the Creative Commons Attribution 4.0 License.
the Creative Commons Attribution 4.0 License.
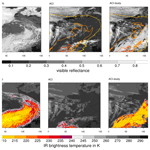
Aerosol–cloud–radiation interaction during Saharan dust episodes: the dusty cirrus puzzle
Vanessa Bachmann
Florian Filipitsch
Jochen Förstner
Christian M. Grams
Gholam Ali Hoshyaripour
Julian Quinting
Anika Rohde
Heike Vogel
Annette Wagner
Bernhard Vogel
Dusty cirrus clouds are extended optically thick cirrocumulus decks that occur during strong mineral dust events. So far they have mostly been documented over Europe associated with dust-infused baroclinic storms. Since today's global numerical weather prediction models neither predict mineral dust distributions nor consider the interaction of dust with cloud microphysics, they cannot simulate this phenomenon. We postulate that the dusty cirrus forms through a mixing instability of moist clean air with drier dusty air. A corresponding sub-grid parameterization is suggested and tested in the ICOsahedral Nonhydrostatic model with Aerosol and Reactive Trace gases (ICON-ART). Only with the help of this parameterization is ICON-ART able to simulate the formation of the dusty cirrus, which leads to substantial improvements in cloud cover and radiative fluxes compared to simulations without this parameterization. A statistical evaluation over six Saharan dust events with and without observed dusty cirrus shows robust improvements in cloud and radiation scores. The ability to simulate dusty cirrus formation removes the linear dependency on mineral dust aerosol optical depth from the bias of the radiative fluxes. For the six Saharan dust episodes investigated in this study, the formation of dusty cirrus clouds is the dominant aerosol–cloud–radiation effect of mineral dust over Europe.
- Article
(13738 KB) -
Supplement
(6109 KB) - BibTeX
- EndNote
The term “dusty cirrus” is used by meteorologists, especially in Europe, for extended cirrus cloud decks that typically occur during strong Saharan dust episodes (Kollath, 2010; Roesli et al., 2020; Fierli et al., 2022). A characteristic property of this type of dusty cirrus is the cellular structure that hints at convective overturning within the cirrus cloud layer.
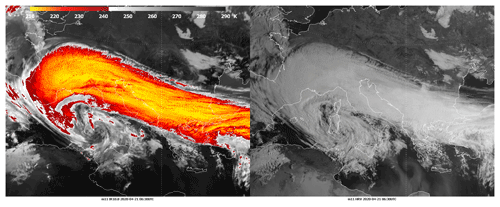
Figure 1Meteosat Second Generation (MSG) spinning enhanced visible and infrared imager (SEVIRI) infrared (IR) and high-resolution visible (HRV) of 21 April 2020, 06:30 UTC, with an extended dusty cirrus cloud deck over central Europe (from Roesli et al., 2020, with permission from EUMETSAT).
Dusty cirrus decks are associated with dust-infused baroclinic storms (DIBS; Fromm et al., 2016), which are far-equatorward-reaching midlatitude cyclones that transport huge amounts of mineral dust from Africa to Europe. Due to the strong ascending motions in these baroclinic storms, the mineral dust can reach the upper troposphere and affect, or even cause, the formation of cirrus clouds (Ansmann et al., 2019). However, not all DIBS produce extended dusty cirrus cloud decks. It has been hypothesized that these extended dusty cirrus decks form through longwave cooling at an elevated dust layer. The longwave cooling leads to destabilization in the upper troposphere and a subsequent formation of a shallow convective cirrus cloud deck (Nagy, 2009; Kollath, 2010). This hypothesis is largely based on the cellular structure of the cirrus cloud, which is visible in high-resolution satellite images (Fig. 1). The basic mechanism is also supported by idealized numerical simulations (Fusina and Spichtinger, 2010; Spichtinger, 2014). Previous studies of cirrus clouds associated with dust events in Europe (Rieger et al., 2017; Weger et al., 2018) or Asia (Wang et al., 2015; Caffrey et al., 2018; Pan et al., 2019) have not focused on the special characteristics of the dusty cirrus. Dusty cirrus clouds with extended cloud decks are rare. In Europe, roughly one event per year is observed.
Dusty cirrus clouds pose a challenge for numerical weather prediction (NWP) and climate models. Today's global NWP models are in general unable to predict these dust-induced clouds. This is not surprising, as operational NWP systems do currently not explicitly predict mineral dust but employ an aerosol climatology to take into account the average effect of mineral dust and other aerosols on radiation and cloud formation. Hence, operational NWP systems know next to nothing about the actual distribution of mineral dust in the atmosphere, especially during episodes. Global aerosol and chemistry forecasting models, like CAMS (Morcrette et al., 2009; Rémy et al., 2019) or GEOS-5 (Nowottnick et al., 2011; Bunn et al., 2020) on the other hand, do predict mineral dust, but in such models, the dust is usually not explicitly coupled to cloud formation; i.e., aerosol–cloud interaction (ACI) is not taken into account. Climate models suffer from very coarse grid spacing, and the formation mechanisms of cirrus clouds and the aerosol–cloud interaction are therefore highly parameterized (Kuebbeler et al., 2014; Wang et al., 2014; Penner et al., 2018). A recent study suggests that climate models underestimate the aerosol–cloud interaction for cirrus clouds (Maciel et al., 2022). Besides the effect on ice nucleation and cirrus formation, mineral dust can modify clouds and rainfall by acting as cloud condensation nuclei, (e.g., Hui et al., 2008) and through a modulation of synoptic-scale and mesoscale atmospheric circulations (e.g., Jin et al., 2021; Parajuli et al., 2022).
Not being able to predict dust-induced cirrus formation can lead to large forecast errors for the solar irradiance at the surface, even in day-ahead forecasts. The erroneous forecasts can subsequently lead to an overestimation in the prediction of photovoltaic (PV) power generation (e.g., Rieger et al., 2017). With the rising relevance of PV for the energy supply in Europe and worldwide, this poses significant challenges for system operators in the energy market and the management of the power grid itself (Antonanzas et al., 2016).
Therefore, it is of high relevance to improve the NWP systems and enable them to predict these rare but important dusty cirrus cloud decks. In the following, we present a new parameterization for dusty cirrus in the model system ICOsahedral Nonhydrostatic model with Aerosol and Reactive Trace gases (ICON-ART), which is based on the hypothesis of a mixing instability between moist clean air with the drier Saharan dust layer. We show that the combination of explicitly predicting mineral dust with a state-of-the-art aerosol model and our new parameterization of aerosol–cloud effects leads to skillful simulations of dusty cirrus. The ICON-ART system is then evaluated for several Saharan dust events with and without dusty cirrus occurrence.
In Sect. 2, we introduce the ICON-ART model and the dusty cirrus parameterization, which augments the cloud scheme in the ICON model. Section 3 is dedicated to an analysis of three dusty cirrus cases, and a statistical analysis of six Saharan dust cases with and without dusty cirrus is presented. We end with a summary and conclusions.
2.1 ICON, ICON-ART, and ICON-D2-ART
ICON is a non-hydrostatic compressible atmospheric model, which uses a triangular icosahedral mesh (Zängl et al., 2015). ICON is developed and maintained jointly by the Deutscher Wetterdienst (DWD), the Max Planck Institute for Meteorology (MPI-M), the German Climate Computing Center (DKRZ), and the Karlsruhe Institute of Technology (KIT). The operational NWP system at DWD consists of a global ICON model, currently at 13 km grid spacing, with a European two-way nest at 6.5 km grid spacing (called ICON-EU), and the regional ICON-D2 with approximately 2 km grid spacing over central Europe (Reinert et al., 2022). ART is a component of ICON that enables treatment of atmospheric chemistry and aerosols (Rieger et al., 2015; Schröter et al., 2018). In the following, we apply ICON-ART in a regional configuration similar to the operational ICON-D2, which we call ICON-D2-ART. The model domain has 542 040 cells in each of the 65 model levels. ICON uses a vertically stretched grid. For ICON-D2 the vertical grid spacing in the lowest levels is smaller than 100 m, but near the tropopause it is approximately 500 m (see Reinert et al., 2022, p. 121). The domain top is at 22 km height. In this study, we use ART only to simulate mineral dust. The aerosol model uses a modal distribution with a two-moment formulation (Rieger et al., 2017; Gasch et al., 2017; Hoshyaripour et al., 2019). Mineral dust is represented by three log-normal modes with standard deviations of 1.7, 1.6, and 1.5 for modes dustA, dustB, and dustC, respectively. While the standard deviations are kept constant, the median diameters are variable depending on the simulated mass and number concentration. Chemical aging of dust is not taken into account in this study, although ICON-ART is in principle able to treat coated aerosol particles (Muser et al., 2020). DWD and KIT maintain a pre-operational global dust forecasting system based on ICON-ART with a grid of approximately 40 km with 90 vertical levels and a two-way nest with 20 km grid spacing (ICON-EU-ART). In this study we use boundary conditions for ICON-D2-ART from the ICON-EU-ART nest of the analysis cycle of this global mineral dust forecasting system.
The model physics of ICON-D2-ART is largely based on the physical parameterizations of COSMO-DE as described in Baldauf et al. (2011), but in ICON-D2-ART the two-moment mixed-phased cloud microphysics of Seifert and Beheng (2006) as described in Seifert et al. (2012) is used. The most important recent change to the two-moment microphysics is that the PDA08 ice nucleation scheme (Phillips et al., 2008) has been replaced with a parameterization of the ice nucleation active surface site (INAS) density (Ullrich et al., 2017). The INAS approach greatly simplifies the coupling of the ice microphysics to the predicted mineral dust modes because only the total surface area of dust is needed as an input to the ice nucleation parameterization in addition to the supersaturation. In the setup of ICON-D2-ART used in the current study, mineral dust is not depleted by ice nucleation. Therefore we use an additional tracer variable to track activated ice nuclei as described in Köhler and Seifert (2015) to avoid an overestimation of heterogenous ice nucleation. Homogeneous ice nucleation of liquid aerosols is parameterized based on Kärcher et al. (2006).
Radiative fluxes in ICON are calculated using the ecRad radiation scheme (Hogan and Bozzo, 2016, 2018; Rieger et al., 2019). ICON makes use of the Tegen et al. (1997) aerosol climatology. In the present study, ICON-D2-ART with prognostic mineral dust applies the monthly climatological values for the sulfate, organic and black carbon, and sea salt aerosol modes. Furthermore, we have reduced the sulfate, organic carbon, and black carbon aerosol optical depth compared to the original Tegen climatology to take into account the reduction in anthropogenic aerosol sources in Europe in the last few decades. For the prognostic dust, the radiative transfer parameters are calculated online depending on the optical properties and the size distributions (Hoshyaripour et al., 2019). For resolved clouds the effective radius is calculated consistent with the microphysical assumptions of the two-moment scheme. Ice optical properties of Fu (1996) are used. For effective radii larger than 100 µm, which is outside the tables currently provided by ecRad, the effective radius is rescaled with the assumption const., where qi is the ice mass fraction and r the ice effective radius. As an offline diagnostic the RTTOV forward operator (Saunders et al., 2018) is used to simulate Meteosat Second Generation (MSG) observations of the spinning enhanced visible and infrared imager (SEVIRI). RTTOV is called with model-consistent cloud information including the effective radii from the two-moment microphysics. For the visible channel at 0.6 µm, the method for fast satellite image synthesis (MFASIS) operator (Scheck et al., 2018; Geiss et al., 2021) is applied as part of RTTOV.
ICON does come with a diagnostic sub-grid cloud-cover scheme, which is similar in spirit to the schemes of Smith (1990) and Le Trent and Li (1991), and provides cloud fraction and sub-grid liquid and ice water content for the radiation calculation. This sub-grid cloud-cover scheme is usually not explicitly coupled to the aerosol information provided by ICON-ART; i.e., aerosol–cloud interaction happens only on the grid scale but is neglected for sub-grid clouds. A parameterization, which changes this, is described in the following.
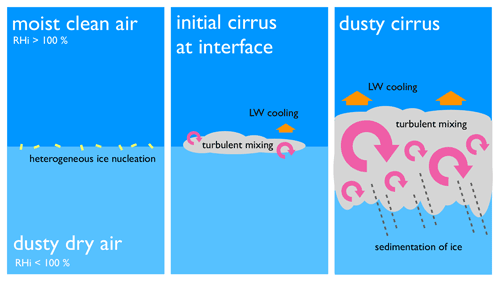
Figure 2Conceptual model summarizing the physical processes that lead to the formation of the dusty cirrus at the interface between a dry Saharan dust layer and a moist atmospheric layer above. The conceptual model assumes cold environmental conditions typical for the upper troposphere and near-neutral to weakly stable stratification.
2.2 A sub-grid parameterization of dusty cirrus
The sub-grid parameterization of dusty cirrus is based on the concept of a fundamental mixing instability of cold moist clean air with a drier air mass containing ice-nucleating particles (INPs). During the Saharan dust events, and especially as part of a dust-infused baroclinic storm (DIBS), the dynamical lifting can lead to a stratification with moist clean air in the upper troposphere located above drier dusty air below. The clean moist air has very few INPs, whereas the dusty air has INPs but lacks the moisture to form a cloud. At the interface between the two layers, heterogeneous ice nucleation can occur if the air mass has cooled to a sufficiently low temperature. This can be achieved, e.g., through lifting of the air layers by ascending motion underneath (Wernli et al., 2016). Small amounts of mineral dust, which are mixed into the moist air above the rather dry dust-carrying layer, can initiate the formation of an ice cloud at the interface between the two layers (see Fig. 2). Longwave cooling at this thin cirrus layer can further destabilize the layer and leads to turbulent mixing. This will entrain more dust from below into the moist layer and further thicken the cirrus cloud layer. At some point, the convective overturning becomes strong enough that even homogeneous nucleation may become relevant Spichtinger (e.g., 2014). We favor the hypothesis of a mixing instability driven by heterogeneous nucleation over the original hypothesis of longwave cooling at the dust layer postulated earlier (Nagy, 2009; Kollath, 2010) because it explains more naturally why the dusty cirrus cloud forms above rather than in the Saharan dust layer. Longwave cooling at the dust layer could play a role in the formation of the very first cirrus cloud at the moist–dusty interface, but on the other hand, the moist layer above reduces the longwave effect of the dust below. The longwave cooling at the dusty layer can contribute to the destabilization of the background profile, but lifting of the air masses, e.g., in a warm conveyor belt, leads by itself to a near-neutral or at least weakly stable stratification in the upper troposphere (e.g., Gierens et al., 2022). Given a weakly stable stratification, the longwave cooling at the thin ice cloud that forms at the interface between the moist clean air and the drier dusty air is sufficient to further destabilize the layer and will eventually lead to the formation of the dusty cirrus deck. Hence, it remains unclear whether the radiative effect of the dust is important in this process. In our hypothesis, the main role of the mineral dust is to provide INPs for the cloud formation, whereas the cloud itself causes the longwave cooling that leads to the turbulent mixing of the layers and the development of the shallow convective cirrus.
To characterize the amount of dust in the Saharan dust layer, we use the mass concentration of mineral dust cmode with mode∈{dustA, dustB, dustC}. That sufficient amounts of mineral dust reach the upper troposphere is the most important predictor in our parameterization. The moisture is quantified by the ice saturation ratio , where pv is the vapor pressure, and psat,ice is the saturation vapor pressure over ice. As a measure of atmospheric stability we use the temperature lapse rate
Note that ICON uses top-down indices; i.e., level k+1 is below level k. Dusty cirrus occurs in model level k if the following conditions are fulfilled:
with empirically determined thresholds = 50 µg kg−3, = 0.7, and γ∗ = −6.5 K km−1 and with N=4 corresponding to a vertical depth of approximately 1500 m. The non-locality of this parameterization corresponds to the convective overturning and the mixing instability described above.
Table 1Overview of ICON-D2-ART simulation periods. The initial condition is at 00:00 UTC on the initial date. The table gives the maximum mineral dust aerosol optical depth (AOD) of ICON-D2-ART and an estimate of the maximum height of the dusty layer as observed by German ceilometer networks. The occurrence of dusty cirrus is estimated based on satellite data.
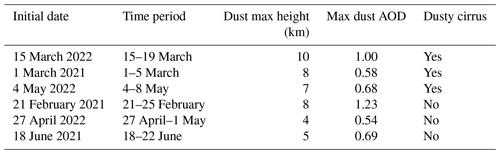
Note that does not include the smallest dust mode dustA, and the largest mode dustC has double the weight of dustB. This choice helps to avoid false alarms in the prediction of the dusty cirrus. The fact that the larger dust modes, dustB and dustC, are better predictors for the occurrence of a dusty cirrus than dustA is consistent with the increased ability of large mineral dust particles to act as INPs, whereas smaller particles are less relevant for the formation of ice clouds by heterogeneous nucleation (DeMott et al., 2010, 2015).
The ice saturation threshold is rather low with . We assume that the process starts at scales considerably smaller than the resolved scales of the model. Fluctuation due to gravity waves or shear-induced clear-air turbulence can locally initiate the cirrus cloud formation at the air mass interface. Once an initial cirrus layer has formed, this triggers the microphysical mixing instability as described above.
The dusty cirrus is further characterized by a cloud fraction of 1, C=1; a maximum ice water content of = 50 mg m−3; and an ice particle number density of 500 × 10−3 m−3. For the ice water content of the dusty cirrus in a model level k we apply a linear tapering with
with µg kg−3 and .
According to our hypothesis and consistent with observations, convective overturning on scales smaller than 10 km is a crucial ingredient for the formation of the dusty cirrus cloud deck. Given the horizontal grid spacing of 2 km, with an effective resolution larger than 10 km, and a vertical grid spacing of approximately 500 m in the upper troposphere, it is reasonable that ICON-D2-ART is not able to explicitly simulate the chain of processes that leads to the formation of the dusty cirrus. It seems therefore appropriate to describe the dusty cirrus by a parameterization as part of the sub-grid cloud scheme as formulated above.
In this study, six Saharan dust episodes over Europe are evaluated. Each simulation spans a time period of 5 d and is initialized from the analysis cycle of the global dust forecasting system of DWD, which also provides the lateral boundary conditions. The three dust episodes without dusty cirrus formation were selected such that they have a similar dust intensity as the dusty cirrus episodes (Table 1). The non-dusty cirrus episodes have on average shallower dust layers. A list of dust episodes of the years 2021 and 2022 is provided in the Supplement.
For each dust episode, an ICON-D2 simulation without prognostic dust acts as a control run mimicking the behavior of a standard operational NWP system (“control”). In addition, three ICON-D2-ART simulations are discussed: first, a simulation with only aerosol–radiation interaction (ARI); second, a simulation with ARI and grid-scale aerosol–cloud interaction (ACI) based on the two-moment microphysics scheme; and third, a simulation with aerosol–radiation interaction, grid-scale aerosol–cloud interaction, and the sub-grid dusty cirrus parameterization (ACI–dusty). The simulation periods are summarized in Table 1, and the differences between the simulations are detailed in Table 2. In the following we discuss the three dusty cirrus events.
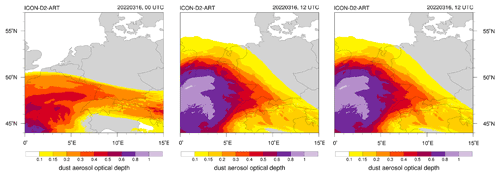
Figure 3ICON-D2-ART mineral dust aerosol optical depth (AOD) on 16 March 2022, 00:00 and 12:00 UTC, and 17 March 00:00 UTC.
3.1 Dusty cirrus case of 15–19 March 2022
On 15 March 2022, 00:00 UTC, the center of storm Celia is located west of the Strait of Gibraltar. In the mid- to upper-troposphere it is associated with a deep trough that reaches equatorward as far as the western Sahara. Located south of a quasi-stationary anticyclone over Europe, the trough decouples from the westerly flow and forms a cutoff on 16 March 2022. On the eastern flank of the trough in a region of quasi-geostrophic forcing for ascent, large amounts of Saharan dust are transported poleward towards Spain and lifted from the lower into the mid to upper troposphere. Over the next 2 d, the dusty air mass moves further eastward over France and Germany with a dust aerosol optical depth (AOD) exceeding 0.8 in the ICON-D2-ART simulation (Fig. 3).
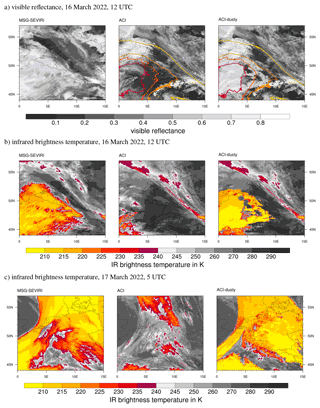
Figure 4MSG–SEVIRI visible reflectance (a) and infrared brightness temperature of the 10.8 µm channel (b) vs. ICON-D2-ART on 16 March 2022, 12:00 UTC, and infrared brightness temperature on 17 March 2022, 05:00 UTC (c). Shown are MSG–SEVIRI (left) vs. ICON-D2-ART ACI (center) and ACI–dusty (right) simulations. The isolines in (a) are the mineral dust AODs predicted by ICON-D2-ART (interval of 0.2 starting at 0.1).
The dusty air mass is associated with an extended cirrus cloud deck, which covers most of France and Switzerland on 16 March, 12:00 UTC, as can be seen in the Meteosat SEVIRI visible image shown in Fig. 4a (left). The infrared brightness temperature from SEVIRI reaches 215 K over large parts of the cirrus cloud deck (Fig. 4b; left). The ACI simulation of ICON-D2-ART shows clear-sky conditions over most of France and Switzerland and mid-level clouds with brightness temperatures larger than 240 K in the northern part of France (Fig. 4a and b; center). This simulation fails to simulate the cirrus associated with the Saharan dust, although the simulated dust AOD matches the spatial extension of the cirrus cloud. This changes with the sub-grid dusty cirrus parameterization in the ACI–dusty simulation, which enables ICON-D2-ART to simulate the cirrus cloud deck that is associated with the Saharan dust (Fig. 4a; right). The infrared brightness temperature is somewhat too low for 16 March, 12:00 UTC, but the spatial extent agrees well with the SEVIRI observations (Fig. 4b; right cf. left). On 17 March, 00:00 UTC, when cirrus clouds extend over the Netherlands, the North Sea, Denmark, and most of Germany, we find a good agreement between SEVIRI and the ACI–dusty simulation (Fig. 4c). The purely grid-scale ACI simulation is not able to simulate the cirrus clouds and shows only low- and mid-level clouds.
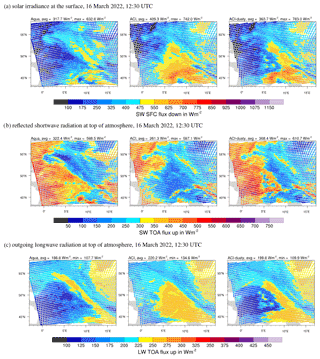
Figure 5CERES SSF radiative fluxes from Aqua (left) vs. ICON-D2-ART ACI (center) and ACI–dusty (right) simulations for 16 March 2022, 12:30 UTC.
An overpass of the Aqua satellite operated by NASA provides CERES radiative flux data for 16 March at 12:30 UTC as shown in Fig. 5. Throughout this study the CERES SSF level-2 edition 4A instantaneous fluxes are used (Su et al., 2015a, b; Kratz et al., 2020). The cirrus cloud deck over France leads to reduced outgoing longwave flux and a large reflected shortwave flux at the top of the atmosphere (TOA). The solar irradiance at the surface is greatly reduced to values below 200 W m−2 under the cirrus cloud deck. Consequently, the ACI simulation without the cirrus cloud deck overestimates the shortwave surface flux and the outgoing longwave radiation (OLR) and underestimates the reflected shortwave at TOA. The errors in the solar irradiance exceed a factor of 2 over large areas in the ACI simulation. In the ACI–dusty simulation with the sub-grid dusty cirrus scheme, the cirrus cloud deck is properly represented, and all three radiative fluxes are reasonably consistent with the CERES observations.
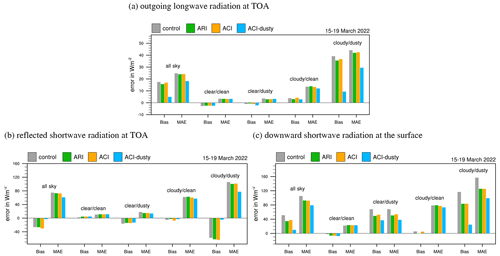
Figure 6Bias and mean absolute error (MAE) of ICON-D2-ART compared to CERES SSF level-2 radiative fluxes for 15–19 March 2022. Shown are the outgoing longwave radiation at the top of the atmosphere (TOA), the reflected shortwave at TOA, and the solar irradiance at the surface (from the top to bottom). Clear sky is defined as a cloud cover smaller than 5 %, cloudy conditions are defined by a cloud cover larger than 5 %, dusty conditions are defined by a dust AOD larger than 0.1, and clean conditions are defined by an AOD smaller than 0.1 (see Sect. 3.1 for details).
To quantify the errors in the radiative fluxes, we make use of all CERES data from Aqua and Terra overpasses over the ICON-D2-ART domain during the 5 d period. These are a total of 35 overpasses but only 17 during daytime with non-zero shortwave data. The CERES data are processed in 20 min time windows with radiative flux output from ICON-D2-ART every 20 min. Based on this data, the biases and mean absolute errors (MAEs) of the ICON-D2-ART simulations can be calculated using each single CERES SSF footprint of 25 km diameter. For each CERES footprint, 64 ICON triangles around its center are averaged. Here and in the following the bias is identical to the mean error (ME). Figure 6 shows the result stratified by clear-sky/clean, clear-sky/dusty, cloudy/clean, and cloudy/dusty conditions. Clear sky is defined as both observation and model, having a cloud cover smaller than 5 %. As observed cloud cover, the MODIS cloud cover as provided with the CERES SSF data is used. Clean and dusty pixels are defined solely based on ICON-D2-ART because MODIS does not provide an AOD for cloudy pixels. The dust AOD threshold for dusty pixels is set to 0.1. Due to the explicit representation of the direct aerosol–radiation effect, we would expect that all three ICON-D2-ART simulations with prognostic mineral dust can improve the radiative fluxes in clear-sky/dusty conditions but show little changes in the clear-sky/clean situations. This is confirmed for the shortwave surface flux in Fig. 6c, but only a minor improvement is seen in the TOA fluxes. For cloudy/dusty pixels the ARI and ACI simulations show an improvement over the control run, but this is most likely also due to the direct aerosol effect because no significant difference exists between ARI and ACI. In fact, ACI with grid-scale aerosol–cloud interaction is slightly worse than the ARI simulation. The dusty cirrus parameterization used in the ACI–dusty simulation greatly improves all three radiative fluxes for cloudy/dusty pixels and also in the all-sky statistics. Especially the biases are dramatically reduced for ACI–dusty. The MAE is for all simulations rather high in cloudy situations because it suffers from a double-penalty problem and the fact that the clouds are far from perfect on small scales, but the reduction in MAE in the ACI–dusty simulation is nevertheless a great improvement over the other three simulations.
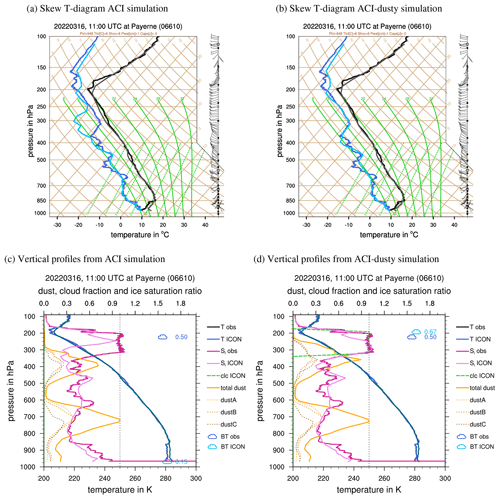
Figure 7Vertical profiles from radiosonde measurements at Payerne (Switzerland) on 16 March 2022, 11:00 UTC, and the corresponding ICON-D2-ART profiles. Skew-T log-p diagrams (a, b) showing the temperature and dew point temperature from observations (black and dark blue) and ICON-D2-ART (grey and light blue). Lower plots (c, d) show the temperature from observations (dark blue) and ICON-D2-ART (blue), ice saturation ratio (obs: dark pink, model: light pink), and total dust concentration (solid orange) and dust modes (dashed; dustA: light orange, dustB: orange, dustC: dark orange). Dust concentrations are normalized with a constant value of 100 µg m−3. Cloud symbols indicate pressure height where the IR brightness temperature (SEVIRI: dark blue, ICON-D2-ART: light blue) matches the temperature of the sounding; numbers next to it indicate visible reflectance. The dashed green line is the cloud fraction predicted by ICON-D2-ART.
To gain some understanding of the vertical structure of this dusty cirrus event, Fig. 7 shows a comparison of the radiosonde measurement at Payerne, Switzerland, on 16 March, 12:00 UTC, with the corresponding profiles from ICON-D2-ART. The skew-T log-p diagram for the ACI simulation (Fig. 7a) shows a good agreement for the temperature profile and also for dew point temperature, except for a layer between 250 and 375 hPa in which the dew point temperature of the ACI simulation is 10 K too low. Initially, we thought that the lack of moisture in the layer, in which the dusty cirrus is observed, is the reason that the ACI simulation fails to predict the cirrus cloud deck. All attempts to track down this error, for example, in the initial or boundary conditions, proved to be futile, though. Interestingly, the ACI–dusty simulation corrects this error in the dew point temperature and shows a good agreement for the whole profile. This is even more remarkable as the dusty cirrus scheme itself does not directly modify the (grid-scale) moisture profile. As described in the previous section, it is a sub-grid cloud scheme that affects only the radiative fluxes. Hence, the only possible explanation is that the longwave cooling at the parameterized sub-grid dusty cirrus changes the dynamics and the moisture transport in the model in such a way that it becomes much more consistent with the observations.
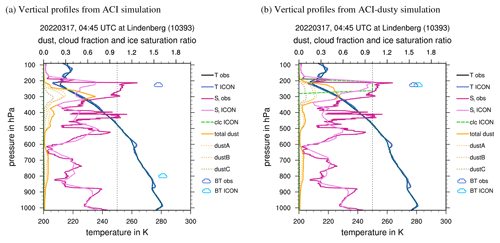
Figure 8As Fig. 7c and d but for radiosonde measurements at Lindenberg (Germany) on 17 March 2022, 05:00 UTC, and the corresponding ICON-D2-ART profiles.
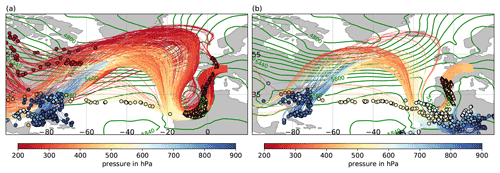
Figure 9The 5 d backward trajectories representing (a) the moist layer and (b) the dust layer in the Payerne region on 16 March, 12:00 UTC. Trajectories, air mass locations at −120 h (circles), and air mass locations at −24 h (triangles) are colored according to their pressure height (see color bar). Green contours show 500 hPa geopotential height in gpm at −24 h, i.e., on 15 March, 12:00 UTC.
Another set of variables for the same sounding at Payerne is shown in Fig. 7c and d. Here we focus on the vertical profiles of the ice saturation ratio and the dust concentration. Although standard radiosondes do not measure cloudiness or ice water content, the ice saturation ratio allows us to identify the location of the dusty cirrus in the observations. Due to the high IWC, the dusty cirrus layer is close to ice saturation, i.e., sice=1. The ACI simulation underestimates the ice saturation ratio in the dusty cirrus layer and is consequently not able to simulate any cloud formation. The sub-grid dusty cirrus parameterization represents the dusty cirrus as a cloud cover of 1 (dashed green line in Fig. 7d). To make the connection with the SEVIRI data, the infrared brightness temperature from SEVIRI and the RTTOV forward calculation is depicted by a small blue cloud symbol (darker colors represent the observations). For optically thick clouds this can be identified with the cloud-top pressure. First, this sounding confirms the concept of moist air located over drier dusty air; i.e., the dusty cirrus initially forms above, not in the dust layer. Second, it shows that the dusty cirrus layer of ACI–dusty is consistent with the observed profile, and the cloud top is consistent with SEVIRI. Third, it is remarkable that the simulated ice saturation ratio increases and reaches almost 1 in the dusty cirrus layer, although the dusty cirrus parameterization does not directly change the water vapor. That the increase in moisture has dynamical causes is consistent with the fact that the dust profiles change significantly as well when the dusty cirrus parameterization is employed. Compared to the ACI simulation the ACI–dusty case has a stronger vertical transport of mineral dust, and the dust mixes more efficiently with the moist layers above. This is consistent with our conceptual model but was not necessarily expected from this very simple sub-grid parameterization that does not explicitly modify the vertical transport of dust or moisture.
This behavior is also confirmed by the radiosonde profile at Lindenberg (Germany) from 17 March, 05:00 UTC (Fig. 8). The observed sounding shows as a cirrus cloud layer between 200 and 300 hPa at ice saturation, similar to the Payerne sounding. The maximum of the dust layer is here at 300 hPa and no longer separated from the cloud layer. Hence, an observation like this could be interpreted as if the cloud has formed in the dust layer. The comparison with the earlier sounding at Payerne suggests, though, that the Lindenberg sounding is characteristic of the mature state of the dusty cirrus when the mixing of the moist layer and the dusty layer has already happened.
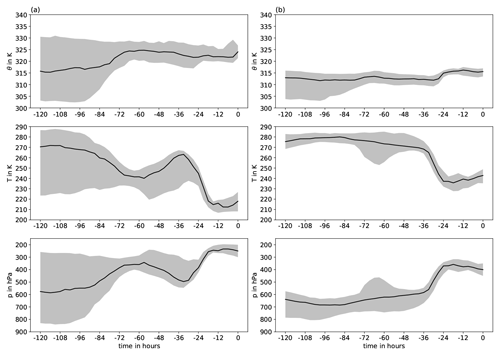
Figure 10Temporal evolution of potential temperature (TH), temperature (T), and pressure (p) along backward trajectories representing (a) the moist layer and (b) the dust layer in the Payerne region on 16 March, 12:00 UTC. The line shows the median of all trajectories, and shading denotes the interquartile range.
To substantiate the concept of moist clean air above drier dusty air prior to the dusty cirrus formation, we calculate 5 d kinematic backward trajectories with the Lagrangian analysis tool (Lagranto; Sprenger and Wernli, 2015). The underlying data are 3-hourly ERA5 reanalysis (Hersbach et al., 2020) on model levels and a regular 0.5∘ × 0.5∘ latitude–longitude grid. The backward trajectories are started from an equidistant grid of 25 km spacing surrounding Payerne (46–49.5∘ N, 6–10∘ E) and every 50 hPa between 450 and 200 hPa. The levels between 450 and 350 hPa represent the dust layer, and levels between 300 and 200 hPa represent the moist layer above (cf. Fig. 7c and d). The trajectory analysis reveals that the bulk of air parcels in the moist layer reaching Payerne on 16 March, 12:00 UTC, ascended almost 5 d earlier over the Caribbean and were transported eastward with the midlatitude jet (Fig. 9a). Humidity in this layer was anomalously high, reflected by values of specific humidity in the upper 10 % of the climatological distribution in the month of March based on ERA5 (not shown). The characteristic U-shape of the trajectories over the eastern North Atlantic shows their transport around the upper-level trough associated with storm Celia. As already indicated in the synoptic description, the air masses were lifted from 450 to 250 hPa ahead of the upper-level trough 36 to 18 h (between 15 March, 00:00 to 18:00 UTC) before arriving over Payerne (Fig. 10a and triangles in Fig. 9a showing air parcel locations 24 h prior to arriving over Payerne). We note that this lifting of the moist layer also occurred spatially in the region of initial dusty cirrus formation over France. Since the potential temperature remained nearly constant during this ascent, we conclude that the lifting was mostly dry adiabatic and – as discussed in the following – initiated through the injection of the dusty layer underneath via an ascending air stream. Concerning the dry dusty layer, the majority of air parcels ascended from the lower troposphere over North Africa during the 5 d period (Fig. 9b). Several air parcels start below 800 hPa and reach above 400 hPa a few days later, reflecting ascent in a warm conveyor belt (WCB; Wernli, 1997) in the warm sector of the forming cyclone. Only a smaller fraction, probably reminiscent of the moist layer, already ascended over the Caribbean. About 36 to 24 h prior to reaching the region of Payerne, the air parcels ascended on average from 600 to above 400 hPa, accompanied by latent heat release, reflected by an increase in potential temperature (Fig. 10b). This coincides with the lifting of the moist layer above and suggests that WCB ascent also lifted the air column above, corroborating the notion of two distinct air layers and the formation of a cirrus cloud deck through lifting and thereby adiabatic cooling and the injection of ice-nucleating particles into the moist layer (see Wernli et al., 2016). Our main conclusion is therefore that the lifting of an anomalously upper-tropospheric moist air layer originating from the Caribbean through a synoptic-scale ascending WCB airstream underneath emerging from the Sahara and the associated injection of dust particles as ice-nucleating particles at the interface of the moist and dusty layers have created the conditions for the formation of the dusty cirrus cloud deck. Although a climatological investigation is beyond the scope of this study, we hypothesize that the complex interaction of the various components (upper-level moist layer, synoptic forcing, dust transport in WCB) makes dusty cirrus events so rare.
3.2 Dusty cirrus case of 1–5 March 2021
On 2–3 March 2021, the synoptic situation is similar to the case of 16 March 2022, with a trough in 500 hPa extending from the British Isles over Spain to Morocco. The southerly flow at the eastward flank of the trough advects mineral dust directly from the Saharan desert and North Africa to Europe. The event is not as massive as the 16 March 2022 event, but dust AODs simulated by ICON-D2-ART do exceed 0.5 over southern France on 3 March 2021, 12:00 UTC (Fig. 11), and high dust AOD extends along a northeast line into Switzerland and southern Germany.
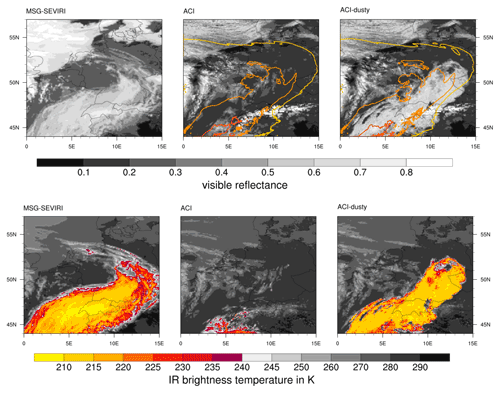
Figure 12MSG–SEVIRI visible reflectance (top) and infrared brightness temperature of the 10.8 µm channel (center) vs. ICON-D2-ART on 3 March 2021, 12:00 UTC. The isolines are the mineral dust AODs predicted by ICON-D2-ART (interval of 0.2 starting at 0.1).
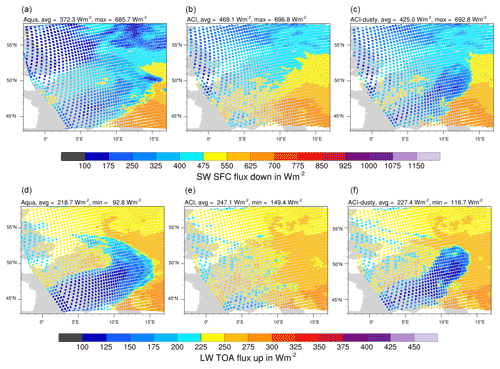
Figure 13CERES SSF outgoing longwave radiation (a–c) and solar irradiance at the surface (d–f) from Aqua and ICON-D2-ART for 3 March 2021, 11:55 UTC.
The MSG–SEVIRI images for 3 March 2021, 12:00 UTC, show a cirrus cloud band associated with the Saharan dust event, which agrees well with the simulated dust plume of ICON-D2-ART (Fig. 12). As in the previous case, the ACI simulation fails to predict the dusty cirrus and shows clear-sky conditions instead (including the snow-covered Alps). The ACI–dusty simulation does have the cirrus cloud band, but the boundaries of the cloud deck are too sharp, and the infrared brightness temperature is too homogeneous compared to the SEVIRI observations. These are probably deficiencies of the simple diagnostic parameterization that can not capture any transient behavior during the formation and decay of the dusty cirrus. Nevertheless, the sub-grid parameterization clearly improves the simulation of this dusty cirrus event by ICON-D2-ART. These improvements are also seen in the comparison to the CERES SSF data from the Aqua overpass of 3 March 2022, 11:55 UTC (Fig. 13). Whereas the ACI simulation completely misses the radiative signature of the dusty cirrus cloud deck, the ACI–dusty matches the CERES data remarkably well (the shortwave TOA flux is shown in Fig. S12 in the Supplement). For the broadband fluxes the tapering of the IWC of Eq. (6) seems to work better than for the RTTOV forward simulation of the MSG–SEVIRI channels. Both simulations have problems to properly represent the low clouds over the North Sea and England, but this is most likely not related to Saharan dust. For the validation of the vertical distribution of cloud occurrence, we can also make use of ceilometer data for this event. Results show a significant improvement of the modeled vertical cloud fraction for the ACI–dusty simulation compared to the other simulations (see Supplement and Fig. S3 for details).
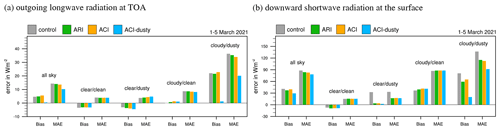
Figure 14Bias and mean absolute error (MAE) of ICON-D2-ART compared to CERES SSF level-2 radiative fluxes for 1–5 March 2021. Shown are the outgoing longwave radiation at the top of the atmosphere (a) and the solar irradiance at the surface (b).
The good agreement of ACI–dusty with the CERES SSF fluxes is also confirmed by the scores for all Aqua and Terra overpasses from 1–5 March 2021 shown in Fig. 14. For this time period all three ICON-D2-ART simulations with prognostic dust show a clear improvement in bias and MAE of the shortwave flux at the surface for clear-sky/dusty pixels (for scores of reflected shortwave at TOA see Fig. S5). For cloudy/dusty pixels the ACI–dusty simulation drastically improves the representation of the radiative fluxes. For all-sky conditions the bias of the outgoing longwave radiation is reduced almost to 0 for ACI–dusty, but significant biases remain for the shortwave fluxes due to issues with low clouds in ICON during this period. Validation of the case with radiosondes shows very similar results as in the case of 15–20 March 2022 (Figs. S14–S16).
3.3 Dusty cirrus case of 4–8 May 2022
The Saharan dust case of 4–8 May 2022 differs from the two previous cases in that the dust transport is more to the east, and dust is advected from North Africa to Italy and the larger Alpine region. In the ICON-D2-ART domain, only the southeastern part is affected by high dust AOD, which is rather persistent over several days (Fig. 15). The dust event is associated with extended cirrus clouds, but in this case, all four simulations do have some cirrus cloud coverage over the Alps. In ACI–dusty the cirrus clouds are thicker and have a lower IR brightness temperature compared to ACI (Fig. 16). Compared to the SEVIRI observations ACI–dusty significantly overestimates the cirrus cloud deck, and ACI underestimates the spatial extent but matches the IR brightness temperature better than ACI–dusty. Hence, in this case, the dusty cirrus parameterization intensifies the cirrus deck in ICON-D2-ART but overestimates the impact of the dust. This might be due to the simple diagnostic nature of the scheme that is unable to represent the microphysical aging and dissipation of the cirrus cloud. An Aqua overpass for 6 May, 12:55 UTC, confirms the behavior seen with SEVIRI and is shown in Fig. S13.
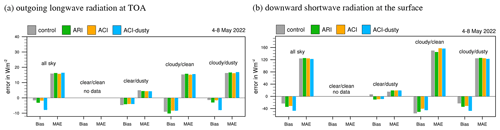
Figure 17Bias and mean absolute error (MAE) of ICON-D2-ART compared to CERES SSF level-2 radiative fluxes for 4–8 May 2022. Shown are the outgoing longwave radiation at the top of the atmosphere (a) and the solar irradiance at the surface (b).
In the statistical evaluation of all CERES overpasses from 4–8 May 2022 shown in Fig. 17, the ACI and ARI simulations are clearly superior to ACI–dusty (for reflected shortwave at TOA see Fig. S8). Hence, the dusty cirrus parameterization leads to an unrealistic intensification of the cirrus formation during this dust episode. The result is a significant negative bias in the OLR and increased biases in the shortwave fluxes compared to the other ICON simulations. This suggests that the dusty cirrus parameterization is too simple to describe all dust events and the related cirrus clouds correctly. Given the simplicity of the parameterization, this is not surprising, though. The two main processes that are missing are, first, the transient behavior of the cirrus cloud depth during formation and decay of the cirrus deck and, second, the microphysical processes in the cirrus cloud, like aggregation and sedimentation. The lack of microphysical processes contributes to a prolonged lifetime and could cause the overestimation that we see in this case.
3.4 Statistical evaluation of six Saharan dust events
So far we have focused on case studies of Saharan dust events, which show some observational evidence for dusty cirrus occurrence. For any practical application of the dusty cirrus parameterization, it is essential that it does not deteriorate the forecasts during dust events without dusty cirrus formation; i.e., the false alarm rate for dusty cirrus needs to be small. To check this, we have performed simulations for three more Saharan dust events without observational evidence for dusty cirrus (see Table 1).
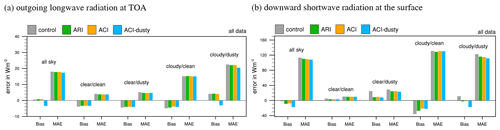
Figure 18Bias and mean absolute error (MAE) of ICON-D2-ART compared to CERES SSF level-2 radiative fluxes for all six Saharan dust episodes. Shown are the outgoing longwave radiation at the top of the atmosphere (a), the reflected shortwave at TOA, and the solar irradiance at the surface (b).
Figure 18 shows the bias and mean absolute errors (MAEs) for the radiative fluxes validated by CERES SSF data (for reflected shortwave at TOA see Fig. S10). For the solar irradiance at the surface, we see a clear improvement in clear/dusty conditions dominated by the direct radiation effect of mineral dust. Hence, the improvement is already seen in ARI without further gain from ACI or ACI–dusty. The impact of mineral dust in clear/clean conditions is small, as expected. For cloudy/dusty conditions we find an improvement by ARI (because this includes partially cloudy pixels) and further improvements in MAE from ACI–dusty. The mean bias is the smallest for ARI and ACI and becomes negative for ACI–dusty. But a close inspection reveals that the small mean bias for ARI and ACI is an error compensation between cases in early spring, which have a positive bias in those simulations, and late spring, which have a negative bias. This change in bias behavior is related to the occurrence of deep convection in May/June, which contributes to a negative bias due to the overestimation of convective anvils in ICON-D2. Hence, the small bias of ARI and ACI should not be interpreted as a general forecast improvement. Similar arguments apply to OLR and reflected shortwave radiation at TOA. For both TOA fluxes we find the lowest MAE for ACI–dusty, but the mean bias becomes negative for OLR and increases for reflected shortwave radiation. Again, this is most likely related to an overestimation of deep convective anvils in ICON-D2, which leads to an error compensation in ARI and ACI. Hence, from this validation with CERES SSF level-2 data we find a small overall improvement from ACI–dusty compared to the other simulations, but further work would be necessary to, first, reduce the false alarms of the dusty cirrus parameterization and, second, improve the representation of convective anvils in ICON-D2.
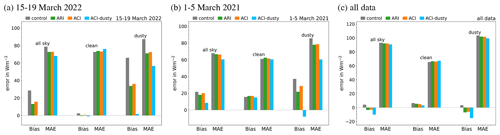
Figure 19Bias and mean absolute error (MAE) of solar irradiance at the surface using DWD's pyranometer network for the dust episodes from 15–19 March 2022 (a), from 1–5 March 2021 (b), and for all data (c).
The retrieval of solar irradiance at the surface from satellite data can lead to considerable uncertainties for cloudy pixels (Kratz et al., 2020). To further support our results we have performed an additional validation with the 27 pyranometers of DWD's radiation station network in Germany. Due to the rather short time periods of 5 d, we refrain from a decomposition in clear-sky and cloudy conditions for the pyranometer data. As for the validation using CERES data, dusty conditions are defined based on ICON-D2-ART dust AOD exceeding 0.1. Figure 19 shows the results for the two major dusty cirrus episodes and for all six dust episodes investigated in this study. The biases and MAEs compare well with the results against CERES in Figs. 6, 14, and 18. This not only confirms our findings but also confirms that CERES level-2 instantaneous fluxes can indeed be used for this kind of episode-based analysis. The pyranometer results for the other dust episodes are provided in the Supplement (Fig. S2).
Note that the overall improvement seen with the dusty cirrus parameterization may become smaller for longer time periods and with more non-dusty cirrus episodes. Due to the fact that the dusty cirrus is a rare event, false alarms and double-penalty issues make it a challenging forecasting problem. The double-penalty problem occurs due to predictability limits on scales smaller than 100 km, when the verification is done on the cloud scale. Hence, further investigations and improvements of the scheme would be necessary before it can be generally applied.
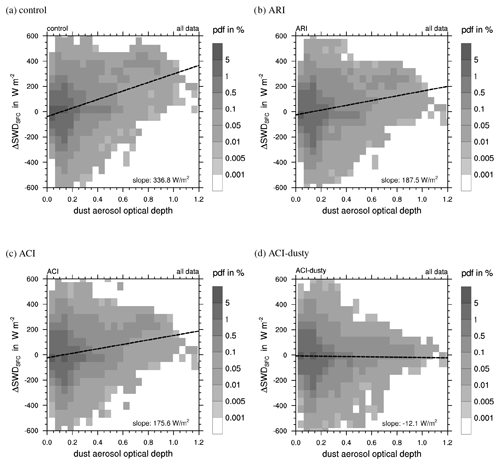
Figure 20Joint histogram of errors in solar irradiance at the surface as a function of mineral dust AOD predicted by ICON-D2-ART for all six Saharan dust episodes shown in Table 1. Errors are defined as (model-obs) for individual CERES footprints with a diameter of 25 km.
More insight into aerosol–cloud–radiative effects can be gained from an analysis of the errors on individual CERES footprints. Figure 20 shows the (model-obs) errors as a function of the mineral dust AOD simulated by ICON-D2-ART. Hence, this analysis gives an indication of whether the modeled radiative flux has the correct sensitivity to mineral dust AOD. The control ICON-D2 simulation shows large positive errors for high dust AOD. This corresponds to the lack of direct and indirect aerosol effects during the Saharan dust episodes. A linear regression gives a slope of 336.8 W m−2 for the sensitivity . Taking into account the direct aerosol–radiative effect in ARI reduces these errors significantly and reduces the slope to 187.5 W m−2, but large errors remain especially for high AOD, which correspond to the occurrence of aerosol–cloud interaction. Considering grid-scale aerosol–cloud effects due to mineral dust acting as INPs has only a marginal impact on ICON-D2-ART and gives only a reduction in the slope to 175.6 W m−2. Only the newly developed sub-grid dusty cirrus parameterization is able to correct the large errors in solar irradiance for large AODs, which obviously correspond to the pixels below the extended cirrus clouds decks. Interestingly, the slope becomes almost 0; actually it is already slightly negative with a value of −12.1 W m−2. Similar behavior is found for OLR (see Fig. S11), but the impact of the direct aerosol is much weaker with a decrease in slope from 84.4 W m−2 for control to 75.3 W m−2 for ARI. The improvement from ACI is again marginal with a slope of 71.3 W m−2, whereas the dust cirrus parameterization removes the dependency on mineral dust AOD, resulting in a slope of −4.9 W m−2.
The fact that on the one hand grid-scale ACI has only a negligible impact and, on the other hand, the dusty cirrus parameterization fully removes the aerosol sensitivity is remarkable. It shows that for the six Saharan dust episodes of the current study, dusty cirrus formation is the dominant aerosol–cloud–radiative effect of mineral dust over Europe. This is somewhat unexpected because enhanced mineral dust concentrations during Saharan dust episodes should lead to more INPs being available for ice formation. It is often argued that high INP numbers increase the ice particle number concentration and subsequently increase ice water content and ice water path (e.g., Seifert et al., 2012). Our simulations suggest that this sensitivity is very weak in ICON-D2-ART. A possible physical explanation is the so-called freezing–relaxation feedback, which is usually described for homogeneous ice nucleation (Kärcher and Seifert, 2016; Kärcher and Jensen, 2017), but which does in principle also apply to heterogeneous ice nucleation. Freezing–relaxation means that within an active updraft the ice supersaturation increases until enough ice particles are present to efficiently deplete the supersaturation. This establishes a nonlinear feedback or buffering mechanism, which can dramatically reduce the sensitivity of the ice particle number concentration to INPs. Hence, a low number of INPs will lead to larger ice supersaturation but not necessarily to a significant decrease in ice particle number concentration. Similarly, a high number of INPs, as during a Saharan dust event, will reduce the local supersaturations within the updrafts but has little effect on the actual cloud properties like ice particle number, ice water content, or ice effective radius. This behavior is consistent with the recent findings of Chen et al. (2022), who showed that aerosol–cloud effects due to volcanic aerosol perturbations in low-level liquid clouds are dominated by changes in cloud cover rather than by cloud brightening. Similarly, in our case the formation of the dusty cirrus and the corresponding increase in cloud cover dominate over changes in cloud optical properties due to mineral dust.
Outbreaks of Saharan dust reaching Europe are occasionally accompanied by the formation of a large-scale cirrus cloud, a phenomenon known as dusty cirrus. Since today's global numerical weather prediction models neither predict mineral dust distributions nor consider the interaction of dust with cloud microphysics, they cannot simulate this phenomenon. Among other things, this leads to significant forecast errors regarding photovoltaic power generation. Avoiding such forecast errors will become more and more important.
We have developed a simple sub-grid-scale cloud parameterization scheme that allows simulating and therefore predicting dusty cirrus clouds. As the physical mechanism that leads to the formation of the dusty cirrus, we postulate a microphysical mixing instability of clean moist air above drier dusty air in the upper troposphere combined with synoptic-scale lifting of the air layers, e.g., through a WCB airstream underneath. Once a cirrus cloud has formed at the interface between moist and dusty air, the dominant longwave cooling at the cloud top generates turbulence and mixing, which thicken the cloud layer.
The new dusty cirrus parameterization was included in ICON-ART, and case and sensitivity studies were performed. ICON-ART allows calculating the interaction of mineral dust with radiation. When the two-moment cloud microphysics scheme is used, the interaction of mineral dust with grid-scale microphysical processes is also calculated.
Although in one of the simulated cases, the dusty cirrus parameterization produced too thick, too extended cirrus layers and false alarms, we show that the new parameterization leads to a considerable improvement in the forecast of longwave and shortwave radiative fluxes at the top of the atmosphere and at the surface. Moreover, it leads to an improvement in the simulated cloud cover. In contrast, the two-moment microphysics scheme alone is not able to simulate the formation of the dusty cirrus, although it is coupled to the prognostic mineral dust. In total, six cases were simulated. In three of them, dusty cirrus occurred, and in three of them, mineral dust was present without dusty cirrus formation. Our new parameterization does not diminish the forecast quality in cases when dust outbreaks happened but no dusty cirrus occurred.
It remains to be proven that the concept of a microphysical mixing instability accompanied by an air mass configuration of moist air over dusty air applies not only to dusty cirrus formation in Europe but also to dusty cirrus formation in other parts of the world.
The ICON code is available under two different licenses: a personal non-commercial scientific license and an institutional license that requires a cooperation agreement with DWD. More details on the licenses and instructions on how to obtain the ICON code can be found at https://code.mpimet.mpg.de/projects/iconpublic/wiki/Instructions_to_obtain_the_ICON_model_code_with_a_personal_non-commercial_research_license (MPI-M, 2023).
The raw model output analyzed for this study exceeds 20 TB and cannot easily be made available. A subset of the model output to reproduce many of the figures is available as a Zenodo archive (Seifert, 2023, https://doi.org/10.5281/zenodo.7976168). Meteosat (MSG)–SEVIRI data were obtained from EUMETSAT (2009, https://navigator.eumetsat.int/product/EO:EUM:DAT:MSG:HRSEVIRI). CERES SSF data were obtained from NASA's Langley Research Center (LaRC) Atmospheric Sciences Data Center (ASDC) at https://doi.org/10.5067/TERRA/CERES/SSF_Terra-FM1_L2.004A (NASA/LARC/SD/ASDC, 2014b) and https://doi.org/10.5067/AQUA/CERES/SSF-FM3_L2.004A (NASA/LARC/SD/ASDC, 2014a).
The supplement related to this article is available online at: https://doi.org/10.5194/acp-23-6409-2023-supplement.
AS performed the ICON-D2-ART simulations and carried out the evaluation using satellite and radiosonde data. AS developed and implemented the dusty cirrus parameterization. VB and JF maintain the ICON-ART model at DWD and performed the global ICON-ART simulations that serve as initial and boundary conditions for the current study. VB developed the ICON-D2-ART hindcast setup used in this study. BV, GAH, HV, and AR developed the dust processes and the dust–radiation interactions in ICON-ART, including the optical properties for ecRad as used in this study. FF performed the validation with DWD's pyranometer network, and AW provided the validation with DWD's ceilometer network. AS wrote the initial version of the manuscript. CMG and JQ performed the trajectory analysis. All authors contributed to the interpretation of the results and the writing of the manuscript.
The contact author has declared that none of the authors has any competing interests.
Publisher's note: Copernicus Publications remains neutral with regard to jurisdictional claims in published maps and institutional affiliations.
We thank both anonymous reviewers and the editor for their comments on the manuscript. Axel Seifert is grateful to Bjorn Stevens, Jens Reichardt, Juan Pedro Mellado, Alberto de Lozar, and Günther Zängl for helpful discussion. We thank Ivan Smiljanić of EUMETSAT for pointing us to the dusty cirrus case of 6 May 2022. We thank Annika Schomburg, Leonhard Scheck, Thomas Deppisch, Christina Stumpf, and Alberto de Lozar for their work on and help with the RTTOV forward operator. Annika Schomburg processed and provided the SEVIRI data for this study.
All simulations were performed on the NEC Aurora of DWD. Most plots were created with the NCAR Command Language (NCAR, 2019). This work contributes to and is partly funded by the project PermaStrom within the seventh Energieforschungsprogramm of the German Federal Ministry of Economic Affairs and Climate Action (Bundesministerium für Wirtschaft und Klimaschutz, BMWK). The contributions of Christian M. Grams and Julian Quinting are funded by the Helmholtz Association as part of the Young Investigator Group Sub-seasonal Predictability: Understanding the Role of Diabatic Outflow (SPREADOUT)
This research has been supported by the Helmholtz-Gemeinschaft (SPREADOUT, grant no. VH-NG-1243) and the BMWK project “PermaStrom” (grant no. 03EI4010A).
This paper was edited by Yuan Wang and reviewed by two anonymous referees.
Ansmann, A., Mamouri, R.-E., Bühl, J., Seifert, P., Engelmann, R., Hofer, J., Nisantzi, A., Atkinson, J. D., Kanji, Z. A., Sierau, B., Vrekoussis, M., and Sciare, J.: Ice-nucleating particle versus ice crystal number concentrationin altocumulus and cirrus layers embedded in Saharan dust:a closure study, Atmos. Chem. Phys., 19, 15087–15115, https://doi.org/10.5194/acp-19-15087-2019, 2019. a
Antonanzas, J., Osorio, N., Escobar, R., Urraca, R., de Pison, F. M., and Antonanzas-Torres, F.: Review of photovoltaic power forecasting, Sol. Energy, 136, 78–111, https://doi.org/10.1016/j.solener.2016.06.069, 2016. a
Baldauf, M., Seifert, A., Förstner, J., Majewski, D., Raschendorfer, M., and Reinhardt, T.: Operational convective-scale numerical weather prediction with the COSMO model: Description and sensitivities, Mon. Weather Rev., 139, 3887–3905, 2011. a
Bunn, P. T. W., Holmgren, W. F., Leuthold, M., and Castro, C. L.: Using GEOS-5 forecast products to represent aerosol optical depth in operational day-ahead solar irradiance forecasts for the southwest United States, J. Renew. Sustain. Ener., 12, 053702, https://doi.org/10.1063/5.0020785, 2020. a
Caffrey, P. F., Fromm, M. D., and Kablick III, G. P.: WRF-Chem simulation of an East Asian dust-infused baroclinic storm (DIBS), J. Geophys. Res., 123, 6880–6895, https://doi.org/10.1029/2017JD027848, 2018. a
Chen, Y., Haywood, J., Wang, Y., Malavelle, F., Jordan, G., Partridge, D., Fieldsend, J., De Leeuw, J., Schmidt, A., Cho, N., Oreopoulos, L., Platnick, S., Grosvenor, D., Field, P., and Lohmann, U.: Machine learning reveals climate forcing from aerosols is dominated by increased cloud cover, Nat. Geoscience, 15, 609–614, 2022. a
DeMott, P. J., Prenni, A. J., Liu, X., Kreidenweis, S. M., Petters, M. D., Twohy, C. H., Richardson, M., Eidhammer, T., and Rogers, D.: Predicting global atmospheric ice nuclei distributions and their impacts on climate, P. Natl. Acad. Sci. USA, 107, 11217–11222, https://doi.org/10.1073/pnas.0910818107, 2010. a
DeMott, P. J., Prenni, A. J., McMeeking, G. R., Sullivan, R. C., Petters, M. D., Tobo, Y., Niemand, M., Möhler, O., Snider, J. R., Wang, Z., and Kreidenweis, S. M.: Integrating laboratory and field data to quantify the immersion freezing ice nucleation activity of mineral dust particles, Atmos. Chem. Phys., 15, 393–409, https://doi.org/10.5194/acp-15-393-2015, 2015. a
EUMETSAT: High Rate SEVIRI Level 1.5 Image Data - MSG - 0 degree, European Organisation for the Exploitation of Meteorological Satellites [data set], Darmstadt, Germany, https://navigator.eumetsat.int/product/EO:EUM:DAT:MSG:HRSEVIRI (last access: 7 June 2023), 2009. a
Fierli, F., Martinez, M.-A., Asmus, J., and Roesli, H.-P.: Widespread dust intrusion across Europe, EUMETSAT, https://www.eumetsat.int/widespread-dust-intrusion-across-europe (last access: 30 October 2022), 2022. a
Fromm, M., Kablick III, G., and Caffrey, P.: Dust-infused baroclinic cyclone storm clouds: The evidence, meteorology, and some implications, Geophys. Res. Lett., 43, 12,643–12,650, https://doi.org/10.1002/2016GL071801, 2016. a
Fu, Q.: An accurate parameterization of the solar radiative properties of cirrus clouds for climate models, J. Climate, 9, 2058–2082, 1996. a
Fusina, F. and Spichtinger, P.: Cirrus clouds triggered by radiation, a multiscale phenomenon, Atmos. Chem. Phys., 10, 5179–5190, https://doi.org/10.5194/acp-10-5179-2010, 2010. a
Gasch, P., Rieger, D., Walter, C., Khain, P., Levi, Y., Knippertz, P., and Vogel, B.: Revealing the meteorological drivers of the September 2015 severe dust event in the Eastern Mediterranean, Atmos. Chem. Phys., 17, 13573–13604, https://doi.org/10.5194/acp-17-13573-2017, 2017. a
Geiss, S., Scheck, L., de Lozar, A., and Weissmann, M.: Understanding the model representation of clouds based on visible and infrared satellite observations, Atmos. Chem. Phys., 21, 12273–12290, https://doi.org/10.5194/acp-21-12273-2021, 2021. a
Gierens, K., Wilhelm, L., Hofer, S., and Rohs, S.: The effect of ice supersaturation and thin cirrus on lapse rates in the upper troposphere, Atmos. Chem. Phys., 22, 7699–7712, https://doi.org/10.5194/acp-22-7699-2022, 2022. a
Hersbach, H., Bell, B., Berrisford, P., Hirahara, S., Horányi, A., Mu noz-Sabater, J., Nicolas, J., Peubey, C., Radu, R., Schepers, D., Simmons, A., Soci, C., Abdalla, S., Abellan, X., Balsamo, G., Bechtold, P., Biavati, G., Bidlot, J., Bonavita, M., De Chiara, G., Dahlgren, P., Dee, D., Diamantakis, M., Dragani, R., Flemming, J., Forbes, R., Fuentes, M., Geer, A., Haimberger, L., Healy, S., Hogan, R. J., Hólm, E., Janisková, M., Keeley, S., Laloyaux, P., Lopez, P., Lupu, C., Radnoti, G., de Rosnay, P., Rozum, I., Vamborg, F., Villaume, S., and Thépaut, J.-N.: The ERA5 Global Reanalysis, Q. J. Roy. Meteor. Soc., 146, 1999–2049, https://doi.org/10.1002/qj.3803, 2020. a
Hogan, R. and Bozzo, A.: ECRAD: A new radiation scheme for the IFS, Tech. Rep. 787, ECMWF, https://doi.org/10.21957/whntqkfdz, 2016. a
Hogan, R. J. and Bozzo, A.: A flexible and efficient radiation scheme for the ECMWF model, J. Adv. Model Earth Sy., 10, 1990–2008, https://doi.org/10.1029/2018MS001364, 2018. a
Hoshyaripour, G. A., Bachmann, V., Förstner, J., Steiner, A., Vogel, H., Wagner, F., Walter, C., and Vogel, B.: Effects of Particle Nonsphericity on Dust Optical Properties in a Forecast System: Implications for Model-Observation Comparison, J. Geophys. Res., 124, 7164–7178, https://doi.org/10.1029/2018JD030228, 2019. a, b
Hui, W. J., Cook, B. I., Ravi, S., Fuentes, J. D., and D'Odorico, P.: Dust-rainfall feedbacks in the West African Sahel, Water Resour. Res., 44, W05202, https://doi.org/10.1029/2008WR006885, 2008. a
Jin, Q., Wei, J., Lau, W. K., Pu, B., and Wang, C.: Interactions of Asian mineral dust with Indian summer monsoon: Recent advances and challenges, Earth-Sci. Rev., 215, 103562, https://doi.org/10.1016/j.earscirev.2021.103562, 2021. a
Kärcher, B. and Jensen, E.: Microscale characteristics of homogeneous freezing events in cirrus clouds, Geophys. Res. Lett., 44, 2027–2034, https://doi.org/10.1002/2016GL072486, 2017. a
Kärcher, B. and Seifert, A.: On homogeneous ice formation in liquid clouds, Q. J. Roy. Meteor. Soc., 142, 1320–1334, https://doi.org/10.1002/qj.2735, 2016. a
Kärcher, B., Hendricks, J., and Lohmann, U.: Physically based parameterization of cirrus cloud formation for use in global atmospheric models, J. Geophys. Res., 111, D01205, https://doi.org/10.1029/2005JD006219, 2006. a
Köhler, C. G. and Seifert, A.: Identifying sensitivities for cirrus modelling using a two-moment two-mode bulk microphysics scheme, Tellus B, 67, 24494, https://doi.org/10.3402/tellusb.v67.24494, 2015. a
Kollath, K.: Cellular convection in cirrus clouds as a possible effect of dust aerosols, EUMETSAT, https://www.eumetsat.int/media/46886 (last access: 30 October 2022), 2010. a, b, c
Kratz, D. P., Gupta, S. K., Wilber, A. C., and Sothcott, V. E.: Validation of the CERES Edition-4A Surface-Only Flux Algorithms, J. Appl. Meteorol. Clim., 59, 281–295, https://doi.org/10.1175/JAMC-D-19-0068.1, 2020. a, b
Kuebbeler, M., Lohmann, U., Hendricks, J., and Kärcher, B.: Dust ice nuclei effects on cirrus clouds, Atmos. Chem. Phys., 14, 3027–3046, https://doi.org/10.5194/acp-14-3027-2014, 2014. a
Le Trent, H. and Li, Z.-X.: Sensitivity of an atmospheric general circulation model to prescribed SST changes: Feedback effects associated with the simulation of cloud optical properties, Clim. Dynam., 5, 175–187, 1991. a
Maciel, F. V., Diao, M., and Patnaude, R.: Examination of aerosol indirect effects during cirrus cloud evolution, Atmos. Chem. Phys., 23, 1103–1129, https://doi.org/10.5194/acp-23-1103-2023, 2023. a
Morcrette, J.-J., Boucher, O., Jones, L., Salmond, D., Bechtold, P., Beljaars, A., Benedetti, A., Bonet, A., Kaiser, J. W., Razinger, M., Schulz, M., Serrar, S., Simmons, A. J., Sofiev, M., Suttie, M., Tompkins, A. M., and Untch, A.: Aerosol analysis and forecast in the European Centre for Medium-Range Weather Forecasts Integrated Forecast System: Forward modeling, J. Geophys. Res., 114, D06206, https://doi.org/10.1029/2008JD011235, 2009. a
MPI-M: Instructions for obtaining the ICON Code, https://code.mpimet.mpg.de/projects/iconpublic/wiki/Instructions_to_obtain_the_ICON_model_code_with_a_personal_non-commercial_research_license, last access: 26 May 2023. a
Muser, L. O., Hoshyaripour, G. A., Bruckert, J., Horváth, Á., Malinina, E., Wallis, S., Prata, F. J., Rozanov, A., von Savigny, C., Vogel, H., and Vogel, B.: Particle aging and aerosol–radiation interaction affect volcanic plume dispersion: evidence from the Raikoke 2019 eruption, Atmos. Chem. Phys., 20, 15015–15036, https://doi.org/10.5194/acp-20-15015-2020, 2020. a
Nagy, A.: Investigating weather situations which bring Saharan dust over Hungary based on MSG satellite images, Master's thesis, ELTE University, Budapest, 2009 (in Hungarian). a, b
NASA/LARC/SD/ASDC: CERES Single Scanner Footprint (SSF) TOA/Surface Fluxes, Clouds and Aerosols Aqua-FM3 Edition4A, NASA Langley Atmospheric Science Data Center DAAC [data set], https://doi.org/10.5067/AQUA/CERES/SSF-FM3_L2.004A, 2014a. a
NASA/LARC/SD/ASDC: CERES Single Scanner Footprint (SSF) TOA/Surface Fluxes, Clouds and Aerosols Terra-FM1 Edition4A, NASA Langley Atmospheric Science Data Center DAAC [data set], https://doi.org/10.5067/TERRA/CERES/SSF_Terra-FM1_L2.004A, 2014b. a
NCAR: The NCAR Command Language, Version 6.6.2, UCAR/NCAR/CISL/TDD [code], Boulder, Colorado, https://doi.org/10.5065/D6WD3XH5, 2019. a
Nowottnick, E., Colarco, P., da Silva, A., Hlavka, D., and McGill, M.: The fate of saharan dust across the atlantic and implications for a central american dust barrier, Atmos. Chem. Phys., 11, 8415–8431, https://doi.org/10.5194/acp-11-8415-2011, 2011. a
Pan, B., Yao, Z., Wang, M., Pan, H., Bu, L., Kumar, K. R., Gao, H., and Huang, X.: Evaluation and utilization of CloudSat and CALIPSO data to analyze the impact of dust aerosol on the microphysical properties of cirrus over the Tibetan Plateau, Adv. Space Res., 63, 2–15, https://doi.org/10.1016/j.asr.2018.07.004, 2019. a
Parajuli, S. P., Stenchikov, G. L., Ukhov, A., Mostamandi, S., Kucera, P. A., Axisa, D., Gustafson Jr., W. I., and Zhu, Y.: Effect of dust on rainfall over the Red Sea coast based on WRF-Chem model simulations, Atmos. Chem. Phys., 22, 8659–8682, https://doi.org/10.5194/acp-22-8659-2022, 2022. a
Penner, J. E., Zhou, C., Garnier, A., and Mitchell, D. L.: Anthropogenic Aerosol Indirect Effects in Cirrus Clouds, J. Geophys. Res., 123, 11652–11677, https://doi.org/10.1029/2018JD029204, 2018. a
Phillips, V. T., DeMott, P. J., and Andronache, C.: An empirical parameterization of heterogeneous ice nucleation for multiple chemical species of aerosol, J. Atmos. Sci., 65, 2757–2783, 2008. a
Reinert, D., Prill, F., Frank, H., Denhard, M., Baldauf, M., Schraff, C., Gebhardt, C., Marsigli, C., and Zängl, G.: DWD database reference for the global and regional ICON and ICON-EPS forecasting system, Technical report and database description, version 2.1.8, Deutscher Wetterdienst, https://www.dwd.de/SharedDocs/downloads/DE/modelldokumentationen/nwv/icon/icon_dbbeschr_aktuell.html (last access: 30 October 2022), 2022. a, b
Rémy, S., Kipling, Z., Flemming, J., Boucher, O., Nabat, P., Michou, M., Bozzo, A., Ades, M., Huijnen, V., Benedetti, A., Engelen, R., Peuch, V.-H., and Morcrette, J.-J.: Description and evaluation of the tropospheric aerosol scheme in the European Centre for Medium-Range Weather Forecasts (ECMWF) Integrated Forecasting System (IFS-AER, cycle 45R1), Geosci. Model Dev., 12, 4627–4659, https://doi.org/10.5194/gmd-12-4627-2019, 2019. a
Rieger, D., Bangert, M., Bischoff-Gauss, I., Förstner, J., Lundgren, K., Reinert, D., Schröter, J., Vogel, H., Zängl, G., Ruhnke, R., and Vogel, B.: ICON–ART 1.0 – a new online-coupled model system from the global to regional scale, Geosci. Model Dev., 8, 1659–1676, https://doi.org/10.5194/gmd-8-1659-2015, 2015. a
Rieger, D., Steiner, A., Bachmann, V., Gasch, P., Förstner, J., Deetz, K., Vogel, B., and Vogel, H.: Impact of the 4 April 2014 Saharan dust outbreak on the photovoltaic power generation in Germany, Atmos. Chem. Phys., 17, 13391–13415, https://doi.org/10.5194/acp-17-13391-2017, 2017. a, b, c
Rieger, D., Köhler, M., Hogan, R. J., Schäfer, S. A. K., Seifert, A., de Lozar, A., and Zängl, G.: ecRad in ICON, Reports on ICON, Issue 4, Deutscher Wetterdienst, https://doi.org/10.5676/DWD_pub/nwv/icon_004, 2019. a
Roesli, H.-P., Putsay, M., and Smiljanic, I.: Extensive DIBS in the Deformation Zone, EUMETSAT, https://www.eumetsat.int/extensive-dibs-deformation-zone (last access: 30 October 2022), 2020. a
Saunders, R., Hocking, J., Turner, E., Rayer, P., Rundle, D., Brunel, P., Vidot, J., Roquet, P., Matricardi, M., Geer, A., Bormann, N., and Lupu, C.: An update on the RTTOV fast radiative transfer model (currently at version 12), Geosci. Model Dev., 11, 2717–2737, https://doi.org/10.5194/gmd-11-2717-2018, 2018. a
Scheck, L., Weissmann, M., and Mayer, B.: Efficient Methods to Account for Cloud-Top Inclination and Cloud Overlap in Synthetic Visible Satellite Images, J. Atmos. Ocean. Tech., 35, 665–685, https://doi.org/10.1175/JTECH-D-17-0057.1, 2018. a
Schröter, J., Rieger, D., Stassen, C., Vogel, H., Weimer, M., Werchner, S., Förstner, J., Prill, F., Reinert, D., Zängl, G., Giorgetta, M., Ruhnke, R., Vogel, B., and Braesicke, P.: ICON-ART 2.1: a flexible tracer framework and its application for composition studies in numerical weather forecasting and climate simulations, Geosci. Model Dev., 11, 4043–4068, https://doi.org/10.5194/gmd-11-4043-2018, 2018. a
Seifert, A.: ICON-D2-ART output for “Aerosol-cloud-radiation interaction during Saharan dust episodes: the dusty cirrus puzzle”, Zenodo [data set], https://doi.org/10.5281/zenodo.7976168, 2023. a
Seifert, A. and Beheng, K. D.: A two-moment cloud microphysics parameterization for mixed-phase clouds. Part 1: Model description, Meteorol. Atmos. Phys., 92, 45–66, https://doi.org/10.1007/s00703-005-0112-4, 2006. a
Seifert, A., Köhler, C., and Beheng, K. D.: Aerosol-cloud-precipitation effects over Germany as simulated by a convective-scale numerical weather prediction model, Atmos. Chem. Phys., 12, 709–725, https://doi.org/10.5194/acp-12-709-2012, 2012. a, b
Smith, R.: A scheme for predicting layer clouds and their water content in a general circulation model, Q. J. Roy. Meteor. Soc., 116, 435–460, https://doi.org/10.1002/qj.49711649210, 1990. a
Spichtinger, P.: Shallow cirrus convection – a source for ice supersaturation, Tellus A, 66, 19937, https://doi.org/10.3402/tellusa.v66.19937, 2014. a, b
Sprenger, M. and Wernli, H.: The LAGRANTO Lagrangian analysis tool – version 2.0, Geosci. Model Dev., 8, 2569–2586, https://doi.org/10.5194/gmd-8-2569-2015, 2015. a
Su, W., Corbett, J., Eitzen, Z., and Liang, L.: Next-generation angular distribution models for top-of-atmosphere radiative flux calculation from CERES instruments: methodology, Atmos. Meas. Tech., 8, 611–632, https://doi.org/10.5194/amt-8-611-2015, 2015a. a
Su, W., Corbett, J., Eitzen, Z., and Liang, L.: Next-generation angular distribution models for top-of-atmosphere radiative flux calculation from CERES instruments: validation, Atmos. Meas. Tech., 8, 3297–3313, https://doi.org/10.5194/amt-8-3297-2015, 2015b. a
Tegen, I., Hollrig, P., Chin, M., Fung, I., Jacob, D., and Penner, J.: Contribution of different aerosol species to the global aerosol extinction optical thickness: Estimates from model results, J. Geophys. Res., 102, 23895–23915, https://doi.org/10.1029/97JD01864, 1997. a
Ullrich, R., Hoose, C., Möhler, O., Niemand, M., Wagner, R., Höhler, K., Hiranuma, N., Saathoff, H., and Leisner, T.: A new ice nucleation active site parameterization for desert dust and soot, J. Atmos. Sci., 74, 699–717, 2017. a
Wang, M., Liu, X., Zhang, K., and Comstock, J. M.: Aerosol effects on cirrus through ice nucleation in the Community Atmosphere Model CAM5 with a statistical cirrus scheme, J. Adv. Model Earth Sy., 6, 756–776, https://doi.org/10.1002/2014MS000339, 2014. a
Wang, W., Sheng, L., Jin, H., and Han, Y.: Dust aerosol effects on cirrus and altocumulus clouds in Northwest China, J. Meteorol. Res.-P. R. C., 29, 793–805, https://doi.org/10.1007/s13351-015-4116-9, 2015. a
Weger, M., Heinold, B., Engler, C., Schumann, U., Seifert, A., Fößig, R., Voigt, C., Baars, H., Blahak, U., Borrmann, S., Hoose, C., Kaufmann, S., Krämer, M., Seifert, P., Senf, F., Schneider, J., and Tegen, I.: The impact of mineral dust on cloud formation during the Saharan dust event in April 2014 over Europe, Atmos. Chem. Phys., 18, 17545–17572, https://doi.org/10.5194/acp-18-17545-2018, 2018. a
Wernli, H.: A Lagrangian-Based Analysis of Extratropical Cyclones. II: A Detailed Case-Study, Q. J. Roy. Meteor. Soc., 123, 1677–1706, 1997. a
Wernli, H., Boettcher, M., Joos, H., Miltenberger, A. K., and Spichtinger, P.: A Trajectory-Based Classification of ERA-Interim Ice Clouds in the Region of the North Atlantic Storm Track, Geophys. Res. Lett., 43, 6657–6664, https://doi.org/10.1002/2016GL068922, 2016. a, b
Zängl, G., Reinert, D., Rípodas, P., and Baldauf, M.: The ICON (ICOsahedral Non-hydrostatic) modelling framework of DWD and MPI-M: Description of the non-hydrostatic dynamical core, Q. J. Roy. Meteor. Soc., 141, 563–579, https://doi.org/10.1002/qj.2378, 2015. a