the Creative Commons Attribution 4.0 License.
the Creative Commons Attribution 4.0 License.
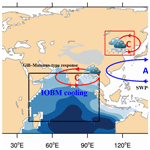
Role of the Indian Ocean basin mode in driving the interdecadal variations of summer precipitation over the East Asian monsoon boundary zone
Jing Wang
Fei Cheng
Chengyu Song
Qiaoping Li
Yihui Ding
Xiangde Xu
Based on long-term observational and reanalysis datasets from 1901 through 2014, this study investigates the characteristics and physical causes of the interdecadal variations in the summer precipitation over the East Asian monsoon boundary zone (EAMBZ), which is a peculiar domain defined from the perspective of the interplay between climatic systems (i.e., mid-latitude westerly and East Asian summer monsoon). Observational evidence reveals that, similarly to previous studies, the EAMBZ precipitation featured prominent interdecadal fluctuations, e.g., with dry summers during the periods preceding 1927, 1939–1945, 1968–1982, and 1998–2010 and wet summers during the periods of 1928–1938, 1946–1967, and 2011 onwards. Further analyses identify that, amongst the major interdecadal oceanic forcings (e.g., Atlantic multidecadal oscillation and Pacific decadal oscillation), the Indian Ocean basin mode (IOBM) is a significant oceanic forcing responsible for the interdecadal variations of the EAMBZ precipitation, playing an independent and critical modulation role. When the cold phase of the IOBM occurs, an anomalous cyclonic circulation is excited around the northeast corner of the tropical Indian Ocean, which further induces a north-low–south-high meridional seesaw pattern over the Northeast China–subtropical western Pacific (SWP) sector. Such seesaw pattern is conducive to the enhanced EAMBZ precipitation by linking favorable environments for the transportation of water vapor from the SWP and the convergence over the EAMBZ at interdecadal timescales. For this reason, a physical–empirical model for the EAMBZ precipitation is developed in terms of the IOBM cooling. Despite the fact that the extreme summer EAMBZ precipitation cannot be captured by this model, it can still well capture its interdecadal fluctuations and reflect their steady relationship. The key physical pathway connecting the IOBM cooling with the interdecadal variations of the summer EAMBZ precipitation is supported by the numerical results based on the large ensemble experiment and the Indian Ocean pacemaker experiment. Our findings may provide new insights into the understanding of the causes of the interdecadal variations in the summer EAMBZ precipitation, which may favor the long-term policy decision-making for the local hydrometeorological planning.
- Article
(11074 KB) - Full-text XML
-
Supplement
(1208 KB) - BibTeX
- EndNote
The monsoonal airflows and mid-latitude westerlies are crucial components of the Asian climate system (Li and Zeng, 2002; Ding and Chan, 2005; Wang et al., 2008; Wu et al., 2012; Huang et al., 2015; Wang et al., 2017; Chen et al., 2018; J. Huang et al., 2019). These two subsystems can synergistically induce regional precipitation fluctuations over subtropical and mid-latitude Asia during the Northern Hemisphere late spring (May) and summer (June–July–August; JJA) (Qian et al., 2009; Chen et al., 2021; Song et al., 2022; J. Wang et al., 2022). For example, Song et al. (2022) found that May precipitation over the southeastern extension of the Tibetan Plateau (TP) features notable year-to-year variations, which are physically linked to a unique interplay between the upstream mid-latitude westerlies and the Bay of Bengal summer monsoon.
During the early stage of the northern summer, however, the mid-latitude westerlies shift poleward to the north of the TP abruptly (Yeh et al., 1959; Schiemann et al., 2009). In this context, westerlies of mid-latitude synoptic disturbance and southerlies of the East Asian summer monsoon (EASM) collide with each other frequently over the East Asian monsoon boundary zone (EAMBZ) (Qian et al., 2009; Wang et al., 2017; Chen et al., 2018; J. Huang et al., 2019; Zeng and Zhang, 2019; Chen et al., 2021; Q. Wang et al., 2021, 2022, 2023). It is essential to point out that although the EAMBZ domain largely overlaps the Northeast Asian area suggested by Si et al. (2021), the EAMBZ is defined from the perspective of the interaction between the mid-latitude westerly and the EASM (see Fig. 1 in Chen et al., 2021; also see the red box in Fig. 1 and associated description in Sect. 2.5.1), not from a geographical notion. Accordingly, the EAMBZ is a transitional climate zone between the EASM-controlled moist region and the westerly-dominated arid region over central Asia (F.-H. Chen et al., 2010; Chen et al., 2018, 2021), stretching from the eastern flank of the TP to Mongolia and Northeast China. Notably, the EAMBZ is a distinguished region with an agrarian economy and animal husbandry, which is largely susceptible to water resource variations (Ou and Qian, 2006; Lu and Jia, 2013). Nevertheless, many studies reported that in the past century, the semi-arid EAMBZ underwent the most profound warming over East Asia, suffering from serious aridification and a high risk of desertification (J. Huang et al., 2017, 2019, 2020). In this regard, the EAMBZ is deemed one of the hotspots highly sensitive to precipitation fluctuations (Qian et al., 2009; Lu and Jia, 2013; J. Huang et al., 2019). Given that the EAMBZ is of an ecologically fragile environment with water shortage, a deep understanding of the reasons for historical changes in summer EAMBZ precipitation could be a prerequisite for in situ ecological improvement and socioeconomic development.
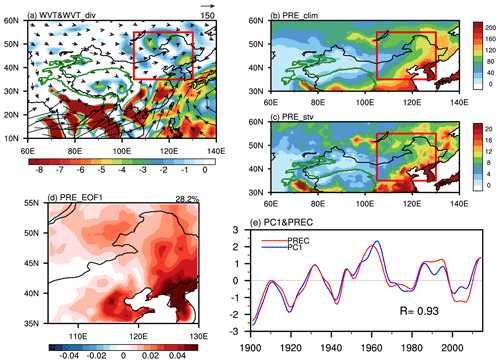
Figure 1The climatological JJA-averaged (a) < WVT > (vectors; kg m−1 s−1) and < WVT_div > (shading; 10−5 kg m−2 s−1), (b) precipitation (millimeters per month), and (c) interdecadal standard deviation of precipitation (millimeters per month) during the period 1901–2014. The red box (35–55° N, 105–130° E) outlines the research domain of the EAMBZ (the same hereinafter). (d) Spatial pattern of the first empirical orthogonal function (EOF1) mode of JJA-mean EAMBZ precipitation. (e) Normalized time series of the JJA-mean EAMBZ precipitation index (IEAMBZP) (red line) and associated first principal component (PC1) (blue line), with the number denoting the temporal correlation coefficient (TCC) between the corresponding time series. In panels (c)–(e), variables are detrended and 11-year low-pass filtered. The green outline in panels (a)–(c) represents the terrain of the Tibetan Plateau (TP) at 2000 m (the same hereinafter). The precipitation is derived from the CRU TS3.26 precipitation data, while other variables are from the 20CRv2c datasets.
Existing studies have well-documented physical mechanisms responsible for the interannual variability of summer EAMBZ precipitation, highlighting the external moisture supply pathways, the modulators for the wet–dry condition variations (e.g., the mid-latitude westerlies within the Asian westerly jet, the western North Pacific subtropical high, and the EASM), and the remote modulation roles of large-scale teleconnected modes (e.g., Silk Road pattern/circumglobal teleconnection propagating along the westerly jet and the Eurasian teleconnection) and sea surface temperature (SST) anomaly patterns (Huang et al., 2015; Wang et al., 2017; Chen et al., 2018, 2021; Zhao et al., 2019a, b, 2020; Q. Wang et al., 2021, 2022, 2023). For instance, Q. Wang et al. (2022) suggested that the positive phase of the Eurasian teleconnection is connected with a low-pressure anomaly in the lower troposphere in the EAMBZ and the Mongolia region, thus favoring enhanced summertime precipitation over the EAMBZ; meanwhile, the circumglobal teleconnection is positively coupled with the EAMBZ precipitation, with ascending motion anomalies over the EAMBZ during its positive phase. Chen et al. (2021) established that the circulations (i.e., the mid-latitude westerlies and EASM) and the forcing of SST anomalies (SSTAs) can collectively regulate the summer EAMBZ precipitation variability. The variability of westerlies is largely modulated by the Silk Road pattern and the meridional displacement of the westerly jet, while the EASM variability is mainly modulated by the prior wintertime El Niño–Southern Oscillation. The synchronized effects of the EASM and westerlies largely contribute to the rainfall variability in the EAMBZ. Note that Chen et al. (2021) also pointed out that the Indian Ocean basin mode (IOBM) is simultaneously correlated with the EASM in boreal summer on the interannual timescale, which may be considered as a salient oceanic modulator for the summer EAMBZ precipitation variability. Nevertheless, they paid little attention to the physical mechanisms of how the IOBM regulates the year-to-year EAMBZ precipitation. Moreover, Zhao et al. (2019a) found that the tropical northern Atlantic SSTAs have significant impacts on the August rainfall over the monsoon transitional zone in China by inducing a wave train over Eurasia and the western North Pacific anomalous anticyclone.
Compared with the extensively explored interannual variability of the JJA EAMBZ precipitation, less effort has been devoted to its interdecadal variability. To understand and predict the summer EAMBZ precipitation, exploring its interdecadal variations and the underlying physical causes is also critical, which is the main focus of the present study. Previous studies suggested that the warm-season precipitation over many Asian areas features interdecadal fluctuations. For example, J. Wang et al. (2022) reported that the late-spring (May) southeastern TP underwent wet conditions for 1928–1961 and 1989–2003 and experienced dry conditions preceding 1927, 1962–1988, and 2004 onwards. Si and Ding (2016) documented that East Asia experienced dry summers from the early 1920s to the 1940s and wet summers from the late 1900s to the early 1920s, in the 1950s, and from the 1980s to the 1990s. Piao et al. (2021) found that the decadal-filtered summer precipitation over Northeast Asia underwent a sudden decease around the late 1990s. The interdecadal oceanic forcings for the interdecadal changes of the Asian summer rainfall are also extensively investigated, highlighting the crucial modulation roles of basin-scale SST modes of Atlantic multidecadal oscillation (Si et al., 2021), Pacific decadal oscillation/interdecadal Pacific oscillation (IPO) (Si and Ding, 2016), and IOBM (Zhang et al., 2018). Among these interdecadal oceanic forcings, it is essential to emphasize the IOBM, a dominant mode of SST variability in the tropical Indian Ocean (TIO) sector, which usually follows up a wintertime El Niño–Southern Oscillation event and persists into the summer through the capacitor effect (Klein et al., 1999; Yang et al., 2007; Xie et al., 2009). It is worth noting that the IOBM also features a basin-scale warming/cooling at interdecadal timescales (Han et al., 2014), exerting active impacts on the mid-latitude Asian climate (e.g., Wu et al., 2016; Li and Ma, 2018; Zhang et al., 2018; S. Wang et al., 2022). As for the interdecadal variations of the summer EAMBZ precipitation, we hope to answer the following two questions. (1) Did the JJA EAMBZ precipitation feature interdecadal variations? If so, (2) is there any intimate connection between the IOBM and the EAMBZ precipitation at interdecadal timescales? As such, this study shall extend previous studies by exploring to what extent and how the JJA IOBM modulates the concurrent EAMBZ precipitation variability at interdecadal timescales, with the aim of providing a novel understanding for the rainfall variability over the mid-latitude semi-arid zone in Asia. Note that we employ datasets with a centennial scale in this study (e.g., the precipitation data produced by the Climatic Research Unit (CRU) and the atmospheric circulation data from the Twentieth Century Reanalysis datasets). In comparison with the short-term datasets since the latter half of the 20th century, these long-term datasets can separate the interdecadal variability of EAMBZ precipitation from the externally forced global climate change caused by anthropogenic (e.g., greenhouse gas) and natural forcings (e.g., volcanic eruption) more effectively (Wu et al., 2016), which were widely used to investigate the physical causes of how internal fluctuations of the climate system modulate the interdecadal variations of precipitation over Asia (e.g., Wu et al., 2016; Zhang et al., 2018; Sun et al., 2019a; Jiang et al., 2021; J. Wang et al., 2022).
The remainder of this paper is arranged as follows. Section 2 describes the datasets and methods used in this study. Section 3 elucidates the characteristics of the interdecadal variations of summertime EAMBZ precipitation and the associated background circulations, illustrates the mechanisms of how the IOBM modulates the EAMBZ precipitation, establishes a linear regression model using the IOBM to predict the interdecadal precipitation anomalies over the EAMBZ, and verifies the IOBM-related physical processes using numerical model simulations. A summary of the major findings and further discussions are provided in Sect. 4.
2.1 Observational data
Several monthly mean observational datasets are utilized in the present study, including (1) the global land high-resolution gridded CRU time series precipitation dataset version 3.26 (CRU TS3.26; spatial resolution: 0.5° × 0.5°; Harris et al., 2014) for 1901–2017, (2) the Extended Reconstructed SST version 5 (ERSSTv5; spatial resolution: 2° × 2°; B. Huang et al., 2017) for 1854–present derived from the National Oceanic and Atmospheric Administration (NOAA), and (3) atmospheric variables derived from NOAA–Cooperative Institute for Research in Environmental Sciences (CIRES) Twentieth Century Reanalysis version 2c (20CRv2c; spatial resolution: 2° × 2°; Compo et al., 2011), except for the precipitation data, with 192 points in longitude and 94 points in latitude, for 1851–2014. Note that all observational datasets cover the common time period of 1901–2014, which is the focused period in the present research.
2.2 Rossby wave source
Following Sardeshmukh and Hoskins (1988), the Rossby wave source (RWS) is calculated as
where Vχ is the divergent wind, ζ is the relative vorticity, and f is the planetary vorticity.
2.3 Moisture flux and associated divergence
The vertically integrated horizontal water vapor transport (< WVT >) and WVT-associated divergence (< WVT_div >) are calculated using the following equations (Sun et al., 2019b; J. Wang et al., 2022):
where ∇p⋅() denotes the horizontal divergence in the pressure coordinates, g is the gravitational acceleration, Ps is the surface pressure, q is the specific humidity, and V= (u, v) is the horizontal wind vector (u and v represent the zonal and meridional winds, respectively).
2.4 Statistical methods
This study focuses on interdecadal fluctuations in variables. The data are 11-year low-pass filtered by adopting a Lanczos filter (Duchon, 1979) to extract the corresponding interdecadal signal. Several statistical methods are used, including empirical orthogonal function (EOF) analysis, composite analysis, correlation analysis, and linear regression analysis. A two-tailed Student t test is used to evaluate the statistical significance. Considering the 11-year low-pass-filtered method can significantly reduce the degrees of freedom of the data, the following approximation is therefore deployed to calculate the effective degrees of freedom (Neff):
where N is the sample size, and ρXX(j) and ρYY(j) are the autocorrelations of two sampled time series X and Y, respectively, at time lag j (Li et al., 2013).
In this study, we focus on the boreal summer season (JJA). All variables in observations and model simulations are linearly detrended before further calculations and analyses to exclude potential impacts of long-term trends.
2.5 Definitions
2.5.1 The research domain of the EAMBZ
From the long-term (1901–2014) perspective of the climatological mean state of converged < WVT > and pronounced precipitation over mid-latitude Asia, the EAMBZ (box in Fig. 1a and b; 35–55° N, 105–130° E) is defined as the collision and convergence zone between JJA dry westerly < WVT > and moist southwesterly < WVT > (Fig. 1a). As such, there exist wetter conditions over the EASM-dominated part and drier conditions over the westerly-controlled part (Fig. 1b), suggesting the semi-arid transitional feature of the EAMBZ (Xing and Wang, 2017). Our defined research domain of the EAMBZ largely matches the monsoon boundary zone defined by Chen et al. (2021), covering Inner Mongolia, Gansu, Ningxia, Shaanxi, Shanxi, Hebei, Beijing, Tianjin, Shandong, Jilin, Liaoning, and Heilongjiang in China, as well as eastern Mongolia and the Korean Peninsula. Note that our focused EAMBZ domain differs from the Northeast Asian domain (29–50° N, 108–140° E) suggested by Si et al. (2021). Although they are extensively overlapped, the EAMBZ is located more westward and northward and defined from the perspective of the interplay between climatic systems, not from a pure geographical perspective. Since the areal mean precipitation over the EAMBZ in boreal summer is the highest of the year accompanying the largest standard deviation (i.e., largest rainfall variability) (Fig. S1 in the Supplement), the summer season is focused in the present study.
2.5.2 Climate indices
The IOBM index (IIOBM) is defined as areal mean SSTAs over the TIO domain of 20° S–20° N, 40–100° E (Xie et al., 2009). The IPO index is calculated using a method identical to that defined in Henley et al. (2015), that is, the difference between SSTAs averaged over the central equatorial Pacific (10° S–10° N, 170° E–90° W) and the average of SSTAs in the northwest (25–45° N, 140° E–145° W) and the southwest Pacific (50–15° S, 150° E–160° W). In observations, considering the coupled nature of the IOBM and IPO at interdecadal timescales in boreal summer (cf. Fig. 2a in Wu et al., 2016), we hence remove the potential influence of the contemporaneous IPO on precipitation by eliminating the forcing of the IPO from the data of climate variables based on the partial regression technique, which is widely used in previous studies (e.g., Dou and Wu, 2018; J. Wang et al., 2022).
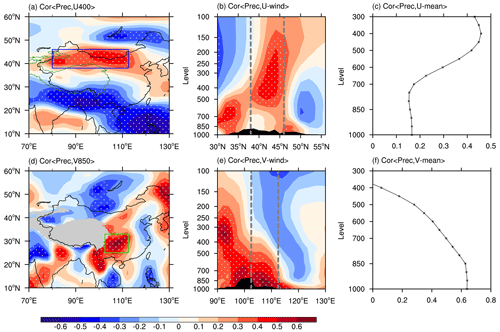
Figure 2Correlation maps of the JJA-averaged IEAMBZP with the simultaneous (a) 400 hPa zonal wind and (d) 850 hPa meridional wind, (b) height–latitude cross-section of zonal winds averaged over 80–112.5° E, and (e) height–longitude cross-section of meridional winds averaged over 25–33° N, during the period 1901–2014. The blue box (38–46° N, 80–112.5° E) in panel (a) and the green box (25–33° N, 102.5–112.5° E) in panel (d) represent the upstream westerly domain and the monsoonal southerly domain significantly tied to the interdecadal variations of precipitation over the EAMBZ, respectively (the same hereinafter). The gray dashed vertical lines in panels (b) and (e) represent the latitudinal and longitudinal range of the westerly and the monsoonal southerly domain, respectively. (c) Profile of correlation coefficients between the JJA-averaged IEAMBZP and the simultaneous area-averaged zonal winds over the upstream westerly domain at multiple levels during the period 1901–2014. (f) As in panel (c) but for the meridional winds over the monsoonal southerly domain. All variables are detrended and 11-year low-pass filtered. Areas with significant values exceeding the 95 % confidence level are stippled. The black shading indicates the topography. The gray shaded areas denote the TP areas above 2000 m (the same hereinafter). The IEAMBZP is calculated based on the CRU TS3.26 precipitation data, while other variables are from the 20CRv2c datasets.
2.6 Model simulations
To validate our proposed mechanisms of how the TIO SSTAs (i.e., IOBM-associated SSTAs) remotely modulate the summer EAMBZ precipitation on interdecadal timescales, following the method of Zhang et al. (2019) and Yang et al. (2020), we adopt monthly mean outputs from two experiments of the Community Earth System Model version 1 (CESM1), which is a fully coupled Earth system model incorporating components of atmosphere, ocean, land, and sea ice (Hurrell et al., 2013).
The first experiment is the CESM1 Large Ensemble Numerical Simulation (referred to as CESM1_LENS; Kay et al., 2015). Among a total of 40 ensemble members in CESM1_LENS (Yang et al., 2020), we use the first 35 individual members according to many previous studies (e.g., Touma et al., 2021; J. Wang et al., 2023), which were completed at the climate modeling center of National Center for Atmospheric Research (NCAR). Note that all ensemble members in CESM1_LENS were imposed with the same radiative forcing scenario (Taylor et al., 2012), with historical forcing for 1920–2005, and high-emission forcing scenario (i.e., Representative Concentration Pathway 8.5) for 2006–2080 (Moss et al., 2010; Touma et al., 2021). The ensemble members were further generated with slightly differentiated perturbations of atmospheric states (Kay et al., 2015; Touma et al., 2021). The second experiment is the CESM1 Indian Ocean Pacemaker Ensemble Simulation (referred to as CESM1_IOPES), with 10 ensemble members (Zhang et al., 2019; Yang et al., 2020). We adopt CESM1_IOPES to highlight the impact of SSTAs over the broader TIO domain (15° S–15° N, African coast to 174° E). For the convenience of subsequent calculations and analyses, the African coast is designated as 40° E in this study, and a small change in the longitudes regarding the African coast may not affect the main results.
As indicated by Yang et al. (2020), the CESM1_LENS 35-member ensemble mean results can better provide an estimate of the influence due to external perturbations such as greenhouse gases on the climate system. Furthermore, the 10-member ensemble mean results in CESM1_IOPES contain the responses to both the time-evolving radiative forcing due to external perturbations and the restored observed time-varying SSTAs over the broader TIO domain (Yang et al., 2020). Note that though the ozone forcing data used in CESM1_IOPES differ from those in CESM1_LENS, the differences in the corresponding simulated tropical and extratropical climates were indistinguishable (e.g., Schneider et al., 2015; Schneider and Deser, 2018; Zhang et al., 2019; Yang et al., 2020). Therefore, by subtracting the CESM1_LENS ensemble mean from the CESM1_IOPES ensemble mean (i.e., removing the shared radiative forcing described above), we can obtain the response of the climate system to the internal variability stemming from the time-varying SSTAs over the specific TIO, isolating the intrinsic climate variability driven by TIO SSTAs by excluding the impacts of the time-evolving external radiative forcing. More details about CESM1_LENS and CESM1_IOPES can be found in Kay et al. (2015) and Yang et al. (2020), respectively. The variables employed here comprise precipitation and wind in the atmosphere component of the Community Atmospheric Model version 5, with a spatial resolution of 1.25° in longitude and 0.9° in latitude, and SST in the ocean component of the Parallel Ocean Program version 2, with 320 grids in longitude and 384 grids in latitude. Before further analyses, model outputs are interpolated at a resolution of 2° × 2° using a bilinear interpolation method (Mastyło, 2013) identical to that of 20CRv2c. In the current study, we focus on the historical simulation period of 1920–2005.
Here, it is important to stress the following two points. First, although the TIO domain in CESM1_IOPES is broader than that for defining IIOBM, there exist highly consistent temporal variations in SSTAs between them in observations (Fig. S2) and simulations (Fig. S3) at interdecadal timescales, with temporal correlation coefficients (TCCs) of 0.93 and 0.87 (P<0.01), respectively. Second, when selecting the SSTAs over the broader TIO domain (purple box in Fig. S4) as a metric, it can be found that the observed (Fig. S4a) and modeled (Fig. S4b) large and intense loadings of the positive SSTAs are still concentrated around the narrower TIO domain (black box in Fig. S4). As such, it is plausible to adopt the above-mentioned Indian Ocean pacemaker experiment with broader TIO SSTAs to validate our proposed mechanisms tied to the interdecadal IOBM variations.
3.1 Observed interdecadal variations of the summer precipitation over the EAMBZ and related background circulations
Figure 1c plots the spatial distribution of the interdecadal standard deviation of precipitation. This distribution is quite similar to that of the climatology (Fig. 1b), suggesting relatively strong (weak) interdecadal precipitation fluctuations over the EASM-dominated (westerly-controlled) part of the EAMBZ. Moreover, we show the first EOF mode of JJA-mean EAMBZ precipitation (Fig. 1d), which accounts for 28 % of the total variance and distinguishes from the remaining eigenvectors according to the criterion defined by North et al. (1982). The leading EOF mode bears close resemblance to the standard deviation of the EAMBZ precipitation on interdecadal timescales (Fig. 1c and d), with larger loadings occupying the Bohai Sea and Korean Peninsula and their adjoining regions. The interdecadal TCC between the principal component of the EOF1 and area-averaged precipitation over the research domain of the EAMBZ (35–55° N, 105–130° E) (EAMBZ precipitation index (IEAMBZP for short); Fig. 1e) is 0.93 (P<0.001). The aforementioned results indicate that our defined IEAMBZP can serve as a good indicator of the predominant fluctuations in the precipitation anomalies over the EAMBZ at interdecadal timescales. As such, from the time series of 11-year low-passed-filtered IEAMBZP (Fig. 1e), we can observe that the summer EAMBZ precipitation delineates notable interdecadal fluctuations. For example, the EAMBZ experienced dry summers during the periods preceding 1927, 1939–1945, 1968–1982, and 1998–2010 but underwent wet summers during the periods of 1928–1938, 1946–1967, and 2011 onwards. Note that, to some extent, the observed major interdecadal fluctuation periods of summertime EAMBZ precipitation are dissimilar from those tied to summertime Northeast Asian precipitation revealed by observations (1900–2012) from 11 local meteorological stations (Si et al., 2021), e.g., the above-normal precipitation over the EAMBZ (Fig. 1e) vs. the below-normal precipitation over Northeast Asia around 1990 (Si et al., 2021; their Fig. 2a).
Before examining the modulation of the IOBM on the interdecadal EAMBZ precipitation fluctuations, it is essential to scrutinize the JJA-mean IEAMBZP-associated circulation anomalies. The highest mid-latitude positive correlation region can be discerned north of the TP (38–46° N, 80–112.5° E; blue box in Fig. 2a), suggesting that the interdecadal enhancement of the summer EAMBZ precipitation is intimately correlated with the acceleration of the upstream mid-latitude westerlies at 400 hPa. In light of the method of Chen et al. (2021) and J. Wang et al. (2022), we correlate the IEAMBZP with the zonal winds averaged over the longitudinal range of the EAMBZ at multiple levels (Fig. 2b) to further check whether the most significant correlation occurs at 400 hPa. Evidently, on interdecadal timescales, the largest positive correlation between precipitation and mid-latitude westerlies within 38–46° N does occur at the mid-tropospheric level of 400 hPa, with a TCC of 0.46 (P<0.01) between the IEAMBZP and areal mean 400 hPa zonal winds over the upstream westerly-dominated domain (Fig. 2c). Note that this correlation pattern exhibits a barotropic structure (Fig. 2b). Additionally, we correlate the IEAMBZP with the 850 hPa meridional winds . The IEAMBZP is positively correlated with the key monsoonal southerly domain east of the TP (25–33° N, 102.5–112.5° E; green box in Fig. 2d), which is located in the western portion of the EASM domain (Ying et al., 2023). The interdecadal correlation pattern between meridional winds and the summer EAMBZ precipitation at multiple levels exhibits a baroclinic structure, with the significant positive correlations confined below 500 hPa (Fig. 2e). Note that the strongest positive correlation is detected at 850 hPa within 102.5–112.5° E, with a TCC of 0.63 (Fig. 2f; P<0.001) between IEAMBZP and areal mean 850 hPa meridional winds over the key EASM-controlled domain (Fig. 2d).
Figure 3 gives the JJA-mean IEAMBZP-regressed circulation anomalies at interdecadal timescales. The interdecadal enhancement of the EAMBZ precipitation is significantly linked to a localized quasi-barotropic cyclonic (low-pressure) anomaly. At 400 hPa, significant westerly anomalies prevail in its southern flank, inducing the acceleration of westerlies upstream of the EAMBZ (Fig. 3a). At 850 hPa, the enhanced EAMBZ precipitation is connected to a north–south meridional seesaw pattern, with a significant anticyclonic (high-pressure) anomaly over the subtropical western Pacific (SWP) and a significant cyclonic anomaly over the EAMBZ (Fig. 3b), exhibiting a somewhat barotropic structure (Fig. 3a and b). Significant southerly anomalies prevail in the western flank of this SWP clockwise gyre anomaly (SWPCGA). Moreover, from the perspective of < WVT > (Fig. 3c), the magnitudes of southerly < WVT > anomalies over the key EASM-controlled domain tied to the SWPCGA are much greater than the westerly < WVT > anomalies over the westerly-dominated domain. Note that the southerly < WVT > anomalies are significantly divergent, pushing copious amounts of warm and moist vapor over the SWP into the EAMBZ. Then, with the aid of the local counterclockwise < WVT > gyre pattern (Fig. 3c), the EASM southerlies from the low latitudes, which bring warm temperature advection anomalies, may easily collide with the mid-level cold temperature advection anomalies brought by mid-latitude enhanced westerlies (Fig. 4a and b), manifesting the extratropical–tropical interplay around the EAMBZ on interdecadal timescales. Such interplay is basically aligned with that on interannual timescales (cf. Chen et al., 2021). Under such environments, atmospheric instability over the EAMBZ can be triggered to generate in situ significant ascending motion anomalies responsible for increased precipitation (Fig. 5a). Note that considering the greater magnitudes of anomalies of < WVT > and warm temperature advection connected to the southerlies over the key EASM-controlled domain, we presume that the monsoonal southerlies play a predominant dynamical role in the interdecadal enhancement of precipitation over the EAMBZ. To verify this presumption, we further propose an East Asian monsoon index (IMI for short), defined as the areal mean meridional winds at 850 hPa over the key monsoonal southerly domain, and a westerly index (IWI for short), defined as the areal mean 400 hPa zonal winds over the upstream westerly-dominated region. The IMI-regressed results can well and realistically reproduce the magnitudes and distributions of the anomalous upward motions tied to IEAMBZP (Fig. 5b vs. Fig. 5a). However, the magnitudes of IWI-regressed results are highly weakened, along with the major loadings shifting more southward (Fig. 5c). The above results could allow us to conclude that the anomalous southerlies over the key monsoonal southerly domain could be the predominant driving factor for the interdecadal enhancement of summer EAMBZ precipitation, whereas the upstream accelerated westerlies play a secondary dynamical amplification role.
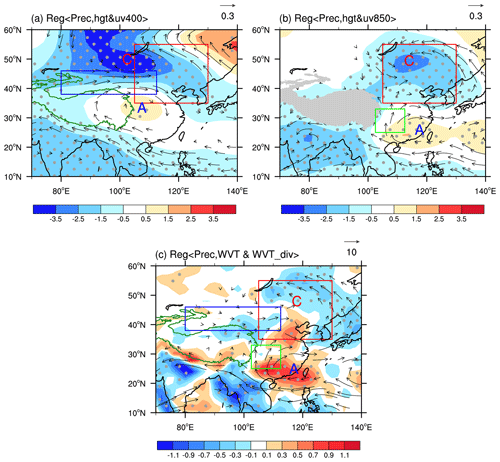
Figure 3Regression maps of the JJA-mean anomalies of (a) 400 hPa geopotential height (Z400; shading; m) and wind field (UV400; vectors; m s−1), (b) 850 hPa geopotential height (Z850; shading; m) and wind field (UV850; vectors; m s−1), and (c) < WVT > (vectors; kg m−1 s−1) and < WVT_div > (shading; 10−5 kg m−2 s−1) onto the concurrent IEAMBZP during the period 1901–2014. All variables are detrended and 11-year low-pass filtered. Letter A (C) represents the center of anticyclonic (cyclonic) anomaly (the same hereinafter). Areas with significant values of Z400, Z850, and <ẆVT_div > that exceed the 95 % confidence level are stippled, respectively. Only vectors that are significant at the 95 % confidence level are shown. The IEAMBZP is calculated based on the CRU TS3.26 precipitation data, while other variables are from the 20CRv2c datasets.
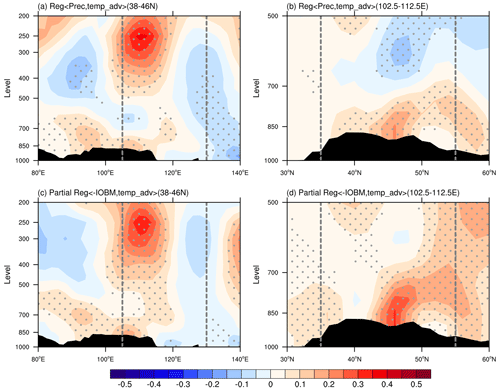
Figure 4(a) Height–longitude cross-section (averaged over 38–46° N) and (b) height–latitude cross-section (averaged over 102.5–112.5° E) of the JJA-mean temperature advection anomalies (shading; 10−5 K s−1) regressed onto the concurrent IEAMBZP during the period 1901–2014. (c, d) As in panels (a) and (b) but for patterns of the partial regression coefficient between temperature advection and negative IIOBM without the IPO forcing. The gray vertical lines in panels (a, c) and (b, d) represent the longitudinal and latitudinal range of the research domain of the EAMBZ, respectively. The black shading indicates the topography. All variables are detrended and 11-year low-pass filtered. Areas with significant values exceeding the 95 % confidence level are stippled. The IEAMBZP and IPO index are calculated based on the CRU TS3.26 precipitation data and the ERSSTv5 dataset, respectively, whilst other variables are from the 20CRv2c datasets.
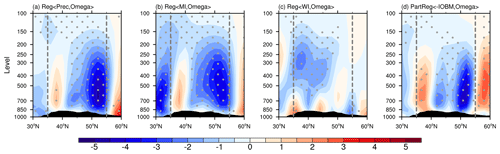
Figure 5Height–latitude cross-section (averaged over 105–130° E) of the JJA-mean vertical velocity anomalies (10−3 Pa s−1) regressed onto the concurrent (a) IEAMBZP, (b) IMI, and (c) IWI during the period 1901–2014. (d) As in panel (a) but for the partial regressed anomalies onto the negative IIOBM with the IPO forcing removed. The gray vertical lines represent the latitudinal range of the EAMBZ. The black shading indicates the topography. All variables are detrended and 11-year low-pass filtered. Areas with significant values exceeding the 95 % confidence level are stippled. The IEAMBZP and IPO index are calculated based on the CRU TS3.26 precipitation data and the ERSSTv5 dataset, respectively, whilst other variables are from the 20CRv2c datasets.
3.2 Interdecadal relationship between the IOBM and the summer EAMBZ precipitation
Many previous studies have substantiated that the IOBM can remotely modulate summer rainfall fluctuations over mid-latitude Asia at interdecadal timescales (e.g., Zhang et al., 2018; S. Wang et al., 2022; Wu et al., 2022). Note that the existing studies primarily highlighted the impacts of the IOBM on the summer rainfall variations over the northwest portion of mid-latitude Asia (e.g., S. Wang et al., 2022; Wu et al., 2022). As for the work of Zhang et al. (2018), although this study focused the northeast portion of mid-latitude Asia including the EAMBZ, it highlighted the combined roles of the IOBM, Atlantic multidecadal oscillation, and Pacific decadal oscillation. In the present study, however, we identify that it is the IOBM that may exert profoundly simultaneous impacts on the interdecadal variations of the EAMBZ precipitation in boreal summer, which is revealed in the following.
Figure 6a exhibits the correlation pattern between the JJA-mean IEAMBZP and the contemporaneous global gridded SST at interdecadal timescales. The most pronounced and significant correlations are found in the TIO sector, which largely matches the domain for delineating the IOBM mode (black frame in Fig. 6a). There exists a salient out-of-phase relationship between the interdecadal EAMBZ precipitation changes and the IOBM mode, with a TCC of −0.57 between IEAMBZP and IIOBM (Fig. 6b; P<0.01). This result suggests that IOBM warming (cooling) is significantly connected with dry (wet) EAMBZ summers, which serves as a critical oceanic modulator. On interdecadal timescales, the IOBM can remotely spark conducive dynamical circumstances for increased precipitation over the EAMBZ; i.e., the collision between cold and warm airflows around the EAMBZ (Fig. 4c and d) and the locally significant convergent ascending motion anomalies resembled those tied to the positive IEAMBZP (Fig. 5d vs. Fig. 5a). However, the extratropical cold (tropical warm) temperature advection anomalies west (south) of the EAMBZ, which are tied to the strengthened westerlies (southerlies), are quite insignificant (significant) (Fig. 4c and d). This indicates that the IOBM may exert a more profound influence on the southerly wind anomalies over the EASM-controlled domain, which is more important for enhanced EAMBZ precipitation, whereas the IOBM may insignificantly modulate the westerly anomalies over the westerly-dominated region. The possible underlying mechanisms of how the IOBM links the summertime circulation anomalies responsible for the interdecadal fluctuations in the EAMBZ precipitation are explained in the next subsection.
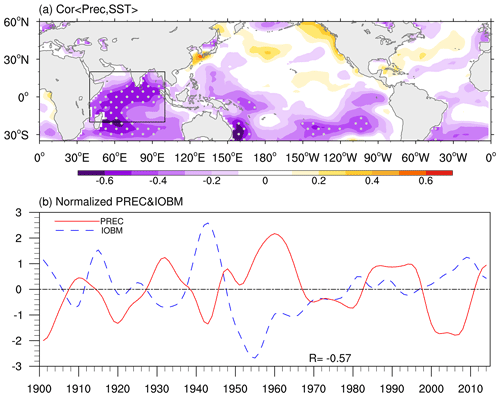
Figure 6(a) Correlation map of the JJA-mean IEAMBZP with the concurrent near-global SST (35° S–60° N) during the period 1901–2014. The black frame (20° S–20° N, 40–100° E) outlines the domain for delineating the IOBM mode (the same hereinafter). Areas with significant values exceeding the 99 % confidence level are stippled. (b) Normalized time series of the JJA-mean IEAMBZP (red line) and IIOBM (blue line) from 1901–2014. The numeral at the bottom represents the TCC between the corresponding time series. All variables are detrended and 11-year low-pass filtered. The SST is from the ERSSTv5 dataset. The IEAMBZP and IIOBM are calculated based on the CRU TS3.26 precipitation data and the ERSSTv5 datasets, respectively.
3.3 Possible mechanisms
Figure 7 shows partial regression of the JJA-mean anomalies of SST and large-scale precipitation over the TIO and its neighboring areas onto the IIOBM at interdecadal timescales with the IPO forcing removed. Corresponding to higher IIOBM years, warm SSTAs cover almost all areas of the TIO, with large loadings appearing in the central-southern TIO and relatively small loadings appearing in the northern TIO (Fig. 7a), which are aligned with the previous studies (Wu et al., 2016; Y. Huang et al., 2019). Moreover, there is striking suppressed precipitation around the northeast corner of the TIO domain (Fig. 7b), suggesting profoundly localized atmospheric responses (viz. the release of regional anomalous atmospheric cooling) to the warm TIO SSTAs. Note that corresponding to cold TIO SST years, there exist positive precipitation anomalies around the northeast corner of the TIO, suggesting the release of anomalous atmospheric heating (figure not shown). Due to the significant out-of-phase relationship between summertime IOBM and EAMBZ precipitation at interdecadal timescales, we adopt negative IIOBM-regressed patterns to express the influence of cold SSTAs over the TIO region. Figure 8 displays the anomalous patterns of the RWS, velocity potential, and divergent horizontal winds regressed onto the negative IIOBM. The velocity potential anomalies with larger negative (positive) loadings in the upper (lower) troposphere are concentrated surrounding the northeast corner of the TIO. Under these circumstances, local upper (lower) tropospheric divergence (convergence) and negative (positive) RWS anomalies can be observed (Fig. 8), suggesting enhanced ascending motions and convection activities in situ and thereby exciting the localized increased precipitation/atmospheric heating. The above results indicate that IOBM cooling may transmit its interdecadal influence via the intermediate atmospheric bridge of enhanced convective activities around the northeast corner of the TIO, exerting a remote modulation on an interdecadal enhancement of the EAMBZ rainfall.
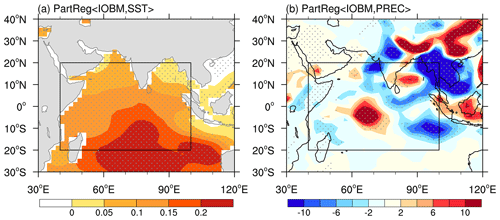
Figure 7Partial regression of the JJA-mean (a) SST (°) and (b) precipitation (millimeters per month) anomalies over the TIO and its neighboring areas onto the concurrent IIOBM with the IPO forcing removed for the period 1901–2014. All variables are detrended and 11-year low-pass filtered. Areas with significant values exceeding the 95 % confidence level are stippled. The IPO index is calculated based on the ERSSTv5 dataset. The SST and the precipitation are derived from the ERSSTv5 dataset and the 20CRv2c dataset, respectively.
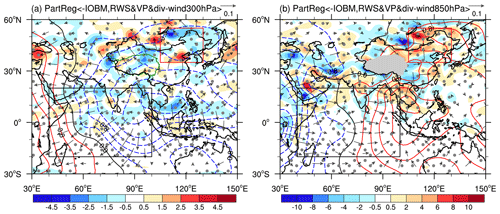
Figure 8Partial regression of the JJA-mean (a) 300 and (b) 850 hPa RWS (shading; 10−11 s−2), velocity potential (contours; interval: 0.4; 105 m2 s−1), and divergent horizontal wind (vectors; m s−1) anomalies against the concurrent negative IIOBM with the IPO forcing removed during the period 1901–2014. All variables are detrended and 11-year low-pass filtered. Areas with significant values of RWS exceeding the 95 % confidence level are stippled. The IPO index is calculated based on the ERSSTv5 dataset, whilst other variables are from the 20CRv2c datasets.
Next, we further discuss the physical pathway linking IOBM cooling with the far-reaching downstream circulation anomalies responsible for the interdecadal enhancement of EAMBZ precipitation, as shown in Fig. 9. Because the cyclonic anomaly at 400 hPa shifts more eastward compared to the IEAMBZP-regressed counterpart (Fig. 9a vs. Fig. 3a), only fractional westerly anomalies occupy the eastern part of the westerly-dominated region. The TCC between IIOBM and IWI is nearly equal to zero (), thus linking the insignificant cold temperature advection displayed in Fig. 4c. Nevertheless, in the lower troposphere, a north-low–south-high meridional seesaw pattern over the Northeast China–SWP sector is found to be linked with IOBM cooling (Fig. 9b). Note that this negative IIOBM-regressed seesaw pattern exhibits a quasi-barotropic structure, with a counterclockwise < WVT > gyre in the north and a SWPCGA in the south (Fig. 9c), which is highly similar to that shown in Fig. 3. Significant anomalies of 850 hPa meridional winds and southerly < WVT > prevail over the key monsoonal southerly domain, lying on the western flank of SWPCGA (Fig. 9b and c). The TCC between IIOBM and IMI is −0.33, which is significant at 0.05 on interdecadal timescales, thereby linking the significant warm temperature advection anomalies indicated in Fig. 4d.
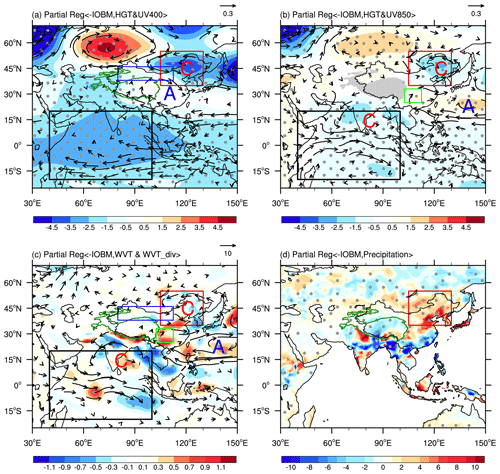
Figure 9Partial regression of the JJA-mean (a) Z400 (shading; m) and UV400 (vectors; m s−1), (b) Z850 (shading; m) and UV850 (vectors; m s−1), (c) < WVT > (vectors; kg m−1 s−1) and < WVT_div > (shading; 10−5 kg m−2 s−1), and precipitation (millimeters per month) anomalies onto the concurrent negative IIOBM with the IPO forcing removed during the period 1901–2014. All variables are detrended and 11-year low-pass filtered. Areas with significant values of Z400, Z850, and < WVT_div > that exceed the 95 % confidence level are stippled, respectively. Only vectors that are significant at the 95 % confidence level are shown. The IPO index is calculated based on the ERSSTv5 dataset; the precipitation is derived from the CRU TS3.26 precipitation data, whilst other variables are from the 20CRv2c datasets.
One may ask how IOBM cooling induces the above-mentioned meridional seesaw pattern. Previously, we have revealed that negative SSTAs over the TIO may exert remote interdecadal impacts through an atmospheric bridge, i.e., vigorous convective activities around the northeast corner of the TIO (Figs. 7 and 8). In effect, there exists a low-level cyclonic anomaly in situ (Fig. 9b). Such cyclonic anomaly can be interpreted as a typical Gill–Matsuno-type response (Matsuno, 1966; Gill, 1980) to the regional anti-symmetric atmospheric heating caused by IOBM cooling with the coldest center located south of the Equator, which is more clear within the lower levels (Fig. 9b). As a result, consistent easterly anomalies appear from the SWP to its northern flank around 15° N, denoting the active role of depressed air pressure. The consistent easterly anomalies over the SWP could lead to local anticyclonic wind shear anomalies (Wang et al., 2019). In such a scenario, a quasi-barotropic SWPCGA can be induced (Fig. 9c). Further, local downward motions tied to SWPCGA could induce significant upward motions to its north via a meridional overturning circulation (J. Wang et al., 2021), thus exciting a quasi-barotropic cyclonic anomaly and a counterclockwise < WVT > gyre pattern centered over Northeast China (Fig. 9a–c). Therefore, positive summertime rainfall anomalies over the EAMBZ at interdecadal timescales can be induced (Fig. 9d). Notably, circulation and precipitation anomalies during the warm phase years of the IOBM (Fig. S5) highly mirror those tied to the IOBM cooling with opposite signs.
3.4 Results from CESM1 simulations
In this subsection, we use the pacemaker experimental data based on the ensemble mean of CESM1_IOPES and CESM1_LENS to validate our proposed mechanisms regarding the modulation of IOBM cooling on the interdecadal enhancement of summer EAMBZ precipitation. Considering the predominant role of southerly anomalies over the key monsoonal southerly domain, we therefore emphasize the low-level (850 hPa) atmospheric anomalies at interdecadal timescales tied to the IOBM-like SST cooling, as depicted in Fig. 10. We can observe a clearly anomalous cyclonic circulation around the northeast corner of the TIO, accompanied by local positive precipitation anomalies and easterly anomalies that stretch from the SWP to its northern flank, which generally resembled those in the observation (Fig. 9). In this circumstance, a similar north-low–south-high meridional seesaw pattern over the Northeast China–SWP sector can be simulated to spark and sustain the enhanced EAMBZ precipitation in boreal summer (Fig. 10). In summary, by and large, the ensemble mean composite results can well reproduce the observed anomalous circulation and precipitation driven by IOBM-related SSTAs, confirming the crucial role of IOBM cooling in driving enhanced summer precipitation over the EAMBZ at interdecadal timescales.
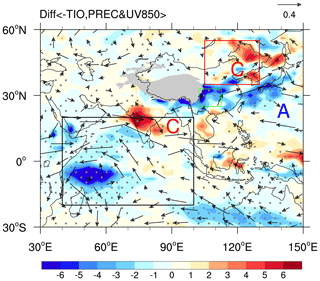
Figure 10Simulated composite differences of JJA-mean UV850 (vectors; m s−1) and precipitation (shading; millimeters per month) between cold and warm SST years over the broader TIO domain in CESM1_IOPES (15° S–15° N, 40–174° E; purple box in Fig. S4). The warm and cold TIO SST years are selected based on the ± 0.5 standard deviations of the simulated time-evolving SSTAs during 1920–2005, as shown in Fig. S3 (red line). All variables are detrended and 11-year low-pass filtered. Areas with significant values of precipitation that exceed the 95 % confidence level are stippled. Only vectors that are significant at the 95 % confidence level are shown. The simulated anomalies of UV850 and precipitation are calculated based on the difference between the CESM1_IOPES ensemble mean and the CESM1_LENS ensemble mean (former minus latter), highlighting the internally driven impacts of TIO SSTAs.
3.5 Estimation of the interdecadal variations of summer EAMBZ precipitation
In the last three subsections, we suggest that the IOBM cooling can serve as a significant oceanic modulator for increased summer EAMBZ precipitation at interdecadal timescales based on observation evidence and pacemaker experiments, as well as present the corresponding physical mechanisms. To estimate their steady antiphase relationship, in the following, the negative IIOBM is selected to construct a physically based empirical model by using the simple linear regression analysis and the cross-validation method (You and Jia, 2018; Chang et al., 2021; Jeong et al., 2021), representing the impact of IOBM cooling. The physically based model is given as follows:
where β0 and β1 are regression coefficients, and ε denotes the residuals. The time series of IEAMBZP and IIOBM are detrended and 11-year low-pass filtered beforehand.
Following the method of Jeong et al. (2021), a leave-one-out cross-validation strategy is employed to determine the robustness of the hindcast estimates. The normalized time series of summer IEAMBZP and associated leave-one-out cross-validated hindcast estimates are shown in Fig. 11. The TCC between the physically based predicted hindcast estimates (blue line) and the observed IEAMBZP (red line) for 1901–2014 can reach 0.56 (P<0.05), suggesting that the physically based model can well capture the interdecadal IEAMBZP variations and reflect their steady relationship.
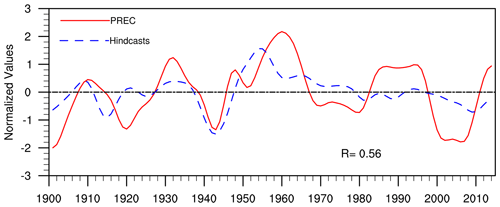
Figure 11Normalized time series of the JJA-mean IEAMBZP (red line) and associated leave-one-out cross-validated hindcast estimates (blue line) for 1901–2014, with the number denoting the TCC between the corresponding time series.
Although our proposed physically based empirical model could confirm the concurrently intimately interdecadal relationship between IOBM and EAMBZ precipitation, we should acknowledge the shortcomings of the model. First, the amplitudes of the hindcast estimates are fairly lower, which cannot well capture the extreme precipitation years (e.g., years around 1960; Fig. 11). Second, the simultaneous forcing of the IOBM cannot be served as a predictor for summertime EAMBZ precipitation variations. As such, this model inherently lacks the ability to predict the interdecadal EAMBZ precipitation anomalies in advance.
The EAMBZ is a peculiar domain defined from the perspective of the interplay between climatic systems (i.e., mid-latitude westerly and EASM). In this study, by analysis of the long-term observational and reanalysis datasets during 1901–2014, the temporal characteristics of interdecadal variations in the summer EAMBZ precipitation and associated circulation background are revealed. The potential modulation of the IOBM on the variations is further discussed. As a summary of our major findings, Fig. 12 schematically synthesizes how the IOBM-associated SST mode remotely drives the interdecadal precipitation fluctuations via a tropical route.
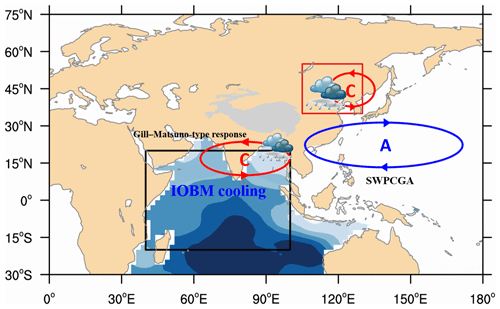
Figure 12Schematic diagram showing how the IOBM-related SST anomaly pattern drives the summer EAMBZ precipitation fluctuations at interdecadal timescales. Blue shading illustrates the IOBM cooling. Letter A (C) indicates the center of the anticyclonic (cyclonic) gyre anomaly.
The summer EAMBZ precipitation exhibited salient interdecadal fluctuations, e.g., with dry summers during the periods preceding 1927, 1939–1945, 1968–1982, and 1998–2010, as well as wet summers during the periods of 1928–1938, 1946–1967, and 2011 onwards. It is indicated that the cold airflows brought by the mid-latitude-accelerated upstream westerlies over the westerly-dominated domain collide and converge with the warm and humid airflows brought by the enhanced southerlies over the key EASM-controlled domain, suggesting the local extratropical–tropical interplay. Further diagnostic results suggest that the monsoonal southerly anomalies could be viewed as the predominant driving factor for the interdecadal enhancement of EAMBZ precipitation, whereas the upstream westerlies play a secondary dynamical amplification role. Such circulation anomalies are closely linked to a north-low–south-high meridional seesaw pattern over the Northeast China–SWP sector, which provides favorable environments for the transportation of water vapor from the SWP and the convergence over the EAMBZ to spark enhanced summer EAMBZ precipitation at interdecadal timescales.
We further identify that, amongst the major interdecadal oceanic forcings (e.g., Atlantic multidecadal oscillation and Pacific decadal oscillation), the IOBM-related SST anomaly pattern is a salient oceanic forcing for the interdecadal variations of the summer EAMBZ precipitation via the Gill–Matsuno mechanism, playing an independent and critical modulation role. When the cold phase of the IOBM occurs, an anomalous cyclonic circulation is excited around the northeast corner of the TIO in terms of the regional anti-symmetric atmospheric heating. As a response, consistent easterly anomalies appear from the SWP to its northern flank, leading to local anticyclonic wind shear anomalies and thus inducing a SWPCGA pattern and a resultant counterclockwise gyre pattern centered over Northeast China. On interdecadal timescales, such meridional seesaw pattern tied to the IOBM cooling is responsible for enhanced summer precipitation over the EAMBZ by linking the predominant driving factor of strengthened monsoonal southerly anomalies west of the SWPCGA pattern. As such, the water vapor transportation from the SWP and the convergence over the EAMBZ can be triggered to induce and sustain the enhancement of local precipitation. Correspondingly, a physically based model based on the negative IIOBM is constructed, which can well capture the interdecadal fluctuations in the EAMBZ precipitation and reflect their steady relationship. Furthermore, the results based on the large ensemble experiment and the Indian Ocean pacemaker experiment also confirm the crucial physical pathway linking the SST variations over the TIO with the summer precipitation over the EAMBZ via the influence of SST variations on the aforementioned meridional seesaw pattern at interdecadal timescales.
The following two points deserve further discussion. First, although results from CESM1_LENS and CESM1_IOPES can reasonably confirm our proposed physical pathway of how IOBM cooling exerts a distant modulation on the interdecadal enhancement of summer precipitation over the EAMBZ, we can still notice the weakness of the model simulations. That is, positive precipitation anomalies around the northeast corner of the TIO and the easterly anomalies exhibit weaker magnitudes compared to the observations (Fig. 10 vs. Figs. 7b and 9b). Besides, systematic biases exist regarding the simulated positions of the upper (lower) tropospheric divergence (convergence) and negative (positive) RWS anomalies (Fig. S6), manifesting themselves in the eastward displacement tendency in contrast to those around the northeast corner of the TIO (Fig. 8).
Second, this study merely identifies the physical linkage between the interdecadal summer EAMBZ precipitation and the contemporaneous SST mode over the TIO basin from the tropical route. Nonetheless, the contemporaneous IOBM is not a predictor. According to many previous studies (e.g., Wang et al., 2015; Li et al., 2023), the physically based empirical model based on multiple predictors may better improve the forecast skill. Thus, it is urgent to find out more salient precursor forcings of the lower boundary anomalies (e.g., sea ice, Han et al., 2021) and figure out associated mechanisms for interdecadal EAMBZ precipitation changes to construct an effective prediction model.
The CRU time series precipitation data version 3.26 (CRU TS3.26) from CRU at the University of East Anglia are available online (https://catalogue.ceda.ac.uk/uuid/3f8944800cc48e1cbc29a5ee12d8542d; CRU, 2022). The ERSSTv5 data from the US NOAA are available from the following website: https://www1.ncdc.noaa.gov/pub/data/cmb/ersst/v5/netcdf/ (NOAA, 2020). The 20CRv2c datasets from NOAA-CIRES are available from the following website: https://psl.noaa.gov/data/gridded/data.20thC_ReanV2c.html (NOAA-CIRES, 2022). The model simulation datasets regarding CESM1_LENS are available online (https://www.cesm.ucar.edu/community-projects/lens/data-sets; NCAR, 2023a). The model simulation datasets regarding CESM1_IOPES are available online (https://www.earthsystemgrid.org/dataset/ucar.cgd.ccsm4.IOD-PACEMAKER.html; NCAR, 2023b). Codes are available from the corresponding author on reasonable request.
The supplement related to this article is available online at: https://doi.org/10.5194/acp-24-5099-2024-supplement.
YL designed the research; JW wrote the first draft of the paper; FC and CS downloaded and analyzed the data and plotted the figures used in this study. All authors, including QL, YD, and XX, contributed to the discussion of the results and reviewed the manuscript.
The contact author has declared that none of the authors has any competing interests.
Publisher’s note: Copernicus Publications remains neutral with regard to jurisdictional claims made in the text, published maps, institutional affiliations, or any other geographical representation in this paper. While Copernicus Publications makes every effort to include appropriate place names, the final responsibility lies with the authors.
Yanju Liu acknowledges the support by the Key Innovation Team of the China Meteorological Administration “Climate Change Detection and Response” (grant no. CMA2022ZD03).
This study was supported by the Second Tibetan Plateau Scientific Expedition and Research (STEP) program (grant nos. 2019QZKK010204-02 and 2019QZKK0102), Guangdong Major Project of Basic and Applied Basic Research (grant no. 2020B0301030004), and Innovation and Development Special Project of the China Meteorological Administration (grant no. CXFZ2022J039).
This paper was edited by Peer Nowack and reviewed by two anonymous referees.
Chang, L., Wu, Z., and Xu, J.: Contribution of Northeastern Asian stratospheric warming to subseasonal prediction of the early winter haze pollution in Sichuan Basin, China, Sci. Total Environ., 751, 141823, https://doi.org/10.1016/j.scitotenv.2020.141823, 2021.
Chen, F.-H., Chen, J.-H., Holmes, J., Boomer, I., Austin, P., Gates, J. B., Wang, N.-L., Brooks, S. J., and Zhang, J.-W.: Moisture changes over the last millennium in arid central Asia: A review, synthesis and comparison with monsoon region, Quaternary Sci. Rev., 29, 1055–1068, https://doi.org/10.1016/j.quascirev.2010.01.005, 2010.
Chen, J., Huang, W., Jin, L., Chen, J., Chen, S., and Chen, F.: A climatological northern boundary index for the East Asian summer monsoon and its interannual variability, Sci. China Earth Sci., 61, 13–22, https://doi.org/10.1007/s11430-017-9122-x, 2018.
Chen, J., Huang, W., Feng, S., Zhang, Q., Kuang, X., Chen, J., and Chen, F.: The modulation of westerlies-monsoon interaction on climate over the monsoon boundary zone in East Asia, Int. J. Climatol., 41, E3049–E3064, https://doi.org/10.1002/joc.6903, 2021.
Compo, G. P., Whitaker, J. S., Sardeshmukh, P. D., Matsui, N., Allan, R. J., Yin, X., Gleason, B. E., Vose, R. S., Rutledge, G., Bessemoulin, P., Brönnimann, S., Brunet, M., Crouthamel, R. I., Grant, A. N., Groisman, P. Y., Jones, P. D., Kruk, M. C., Kruger, A. C., Marshall, G. J., Maugeri, M., Mok, H. Y., Nordli, Ø., Ross, T. F., Trigo, R. M., Wang, X. L., Woodruff, S. D., and Worley, S. J.: The Twentieth Century Reanalysis Project, Q. J. R. Meteorol. Soc., 137, 1–28, https://doi.org/10.1002/qj.776, 2011.
CRU: CRU TS3.26, monthly, CRU [data set] https://catalogue.ceda.ac.uk/uuid/3f8944800cc48e1cbc29a5ee12d8542d, last access: 5 July 2022.
Ding, Y. and Chan, J. C. L.: The East Asian summer monsoon: An overview, Meteorol. Atmos. Phys., 89, 117–142, https://doi.org/10.1007/s00703-005-0125-z, 2005.
Dou, J. and Wu, Z.: Southern Hemisphere origins for interannual variations of snow cover over the western Tibetan Plateau in boreal summer, J. Clim., 31, 7701–7718, https://doi.org/10.1175/JCLI-D-17-0327.1, 2018.
Duchon, C. E.: Lanczos filtering in one and two dimensions, J. Appl. Meteorol. Clim., 18, 1016–1022, https://doi.org/10.1175/1520-0450(1979)018<1016:LFIOAT>2.0.CO;2, 1979.
Gill, A. E.: Some simple solutions for heat-induced tropical circulation, Q. J. R. Meteorol. Soc., 106, 447–462, https://doi.org/10.1002/qj.49710644905, 1980.
Han, T., Zhang, M., Zhu, J., Zhou, B., and Li, S.: Impact of early spring sea ice in Barents Sea on midsummer rainfall distribution at Northeast China, Clim. Dynam., 57, 1023–1037, https://doi.org/10.1007/s00382-021-05754-4, 2021.
Han, W., Vialard, J., McPhaden, M. J., Lee, T., Masumoto, Y., Feng, M., and de Ruijter, W. P. M.: Indian Ocean decadal variability: A review, Bull. Am. Meteorol. Soc., 95, 1679–1703, https://doi.org/10.1175/BAMS-D-13-00028.1, 2014.
Harris, I., Jones, P. D., Osborn, T. J., and Lister, D. H.: Updated high-resolution grids of monthly climatic observations – the CRU TS3.10 Dataset, Int. J. Climatol., 34, 623–642, https://doi.org/10.1002/joc.3711, 2014.
Henley, B. J., Gergis, J., Karoly, D. J., Power, S., Kennedy, J., and Folland, C. K.: A Tripole Index for the Interdecadal Pacific Oscillation, Clim. Dynam., 45, 3077–3090, https://doi.org/10.1007/s00382-015-2525-1, 2015.
Huang, B., Thorne, P. W., Banzon, V. F., Boyer, T., Chepurin, G., Lawrimore, J. H., Menne, M. J., Smith, T. M., Vose, R. S., and Zhang, H.-M.: Extended Reconstructed Sea Surface Temperature, version 5 (ERSSTv5): Upgrades, validations, and intercomparisons, J. Clim., 30, 8179–8205, https://doi.org/10.1175/JCLI-D-16-0836.1, 2017.
Huang, J., Ma, J., Guan, X., Li, Y., and He, Y.: Progress in semi-arid climate change studies in China, Adv. Atmos. Sci., 36, 922–937, https://doi.org/10.1007/s00376-018-8200-9, 2019.
Huang, J., Zhang, G., Zhang, Y., Guan, X., Wei, Y., and Guo, R.: Global desertification vulnerability to climate change and human activities, Land Degrad. Dev., 31, 1380–1391, https://doi.org/10.1002/ldr.3556, 2020.
Huang, J., Li, Y., Fu, C., Chen, F., Fu, Q., Dai, A., Shinoda, M., Ma, Z., Guo, W., Li, Z., Zhang, L., Liu, Y., Yu, H., He, Y., Xie, Y., Guan, X., Ji, M., Lin, L., Wang, S., Yan, H., and Wang, G.: Dryland climate change: Recent progress and challenges, Rev. Geophys., 55, 719–778, https://doi.org/10.1002/2016RG000550, 2017.
Huang, W., Chen, J., Zhang, X., Feng, S., and Chen, F.: Definition of the core zone of the “westerlies-dominated climatic regime”, and its controlling factors during the instrumental period, Sci. China Earth Sci., 58, 676–684, https://doi.org/10.1007/s11430-015-5057-y, 2015.
Huang, Y., Wu, B., Li, T., Zhou, T., and Liu, B.: Interdecadal Indian Ocean basin mode driven by interdecadal Pacific oscillation: A season-dependent growth mechanism, J. Clim., 32, 2057–2073, https://doi.org/10.1175/JCLI-D-18-0452.1, 2019.
Hurrell, J. W., Holland, M. M., Gent, P. R., Ghan, S., Kay, J. E., Kushner, P. J., Lamarque, J. F., Large, W. G., Lawrence, D., Lindsay, K., Lipscomb, W. H., Long, M. C., Mahowald, N., Marsh, D. R., Neale, R. B., Rasch, P., Vavrus, S., Vertenstein, M., Bader, D., Collins, W. D., Hack, J. J., Kiehl, J., and Marshall, S.: The Community Earth System Model: A framework for collaborative research, Bull. Am. Meteorol. Soc., 94, 1339–1360, https://doi.org/10.1175/BAMS-D-12-00121.1, 2013.
Jeong, J. I., Park, R. J., Yeh, S.-W., and Roh, J.-W.: Statistical predictability of wintertime PM2.5 concentrations over East Asia using simple linear regression, Sci. Total Environ., 776, 146059, https://doi.org/10.1016/j.scitotenv.2021.146059, 2021.
Jiang, J., Zhou, T., Chen, X., and Wu, B.: Central Asian precipitation shaped by the tropical Pacific decadal variability and the Atlantic multidecadal variability, J. Clim., 34, 7541–7553, https://doi.org/10.1175/JCLI-D-20-0905.1, 2021.
Kay, J. E., Deser, C., Phillips, A., Mai, A., Hannay, C., Strand, G., Arblaster, J. M., Bates, S. C., Danabasoglu, G., Edwards, J., Holland, M., Kushner, P., Lamarque, J. F., Lawrence, D., Lindsay, K., Middleton, A., Munoz, E., Neale, R., Oleson, K., Polvani, L., and Vertenstein, M.: The community earth system model (CESM) large ensemble project: A community resource for studying climate change in the presence of internal climate variability, Bull. Am. Meteorol. Soc., 96, 1333–1349, https://doi.org/10.1175/BAMS-D-13-00255.1, 2015.
Klein, S. A., Soden, B. J., and Lau, N.-C.: Remote sea surface temperature variations during ENSO: Evidence for a tropical atmospheric bridge, J. Clim., 12, 917–932, https://doi.org/10.1175/1520-0442(1999)012<0917:RSSTVD>2.0.CO;2, 1999.
Li, J. and Zeng, Q.: A unified monsoon index, Geophys. Res. Lett., 29, 115-111–115-114, https://doi.org/10.1029/2001GL013874, 2002.
Li, J., Sun, C., and Jin, F.-F.: NAO implicated as a predictor of Northern Hemisphere mean temperature multidecadal variability, Geophys. Res. Lett., 40, 5497–5502, https://doi.org/10.1002/2013GL057877, 2013.
Li, J., Zheng, C., Yang, Y., Lu, R., and Zhu, Z.: Predictability of spatial distribution of pre-summer extreme precipitation days over southern China revealed by the physical-based empirical model, Clim. Dynam., 61, 2299–2316, https://doi.org/10.1007/s00382-023-06681-2, 2023.
Li, M. and Ma, Z.: Decadal changes in summer precipitation over arid northwest China and associated atmospheric circulations, Int. J. Climatol., 38, 4496–4508, https://doi.org/10.1002/joc.5682, 2018.
Lu, W. and Jia, G.: Fluctuation of farming-pastoral ecotone in association with changing East Asia monsoon climate, Climatic Change, 119, 747–760, https://doi.org/10.1007/s10584-013-0761-0, 2013.
Mastyło, M.: Bilinear interpolation theorems and applications, J. Funct. Anal., 265, 185–207, https://doi.org/10.1016/j.jfa.2013.05.001, 2013.
Matsuno, T.: Quasi-geostrophic motions in the equatorial area, J. Meteorol. Soc. Jpn., 44, 25–43, https://doi.org/10.2151/jmsj1965.44.1_25, 1966.
Moss, R. H., Edmonds, J. A., Hibbard, K. A., Manning, M. R., Rose, S. K., van Vuuren, D. P., Carter, T. R., Emori, S., Kainuma, M., Kram, T., Meehl, G. A., Mitchell, J. F. B., Nakicenovic, N., Riahi, K., Smith, S. J., Stouffer, R. J., Thomson, A. M., Weyant, J. P., and Wilbanks, T. J.: The next generation of scenarios for climate change research and assessment, Nature, 463, 747–756, https://doi.org/10.1038/nature08823, 2010.
NCAR: CESM1_LENS, NCAR [data set], https://www.cesm.ucar.edu/community-projects/lens/data-sets (last access: 28 April 2023), 2023a.
NCAR: CESM1_IOPES, NCAR [data set], https://www.earthsystemgrid.org/dataset/ucar.cgd.ccsm4.IOD-PACEMAKER.html (last access: 4 May 2023), 2023b.
NOAA: ERSSTv5, NOAA [data set], https://www1.ncdc.noaa.gov/pub/data/cmb/ersst/v5/netcdf/, last access: 15 October 2020.
NOAA-CIRES: 20CRv2c, NOAA-CIRES [data set], https://psl.noaa.gov/data/gridded/data.20thC_ReanV2c.html, last access: 26 June 2022.
North, G. R., Bell, T. L., Cahalan, R. F., and Moeng, F. J.: Sampling errors in the estimation of empirical orthogonal functions, Mon. Weather Rev., 110, 699–706, https://doi.org/10.1175/1520-0493(1982)110<0699:seiteo>2.0.co;2, 1982.
Ou, T. H. and Qian, W. H.: Vegetation variations along the monsoon boundary zone in East Asia, Chinese J. Geophys., 49, 698–705, https://doi.org/10.1002/cjg2.876, 2006.
Piao, J., Chen, W., and Chen, S.: Water vapour transport changes associated with the interdecadal decrease in the summer rainfall over Northeast Asia around the late-1990s, Int. J. Climatol., 41, E1469–E1482, https://doi.org/10.1002/joc.6780, 2021.
Qian, W., Ding, T., Hu, H., Lin, X., and Qin, A.: An overview of dry-wet climate variability among monsoon-westerly regions and the monsoon northernmost marginal active zone in China, Adv. Atmos. Sci., 26, 630–641, https://doi.org/10.1007/s00376-009-8213-5, 2009.
Sardeshmukh, P. D. and Hoskins, B. J.: The generation of global rotational flow by steady idealized tropical divergence, J. Atmos. Sci., 45, 1228–1251, https://doi.org/10.1175/1520-0469(1988)045<1228:TGOGRF>2.0.CO;2, 1988.
Schiemann, R., Lüthi, D., and Schär, C.: Seasonality and interannual variability of the westerly jet in the Tibetan Plateau region, J. Clim., 22, 2940–2957, https://doi.org/10.1175/2008jcli2625.1, 2009.
Schneider, D. P. and Deser, C.: Tropically driven and externally forced patterns of Antarctic sea ice change: Reconciling observed and modeled trends, Clim. Dynam., 50, 4599–4618, https://doi.org/10.1007/s00382-017-3893-5, 2018.
Schneider, D. P., Deser, C., and Fan, T.: Comparing the impacts of tropical SST variability and polar stratospheric ozone loss on the southern ocean westerly winds, J. Clim., 28, 9350–9372, https://doi.org/10.1175/JCLI-D-15-0090.1, 2015.
Si, D. and Ding, Y.: Oceanic forcings of the interdecadal variability in East Asian summer rainfall, J. Clim., 29, 7633–7649, https://doi.org/10.1175/JCLI-D-15-0792.1, 2016.
Si, D., Jiang, D., Hu, A., and Lang, X.: Variations in northeast Asian summer precipitation driven by the Atlantic multidecadal oscillation, Int. J. Climatol., 41, 1682–1695, https://doi.org/10.1002/joc.6912, 2021.
Song, C., Wang, J., Liu, Y., Zhang, L., Ding, Y., Li, Q., Shen, X., Song, Y., and Yan, Y.: Toward role of westerly-monsoon interplay in linking interannual variations of late spring precipitation over the southeastern Tibetan Plateau, Atmos. Sci. Lett., 23, e1074, https://doi.org/10.1002/asl.1074, 2022.
Sun, B., Li, H., and Zhou, B.: Interdecadal variation of Indian Ocean basin mode and the impact on Asian summer climate, Geophys. Res. Lett., 46, 12388–12397, https://doi.org/10.1029/2019GL085019, 2019a.
Sun, B., Wang, H., Zhou, B., and Li, H.: Interdecadal variation in the synoptic features of mei-yu in the Yangtze River valley region and relationship with the Pacific decadal oscillation, J. Clim., 32, 6251–6270, https://doi.org/10.1175/jcli-d-19-0017.1, 2019b.
Taylor, K. E., Stouffer, R. J., and Meehl, G. A.: An overview of CMIP5 and the experiment design, Bull. Am. Meteorol. Soc., 93, 485–498, https://doi.org/10.1175/BAMS-D-11-00094.1, 2012.
Touma, D., Stevenson, S., Lehner, F., and Coats, S.: Human-driven greenhouse gas and aerosol emissions cause distinct regional impacts on extreme fire weather, Nat. Commun., 12, 212, https://doi.org/10.1038/s41467-020-20570-w, 2021.
Wang, B., Wu, Z., Li, J., Liu, J., Chang, C.-P., Ding, Y., and Wu, G.: How to measure the strength of the East Asian summer monsoon, J. Clim., 21, 4449–4463, https://doi.org/10.1175/2008jcli2183.1, 2008.
Wang, B., Xiang, B., Li, J., Webster, P. J., Rajeevan, M. N., Liu, J., and Ha, K.-J.: Rethinking Indian monsoon rainfall prediction in the context of recent global warming, Nat. Commun., 6, 7154, https://doi.org/10.1038/ncomms8154, 2015.
Wang, J., Liu, Y., Ding, Y., and Wu, Z.: Towards influence of Arabian Sea SST anomalies on the withdrawal date of Meiyu over the Yangtze-Huaihe River basin, Atmos. Res., 249, 105340, https://doi.org/10.1016/j.atmosres.2020.105340, 2021.
Wang, J., Zhu, Z. W., Qi, L., Zhao, Q. H., He, J. H., and Wang, J. X. L.: Two pathways of how remote SST anomalies drive the interannual variability of autumnal haze days in the Beijing–Tianjin–Hebei region, China, Atmos. Chem. Phys., 19, 1521–1535, https://doi.org/10.5194/acp-19-1521-2019, 2019.
Wang, J., Liu, Y., Song, C., Ding, Y., Li, Q., Wu, P., Xu, Y., and Xu, X.: Synergistic impacts of westerlies and monsoon on interdecadal variations of late spring precipitation over the southeastern extension of the Tibetan Plateau, Int. J. Climatol., 42, 7342–7361, https://doi.org/10.1002/joc.7648, 2022.
Wang, J., Liu, Y., Yang, Y., Wu, P., Yang, J., Liang, P., Song, C., Zhang, S., and Ding, Y.: Impact of early winter North Atlantic Oscillation on the dramatic alternation of seesaw haze intensity between late winter months in the North China Plain, Atmos. Res., 281, 106483, https://doi.org/10.1016/j.atmosres.2022.106483, 2023.
Wang, L., Chen, W., Huang, G., and Zeng, G.: Changes of the transitional climate zone in East Asia: Past and future, Clim. Dynam., 49, 1463–1477, https://doi.org/10.1007/s00382-016-3400-4, 2017.
Wang, Q., Wang, L., Huang, G., Piao, J., and Chotamonsak, C.: Temporal and spatial variation of the transitional climate zone in summer during 1961–2018, Int. J. Climatol., 41, 1633–1648, https://doi.org/10.1002/joc.6902, 2021.
Wang, Q., Wang, L., Huang, G., and Wang, T.: Mechanism of the summer rainfall interannual variability in transitional climate zone in East Asia: Roles of teleconnection patterns and associated moisture processes, Clim. Dynam., 61, 1177–1192, https://doi.org/10.1007/s00382-022-06618-1, 2022.
Wang, Q., Huang, G., Wang, L., Piao, J., Ma, T., Hu, P., Chotamonsak, C., and Limsakul, A.: Mechanism of the summer rainfall variation in Transitional Climate Zone in East Asia from the perspective of moisture supply during 1979–2010 based on the Lagrangian method, Clim. Dynam., 60, 1225–1238, https://doi.org/10.1007/s00382-022-06344-8, 2023.
Wang, S., Huang, J., Huang, G., Luo, F., Ren, Y., and He, Y.: Enhanced impacts of Indian Ocean sea surface temperature on the dry/wet variations over Northwest China, J. Geophys. Res.-Atmos., 127, e2022JD036533, https://doi.org/10.1029/2022JD036533, 2022.
Wu, B., Zhou, T., and Li, T.: Impacts of the Pacific–Japan and circumglobal teleconnection patterns on the interdecadal variability of the East Asian summer monsoon, J. Clim., 29, 3253–3271, https://doi.org/10.1175/JCLI-D-15-0105.1, 2016.
Wu, G., Guan, Y., Liu, Y., Yan, J., and Mao, J.: Air–sea interaction and formation of the Asian summer monsoon onset vortex over the Bay of Bengal, Clim. Dynam., 38, 261–279, https://doi.org/10.1007/s00382-010-0978-9, 2012.
Wu, P., Liu, Y., Ding, Y., Li, X., and Wang, J.: Modulation of sea surface temperature over the North Atlantic and Indian-Pacific warm pool on interdecadal change of summer precipitation over northwest China, Int. J. Climatol., 42, 8526–8538, https://doi.org/10.1002/joc.7743, 2022.
Xie, S.-P., Hu, K., Hafner, J., Tokinaga, H., Du, Y., Huang, G., and Sampe, T.: Indian Ocean capacitor effect on Indo–western Pacific climate during the summer following El Niño, J. Clim., 22, 730–747, https://doi.org/10.1175/2008jcli2544.1, 2009.
Xing, W. and Wang, B.: Predictability and prediction of summer rainfall in the arid and semi-arid regions of China, Clim. Dynam., 49, 419–431, https://doi.org/10.1007/s00382-016-3351-9, 2017.
Yang, D., Arblaster, J. M., Meehl, G. A., England, M. H., Lim, E.-P., Bates, S., and Rosenbloom, N.: Role of tropical variability in driving decadal shifts in the Southern Hemisphere summertime eddy-driven jet, J. Clim., 33, 5445–5463, https://doi.org/10.1175/JCLI-D-19-0604.1, 2020.
Yang, J., Liu, Q., Xie, S.-P., Liu, Z., and Wu, L.: Impact of the Indian Ocean SST basin mode on the Asian summer monsoon, Geophys. Res. Lett., 34, L02708, https://doi.org/10.1029/2006GL028571, 2007.
Yeh, T.-C., Dao, S.-Y., and Li, M.-T. (Eds.): The abrupt change of circulation over the Northern Hemisphere during June and October, The Atmosphere and the Sea in Motion, Rockefeller Institute Press and Oxford University Press, New York, 249–267, ISBN 9780874700336, 1959.
Ying, K., Jiang, D., Zheng, X., Frederiksen, C. S., Peng, J., Zhao, T., and Zhong, L.: Seasonal predictable source of the East Asian summer monsoon rainfall in addition to the ENSO–AO, Clim. Dynam., 60, 2459–2480, https://doi.org/10.1007/s00382-022-06461-4, 2023.
You, Y. and Jia, X.: Interannual variations and prediction of spring precipitation over China, J. Clim., 31, 655–670, https://doi.org/10.1175/JCLI-D-17-0233.1, 2018.
Zeng, J. and Zhang, Q.: A humidity index for the summer monsoon transition zone in East Asia, Clim. Dynam., 53, 5511–5527, https://doi.org/10.1007/s00382-019-04876-0, 2019.
Zhang, L., Han, W., Karnauskas, K. B., Meehl, G. A., Hu, A., Rosenbloom, N., and Shinoda, T.: Indian Ocean warming trend reduces Pacific warming response to anthropogenic greenhouse gases: An interbasin thermostat mechanism, Geophys. Res. Lett., 46, 10882–10890, https://doi.org/10.1029/2019GL084088, 2019.
Zhang, Z., Sun, X., and Yang, X.-Q.: Understanding the interdecadal variability of East Asian summer monsoon precipitation: Joint influence of three oceanic signals, J. Clim., 31, 5485–5506, https://doi.org/10.1175/jcli-d-17-0657.1, 2018.
Zhao, W., Chen, S., Chen, W., Yao, S., Nath, D., and Yu, B.: Interannual variations of the rainy season withdrawal of the monsoon transitional zone in China, Clim. Dynam., 53, 2031–2046, https://doi.org/10.1007/s00382-019-04762-9, 2019a.
Zhao, W., Chen, W., Chen, S., Yao, S.-L., and Nath, D.: Inter-annual variations of precipitation over the monsoon transitional zone in China during August–September: Role of sea surface temperature anomalies over the tropical Pacific and North Atlantic, Atmos. Sci. Lett., 20, e872, https://doi.org/10.1002/asl.872, 2019b.
Zhao, W., Chen, W., Chen, S., Nath, D., and Wang, L.: Interdecadal change in the impact of North Atlantic SST on August rainfall over the monsoon transitional belt in China around the late 1990s, Theor. Appl. Climatol., 140, 503–516, https://doi.org/10.1007/s00704-020-03102-w, 2020.