the Creative Commons Attribution 4.0 License.
the Creative Commons Attribution 4.0 License.
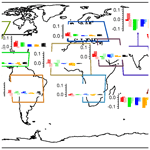
Intercomparison of aerosol optical depths from four reanalyses and their multi-reanalysis consensus
Jeffrey S. Reid
Melanie Ades
Angela Benedetti
Peter R. Colarco
Arlindo da Silva
Tom F. Eck
Johannes Flemming
Edward J. Hyer
Zak Kipling
Samuel Rémy
Tsuyoshi Thomas Sekiyama
Taichu Tanaka
Keiya Yumimoto
Jianglong Zhang
The emergence of aerosol reanalyses in recent years has facilitated a comprehensive and systematic evaluation of aerosol optical depth (AOD) trends and attribution over multi-decadal timescales. Notable multi-year aerosol reanalyses currently available include NAAPS-RA from the US Naval Research Laboratory, the NASA MERRA-2, JRAero from the Japan Meteorological Agency (JMA), and CAMSRA from Copernicus/ECMWF. These aerosol reanalyses are based on differing underlying meteorology models, representations of aerosol processes, as well as data assimilation methods and treatment of AOD observations. This study presents the basic verification characteristics of these four reanalyses versus both AERONET and MODIS retrievals in monthly AOD properties and identifies the strength of each reanalysis and the regions where divergence and challenges are prominent. Regions with high pollution and often mixed fine-mode and coarse-mode aerosol environments, such as South Asia, East Asia, Southeast Asia, and the Maritime Continent, pose significant challenges, as indicated by higher monthly AOD root mean square error. Moreover, regions that are distant from major aerosol source areas, including the polar regions and remote oceans, exhibit large relative differences in speciated AODs and fine-mode versus coarse-mode AODs among the four reanalyses. To ensure consistency across the globe, a multi-reanalysis consensus (MRC, i.e., ensemble mean) approach was developed similarly to the International Cooperative for Aerosol Prediction Multi-Model Ensemble (ICAP-MME). Like the ICAP-MME, while the MRC does not consistently rank first among the reanalyses for individual regions, it performs well by ranking first or second globally in AOD correlation and RMSE, making it a suitable candidate for climate studies that require robust and consistent assessments.
- Article
(14274 KB) - Full-text XML
-
Supplement
(3885 KB) - BibTeX
- EndNote
Four global aerosol reanalyses are intercompared and verified with observations for their skill in simulating aerosol optical depth.
The study identifies the strength of each reanalysis and the regions where there are notable differences and challenges.
The multi-reanalysis consensus, based on the four reanalyses, consistently ranks as one of the best regionally and globally.
In recent years, global aerosol reanalyses have been developed by major operational and research centers, owing to the availability of long-record satellite remote sensing aerosol products and advancements in aerosol data assimilation and modeling. These reanalyses are based on their operational counterparts that are included in the “core four” members of the International Cooperative for Aerosol Prediction multi-model ensemble (ICAP-MME C4C; Sessions et al., 2015; Xian et al., 2019; Reid et al., 2022). The reanalyses include the Copernicus Atmosphere Monitoring Service Reanalysis (CAMSRA; Inness et al., 2019) produced by the European Centre for Medium-Range Weather Forecasts (ECMWF), the Japanese Reanalysis for Aerosol (JRAero) (Yumimoto et al., 2017) developed by the Japan Meteorological Agency (JMA), the NASA Modern-Era Retrospective Analysis for Research and Applications version 2 (MERRA-2; Randles et al., 2017), and the Navy Aerosol Analysis and Prediction System reanalysis (NAAPS-RA; Lynch et al., 2016) developed by the US Naval Research Laboratory (NRL).
The aerosol reanalyses are similar to their operational counterparts and characterized by a high degree of independence in their underlying meteorology, aerosol sources, sinks, microphysics, and chemistry, as well as in their assimilation methods for aerosol optical depth (AOD) observations. A summary of the configurations of these four reanalyses is presented in Table 1 for general features and Table 2 for microphysical and optical treatments of different aerosol species. Notably, the use of operational Terra and Aqua Moderate Resolution Imaging Spectrometer data (MODIS Dark Target and Deep Blue; Levy et al., 2013; Hsu et al., 2013) is consistent across these reanalyses, although preprocessing treatments vary. These treatments include quality control, bias correction, and aggregation as well as sampling. Additionally, several other products, such as the Multi-angle Imaging Spectroradiometer (MISR; Kahn et al., 2010), Advanced Very High Resolution Radiometer (AVHRR; e.g., Ignatov and Stowe, 2002), and Advanced Along-Track Scanning Radiometer (AATSR; Popp et al., 2016) are assimilated into some of these reanalyses, although these additional remote sensing data probably have only a small impact during the MODIS era, as their data volume is small compared with that of MODIS. Therefore, between their underlying meteorology, physics, and data assimilation, these reanalyses are characterized by a high degree of independence overall.
Like atmospheric reanalysis products, aerosol reanalysis products, whether used individually or in combination, have been employed for diverse applications. They provide comprehensive aerosol climatology and statistics to aid in understanding aerosol conditions across various regions and the world (e.g., Reid et al., 2012; Xian et al., 2020; Ningombam et al., 2021; Ohno et al., 2022; Rubin et al., 2023). They are widely used to address a multitude of scientific inquiries in the fields of aerosol radiative forcing (e.g., Randles et al., 2017; Markowicz et al., 2016, 2021a, b; Ohno et al., 2022; Zhang and Zhou, 2023), aerosol–cloud interaction (e.g., McCoy et al., 2017; Ross et al., 2018; Eck et al., 2018), aerosol–cryosphere interaction (e.g., Khan et al., 2018, 2019, 2020; Roychoudhury et al., 2022), air quality and its impact on health (e.g., Tong et al., 2023; Cui et al., 2022; Jenwitheesuk et al., 2022; Lacima et al., 2023), and biogeochemical cycles (e.g., Rahav et al., 2020; Borchardt et al., 2019; Mescioglu et al., 2019), among others. These reanalyses have been rigorously evaluated by the developing centers and various studies from different perspectives, including AOD and other aerosol optical properties, mass concentrations, and vertical distribution profiles. However, to date, no intercomparison among the four reanalyses has been conducted.
This study presents an intercomparison of the four available global aerosol reanalyses to evaluate their skill in simulating monthly average AOD. Additionally, this study includes the development of a multi-reanalysis-consensus (MRC) product using a multi-model-consensus approach, similar to the ICAP multi-model ensemble (ICAP-MME; Sessions et al., 2015; Xian et al., 2019). The MRC is an ensemble mean (i.e., mathematical average) of the four individual reanalyses, with a spatial resolution of 1°×1° lat/long and monthly temporal resolution. The study provides speciated AODs as well as fine-mode (FM), coarse-mode (CM), and total AODs at 550 nm for the period of 2003–2019 from three reanalyses, and all four reanalyses are available for the period of 2011–2019. In addition, a companion study focuses on global and regional AOD trends derived from these reanalyses. The validation of AODs from the MRC and the four component members is performed using ground-based AEROsol Robotic NETwork (AERONET; Holben et al., 1998) observations, with MODIS AOD for spatial distribution evaluation. The validation results, as well as the AOD climatology and divergence of the reanalyses, are presented in Sect. 3. The study concludes with a summary of the findings in Sect. 4.
This study intercompares the monthly average modal (total, FM, and CM) and speciated AOD products from four aerosol reanalyses (RA) and their consensus, and evaluates the RA AODs with AERONET and the combined MODIS Dark Target/Deep Blue retrievals (Levy et al., 2013; Hsu et al., 2013).
2.1 Individual product lines
Descriptions of the four reanalysis datasets, including CAMSRA, JRAero, MERRA-2, and NAAPS-RA v1, are provided in this section. Table 1 provides a summary of the basic features of the four reanalyses and the MRC used in this study. Table 2 offers a summary of the parameters employed to depict the microphysical and optical properties of aerosol species from these reanalyses. Furthermore, Table 3 samples hygroscopic enhancement factor values that influence optical property calculations due to the hygroscopic growth of particles at various relative humidity levels. In addition to utilizing different meteorological data, aerosol source data, AOD observations, and constructing aerosol species, notable differences exist even among similar species regarding treatments related to aerosol microphysics, optical properties, and water uptake ability for hydrophilic species.
2.1.1 CAMSRA
The Copernicus Atmosphere Monitoring Service (CAMS) Reanalysis (CAMSRA; Inness et al., 2019) is run at the European Centre for Medium-Range Weather Forecasts (ECMWF) and is a global reanalysis of atmospheric composition species, including aerosols. It builds on the previous reanalyses of the Monitoring Atmospheric Composition and Climate (MACC) project (Inness et al., 2019) and the CAMS interim reanalysis (Flemming et al., 2017). The CAMSRA is publicly available for 2003–2022 and is being continuously updated.
The CAMSRA is based on the Integrated Forecasting System (IFS) used by ECMWF for numerical weather prediction and meteorological reanalysis. Two additional modules are incorporated into the IFS for the CAMSRA, one to calculate the processes and reactions of the chemical species and one to represent the prognostic aerosol species. The aerosol scheme includes prescribed and online emissions, dry and wet deposition, production of sulfate from a gas-phase sulfur dioxide precursor, and the aging of hydrophobic organic matter (OM) and black carbon (BC) to hydrophilic. The prescribed anthropogenic emissions come from the MACCity inventory (Granier et al., 2011) and the biomass burning (BB) emissions from the Global Fire Assimilation System, version 1.2 (GFASv1.2; Kaiser et al., 2012). GFASv1.2 is a separate system to the IFS that uses satellite retrievals of fire radiative power to produce the BB emissions that are then input as fixed emissions to the aerosol scheme. The transport of the aerosol species by advection, convection, and diffusion is calculated using the meteorological component of the IFS and the wind fields from the meteorology are also used as parameters to estimate the online sea salt (Monahan et al., 1986) and dust (Ginoux et al., 2001) surface emissions. One key difference between the CAMSRA setup of the IFS and that used for numerical weather prediction is that for the CAMSRA the radiative impact of aerosol particles and ozone on meteorology is also taken into account.
The observations used in the CAMSRA for aerosols are of the total AOD at 550 nm. These come from MODIS collection 6 satellite retrievals for the entire period covered by CAMSRA and from the Advanced Along-Track Scanning Radiometer for the period 2003–2012. These AOD observations are simultaneously assimilated with trace gas and meteorological observations using the 4D variational data assimilation system of the IFS with a 12 h assimilation window. The products available from the CAMSRA include speciated AODs at a 3 h temporal and approximately 0.7° spatial resolution, whereas monthly mean AODs at 550 nm were used in this study.
2.1.2 JRAero
The Japanese Reanalysis for Aerosol (JRAero) was developed by the Meteorological Research Institute (MRI) of the Japan Meteorological Agency and Kyushu University using the global aerosol transport model MASINGAR Mk-2 (Yukimoto et al., 2012) and a two-dimensional variational (2D-Var) data assimilation method. The model uses the MRI-AGCM3 atmospheric general circulation model and considers major tropospheric aerosol components, including black carbon (BC), organic carbon (OC), mineral dust, sea salt, and sulfate aerosols, as well as their precursors.
JRAero assimilates global AOD from a bias-corrected MODIS Level-3 AOD product provided by the US Naval Research Laboratory (NRL) and the University of North Dakota (https://modaps.modaps.eosdis.nasa.gov/services/about/products/c61-nrt/MCDAODHD.html last access: 6 May 2024) every 6 h. Anthropogenic and biomass burning emissions were estimated using the MACCity (MACC/CityZEN EU projects) emission inventory (http://accent.aero.jussieu.fr/MACC_metadata.php, last access: 5 May 2024) and the Global Fire Assimilation System (GFAS) dataset (https://ads.atmosphere.copernicus.eu/cdsapp#\!/dataset/cams-global-fire-emissions-gfas?tab=overview, last access: 6 May 2024). The reanalysis has a resolution of TL159 (about 1.1°×1.1°) with 48 vertical layers from the ground to 0.4 hPa. Validation results and additional information can be found in Yumimoto et al. (2017).
2.1.3 MERRA-2
The NASA Modern-Era Retrospective Analysis for Research and Applications version 2 (MERRA-2; Gelaro et al., 2017) is an atmospheric and aerosol reanalysis produced with the NASA Goddard Earth Observing System (GEOS) earth system model. Aerosol data assimilation brings in data from the MODIS and MISR satellite sensors (after 2000) and includes AERONET ground-based sun photometer observations (through 2014). The Goddard Chemistry, Aerosol, Radiation, and Transport model (GOCART; Chin et al., 2022; Colarco et al., 2010) is run online and radiatively coupled in the MERRA-2 system, and provides simulations of dust, sea salt, sulfate, as well as black and organic carbon aerosol species.
Black and organic carbon are each partitioned into hydrophobic and hydrophilic modes, and a single bulk sulfate aerosol species is carried. Dust and sea salt are partitioned into five non-interacting size bins, with dust emissions based on the model 10 m wind speed and a topographic source function following Ginoux et al. (2001), and sea salt emissions driven by the surface wind friction speed modified from Gong (2003) and with a sea-surface temperature adjustment based on Jaeglé et al. (2011). Explosive volcanic sulfur emissions are included through 2010 based on Diehl et al. (2012), with a repeating annual cycle of degassing volcanic emissions subsequent. Other emissions are as summarized in Table 1.
The analysis of AOD is performed on quality-controlled MODIS, MISR, and AERONET data as described in Randles et al. (2017) and Buchard et al. (2015). The AOD analysis is performed by means of analysis splitting, where first a 2D analysis of AOD is performed using error covariances derived from innovation data. Three-dimensional analysis increments for aerosol mass concentration are then computed using the local displacement ensemble (LDE) methodology, which accommodates misplacement of the aerosol plumes due to source or transport issues. The ensemble perturbations are generated at the full model resolution, without the need for multiple model runs. Online quality control is performed as in Dee et al. (2001), with observation and background errors estimated as in Dee and da Silva (1999). Randles et al. (2017) and Buchard et al. (2017) describe the overall methodology and validation of the MERRA-2 AOD reanalysis. For this study, monthly mean speciated AODs and total AOD at 550 nm with 0.5° latitude and 0.625° longitude spatial resolution were used.
2.1.4 NAAPS-RA v1
The Navy Aerosol Analysis and Prediction System (NAAPS; Lynch et al., 2016) is a global offline chemical transport model developed at the US Naval Research Laboratory. NAAPS simulates the life cycles of aerosol particles and their gaseous precursors. The particle species include anthropogenic and biogenic fine (ABF, i.e., a mix of sulfate, organic aerosols, and BC from non-BB sources), BB smoke, eolian dust, and sea salt aerosols. The transport, hygroscopic growth of particles, dry and wet removal processes of these particles, and emissions of wind-blown particles are driven by the meteorological fields from the Navy Global Environmental model (NAVGEM; Hogan et al., 2014) and previously the Navy Operational Global Atmospheric Prediction System (NOGAPS; Hogan and Rosmond, 1991). Secondary organic aerosol (SOA) processes are represented with a first-order-approximation method in which production of SOA from its precursors is assumed to be instant and is pre-treated outside the model. Anthropogenic emissions come from the MACC inventory from ECMWF (Granier et al., 2011). BB smoke emission is derived from the Fire Locating and Modeling of Burning Emissions (FLAMBE; Reid et al., 2009), which is constructed based on the MODIS fire hotspot data. In the reanalysis version, additional orbital corrections and regional emission factors are incorporated. Eolian dust emissions are determined based on the surface friction velocity to the fourth power, and surface erodibility, which is adopted from Ginoux et al. (2001) with regional tuning. Dust emission occurs when specific conditions related to surface wetness and friction velocity thresholds are met. The representation of sea spray process adheres to Witek et al. (2007), with sea salt emission being governed by sea surface wind conditions.
The NAAPS reanalysis (NAAPS-RA) v1 (Lynch et al., 2016) is derived from NAAPS, with assimilation of quality-assured and quality-controlled MODIS (Zhang and Reid, 2006; Hyer et al., 2011) and MISR AOD products (Shi et al., 2011) using 2D-var data assimilation method (Zhang et al., 2008). It provides 3D mass concentration, extinction, and 2D 550 nm AOD from these aerosol species with 1°×1° lat/long spatial and 6 h temporal resolution for 2003–2022. The BB smoke source and dust sources are regionally tuned to best match the FM and CM AODs with AERONET AODs. Aerosol wet removals within the tropical region were regulated with a satellite precipitation product (Xian et al., 2009) to mitigate the model's deficiency in simulating convective precipitation. The reanalysis shows a similar decadal trend of AOD found in satellite products (e.g., Zhang et al., 2017) and was verified with various field campaign data (e.g., Reid et al., 2016, 2023; Atwood et al., 2017; Edwards et al., 2022) in addition to ground- and space-based observations.
2.2 Multi-reanalysis consensus
The multi-reanalysis consensus (MRC) product is a result of combining the four individual aerosol reanalysis products described above. This method follows the multi-model-ensemble approach used by the International Cooperative for Aerosol Prediction (ICAP) and is based on the work by Sessions et al. (2015) and Xian et al. (2019). The data from each RA with spatial resolution different from 1°×1° lat/long degree are first projected onto the global map with 1°×1° lat/long degree resolution using linear interpolation. Then the MRC value is determined by calculating the average of the values from the four RAs. No weighting among the RAs is applied, or the four RAs are weighted equally in deriving the MRC. (Regionally weighted ensemble products based on the verification results shown here can be developed in the future.) The MRC provides speciated and total AOD at 550 nm with a 1°×1° lat/long degree and monthly resolution for the period 2003–2019. The MRC data for the period spanning from 2003 to 2010 rely on three RAs, while for the period from 2011 to 2019, it incorporates all four RAs, considering that JRAero data are only accessible starting from 2011.
Table 1Summary of the characteristics of the aerosol reanalyses. Note: DAQ means data assimilation quality; VOC stands for volatile organic compound.
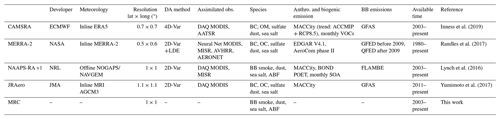
Table 2Parameters representing microphysical and optical properties of aerosol species from the four aerosol reanalyses. NA – not available.
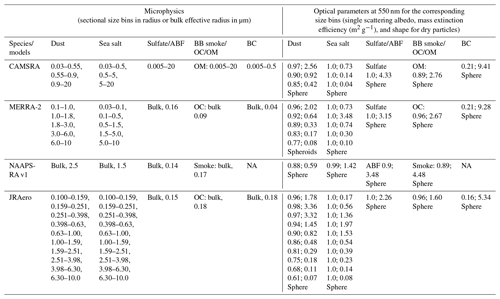
Table 3Hygroscopic enhancement factor (f) at different relative humidity (RH) levels for various aerosol species in the four RAs. In MERRA-2, f for sea salt varies with size bins, and thus a range for f is presented here. Notably, NAAPS-RA v1 does not explicitly contain BC species. More specific details can be found in the references provided in Table 1.
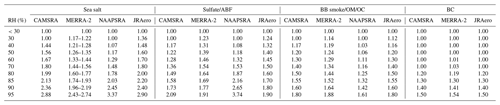
2.3 AERONET
AERONET is a global ground-based sun photometer network managed by NASA. Sun and sky radiance at multiple wavelengths, covering the near-ultraviolet to near-infrared spectra, are measured (Holben et al., 1998). Level-2 AERONET (version 3) daily data (Giles et al., 2019), which are cloud screened and quality assured, are used in this study. The estimated uncertainty in AERONET-measured AOD, due primarily to calibration uncertainty, is ∼0.01–0.02 at optical air mass of one for network field instruments (with the highest errors in the UV; Eck et al., 1999).
The 550 nm FM and CM AODs and total AODs are derived with the spectral deconvolution method (SDA; O'Neill et al., 2001, 2003). The AERONET SDA product has been verified using in situ measurements (see, for example, Kaku et al., 2014). The spectral separation of FM and CM particles is determined based on their distinctive optical properties and complete size distributions. As part of this separation, a diameter of approximately 1 µm serves as an approximate threshold to differentiate FM and CM particles. This optical separation is different from the submicron fraction (SMF) method that uses a specified cutoff radius of the particle size distribution in the AERONET (AOD and sky radiance) inversion and allows more data to be available compared with the SMF method. The FM fraction based on SDA is generally comparable to, and slightly greater than, that based on SMF (O'Neill et al., 2023).
This study uses AERONET sites that have more than 5 years of observations and more than 1000 daily data between 2011 and 2019 for verification purposes. Monthly AOD was derived for months that have more than 15 d of daily data. Then, only sites with a total number of months of more than 45 (upper three quartiles of sites regarding total number of monthly data) were selected. This resulted in a total number of 200 sites globally. The list of sites along with lat/long coordinates and elevation details for the studied regions is accessible in Table S1 in the Supplement. Additionally, the locations of all sites can be identified in Fig. 8.
2.4 MODIS AOD
Three MODIS AOD products are used as reference datasets to show global distribution of AOD climatology and the divergence among the retrieval products in comparison with the RAs. The level-3 MODIS AOD data for Dark Target (DT) were constructed using collection 6.1 Aqua MODIS level-2 DT data. The level-2 MODIS-DT aerosol retrievals are available at a 10×10 km2 spatial resolution over both land and ocean. These aerosol retrievals were initially averaged on a daily basis at a spatial resolution of 0.5×0.5° lat/long. Only data with a quality flag of “marginal” or better were used in the analysis. Additionally, retrievals with a cloud fraction larger than 80 % were excluded to minimize cloud contamination, as suggested by Zhang et al. (2005). The level-3 MODIS-DT AOD data (0.5×0.5° lat/long) were then constructed using the daily averaged AOD data.
Similar approaches were applied to C6.1 Aqua MODIS level-2 Deep Blue (DB) AOD data. Unlike the MODIS-DT aerosol retrievals, which are available over regions with low surface reflectance, the DB retrievals are also available over some bright regions, such as desert regions. No over-ocean aerosol retrievals, however, are included in the MODIS level-2 aerosol data. The level-2 MODIS-DB aerosol data were used to construct daily averages at a spatial resolution of 0.5×0.5° (lat/long). No quality flag and cloud fraction thresholds were applied. The level-3 MODIS-DB AOD data (0.5×0.5° lat/long) were constructed using the daily averaged AOD data.
The third MODIS AOD product is a data-assimilation-quality AOD dataset. It was based on C6.1 DT and DB retrieval products (Levy et al., 2013). Strict quality-control and bias-correction processes were applied as described in Zhang and Reid (2006) and Shi et al. (2011) for over water, Hyer et al. (2011) for over land, and Shi et al. (2013) for over desert regions. These quality-control processes were updated for the C6.1 data and the final MODIS C6.1 AOD (550 nm) data are a level-3 product with 1°×1° lat/long spatial and 6 h temporal resolution. This product has a cutoff at 40° S to filter out potential cloud-contaminated data south of this latitude. The 6 h-averaged AOD data were then binned into monthly means.
Note that MODIS AOD products are well known in the context of low bias significant aerosol events (e.g., Reid et al., 2022; Gumber et al., 2023) and slightly high bias clean environment (e.g., Wei et al., 2019), which could affect AOD climatology to some degree.
2.5 Analysis method
This study aims to investigate the divergence and utility of RAs for climate-scale studies by exploring the AOD at 550 nm. To achieve this goal, the AOD data from the RAs as well as MODIS were spatially and temporally binned into 1°×1° degrees and monthly resolutions. For the purpose of verification and intercomparison analysis, only the data between 2011 and 2019 were used as that is the period when all the RAs have data. The study focuses on the 550 nm AOD parameter since it is available for all four aerosol RAs and MODIS. Furthermore, the AERONET FM and CM AODs at 550 nm were obtained using the SDA method described in Sect. 2.3.
The study examines the performance of RAs globally and regionally. A total of 16 regions, including the globe, are defined for regional aerosol property analysis. They include East Asia, Southeast Asia, South Asia, the Maritime Continent, Australia, southwest Asia, Europe, northwest Africa, South Africa, western North America, eastern North America, Central America, and South America, as indicated by the rectangular boxes in Fig. 5, as well as the Arctic (north of 70° N), and Antarctic (south of 75° S). There is no AERONET site satisfying site selection criteria as described in Sect. 2.3 in the Arctic and Antarctic, so these two regions are excluded for regional verification, though they are included in other analyses.
Regarding the aerosol species, the study focuses on BB smoke, ABF in NAAPS-RA, and its equivalent of sulfate for MERRA-2, CAMSRA, and JRAero, as well as dust and sea salt. The definition of species follows the ICAP practices (Sessions et al., 2015; Xian et al., 2019) for the operational counterparts of these RAs and previous applications of these RAs (e.g., Xian et al., 2022), in which the sum of OM and BC AODs from CAMSRA, and the sum of OC and BC AODs from MERRA-2 and JRAero, is used to approximate BB smoke AODs. Although this separation of species may be somewhat arbitrary, the study takes into account the fact that different aerosol types and sources may be represented differently in each RA. For example, the NAAPS-RA model characterizes aerosol species by emission source rather than chemical speciation, which makes it unique. In contrast, CAMSRA, MERRA-2, and JRAero characterize OM or OC, BC, and inorganic species, merging contributions from various anthropogenic, biomass burning, and biogenic sources.
The study also assumes that all sea salt and dust are CM, while other aerosol species are FM. The segregation of sea salt and dust to the CM category is based on the fact that only a small portion of total sea salt or dust AOD at 550 nm is attributed to their FM components. For example, FM sea salt represents about 17 %, 10 %, and 11 % of total sea salt AOD globally in MERRA-2, CAMSRA, and JRAero, respectively. The numbers are about 30 %, 39 %, and 32 % for dust, while the FM fraction of dust during dust storms in Africa varies between 20 % and 25 % according to AERONET. The FM fraction of dust from MERRA-2, CAMSRA, and JRAero might be biased high as these global models tend to overestimate FM dust and underestimate CM dust (e.g., O'Sullivan et al., 2020; Kramer et al., 2020). In contrast, NAAPS-RA assumes all sea salt and dust are CM. Verification results based on the FM and CM AODs derived using the FM fractions of sea salt and dust from MERRA-2, CAMSRA, and JRAero can be found in the Supplement (Figs. S2–S4). Generally, the validation of FM and CM AODs with AERONET data shows a degradation in performance for the three RAs compared with the verification results presented below, as discussed in Sect. 3.3.1.
For every AERONET site, the time series of monthly modal AOD from each RA is first extracted from the model grid that encompasses the site's location. Bias, root mean square error (RMSE), and coefficient of determination (r2) are then computed for each site and each RA. The regional validation outcome is derived from the average of validation statistics across all sites within the region. (See Table S1 for the sites included in each region.) Following the criteria for site selection outlined in Sect. 2.3, only 200 sites are available globally, and certain regions have only a few sites (a minimum of three sites, such as in South Africa) to represent the entire region; hence, no site weighting within a region is applied. It is acknowledged that this averaging method could bias the global validation result toward regions densely populated with sites, notably North America and Europe. The AOD validation results for total, FM, and CM AOD at 550 nm are presented accordingly.
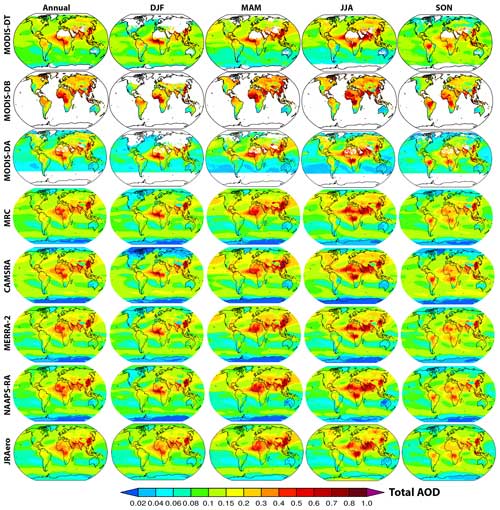
Figure 1Annual and seasonal total 550 nm AOD climatology from three MODIS products, the four RAs, and the MRC over 2003–2019, except JRAero for 2011–2019. MODIS-DA is the data-assimilation-quality AOD dataset described in Sect. 2.4. In the MODIS plots, the white area means a lack of data attributed to either no valid retrievals or quality-control filtering. Notably, MODIS-DB data are only available over land.
3.1 Total and speciated AOD climatology
The climatological annual and seasonal mean total AODs at 550 nm from the three MODIS AOD datasets and the four aerosol RAs as well as the MRC are presented in Fig. 1. In general, there are very similar spatial AOD distribution patterns and AOD magnitude among the RAs and MODIS datasets for all four seasons. This is expected as MODIS total AOD is assimilated into all of these RA products as well as used to tune the model components such as emissions. High AOD regions include the dust-dominated Sahara in March–April–May (MAM) and June–July–August (JJA), Sahel in December–January–February (DJF) and MAM, southwest Asia and Taklamakan in MAM and JJA, anthropogenic pollution-dominated East Asia and South Asia throughout the year, BB smoke-dominated South Africa, South America in JJA and September–October–November (SON), Southeast Asia in MAM, the Maritime Continent in SON, and high-latitude North America and Eurasia in JJA. For the annual mean, MODIS AODs from all three products are relatively high compared with the MRC in the Northern Hemisphere's high latitudes due to seasonal sampling bias. MODIS was able to retrieve AOD during biomass burning active season, i.e., boreal summer to fall, but it could not retrieve AOD during northern winter in the high latitudes due to the lack of sunlight and the high snow/ice coverage. The high AOD over high-latitude Eurasia and North America in the MODIS annual mean is a general reflection of MODIS summertime AOD, which is captured by all the RAs in their summertime mean AODs.
It is worth noting that MODIS-DB AOD generally exhibits slightly higher values compared with MODIS-DT AOD, except in high terrain regions (e.g., western North America). On the other hand, MODIS-DA AOD tends to be slightly lower (approximately 0.02 magnitude) than MODIS-DT AOD over oceanic regions due to bias-correction procedures. When compared with MODIS-DT, AODs from the RAs tend to align more closely, especially over oceanic areas. Furthermore, RAs typically exhibit lower AODs compared with MODIS-DB over regions affected by African and Arabian dust. Overall, the divergence in total AOD climatology among the RAs is comparable to or even smaller than the divergence observed in the MODIS products.
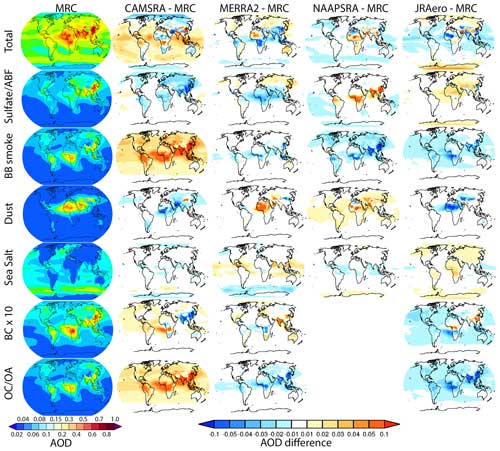
Figure 2Annual mean total and speciated AODs of the MRC and the AOD difference between the individual RA and the MRC based on the 2011–2019 average. BB smoke is approximated as the sum of OC/OM and BC in CAMSRA, MERRA-2, and JRAero.
Previous experience with multi-model ensembles suggests that the consensus of multi-models, in general, shows better skill than individual contributing models (Sessions et al., 2015; Xian et al., 2019; Reid et al., 2022). A similar verification conclusion is also drawn in Sect. 3.3. Therefore, the total and speciated AODs from the MRC based on the 2011–2019 average are used as a baseline here and are shown in Fig. 2. As expected, sulfate/ABF AOD is relatively high over population-dense and industrially polluted regions, dust AOD is high over major desert and arid regions, and sea salt AOD is relatively high over mid-to-high-latitude oceans. BB smoke and its components BC and OC/OM are relatively high over major BB source regions in South Africa, South America, Southeast Asia, the Maritime Continent, Siberia, and high-latitude North American areas. BC and OC/OM AOD are also relatively high over South Asia and East Asia, where sources other than BB, such as anthropogenic emission, are the main contributors, as suggested by contrasting smoke AOD contribution to the total AOD between NAAPS-RA and other RAs in these regions. (See Figs. 3 and 10, noting that smoke AOD is driven by BB in NAAPS-RA, while smoke AOD is a sum of BC and OC/OM from the other RAs.)
Shown also in Fig. 2 are the total and speciated AOD differences between the individual RA and the MRC. For total AOD, CAMSRA is apparently higher than the other three RAs over the ocean, which is consistent with the findings on its operational counterpart of high biased FM AOD verified with Maritime Aerosol Network over the ocean in Reid et al. (2022). This high bias is attributed to its universally higher OM/smoke AOD compared with other RAs and suggests that CAMSRA may have higher BB emissions and/or higher secondary production of OM compared with other RAs. Sulfate AOD is relatively low in CAMSRA except for some highly biased hotspots around outgassing volcanoes (in particular Mauna Loa and near Mexico City) as mentioned in Inness et al. (2019). Differences in species definitions affect the comparison with NAAPS-RA: NAAPS-RA ABF AOD is higher than sulfate AOD in other RAs especially in East Asia, South Asia, central Africa, and northern South America, and these deviations are counterbalanced by opposite deviations in the BB AOD. This is expected as ABF in NAAPS-RA includes additional aerosol sources besides sulfate, and some of these sources are included in the BB AOD for other models. For dust AOD, MERRA-2 is relatively higher over North Africa and the Arabian Peninsula, and NAAPS-RA is relatively higher over most regions, including oceanic areas, while CAMSRA and JRAero are relatively lower over most regions except around the Gobi Desert for CAMSRA and Iran for JRAero. As for sea salt AOD, MERRA-2 is relatively higher over the tropical oceans and lower over the southern oceans. JRAero sea salt AOD is relatively higher over most continents, which is probably biased.
The differences in speciated AOD result in significant variations in their contributions to the total AOD, as illustrated in Fig. 3. For instance, the considerably higher BB smoke AOD in CAMSRA compared to other RAs makes BB smoke the predominant contributor to the total AOD in the CAMSRA over most continents, adjacent water bodies, and polar regions, except for regions where dust is dominant. Sulfate AOD, on the other hand, contributes more to the total AOD particularly over oceanic regions in the JRAero compared with other RAs. Both MERRA-2 and JRAero exhibit higher sulfate contributions along the western coasts of South America and North America, suggesting possible increased production of dimethyl sulfide (DMS) in those areas. Dust AOD, on the other hand, contributes more to the total AOD particularly over oceanic regions in NAAPS-RA compared with other RAs. Sea salt AOD is found to contribute more to the total AOD in the high-latitude oceans and the Antarctic in NAAPS-RA compared with the other RAs. The OC/OM AOD contribution to the total AOD closely mirrors the distribution of BB smoke, as anticipated. The contribution of BC to the total AOD is generally small, ranging between 5 % and 10 % in BB regions, except for central South Africa where it reaches 10 %–15 %. Despite the higher ratio of BB smoke AOD to the total AOD in CAMSRA, the ratio of BC to the total AOD over East Asia and South Asia is smaller in CAMSRA compared with MERRA-2 and JRAero, suggesting that BC emissions from anthropogenic sources may be lower in CAMSRA (also Fig. 2). Finally, the contributions of FM and CM AOD to the total AOD are also depicted in Fig. 3. It is consistent among the RAs that FM is the dominant contributor over most land regions, except for regions where dust is dominant such as North Africa, the Arabian Peninsula, the Middle East, and the Gobi Desert. In all the RAs, CM is the dominant contributor over oceanic regions, except for regions influenced by continental BB smoke and pollution outflow. The contribution of CM in CAMSRA is generally smaller in tropical to mid-latitude oceans compared with other RAs, due to its higher contribution from BB smoke. It is also noted that CM is dominant over FM in the Antarctic in NAAPS-RA, while FM is dominant in the Antarctic in the other three RAs, though total AOD is very small (annual and seasonal means <0.04 from MRC) and hard to validate due to lack of observational data.
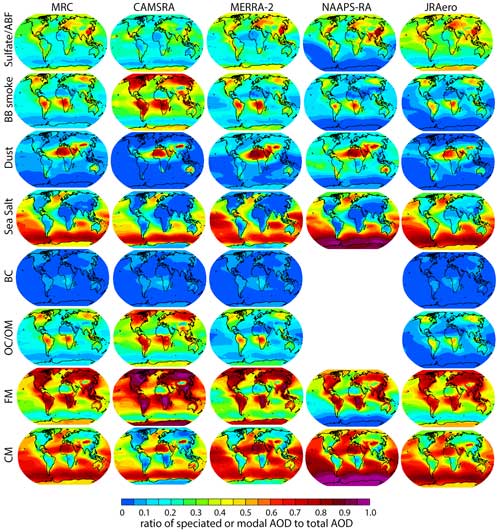
Figure 3Ratio of speciated AODs as well as FM and CM AODs to the total AOD from the MRC and the individual RAs based on the 2011–2019 annual average.
Table 4 provides a summary of global-average total AOD and speciated AODs, as well as the contributions of speciated AOD to the total AOD for all the RAs. Overall, the annual and global mean total AODs are similar, hovering around 0.14 for most RAs. All land and ocean mean AODs are within 0.006 of the MRC with the exception of CAMSRA over ocean, which is higher than the MRC by +0.024.
Speciated AODs, especially smoke AOD and OM/OC AOD, display greater divergence among the RAs. Smoke and OM AODs from CAMSRA are 2–3 times higher than those from the other RAs. Smoke AOD contributes to 41 % of the total AOD in CAMSRA, while ranging from 16 % to 22 % in other RAs. Moreover, the standard deviation of smoke and OM AODs with respect to the 12 months is also higher in CAMSRA than in other RAs. The contribution of dust AOD to the total AOD varies from 13 % to 28 % for all the RAs, with NAAPS dust AOD being the highest among the RAs and about 2 times that of CAMSRA, which has the lowest dust AOD among the RAs. The contribution of sulfate/ABF AOD to the total AOD ranges from 23 % to 34 %, with the highest contribution observed in JRAero, even larger than the ABF AOD contribution in NAAPS-RA. Sea salt AOD contributes 25 %–35 % to the total AOD in the RAs with JRAero being the highest. BC AOD, on the other hand, contributes only 3 %–4 % of the total AOD across the RAs. The FM's contribution to the overall AOD varies across different datasets. In MERRA-2, NAAPS-RA, and JRAero, FM accounts for 44 %–51 % of the total AOD. However, in CAMSRA, its contribution is notably higher, at 63 %, primarily due to its significant contribution from OM. Conversely, CM's contribution to the total AOD is consistent across the three RAs, ranging from 49 % to 56 %. In contrast, CM's contribution is lower, at 37 %, in CAMSRA.
Table 4Global area-weighted mean modal (total, FM, and CM) and speciated AOD, as well as standard deviation of monthly AOD based on 2011–2019 data. Percentage numbers in parentheses are contributions of speciated AOD to the total AOD. Global mean total AODs over land and water are shown in the last two rows.
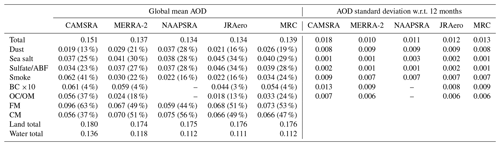
3.2 Geographical divergence of speciated AOD among the four RAs
The divergence of the global-average total and speciated AODs is already documented in Table 4. Figure 4 provides the geographical distribution of the relative spread of speciated annual mean AODs from the RAs to their means. Spread, in this context, is defined as the ratio of the standard deviation of the RA AODs to their means. It is noteworthy that the relative spread of the total AOD from the four RAs is generally small, except for polar regions and specific hotspots where known issues exist. For instance, biases in CAMSRA AOD have been identified over Hawaii and Mexico's volcanic outgassing regions. In polar regions, there are limited satellite observations to constrain model fields, resulting in a larger spread, which is consistent with the findings of Xian et al. (2022) on AODs from CAMSRA, MERRA-2, and NAAPS-RA over the Arctic. Similarly, over high terrains with snow and ice covers, such as the Himalayas and the Andes, and over desert regions, such as the Australian deserts, and the Bodélé Depression region in the Sahara, both retrievals and models face challenges, leading to a larger spread. Moreover, over the Maritime Continent, where high cloud coverage poses challenges to remote sensing retrievals for both AOD and BB smoke emissions, the spread is also relatively large.
The aforementioned characteristics are also evident in the spread of speciated AODs. However, the spreads of the speciated AODs among the RAs are much larger compared with the total AOD, particularly in regions that are remote from aerosol sources. This suggests that the efficiency of removal processes during long-range transport may differ. This is also relevant to the fact that data assimilation constrains the total AOD, but speciated AOD remains unconstrained. Moreover, the disparities in definitions of species, such as sulfate/ABF, BB smoke, and OC/OM, as discussed in Sect. 2.5, can also influence the spread of these FM species. The relative spread of speciated AODs being much larger than that of the total AOD is broadly consistent with the AeroCom results, where global climate models (without data assimilation) were intercompared in terms of aerosol optical properties and life cycles (Kinne et al., 2006; Textor et al., 2006; Gliß et al., 2021).
3.3 Evaluation with AERONET AOD
This section presents evaluation of the monthly performance of the four RAs plus the MRC at the AERONET sites on regional and global scales. Both skill and consistency of the different RAs and consensuses are evaluated.
3.3.1 Bias, RMSE, and correlation between the RAs and AERONET
The regional and global mean modal AOD bias, RMSE, and coefficient of determination for the four RAs and the MRC are shown as bar graphs on global maps in Figs. 5–7. Regarding regional bias, all the RAs, except for CAMSRA, have large negative biases (on the order of −0.1) in total AOD over Southeast Asia, South Asia, and the Maritime Continent (Fig. 5). The much smaller negative bias in total AOD over these regions in CAMSRA is a result of the cancellation of a positive bias in FM, possibly due to high biased OM/smoke AOD, and a negative bias in CM. The large negative biases over these regions in the other RAs are mainly attributed to large negative biases in FM AOD in general. It is also noted that CAMSRA is biased relatively high in total AOD due to high FM bias over East Asia. Over other regions and the globe, all the RAs have relatively small biases and in general slight positive biases, with CAMSRA having the largest positive bias, due mainly to relatively high OM/smoke AOD. The cancellation effect of positive FM bias and negative CM bias in CAMSRA are also visible.
Total AOD RMSEs are relatively high over all Asian regions and North Africa compared with other regions for all the RAs (Fig. 6). The contribution of FM to the total AOD RMSE is larger than that from CM globally, except over dust-influenced regions, including North Africa and, for most models, southwest Asia and Central America. The correlations of the total AOD between the RAs and AERONET data are mostly reasonable for all the regions (Fig. 7). Some relatively low-performance regions (total AOD r2 less than 0.60 for at least one RA) include South Asia, southwest Asia, Australia, Europe, and East Asia. The relatively low correlations over Australia and Europe are due to the low climatological mean and variance, while the other low-performance regions are all mixed pollution and dust environment that is challenging for all RAs. Some relatively high-performance regions (total AOD r2 greater than 0.85 for at least two RA members) include Central America, peninsular Southeast Asia, and the Maritime Continent. Total and CM AOD r2 are high over Central America, because it is a receptor region for African dust, and RAs perform well in general during long-range transport over oceans where data assimilation is very effective in correcting model AOD fields. Total and FM AOD r2 are high over peninsular Southeast Asia and the Maritime Continent, suggesting that the RAs can capture the large interannual variabilities in the regional dominant aerosol species, BB smoke, associated with the impact of ENSO on fire activities in the regions (e.g., Reid et al., 2012; Xian, et al., 2013). Overall, the MRC exhibits superior r2 compared with individual RAs for modal AODs regionally and globally.
For remote marine sites, including Ascension Island in the mid-basin of the South Atlantic, Ragged Point in the western tropical Atlantic, Mauna Loa in Hawaii, MCO-Hanimaadhoo in the North Indian Ocean, and Réunion in the South Indian Ocean, the RAs exhibit similar performance at these sites to the performance over the upwind land or coastal regions (Fig. S1). An exception is Mauna Loa. Mauna Loa is situated at an elevation of 3.4 km, well above the marine boundary layer and remote from continental sources. At this location, all the RAs exhibit a significant positive bias. One possible explanation for this bias is the topographic effect, as the coarse spatial resolutions of the models may not be able to resolve the site's high elevation or its sharp elevation gradient compared with the surroundings. Additionally, uncertainties in the removal processes during long-range transport may also be contributing to the high bias. It is also worth noting that all the RAs do especially well at the Ragged Point site, with total AOD r2 close to or higher than 0.92. This site is a receptor site of African dust in the western tropical Atlantic. This suggests that the RAs capture the long-range transport of dust from Africa quite well. This is related to the fact that data assimilation systems have more chance to correct the model fields with observations in the long-range transport over the ocean.
When considering the contribution of dust and sea salt aerosols to FM AOD in CAMSRA, MERRA-2, and JRAero, the verification statistics (bias, RMSE, and r2) for the total AOD of these RAs remain unchanged as expected (Figs. S2–S4). However, there is a noticeable shift in the positive bias of FM AOD (and negative bias of CM AOD) for these RAs, particularly in regions influenced by dust, such as North Africa, the Arabian Peninsula, East Asia, Central America, South Asia, and Europe. Specifically, the positive bias in FM AOD becomes more pronounced and the negative bias in CM AOD becomes more negative in these regions, especially for CAMSRA. It is worth noting that in MERRA-2, there is a change in sign, where the FM AOD bias switches from negative to positive in North Africa and the Arabian Peninsula, while the CM AOD bias changes from positive to negative in these regions. Additionally, the negative FM AOD bias becomes smaller; however, the negative CM AOD bias worsens in South Asia within both MERRA-2 and JRAero datasets (Fig. S2). In general, when taking into account the contribution of dust and sea salt aerosols to FM AOD (by default, dust and sea salt AODs are treated as CM AODs in this study) in CAMSRA, MERRA-2, and JRAero, we observe a worsening of both FM and CM AOD biases in these three datasets. Similarly, the RMSE for both FM and CM AODs over regions influenced by dust deteriorates as well (Fig. S3). The r2 for FM and CM AODs in these regions also worsens overall, with the exception of an improvement in FM AOD over Central America. FM sea salt's impact on the verification score is small as the majority of AERONET sites are on land and FM sea salt only contributes on the order of ∼10 % to total sea salt AOD in the three RAs.
3.3.2 Rankings of the RAs with respect to validation statistics
To expand the validation result from regional averages to individual sites, including remote sites that are not included in the regional analysis, rankings of the RAs in terms of RMSE of monthly total AOD at all the AERONET sites are displayed in Fig. 8. It shows that there are cases in which individual RA ranks first over some regions. For example, CAMSRA ranks relatively better than others in South Asia and Southeast Asia, MERRA-2 ranks better over North Africa and the Arabian Peninsula, NAAPS-RA ranks better over North America and Europe, while JRAero performs relatively better over southern North America and the Caribbean. Individual RA has mixed results for sites in other regions. AOD RMSE of the MRC is not always the lowest for a given site, but it is relatively low and stable over the globe. This is consistent with the regional RMSE result (Fig. 6). The consensus wins because of its averaging of independent models. This is consistent with our findings with the ICAP models (Sessions et al., 2015; Xian et al., 2019).
Challenging sites for these RAs are found as marked by the magenta color in Fig. 8. These sites exhibit an r2 value of less than 0.25 and are associated with relatively large AOD bias and/or RMSE. Often, when a challenge occurs, it is a common challenge to all models and no specific model is much better than the others. Some of the causes for the challenges include lack of representation or large uncertainty in local emissions (e.g., Modena in northern Italy, Mainz in Germany, Cairo in Egypt, Trelew and CEILAP-RG sites in Argentina) and/or topographic effects that are not resolved in these RAs due mostly to coarse-mode spatial resolutions (e.g., Mauna Loa), as well as sites that are impacted by mixed pollution and dust (e.g., Dushanbe in Tajikistan).
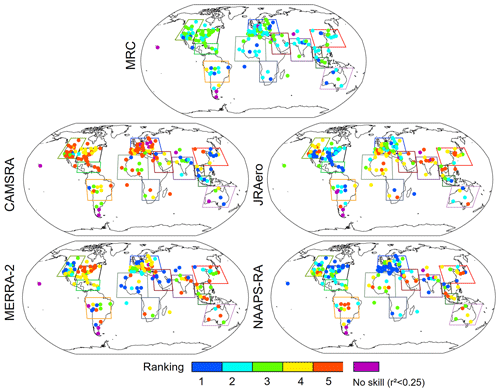
Figure 8Ranking of aerosol RAs in terms of RMSE of monthly total AOD at 550 nm over all the AERONET sites. Rectangles are used to delineate regions for regional validation, as depicted in Figs. 5–7. A lower RMSE indicates better performance, with a ranking of 1 being the most desirable. AERONET sites with a coefficient of determination (r2) less than 0.25 are marked in magenta, indicating a lack of skill from the model.
Ranking analyses were also conducted on the RMSEs of FM and CM AODs, absolute bias, and r2 of modal AODs. Figure 9 presents the MRC rankings for all these comparison statistics. In line with the MRC ranking for the total AOD's RMSE, the MRC rankings for other metrics are predominantly ranked first or second, except for the absolute biases, where MRC rankings are often ranked third over North America, South America, and Europe for total and FM AODs. For these modes and over these regions, all the RAs have positive biases relative to AERONET. When the biases are in the same sign (positive or negative), it is mathematically natural for MRC to rank in the middle. For CM and FM AODs, there are more sites with r2<0.25 compared with the total AOD. These sites mostly have small values of CM or FM AODs and reside in regions of opposite-mode dominance, such as FM in the Saharan region as well as CM in northern Europe and North America. From another perspective, the MRC ranking with respect to correlations is superior to RMSE and then absolute bias. In other words, the MRC better captures aerosol variance than the individual models, but it is nevertheless subject to overall model biases. The MRC ranking for CM AOD is slightly superior to that of the total AOD and then FM AOD. While the MRC ranking is not consistently at the top for a given site or region, it is relatively high and stable, ranking first for the global average. No individual RAs could compete with the MRC in that sense.
3.4 Seasonality of regional AODs
In Sect. 3.1 we depict the spatial distribution of the total AODs from all the RAs across the four seasons. In this section, we provide monthly time series of AOD and AOD interannual variabilities for 16 regions (Fig. 10), along with the contributions of speciated AOD to the total AOD for these regions for four seasons and the annual mean. All the RAs exhibit a similar seasonality and interannual variability in the total AOD for all regions, except for the Antarctic and Arctic, particularly during their winter seasons. This disparity arises from the absence of passive satellite AOD data during polar winter, which limits the effect of data assimilation on model AOD (see Xian et al., 2022 for the Arctic region). Even during polar summer, AOD retrievals are often unavailable due to high reflectance from surface ice/snow. The total AOD in JRAero exhibits exceptionally high levels, primarily attributed to elevated sea salt and sulfate AODs (Fig. S5). This anomaly stems from the MASINGAR model used to produce JRAero, which tended to underestimate the removal of aerosols via cumulus convection. Consequently, this led to an overestimation of aerosol concentrations in the polar regions and the upper atmosphere. The underestimation of the removal process has been resolved in the current MASINGAR model and the overestimation of AOD over the polar regions will be improved with the JRAero version upgrade. Nevertheless, the polar regions demonstrate the most significant divergence among the RAs in the seasonal cycle and speciation of AOD.
The regions that are dominated by BB smoke, including South Africa, South America, the Maritime Continent, peninsular Southeast Asia, and western North America, exhibit consistent peak seasons of the total AOD with their respective burning seasons. The Maritime Continent and peninsular Southeast Asia experience extremely large interannual variations in peak monthly AOD, owing to a strong positive correlation between burning activities and El Nĩno cycles (e.g., Reid et al., 2012; Xian et al., 2013). The contributions of sulfate/ABF AOD induced by pollution are dominant in East Asia and South Asia, while other aerosol species also make a significant contribution to the total AOD. In Europe and eastern North America, sulfate/ABF is also the dominant species; however, the monthly total AOD values are much smaller. All the RAs capture the dominance of dust species in summertime over southwest Asia and northwest Africa. The relatively high AOD in spring in northwest Africa is partially due to BB in Sahel. In Australia, the peak AOD from October to December is associated with BB smoke. In Central America, the relatively high AOD in spring results from BB smoke. Although quite diverse in AOD magnitude, all RAs tend to have a summertime total AOD peak attributed to dust. For the global average, sea salt AOD has a significant contribution to the total AOD as the area of ocean overwhelms the area of land. Monthly time series of the speciated AODs for all the regions are available in Fig. S5. Overall, the seasonality and interannual variability in total AOD for most regions is very similar among the RAs. Moreover, all RAs have the same dominant species for most regions, but the contributions from different species can be quite different in these RAs. This is a result of the fact that the total AOD is constrained within these RAs through data assimilation, while speciated AODs are not. Aerosol speciation and the contribution of each species to the total AOD are determined by the construction of the aerosol forecast models, which are very independent in these RAs.
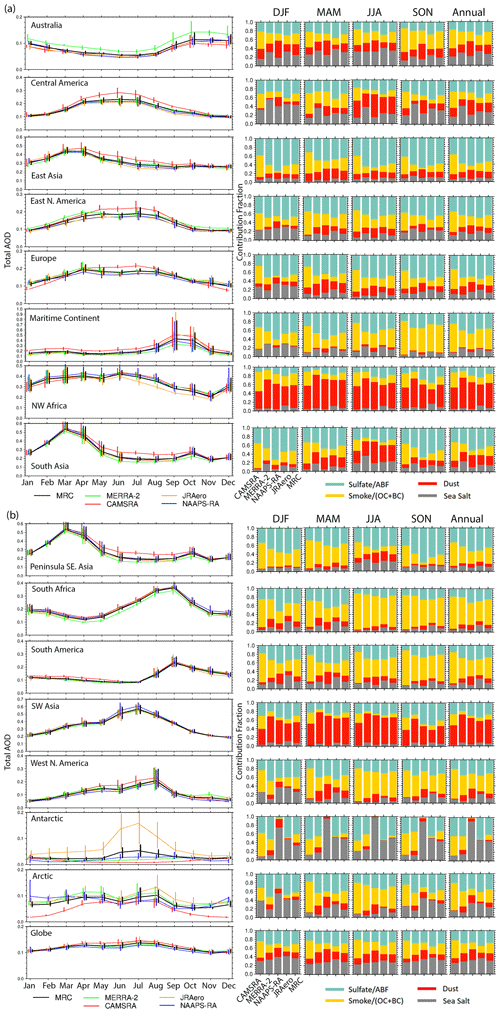
Figure 10Climatological seasonal cycle of regional mean total AOD (left) and the contribution fraction of speciated AOD to the total AOD for the corresponding regions and seasons from the four RAs and the MRC (right). In the seasonal cycle plots, bars denote the interquartile range of monthly mean AOD, illustrating interannual variabilities for the period 2011–2019.
3.5 Urban versus rural areas
To evaluate the RAs for urban versus rural areas, three paired sites were selected. Beijing (China), Yonsei University (South Korea), and Kanpur (India) represent urban areas, while their corresponding rural areas are represented by the Xiang He, Anmyon, and Gandhi College sites among the available AERONET sites. Figure 11 shows the monthly time series of modal AODs from the RAs and the MRC, along with their validation statistics against AERONET data. The dominant aerosol mode is FM at all these sites, due mostly to pollution. These sites are also subject to the influence of dust storms in spring, which contribute to CM AOD. The modal AODs from the four RAs and the MRC generally follow those of AERONET seasonally. The spread among the RAs is relatively large for the Chinese and Indian sites. The spread is relatively small for the Korean sites, with the spread being slightly less for the rural site Anmyon than for its corresponding urban site Yonsei University. Regarding bias, RMSE, and r2, there is no significant difference between the urban and the corresponding rural sites for each RA and the MRC, despite that r2 of the total AOD tends to be higher for the rural sites than the urban sites. The r2 of FM AOD also tends to be higher than that of the CM. The RAs and the MRC also capture the decreasing AOD trend in the latter half of the 2011–2019 period for the Chinese and Korean sites. (A more detailed trend analysis will be provided in a companion paper.) For the ranking of all RAs in terms of bias, RMSE, and r2, each individual RA has a few first rankings. MERRA-2 is especially better compared with other RAs at CM/dust AOD for the Indian sites. But in terms of the number of ranking first, the MRC is the winner for all the sites (having at least five out of nine statistical variables ranking first for each site).
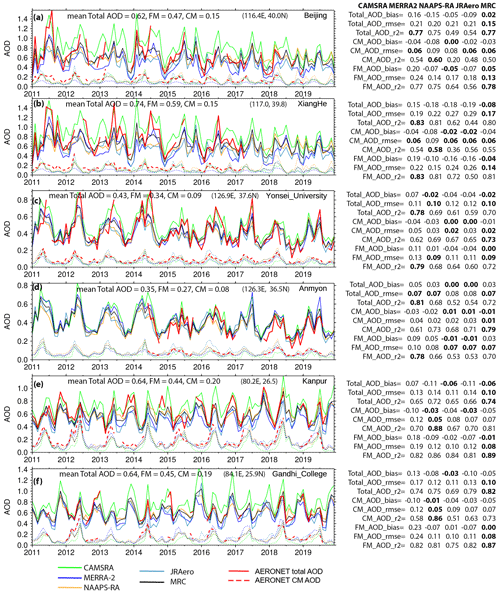
Figure 11Evaluation of total, FM, and CM monthly AODs from the RAs at urban versus rural AERONET sites. Sites (a), (c), and (e) represent urban locations in China, Korea, and India, respectively, while sites (b), (d), and (f) denote their corresponding rural sites. Mean total, FM, and CM AODs from AERONET data are presented in the upper panels of the time series plots for each site. The right column displays verification statistics for the four RAs and the MRC, including bias, RMSE, and r2. Values in bold indicate the lowest bias or RMSE, or the highest r2, signifying the best ranking among all the RAs.
This study compares the monthly average total and speciated aerosol optical depths (AODs) from four different aerosol reanalyses (RAs). These RAs include the Copernicus Atmosphere Monitoring Service ReAnalysis (CAMSRA) developed by Copernicus/ECMWF, the Japanese Reanalysis for Aerosol (JRAero) developed at the Japan Meteorological Agency (JMA), the Modern-Era Retrospective Analysis for Research and Applications version 2 (MERRA-2) developed by NASA, and the Navy Aerosol Analysis and Prediction System reanalysis (NAAPS-RA) version 1 developed by the US Naval Research Laboratory. The consensus of the four RAs is also developed for intercomparison. The AODs from these RAs are evaluated with AEROsol Robotic NETwork (AERONET) and the MODIS Dark Target/Deep Blue retrievals (Levy et al., 2013; Sayer et al., 2014) using data from 2011 to 2019. The following are the conclusions drawn from this study:
-
Global distribution and magnitude of the total AOD demonstrate a high level of similarity among all four RAs. The spread of the total AOD among the RAs is small over most regions. Exceptions, where the RAs diverge in total AOD, are polar regions and areas affected by specific factors that include volcanic outgassing, high terrain, and certain desert regions.
-
The relative spread of speciated AODs is considerably larger than that of the total AOD. CAMSRA consistently yields higher values for biomass burning (BB) smoke or organic matter (OM) AOD in comparison with other RAs. Meanwhile, NAAPS-RA exhibits generally higher dust AOD values. JRAero has comparatively high biased inland sea salt AOD. The divergence of speciated AODs in regions remote from aerosol sources is large, implying different efficiencies in removal during long-range transport. This phenomenon results from the fact that data assimilation in these RAs constrains total AOD but not speciated AOD.
-
The seasonality and interannual variability in total AOD in the 16 regions under study, with the exception of the Antarctic and Arctic, demonstrate a high degree of similarity across the various RAs and align with the observations. While the dominant species of aerosols are consistent across most regions in all RAs, the relative contributions from individual species can vary significantly.
-
The accuracy of the RAs, as measured by RMSE, bias, and correlation of the total, fine-mode (FM), and coarse-mode (CM) AODs (i.e., modal AODs), has been verified with AERONET. It is evident that each RA exhibits its own unique regional strengths. Specifically, CAMSRA performs better in South Asia and Southeast Asia, MERRA-2 excels in African and Arabian Peninsula dust regions, NAAPS-RA shows relatively better performance over Europe and the eastern United States, and JRAero performs relatively better over southern North America and the Caribbean. Common challenges to all the RAs often include lack of representation or large uncertainty in local emissions, and/or topographic effects, as well as situations where both FM and CM states are mixed. There is no significant difference in RA performance for urban versus rural areas, despite that rural areas tend to have slightly higher AOD correlations with observations. RAs show the worst performance in areas impacted by mixed FM and CM aerosols, such as South Asia and East Asia. Areas that experience substantial interannual variability in AOD, for instance, Southeast Asia and the Maritime Continent, also have high bias and RMSE. However, correlations remain relatively strong, indicating that the RAs effectively capture the interannual and seasonal variations in AOD linked to BB events in these regions. The polar regions present a challenge due to limited observations.
-
The multi-reanalysis consensus (MRC), an ensemble mean of the four RAs, is not consistently the best performer in terms of RMSE, bias, and correlation of modal AODs for a given site or region. However, the MRC generally performs relatively well and remains stable, ranking first or second regionally and first globally among all the RAs, especially for correlation and RMSE. The MRC ranking with respect to correlations is superior to RMSE and then absolute bias. The MRC ranking for CM AOD is slightly superior to that of the total AOD and then FM AOD. The MRC method gains an advantage due to its ability to average independent models.
The findings presented in this study offer a comprehensive overview of the current state-of-the-art aerosol RAs in the context of monthly AOD. The strengths and weaknesses of individual RAs and their collective implications will provide valuable information for diverse potential users. Compared with intercomparisons of satellite AOD products, which have shown a typical bias of 15 %–25 % (which regionally can reach ±50 %) and AOD divergence of 10 % over ocean to 100 % over certain land areas among 14 satellite products in Schutgens et al. (2020), as well as the intercomparisons of different MODIS products shown in Fig. 1, the biases and divergence of AODs from the four RAs are moderate. The MRC product, which is currently a simple ensemble mean of the four RAs, could be potentially improved with regionally weighted member contributions according to the strengths of the RAs or with aerosol scenario/species-weighted member contributions.
The results of the intercomparison highlight areas for improvement in the next generation of aerosol RAs. These improvements may include tuning of emission sources and sinks, finer spatiotemporal resolutions, incorporation of additional aerosol species, such as nitrate aerosols and dust with different mineralogy, separation of BC and OC from BB emissions in some RAs, as well as application and enhancement of BB plume rise models. Moreover, some centers are planning to incorporate new observational data such as the OMI aerosol index to constrain the amount of absorptive aerosols, an index which has the potential to enhance simulations of BB smoke and dust aerosols (Zhang et al., 2021; Sorenson et al., 2023). Vertical profiles of aerosol backscatter measured by CALIOP and future space-borne lidars may also be incorporated into RAs to help constrain aerosol vertical distribution. Anticipated advancements in emission inventories, retrieval algorithms, space-borne sensors, upcoming satellite missions, and improvements in meteorological as well as aerosol modeling are expected to drive progress in aerosol RA.
ABF | Anthropogenic and biogenic fine aerosols |
AERONET | Aerosol Robotic Network |
AOD | Aerosol optical depth |
AVHRR | Advanced Very High Resolution Radiometer |
BB | Biomass burning |
BC | Black carbon |
CALIOP | Cloud-Aerosol Lidar with Orthogonal Polarization |
CAMSRA | Copernicus Atmosphere Monitoring System |
Reanalysis | |
CM | Coarse mode |
FLAMBE | Fire Locating and Modeling of Burning Emissions |
FM | Fine mode |
ICAP | International Cooperative for Aerosol Predictions |
JRAero | Japanese Reanalysis for Aerosol |
MASINGAR | Model of Aerosol Species IN the Global |
AtmospheRe | |
MERRA-2 | Modern-Era Retrospective Analysis for Research |
and Applications version 2 | |
MISR | Multi-angle Imaging SpectroRadiometer |
MME | Multi-model ensemble |
MODIS | Moderate Resolution Imaging Spectroradiometer |
MODIS-DA | MODIS data-assimilation-quality data |
MODIS-DB | MODIS Deep Blue |
MODIS-DT | MODIS Dark Target |
MRC | Multi-reanalysis consensus |
NAAPS-RA v1 | Naval Aerosol Analysis and Prediction |
System Reanalysis version 1 | |
OC | Organic carbon |
OM | Organic matter |
OMI | Ozone Monitoring Instrument |
PMAp | Polar Multi-Sensor Aerosol product |
QFED | Quick Fire Emissions Dataset |
RA | Reanalysis |
RMSE | Root mean square error |
SDA | Spectral deconvolution method |
Root mean square error (RMSE):
where τ represents monthly AOD and n is the total number (i.e., months) of observational or model data.
Bias:
Mean error:
Mean absolute error:
Coefficient of determination:
where and are the mean values of variables x and y.
Multi-reanalysis consensus (MRC):
where m is the total number of the individual reanalyses, which is 4 for this study.
Spread among the RAs is defined as the standard deviation of all the individual models, i.e.,
where xi is individual reanalysis and is the MRC.
All the data supporting the findings of this paper can be accessed via the provided links or by requesting them using the contact information provided within those links.
AERONET version 3 level-2 data: http://aeronet.gsfc.nasa.gov (AERONET, 2024)
MODIS data-assimilation-quality AOD:
https://doi.org/10.5067/MODIS/MCDAODHD.NRT.061 (MODAPS, 2017)
CAMSRA AOD: https://ads.atmosphere.copernicus.eu/cdsapp#!/dataset/cams-global-reanalysis-eac4-monthly?tab=overview (Inness et al., 2019)
JRAero product: https://www.riam.kyushu-u.ac.jp/taikai/JRAero (Yumimoto et al., 2017)
MERRA-2 AOD: https://doi.org/10.5067/FH9A0MLJPC7N (GMAO, 2015)
NAAPS-RA AOD: https://usgodae.org//cgi-bin/datalist.pl?dset=nrl_naaps_reanalysis&summary=Go (Lynch et al., 2016)
MRC AOD: https://nrlgodae1.nrlmry.navy.mil/cgi-bin/datalist.pl?dset=nrl_mre4_post&summary=Go (GODAE, 2024).
The supplement related to this article is available online at: https://doi.org/10.5194/acp-24-6385-2024-supplement.
PX and JSR designed the study. PX performed the data analysis and wrote the paper with contributions from MA, PRC, KY, TFE, EJH, and JZ to data descriptions and information collection. All authors contributed to the discussion of the results and revising the paper.
The contact author has declared that none of the authors has any competing interests.
Publisher’s note: Copernicus Publications remains neutral with regard to jurisdictional claims made in the text, published maps, institutional affiliations, or any other geographical representation in this paper. While Copernicus Publications makes every effort to include appropriate place names, the final responsibility lies with the authors.
This article is part of the special issue “The SPARC Reanalysis Intercomparison Project (S-RIP) Phase 2 (ACP/WCD inter-journal SI)”. It is not associated with a conference.
We thank the NASA AERONET and MODIS teams for the AOD data used in the study. We extend our gratitude to NASA GMAO, ECMWF, JMA, US ONR, and NRL for providing access to the aerosol reanalysis products. We appreciate Stefan Kinne and the anonymous reviewer for their constructive comments that improved the paper.
This research has been supported by the Office of Naval Research Code 322 through the atmospheric reanalysis project. Partial support came from NASA's Interdisciplinary Science (IDS) program (grant no. 80NSSC20K1260).
This paper was edited by Stelios Kazadzis and reviewed by Stefan Kinne and one anonymous referee.
Atwood, S. A., Reid, J. S., Kreidenweis, S. M., Blake, D. R., Jonsson, H. H., Lagrosas, N. D., Xian, P., Reid, E. A., Sessions, W. R., and Simpas, J. B.: Size-resolved aerosol and cloud condensation nuclei (CCN) properties in the remote marine South China Sea – Part 1: Observations and source classification, Atmos. Chem. Phys., 17, 1105–1123, https://doi.org/10.5194/acp-17-1105-2017, 2017.
Buchard, V., da Silva, A. M., Colarco, P. R., Darmenov, A., Randles, C. A., Govindaraju, R., Torres, O., Campbell, J., and Spurr, R.: Using the OMI aerosol index and absorption aerosol optical depth to evaluate the NASA MERRA Aerosol Reanalysis, Atmos. Chem. Phys., 15, 5743–5760, https://doi.org/10.5194/acp-15-5743-2015, 2015.
Buchard, V., Randles, C. A., da Silva, A. M., Darmenov, A., Colarco, P. R., Govindaraju, R., Ferrare, R., Hair, J., Beyersdorf, A. J., Ziemba, L. D., and Yu, H.: The MERRA-2 Aerosol Reanalysis, 1980 Onward. Part II: Evaluation and Case Studies, J. Climate, 30, 6851–6872, https://doi.org/10.1175/jcli-d-16-0613.1, 2017.
Chin, M., Ginoux, P., Kinne, S., Torres, O., Holben, B. N., Duncan, B. N., Martin, R. V., Logan, J. A., Higurashi, A., and Nakajima, T.: Tropospheric aerosol optical thickness from the GOCART model and comparisons with satellite and sun photometer measurements, J. Atmos. Sci., 59, 461–483, https://doi.org/10.1175/1520-0469(2002)059<0461:TAOTFT>2.0.CO;2, 2002.
Colarco, P., da Silva, A., Chin, M., and Diehl, T.: Online simulations of global aerosol istributions in the NASA GEOS-4 model and comparisons to satellite and groundbased aerosol optical depth, J. Geophys. Res., 115, D14207, https://doi.org/10.1029/2009jd012820, 2010.
Cui, C., Liu, Y., Chen, L., Liang, S., Shan, M., Zhao, J., Liu, Y., Yu, S., Sun, Y., Mao, J., Zhang, H., Gao, S., and Zhenxing Ma, Z.: Assessing public health and economic loss associated with black carbon exposure using monitoring and MERRA-2 data, Environ. Pollut., 313, 120190, https://doi.org/10.1016/j.envpol.2022.120190, 2022.
Dee, D. P. and da Silva, A. M.: Maximum-likelihood estimation of forecast and observation error covariance parameters. Part I: Methodology, Mon. Weather Rev., 127, 1811–1834, https://doi.org/10.1175/1520-0493(1999)127<1822:MLEOFA>2.0.CO;2, 1999.
Dee, D., Rukhovets, L., Todling, R., da Silva, A. M., and Lawson, J. W.: An adaptive buddy check for observational quality control, Q. J. Roy. Meteor. Soc., 127, 2451–2471, https://doi.org/10.1002/qj.49712757714, 2001.
Diehl, T., Heil, A., Chin, M., Pan, X., Streets, D., Schultz, M., and Kinne, S.: Anthropogenic, biomass burning, and volcanic emissions of black carbon, organic carbon, and SO2 from 1980 to 2010 for hindcast model experiments, Atmos. Chem. Phys. Discuss., 12, 24895–24954, https://doi.org/10.5194/acpd-12-24895-2012, 2012.
Eck, T. F., Holben, B. N., Reid, J. S., Dubovik, O., Smirnov, A., O'Neill, N. T., Slutsker, I., and Kinne, S.: Wavelength dependence of the optical depth of biomass burning, urban, and desert dust aerosols, J. Geophys. Res., 104, 31333–31349, 1999.
Eck, T. F., Holben, B. N., Reid, J. S., Xian, P., Giles, D. M., Sinyuk, A., Smirnov, A., Schafer, J. S., Slutsker, I., Kim, J., Koo, J.-H., Choi, M., Kim, K. C., Sano, I., Arola, A., Sayer, A. M., Levy, R. C., Munchak, L. A., O’Neill, N. T., Lyapustin, A., Hsu, N. C., Randles, C. A., Da Silva, A. M., Buchard, V., Govindaraju, R. C., Hyer, E., Crawford, J. H., Wang, P., and Xia, X.: Observations of the interaction and transport of fine mode aerosols with cloud and/or fog in Northeast Asia from Aerosol Robotic Network and satellite remote sensing, J. Geophys. Res.-Atmos., 123, 5560–5587, https://doi.org/10.1029/2018JD028313, 2018.
Edwards, E.-L., Reid, J. S., Xian, P., Burton, S. P., Cook, A. L., Crosbie, E. C., Fenn, M. A., Ferrare, R. A., Freeman, S. W., Hair, J. W., Harper, D. B., Hostetler, C. A., Robinson, C. E., Scarino, A. J., Shook, M. A., Sokolowsky, G. A., van den Heever, S. C., Winstead, E. L., Woods, S., Ziemba, L. D., and Sorooshian, A.: Assessment of NAAPS-RA performance in Maritime Southeast Asia during CAMP2Ex, Atmos. Chem. Phys., 22, 12961–12983, https://doi.org/10.5194/acp-22-12961-2022, 2022.
Flemming, J., Benedetti, A., Inness, A., Engelen, R. J., Jones, L., Huijnen, V., Remy, S., Parrington, M., Suttie, M., Bozzo, A., Peuch, V.-H., Akritidis, D., and Katragkou, E.: The CAMS interim Reanalysis of Carbon Monoxide, Ozone and Aerosol for 2003–2015, Atmos. Chem. Phys., 17, 1945–1983, https://doi.org/10.5194/acp-17-1945-2017, 2017.
Gelaro, R., McCarty, W., Suarez, M. J., Todling, R., Molod, A., Takacs, L., Randles, C. A., Darmenov, A., Bosilovich, M. G., Reichle, R., Wargan, K., Coy, L., Cullather, R., Draper, C., Akella, S., Buchard, V., Conaty, A., Silva, A. M. da, Gu, W., Kim, G.-K., Koster, R., Lucchesi, R., Merkova, D., Nielsen, J. E., Partyka, G., Pawson, S., Putman, W., Rienecker, M., Schubert, S. D., Sienkiewicz, M., and Zhao, B.: The Modern-Era Retrospective Analysis for Research and Applications, Version 2 (MERRA-2), J. Climate, 30, 5419–5454, https://doi.org/10.1175/jcli-d-16-0758.1, 2017.
Giles, D. M., Sinyuk, A., Sorokin, M. G., Schafer, J. S., Smirnov, A., Slutsker, I., Eck, T. F., Holben, B. N., Lewis, J. R., Campbell, J. R., Welton, E. J., Korkin, S. V., and Lyapustin, A. I.: Advancements in the Aerosol Robotic Network (AERONET) Version 3 database – automated near-real-time quality control algorithm with improved cloud screening for Sun photometer aerosol optical depth (AOD) measurements, Atmos. Meas. Tech., 12, 169–209, https://doi.org/10.5194/amt-12-169-2019, 2019.
Ginoux, P., Chin, M., Tegen, I., Prospero, J. M., Holben, B., Dubovik, O., and Lin, S.-J.: Sources and distributions of dust aerosols simulated with the GOCART model, J. Geophys. Res., 106, 20255–20273, 2001.
Gliß, J., Mortier, A., Schulz, M., Andrews, E., Balkanski, Y., Bauer, S. E., Benedictow, A. M. K., Bian, H., Checa-Garcia, R., Chin, M., Ginoux, P., Griesfeller, J. J., Heckel, A., Kipling, Z., Kirkevåg, A., Kokkola, H., Laj, P., Le Sager, P., Lund, M. T., Lund Myhre, C., Matsui, H., Myhre, G., Neubauer, D., van Noije, T., North, P., Olivié, D. J. L., Rémy, S., Sogacheva, L., Takemura, T., Tsigaridis, K., and Tsyro, S. G.: AeroCom phase III multi-model evaluation of the aerosol life cycle and optical properties using ground- and space-based remote sensing as well as surface in situ observations, Atmos. Chem. Phys., 21, 87–128, https://doi.org/10.5194/acp-21-87-2021, 2021.
Global Modeling and Assimilation Office (GMAO): MERRA-2 tavgM_2d_aer_Nx: 2d, Monthly mean, Time-averaged, Single-Level, Assimilation, Aerosol Diagnostics V5.12.4, Greenbelt, MD, USA, Goddard Earth Sciences Data and Information Services Center (GES DISC) [data set], https://doi.org/10.5067/FH9A0MLJPC7N, 2015.
Global Ocean Data Assimiliation Experiment (GODAE): MRC AOD, https://nrlgodae1.nrlmry.navy.mil/cgi-bin/datalist.pl?dset=nrl_mre4_post&summary=Go, last access: 28 May 2024.
Gong, S.: A parameterization of sea-salt aerosol source function for sub- and super-micron particles, Global Biogeochem. Cy., 17, 1097, https://doi.org/10.1029/2003gb002079, 2003.
Granier, C., Bessagnet, B., Bond, T., D'Angiola, A., van der Gon, H. D., Frost, G. J., Heil, A., Kaiser, J. W., Kinne, S., Klimont, Z., Kloster, S., Lamarque, J.-F., Liousse, C., Masui, T., Meleux, F., Mieville, A., Ohara, T., Raut, J.-C., Riahi, K., Schultz, M. G., Smith, S. J., Thompson, A., van Aardenne, J., van der Werf, G. R., and van Vuuren, D. P.: Evolution of anthropogenic and biomass burning emissions of air pollutants at global and regional scales during the 1980–2010 period, Climate Change, 109, 163–190, 2011.
Gumber, A., Reid, J. S., Holz, R. E., Eck, T. F., Hsu, N. C., Levy, R. C., Zhang, J., and Veglio, P.: Assessment of severe aerosol events from NASA MODIS and VIIRS aerosol products for data assimilation and climate continuity, Atmos. Meas. Tech., 16, 2547–2573, https://doi.org/10.5194/amt-16-2547-2023, 2023.
Hogan, T. F. and Rosmond, T. E.: The description of the Navy Operational Global Atmospheric Prediction System's spectral forecast model, Mon. Weather Rev., 119, 1786–1815, 1991.
Hogan, T. F., Liu, M., Ridout, J. A., Peng, M. S., Whitcomb, T. R., Ruston, B. C., Reynolds, C. A., Eckermann, S. D., Moskaitis, J. R., Baker, N. L., McCormack, J. P., Viner, K. C., McLay, J. G., Flatau, M. K., Xu, L., Chen, C., and Chang, S. W.: The Navy Global Environmental Model. Oceanography, 27, 116–125, 2014
Holben, B. N., Eck, T. F., Slutsker, I., Tanre, D., Buis, J. P., Setzer, A., Vermote, E., Reagan, J. A., Kaufman, Y. J., Nakajima, T., Lavenu, F., Jankowiak, I., and Smirnov, A.: AERONET – A federated instrument network and data archive for aerosol characterization, Remote Sens. Environ., 66, 1–16, 1998.
Hsu, N. C., Jeong, M.-J., Bettenhausen, C., Sayer, A. M., Hansell, R., Seftor, C. S., Huang, J., and Tsay, S.-C.: Enhanced Deep Blue aerosol retrieval algorithm: The second generation, J. Geophys. Res.-Atmos., 118, 9296–9315, https://doi.org/10.1002/jgrd.50712, 2013.
Hyer, E. J., Reid, J. S., and Zhang, J.: An over-land aerosol optical depth data set for data assimilation by filtering, correction, and aggregation of MODIS Collection 5 optical depth retrievals, Atmos. Meas. Tech., 4, 379–408, https://doi.org/10.5194/amt-4-379-2011, 2011.
Ignatov, A. and Stowe, L.: Aerosol Retrievals from Individual AVHRR Channels. Part I: Retrieval Algorithm and Transition from Dave to 6S Radiative Transfer Model, J. Atmos. Sci., 59, 313–334, https://doi.org/10.1175/1520-0469(2002)059<0313:ARFIAC>2.0.CO;2, 2002.
Inness, A., Ades, M., Agustí-Panareda, A., Barré, J., Benedictow, A., Blechschmidt, A.-M., Dominguez, J. J., Engelen, R., Eskes, H., Flemming, J., Huijnen, V., Jones, L., Kipling, Z., Massart, S., Parrington, M., Peuch, V.-H., Razinger, M., Remy, S., Schulz, M., and Suttie, M.: The CAMS reanalysis of atmospheric composition, Atmos. Chem. Phys., 19, 3515–3556, https://doi.org/10.5194/acp-19-3515-2019, 2019 (data available at: https://ads.atmosphere.copernicus.eu/cdsapp#!/dataset/cams-global-reanalysis-eac4-monthly?tab=overview, last access: 28 May 2024).
Jaeglé, L., Quinn, P. K., Bates, T. S., Alexander, B., and Lin, J.-T.: Global distribution of sea salt aerosols: new constraints from in situ and remote sensing observations, Atmos. Chem. Phys., 11, 3137–3157, https://doi.org/10.5194/acp-11-3137-2011, 2011.
Jenwitheesuk, K., Peansukwech, U., and Jenwitheesuk, K.: Predictive MERRA-2 aerosol diagnostic model for oral, oropharyngeal and laryngeal cancer caused by air pollution in Thai population, Toxicology Reports, 9, 970–978, https://doi.org/10.1016/j.toxrep.2022.04.015, 2022.
Kahn, R. A., Gaitley, B. J., Garay, M. J., Diner, D. J., Eck, T. F., Smirnov, A., and Holben, B. N.: Multiangle Imaging SpectroRadiometer global aerosol product assessment by comparison with the Aerosol Robotic Network, J. Geophys. Res., 115, D23209, https://doi.org/10.1029/2010JD014601, 2010.
Kaiser, J. W., Heil, A., Andreae, M. O., Benedetti, A., Chubarova, N., Jones, L., Morcrette, J.-J., Razinger, M., Schultz, M. G., Suttie, M., and van der Werf, G. R.: Biomass burning emissions estimated with a global fire assimilation system based on observed fire radiative power, Biogeosciences, 9, 527–554, https://doi.org/10.5194/bg-9-527-2012, 2012.
Kaku, K. C., Reid, J. S., O'Neill, N. T., Quinn, P. K., Coffman, D. J., and Eck, T. F.: Verification and application of the extended spectral deconvolution algorithm (SDA+) methodology to estimate aerosol fine and coarse mode extinction coefficients in the marine boundary layer, Atmos. Meas. Tech., 7, 3399–3412, https://doi.org/10.5194/amt-7-3399-2014, 2014.
Khan, A. L., McMeeking, G. R., Schwarz, J. P., Xian, P., Welch, K. A., Berry Lyons, W., and McKnight, D. M.: Near-surface refractory black carbon observations in the atmosphere and snow in the McMurdo Dry Valleys, Antarctica, andpotential impacts of foehn winds, J. Geophys. Res.-Atmos., 123, 2877–2887, https://doi.org/10.1002/2017JD027696, 2018.
Khan, A. L., Klein, A. G., Katich, J. M. and Xian, P.: Local Emissions and Regional Wildfires Influence Refractory Black Carbon Observations Near Palmer Station, Antarctica, Front. Earth Sci., 7, 49, https://doi.org/10.3389/feart.2019.00049, 2019.
Khan, A. L., Rittger, K., Xian, P., Katich, J. M., Armstrong, R., Kayastha, R., Dana, J., and McKnight, D. M.: Biofuel burning influences refractory black carbon concentrations in seasonal snow at lower elevations of the Dudh Koshi River Basin of Nepal, Front. Earth Sci., 8, https://doi.org/10.3389/feart.2020.00371, 2020.
Kinne, S., Schulz, M., Textor, C., Guibert, S., Balkanski, Y., Bauer, S. E., Berntsen, T., Berglen, T. F., Boucher, O., Chin, M., Collins, W., Dentener, F., Diehl, T., Easter, R., Feichter, J., Fillmore, D., Ghan, S., Ginoux, P., Gong, S., Grini, A., Hendricks, J., Herzog, M., Horowitz, L., Isaksen, I., Iversen, T., Kirkevåg, A., Kloster, S., Koch, D., Kristjansson, J. E., Krol, M., Lauer, A., Lamarque, J. F., Lesins, G., Liu, X., Lohmann, U., Montanaro, V., Myhre, G., Penner, J., Pitari, G., Reddy, S., Seland, O., Stier, P., Takemura, T., and Tie, X.: An AeroCom initial assessment – optical properties in aerosol component modules of global models, Atmos. Chem. Phys., 6, 1815–1834, https://doi.org/10.5194/acp-6-1815-2006, 2006.
Kramer, S. J., Alvarez, C., Barkley, A. E., Colarco, P. R., Custals, L., Delgadillo, R., Gaston, C. J., Govindaraju, R., and Zuidema, P.: Apparent dust size discrepancy in aerosol reanalysis in north African dust after long-range transport, Atmos. Chem. Phys., 20, 10047–10062, https://doi.org/10.5194/acp-20-10047-2020, 2020.
Lacima, A., Petetin, H., Soret, A., Bowdalo, D., Jorba, O., Chen, Z., Méndez Turrubiates, R. F., Achebak, H., Ballester, J., and Pérez García-Pando, C.: Long-term evaluation of surface air pollution in CAMSRA and MERRA-2 global reanalyses over Europe (2003–2020), Geosci. Model Dev., 16, 2689–2718, https://doi.org/10.5194/gmd-16-2689-2023, 2023.
Levy, R. C., Mattoo, S., Munchak, L. A., Remer, L. A., Sayer, A. M., Patadia, F., and Hsu, N. C.: The Collection 6 MODIS aerosol products over land and ocean, Atmos. Meas. Tech., 6, 2989–3034, https://doi.org/10.5194/amt-6-2989-2013, 2013.
Lynch, P., Reid, J. S., Westphal, D. L., Zhang, J., Hogan, T. F., Hyer, E. J., Curtis, C. A., Hegg, D. A., Shi, Y., Campbell, J. R., Rubin, J. I., Sessions, W. R., Turk, F. J., and Walker, A. L.: An 11-year global gridded aerosol optical thickness reanalysis (v1.0) for atmospheric and climate sciences, Geosci. Model Dev., 9, 1489–1522, https://doi.org/10.5194/gmd-9-1489-2016, 2016 (data available at: https://usgodae.org//cgi-bin/datalist.pl?dset=25nrl_naaps_reanalysis&summary=Go, last access: 28 May 2024).
Markowicz, K. M., Pakszys, P., Ritter, C., Zielinski, T., Udisti, R., Cappelletti, D., Mazzola, M., Shiobara, M., Xian, P., Zawadzka, O., Lisok, J., Petelski, T., Makuch, P., and Karasinski, G.: Impact of North American intense fires on aerosol optical properties measured over the European Arctic in July 2015, J. Geophys. Res.-Atmos., 121, 14487–14512, https://doi.org/10.1002/2016JD025310, 2016.
Markowicz, K. M., Zawadzka-Manko, O., Lisok, J., Chilinski, M., T., and Xian, P.: The impact of moderately absorbing aerosol on surface sensible, latent, and net radiative fluxes during the summer of 2015 in Central Europe, J. Aerosol Sci., 151, 105627, https://doi.org/10.1016/j.jaerosci.2020.105627, 2021a.
Markowicz, K. M., Lisok, J., and Xian, P.: Simulation of long-term direct aerosol radiative forcing over the arctic within the framework of the iAREA project, Atmos. Environ., 244, 117882, https://doi.org/10.1016/j.atmosenv.2020.117882, 2021b.
McCoy, D. T.,Bender, F. A.-M., Mohrmann, J. K. C., Hartmann, D. L., Wood, R., and Grosvenor, D. P.: The global aerosol-cloud first indirect effect estimated using MODIS, MERRA, and AeroCom, J. Geophys. Res.-Atmos., 122, 1779–1796, https://doi.org/10.1002/2016JD026141, 2017.
Mescioglu, E., Rahav, E., Belkin, N., Xian, P., Eizenga, J. M., Vichik, A., Herut, B., and Paytan, A.: Aerosol Microbiome over the Mediterranean Sea Diversity and Abundance, Atmosphere, 10, 440, https://doi.org/10.3390/atmos10080440, 2019.
Monahan, E. C., Spiel, D. E., and Davidson, K. L.: A Model of Marine Aerosol Generation Via Whitecaps and Wave Disruption, edited by: Monahan, E. C. Niocaill, G. M., Oceanic Whitecaps, Oceanographic Sciences Library, vol 2., Springer, Dordrecht, https://doi.org/10.1007/978-94-009-4668-2_16, 1986.
Ningombam, S. S., Dumka, U. C., Mugil, S. K., Kuniyal, J. C., Hooda. R. K., Gautam, A. S., and Tiwari, S.: Impacts of Aerosol Loading in the Hindu Kush Himalayan Region Based on MERRA-2 Reanalysis Data, Atmosphere, 12, 2073–4433, 2021.
Ohno, T., Irie, H., Momoi, M., and da Silva, A. M.: Quantitative evaluation of mixed biomass burning and anthropogenic aerosols over the Indochina Peninsula using MERRA-2 reanalysis products validated by sky radiometer and MAX-DOAS observations, Prog. Earth Planet. Sci., 9, 61, https://doi.org/10.1186/s40645-022-00520-4, 2022.
O'Neill, N.T., Eck, T. F., Holben, B. N., Smirnov, A., Dubovik, O., and Royer, A.: Bimodal size distribution influences on the variation of Angstrom derivatives in spectral and optical depth space, J. Geophys. Res., 106, 9787–9806, 2001.
O'Neill, N. T., Eck, T. F., Smirnov, A., Holben, B. N., and Thulasiraman, S.: Spectral discrimination of coarse and fine mode optical depth, J. Geophys. Res., 108, D05212, https://doi.org/10.1029/2002JD002975, 2003.
O'Neill, N. T., Ranjbar, K., Ivănescu, L., Eck, T. F., Reid, J. S., Giles, D. M., Pérez-Ramírez, D., and Chaubey, J. P.: Relationship between the sub-micron fraction (SMF) and fine-mode fraction (FMF) in the context of AERONET retrievals, Atmos. Meas. Tech., 16, 1103–1120, https://doi.org/10.5194/amt-16-1103-2023, 2023.
O'Sullivan, D., Marenco, F., Ryder, C. L., Pradhan, Y., Kipling, Z., Johnson, B., Benedetti, A., Brooks, M., McGill, M., Yorks, J., and Selmer, P.: Models transport Saharan dust too low in the atmosphere: a comparison of the MetUM and CAMS forecasts with observations, Atmos. Chem. Phys., 20, 12955–12982, https://doi.org/10.5194/acp-20-12955-2020, 2020.
Popp, T., deLeeuw, G., Bingen, C., Brühl, C., Capelle, V., Chedin, A., Clarisse, L., Dubovik, O., Grainger, R., Griesfeller, J., Heckel, A., Kinne, S., Klüser, L., Kosmale, M., Kolmonen, P., Lelli,L., Litvinov, P., Mei, L., North, P., Pinnock,S., Povey, A., Robert, C., Schulz, M., Sogacheva, L., Stebel, K., Zweers, D. S., Thomas, G., Gijsbert Tilstra, L., Vandenbussche, S., Veefkind, P., Vountas, M., and Xue, Y.: Development, production and evaluation of aerosol climate data records from european satellite observations (Aerosol_cci), Remote Sens., 8, 421, https://doi.org/10.3390/rs8050421, 2016.
Randles, C. A., daSilva, A. M., Buchard, V., Colarco, P. R., Darmenov, A., Govindaraju, R., Smirnov, A., Holben, B., Ferrare, R., Hair, J., Shinozuka, Y., and Flynn, C. J.: The MERRA-2 aerosol reanalysis, 1980 onward. Part I: System description and data assimilation evaluation, J. Climate, 30, 6823–6850, https://doi.org/10.1175/JCLI-D-16-0609.1, 2017.
Reid, J. S., Gumber, A., Zhang, J., Holz, R. E., Rubin, J. I., Xian, P., Smirnov, A., Eck, T. F., O'Neill, N. T., Levy, R. C., Reid, E. A., Colarco, P. R., Benedetti, A., and Tanaka, T.: A Coupled Evaluation of Operational MODIS and Model Aerosol Products for Maritime Environments Using Sun Photometry: Evaluation of the Fine and Coarse Mode, Remote Sens.-, 14, 2978, https://doi.org/10.3390/rs14132978, 2022.
Reid, J. S., Maring, H. B., Narisma, G. T., Heever, S. van den, Girolamo, L. D., Ferrare, R., Lawson, P., Mace, G. G., Simpas, J. B., Tanelli, S., Ziemba, L., Diedenhoven, B. van, Bruintjes, R., Bucholtz, A., Cairns, B., Cambaliza, M. O., Chen, G., Diskin, G. S., Flynn, J. H., Hostetler, C. A., Holz, R. E., Lang, T. J., Schmidt, K. S., Smith, G., Sorooshian, A., Thompson, E. J., Thornhill, K. L., Trepte, C., Wang, J., Woods, S., Yoon, S., Alexandrov, M., Alvarez, S., Amiot, C. G., Bennett, J. R., M., B., Burton, S. P., Cayanan, E., Chen, H., Collow, A., Crosbie, E., DaSilva, A., DiGangi, J. P., Flagg, D. D., Freeman, S. W., Fu, D., Fukada, E., Hilario, M. R. A., Hong, Y., Hristova-Veleva, S. M., Kuehn, R., Kowch, R. S., Leung, G. R., Loveridge, J., Meyer, K., Miller, R. M., Montes, M. J., Moum, J. N., Nenes, T., Nesbitt, S. W., Norgren, M., Nowottnick, E. P., Rauber, R. M., Reid, E. A., Rutledge, S., Schlosser, J. S., Sekiyama, T. T., Shook, M. A., Sokolowsky, G. A., Stamnes, S. A., Tanaka, T. Y., Wasilewski, A., Xian, P., Xiao, Q., Xu, Z., and Zavaleta, J.: The coupling between tropical meteorology, aerosol lifecycle, convection, and radiation, during the Cloud, Aerosol and Monsoon Processes Philippines Experiment (CAMP2Ex), B. Am. Meteor. Soc., 1, https://doi.org/10.1175/BAMS-D-21-0285.1, 2023.
Reid, J. S., Hyer, E. J., Prins, E. M., Westphal, D. L., Zhang, J., Wang, J., Christopher, S. A., Curtis, C. A., Schmidt, C. C., Eleuterio, D. P., Richardson, K. A., and Hoffman, J. P.: Global Monitoring and Forecasting of Biomass-Burning Smoke: Description of and Lessons from the Fire Locating and Modeling of Burning Emissions (FLAMBE) Program, IEEE J. Sel. Top. Appl., 2, 144–162, 2009.
Reid, J. S., Xian, P., Hyer, E. J., Flatau, M. K., Ramirez, E. M., Turk, F. J., Sampson, C. R., Zhang, C., Fukada, E. M., and Maloney, E. D.: Multi-scale meteorological conceptual analysis of observed active fire hotspot activity and smoke optical depth in the Maritime Continent, Atmos. Chem. Phys., 12, 2117–2147, https://doi.org/10.5194/acp-12-2117-2012, 2012.
Reid, J. S., Xian, P., Holben, B. N., Hyer, E. J., Reid, E. A., Salinas, S. V., Zhang, J., Campbell, J. R., Chew, B. N., Holz, R. E., Kuciauskas, A. P., Lagrosas, N., Posselt, D. J., Sampson, C. R., Walker, A. L., Welton, E. J., and Zhang, C.: Aerosol meteorology of the Maritime Continent for the 2012 7SEAS southwest monsoon intensive study – Part 1: regional-scale phenomena, Atmos. Chem. Phys., 16, 14041–14056, https://doi.org/10.5194/acp-16-14041-2016, 2016.
Ross, A. D., Holz, R. E., Quinn, G., Reid, J. S., Xian, P., Turk, F. J., and Posselt, D. J.: Exploring the first aerosol indirect effect over Southeast Asia using a 10-year collocated MODIS, CALIOP, and model dataset, Atmos. Chem. Phys., 18, 12747–12764, https://doi.org/10.5194/acp-18-12747-2018, 2018.
Roychoudhury, C., He, C., Kumar, R., McKinnon, J. M., and Arellano Jr., A. F.: On the relevance of aerosols to snow cover variability over High Mountain Asia, Geophys. Res. Lett., 49, e2022GL099317, https://doi.org/10.1029/2022GL099317, 2022.
Rubin, J. I., Reid, J. S., Xian, P., Selman, C. M., and Eck, T. F.: A global evaluation of daily to seasonal aerosol and water vapor relationships using a combination of AERONET and NAAPS reanalysis data, Atmos. Chem. Phys., 23, 4059–4090, https://doi.org/10.5194/acp-23-4059-2023, 2023.
Sayer, A. M., Munchak, L. A., Hsu, N. C., Levy, R. C., Bettenhausen, C., and Jeong, M. J.: MODIS Collection 6 aerosol products: comparison between Aqua's e-Deep Blue, Dark Target, and “merged” data sets, and usage recommendations, J. Geophys. Res.-Atmos., 119, 13–965, 2014.
Schutgens, N., Sayer, A. M., Heckel, A., Hsu, C., Jethva, H., de Leeuw, G., Leonard, P. J. T., Levy, R. C., Lipponen, A., Lyapustin, A., North, P., Popp, T., Poulsen, C., Sawyer, V., Sogacheva, L., Thomas, G., Torres, O., Wang, Y., Kinne, S., Schulz, M., and Stier, P.: An AeroCom–AeroSat study: intercomparison of satellite AOD datasets for aerosol model evaluation, Atmos. Chem. Phys., 20, 12431–12457, https://doi.org/10.5194/acp-20-12431-2020, 2020.
Sessions, W. R., Reid, J. S., Benedetti, A., Colarco, P. R., da Silva, A., Lu, S., Sekiyama, T., Tanaka, T. Y., Baldasano, J. M., Basart, S., Brooks, M. E., Eck, T. F., Iredell, M., Hansen, J. A., Jorba, O. C., Juang, H.-M. H., Lynch, P., Morcrette, J.-J., Moorthi, S., Mulcahy, J., Pradhan, Y., Razinger, M., Sampson, C. B., Wang, J., and Westphal, D. L.: Development towards a global operational aerosol consensus: basic climatological characteristics of the International Cooperative for Aerosol Prediction Multi-Model Ensemble (ICAP-MME), Atmos. Chem. Phys., 15, 335–362, https://doi.org/10.5194/acp-15-335-2015, 2015.
Shi, Y., Zhang, J., Reid, J. S., Hyer, E. J., and Hsu, N. C.: Critical evaluation of the MODIS Deep Blue aerosol optical depth product for data assimilation over North Africa, Atmos. Meas. Tech., 6, 949–969, https://doi.org/10.5194/amt-6-949-2013, 2013.
Shi, Y., Zhang, J., Reid, J. S., Holben, B., Hyer, E. J., and Curtis, C.: An analysis of the collection 5 MODIS over-ocean aerosol optical depth product for its implication in aerosol assimilation, Atmos. Chem. Phys., 11, 557–565, https://doi.org/10.5194/acp-11-557-2011, 2011.
Sorenson, B. T., Zhang, J., Reid, J. S., Xian, P., and Jaker, S. L.: Ozone Monitoring Instrument (OMI) UV aerosol index data analysis over the Arctic region for future data assimilation and climate forcing applications, Atmos. Chem. Phys., 23, 7161–7175, https://doi.org/10.5194/acp-23-7161-2023, 2023.
The Naval Research Laboratory and the University of North Dakota/MODIS Adaptive Processing System (MODAPS): Terra Product Descriptions: MCDAODHD, NASA [data set], https://doi.org/10.5067/MODIS/MCDAODHD.NRT.061, 2017.
Textor, C., Schulz, M., Guibert, S., Kinne, S., Balkanski, Y., Bauer, S., Berntsen, T., Berglen, T., Boucher, O., Chin, M., Dentener, F., Diehl, T., Easter, R., Feichter, H., Fillmore, D., Ghan, S., Ginoux, P., Gong, S., Grini, A., Hendricks, J., Horowitz, L., Huang, P., Isaksen, I., Iversen, I., Kloster, S., Koch, D., Kirkevåg, A., Kristjansson, J. E., Krol, M., Lauer, A., Lamarque, J. F., Liu, X., Montanaro, V., Myhre, G., Penner, J., Pitari, G., Reddy, S., Seland, Ø., Stier, P., Takemura, T., and Tie, X.: Analysis and quantification of the diversities of aerosol life cycles within AeroCom, Atmos. Chem. Phys., 6, 1777–1813, https://doi.org/10.5194/acp-6-1777-2006, 2006.
Tong, D. Q., Gill, T. E., Sprigg, W. A., Van Pelt, R. S., Baklanov, A. A., Barker, B. M., Bell, J. E., Castillo, J., Gassó, S., Gaston, C. J., Griffin, D. W., Huneeus, N., Kahn, R. A., Kuciauskas, A. P., Ladino, L. A., Li, J., Mayol-Bracero, O. L., McCotter, O. Z., Méndez-Lázaro, P. A., Mudu, P., Nickovic, S., Oyarzun, D., Prospero, J., Raga, G. B., Raysoni, A. U., Ren, L., Sarafoglou, N., Sealy, A., Sun, Z., and Vimic, A. V.: Health and Safety Effects of Airborne Soil Dust in the Americas and Beyond, Rev. Geophys., 61, e2021RG000763, https://doi.org/10.1029/2021RG000763, 2023.
Wei, J., Peng, Y., Guo, J., and Sun, L.: Performance of MODIS Collection 6.1 Level 3 aerosol products in spatial-temporal variations over land. Atmos. Environ., 206, 30–44, https://doi.org/10.1016/j.atmosenv.2019.03.001, 2019.
Xian, P., Klotzbach, P. J., Dunion, J. P., Janiga, M. A., Reid, J. S., Colarco, P. R., and Kipling, Z.: Revisiting the relationship between Atlantic dust and tropical cyclone activity using aerosol optical depth reanalyses: 2003–2018, Atmos. Chem. Phys., 20, 15357–15378, https://doi.org/10.5194/acp-20-15357-2020, 2020.
Xian, P., Reid, J. S., Hyer, E. J., Sampson, C. R., Rubin, J. I., Ades, M., Asencio, N., Basart, S., Benedetti, A., Bhattacharjee, P. S., Brooks, M. E., Colarco, P. R., da Silva, A. M., Eck, T. F., Guth, J., Jorba, O., Kouznetsov, R., Kipling, Z., Sofiev, M., Perez Garcia-Pando, C., Pradhan, Y., Tanaka, T.,Wang, J., Westphal, D. L., Yumimoto, K., and Zhang, J.: Current state of the global operational aerosol multi-model ensemble: an update from the International Cooperative for Aerosol Prediction (ICAP), Q. J. Roy. Meteoror. Soc., 145, 176–209, https://doi.org/10.1002/qj.3497, 2019.
Xian, P., Reid, J. S., Turk, J. F., Hyer, E. J., and Westphal, D. L.: Impact of models versus satellite measured tropical precipitation on regional smoke optical thickness in an aerosol transport model, Geophys. Res. Lett., 36, L16805, https://doi.org/10.1029/2009GL038823, 2009.
Xian, P. Reid, J. S., Atwood, S. A., Johnson, R. S., Hyer, E. J., Westphal, D. L., and Sessions, W.: Smoke aerosol transport patterns over the Maritime continent, Atmos. Res., 122, 469–485, https://doi.org/10.1016/j.atmosres.2012.05.006, 2013.
Xian, P., Zhang, J., O'Neill, N. T., Toth, T. D., Sorenson, B., Colarco, P. R., Kipling, Z., Hyer, E. J., Campbell, J. R., Reid, J. S., and Ranjbar, K.: Arctic spring and summertime aerosol optical depth baseline from long-term observations and model reanalyses – Part 1: Climatology and trend, Atmos. Chem. Phys., 22, 9915–9947, https://doi.org/10.5194/acp-22-9915-2022, 2022.
Witek, M. L., Flatau, P. J., Quinn, P. K., and Westphal, D. L.: Global sea-salt modeling: Results and validation against multicampaign shipboard measurements, J. Geophys. Res., 112, D08215, https://doi.org/10.1029/2006JD007779, 2007.
Yumimoto, K., Tanaka, T. Y., Oshima, N., and Maki, T.: JRAero: the Japanese Reanalysis for Aerosol v1.0, Geosci. Model Dev., 10, 3225–3253, https://doi.org/10.5194/gmd-10-3225-2017, 2017 (data available at: https://www.riam.kyushu-u.ac.jp/taikai/JRAero, last access: 28 May 2024).
Yukimoto, S., Adachi, Y., Hosaka, M., Sakami, T., Yoshimura, H., Hirabara, M., Tanaka, T. Y., Shindo, E., Tsujino, H., Deushi, M., Mizuta, R., Yabu, S., Obata, A., Nakano, H., Koshiro, T., Ose, T., and Kitoh, A.: A New Global Climate Model of the Meteorological Research Institute: MRI-CGCM3 –Model Description and Basic Performance, J. Meteorol. Soc. Jpn., 90A, 23–64, https://doi.org/10.2151/jmsj.2012-A02, 2012.
Zhang, J., Reid, J. S., and Holben, B. N.: An analysis of potential cloud artifacts in MODIS over ocean aerosol optical thickness products, Geophys. Res. Lett., 32, L15803, https://doi.org/10.1029/2005GL023254, 2005.
Zhang, J. L. and Reid, J. S.: MODIS aerosol product analysis for data assimilation: Assessment of over-ocean level 2 aerosol optical thickness retrievals, J. Geophys. Res.-Atmos., 111, D22207, https://doi.org/10.1029/2005JD006898, 2006.
Zhang, J. L., Reid, J. S., Westphal, D. L., Baker, N. L., and Hyer, E. J.: A system for operational aerosol optical depth data assimilation over global oceans, J. Geophys. Res., 113, D10208, https://doi.org/10.1029/2007JD009065, 2008.
Zhang, J., Reid, J. S., Alfaro-Contreras, R., and Xian P.: Has China been exporting less particulate air pollution over the past decade?, Geophys. Res. Lett., 44, 2941–2948, https://doi.org/10.1002/2017GL072617, 2017.
Zhang, J., Spurr, R. J. D., Reid, J. S., Xian, P., Colarco, P. R., Campbell, J. R., Hyer, E. J., and Baker, N. L.: Development of an Ozone Monitoring Instrument (OMI) aerosol index (AI) data assimilation scheme for aerosol modeling over bright surfaces – a step toward direct radiance assimilation in the UV spectrum, Geosci. Model Dev., 14, 27–42, https://doi.org/10.5194/gmd-14-27-2021, 2021.
Zhang, X. and Zhou, Y.: Aerosol direct radiative forcing over China: A 40-year MERRA-2-based evaluation, Atmos. Environ., 299, 119659, https://doi.org/10.1016/j.atmosenv.2023.119659, 2023.
- Abstract
- Key points
- Introduction
- Data and methods
- Results
- Conclusions
- Appendix A: Abbreviations
- Appendix B: Definitions of terminologies
- Data availability
- Author contributions
- Competing interests
- Disclaimer
- Special issue statement
- Acknowledgements
- Financial support
- Review statement
- References
- Supplement
- Abstract
- Key points
- Introduction
- Data and methods
- Results
- Conclusions
- Appendix A: Abbreviations
- Appendix B: Definitions of terminologies
- Data availability
- Author contributions
- Competing interests
- Disclaimer
- Special issue statement
- Acknowledgements
- Financial support
- Review statement
- References
- Supplement