sonde-derived tropospheric trends). Our comparisons imply that the correlation between lower-tropospheric and upper-tropospheric ozone trends is not a strict one-to-one mapping, but there are nevertheless some similarities between these regions.
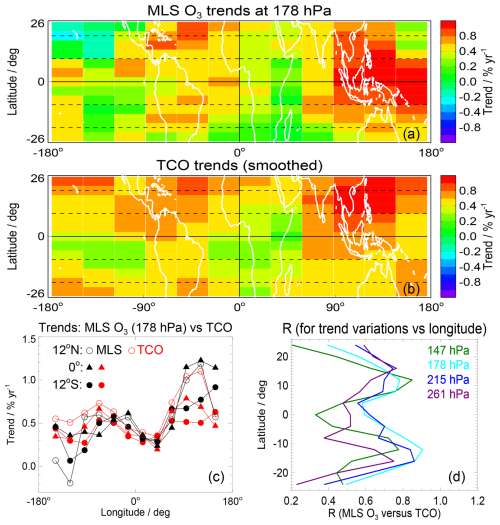
Figure 5(a) The top map shows MLS ozone trends (2005–2020) at 178 hPa; (b) the bottom map displays horizontally smoothed tropospheric column ozone trends for the same time period, following the analyses of Ziemke et al. (2019); (c) cross sections of the above mapped trends in 4° wide latitude bins centered at 12° N, 0°, and 12° S (see legend) for MLS (black) and TCO (red); and (d) correlation coefficient values R (on the x axis) between the MLS ozone trends at different pressures (see legend) and the TCO trends as a function of longitude, at different tropical latitudes (y axis). This panel provides a broader picture of the trend correlations, which exhibit a minimum near the Equator and maxima near 12° S and 12° N.
We have also analyzed the level of explained variance in the regression fits for these binned trend results. Figure 6 shows the square of the correlation coefficient values (R2) as a function of latitude and longitude for different explanatory variables used in the binned O3 fits at 147 hPa, based on fit comparisons to the MLS series (top six panels), and for the regression fit versus the WACCM-CEDS series (bottom six panels). We have ignored the solar component in these plots as it was found to be of negligible importance; we display the remaining contributions, namely the annual, semi-annual, short-term (sum of the 3-month and 4-month terms), QBO, and ENSO terms, as well as the contribution from the full regression fit, which shows that most (but certainly not all) of the time series variance can be explained by such a regression model. The annual term and semi-annual terms can generally explain a large part of the variance, usually followed in importance by the ENSO term, over most of the Pacific. The QBO component is very small in the upper troposphere, even though it is a well-known and large contributor to stratospheric trace gas variability in the lowermost stratosphere. There is also a significant annual cycle in the tropical lowermost stratosphere related to variations in vertical velocities and in the Brewer–Dobson circulation (Randel et al., 2007; Witte et al., 2008). The R2 patterns observed in the MLS panels are reproduced in a broad sense by the fits to the community climate model (CCM), as shown in the bottom six panels; this is also a result of the close match between the CCM and the MLS O3 time series, shown earlier in this work. The ENSO model pattern for O3 does not match the MLS-derived pattern that well over Indonesia, but this comparison is generally better in the Pacific region between −90 and −180°. A somewhat weaker R2 value in the model simulation also exists in parts of the Eastern Hemisphere for the semi-annual term. The combination of these differences helps to explain the somewhat poorer overall fits (and variance contributions) for the model than for MLS. For the most part, it does not matter much which model run is used for these analyses or even which pressure level is used; indeed, the results at 215 hPa (see Fig. S5) are generally similar to those in Fig. 6.
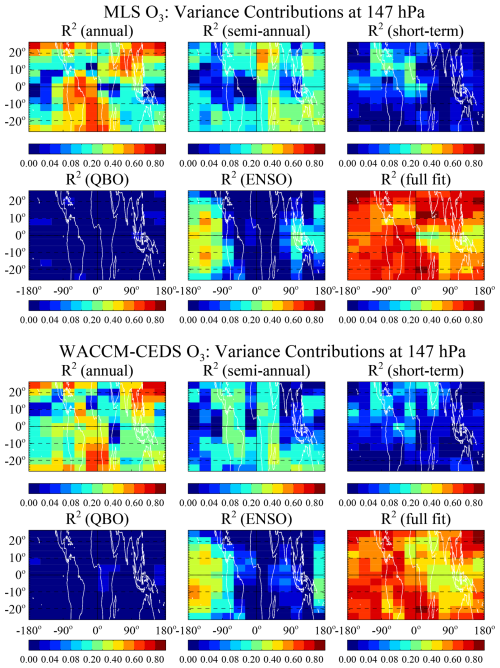
Figure 6Contributions to the time series variance from the main fitted components of the regression to the gridded tropical MLS ozone time series at 147 hPa (top six panels) and the same for the WACCM-CEDS time series (bottom six panels). The titles in each panel indicate that the explained variance is from specific components (annual, semi-annual, short term, meaning 3 and 4 months, QBO, ENSO, and full fit).
To pursue the ENSO-related patterns further, one can obtain a (mapped) sensitivity coefficient to ENSO from the regression fits regarding this component's importance in ppbv K−1 (where “K” relates to tropical sea surface temperatures changes). The O3 ENSO sensitivity is shown in Fig. 7 for the 2005–2020 MLS and WACCM-CEDS results at 147 and 215 hPa. This provides more information about the sign of the sensitivity over different regions, and we observe generally positive (negative) sensitivity in the Eastern (Western) Hemisphere, for both MLS and WACCM-CEDS cases; moreover, at 147 hPa, there are two strong negative minima on each side of the Equator in the central Pacific region. A positive change (or a negative change) in tropical Pacific sea surface temperatures during El Niño (La Niña) conditions will correlate with ozone increases (decreases) in the regions with positive (negative) sensitivity coefficients. The model results are quite consistent with those from MLS in terms of the ENSO-related sensitivity coefficient patterns and magnitudes, although the model response is often slightly smaller than seen in the MLS result. As we discuss further below, such ozone sensitivity patterns have been described and interpreted before. Figure 16 provides the same analysis but for the CO sensitivity to ENSO. These maps show a positive CO ENSO sensitivity coefficient throughout the tropics, with local maxima in both the Eastern Hemisphere and Western Hemisphere, rather than the O3 dipole (positive/negative) structure shown in Fig. 7. The model CO ENSO sensitivity broadly matches the MLS results, although it is not as strong; the different patterns in the Western Hemisphere, compared to the O3 sensitivity to ENSO, might be caused by differences in O3 and CO vertical profile gradients in these regions, but this would require further detailed investigations. We also note that, especially in the MLS case, the peak magnitudes of the CO ENSO sensitivity coefficients in Fig. 16 match the peak magnitudes of the positive O3 ENSO sensitivity in Fig. 7.
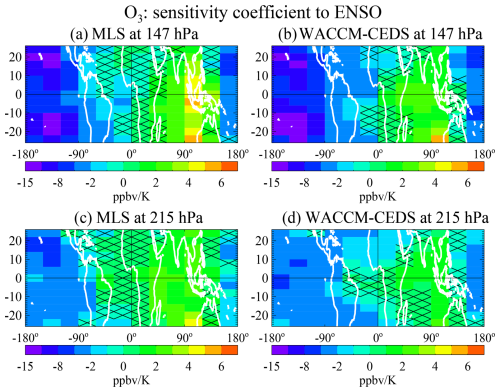
Figure 7Sensitivity coefficient to ENSO for ozone at 147 hPa (a, b) and 215 hPa (c, d); MLS results are shown in panels (a) and (c) and the WACCM-CEDS results in panels (b) and (d). The black crosses show the grid boxes for which the sensitivity is not significantly different from zero (based on the 2σ error estimates). Note that this color bar is asymmetric, with larger negative values than positive values.
3.1.4 O3 discussion
We have found some climatological differences between the MLS observations of O3 in the tropical upper troposphere and the WACCM-CEDS simulation, as well as both CAM-chem simulations. The models underestimate the mean MLS O3 values at 215 hPa; at 147 hPa, the models are biased high by about 15 %–25 %, and we have no reason to believe that such positive biases result from an average negative bias in the corresponding MLS values.
The averaged zonal mean tropical UT O3 trend from MLS for 2005–2020 is 0.39 ± 0.28 % yr−1 (or about 0.22 ± 0.16 ppbv yr−1), where the error bars indicate 2σ uncertainties. We note that the MLS ozone profile trend detection capability lies within the most stable among ozone sounders, based on the satellite- and ground-based ozone intercomparison work by Hubert et al. (2016). In addition, differences between stratospheric ozone columns from MLS and the Aura Ozone Monitoring Instrument (OMI) exhibit no significant drift (Ziemke et al., 2019), thus providing added confidence in the temporal stability of both measurement systems; also, we expect a similar level of confidence in the stability of MLS CO, since CO is retrieved using the same radiometer as the MLS standard ozone product. We get excellent agreement with MLS tropical UT zonal mean trends from the CAM-chem-CEDS O3 zonal mean trends (0.38 ± 0.28 % yr−1) and somewhat poorer agreement from the smaller WACCM-CEDS trends (0.21 ± 0.23 % yr−1). We also show that the zonal mean MLS O3 tropical UT trend results for different time periods, with start and end years adjusted by 1–2 years, do not significantly depart from the 2005–2020 results; there is more sensitivity to the choice of period in the CAM-chem-CEDS trend results over the southern tropics.
In terms of mapped ozone trends, the largest MLS-derived tropical trends (up to +1.4 % yr−1) are observed over Indonesia and east of that region, as well as over the northern Atlantic region. The mapped model O3 UT trends broadly match the MLS trends, albeit with somewhat smaller variations. The significant model maxima over Southeast Asia and the North Atlantic are similar to the significant MLS patterns in those regions. More qualitatively, the Indonesian region displays smaller model O3 trends than those derived from MLS data; parts of the western Pacific region exhibit some negative trends in the MLS and model trends, but not with good spatial correlation. The mapped MLS-based UT O3 trends and TCO trends for the same period (see Fig. 5), based on the analyses of Ziemke et al. (2019), provide good correlations in parts of the tropics, with similar values and longitudinal patterns; however, the MLS UT O3 trend maxima over the western Pacific are symmetric about the Equator, whereas the TCO maxima in that region are found in the northern part only. Since the TCO measurement weighting does not favor the UT region, we would not necessarily expect a really high correlation versus the MLS UT trends.
There have been large differences between past satellite-based tropospheric O3 trends (Gaudel et al., 2018). Leventidou et al. (2018) pointed out that tropical tropospheric ozone column trends derived from a combination of European satellite measurements from 1996 to 2015 showed regional increases as large as 1 % yr−1–2 % yr−1, with some negative trends over the oceans, but with significant uncertainties (see also Heue et al., 2016, and Ebojie et al., 2016). The TCO analyses by Ziemke et al. (2019) using combined OMI and MLS ozone columns showed that TCO trends are larger for 2005–2016 than in the 2 decades before 2005; for 2005–2016, the derived TCO tropical trends are ∼ 0.4 % yr−1–0.7 % yr−1 (see also Gaudel et al., 2020). These two investigations found regional differences in TCO trends, with maxima over India, Southeast Asia, the eastern Pacific region, and the tropical Atlantic, with near-zero or slightly negative TCO trends over the western Pacific. Similar TCO trends (based on combined OMI and MLS data) were also given by Liu et al. (2022) for the 2005–2018 period.
A recent study (Gaudel et al., 2024) of tropical tropospheric ozone trends from several satellite-based and in situ data sets between 1994 and 2019 yields the maximum mid- and upper-tropospheric increases above India, Southeast Asia and Malaysia, with values from 3.4 ± 0.8 to 6.8 ± 1.8 ppbv decade−1. The tropical UT O3 trend results obtained here from MLS data, converted to the same units, are 2.2 ± 1.6 ppbv decade−1, which is consistent with the above results, considering also that the maximum mapped UT trends obtained here (about 1.4 % yr−1) translate to ∼ 8 ppbv decade−1. The MLS-derived results for zonal mean tropical UT trends versus latitude are tabulated in Table S1 in both sets of units. The OMI/MLS tropical trends (2004–2021) from the above reference are listed as 2 ± 5 % decade−1 for 0 to 20° S and 3 ± 2 % decade−1 for 0 to 20° N; these numbers are consistent with the slightly larger UT averages from MLS, which show an increase from ∼ 3 % decade−1 near 20° S to ∼ 4 % decade−1 near 20° N (see Table S1 in the Supplement for more details).
Table 2Trend results from recent tropical upper-tropospheric O3 data analyses. See Sect. 3.1.4 for other references and comments.
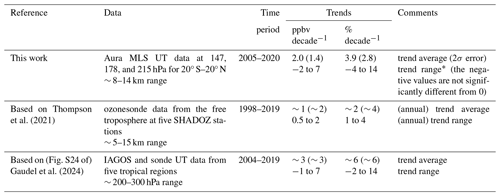
∗ Trend range for MLS is taken from the minimum and maximum values in mapped tropical UT trends.
Regarding other past O3 trend results for the upper troposphere, IAGOS-derived trends were previously discussed by Cohen et al. (2018) for the 1994–2013 period, but with an emphasis on the extra-tropics. The IAGOS trend analysis by Gaudel et al. (2020) for five tropical regions over 1994–2016 gave positive UT trends averaging ∼ 0.6 % yr−1, with largest values over Southeast Asia. As mentioned above, the MLS results also show peak ozone trends over this general region. Wang et al. (2022) showed that ozone trends from ozonesonde profiles for a very similar time period agree broadly with IAGOS results, although the sonde spatiotemporal coverage is limited, and there can be significant scatter in trends from various sonde sites. Their non-satellite UT data sets and derived trends are similar to those from Gaudel et al. (2020). Thompson et al. (2021) observed significant seasonal variations in tropical ozonesonde trends (based on data for 1994–2019 from the Southern Hemisphere Additional Ozonesondes, or SHADOZ network); these authors noted that dynamical influences (besides emissions changes) likely play a role in these tropical tropospheric trends, which average ∼ 0.1 % yr−1–0.4 % yr−1 (for annual trends), but with trends in certain regions/seasons (February to May in particular) as large as 1 % yr−1–2.5 % yr−1. While model studies in a recent paper by Ma et al. (2024) also confirm that lower-stratospheric O3 and related dynamical transport effects can significantly impact long-term UT O3 trends, their results suggest that, for the tropics, the largest influence (of order 60 %–70 % or more) comes from the tropospheric O3 source. Table 2 provides trend averages and trend ranges from MLS, along with those from two of the above references regarding UT O3 trends, not including column results or references with redundancy or poorer matches to the MLS period. For tropical tropospheric O3 column trends, a comprehensive review is given by Gaudel et al. (2024). The trends in Table 2 are consistent with MLS tropical UT trends, which are based on more dense and daily coverage. Given the different periods and tropical sampling patterns between IAGOS and sondes versus MLS, these UT trend comparisons give as reasonable an agreement as one might expect; see also the significant impacts on O3 trends from in situ sampling limitations mentioned by Gaudel et al. (2024).
Zhang et al. (2016) and Wang et al. (2022) have ascribed the positive sign of post-2000 tropical ozone trends to an equatorward redistribution of surface emissions over the years. Moreover, Wang et al. (2022) discussed how increases in aircraft emissions of nitrogen oxides should also have contributed to enhancements in UT ozone. The UT zonal mean model O3 trends shown in our work are typically larger (by ∼ 30 %–50 %) in the NH tropics than in the SH tropics. This is also true for the model simulation (also from CESM2) provided by Wang et al. (2022); these authors also point out that uncertainties in estimates of ozone precursor emission inventories (including those for volatile organic carbons species, or VOCs) may well contribute to differences between modeled and observational ozone trends. While VOC source strengths might be difficult to invoke as a major source of uncertainty for the tropical regions, other potential model issues (e.g., larger than currently expected uncertainties in lightning-generated ozone in the tropical upper troposphere) may be worth further consideration. We note that there are large differences (a range of a factor of 2 or more) between the tropospheric ozone burden changes predicted by various global models in the work by Wang et al. (2022). Also, Liu et al. (2022) show that significant regional differences in ozone column trends exist in their model results (using the NASA Goddard Earth Observing System Chemistry Climate Model, GEOSCCM), with near-zero trends over the tropical western Pacific; their modeled TCO trend results underestimate the observed positive TCO trends.
Regarding ozone UT variability, we found that the annual, semi-annual, and ENSO terms dominate the variability in the tropical upper troposphere. The TCO interannual variability has been known to be heavily influenced by ENSO (Ziemke and Chandra, 2003; Ziemke et al., 2010). Oman et al. (2013) found that the ENSO relationship for ozone could be simulated by a chemical climate model driven by observed SSTs. The observed and matching simulated sensitivity coefficients imply increased downwelling from the stratosphere and suppressed convection during El Niño periods for regions of positive sensitivity (Chandra et al., 1998; Sudo and Takahashi, 2001; Oman et al., 2013). The MLS UT ozone variations and their relation to ENSO were discussed by Oman et al. (2013), who showed patterns of ozone sensitivity to ENSO at 147 hPa (their Fig. 6) that resemble the ones we produced here (in Fig. 7) from analyses of MLS data over almost twice as long a period.
3.2 Tropical UT CO
3.2.1 CO climatologies
For CO, a set of annual mean climatological plots similar to those from Fig. 1 is provided in Fig. 8. We observe that the model CO values follow the patterns of the MLS UT CO fields fairly well, and the zonal mean model biases are usually around −10 % to −20 %; the model biases are most often negative and more so in the northern tropics at 215 hPa. The model mean CO biases shown in Fig. 8 are well within the MLS CO systematic uncertainties mentioned in Sect. 2.1; the CAM-chem-CEDS climatological UT CO is slightly closer to the MLS UT CO climatology than is the WACCM-CEDS CO climatology. As in the case of ozone, the aforementioned model versus data CO biases are found to exist not only for annual averages, but also on a month-to-month basis. The SPARC Data Initiative report (SPARC, 2017) and the more recent update by Hegglin et al. (2021) showed that MLS CO values in the tropical UT are within about 10 %–15 % of the mean values that include other data from ACE-FTS and the Michelson Interferometer for Passive Atmospheric Sounding (MIPAS). However, the MLS mean values are larger than the multi-instrument mean at 100 hPa by about 10 %–20 %, which can account for more than half of the MLS–model bias at this level (not shown here). Also, just considering the theoretical systematic uncertainty estimates provided in Sect. 2.1, it is possible that most (or even all) of the bias between models and MLS at 100 hPa is caused by a positive bias in the MLS CO data. However, an earlier WACCM-CEDS version (WACCM4) underestimated CO and other hydrocarbon data in the southern tropical UT, as described by Park et al. (2013); those authors noted that model deficiencies in emission source strengths or in the upward rate of transport could potentially explain these model underestimates. In summary, while we cannot pin down the exact causes for the mean biases between the UT CO climatologies from MLS and the models shown here, a combination of MLS and model systematic errors likely provides a reasonable explanation.
3.2.2 CO zonal mean trends
For CO, the zonal mean time series provided in Fig. S2 show that there are some slight differences in the trends between observed and modeled (WACCM-CEDS) CO, with more negative trends in the MLS series than in the model series. The large variability seen in the MLS CO series shows correlation with WACCM-CEDS (see the large correlation coefficient values, R, in the 12° S series for 147 and 215 hPa). We know that the largest CO peaks in these time series are tied to surface emissions, convection, and subsequent transport into the upper troposphere and lower stratosphere (UTLS), with a strong connection to El Niño-related droughts and intense fire (biomass burning) events (see, e.g., Schoeberl et al., 2006; Jiang et al., 2007; Liu et al., 2013; Park et al., 2021; Duncan et al., 2003, 2007). The largest CO peaks in the MLS upper-tropospheric tropical record have been correlated with El Niño events in late 2006, in 2009–2010 and especially from late 2015 into 2016 (see Park et al., 2021, and references therein, for further information). At 12° N, the observed CO variability is somewhat smaller than at 12° S, and the model variability is much more muted, while the model versus MLS phasing agreement is quite poor, especially at 215 hPa (where R is very small and the MLS time series annual phase is very poorly matched by the model). We have checked that this poor correlation is not tied to an issue involving the smoothing of model profiles to account for the MLS averaging kernels; indeed, Fig. S3 shows the small relative impact resulting from a smoothed (versus vertically interpolated) model series on the average CO profile at 215 hPa and 12° N, as well as regarding the smoothed time series and its phasing.
For the UT CO zonal mean trends, Fig. 9 provides results in a similar way to Fig. 2 for ozone but for just the two MLS CO retrieval levels at 147 and 215 hPa. In contrast to ozone, we can see that the MLS-derived tropical UT CO values have typically decreased from 2005 to 2020; these CO trends display negligible latitude dependence. Using the same approach as for ozone, but based on the Fig. 9 results, we obtain an average MLS-based UT CO trend of −0.25 ± 0.30 % yr−1 (equivalent to −0.20 ± 0.23 ppbv yr−1). The trends at 215 hPa (−0.16 % yr−1) are a factor of 2 smaller than those at 147 hPa (−0.34 % yr−1), although both of these numbers agree within the (2σ) trend uncertainties of 0.3 % yr−1; based on the error bars, the CO trend from MLS at 147 hPa is different from zero, while the corresponding MLS trend at 215 hPa is not. In contrast, the average CAM-chem-CAMS UT CO trend at these levels is 0.22 ± 0.19 % yr−1, with little difference between 147 and 215 hPa. The two simulations that use CEDS emissions (WACCM-CEDS and CAM-chem-CEDS) yield smaller trends for CO, namely 0.0 ± 0.14 % yr−1, with slightly negative average trends at 147 hPa and slightly positive average trends at 215 hPa. This difference in trends can be explained by significant decreases in Chinese anthropogenic emissions in CEDSv2, despite the increasing anthropogenic tropical CO emissions in both CAMS-GLOB-ANT v5.1 and CEDSv2 (see Fig. S4).
Furthermore, larger MLS CO abundances in 2020 explain why the MLS CO UT trends are more negative if one stops the analyses in 2018 or 2019, as can be seen from Fig. 10, which is analogous to the ozone trend sensitivity study provided in Fig. 3. Regarding another aspect of CO trend sensitivities, we considered the issue of large peaks in the observed MLS CO time series (see examples in Fig. S2) typically resulting from El Niño-related biomass burning events, followed by convective uplift and CO advective transport into the UTLS. If the model has smaller peaks than the MLS data show, it may be that this could explain some differences or even a change of sign in the trends. This would stand out more if the large peaks occurred close to the beginning or end of the time series. As a sensitivity test, we artificially suppressed the peaks in these series by setting any CO value larger than 2.5 times the (1σ) variability to a value of 1.5 times this variability, and we found the impact on the linear trends to be negligible (well within the error bars shown here). Such a sensitivity study gives added confidence in the robustness of these trends.
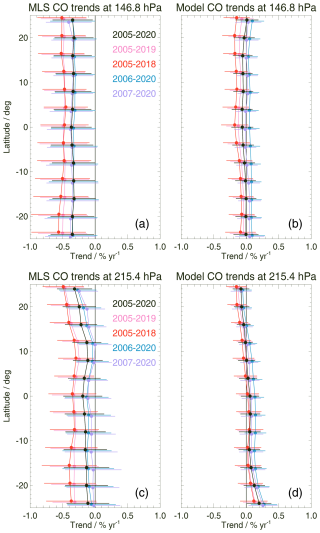
Figure 10Same as Fig. 3 but for CO tropical zonal mean trends from MLS and CAM-chem-CEDS at the MLS CO UT retrieval levels of 147 and 215 hPa.
In Fig. 11, we show the MLS, WACCM-CEDS, and CAM-chem-CEDS climatological mean CO changes over the annual cycle at 215 hPa for 12° N and 12° S, along with the range of variability (twice the standard deviations about the means). The fits from the models to the MLS CO behavior at 12° S are quite good. The MLS CO curves show the two maxima previously observed in seasonal analyses of biomass burning events, with related upward injections of CO and their subsequent transport to the UT being implicated. Based on fire counts from satellite data (see, e.g., Duncan et al., 2003, 2007), a March biomass burning maximum has been associated with the Northern Hemisphere (mainly from Southeast Asia but also from northern Africa); outflow from the Asian monsoon contributes to the August NH maximum. The September/October maximum arises from the Southern Hemisphere (Indonesia, Malaysia, southern Africa, Brazil). We should also note (more broadly) that the climatological double maximum CO structure measured by MLS near 215 hPa over the broader (20° S–20° N) tropics is well matched by MIPAS CO zonal means (see SPARC, 2017, Chap. 4). At 12° N, however, the lack of correlation between the model variations and those deduced from MLS in Fig. 11 appears to stem from the poorly modeled double maximum structure; we also find that these poorer fits occur more generally throughout the northern tropics. The model underestimates the boreal winter buildup of CO (Gaubert et al., 2020, 2023), which may explain a poor representation of the Northern Hemisphere March/April maximum. Also, biomass burning emission biases can vary regionally, and this might explain some of the model–data differences, with some regions providing somewhat better comparisons than others. We do not ascribe the larger model–MLS discrepancies at 215 hPa in the northern tropics to an undue influence of the MLS a priori on the retrievals in this region, as the (averaged) a priori MLS values (although not shown in Fig. 11) follow the WACCM-CEDS fields quite well, and the MLS CO retrievals are producing significantly different variations. To explore this hemispherical asymmetry further, we show CO column comparisons between zonal mean time series from MOPITT, CAM-chem-CEDS, and WACCM-CEDS in Fig. 12; all CO columns are averaged over the same latitudes (10–14° N and 10–14° S). We obtain much better agreement in the phasing of these CO column comparisons for 12° N than we do in the model versus MLS CO comparisons at 215 hPa in Fig. 11. This is clearly seen in the time series evolution, as well as in the correlation coefficients shown in both of these figures, although R is smaller at 12° N than at 12° S in Fig. 12 (but still about 0.75 to 0.8). We also look at this issue for the gridded fields and provide R values for the 12° N and 12° S bins in Fig. 13, where we superpose the column CO model results versus MOPITT and the 215 hPa model results versus MLS as a function of longitude. Again, we observe that R is much higher for the CO total columns than for the 215 hPa level, especially so in the northern tropics. The patterns versus longitude indicate that poorer correlations exist over the Atlantic Ocean (just west of the Greenwich meridian) than over land masses. We do not have clear explanations for the exact patterns in Fig. 13, except for the suggestion that regions with strong land convection might show better UT correlations between models and data, while outflow regions (downwind of convection) in the upper troposphere could be more poorly modeled. The models do not follow the observed UT CO seasonal behavior in a narrow UT region of the northern tropics, even if the modeled seasonal total columns compare well to MOPITT columns in that region. More in-depth analysis would be needed to probe whether this might be caused by a poor representation of emissions and/or transport to this region. Alternatively, it might be that currently unaccounted for variations of the MLS vertical averaging kernels could affect the (properly smoothed) model values in the Northern Hemisphere tropics at 215 hPa, in ways that are somehow significantly different than what we show in Fig. S3; this is highly unlikely, given that the smoothed model plots in this figure hardly change if we replace the tropical MLS averaging kernel values used in that plot by kernels appropriate for 70° N. Another potential issue might be poorly understood cloud impacts on the 215 hPa MLS retrievals, specifically in the Northern Hemisphere tropics; although this is speculative, it might be worth exploring in the future.
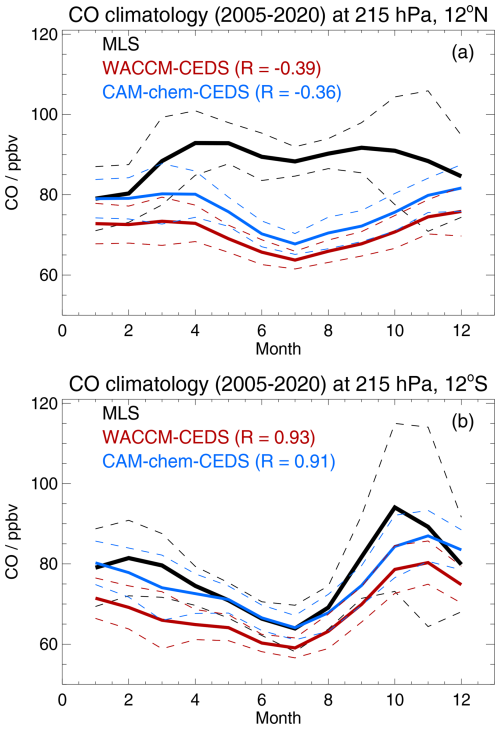
Figure 11CO climatology at 215 hPa (using the 2005–2020 period) from MLS, WACCM-CEDS, and CAM-chem-CEDS for 4° wide latitude bins centered at (a) 12° N and (b) 12° S. The thick solid lines represent the mean values from MLS (black), WACCM-CEDS (red), and CAM-chem-CEDS (blue), with corresponding variability estimates (twice the standard deviations) given by the colored dashed lines about each mean.
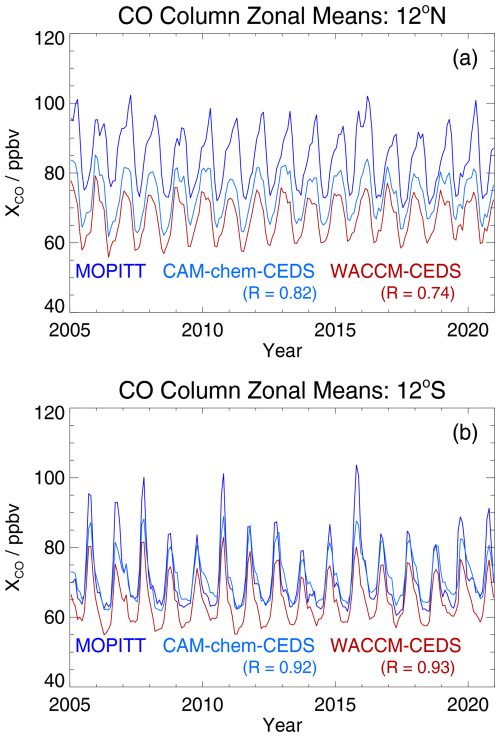
Figure 12CO column comparisons between zonal mean time series from MOPITT (purple) XCO (see text) and from CAM-chem-CEDS (blue) and WACCM-CEDS (red) for 4° wide latitude bins centered at (a) 12° N and (b) 12° S.
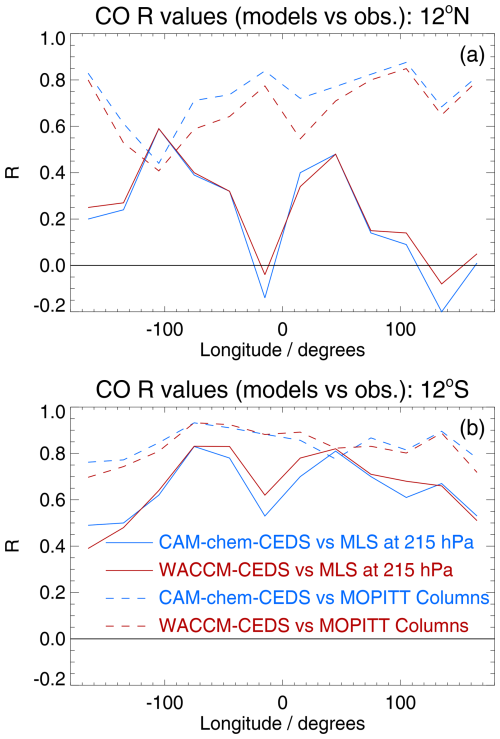
Figure 13Correlation coefficient values (R) for the zonal mean time series from the model CO columns (CAM-chem-CEDS in blue, WACCM-CEDS in red) versus MOPITT columns (dashed) and from the same two models' CO mixing ratios versus MLS CO at 215 hPa (solid) for 4° wide latitude bins centered at (a) 12° N and (b) 12° S.
3.2.3 CO mapped trends and variability
In Fig. 14, we show the mapped CO trend results for MLS and all three simulations (WACCM-CEDS, CAM-chem-CAMS, and CAM-chem-CEDS) at 147 and 215 hPa. As seen above, MLS CO trends in the UT are generally negative, with the more statistically significant result occurring at 147 hPa (where the trends are more negative than at 215 hPa). There is an indication of slightly positive trends over or near western Africa, mainly at 215 hPa, although this is not statistically significant. The binned model results from CAM-chem-CAMS confirm the zonal mean view from this model, with mostly positive trends, in contrast to the generally negative tendencies in the MLS trend results. The average trend (from both pressure levels) based on all grid cells for MLS is −0.25 % yr−1, as opposed to +0.24 % yr−1 obtained from CAM-chem-CAMS. Note that these values lie well outside twice the standard errors in the means (of 0.1 % yr−1), although one should understand that there are limitations in the use of such a small error bar, given the existence of correlations in atmospheric variability between the various bins. When the CEDS emissions are used, as done for WACCM-CEDS and CAM-chem-CEDS, there is a general decrease in the UT CO trends, with some small negative values, although the vast majority of the model CO trends obtained here are not statistically different from zero within any given bin. The averaged UT mapped trend for CAM-chem-CEDS is 0.0 % yr−1, with twice the standard error in the mean also about 0.1 % yr−1. While the use of the model CEDS emissions does lead to a better model agreement with the gridded MLS UT CO trends, the MLS-derived trends are still, on the whole, more negative than these simulated CO trends.
For CO, we repeat in Fig. 15 the explained variance analysis provided in Fig. 6 for O3. Overall, the full fits explain less of the variability in the CO case, in part because of the large ENSO-related peaks that occur throughout the MLS and WACCM-CEDS records, which the regression model, as designed, can only imperfectly match. Also, there are regions in the southern tropics where the annual cycle in the model is better fit by the regression than in the MLS case, and this translates to a somewhat better overall full fit. For both MLS and model, the semi-annual cycle component shows peaks over the South Atlantic region, which is likely linked to biomass burning in Africa and related CO transport to the UT following convective activity (e.g., Duncan et al., 2007; Park et al., 2013, 2021). As for the ozone case, the QBO-related UT variability in the tropics is very small (as seen from the QBO R2 contributions). For both MLS and model representations, the ENSO-related correlation patterns are broadly similar to the ozone case, in that there is larger variance in the more extreme longitudes of both western and eastern sides. As for O3, there are somewhat smaller variance contributions in the Eastern Hemisphere from ENSO and the semi-annual term than in the MLS case. At 215 hPa (see Fig. S6), the ENSO variance contribution is slightly larger than at 147 hPa only in a small number of bins, but the overall ENSO-related patterns are not stronger, as seen also in the CO sensitivity coefficients to ENSO in Fig. 16 below, which shows only slight differences between the two pressure levels.
3.2.4 CO discussion
Regarding the CO climatology, the models underestimate the MLS UT values by up to 20 %, and these differences could be readily caused by systematic biases in either MLS or the models, or both. Park et al. (2013) also found that model CO values from a (WACCM4) simulation at 147 hPa were smaller than the ACE-FTS (and MLS) CO abundances, especially in the SH sub-tropics; they attributed this to a possible underestimation of surface emissions or transport via deep convection. We note that low biases in simulated tropospheric CO have also been found before at northern latitudes and may arise from various factors, such as underestimated CO emissions, high biases in modeled tropospheric OH (Strode et al., 2016; Gaubert et al., 2023), or issues with simulated CO dry deposition rates (Stein et al., 2014). Based on our model–MLS comparisons of UT CO seasonal changes, we find significantly poorer matches at 215 hPa in the northern tropics than in the southern tropics. The detailed causes of this discrepancy are currently not clear to us, given the better matches (correlation coefficients) we obtain between MOPITT total CO columns and modeled CO columns. Potential causes could include model inaccuracies (possibly related to convection and/or CO emissions and subsequent transport in this fairly narrow latitude region), stratosphere–troposphere exchange, or an alternate explanation having to do with poorly understood limitations of the MLS data in this same region.
For the CO trends from 2005–2020, the average tropical MLS UT trend is −0.25 ± 0.30 % yr−1, whereas the corresponding trends from CAM-chem-CEDS and WACCM-CEDS are close to zero (0.0 ± 0.14 % yr−1) for this region; these average trend results are statistically in agreement, even if the MLS CO trends tend to generally be more negative than the simulation results. Note, however, that the MLS-derived CO UT trends for 2005–2023 are closer to zero (about −0.1 % yr−1), as we mention in the Conclusions section, but we have no model simulations (or model trends) for that extended period. The CAM-chem-CAMS simulations (which use CAMS anthropogenic CO emissions; see Sect. 2.2) yield statistically significant positive average tropical UT CO trends (+0.22 ± 0.19 % yr−1). More specifically, these simulated latitude-dependent trends are significantly different from the MLS CO trends in the 12–24° N latitude bins. Larger MLS CO abundances in 2020 explain why the MLS CO UT trends are more negative if one stops the analyses in 2018 or 2019. The mapped MLS CO trends in the UT are also negative, with the more statistically significant result (stronger negative trends) occurring at 147 hPa.
While there have not been any past decades-long trend estimates for CO in the broad tropical UT region, our results yield somewhat smaller rates of decrease than other trends mentioned in the Introduction, for example, −0.5 % yr−1 to −2 % yr−1, based on IAGOS UT data at northern midlatitudes (Cohen et al., 2018). Column CO in the free troposphere has generally shown decreasing trends since the turn of the century, typically between −0.5 % yr−1 and −1.5 % yr−1, as observed in particular by MOPITT and AIRS (Worden et al., 2013a; Strode et al., 2016; Buchholz et al., 2021; Hedelius et al., 2021); however, these trends are not necessarily expected to agree with UT CO trends, since they represent two different altitude regimes. Liu et al. (2022) presented a recent analysis of MOPITT CO data from 2005–2018, along with tropospheric model comparisons to observed CO and O3 time series. These authors found (as shown here and described for MLS and ACE-FTS data by Park et al., 2021) that their modeled and observational time series both exhibit large interannual variability, with some of the largest interannual changes driven by El Niño events in 2006 and 2015 and related biomass burning and CO enhancements tied to droughts over the Indonesian region (see also Logan et al., 2008; Zhang et al., 2011; Livesey et al., 2013; Worden et al., 2013b; Park et al., 2013; Field et al., 2016). Liu et al. (2022) found that modeled CO column trends over various regions of the globe were generally negative, although a lower-latitude region (India) exhibited a positive model trend. Jiang et al. (2017) provide some arguments (and other references) pointing to flat biomass burning emission trends over Africa for the first 10–15 years since the turn of the century. Not including the strong tropical anomaly caused by El Niño in 2015, they infer a negative trend in global biomass burning emissions. Uncertainties in the temporal evolution of OH (a major sink for CO) could also explain model CO trend issues. However, Jiang et al. (2017) implied that changes in global OH abundances could not readily explain global CO decreases, given constraints from methyl chloroform surface data (this species also having OH as a major sink, as discussed by Montzka et al., 2011) and despite large uncertainties in OH, especially during the last decade. Rather, these authors conclude that decreasing CO emissions from anthropogenic and biomass burning sources are the main cause of tropospheric CO decreases, although some regional increasing emission trends do exist. While a systematic model bias cannot readily lead to a significant discrepancy in model trend estimates (in percent per year) versus observations, time-dependent emission biases could (e.g., Gaubert et al., 2023). To first order, the decreasing UT CO tropical trends derived from MLS for 2005–2020 agree with (but tend to be smaller in magnitude than) total column CO trends discussed previously in the literature. As discussed by others, some temporal non-linearity in CO trends may be responsible for some of the differences between past tropospheric CO trend results over different periods.
For CO in particular, the temporal variability that MLS has observed in the upper troposphere is difficult to fit completely using standard linear regression, given the existence of short-term variability in the troposphere (e.g., Dunkerton and Crum, 1995; Ziemke et al., 2015), as well as large episodic and somewhat random enhancements in the UT CO abundances. Regarding this CO variability, we note that ACE-FTS UT CO monthly zonal mean time series track those from MLS, as shown by Park et al. (2021); this helps to validate the UT time series and variability from MLS. We find that the CO sensitivity to ENSO is much more spatially uniform in sign than the O3 sensitivity; UT O3 generally increases toward the tropopause, while CO decreases, leading to opposite sensitivities to increased upwelling phase over the Pacific (Figs. 7 and 16). In some regions, the CO sensitivity has the same sign as for ozone, and in other regions, it differs; moreover, the model's UT CO sensitivity coefficient to ENSO seems to broadly match the observational sensitivity from MLS, as it shows positive values throughout the tropics. These different behaviors between tropical UT O3 and CO seem to mainly reflect a stronger (and positive) sensitivity to biomass burning events in the case of CO.
We have analyzed tropical ozone (O3) and carbon monoxide (CO) distributions in the upper troposphere (UT) and their temporal changes for 2005–2020 using Aura Microwave Limb Sounder (MLS) observations and chemistry climate model simulations. Upper-tropospheric trends and variability diagnostics were obtained from multiple linear regression analyses.
4.1 Tropical UT O3
We have compared the model and MLS annual ozone climatologies, focusing on the 147 and 215 hPa pressure levels; the model abundances are typically ∼ 5 %–15 % smaller than MLS O3 at 215 hPa but larger than the MLS values at 147 hPa by ∼ 20 %. MLS O3 has an averaged UT zonal mean trend at 20° S–20° N of +0.39 ± 0.28 % yr−1. We obtain excellent agreement with the above result from the (averaged) CAM-chem-CEDS O3 zonal mean trends (0.38 ± 0.28 % yr−1) and somewhat poorer agreement from the smaller WACCM-CEDS trends (0.21 ± 0.23 % yr−1). We note that the MLS tropical UT zonal mean O3 trends for 2005–2023 are 0.34 ± 0.22 % yr−1, so these trends have only changed by a small amount versus the 2005–2020 results; it is useful that the trend error bars are reduced by about 23 % for the analysis using 3 more years (the same holds for the CO 2005–2023 trends mentioned below). However, we cannot readily update any of the model simulations (and related trend comparisons) with more analysis years at the time of this writing.
Our analyses for various latitude–longitude bins produce positive mapped O3 trends of up to 1.4 % yr−1 over Indonesia and east of that region, as well as over tropical Africa and the tropical Atlantic. Positive tropical UT mapped O3 trends are generally captured by the model simulations, although in a more muted way. We find broad similarities (and some differences) between the mapped MLS UT O3 trends and corresponding mapped trends of tropospheric column ozone for the same time period.
4.2 Tropical UT CO
The model climatologies generally show an underestimate versus the MLS CO climatology, with model average biases usually about −10 % to −20 %. Also, in the Northern Hemisphere tropics, we find significantly poorer model fits to the observed phasing of CO seasonal changes at 215 hPa than at 147 hPa. This discrepancy is much smaller for the comparison of modeled and Measurements of Pollution in the Troposphere (MOPITT) V9J CO columns. The MLS zonal mean CO UT trend is −0.25 ± 0.30 % yr−1, while the corresponding model CO trends are close to zero (0.0 ± 0.14 % yr−1) when the anthropogenic emissions used in CAM-chem and WACCM are taken from the Community Emissions Data System (CEDS) version 2. The non-CEDS version of CAM-chem (the CAM-chem-CAMS simulation) yields averaged CO UT trends of 0.22 ± 0.19 % yr−1, in contrast to the negative tendencies prevalent in the MLS CO trends throughout the tropics. These three average CO trend results agree within the limits of the (2σ) error bars provided above, although the model versus MLS agreement is more marginal when non-CEDS CO emissions are used. We note that the MLS tropical UT CO trends for 2005–2023 are −0.09 ± 0.23 % yr−1, so these trends have changed by somewhat more than the ozone trend results and are closer to zero than the 2005–2020 MLS CO UT trends. Unfortunately, the coming end of the MLS data record will soon make such MLS updates impossible. As we noted for O3, we cannot readily update any of the model simulations (and related trend comparisons) with more analysis years.
The negative MLS tropical UT CO trends for 2005–2020 agree with (but tend to be smaller in magnitude than) previously published total column CO trends, although one does not expect complete agreement between UT and column trends. We also find that the sensitivity of UT CO to El Niño–Southern Oscillation (ENSO) is positive at all tropical longitudes, in contrast to the (well-known) dipolar longitudinal structure that exists for the UT O3 ENSO sensitivity.
The MLS-derived upper-tropospheric tropical trends in O3 and CO arise from a well-sampled multi-year data set, with the results showing a first-order correlation to large-scale changes in lower-tropospheric composition (O3 increases and CO decreases). We find that there are broad similarities (and a few differences) between the measured UT trends and corresponding results from model simulations, which incorporate state-of-the-art representations of the complex interplay between emissions, photochemistry, convection, and transport in the upper troposphere and lower stratosphere. These results will contribute to the continuing assessments of tropospheric evolution, in particular the large community efforts regarding TOAR-II and CMIP7.
Changes in O3 precursor emissions have been implicated previously as a driver for global tropospheric O3 changes (e.g., long-term increases), while decreasing CO emissions from anthropogenic and biomass burning sources have been suggested as the main causes of recent decreases in tropospheric CO. We believe that further investigations into how well different models of O3 and CO in the tropical UT match the corresponding MLS UT trends are warranted to provide better understanding of differences between models. There may still be adjustments to make to the models regarding the assumed CO surface emissions, convection, and/or transport-related issues, even though such studies are beyond the scope of this paper. Indeed, biomass burning from Africa or South America and emissions from Asia, followed by transport, can (and will continue to) influence the tropical upper-tropospheric abundances of CO and O3 (e.g., Tsivlidou et al., 2023). On a longer timescale, the troposphere is a region where the relative importance of multiple factors might change over the multi-decadal timescale of climate change; also, longer-term projections from (free running) models may not be representative of changes from a particular decade or two (see Fiore et al., 2022, regarding model ensemble projections). For example, long-term positive trends in the influx of ozone from the stratosphere to the troposphere may be expected as a result of climate change (Meul et al., 2018), probably with more of an influence on the extra-tropical upper troposphere. Regarding the tropics, Stevenson et al. (2013) showed that a number of chemistry climate model simulations of climate change scenarios yielded long-term ozone decreases in the lower troposphere as a result of enhancements in water vapor (implying more ozone destruction), but low-latitude upper-tropospheric ozone could be expected to rise, following increased production from lightning. Obtaining accurate enough observations of large-scale tropospheric composition change over the long-term is expected to represent a continuing but worthy challenge.
The MLS Level 3 monthly subsets of MLS version 5 ozone data can be obtained from https://doi.org/10.5067/Aura/MLS/DATA/3546 (Schwartz et al., 2021a). The MLS Level 3 monthly subsets of MLS version 5 CO data can be obtained from https://doi.org/10.5067/Aura/MLS/DATA/3536 (Schwartz et al., 2021b). The MOPITT version 9 products are available from NASA through the Earthdata portal (https://doi.org/10.5067/TERRA/MOPITT/MOP03JM.009, NASA/LARC/SD/ASDCMOPITT, 2020). The MOPITT CO data were obtained from the NASA Langley Research Center Atmospheric Science Data Center. The text file for the solar flux data set can be accessed at https://www.spaceweather.gc.ca/solar_flux_data/monthly_averages/solflux_monthly_average.txt (last access: 20 January 2022). See Tapping (2013) for the background and methodology for the production of this data set.
The QBO-related equatorial wind monthly time series were obtained from the public website at https://www.atmohub.kit.edu/data/qbo.dat (Naujokat, 1986). The multivariate ENSO index data set was obtained from the NOAA Physical Sciences Laboratory website at https://www.psl.noaa.gov/enso/mei/ (Wolter and Timlin, 2011; Zhang et al., 2019). OMI/MLS tropospheric ozone data were obtained from the NASA satellite tropospheric ozone web page https://acd-ext.gsfc.nasa.gov/Data_services/cloud_slice/ (NASA, 2024). The CESM2.2 model is a publicly released version of the Community Earth System Model that is available at (https://doi.org/10.5065/D67H1H0V, Danabasoglu, 2024). Also, the model simulation results for this work are available at the following website link: https://doi.org/10.5281/zenodo.14510410 (Gaubert and Kinnison, 2024).
The supplement related to this article is available online at: https://doi.org/10.5194/acp-25-597-2025-supplement.
LF analyzed the MLS and model data for trends and variability and prepared the manuscript, along with contributions from all co-authors. DEK, CGB, and BG provided inputs for running the model runs, properly averaged and formatted outputs from the model, and provided pertinent model-related comments and interpreted the results. JRZ provided TCO data sets and comments on the manuscript. NJL, MJS, WGR, and others on the MLS team provided analyses and expertise to enable the production of the Aura MLS data sets. NJL, MJS, and WGR also provided comments on the manuscript. RAF provided programming assistance for the creation of the MLS data sets and for storage and analyses of the MLS and model files.
The contact author has declared that none of the authors has any competing interests.
Publisher’s note: Copernicus Publications remains neutral with regard to jurisdictional claims made in the text, published maps, institutional affiliations, or any other geographical representation in this paper. While Copernicus Publications makes every effort to include appropriate place names, the final responsibility lies with the authors.
This article is part of the special issue “Tropospheric Ozone Assessment Report Phase II (TOAR-II) Community Special Issue (ACP/AMT/BG/GMD inter-journal SI)”. It is not associated with a conference.
We are thankful to the whole MLS team (past and present) for their contributions over the years to the MLS instrument, data, processing, and database management. We very much acknowledge the referees, who helped improve the initial manuscript with quite a list of useful and detailed comments. We acknowledge the encouragement from Owen Cooper and Helen Worden to link our paper to the TOAR-II Copernicus special issue; we also thank Kai-Lan Chang for briefly reviewing aspects of our statistical approach regarding the guidelines adopted for reporting TOAR-linked results. WACCM-CEDS is a component of the CESM, supported by the National Science Foundation (NSF). We acknowledge high-performance computing support from Cheyenne (https://doi.org/10.5065/D6RX99HX) provided by NCAR's Computational and Information Systems Laboratory, sponsored by the NSF. F10.7 data collection and dissemination are supported by the National Research Council of Canada, with the participation of Natural Resources Canada and support by the Canadian Space Agency. Work at the Jet Propulsion Laboratory, California Institute of Technology, was performed under contract with the National Aeronautics and Space Administration (80NM0018D0004).
Lucien Froidevaux, Ryan Fuller, and Douglas E. Kinnison were funded by the NASA Atmospheric Composition Modeling and Analysis Program (ACMAP). Nathaniel J. Livesey, Michael J. Schwartz, and William G. Read (as well as LF) were supported by the Aura Microwave Limb Sounder project. Douglas E. Kinnison, Benjamin Gaubert, and Charles G. Bardeen were funded separately by NASA and NSF grants. Part of this material is based upon work supported by the NSF National Center for Atmospheric Research, which is a major facility sponsored by the U.S. National Science Foundation (under cooperative agreement no. 1852977). The NSF NCAR MOPITT project is supported by the National Aeronautics and Space Administration (NASA) Earth Observing System (EOS) program.
This paper was edited by Gabriele Stiller and reviewed by Yann Cohen and two anonymous referees.
Andela, N., Morton, D. C., Giglio, L., Chen, Y., van der Werf, G. R., Kasibhatla, P. S., DeFries, R. S., Collatz, G. J., Hantson, S., Kloster, S., Bachelet, D., Forrest, M., Lasslop, G., Li, F., Mangeon, S., Melton, J. R., Yue, C., and Randerson, J. T.: A human-driven decline in global burned area, Science, 356, 1356–1362, https://doi.org/10.1126/science.aal4108, 2017.
Bouarar, I., Gaubert, B., Brasseur, G. P., Steinbrecht, W., Doumbia, T., Tilmes, S., Liu, Y., Stavrakou, T., Deroubaix, A., Darras, S., Granier, C., Lacey, F., Müller, J.-F., Shi, X., Elguindi, N., and Wang, T.: Ozone anomalies in the free troposphere during the COVID-19 pandemic, Geophys. Res. Lett., 48, e2021GL094204, https://doi.org/10.1029/2021GL094204, 2021.
Bourassa, A. E., Degenstein, D. A., Randel, W. J., Zawodny, J. M., Kyrölä, E., McLinden, C. A., Sioris, C. E., and Roth, C. Z.: Trends in stratospheric ozone derived from merged SAGE II and Odin-OSIRIS satellite observations, Atmos. Chem. Phys., 14, 6983–6994, https://doi.org/10.5194/acp-14-6983-2014, 2014.
Brasseur, G. P., Gupta, M., Anderson, B. E., Balasubramanian, S., Barrett, S., Duda, D., Fleming, G., Forster, P. M., Fuglestvedt, J., Gettelman, A., Halthore, R. N., Jacob, D., Jacobson, M. Z., Khodayari, A., Liou, K.-N., Lund, M. T., Miake-Lye, R. C., Minnis, P., Olsen, S., Penner, J. E., Prinn, R., Schumann, U., Selkirk, H. B., Sokolov, A., Unger, N., Wolfe, P., Wong, H.-W., Wuebbles, D. W., Yi, B., Yang, P., and Zhou, C.: Impact of aviation on climate, FAA's Aviation Climate Change Research Initiative (ACCRI) Phase II, B. Am. Meteorol. Soc., 97, 561–583, https://doi.org/10.1175/BAMS-D-13-00089.1, 2016.
Buchholz, R. R., Worden H. M., Park, M., Francis, G., Deeter, M. N., Edwards, D. P., Emmons, L. K., Gaubert, B., Gille, J., Martínez-Alonso, S., Tang, W., Kumar, R., Drummond, J. R., Clerbaux, C., George, M., Pierre François Coheur, P.–F., Hurtmans, D., Bowman, K. W., Luo, M., Payne, V. H., Worden, J. R., Chin, M., Levy, R. C., Warner, J., Wei, Z., and Kulawik, S. S.: Air pollution trends measured from Terra: CO and AOD over industrial, fire-prone, and background regions, Remote Sens. Environ., 256, 112275, https://doi.org/10.1016/j.rse.2020.112275, 2021.
Burkholder, J. B., Sander, S. P., Abbatt, J. P. D., Barker, J. R., Cappa, C., Crounse, J. D., Dibble, T. S., Huie, R. E., Kolb, C. E., Kurylo, M. J., Orkin, V. L., Percival, C. J., Wilmouth, D. M., and Wine, P. H.: Chemical kinetics and photochemical data for use in atmospheric studies, Evaluation No. 19, JPL Publication 19-5, Jet Propulsion Laboratory, California Institute of Technology, Pasadena, http://jpldataeval.jpl.nasa.gov (last access: 1 March 2022), 2019.
Calvo, N., Garcia, R. R., Randel, W. J., and Marsh, D.: Dynamical mechanism for the increase in tropical upwelling in the lowermost tropical stratosphere during warm ENSO events, J. Atmos. Sci., 67, 2331–2340, https://doi.org/10.1175/2010JAS3433.1, 2010.
Chandra, S., Ziemke, J. R., Min, W., and Read, W. G.: Effects of 1997–1998 El Niño on tropospheric ozone and water vapor, Geophys. Res. Lett., 25, 3867–3870, https://doi.org/10.1029/98GL02695, 1998.
Coddington, O., Lean, J., Pilewskie, P., Snow, M., and Lindholm, D.: A solar irradiance climate data record, B. Am. Meteorol. Soc., 97, 1265–1282, https://doi.org/10.1175/BAMS-D-14-00265.1, 2016.
Cohen, Y., Petetin, H., Thouret, V., Marécal, V., Josse, B., Clark, H., Sauvage, B., Fontaine, A., Athier, G., Blot, R., Boulanger, D., Cousin, J.-M., and Nédélec, P.: Climatology and long-term evolution of ozone and carbon monoxide in the upper troposphere–lower stratosphere (UTLS) at northern midlatitudes, as seen by IAGOS from 1995 to 2013, Atmos. Chem. Phys., 18, 5415–5453, https://doi.org/10.5194/acp-18-5415-2018, 2018.
Collins, W. J., Derwent, R. G., Garnier, B., C. E. Johnson, C. E., Sanderson, M. G., and Stevenson, D. S.: Effect of stratosphere-troposphere exchange on the future tropospheric ozone trend, J. Geophys. Res., 108, 8528, https://doi.org/10.1029/2002JD002617, 2003.
Cooper, O. R., Parrish, D. D., Ziemke, J. R., Balashov, N. V., Cupeiro, M., Galbally, I., Gilge, S., Horowitz, L., Jensen, N. R., Lamarque, J.-F., Naik, V., Oltmans, S. J., Schwab, J., Shindell, D. T., Thompson, A. M., Thouret, V., Wang, Y., and Zbinden, R. M.: Global distribution and trends of tropospheric ozone: An observation-based review, Elementa, 2, 000029, https://doi.org/10.12952/journal.elementa.000029, 2014.
Crutzen, P. J.: A discussion of the chemistry of some minor constituents in stratosphere and troposphere, Pure Appl. Geophys., 106, 1385–1399, https://doi.org/10.1007/BF00881092, 1973.
Crutzen, P. J. and Andreae, M. O.: Biomass burning in the tropics: Impact on atmospheric chemistry and biogeochemical cycles, Science, 250, 1669–1678, https://doi.org/10.1126/science.250.4988.1669, 1990.
Danabasoglu, G.: CESM2 data and software, UCAR [data set], https://doi.org/10.5065/D67H1H0V, 2020.
Danabasoglu, G., Lamarque, J.-F., Bacmeister, J., Bailey, D. A., DuVivier, A. K., Edwards, J., Emmons, L. K., Fasullo, J., Garcia, R., Gettelman, A., Hannay, C., Holland, M. M., Large, W. G., Lauritzen, P. H., Lawrence, D. M., Lenaerts, J. T. M., Lindsay, K., Lipscomb, W. H., Mills, M. J., Neale, R., Oleson, K. W., Otto-Bliesner, B., Phillips, A. S., Sacks, W., Tilmes, S., van Kampenhout, L., Vertenstein, M., Bertini, A., Dennis, J., Deser, C., Fischer, C., Fox-Kemper, B., Kay, J. E., Kinnison, D. E., Kushner, P. J., Larson, V. E., Long, M. C., Mickelson, S., Moore, J. K., Nienhouse, E., Polvani, L., Rasch, P. J., and Strand, W. G.: The Community Earth System Model Version 2 (CESM2), J. Adv. Model. Earth Syst., 12, e2019MS001916, https://doi.org/10.1029/2019MS001916, 2020.
Darmenov, A. and da Silva, A. M.: The Quick Fire Emissions Dataset (QFED) – Documentation of versions 2.1, 2.2 and 2.4, NASA/TM–2015–104606, 2015;38:183, http://gmao.gsfc.nasa.gov/pubs/tm/ (last access: 15 January 2024), 2014.
Davis, N. A., Callaghan, P., Simpson, I. R., and Tilmes, S.: Specified dynamics scheme impacts on wave-mean flow dynamics, convection, and tracer transport in CESM2 (WACCM6), Atmos. Chem. Phys., 22, 197–214, https://doi.org/10.5194/acp-22-197-2022, 2022.
Deeter, M., Francis, G., Gille, J., Mao, D., Martínez-Alonso, S., Worden, H., Ziskin, D., Drummond, J., Commane, R., Diskin, G., and McKain, K.: The MOPITT Version 9 CO product: sampling enhancements and validation, Atmos. Meas. Tech., 15, 2325–2344, https://doi.org/10.5194/amt-15-2325-2022, 2022.
Doherty, R. M., Stevenson, D. S., Johnson, C. E., Collins, W. J., and Sanderson, M. G.: Tropospheric ozone and El Niño–Southern Oscillation: Influence of atmospheric dynamics, biomass burning emissions, and future climate change, J. Geophys. Res.-Atmos., 111, D19304, https://doi.org/10.1029/2005JD006849, 2006.
Duncan, B. N., Martin, R. V., Staudt, A. C., Yevich, R., and Logan, J. A.: Interannual and seasonal variability of biomass burning emissions constrained by satellite observations, J. Geophys. Res.-Atmos., 108, 4100, https://doi.org/10.1029/2002jd002378, 2003.
Duncan, B. N., Strahan, S. E., Yoshida, Y., Steenrod, S. D., and Livesey, N.: Model study of the cross-tropopause transport of biomass burning pollution, Atmos. Chem. Phys., 7, 3713–3736, https://doi.org/10.5194/acp-7-3713-2007, 2007.
Dunkerton, T. J. and Crum, F. X.: Eastward propagating eastward ∼ 2- to 15-day equatorial convection and its relation to the tropical intraseasonal oscillation, J. Geophys. Res., 100, D12, 25781–25790, https://doi.org/10.1029/95JD02678, 1995.
Ebojie, F., Burrows, J. P., Gebhardt, C., Ladstätter-Weißenmayer, A., von Savigny, C., Rozanov, A., Weber, M., and Bovensmann, H.: Global tropospheric ozone variations from 2003 to 2011 as seen by SCIAMACHY, Atmos. Chem. Phys., 16, 417–436, https://doi.org/10.5194/acp-16-417-2016, 2016.
Efron, B. and Tibshirani, R.: An Introduction to the Bootstrap, Mg. Stat. Pro. 57, Chapman and Hall, 1993.
Emmons, L. K., Walters, S., Hess, P. G., Lamarque, J.-F., Pfister, G. G., Fillmore, D., Granier, C., Guenther, A., Kinnison, D., Laepple, T., Orlando, J., Tie, X., Tyndall, G., Wiedinmyer, C., Baughcum, S. L., and Kloster, S.: Description and evaluation of the Model for Ozone and Related chemical Tracers, version 4 (MOZART-4), Geosci. Model Dev., 3, 43–67, https://doi.org/10.5194/gmd-3-43-2010, 2010.
Emmons, L. K., Schwantes, R. H., Orlando, J. J., Tyndall, G., Kinnison, D., Lamarque, J.-F., Marsh, D., Mills, M. J., Tilmes, S., Bardeen, C., Buchholz, R. R., Conley, A., Gettelman, A., Garcia, R., Simpson, I., Blake, D. R., Meinardi, S., and Pétron, G.: The Chemistry Mechanism in the Community Earth System Model version 2 (CESM2), J. Adv. Model. Earth Syst., 12, e2019MS001882, https://doi.org/10.1029/2019MS001882, 2020.
Feely, R. A., Gammon, R. H., Taft, B. A., Pullen, P. E., Waterman, L. S., Conway, T. J., Gendron, J. F., and Wisegarver, D. P.: Distribution of chemical tracers in the eastern equatorial Pacific during and after the 1982–1983 El Niño/Southern Oscillation Event, J. Geophys. Res.-Oceans, 92, 6545–6558, https://doi.org/10.1029/JC092iC06p06545, 1987.
Field, R. D., van der Werf, G. R., Fanin, T., Fetzer, E. J., Fuller, R., Jethva, H., Levy, R., Livesey, N. J., Luo, M., Torres, O., and Worden, H. M.: Indonesian fire activity and smoke pollution in 2015 show persistent nonlinear sensitivity to El Niño-induced drought, P. Natl. Acad. Sci. USA, 113, 9204–9209, https://doi.org/10.1073/pnas.1524888113, 2016.
Fiore, A. M., Hancock, S. E., Lamarque, J.-F., Correa, G. P., Chang, K.-L., Ru, M., Cooper, O., Gaudel, A., Polvani, L. M., Sauvage, B., and Ziemke, J. R.: Understanding recent tropospheric ozone trends in the context of large internal variability: a new perspective from chemistry-climate model ensembles, Environ. Res.-Climate, 1, 025008, https://doi.org/10.1088/2752-5295/ac9cc2, 2022.
Froidevaux, L., Kinnison, D. E., Wang, R., Anderson, J., and Fuller, R. A.: Evaluation of CESM1 (WACCM) free-running and specified dynamics atmospheric composition simulations using global multispecies satellite data records, Atmos. Chem. Phys., 19, 4783–4821, https://doi.org/10.5194/acp-19-4783-2019, 2019.
Froidevaux, L., Kinnison, D. E., Santee, M. L., Millán, L. F., Livesey, N. J., Read, W. G., Bardeen, C. G., Orlando, J. J., and Fuller, R. A.: Upper stratospheric ClO and HOCl trends (2005–2020): Aura Microwave Limb Sounder and model results, Atmos. Chem. Phys., 22, 4779–4799, https://doi.org/10.5194/acp-22-4779-2022, 2022.
Gaubert, B. and Kinnison, D.: CAM-chem and WACCM outputs, Zenodo [data set], https://doi.org/10.5281/zenodo.14510410, 2024.
Gaubert, B., Worden, H. M., Arellano, A. F. J., Emmons, L. K., Tilmes, S., Barré, J., Martinez Alonso, S., Vitt, F., Anderson, J. L., Alkemade, F., Houweling, S., and Edwards, D. P.: Chemical feedback from decreasing carbon monoxide emissions, Geophys. Res. Lett., 44, 9985–9995, https://doi.org/10.1002/2017GL074987, 2017.
Gaubert, B., Emmons, L. K., Raeder, K., Tilmes, S., Miyazaki, K., Arellano Jr., A. F., Elguindi, N., Granier, C., Tang, W., Barré, J., Worden, H. M., Buchholz, R. R., Edwards, D. P., Franke, P., Anderson, J. L., Saunois, M., Schroeder, J., Woo, J.-H., Simpson, I. J., Blake, D. R., Meinardi, S., Wennberg, P. O., Crounse, J., Teng, A., Kim, M., Dickerson, R. R., He, H., Ren, X., Pusede, S. E., and Diskin, G. S.: Correcting model biases of CO in East Asia: impact on oxidant distributions during KORUS-AQ, Atmos. Chem. Phys., 20, 14617–14647, https://doi.org/10.5194/acp-20-14617-2020, 2020.
Gaubert, B., Edwards, D. P., Anderson, J. L., Arellano, A.F., Barré, J., Buchholz, R.R., Darras, S., Emmons, L. K., Fillmore, D., Granier, C., Hannigan, J. W., Ortega, I., Raeder, K., Soulié, A., Tang, W., Worden, H. M., and Ziskin, D.: Global Scale Inversions from MOPITT CO and MODIS AOD, Remote Sens., 15, 4813, https://doi.org/10.3390/rs15194813, 2023.
Gaudel, A., Cooper, O. R., Ancellet, G., Barret, B., Boynard, A., Burrows, J. P., Clerbaux, C., Coheur, P. F., Cuesta, J., Cuevas, E., Doniki, S., Dufour, G., Ebojie, F., Foret, G., Garcia, O., Granados-Muñoz, M. J., Hannigan, J. W., Hase, F., Hassler, B., Huang, G., Hurtmans, D., Jaffe, D., Jones, N., Kalabokas, P., Kerridge, B., Kulawik, S., Latter, B., Leblanc, T., Le Flochmoën, E., Lin, W., Liu, J., Liu, X., Mahieu, E., McClure-Begley, A., Neu, J. L., Osman, M., Palm, M., Petetin, H., Petropavlovskikh, I., Querel, R., Rahpoe, N., Rozanov, A., Schultz, M. G., Schwab, J., Siddans, R., Smale, D., Steinbacher, M., Tanimoto, H., Tarasick, D. W., Thouret, V., Thompson, A. M., Trickl, T., Weatherhead, E., Wespes, C., Worden, H. M., Vigouroux, C., Xu, X., Zeng, G., and Ziemke, J.: Tropospheric Ozone Assessment Report: Present-day distribution and trends of tropospheric ozone relevant to climate and global atmospheric chemistry model evaluation, Elem. Sci. Anth., 6, 39, https://doi.org/10.1525/elementa.291, 2018.
Gaudel, A., Cooper, O. R., Chang, K.-L., Bourgeois, I., Ziemke, J. R., Strode, S. A., Oman, L. D., Sellitto, P., Nédélec, P., Blot, R., Thouret, V., and Granier, C.: Aircraft observations since the 1990s reveal increases of tropospheric ozone at multiple locations across the Northern Hemisphere, Sci. Adv., 6, eaba8272, https://doi.org/10.1126/sciadv.aba8272, 2020.
Gaudel, A., Bourgeois, I., Li, M., Chang, K.-L., Ziemke, J., Sauvage, B., Stauffer, R. M., Thompson, A. M., Kollonige, D. E., Smith, N., Hubert, D., Keppens, A., Cuesta, J., Heue, K.-P., Veefkind, P., Aikin, K., Peischl, J., Thompson, C. R., Ryerson, T. B., Frost, G. J., McDonald, B. C., and Cooper, O. R.: Tropical tropospheric ozone distribution and trends from in situ and satellite data, Atmos. Chem. Phys., 24, 9975–10000, https://doi.org/10.5194/acp-24-9975-2024, 2024.
Gelaro, R., McCarty, W., Suarez, M. J., Todling, R., Molod, A., Takacs, L., Randles, C. A., Darmenov, A., Bosilovich, M. G., Reichle, R., Wargan, K., Coy, L., Cullather, R., Draper, C., Akella, S., Buchard, V., Conaty, A., da Silva, A. M., Gu, W., Kim, G.-K., Koster, R., Lucchesi, R., Merkova, D., Nielsen, J. E., Partyka, G., Pawson, S., Putman, W., Rienecker, M., Schubert, S. D., Sienkiewicz, M., and Zhao, B.: The Modern-Era Retrospective Analysis for Research and Applications, Version 2 (MERRA2), J. Climate, 30, 5419–5454, https://doi.org/10.1175/JCLI-D-16-0758.1, 2017.
Gettelman, A., Mills, M. J., Kinnison, D. E., Garcia, R. R., Smith, A. K., Marsh, D. R., Tilmes, S., Vitt, F., Bardeen, C. G., McInerny, J., Liu, H.-L., Solomon, S. C., Polvani, L. M., Emmons, L. K., Lamarque, J.-F., Richter, J. H., Glanville, A. S., Bacmeister, J. T., Phillips, A. S., Neale, R. B., Simpson, I. R., DuVivier, A. K., Hodzic, A., and Randel, W. J.: The Whole Atmosphere Community Climate Model version 6 (WACCM6), J. Geophys. Res.-Atmos., 124, 12380–12403, https://doi.org/10.1029/2019JD030943, 2019.
Gratz, L. E., Jaffe, D. A., and Hee, J. R.: Causes of increasing ozone and decreasing carbon monoxide in springtime at the Mt. Bachelor Observatory from 2004 to 2013, Atmos. Environ., 109, 323–330, https://doi.org/10.1016/j.atmosenv.2014.05.076, 2015.
He, H., Stehr, J. W., Hains, J. C., Krask, D. J., Doddridge, B. G., Vinnikov, K. Y., Canty, T. P., Hosley, K. M., Salawitch, R. J., Worden, H. M., and Dickerson, R. R.: Trends in emissions and concentrations of air pollutants in the lower troposphere in the Baltimore/Washington airshed from 1997 to 2011, Atmos. Chem. Phys., 13, 7859–7874, https://doi.org/10.5194/acp-13-7859-2013, 2013.
Hedelius, J. K., Toon, G. C., Buchholz, R. R., Iraci, L. T., Podolske, J. R., Roehl, C. M., Wennberg, P. O., Worden, H. M., and Wunch, D.: Regional and urban column CO trends and anomalies as observed by MOPITT over 16 years, J. Geophys. Res.-Atmos., 126, e2020JD033967, https://doi.org/10.1029/2020JD033967, 2021.
Hegglin, M. I. and Shepherd, T. G.: Large Climate-Induced Changes in Ultraviolet Index and Stratosphere-to Troposphere Ozone Flux, Nat. Geosci., 2, 687–691, https://doi.org/10.1038/ngeo604, 2009.
Hegglin, M. I., Tegtmeier, S., Anderson, J., Bourassa, A. E., Brohede, S., Degenstein, D., Froidevaux, L., Funke, B., Gille, J., Kasai, Y., Kyrölä, E. T., Lumpe, J., Murtagh, D., Neu, J. L., Pérot, K., Remsberg, E. E., Rozanov, A., Toohey, M., Urban, J., von Clarmann, T., Walker, K. A., Wang, H.-J., Arosio, C., Damadeo, R., Fuller, R. A., Lingenfelser, G., McLinden, C., Pendlebury, D., Roth, C., Ryan, N. J., Sioris, C., Smith, L., and Weigel, K.: Overview and update of the SPARC Data Initiative: comparison of stratospheric composition measurements from satellite limb sounders, Earth Syst. Sci. Data, 13, 1855–1903, https://doi.org/10.5194/essd-13-1855-2021, 2021.
Hess, P. G. and Zbinden, R.: Stratospheric impact on tropospheric ozone variability and trends: 1990–2009, Atmos. Chem. Phys., 13, 649–674, https://doi.org/10.5194/acp-13-649-2013, 2013.
Heue, K.-P., Coldewey-Egbers, M., Delcloo, A., Lerot, C., Loyola, D., Valks, P., and van Roozendael, M.: Trends of tropical tropospheric ozone from 20 years of European satellite measurements and perspectives for the Sentinel-5 Precursor, Atmos. Meas. Tech., 9, 5037–5051, https://doi.org/10.5194/amt-9-5037-2016, 2016.
Hoesly, R. M., Smith, S. J., Feng, L., Klimont, Z., Janssens-Maenhout, G., Pitkanen, T., Seibert, J. J., Vu, L., Andres, R. J., Bolt, R. M., Bond, T. C., Dawidowski, L., Kholod, N., Kurokawa, J.-I., Li, M., Liu, L., Lu, Z., Moura, M. C. P., O'Rourke, P. R., and Zhang, Q.: Historical (1750–2014) anthropogenic emissions of reactive gases and aerosols from the Community Emissions Data System (CEDS), Geosci. Model Dev., 11, 369–408, https://doi.org/10.5194/gmd-11-369-2018, 2018.
Hoor, P., Borken-Kleefeld, J., Caro, D., Dessens, O., Endresen, O., Gauss, M., Grewe, V., Hauglustaine, D., Isaksen, I. S. A., Jöckel, P., Lelieveld, J., Myhre, G., Meijer, E., Olivie, D., Prather, M., Schnadt Poberaj, C., Shine, K. P., Staehelin, J., Tang, Q., van Aardenne, J., van Velthoven, P., and Sausen, R.: The impact of traffic emissions on atmospheric ozone and OH: results from QUANTIFY, Atmos. Chem. Phys., 9, 3113–3136, https://doi.org/10.5194/acp-9-3113-2009, 2009.
Hsu, J. and Prather, M. J.: Is the residual vertical velocity a good proxy for stratosphere-troposphere exchange of ozone?, Geophys. Res. Lett., 41, 9024–9032, https://doi.org/10.1002/2014GL061994, 2014.
Huang, L., Fu, R., Jiang, J. H., Wright, J. S., and Luo, M.: Geographic and seasonal distributions of CO transport pathways and their roles in determining CO centers in the upper troposphere, Atmos. Chem. Phys., 12, 4683–4698, https://doi.org/10.5194/acp-12-4683-2012, 2012.
Huang, L., Fu, R., and Jiang, J. H.: Impacts of fire emissions and transport pathways on the interannual variation of CO in the tropical upper troposphere, Atmos. Chem. Phys., 14, 4087–4099, https://doi.org/10.5194/acp-14-4087-2014, 2014.
Huang, L., Jiang, J. H., Murray, L. T., Damon, M. R., Su, H., and Livesey, N. J.: Evaluation of UTLS carbon monoxide simulations in GMI and GEOS-Chem chemical transport models using Aura MLS observations, Atmos. Chem. Phys., 16, 5641–5663, https://doi.org/10.5194/acp-16-5641-2016, 2016.
Hubert, D., Lambert, J.-C., Verhoelst, T., Granville, J., Keppens, A., Baray, J.-L., Bourassa, A. E., Cortesi, U., Degenstein, D. A., Froidevaux, L., Godin-Beekmann, S., Hoppel, K. W., Johnson, B. J., Kyrölä, E., Leblanc, T., Lichtenberg, G., Marchand, M., McElroy, C. T., Murtagh, D., Nakane, H., Portafaix, T., Querel, R., Russell III, J. M., Salvador, J., Smit, H. G. J., Stebel, K., Steinbrecht, W., Strawbridge, K. B., Stübi, R., Swart, D. P. J., Taha, G., Tarasick, D. W., Thompson, A. M., Urban, J., van Gijsel, J. A. E., Van Malderen, R., von der Gathen, P., Walker, K. A., Wolfram, E., and Zawodny, J. M.: Ground-based assessment of the bias and long-term stability of 14 limb and occultation ozone profile data records, Atmos. Meas. Tech., 9, 2497–2534, https://doi.org/10.5194/amt-9-2497-2016, 2016.
Jiang, J. H., Livesey, N. J., Su, H., Neary, L., McConnell, J. C., and Richards, N. A. D.: Connecting surface emissions, convective uplifting, and long-range transport of carbon monoxide in the upper troposphere: New observations from the Aura Microwave Limb Sounder, Geophys. Res. Lett., 34, L18812, https://doi.org/10.1029/2007gl030638, 2007.
Jiang, Z., Worden, J. R., Worden, H., Deeter, M., Jones, D. B. A., Arellano, A. F., and Henze, D. K.: A 15-year record of CO emissions constrained by MOPITT CO observations, Atmos. Chem. Phys., 17, 4565–4583, https://doi.org/10.5194/acp-17-4565-2017, 2017.
Jones, C. D., Collins, M., Cox, P. M., and Spall, S. A.: The Carbon Cycle Response to ENSO: A Coupled Climate–Carbon Cycle Model Study, J. Climate, 14, 4113–4129, https://doi.org/10.1175/1520-0442(2001)014<4113:tccrte>2.0.CO;2, 2001.
Kinnison, D. E., Brasseur, G. P., Walters, S., Garcia, R. R., Sassi, F., Boville, B. A., Marsh, D. Harvey, L., Randall, C., Randel, W., Lamarque, J. F., Emmons, L. K., Hess, Orlando, J., Tyndall, G., and Pan, L.: Sensitivity of chemical tracers to meteorological parameters in the MOZART-3 chemical transport model, J. Geophys. Res., 112, D20302, https://doi.org/10.1029/2006JD007879, 2007.
Khalil, M. A. K. and Rasmussen, R. A.: The global cycle of carbon monoxide: trends and mass balance, Chemosphere, 20, 227–242, https://doi.org/10.1016/0045-6535(90)90098-E, 1990.
Kumar, A., Wu, S., Weise, M. F., Honrath, R., Owen, R. C., Helmig, D., Kramer, L., Val Martin, M., and Li, Q.: Free-troposphere ozone and carbon monoxide over the North Atlantic for 2001–2011, Atmos. Chem. Phys., 13, 12537–12547, https://doi.org/10.5194/acp-13-12537-2013, 2013.
Laken, B. A. and Shahbaz, T.: Satellite-Detected Carbon Monoxide Pollution during 2000–2012: Examining Global Trends and also Regional Anthropogenic Periods over China, the EU and the USA, Climate, 2014, 1–16, https://doi.org/10.3390/cli2010001, 2014.
Lee, D. S., Fahey, D. W., Skowron, A., Allen, M. R., Burkhardt, U., Chen, Q., Doherty, S. J., Freeman, S., Forster, P. M., Fuglestvedt, J., Gettelman, A., De Leon, R. R., Lim, L. L., Lund, M. T., Millar, R. J., Owen, B., Penner, J. E., Pitari, G., Prather, M. J., Sausen, R., and Wilcox, L. J.: The contribution of global aviation to anthropogenic climate forcing for 2000 to 2018, Atmos. Environ., 244, 117834, https://doi.org/10.1016/j.atmosenv.2020.117834, 2021.
Leventidou, E., Weber, M., Eichmann, K.-U., Burrows, J. P., Heue, K.-P., Thompson, A. M., and Johnson, B. J.: Harmonisation and trends of 20-year tropical tropospheric ozone data, Atmos. Chem. Phys., 18, 9189–9205, https://doi.org/10.5194/acp-18-9189-2018, 2018.
Li, L. and Liu, Y.: Space-borne and ground observations of the characteristics of CO pollution in Beijing, 2000–2010, Atmos. Environ., 45, 2367–2372, https://doi.org/10.1016/j.atmosenv.2011.02.026, 2011.
Liu, J., Logan, J. A., Murray, L. T., Pumphrey, H. C., Schwartz, M. J., and Megretskaia, I. A.: Transport analysis and source attribution of seasonal and interannual variability of CO in the tropical upper troposphere and lower stratosphere, Atmos. Chem. Phys., 13, 129–146, https://doi.org/10.5194/acp-13-129-2013, 2013.
Liu, J., Strode, S. A., Liang, Q., Oman, L. D., Colarco, P. R., Fleming, E. L., Manyin, M. E., Douglass, A. R., Ziemke, J. R., Lamsal, L. N., and Li, C.: Change in tropospheric ozone in the recent decades and its contribution to global total ozone, J. Geophys. Res.-Atmos., 127, e2022JD037170. https://doi.org/10.1029/2022JD037170, 2022.
Livesey, N. J. and Read, W. G.: Direct retrieval of line-of-sight atmospheric structure from limb sounding observations, Geophys. Res. Lett., 27, 891–894, https://doi.org/10.1029/1999GL010964, 2000.
Livesey, N. J., Van Snyder, W., Read, W. G., and Wagner, P. A.: Retrieval algorithms for the EOS Microwave Limb Sounder (MLS), IEEE Trans. Geosci. Remote Sens., 44, 1144–1155, https://doi.org/10.1109/TGRS.2006.872327, 2006.
Livesey, N. J., Filipiak, M. J., Froidevaux, L., Read, W. G., Lambert, A., Santee, M. L., Jiang, J. H., Waters, J. W., Cofield, R. E., Cuddy, D. T., Daffer, W. H., Drouin, B. J., Fuller, R. A., Jarnot, R. F., Jiang, Y. B., Knosp, B. W., Li, Q. B., Perun, V. S., Schwartz, M. J., Snyder,W. V., Stek, P. C., Thurstans, R. P.,Wagner, P. A., Pumphrey, H. C., Avery, M., Browell, E. V., Cammas, J.-P., Christensen, L. E., Edwards, D. P., Emmons, L. K., Gao, R.-S., Jost, H.-J., Loewenstein, M., Lopez, J. D., Nédélec, P., Osterman, G. B., Sachse, G. W., and Webster, C. R.: Validation of Aura Microwave Limb Sounder O3 and CO observations in the upper troposphere and lower stratosphere, J. Geophys. Res., 113, D15S02, https://doi.org/10.1029/2007JD008805, 2008.
Livesey, N. J., Logan, J. A., Santee, M. L., Waters, J. W., Doherty, R. M., Read, W. G., Froidevaux, L., and Jiang, J. H.: Interrelated variations of O3, CO and deep convection in the tropical/subtropical upper troposphere observed by the Aura Microwave Limb Sounder (MLS) during 2004–2011, Atmos. Chem. Phys., 13, 579–598, https://doi.org/10.5194/acp-13-579-2013, 2013.
Livesey, N. J., Read, W. G., Wagner, P. A., Froidevaux, L., Santee, M. L., Schwartz, M. J., Lambert, A., Millan Valle, L. F., Pumphrey, H. C., Manney, G. L., Fuller, R. A., Jarnot, R. F., Knosp, B. W., and Lay, R. R.: EOS MLS Version 5.0x Level 2 and 3 data quality and description document, Tech. rep., Jet Propulsion Laboratory D-105336 Rev. B, 30 January 2022, https://mls.jpl.nasa.gov/eos-aura-mls/documentation.php (last access: 31 January 2024), 2022.
Logan, J. A.: Tropospheric ozone: Seasonal behavior, trends, and anthropogenic influence, J. Geophys. Res.-Atmos., 90, 10463–10482, https://doi.org/10.1029/JD090iD06p10463, 1985.
Logan, J. A., Prather, M. J., Wofsy, S. C., and McElroy, M. B.: Tropospheric chemistry: a global perspective, J. Geophys. Res., 86, 7210–7254, https://doi.org/10.1029/JC086iC08p07210, 1981.
Logan, J. A., Megretskaia, I., Nassar, R., Murray, L. T., Zhang, L., Bowman, K. W., Worden, H. M., and Luo, M.: Effects of the 2006 El Niño on tropospheric composition as revealed by data from the Tropospheric Emission Spectrometer (TES), Geophys. Res. Lett., 35, L03816, https://doi.org/10.1029/2007GL031698, 2008.
Ma, X., Huang, J., Hegglin, M., Joeckel, P., and Zhao, T.: Causes of growing middle-upper tropospheric ozone over the Northwest Pacific region, EGUsphere [preprint], https://doi.org/10.5194/egusphere-2023-2411, 2024.
McDuffie, E. E., Smith, S. J., O'Rourke, P., Tibrewal, K., Venkataraman, C., Marais, E. A., Zheng, B., Crippa, M., Brauer, M., and Martin, R. V.: A global anthropogenic emission inventory of atmospheric pollutants from sector- and fuel-specific sources (1970–2017): an application of the Community Emissions Data System (CEDS), Earth Syst. Sci. Data, 12, 3413–3442, https://doi.org/10.5194/essd-12-3413-2020, 2020.
Meinshausen, M., Vogel, E., Nauels, A., Lorbacher, K., Meinshausen, N., Etheridge, D. M., Fraser, P. J., Montzka, S. A., Rayner, P. J., Trudinger, C. M., Krummel, P. B., Beyerle, U., Canadell, J. G., Daniel, J. S., Enting, I. G., Law, R. M., Lunder, C. R., O'Doherty, S., Prinn, R. G., Reimann, S., Rubino, M., Velders, G. J. M., Vollmer, M. K., Wang, R. H. J., and Weiss, R.: Historical greenhouse gas concentrations for climate modelling (CMIP6), Geosci. Model Dev., 10, 2057–2116, https://doi.org/10.5194/gmd-10-2057-2017, 2017.
Meinshausen, M., Nicholls, Z. R. J., Lewis, J., Gidden, M. J., Vogel, E., Freund, M., Beyerle, U., Gessner, C., Nauels, A., Bauer, N., Canadell, J. G., Daniel, J. S., John, A., Krummel, P. B., Luderer, G., Meinshausen, N., Montzka, S. A., Rayner, P. J., Reimann, S., Smith, S. J., van den Berg, M., Velders, G. J. M., Vollmer, M. K., and Wang, R. H. J.: The shared socio-economic pathway (SSP) greenhouse gas concentrations and their extensions to 2500, Geosci. Model Dev., 13, 3571–3605, https://doi.org/10.5194/gmd-13-3571-2020, 2020.
Meul, S., Langematz, U., Kröger, P., Oberländer-Hayn, S., and Jöckel, P.: Future changes in the stratosphere-to-troposphere ozone mass flux and the contribution from climate change and ozone recovery, Atmos. Chem. Phys., 18, 7721–7738, https://doi.org/10.5194/acp-18-7721-2018, 2018.
Miyazaki, K., Bowman, K., Sekiya, T., Takigawa, M., Neu, J. L., Sudo, K., Osterman, G., and Eskes, H.: Global tropospheric ozone responses to reduced NOx emissions linked to the COVID-19 worldwide lockdowns, Sci. Adv., 7, eabf7460, https://doi.org/10.1126/sciadv.abf7460, 2021.
Monks, P. S., Archibald, A. T., Colette, A., Cooper, O., Coyle, M., Derwent, R., Fowler, D., Granier, C., Law, K. S., Mills, G. E., Stevenson, D. S., Tarasova, O., Thouret, V., von Schneidemesser, E., Sommariva, R., Wild, O., and Williams, M. L.: Tropospheric ozone and its precursors from the urban to the global scale from air quality to short-lived climate forcer, Atmos. Chem. Phys., 15, 8889–8973, https://doi.org/10.5194/acp-15-8889-2015, 2015.
Montzka, S. A., Krol, M., Dlugokencky, E., Hall, B., Jöckel, P., and Lelieveld, J.: Small Interannual Variability of Global Atmospheric Hydroxyl, Science, 331, 67–69, https://doi.org/10.1126/science.1197640, 2011.
Murray, L. T., Mickley, L. J., Kaplan, J. O., Sofen, E. D., Pfeiffer, M., and Alexander, B.: Factors controlling variability in the oxidative capacity of the troposphere since the Last Glacial Maximum, Atmos. Chem. Phys., 14, 3589–3622, https://doi.org/10.5194/acp-14-3589-2014, 2014.
Naujokat, B.: An update of the observed quasi-biennial oscillation of the stratospheric winds over the tropics, J. Atmos. Sci., 43, 1873–1877, 1986.
NASA/LARC/SD/ASDCMOPITT: MOPITT CO gridded daily means (Near and Thermal Infrared Radiances) V009, NASA [data set], https://doi.org/10.5067/TERRA/MOPITT/MOP03JM.009, 2020.
NASA: OMI CCD, OMI/MLS and OMPS/MERRA-2 data, NASA [data set], https://acd-ext.gsfc.nasa.gov/Data_services/cloud_slice/ (last access: 5 January 2024), 2024.
Nassar, R., Logan, J. A., Megretskaia, I. A., Murray, L. T., Zhang, L., and Jones, D. B. A.: Analysis of tropical tropospheric ozone, carbon monoxide, and water vapor during the 2006 El Niño using TES observations and the GEOS-Chem model, J. Geophys. Res.-Atmos., 114, D17304, https://doi.org/10.1029/2009jd011760, 2009.
Neely, R. R. and Schmidt, A.: VolcanEESM: Global volcanic sulphur dioxide (SO2) emissions database from 1850 to present – Version 1.0., https://doi.org/10.5285/76ebdc0b-0eed-4f70-b89e-55e606bcd568, 2016.
Neu, J. L., Flury, T., Manney, G. L., Santee, M. L., Livesey, N. J., and Worden, J.: Tropospheric ozone variations governed by changes in stratospheric circulation, Nat. Geosci., 7, 340–344, https://doi.org/10.1038/ngeo2138, 2014.
O'Neill, B. C., Tebaldi, C., van Vuuren, D. P., Eyring, V., Friedlingstein, P., Hurtt, G., Knutti, R., Kriegler, E., Lamarque, J.-F., Lowe, J., Meehl, G. A., Moss, R., Riahi, K., and Sanderson, B. M.: The Scenario Model Intercomparison Project (ScenarioMIP) for CMIP6, Geosci. Model Dev., 9, 3461–3482, https://doi.org/10.5194/gmd-9-3461-2016, 2016.
Oman, L. D., Ziemke, J. R., Douglass, A. R., Waugh, D. W., Lang, C., Rodriguez, J. M., and Nielsen, J. E.: The response of tropical tropospheric ozone to ENSO, Geophys. Res. Lett., 38, L13706, https://doi.org/10.1029/2011gl047865, 2011.
Oman, L. D., Douglass, A. R., Ziemke, J. R., Rodriguez, J. M., Waugh, D. W., and Nielsen, J. E.: The ozone response to ENSO in Aura satellite measurements and a chemistry-climate simulation, J. Geophys. Res., 118, 965–976, https://doi.org/10.1029/2012jd018546, 2013.
Park, M., Randel, W. J., Kinnison, D. E., Emmons, L. K., Bernath, P. F., and Walker, K. A., Boone, C. D., and Livesey, N. J.: Hydrocarbons in the upper troposphere and lower stratosphere observed from ACE-FTS and comparisons with WACCM, J. Geophys. Res-Atmos., 118, 1964–1980, https://doi.org/10.1029/2012JD018327, 2013.
Park, M., Worden, H. M., Kinnison, D. E., Gaubert, B., Tilmes, S., Emmons, L. K., Santee, M. L., Froidevaux, L., and Boone, C. D.: Fate of pollution emitted during the 2015 Indonesian fire season, J. Geophys. Res.-Atmos., 126, e2020JD033474, https://doi.org/10.1029/2020JD033474, 2021.
Patel, A., Mallika, C., Chandrab, N., Patrab, P. K., Steinbacherd, M., Revisiting regional and seasonal variations in decadal carbon monoxide variability: Global reversal of growth rate, Sci. Total Environ., 909, 168476, https://doi.org/10.1016/j.scitotenv.2023.168476, 2024.
Petzold, A., Thouret, V., Gerbig, C., Zahn, A., Brenninkmeijer, C. A. M., Gallagher, M., Hermann, M., Pontaud, M., Ziereis, H., Boulanger, D., Marshall, J., Nédélec, P., Smit, H. G. J., Friess, U., Flaud, J.-M., Wahner, A., Cammas, J.-P., and Volz-Thomas, A.: Global-scale atmosphere monitoring by in-service aircraft – current achievements and future prospects of the European Research Infrastructure IAGOS, Tellus B, 67, 28452,
doi10.3402/tellusb.v67.28452, 2015.
Price, C. and Rind, D.: A simple lightning parameterization for calculating global lightning distributions, J. Geophys. Res.-Atmos., 97, 9919–9933, https://doi.org/10.1029/92JD00719, 1992.
Price, C., Penner, J., and Prather, M.: NOx from lightning: 1. Global distribution based on lightning physics, J. Geophys. Res.-Atmos., 102, 5929–5941, https://doi.org/10.1029/96JD03504, 1997.
Randel, W. J., Park, M., Wu, F., and Livesey, N. J.: A large annual cycle in ozone above the tropical tropopause linked to the Brewer-Dobson circulation, J. Atmos. Sci., 64, 4479–4488, https://doi.org/10.1175/2007JAS2409.1, 2007.
Read, W. G., Shippony, Z., and Snyder, W. V.: The clear-sky unpolarized forward model for the EOS Aura microwave limb sounder (MLS), IEEE Trans. Geosci. Remote Sens., 44, 1367–1379, https://doi.org/10.1109/TGRS.2006.862267, 2006.
Riahi, K., van Vuuren, D. P., Kriegler, E., Edmonds, J., O'Neill, B. C., Fujimori, S., Bauer, N., Calvin, K., Dellink, R., Fricko, O., Lutz, W., Popp, A., Crespo Cuaresma, J., Samir, K. C., Leimbach, M., Jiang, L., Kram, T., Rao, S., Emmerling, J., Ebi, K., Hasegawa, T., Havlik, P., Humpenöder, F., Da Silva, A., Smith, S., Stehfest, E., Bosetti, V., Eom, J., Gernaat, D., Masui, T., Rogelj, J., Strefler, J., Drouet, L., Krey, V., Luderer, G., Harmsen, M., Takahashi, K., Baumstark, L., Doelman, J. C., Kainuma, M., Klimont, Z., Marangoni, G., Lotze-Campen, H., Obersteiner, M., Tabeau, A., and Tavoni, M.: The Shared Socioeconomic Pathways and their energy, land use, and greenhouse gas emissions implications: An overview, Global Environ. Chang., 42, 1045 153–168, https://doi.org/10.1016/j.gloenvcha.2016.05.009, 2017.
Rodgers, C.: Inverse Methods for Atmospheric Sounding: Theory and Practice, Vol. 2 of Series on Atmospheric, Oceanic and Planetary Physics, World Scientific, Singapore, ISBN10 981022740X, 2000.
Rowlinson, M. J., Rap, A., Arnold, S. R., Pope, R. J., Chipperfield, M. P., McNorton, J., Forster, P., Gordon, H., Pringle, K. J., Feng, W., Kerridge, B. J., Latter, B. L., and Siddans, R.: Impact of El Niño–Southern Oscillation on the interannual variability of methane and tropospheric ozone, Atmos. Chem. Phys., 19, 8669–8686, https://doi.org/10.5194/acp-19-8669-2019, 2019.
Schoeberl, M. R., Duncan, B. N., Douglass, A. R., Waters, J., Livesey, N., Read, W., and Filipiak, M.: The carbon monoxide tape recorder, Geophys. Res. Lett., 33, L12811, https://doi.org/10.1029/2006gl026178, 2006.
Schwartz, M., Froidevaux, L., Livesey, N., Read, W., and Fuller, R.: MLS/Aura Level 3 Monthly Binned Ozone (O3) Mixing Ratio on Assorted Grids V005, Greenbelt, MD, USA, Goddard Earth Sciences Data and Information Services Center (GES DISC) [data set], https://doi.org/10.5067/Aura/MLS/DATA/3546, 2021a.
Schwartz, M., Pumphrey, H., Livesey, N., Read, W., and Fuller, R.: MLS/Aura Level 3 Monthly Binned Carbon Monoxide (CO) Mixing Ratio on Assorted Grids V005, Greenbelt, MD, USA, Goddard Earth Sciences Data and Information Services Center (GES DISC) [data set], https://doi.org/10.5067/Aura/MLS/DATA/3536, 2021b.
Schumann, U. and Huntrieser, H.: The global lightning-induced nitrogen oxides source, Atmos. Chem. Phys., 7, 3823–3907, https://doi.org/10.5194/acp-7-3823-2007, 2007.
Soulie, A., Granier, C., Darras, S., Zilbermann, N., Doumbia, T., Guevara, M., Jalkanen, J.-P., Keita, S., Liousse, C., Crippa, M., Guizzardi, D., Hoesly, R., and Smith, S. J.: Global anthropogenic emissions (CAMS-GLOB-ANT) for the Copernicus Atmosphere Monitoring Service simulations of air quality forecasts and reanalyses, Earth Syst. Sci. Data, 16, 2261–2279, https://doi.org/10.5194/essd-16-2261-2024, 2024.
Souri, A. H., Choi, Y., Jeon, W., Woo, J.-H., Zhang, Q., and Kurokawa, J.: Remote sensing evidence of decadal changes in major tropospheric ozone precursors over East Asia, J. Geophys. Res.-Atmos., 122, 2474–2492, https://doi.org/10.1002/2016JD025663, 2017.
SPARC: The SPARC Data Initiative: Assessment of stratospheric trace gas and aerosol climatologies from satellite limb sounders, in: SPARC Report No. 8, edited by: Hegglin, M. I. and Tegtmeier, S., WCRP-5/2017, available at: https://www.sparc-climate.org/publications/sparc-reports/sparc-report-no-8/ (last access: 15 December 2023), 2017.
Stein, O., Schultz, M. G., Bouarar, I., Clark, H., Huijnen, V., Gaudel, A., George, M., and Clerbaux, C.: On the wintertime low bias of Northern Hemisphere carbon monoxide found in global model simulations, Atmos. Chem. Phys., 14, 9295–9316, https://doi.org/10.5194/acp-14-9295-2014, 2014.
Steinbrecht, W., Kubistin, D., Plass-Dülmer, C., Davies, J., Tarasick, D. W., Gathen, P. v. d., Deckelmann, H., Jepsen, N., Kivi, R., Lyall, N., Palm, M., Notholt, J., Kois, B., Oelsner, P., Allaart, M., Piters, A., Gill, M., Van Malderen, R., Delcloo, A. W., Sussmann, R., Mahieu, E., Servais, C., Romanens, G., Stübi,R., Ancellet, G., Godin-Beekmann, S., Yamanouchi, S., Strong, K., Johnson, B., Cullis, P., Petropavlovskikh, I., Hannigan, J. W., Hernandez, J.-L., Diaz Rodriguez, A., Nakano, T., Chouza, F., Leblanc, T., Torres, C., Garcia, O., Röhling, A. N., Schneider, M., Blumenstock, T., Tully, M., Paton-Walsh, C., Jones, N., Querel, R., Strahan, S., Stauffer, R. M., Thompson, A. M., Inness, A., Engelen, R., Chang, K.-L., and Cooper, O. R.: COVID-19 Crisis Reduces Free Tropospheric Ozone Across the Northern Hemisphere, Geophys. Res. Lett., 48, e2020GL091987, https://doi.org/10.1029/2020GL091987, 2021.
Stevenson, D. S., Young, P. J., Naik, V., Lamarque, J.-F., Shindell, D. T., Voulgarakis, A., Skeie, R. B., Dalsoren, S. B., Myhre, G., Berntsen, T. K., Folberth, G. A., Rumbold, S. T., Collins, W. J., MacKenzie, I. A., Doherty, R. M., Zeng, G., van Noije, T. P. C., Strunk, A., Bergmann, D., Cameron-Smith, P., Plummer, D. A., Strode, S. A., Horowitz, L., Lee, Y. H., Szopa, S., Sudo, K., Nagashima, T., Josse, B., Cionni, I., Righi, M., Eyring, V., Conley, A., Bowman, K. W., Wild, O., and Archibald, A.: Tropospheric ozone changes, radiative forcing and attribution to emissions in the Atmospheric Chemistry and Climate Model Intercomparison Project (ACCMIP), Atmos. Chem. Phys., 13, 3063–3085, https://doi.org/10.5194/acp-13-3063-2013, 2013.
Strode, S. A., Worden, H. M., Damon, M., Douglass, A. R., Duncan, B. N., Emmons, L. K., Lamarque, J.-F., Manyin, M., Oman, L. D., Rodriguez, J. M., Strahan, S. E., and Tilmes, S.: Interpreting space-based trends in carbon monoxide with multiple models, Atmos. Chem. Phys., 16, 7285–7294, https://doi.org/10.5194/acp-16-7285-2016, 2016.
Sudo, K. and Takahashi, M.: Simulation of tropospheric ozone changes during 1997–1998 El Niño: Meteorological impact on tropospheric photochemistry, Geophys. Res. Lett., 28, 4091–4094, https://doi.org/10.1029/2001GL013335, 2001.
Sudo, K., Takahashi, M., and Akimoto, H.: Future changes in stratosphere-troposphere exchange and their impacts on future tropospheric ozone simulations, Geophys. Res. Lett., 30, 24, 2256, https://doi.org/10.1029/2003GL018526, 2003.
Tapping, K. F.: The 10.7 cm solar radio flux (F10.7), Space Weather, 11, 394–406, https://doi.org/10.1002/swe.20064, 2013.
Thompson, A. M., Doddridge, B. G., Witte, J. C., Hudson, R. D., Luke, W. T., Johnson, J. E., Johnson, B. J., Oltmans, S. J., and Weller, R.: A tropical Atlantic ozone paradox: Shipboard and satellite views of a tropospheric ozone maximum and wave-one in January–February 1999, Geophys. Res. Lett., 27, 3317–3320, https://doi.org/10.1029/1999GL011273, 2000.
Thompson, A. M., Witte, J. C., Oltmans, S. J., Schmidlin, F. J., Logan, J. A., Fujiwara, M., Kirchhoff, V. W. J., Posny, F., Coetzee, G. J. R., Hoegger, B., Kawakami, S., Ogawa, T., Fortuin, J. P. F., and Kelder, H. M.: Southern Hemisphere Additional Ozonesondes (SHADOZ) 1998–2000 tropical ozone climatology. 2. Tropospheric variability and the zonal wave-one, J. Geophys. Res.-Atmos., 108, 8241, https://doi.org/10.1029/2002JD002241, 2003.
Thompson, A. M., Stauffer, R. M., Wargan, K., Witte, J. C., Kollonige, D. E., and Ziemke, J. R.: Regional and seasonal trends in tropical ozone from SHADOZ profiles: Reference for models and satellite products, J. Geophys. Res.-Atmos., 126, e2021JD034691, https://doi.org/10.1029/2021JD034691, 2021.
Tilmes, S., Hodzic, A., Emmons, L. K., Mills, M. J., Gettelman, A., Kinnison, D. E., Park, M., Lamarque, J.-F., Vitt, F., Shrivastava, M., Campuzano-Jost, P., Jimenez, J. L., and Liu, X.: Climate forcing and trends of organic aerosols in the Community Earth System Model (CESM2), J. Adv. Model. Earth Syst., 11, 4323–4351, https://doi.org/10.1029/2019MS001827, 2019.
Tsivlidou, M., Sauvage, B., Bennouna, Y., Blot, R., Boulanger, D., Clark, H., Le Flochmoën, E., Nédélec, P., Thouret, V., Wolff, P., and Barret, B.: Tropical tropospheric ozone and carbon monoxide distributions: characteristics, origins, and control factors, as seen by IAGOS and IASI, Atmos. Chem. Phys., 23, 14039–14063, https://doi.org/10.5194/acp-23-14039-2023, 2023.
Verma, S., Yadava, P. K., Lal, D. M., Mall, R. K., Kumar, H., and Payra, S.: Role of Lightning NOx in Ozone Formation: A Review, Pure Appl. Geophys., https://doi.org/10.1007/s00024-021-02710-5, 2021.
Voulgarakis, A., Marlier, M. E., Faluvegi, G., Shindell, D. T., Tsigaridis, K., and Mangeon, S.: Interannual variability of tropospheric trace gases and aerosols: The role of biomass burning emissions, J. Geophys. Res.-Atmos., 120, 7157–7173, https://doi.org/10.1002/2014JD022926, 2015.
Wang, P.-H., Fishman, J., Harvey, V. L., and Hitchman, M. H.: Southern tropical upper tropospheric zonal ozone wave-1 from SAGE II observations (1985–2002), J. Geophys. Res.-Atmos., 111, D08305, https://doi.org/10.1029/2005JD006221, 2006.
Wang, H., Lu, X., Jacob, D. J., Cooper, O. R., Chang, K.-L., Li, K., Gao, M., Liu, Y., Sheng, B., Wu, K., Wu, T., Zhang, J., Sauvage, B., Nédélec, P., Blot, R., and Fan, S.: Global tropospheric ozone trends, attributions, and radiative impacts in 1995–2017: an integrated analysis using aircraft (IAGOS) observations, ozonesonde, and multi-decadal chemical model simulations, Atmos. Chem. Phys., 22, 13753–13782, https://doi.org/10.5194/acp-22-13753-2022, 2022.
Warner, J., Carminati, F., Wei, Z., Lahoz, W., and Attié, J.-L.: Tropospheric carbon monoxide variability from AIRS under clear and cloudy conditions, Atmos. Chem. Phys., 13, 12469–12479, https://doi.org/10.5194/acp-13-12469-2013, 2013.
Wasserstein, R. L., Schirm, A. L., and Lazar, N. A.: Moving to a World Beyond “p < 0.05”, The Am. Stat., 73 (Sup1), 1–19, https://doi.org/10.1080/00031305.2019.1583913, 2019.
Waters, J., Froidevaux, L., Harwood, R., Jarnot, R., Pickett, H., Read, W., Siegel, P., Cofield, R., Filipiak, M., Flower, D., Holden, J., Lau, G., Livesey, N., Manney, G., Pumphrey, H., Santee, M., Wu, D., Cuddy, D., Lay, R., Loo, M., Perun, V., Schwartz, M., Stek, P., Thurstans, R., Boyles, M., Chandra, S., Chavez, M., Chen, G.-S., Chudasama, B., Dodge, R., Fuller, R., Girard, M., Jiang, J., Jiang, Y., Knosp, B., LaBelle, R., Lam, J., Lee, K., Miller, D., Oswald, J., Patel, N., Pukala, D., Quintero, O., Scaff, D., Snyder, V., Tope, M., Wagner, P., and Walch, M.: The Earth Observing System Microwave Limb Sounder (EOS MLS) on the Aura satellite, IEEE T. Geosci. Remote Sens., 44, 5, https://doi.org/10.1109/TGRS.2006.873771, 2006.
Witte, J. C., Schoeberl, M. R., Douglass, A. R., and Thompson, A. M.: The Quasi-biennial Oscillation and annual variations in tropical ozone from SHADOZ and HALOE, Atmos. Chem. Phys., 8, 3929–3936, https://doi.org/10.5194/acp-8-3929-2008, 2008.
Wolter, K. and Timlin, M. S.: El Niño/Southern Oscillation behaviour since 1871 as diagnosed in an extended multivariate ENSO index (MEI.ext), Int. J. Climatology, 31, 1074–1087, https://doi.org/10.1002/joc.2336, 2011.
Worden, H. M., Deeter, M. N., Frankenberg, C., George, M., Nichitiu, F., Worden, J., Aben, I., Bowman, K. W., Clerbaux, C., Coheur, P. F., de Laat, A. T. J., Detweiler, R., Drummond, J. R., Edwards, D. P., Gille, J. C., Hurtmans, D., Luo, M., Martínez-Alonso, S., Massie, S., Pfister, G., and Warner, J. X.: Decadal record of satellite carbon monoxide observations, Atmos. Chem. Phys., 13, 837–850, https://doi.org/10.5194/acp-13-837-2013, 2013a.
Worden, J., Wecht, K., Frankenberg, C., Alvarado, M., Bowman, K., Kort, E., Kulawik, S., Lee, M., Payne, V., and Worden, H.: CH4 and CO distributions over tropical fires during October 2006 as observed by the Aura TES satellite instrument and modeled by GEOS-Chem, Atmos. Chem. Phys., 13, 3679–3692, https://doi.org/10.5194/acp-13-3679-2013, 2013b.
Yoon, J. and Pozzer, A.: Model-simulated trend of surface carbon monoxide for the 2001–2010 decade, Atmos. Chem. Phys., 14, 10465–10482, https://doi.org/10.5194/acp-14-10465-2014, 2014.
Zhang, L., Li, Q. B., Jin, J., Liu, H., Livesey, N., Jiang, J. H., Mao, Y., Chen, D., Luo, M., and Chen, Y.: Impacts of 2006 Indonesian fires and dynamics on tropical upper tropospheric carbon monoxide and ozone, Atmos. Chem. Phys., 11, 10929–10946, https://doi.org/10.5194/acp-11-10929-2011, 2011.
Zhang, T., Hoell, A., Perlwitz, J., Eischeid, J., Murray, D., Hoerling, M., and Hamill, T.: Towards Probabilistic Multivariate ENSO Monitoring, Geophys. Res. Lett., 46, 10532–10540, https://doi.org/10.1029/2019GL083946, 2019.
Zhang, Y., Cooper, O. R., Gaudel, A., Thompson, A. M., Nédélec, P., Ogino, S.-Y., and West, J. J.: Tropospheric ozone change from 1980 to 2010 dominated by equatorward redistribution of emissions, Nat. Geosci., 9, 875–879, https://doi.org/10.1038/ngeo2827, 2016.
Zheng, B., Chevallier, F., Ciais, P., Yin, Y., Deeter, M. N., Worden, H. M., Wang, Y., Zhang, Q., and He, K.: Rapid decline in carbon monoxide emissions and export from East Asia between years 2005 and 2016, Environ. Res. Lett., 13, 044007, https://doi.org/10.1088/1748-9326/aab2b3, 2018.
Ziemke, J. R. and Chandra, S.: La Niña and El Niño–induced variabilities of ozone in the tropical lower atmosphere during 1970–2001, Geophys. Res. Lett., 30, 3, 1142, https://doi.org/10.1029/2002GL016387, 2003.
Ziemke, J. R., Chandra, S., Oman, L. D., and Bhartia, P. K.: A new ENSO index derived from satellite measurements of column ozone, Atmos. Chem. Phys., 10, 3711–3721, https://doi.org/10.5194/acp-10-3711-2010, 2010.
Ziemke, J. R., Douglass, A. R., Oman, L. D., Strahan, S. E., and Duncan, B. N.: Tropospheric ozone variability in the tropics from ENSO to MJO and shorter timescales, Atmos. Chem. Phys., 15, 8037–8049, https://doi.org/10.5194/acp-15-8037-2015, 2015.
Ziemke, J. R., Oman, L. D., Strode, S. A., Douglass, A. R., Olsen, M. A., McPeters, R. D., Bhartia, P. K., Froidevaux, L., Labow, G. J., Witte, J. C., Thompson, A. M., Haffner, D. P., Kramarova, N. A., Frith, S. M., Huang, L.-K., Jaross, G. R., Seftor, C. J., Deland, M. T., and Taylor, S. L.: Trends in global tropospheric ozone inferred from a composite record of TOMS/OMI/MLS/OMPS satellite measurements and the MERRA-2 GMI simulation , Atmos. Chem. Phys., 19, 3257–3269, https://doi.org/10.5194/acp-19-3257-2019, 2019.
Ziemke, J. R., Kramarova, N. A., Frith, S. M., Huang, L.-K., Haffner, D. P., and Wargan, K.: NASA satellite measurements show global-scale reductions in free tropospheric ozone in 2020 and again in 2021 during COVID-19, Geophys. Res. Lett., 49, e2022GL098712, https://doi.org/10.1029/2022GL098712, 2022.