the Creative Commons Attribution 4.0 License.
the Creative Commons Attribution 4.0 License.
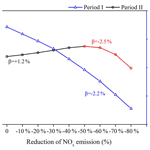
Nonlinear responses of particulate nitrate to NOx emission controls in the megalopolises of China
Mengmeng Li
Zihan Zhang
Quan Yao
Tijian Wang
Shu Li
Bingliang Zhuang
Yong Han
Nitrate is an increasingly important component of fine particulate matter (PM2.5) in Chinese cities. The production of nitrate is not only related to the abundance of its precursor, but it is also supported by the atmospheric photochemical oxidants, raising a new challenge for the current emission control actions in China. This paper uses comprehensive measurements and a regional meteorology–chemistry model with optimized mechanisms to establish the nonlinear responses between particulate nitrate and the emission controls of nitrogen oxides (NOx) in the megalopolises of China. Nitrate is an essential component of PM2.5 in eastern China, accounting for 9.4 %–15.5 % and 11.5 %–32.1 % of the PM2.5 mass for the warm and cold seasons. The hypothetical NOx emission reduction scenarios (−10 % to −80 %) during summer–autumn result in almost linearly lower PM2.5 by −2.2 % in Beijing–Tianjin–Hebei (BTH) and −2.9 % in Yangtze River Delta (YRD) per 10 % reduction of NOx emissions, whereas they lead to a rather complicated response of PM components in winter. Wintertime nitrate is found to increase by +4.1 % in BTH and +5.1 % in YRD per 10 % reduction of NOx emissions, with nearly unchanged nitric acid (HNO3) and higher dinitrogen pentoxide (N2O5) intermediate products produced from the increased atmospheric oxidant levels. An inflexion point appears at 30 %–50 % NOx emission reduction, and a further reduction in NOx emissions is predicted to cause −10.5 % reduction of nitrate for BTH and −7.7 % for YRD per 10 % reduction of NOx emissions. In addition, the 2012–2016 NOx control strategy actually leads to no changes or even increases of nitrate in some areas (8.8 % in BTH and 14.4 % in YRD) during winter. Our results also emphasize that ammonia (NH3) and volatile organic compounds (VOCs) are effective in controlling nitrate pollution, whereas decreasing the sulfur dioxide (SO2) and NOx emissions may have counterintuitive effects on nitrate aerosols. This paper helps understand the nonlinear aerosol and photochemistry feedbacks and defines the effectiveness of proposed mitigations for the increasingly serious nitrate pollution in China.
- Article
(16251 KB) - Full-text XML
-
Supplement
(872 KB) - BibTeX
- EndNote
Secondary inorganic aerosols (SIAs), including sulfate (), nitrate () and ammonium (), account for 30 %–60 % of the total fine particulate matter (PM2.5) mass during haze events in China (R. J. Huang et al., 2014; Zhao et al., 2013). Since the enactment of the Air Pollution Prevention and Control Action Plan in 2013, the Chinese government has taken drastic measures to reduce the emissions of sulfur dioxide (SO2), nitrogen oxides (NOx) and primary PM2.5, leading to significant decreases in sulfate and overall PM2.5 concentrations in cities (Silver et al., 2018; Li et al., 2021a; J. D. Wang et al., 2017). Meanwhile, the nitrogen / sulfur () ratio in PM2.5 increased significantly, and nitrate became the main component of PM2.5 (16 %–45 %) during haze episodes, despite a more than 20 % reduction in the concentrations of its precursor NOx (Shao et al., 2018; Wen et al., 2018; Zhai et al., 2019). The increasingly serious nitrate pollution has emerged to be the new emphasis of air pollution controls in China.
Nitrate formation involves complex multiphase chemical reactions. In the daytime, nitrogen dioxide (NO2) reacts with the hydroxyl radical (OH) to produce nitric acid (HNO3). With excess ammonium (NH3), low temperature and insufficient sulfuric acid, this reaction can proceed quickly and produce high ammonium nitrate (Seinfeld and Pandis, 2006). In the nighttime, however, high-concentration NO2 reacts with ozone (O3) to produce the nitrate radical (NO3) and dinitrogen pentoxide (N2O5). The heterogeneous hydrolysis of N2O5 on wet particles is the main pathway for nocturnal nitrate formation (56 %–97 %) (He et al., 2018; Pathak et al., 2011; Xue et al., 2014).
Nitrate chemistry is not only related to the abundance of its precursor NOx, but it is also supported by the atmospheric oxidants (e.g., OH and O3) produced from the photochemical reactions of NOx and volatile organic compounds (VOCs) (Meng et al., 1997). Using a box model, some studies have determined that the relationship between particulate nitrate and NOx emissions is nonlinear depending on the ozone chemical sensitivity regime (Pun and Seigneur, 2001; Nguyen and Dabdub, 2002). Pun and Seigneur (2001) showed that the daytime HNO3 production was more sensitive to the concentrations of atmospheric oxidants and that in the VOC-limited regime the decrease of HNO3 production due to the NOx emission control might be offset by the increase of OH. Nguyen and Dabdub (2002) calculated the detailed isopleth between nitrate and NOx emissions; they found that the reduction of NOx emissions resulted in a decrease of nitrate in the NOx-limited regime, and an increase of nitrate under extreme conditions in the VOC-limited regime. Despite that, the single-site box model results could not distinguish the regional differences among chemical regimes; the basic hypotheses in box models to predict nitrate production are also unreasonable in the real atmosphere.
As an important precursor for both fine particles and ozone, strict control of NOx emissions has started in China since the 12th Five-Year Plan was established (Zheng et al., 2018). A confounding factor is that, for most cities in China, the production of O3 is usually limited by VOCs (Xie et al., 2014; Dong et al., 2014; Liu et al., 2010). The control of NOx emissions has therefore resulted in an increase of surface O3 concentrations in recent years (Li et al., 2021a; K. Li et al., 2019; Kalsoom et al., 2021), implying complex impacts on nitrate formation. Li et al. (2021a) and Liu and Wang (2020) examined the influencing factors on the surface O3 trends in China from 2013 to 2017 using regional chemical models. They highlighted that the control of NOx emissions explained 11 %–35 % of the increased O3 due to the nonlinear NOx–VOC–O3 chemistry and that for most regions the magnitudes could be comparable to those resulting from the meteorological influences and aerosol effects. Some simulations suggested that the NOx emission increase in 2005–2012 resulted in an increase of nitrate by 3.4 % yr−1 in eastern China (Geng et al., 2017; Wang et al., 2013), and the following NOx emission control resulted in a decrease of nitrate by 3 %–14 % (Wang et al., 2014). Recent evidence from field observations (Fu et al., 2020) and numerical simulations (Dong et al., 2014), however, has suggested that the NOx emission reduction in China could result in an increase of nitrate in winter through increased photochemical oxidants and nocturnal N2O5 chemistry but a decrease in other seasons. In the next 5–10 years, SO2 emissions might level off in China, while NOx emissions will become stringently controlled to ensure further air quality improvements (Zheng et al., 2018). Accurately understanding the nonlinear aerosol and photochemistry feedbacks is crucial to resolve the emerging nitrate pollution and to establish reasonable air pollution control strategies in China.
To address this issue, we use comprehensive measurements and a regional meteorology–chemistry model combined with hypothetical NOx emission scenarios to establish the nonlinear response relationships between particulate nitrate and NOx emission controls in the megalopolises of China. The model configurations, numerical designs and observational data are presented in Sect. 2. Section 3 discusses the results. Finally, a summary is presented in Sect. 4.
2.1 Model setup and experimental designs
This study uses the Weather Research and Forecasting-Chemistry (WRF-Chem) model version 4.1 developed by Grell et al. (2005) to simulate the regional meteorology and atmospheric chemistry. The mesoscale meteorology and air quality simulations of WRF-Chem have been improved in terms of incorporating the satellite-derived land surface parameters (Li et al., 2014, 2017) and optimizing the SIA formation pathways enhanced by mineral aerosols (M. M. Li et al., 2019; X. Huang et al., 2014).
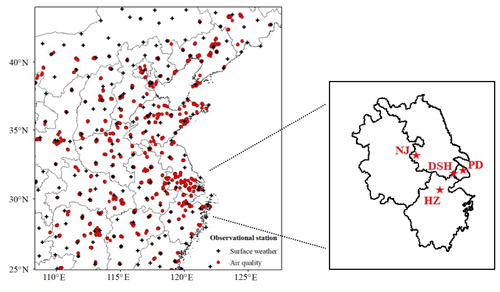
Figure 1WRF-Chem domain configuration and observational stations. Black crosses: surface weather stations; red dots: CNEMC routine air quality monitoring stations; red stars: surface supersites in YRD.
The modeling domain covers two main megalopolises of China and its adjacent areas – the Beijing–Tianjin–Hebei (BTH) region and the Yangtze River Delta (YRD) region (Fig. 1). The modeling framework is configured with 81 × 86 grid cells at 25 km horizontal resolution. The model is run with an 84 h model cycle, with the first 12 h discarded as spin-up time and model outputs of each model cycle to provide chemical initial conditions for the subsequent overlapping 84 h simulation. The 6 h, 1∘ × 1∘ National Centers for Environmental Prediction Final (NCEP/FNL) analysis fields are regularly input for the model initial and lateral boundary meteorological conditions.
The model physical configurations include the YSU boundary layer scheme (Noh et al., 2003), the RRTMG radiation scheme (Iacono et al., 2008), the Noah land surface scheme (Ek et al., 2003) and the Lin microphysics scheme (Lin et al., 1983). We have updated the land cover type and vegetation data in the WRF mesoscale model with the latest land surface parameters derived from the Moderate Resolution Imaging Spectroradiometer (MODIS; Li et al., 2014, 2017).
The atmospheric chemistry is simulated using the Carbon Bond Mechanism version Z (CBMZ) (Zaveri and Peters, 1999) gas-phase chemistry module coupled with the four-bin sectional Model for Simulating Aerosol Interactions and Chemistry (MOSAIC) (Zaveri et al., 2008). The aqueous-phase chemistry is based on the Carnegie Mellon University (CMU) scheme including 50 species and more than 100 reactions (Fahey and Pandis, 2001). Formation of SIA in the default WRF-Chem model accounts for the gas-phase oxidation of SO2 and NO2 and aqueous-phase oxidation of SO2 by hydrogen peroxide (H2O2) and O3 in cloud. We have optimized the SIA formation pathways by including the aqueous SO2 oxidation catalyzed by mineral ions and heterogeneous uptakes of SO2, NO2, NO3, N2O5 and HNO3 on mineral aerosols in the MOSAIC aerosol module (M. M.Li et al., 2019; X. Huang et al., 2014).
Anthropogenic emissions are adopted from the 2016 Multi-resolution Emission Inventory for China (MEIC) and the 2010 MIX-Asia emission inventory for regions outside of mainland China developed by Tsinghua University (http://meicmodel.org, last access: December 2020). Biogenic emissions are calculated online using the Model of Emissions of Gases and Aerosols from Nature (MEGAN; Guenther et al., 2006).
A series of WRF-Chem simulations is designed, as summarized in Table 1. In the baseline simulation (denoted as the B0 scenario), the anthropogenic emissions in China remain unchanged at the usual levels in 2016. Simulation N0 is the same as B0, but it only considers the gas-phase oxidation production of HNO3 (NO2 + OH → HNO3) and its subsequent partitioning to the aerosol phase of nitrate in WRF-Chem. The B0 and N0 simulations are combined to distinguish the contributions of gas-phase oxidation and heterogeneous pathways (i.e., uptakes of N2O5, NO3 and NO2) for the formation of nitrate aerosols during the warm and cold seasons. A group of sensitivity scenarios ( (N=10 %, 20 %, …, and 80 %)) are designed with the perturbed anthropogenic NOx emissions in China reduced by 10 %, 20 %, …, and 80 %, respectively. The differences between B0 and simulations are calculated to illustrate the responses of particulate pollution in China's megacities to the NOx emission reduction scenarios. Another simulation (E1) is designed with the anthropogenic emissions of NOx in China set to the 2012 levels to show the impacts of 2012–2016 NOx control strategy on particulate pollution. Additionally, in order to evaluate the effectiveness of multi-pollutant cooperative controls, three series of simulations (, and CVOCs−N, N=20 %, 40 %, 60 % and 80 %) are also supplemented with the anthropogenic emissions of SO2, NH3 and VOCs in China reduced by 20 %, 40 %, 60 % and 80 %, respectively. The differences between B0 and simulations are calculated to illustrate the responses of nitrate pollution in China's megacities to the multi-pollutant cooperative controls.
For all simulation scenarios, two month-long periods during the Campaign on Air Pollution and Urban Meteorology in Yangtze River Delta (CAPUM-YRD) – 15 August to 16 September (Period I) and 24 November to 26 December (Period II) in 2016 – are simulated to represent the warm and cold seasons, respectively (Shu et al., 2019). The complete simulation consists of 13 84 h model cycles, with the first 6 d as a spin-up for chemistry and the remaining model outputs for analysis.
2.2 Weather and air pollutant data
Surface meteorological observations at 186 land-based automatic stations across China (Fig. 1) are collected for model meteorological validation, including hourly data of 2 m air temperature, 2 m relative humidity and 10 m wind speed. These data are archived at the U.S. National Climatic Data Center (NCDC) (Smith et al., 2011).
Air pollutant data at the national air quality monitoring network and regional supersites of China (Fig. 1) are used for model chemical validation. This nationwide monitoring network contains 1597 sites covering 454 cities in mainland China, as shown in Fig. 1. Six routine air pollutants including PM2.5, particulate matter with aerodynamic diameter less than 10 µm (PM10), SO2, NO2, carbon monoxide (CO) and O3 are monitored and reported hourly by the Chinese National Environmental Monitoring Center (CNEMC) network (available at http://www.cnemc.cn, last access: December 2020).
Additionally, four comprehensive atmospheric environment supersites in YRD including Dianshanhu (DSH; 31.1∘ N, 121.0∘ E), Pudong (PD; 31.2∘ N, 121.5∘ E), Nanjing (NJ; 32.1∘ N, 118.8∘ E) and Hangzhou (HZ; 30.3∘ N, 120.2∘ E) measured the mass concentrations of PM2.5, water-soluble ions (sulfate, nitrate, ammonium, sodium, chloride, potassium, calcium and magnesium), carbonaceous aerosols (elemental carbon (EC) and organic carbon (OC)) and gaseous pollutants (SO2, NO2, CO and O3) during the CAPUM-YRD. Details for the methods and data at the four supersites are described in Shu et al. (2019).
3.1 Model weather and chemical validation
Model evaluations indicate that the WRF-Chem model is able to simulate the weather and atmospheric pollution characteristics in China. The simulated magnitudes of surface temperature by WRF-Chem in general agree with actual observations, with a correlation efficient (R) of 0.89 and 0.94 and a normalized mean bias (NMB) of −0.55 % and −0.80 %, respectively, in Period I and Period II (Table 2). Underestimation of relative humidity (−5.65 % in Period I and −6.56 % in Period II) is common in the WRF simulation, and it might be attributed to the influence of the boundary layer parameterization on the weather forecast (Bhati and Mohan, 2018; Gomez-Navarro et al., 2015). Clear overestimation of wind speed (23.72 % in Period I and 40.64 % in Period II) might be because of the unresolved topography in WRF (Jimenez et al., 2013; Li et al., 2014).
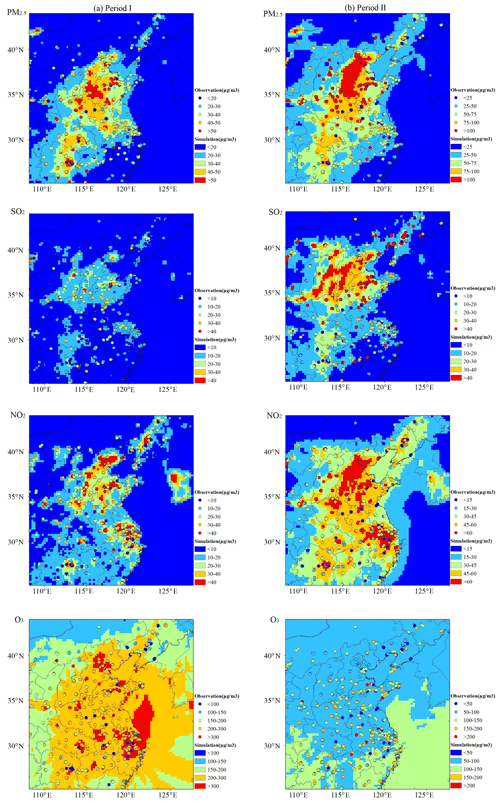
Figure 2Spatial patterns of the surface average PM2.5, NO2, SO2 and daily-maximum O3 concentrations in Period I (a) and Period II (b) from the WRF-Chem modeling (shaded contours) and routine air quality observations (dots).
The predicted concentrations of routine air pollutants also accurately capture the spatial and seasonal patterns of observed surface PM2.5, SO2, NO2 and O3 levels in both seasons (Fig. 2). Both simulations and observations display high air pollutant concentrations in the vicinity of the North China Plain (NCP) and eastern China but with higher O3 levels in the warm season and oppositely higher PM2.5 and other gaseous pollutant concentrations in winter. The model statistical evaluations show a mean bias (MB) of −3.66, −1.14, 4.70 and 18.32 µg m−3 and a NMB of −9.92, −6.46, 16.47 and 7.72 % for PM2.5, SO2, NO2 and O3 in Period I and a relatively larger MB of −27.31, −11.65, 1.27 and −39.01 µg m−3 and a NMB of −29.82, −28.11, 2.40 and −31.05 % in Period II, respectively (Table 3). The uncertainty in emissions data, the absence of secondary organic aerosol in MOSAIC aerosol chemistry or the simulated wind errors (Table 2) may be responsible for the larger atmospheric chemical biases in winter, which has been extensively discussed in some studies (Zhao et al., 2016; Li et al., 2021a).
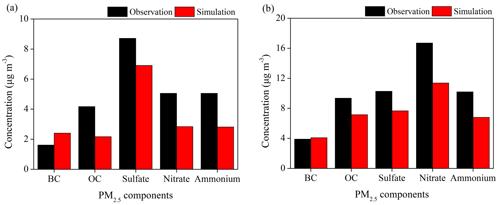
Figure 3Comparisons of surface PM2.5 components from WRF-Chem simulations and observations in Period I (a) and Period II (b) at the four supersites in YRD.
As the most important components of PM2.5, reasonable representation of SIA is imperative to the PM2.5 simulation. Evaluations with measurements of PM2.5 components at the four supersites of eastern China show that the model performs reasonably in simulating the seasonal variations and proportions of aerosol species in PM2.5, but it is biased low by 10 %–40 % in simulating the magnitudes of SIA concentrations (Fig. 3). The model underestimation is −1.8, −2.2 and −2.2 µg m−3 for sulfate, nitrate and ammonium, respectively, in Period I and −2.6, −4.3 and −3.4 µg m−3 in Period II. The model also captures the large change of ratio from the warm to cold seasons, that increases from 0.4 in Period I to 1.6 in Period II. Our previous work (Li et al., 2019) has confirmed that the consideration of the optimized aqueous and heterogeneous SIA formation pathways in WRF-Chem significantly reduces the model biases by 41.4 % for sulfate and 44.6 % for nitrate during the CAPUM-YRD of 2016. Recent studies highlighted that the remaining SIA simulation biases may be attributed to the missing aqueous oxidation of SO2 by NO2 on alkaline aerosols under humid conditions (Wang et al., 2016; Cheng et al., 2016).
3.2 Air pollution and aerosol composition characteristics
Chemical composition analyses of major gaseous and particulate air pollutants suggest large seasonal variations of air pollution characteristics in China (Fig. 2). Mainly emitted from combustion sources, atmospheric pollutants accumulate in the densely industrialized and populated megalopolises of China, with a hotspot along Beijing, Hebei, Shandong and their adjacent cities frequently exceeding China's National Ambient Air Quality Standards. The average concentrations of surface PM2.5, SO2, NO2 and daily-maximum O3 in China's routine air quality monitoring network are 33.8, 15.8, 26.5 and 223.2 µg m−3 for Period I and 80.2, 34.7, 47.7 and 131.4 µg m−3 for Period II. The surface PM2.5, SO2 and NO2 concentrations show obvious increases by 137.6 %, 119.2 % and 80.2 % during winter compared to those of the summer–autumn period (Period I). The maximum surface PM2.5 concentration recorded in the winter period was more than 600 µg m−3, which is the highest value ever recorded in 2016 and led to the “orange” air quality alert.
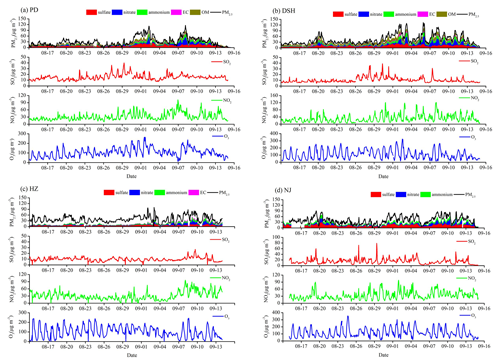
Figure 4Observed aerosol composition and gaseous pollutant concentrations at the four supersites during Period I.
The further analyses of PM2.5 mass concentrations, major PM2.5 components and gases at the four supersites in YRD are presented in Figs. 4–5. Organic matter (OM) is obtained by multiplying the OC concentrations by a factor of 1.6, mainly accounting for the hydrogen and oxygen masses in OM. The measured SIA concentrations exhibit high levels, with average values of 18.8 µg m−3 for Period I and 37.1 µg m−3 for Period II. The three SIA components together account for 32.3 %–57.4 % (48.6 % on average) and 27.7 %–70.9 % (56.9 % on average) of the total PM2.5 mass concentrations and become the primary components of PM2.5 in the two periods. The proportions of sulfate, nitrate and ammonium in total PM2.5 range from 13.5 %–28.9 %, 9.4 %–15.5 % and 9.4 %–14.9 % at the four supersites for Period I and 9.2 %–20.3 %, 11.5 %–32.1 % and 7.0 %–19.8 % for Period II, respectively. The strikingly higher proportion of nitrate than that of sulfate in PM2.5 during winter, with a ratio of 1.6, is in accordance with recent observations during other winter haze periods in China (Shao et al., 2018; Zhang et al., 2018; Zhang et al., 2019). They emphasized that since the enactment of the Clean Air Action Plan in 2013, the PM2.5 components have changed clearly, with decreasing contributions from coal combustion.
The high proportions of sulfate and nitrate in PM2.5 could be related to the high oxidation rates of SO2 and NO2. The observed average values of sulfur oxidation ratio (SOR = ) and nitrogen oxidation ratio (NOR = ) are 0.41 and 0.13 in Period I and 0.33 and 0.21 in Period II. In contrast, the observed SOR is generally higher in summer–autumn than winter, opposite to that of NOR, indicating the enhanced formation of nitrate in winter. Shu et al. (2019) also noted similar seasonal distinctions for SOR and NOR in YRD. They attributed the weakened conversion from NO2 to nitrate in summer to the volatility and evaporative loss of nitrate (Sun et al., 2012). The sharp increase of particles and moderate ambient humidity in winter also benefits the heterogeneous formation of SIA, leading to high NOR and SOR (Wang et al., 2012).
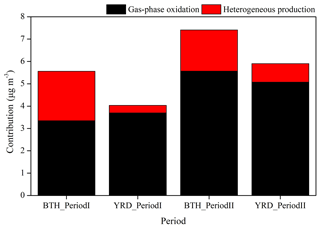
Figure 6Contributions of gas-phase oxidation and heterogeneous production to the surface nitrate concentrations for the BTH and YRD regions in two seasons.
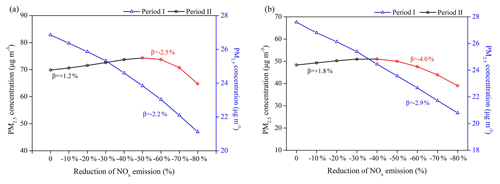
Figure 7Responses of surface PM2.5 concentrations to the NOx emission reduction scenarios in (a) BTH and (b) YRD. The calculated NOx emission control efficiency (β) is also marked in the figure.
Figure 6 illustrates the contributions of gas-phase oxidation and heterogeneous reactions for the nitrate production calculated from B0 and E0 simulations. It is shown that on a daily basis the gas-phase oxidation production of HNO3 and its subsequent partitioning to the aerosol phase is the principal formation route for particulate nitrate, with the average contributions of 60.2 % for BTH and 91.7 % for YRD in Period I and 75.1 % for BTH and 85.9 % for YRD in Period II. The heterogeneous hydrolyses of N2O5 and other nitrogenous gases (calculated as the model differences between B0 and N0 simulations) contribute to the remaining nitrate, particularly in BTH with high aerosol loading. These calculated results (60.2 %–91.7 % for NO2 + OH oxidation and 8.3 %–39.8 % for heterogeneous pathways) are in line with previous assessments in China and globally. Alexander et al. (2009) reported that the global tropospheric nitrate burden is dominated by NO2 + OH (76 %), followed by N2O5 hydrolysis (18 %), but recent results suggested that N2O5 hydrolysis was as important as NO2 + OH (both 41 %) for global nitrate production (Alexander et al., 2020). In major Chinese cities, it was estimated that the conversion of NOx to nitrate was dominated by NO2 + OH oxidation in Shanghai, with a mean contribution of 55 %–77 % in total and an even higher contribution (84 %–92 %) in summer (He et al., 2020). In the NCP, the nitrate contribution of heterogeneous pathways was about 30.8 % (Liu et al., 2020) or even comparable to the partitioning of HNO3 (Wang et al., 2019; H. C. Wang et al., 2017; Luo et al., 2021). The nitrate formation from heterogeneous pathways is moderately underestimated in the optimized WRF-Chem model of this study, possibly due to the uncertainties of heterogeneous uptake coefficients and unclear reaction mechanisms applied in the model (M. M. Li et al., 2019; Xue et al., 2016; He et al., 2014).
3.3 Nonlinear responses of nitrate to NOx emissions and their policy implications
3.3.1 PM2.5–NOx and O3–NOx responses in the warm and cold seasons
NOx is key in atmospheric chemistry and serves as an important precursor for both ozone and secondary aerosols. We conduct a series of simulations with perturbed NOx emissions to assess the responses of PM2.5 mass concentrations to NOx emissions in two megalopolises of China (Fig. 7). The WRF-Chem simulation results show that the responses of surface PM2.5 concentrations to NOx emissions vary in different seasons and display strong nonlinear behavior in winter. To better quantify their effectiveness, we define the NOx emission control efficiency (β), which denotes the percentage changes of surface PM2.5 or its component concentrations in response to the successive 10 % reduction of NOx emissions.
In Period I (August–September), the PM2.5–NOx responses are closer to a linear function, reflecting a stronger sensitivity to the NOx emission changes in the warm season. The surface PM2.5 concentrations decrease almost linearly as we gradually reduce NOx emissions in China, with the average β values of −2.2 % in BTH and −2.9 % in YRD. However, the PM2.5–NOx emission responses in Period II (November–December) display strong nonlinearity and are analogous to a quadratic parabola distribution for both regions. The NOx emission reductions within the first 50 % would even increase surface PM2.5 concentrations by +1.2 % averagely in BTH, and this β value increases to +1.8 % in YRD with the first 40 % reductions of NOx emissions. Subsequently, the PM2.5 responses shift towards a similar linear pattern, with an average β value of −2.5 % in BTH and −4.0 % in YRD.
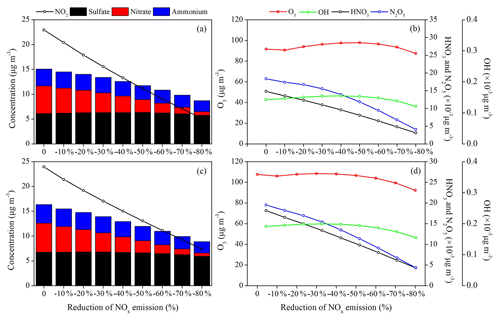
Figure 8Responses of the surface concentrations of SIA components and key atmospheric trace gases (NO2, O3, OH, HNO3 and NO3) to the NOx emission reduction scenarios in (a, b) BTH and (c, d) YRD during Period I.
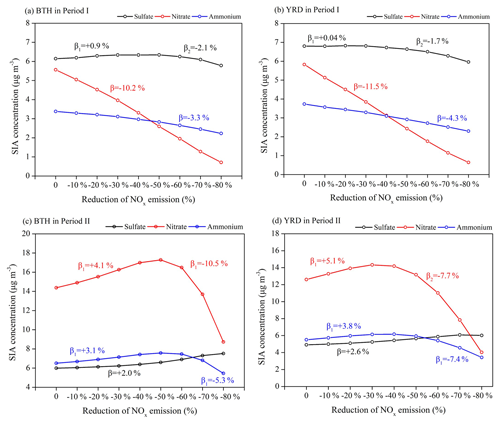
Figure 10Responses of the surface concentrations of SIA components to the NOx emission reduction scenarios and their emission control efficiencies in (a, b) Period I and (c, d) Period II.
The distinct forms of PM2.5–NOx emission responses for the warm and cold seasons are determined by the seasonal ozone chemical sensitivity regimes. The photochemical indicator of with a critical value of 1.0 is used to investigate the season-varying ozone sensitivity in China, which is calculated as the ratio of ozone concentration changes under 20 % NOx emission reduction to that under 20 % VOC emission reduction (Fig. S1 in the Supplement). The results indicate a strong VOC-limited ozone chemistry across China during winter while either a VOC-limited regime over a large portion of the NCP and eastern China or a NOx-limited regime in northern and western China during summer–autumn, as also indicated by previous studies (Xie et al., 2014; Dong et al., 2014; Liu et al., 2010). We find larger O3 and OH production under the NOx emission reduction conditions in both seasons (Figs. 8 and 9), particularly in Period II (November–December), with an average increase rate of +14.7 % and +18.5 % in BTH and +25.2 % and +23.1 % in YRD per 10 % reduction of NOx emissions. The SIA formation chemistry is highly limited by atmospheric oxidants produced from the NOx–VOC–O3 photochemical cycles. The nonlinear O3–NOx responses indicate a rather complicated aerosol and photochemistry feedback in megacities.
3.3.2 Nonlinear responses of particulate nitrate to NOx emissions
The SIA formation is basically driven by the atmospheric oxidant levels, and a reduction of NOx emissions may have counterintuitive effects on SIA components by controlling the atmospheric oxidant levels. The calculated SIA components for each emission scenario in both months show that the surface nitrate aerosols can be substantially decreased/increased with reducing NOx emissions, but the sulfate and ammonium concentrations have moderately smaller changes (Figs. 8–10).
Response of sulfate to the NOx emissions is more predictable and determined by the changes of atmospheric oxidant levels since the conversion of SO2 to sulfate is partly driven by OH in the gas phase and by dissolved H2O2 or O3 in the presence of fog or cloud. In Period I (August–September), the sulfate–NOx response follows a gradual quadratic parabola distribution as that of O3–NOx and OH–NOx response curves (Figs. 8 and 10), with a fitted function in Eq. (1). The β values for surface sulfate change by −0.7 %–+1.2 % in BTH and −1.5 %–+0.2 % in YRD under each NOx emission reduction scenario.
where [] is the surface mean concentration of sulfate (µg m−3), and is the percentage change of NOx emissions (%).
As expected, the production of nitrate reflects a strong sensitivity to NOx, and it decreases linearly with the NOx emission control, with an average β value of −10.2 % in BTH and −11.5 % in YRD, which further leads to a decrease of ammonium concentrations by −3.3 % in BTH and −4.3 % in YRD (Figs. 8 and 10). The formation of nitrate mainly involves the NO2 + OH → HNO3 gas-phase oxidation and the heterogeneous hydrolysis of N2O5 and other nitrogenous gases. The strong sensibility of particulate nitrate in response to the NOx emission decreases can be explained by the synchronously suppressive production of its intermediate products HNO3 and N2O5. For example, when the NOx emission is reduced by 20 %, the surface NO2 concentration in BTH drops by 20.0 %, but the surface O3 and OH concentrations increase slightly by 2.6 % and 5.3 % due to the reduction of NO+O3 titration reaction and the greater VOC availability in the warm season, leading to substantial reductions in surface HNO3 (−16.7 %) and N2O5 (−8.9 %) concentrations.
where [] and [] are the surface mean concentrations (µg m−3) of nitrate and ammonium, respectively.
In Period II (November–December), we find opposite results, with quadratic parabola distributions for the nitrate–NOx response (Eq. 2) and the ammonium–NOx response (Eq. 3) but linearly increasing sulfate concentrations (average β values of +2.0 % in BTH and +2.6 % in YRD; Figs. 9 and 10), leading to small PM2.5 changes in winter. Such nonlinear nitrate–NOx responses can be explained by the substantially increased oxidants as we gradually reduce NOx emissions in each scenario. It is noted that in winter the nitrate–NOx response highly depends on the production of N2O5, which is produced from the chemical reactions and is a crucial intermediate product for nitrate formation. Under the low NOx emission reduction conditions, the production of N2O5 is more sensitive to the atmospheric oxidant concentrations. The significant increases of surface O3 in each NOx emission scenario in the VOC-poor environment (Fig. 9b and d) lead to an enhancement of N2O5 levels from 10 % to more than 100 %. In spite of the HNO3 concentration remaining nearly unchanged or decreasing slightly by less than 5 % in response to NOx control, nitrate is found to increase (average β values of +4.1 % in BTH and +5.1 % in YRD), with higher N2O5 produced from the increased ozone introduced by attenuated titration. An inflexion point appears at the 30 %–50 % NOx emission reduction scenario, and a further reduction in NOx emissions is predicted to cause −10.5 % and −5.3 % reductions of surface particulate nitrate and ammonium for BTH and −7.7 % and −7.4 % for YRD.
These results reveal that the increase in atmospheric oxidants in response to NOx emission control can offset the decreasing precursors concentrations and further enhance the formation of secondary nitrate, as recently found during the COVID-19 pandemic (Huang et al., 2020; Li et al., 2021b).
3.3.3 Impacts of 2012–2016 NOx control strategy on particulate pollution
During the 12th Five-Year Plan period (2011–2015), a series of end-of-pipe pollutant controls (e.g., selective catalytic reduction techniques) were carried out for power, industry and transportation sectors. These measures effectively controlled the national NOx emissions by 22.8 % from 2012 to 2016 (MEIC v1.3) in China. To quantify the effects of recent NOx control measures on the levels of photochemical oxidants and particulate nitrate, we conduct an additional simulation with NOx emissions set to the levels of 2012 in E1.
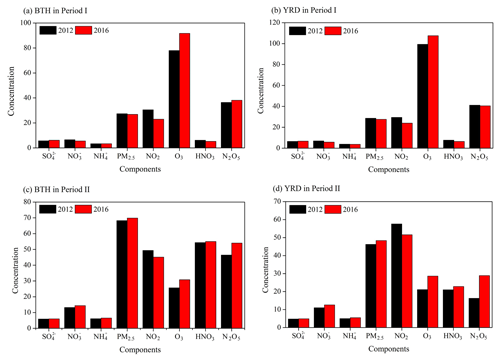
Figure 11Changes in the concentrations of surface PM2.5, SIA components and key atmospheric trace (NO2, O3, HNO3 and N2O5) due to the 2012–2016 NOx emission reductions in China, estimated as the differences between the base simulation and the E1 scenario. The units are parts per trillion (ppt) for HNO3 and N2O5 and micrograms per cubic meter (µg m−3) for other chemical species.
The model simulations (Fig. 11) suggest that reducing China's NOx emissions alone from 2012 to 2016 leads to an average −24.9 % to −8.6 % decrease of NOx concentrations in the surface layer. As previously pointed out, the 2012–2016 NOx emission control measures led to increased O3 and OH levels in winter, which offset the effectiveness of NOx emission reduction in alleviating winter nitrate. No obvious declines in the winter nitrate levels are observed and even increases in some areas (+8.8 % in BTH and 14.4 % in YRD; Figs. S2 and S3 in the Supplement). As shown, the largest PM2.5 responses shift towards the southern Hebei and central China provinces, where the wintertime PM2.5 concentrations are particularly high. The substantial emission changes from 2012 to 2016 lower the PM2.5 air pollution by up to −1.8 % in BTH and −3.5 % in YRD for Period I and oppositely increase the surface PM2.5 by 2.4 % in BTH and 4.7 % in YRD for Period II. The past NOx emission control strategy leads to increased atmospheric oxidant levels and deteriorated particulate pollution in winter due to the nonlinear photochemistry and aerosol chemical feedbacks, without regard of the other emission control measures. This conclusion is also supported by evidence from the recent field observations (Fu et al., 2020).
3.3.4 Responses of particulate nitrate to multi-pollutant cooperative controls
In order to evaluate the effectiveness of multi-pollutant cooperative controls in China, three series of additional simulations (, and CVOCs−N) are also designed to show the responses of nitrate and PM2.5 pollution to the emission controls of NOx, SO2, NH3 and VOCs, respectively. The results (Fig. 12) show that atmospheric NH3 and VOCs are effective in controlling the particulate nitrate pollution for both seasons, whereas decreasing the SO2 and NOx emissions may have counterintuitive effects on the concentration levels of nitrate aerosols.
Atmospheric NH3 acts as a critical neutralizing species for SIA production and efficient haze mitigation (Liu et al., 2019). According to the WRF-Chem simulation, reduction of NH3 emissions may be effective in reducing the nitrate component, with an average β value of −10.0 % in BTH and −10.3 % in YRD for Period I and −8.3 % in BTH and −11.5 % in YRD for Period II, primarily by suppressing the ammonium nitrate formation. Quantitatively, a 10 % reduction in NH3 emissions can alleviate the PM2.5 pollution by −2.7 % during summer–autumn and −3.2 % during winter in the two Chinese megacities. Atmospheric chemistry modeling by Wen et al. (2021) also indicated that controlling NH3 emissions in Beijing would significantly reduce the population-weighted PM2.5 concentrations by 6.2 %–21 %, with 60 %–100 % NH3 reductions in January, implying the need to consider NH3 emission controls when designing the PM2.5 pollution mitigation strategies.
VOCs, which are not a direct precursor for SIA, are effective in SIA controls due to their influences on the atmospheric oxidation cycles (Tsimpidi et al., 2008; Womack et al., 2019; Nguyen and Dabdub, 2002). Our results suggest that decreasing VOC emissions per 10 % would suppress the oxidation formation of nitrate and decrease the nitrate concentrations by −2.5 % in BTH and −1.7 % in YRD for Period I and −5.0 % in BTH and −6.3 % in YRD for Period II. The reduction of VOC emissions would result in a decrease of PM2.5 by −0.7 % during summer–autumn and −1.8 % during winter in the two megacities. Tsimpidi et al. (2008) also showed that the reduction of VOC emissions caused a marginal increase of PM2.5 during summer in the eastern United States, whereas it resulted in a decrease of atmospheric oxidant levels and 5 %–20 % reduction of both inorganic and organic PM2.5 components during winter. Larger and synchronized NOx and VOC emission controls are required to overcome the adverse effects of nonlinear photochemistry and aerosol chemical feedbacks.
The SO2 emission reduction, although effective in reducing sulfate and PM2.5, is not successful in regulating the nitrate pollution due to the chemical competition in nitrate and sulfate formations (Geng et al., 2017; Wang et al., 2013). Changes in nitrate concentration are linearly associated with the SO2 emission reductions, with the average β values of 2.9 % during summer–autumn and 1.3 % during winter. Decreasing SO2 emissions is less effective (a β value of −0.7 %) in mitigating the wintertime haze pollution because the benefit of SO2 reduction is partly offset by the significant increase of nitrate, demonstrating the critical role of multi-pollutant cooperative controls. Lei et al. (2013) evaluated the impacts of SO2 control strategies on nitrate and sulfate production in the United States and also found that the competition for bases in nitrate and sulfate formation significantly affects the nitrate concentrations.
Our results emphasize that future nitrate and PM2.5 pollution mitigation strategies should focus on reducing the chemical precursors and key atmospheric oxidants involved in the production of secondary aerosols. The recent “Three-Year Action Plan for Winning the Blue Sky Defense Battle” calls for stringent emission controls of NOx, SO2, VOCs and NH3 but without specific reduction targets. Such emission changes would emphasize the need to jointly consider multi-pollutant emission controls for mitigating haze air pollution.
Recent air pollution actions have significantly lowered the PM2.5 levels in China via controlling emissions of SO2 and NOx but raised a new question of how effective the NOx emission controls can be in the mitigation of emerging nitrate and ozone air pollution. We use comprehensive measurements and a regional meteorology–chemistry model with optimized mechanisms to establish the nonlinear responses between particulate nitrate and NOx emission controls in the megalopolises of China.
Nitrate is an essential component of PM2.5 in eastern China, accounting for 9.4 %–15.5 % and 11.5 %–32.1 % of the total PM2.5 mass for the warm and cold seasons, respectively. We find that the efficiency of PM2.5 reduction is highly sensitive to NOx emissions, and it varies in different seasons depending on the ozone chemical regimes. The reduction of NOx emissions results in almost linearly lower PM2.5 by −2.2 % in BTH and −2.9 % in YRD per 10 % reduction of NOx emissions during summer–autumn, whereas it increases the atmospheric oxidant levels and leads to a rather complicated response of the PM components in winter. Nitrate is found to increase (average β values of +4.1 % in BTH and +5.1 % in YRD) in winter with higher N2O5 intermediate produced from the increased ozone introduced by attenuated titration, despite the nearly unchanged or slightly decreased HNO3 concentrations in response to NOx control. An inflexion point appears at 30 %–50 % NOx emission reduction, and a further reduction of NOx emissions is predicted to cause −10.5 % reductions of particulate nitrate for BTH and −7.7 % for YRD. In addition, the 2012–2016 NOx emission control strategy leads to −24.9 % to −8.6 % decreases of surface NOx concentrations and no changes or even increases of wintertime nitrate in BTH (+8.8 %) and YRD (14.4 %). Our results also emphasize that atmospheric NH3 and VOCs are effective in controlling the particulate nitrate pollution, whereas decreasing the SO2 and NOx emissions may have counterintuitive effects on nitrate aerosols. These results provide insights for developing mitigation strategies for the ubiquitous nitrate aerosols in the winter haze of China.
The WRF-Chem model version 4.1 is available at https://www2.mmm.ucar.edu/wrf/users/download/get_source.html (last access: December 2020). The NCEP FNL data are accessible in the National Center for Atmospheric Research (NCAR) Research Data Archive (RDA; http://rda.ucar.edu/datasets/ds083.2/, last access: December 2020). The MEIC anthropogenic emission inventories are available at http://www.meicmodel.org (last access: December 2020). The surface weather data are accessible in the Integrated Surface Database (https://www.ncdc.noaa.gov/isd/data-access, last access: December 2020). The surface air pollutant and aerosol species data are provided by the Chinese National Environmental Monitoring Center (http://www.cnemc.cn/en/, last access: December 2020) and archived at https://doi.org/10.6084/m9.figshare.12818807.v1 (Li, 2020).
The supplement related to this article is available online at: https://doi.org/10.5194/acp-21-15135-2021-supplement.
ML developed the model code, designed the numerical experiments and wrote the original draft. ZZ carried out the numerical experiments. QY provided and analyzed some of the data. MX, SL and BZ validated and analyzed the model results. TW and YH reviewed and revised the manuscript.
The contact author has declared that neither they nor their co-authors have any competing interests.
Publisher's note: Copernicus Publications remains neutral with regard to jurisdictional claims in published maps and institutional affiliations.
This study is funded by the National Natural Science Foundation of China (41975153, 42077192 and 41775026), the National Key Basic Research Development Program of China (2019YFC0214603 and 2020YFA0607802) and the Emory University–Nanjing University Collaborative Research Grant.
This research has been supported by the National Natural Science Foundation of China (grant nos. 41975153, 42077192 and 41775026), the National Key Research and Development Program of China (grant nos. 2019YFC0214603 and 2020YFA0607802) and the Emory University–Nanjing University Collaborative Research Grant.
This paper was edited by Maria Kanakidou and reviewed by two anonymous referees.
Alexander, B., Hastings, M. G., Allman, D. J., Dachs, J., Thornton, J. A., and Kunasek, S. A.: Quantifying atmospheric nitrate formation pathways based on a global model of the oxygen isotopic composition (Δ17O) of atmospheric nitrate, Atmos. Chem. Phys., 9, 5043–5056, https://doi.org/10.5194/acp-9-5043-2009, 2009.
Alexander, B., Sherwen, T., Holmes, C. D., Fisher, J. A., Chen, Q., Evans, M. J., and Kasibhatla, P.: Global inorganic nitrate production mechanisms: comparison of a global model with nitrate isotope observations, Atmos. Chem. Phys., 20, 3859–3877, https://doi.org/10.5194/acp-20-3859-2020, 2020.
Bhati, S. and Mohan, M.: WRF-urban canopy model evaluation for the assessment of heat island and thermal comfort over an urban airshed in India under varying land use/land cover conditions, Geoscience Letters, 5, 27, https://doi.org/10.1186/s40562-018-0126-7, 2018.
Cheng, Y. F., Zheng, G. J., Wei, C., Mu, Q., Zheng, B., Wang, Z. B., Gao, M., Zhang, Q., He, K. B., Carmichael, G., Poschl, U., and Su, H.: Reactive nitrogen chemistry in aerosol water as a source of sulfate during haze events in China, Science Advances, 2, e1601530, https://doi.org/10.1126/sciadv.1601530, 2016.
Dong, X. Y., Li, J., Fu, J. S., Gao, Y., Huang, K., and Zhuang, G. S.: Inorganic aerosols responses to emission changes in Yangtze River Delta, China, Sci. Total Environ., 481, 522–532, 2014.
Ek, M. B., Mitchell, K. E., Lin, Y., Rogers, E., Grunmann, P., Koren, V., Gayno, G., and Tarpley, J. D.: Implementation of Noah land surface model advances in the National Centers for Environmental Prediction operational mesoscale Eta model, J. Geophys. Res.-Atmos., 108, 8851, https://doi.org/10.1029/2002jd003296, 2003.
Fahey, K. M. and Pandis, S. N.: Optimizing model performance: variable size resolution in cloud chemistry modeling, Atmos. Environ., 35, 4471–4478, 2001.
Fu, X., Wang, T., Gao, J., Wang, P., Liu, Y. M., Wang, S. X., Zhao, B., and Xue, L. K.: Persistent Heavy Winter Nitrate Pollution Driven by Increased Photochemical Oxidants in Northern China, Environ. Sci. Technol., 54, 3881–3889, 2020.
Geng, G., Zhang, Q., Tong, D., Li, M., Zheng, Y., Wang, S., and He, K.: Chemical composition of ambient PM2.5 over China and relationship to precursor emissions during 2005–2012, Atmos. Chem. Phys., 17, 9187–9203, https://doi.org/10.5194/acp-17-9187-2017, 2017.
Gómez-Navarro, J. J., Raible, C. C., and Dierer, S.: Sensitivity of the WRF model to PBL parametrisations and nesting techniques: evaluation of wind storms over complex terrain, Geosci. Model Dev., 8, 3349–3363, https://doi.org/10.5194/gmd-8-3349-2015, 2015.
Grell, G. A., Peckham, S. E., Schmitz, R., McKeen, S. A., Frost, G., Skamarock, W. C., and Eder, B.: Fully coupled “online” chemistry within the WRF model, Atmos. Environ., 39, 6957–6975, 2005.
Guenther, A., Karl, T., Harley, P., Wiedinmyer, C., Palmer, P. I., and Geron, C.: Estimates of global terrestrial isoprene emissions using MEGAN (Model of Emissions of Gases and Aerosols from Nature), Atmos. Chem. Phys., 6, 3181–3210, https://doi.org/10.5194/acp-6-3181-2006, 2006.
He, H., Wang, Y. S., Ma, Q. X., Ma, J. Z., Chu, B. W., Ji, D. S., Tang, G. Q., Liu, C., Zhang, H. X., and Hao, J. M.: Mineral dust and NOx promote the conversion of SO2 to sulfate in heavy pollution days, Sci. Rep.-UK, 4, 4172, https://doi.org/10.1038/Srep04172, 2014.
He, P., Xie, Z., Chi, X., Yu, X., Fan, S., Kang, H., Liu, C., and Zhan, H.: Atmospheric reveals nocturnal chemistry dominates nitrate production in Beijing haze, Atmos. Chem. Phys., 18, 14465–14476, https://doi.org/10.5194/acp-18-14465-2018, 2018.
He, P. Z., Xie, Z. Q., Yu, X. W., Wang, L. Q., Kang, H., and Yue, F. G.: The observation of isotopic compositions of atmospheric nitrate in Shanghai China and its implication for reactive nitrogen chemistry, Sci. Total Environ., 714, 136727, https://doi.org/10.1016/j.scitotenv.2020.136727, 2020.
Huang, R. J., Zhang, Y. L., Bozzetti, C., Ho, K. F., Cao, J. J., Han, Y. M., Daellenbach, K. R., Slowik, J. G., Platt, S. M., Canonaco, F., Zotter, P., Wolf, R., Pieber, S. M., Bruns, E. A., Crippa, M., Ciarelli, G., Piazzalunga, A., Schwikowski, M., Abbaszade, G., Schnelle-Kreis, J., Zimmermann, R., An, Z. S., Szidat, S., Baltensperger, U., El Haddad, I., and Prevot, A. S. H.: High secondary aerosol contribution to particulate pollution during haze events in China, Nature, 514, 218–222, 2014.
Huang, X., Song, Y., Zhao, C., Li, M. M., Zhu, T., Zhang, Q., and Zhang, X. Y.: Pathways of sulfate enhancement by natural and anthropogenic mineral aerosols in China, J. Geophys. Res.-Atmos., 119, 14165–14179, 2014.
Huang, X., Ding, A., Gao, J., Zheng, B., Zhou, D., Qi, X., Tang, R., Wang, J., Ren, C., Nie, W., Chi, X., Xu, Z., Chen, L., Li, Y., Che, F., Pang, N., Wang, H., Tong, D., Qin, W., Cheng, W., Liu, W., Fu, Q., Liu, B., Chai, F., Davis, S., Zhang, Q., and He, K.: Enhanced secondary pollution offset reduction of primary emissions during COVID-19 lockdown in China, Natl. Sci. Rev., 8, nwaa137, https://doi.org/10.1093/nsr/nwaa137, 2020.
Iacono, M. J., Delamere, J. S., Mlawer, E. J., Shephard, M. W., Clough, S. A., and Collins, W. D.: Radiative forcing by long-lived greenhouse gases: Calculations with the AER radiative transfer models, J. Geophys. Res.-Atmos., 113, D13103, https://doi.org/10.1029/2008jd009944, 2008.
Jimenez, P. A., Dudhia, J., Gonzalez-Rouco, J. F., Montavez, J. P., Garcia-Bustamante, E., Navarro, J., de Arellano, J. V. G., and Munoz-Roldan, A.: An evaluation of WRF's ability to reproduce the surface wind over complex terrain based on typical circulation patterns, J. Geophys. Res.-Atmos., 118, 7651–7669, 2013.
Kalsoom, U., Wang, T. J., Ma, C. Q., Shu, L., Huang, C. W., and Gao, L. B.: Quadrennial variability and trends of surface ozone across China during 2015–2018: A regional approach, Atmos. Environ., 245, 117989, https://doi.org/10.1016/j.atmosenv.2020.117989, 2021.
Lei, H. and Wuebbles, D. J.: Chemical competition in nitrate and sulfate formations and its effect on air quality, Atmos. Environ., 80, 472–477, 2013.
Li, K., Jacob, D. J., Liao, H., Shen, L., Zhang, Q., and Bates, K. H.: Anthropogenic drivers of 2013-2017 trends in summer surface ozone in China, P. Natl. Acad. Sci. USA, 116, 422–427, 2019.
Li, M.: Air qaulity in recent years.zip, figshare [data set], https://doi.org/10.6084/m9.figshare.12818807.v1, 2020.
Li, M. M., Song, Y., Huang, X., Li, J. F., Mao, Y., Zhu, T., Cai, X. H., and Liu, B.: Improving mesoscale modeling using satellite-derived land surface parameters in the Pearl River Delta region, China, J. Geophys. Res.-Atmos., 119, 6325–6346, 2014.
Li, M. M., Wang, T. J., Xie, M., Zhuang, B. L., Li, S., Han, Y., Song, Y., and Cheng, N. L.: Improved meteorology and ozone air quality simulations using MODIS land surface parameters in the Yangtze River Delta urban cluster, China, J. Geophys. Res.-Atmos., 122, 3116–3140, 2017.
Li, M. M., Wang, T. J., Xie, M., Li, S., Zhuang, B. L., Huang, X., Chen, P. L., Zhao, M., and Liu, J. E.: Formation and Evolution Mechanisms for Two Extreme Haze Episodes in the Yangtze River Delta Region of China During Winter 2016, J. Geophys. Res.-Atmos., 124, 3607–3623, 2019.
Li, M. M., Wang, T. J., Shu, L., Qu, Y. W., Xie, M., Liu, J. N., Wu, H., and Kalsoom, U.: Rising surface ozone in China from 2013 to 2017: A response to the recent atmospheric warming or pollutant controls?, Atmos. Environ., 246, 118130, https://doi.org/10.1016/j.atmosenv.2020.118130, 2021a.
Li, M. M., Wang, T. J., Xie, M., Li, S., Zhuang, B. L., Fu, Q. Y., Zhao, M., Wu, H., Liu, J., Saikawa, E., and Liao, K.: Drivers for the poor air quality conditions in North China Plain during the COVID-19 outbreak, Atmos. Environ., 246, 118103, https://doi.org/10.1016/j.atmosenv.2020.118103, 2021b.
Lin, Y. L., Farley, R. D., and Orville, H. D.: Bulk Parameterization of the Snow Field in a Cloud Model, J. Clim. Appl. Meteorol., 22, 1065–1092, 1983.
Liu, L., Bei, N. F., Hu, B., Wu, J. R., Liu, S. X., Li, X., Wang, R. N., Liu, Z. R., Shen, Z. X., and Li, G. H.: Wintertime nitrate formation pathways in the north China plain: Importance of N2O5 heterogeneous hydrolysis, Environ. Pollut., 266, 115287, https://doi.org/10.1016/j.envpol.2020.115287, 2020.
Liu, M. X., Huang, X., Song, Y., Tang, J., Cao, J. J., Zhang, X. Y., Zhang, Q., Wang, S. X., Xu, T. T., Kang, L., Cai, X. H., Zhang, H. S., Yang, F. M., Wang, H. B., Yu, J. Z., Lau, A. K. H., He, L. Y., Huang, X. F., Duan, L., Ding, A. J., Xue, L. K., Gao, J., Liu, B., and Zhu, T.: Ammonia emission control in China would mitigate haze pollution and nitrogen deposition, but worsen acid rain, P. Natl. Acad. Sci. USA, 116, 7760–7765, 2019.
Liu, X. H., Zhang, Y., Xing, J., Zhang, Q. A., Wang, K., Streets, D. G., Jang, C., Wang, W. X., and Hao, J. M.: Understanding of regional air pollution over China using CMAQ, part II. Process analysis and sensitivity of ozone and particulate matter to precursor emissions, Atmos. Environ., 44, 3719–3727, 2010.
Liu, Y. and Wang, T.: Worsening urban ozone pollution in China from 2013 to 2017 – Part 2: The effects of emission changes and implications for multi-pollutant control, Atmos. Chem. Phys., 20, 6323–6337, https://doi.org/10.5194/acp-20-6323-2020, 2020.
Luo, L., Zhu, R. G., Song, C. B., Peng, J. F., Guo, W., Liu, Y. H., Zheng, N. J., Xiao, H. W., and Xiao, H. Y.: Changes in nitrate accumulation mechanisms as PM2.5 levels increase on the North China Plain: A perspective from the dual isotopic compositions of nitrate, Chemosphere, 263, 127915, https://doi.org/10.1016/j.chemosphere.2020.127915, 2021.
Meng, Z., Dabdub, D., and Seinfeld, J. H.: Chemical coupling between atmospheric ozone and particulate matter, Science, 277, 116–119, 1997.
Nguyen, K. and Dabdub, D.: NOx and VOC control and its effects on the formation of aerosols, Aerosol. Sci. Tech., 36, 560–572, 2002.
Noh, Y., Cheon, W. G., Hong, S. Y., and Raasch, S.: Improvement of the K-profile model for the planetary boundary layer based on large eddy simulation data, Bound.-Lay. Meteorol., 107, 401–427, 2003.
Pathak, R. K., Wang, T., and Wu, W. S.: Nighttime enhancement of PM2.5 nitrate in ammonia-poor atmospheric conditions in Beijing and Shanghai: Plausible contributions of heterogeneous hydrolysis of N2O5 and HNO3 partitioning, Atmos. Environ., 45, 1183–1191, 2011.
Pun, B. K. and Seigneur, C.: Sensitivity of particulate matter nitrate formation to precursor emissions in the California San Joaquin Valley, Environ. Sci. Technol., 35, 2979–2987, 2001.
Seinfeld, J. H. and Pandis, S. N.: Atmospheric chemistry and physics: from air pollution to climate change, 2nd edn., John Wiley and Sons, Hoboken, NJ, 2006.
Shao, P. Y., Tian, H. Z., Sun, Y. J., Liu, H. J., Wu, B. B., Liu, S. H., Liu, X. Y., Wu, Y. M., Liang, W. Z., Wang, Y., Gao, J. J., Xue, Y. F., Bai, X. X., Liu, W., Lin, S. M., and Hu, G. Z.: Characterizing remarkable changes of severe haze events and chemical compositions in multi-size airborne particles (PM1, PM2.5 and PM10) from January 2013 to 2016-2017 winter in Beijing, China, Atmos. Environ., 189, 133–144, 2018.
Shu, L., Wang, T. J., Xie, M., Li, M. M., Zhao, M., Zhang, M., and Zhao, X. Y.: Episode study of fine particle and ozone during the CAPUM-YRD over Yangtze River Delta of China: Characteristics and source attribution, Atmos. Environ., 203, 87–101, 2019.
Silver, B., Reddington, C. L., Arnold, S. R., and Spracklen, D. V.: Substantial changes in air pollution across China during 2015-2017, Environ. Res. Lett., 14, 114012, https://doi.org/10.1088/1748-9326/aae718, 2018.
Smith, A., Lott, N., and Vose, R.: The Integrated Surface Database Recent Developments and Partnerships, B. Am. Meteorol. Soc., 92, 704–708, 2011.
Sun, Y. L., Wang, Z. F., Dong, H. B., Yang, T., Li, J., Pan, X. L., Chen, P., and Jayne, J. T.: Characterization of summer organic and inorganic aerosols in Beijing, China with an Aerosol Chemical Speciation Monitor, Atmos. Environ., 51, 250–259, 2012.
Tsimpidi, A. P., Karydis, V. A., and Pandis, S. N.: Response of Fine Particulate Matter to Emission Changes of Oxides of Nitrogen and-Anthropogenic Volatile Organic Compounds in the Eastern United States, J. Air Waste Manage., 58, 1463–1473, 2008.
Wang, G. H., Zhang, R. Y., Gomez, M. E., Yang, L. X., Zamora, M. L., Hu, M., Lin, Y., Peng, J. F., Guo, S., Meng, J. J., Li, J. J., Cheng, C. L., Hu, T. F., Ren, Y. Q., Wang, Y. S., Gao, J., Cao, J. J., An, Z. S., Zhou, W. J., Li, G. H., Wang, J. Y., Tian, P. F., Marrero-Ortiz, W., Secrest, J., Du, Z. F., Zheng, J., Shang, D. J., Zeng, L. M., Shao, M., Wang, W. G., Huang, Y., Wang, Y., Zhu, Y. J., Li, Y. X., Hu, J. X., Pan, B., Cai, L., Cheng, Y. T., Ji, Y. M., Zhang, F., Rosenfeld, D., Liss, P. S., Duce, R. A., Kolb, C. E., and Molina, M. J.: Persistent sulfate formation from London Fog to Chinese haze, P. Natl. Acad. Sci. USA, 113, 13630–13635, 2016.
Wang, H. C., Lu, K. D., Chen, X. R., Zhu, Q. D., Chen, Q., Guo, S., Jiang, M. Q., Li, X., Shang, D. J., Tan, Z. F., Wu, Y. S., Wu, Z. J., Zou, Q., Zheng, Y., Zeng, L. M., Zhu, T., Hu, M., and Zhang, Y. H.: High N2O5 Concentrations Observed in Urban Beijing: Implications of a Large Nitrate Formation Pathway, Environ. Sci. Tech. Let., 4, 416–420, 2017.
Wang, J. D., Zhao, B., Wang, S. X., Yang, F. M., Xing, J., Morawska, L., Ding, A. J., Kulmala, M., Kerminen, V. M., Kujansuu, J., Wang, Z. F., Ding, D. A., Zhang, X. Y., Wang, H. B., Tian, M., Petaja, T., Jiang, J. K., and Hao, J. M.: Particulate matter pollution over China and the effects of control policies, Sci. Total Environ., 584, 426–447, 2017.
Wang, S. X., Xing, J., Zhao, B., Jang, C., and Hao, J. M.: Effectiveness of national air pollution control policies on the air quality in metropolitan areas of China, J. Environ. Sci., 26, 13–22, 2014.
Wang, X. F., Wang, W. X., Yang, L. X., Gao, X. M., Nie, W., Yu, Y. C., Xu, P. J., Zhou, Y., and Wang, Z.: The secondary formation of inorganic aerosols in the droplet mode through heterogeneous aqueous reactions under haze conditions, Atmos. Environ., 63, 68–76, 2012.
Wang, Y., Zhang, Q. Q., He, K., Zhang, Q., and Chai, L.: Sulfate-nitrate-ammonium aerosols over China: response to 2000–2015 emission changes of sulfur dioxide, nitrogen oxides, and ammonia, Atmos. Chem. Phys., 13, 2635–2652, https://doi.org/10.5194/acp-13-2635-2013, 2013.
Wang, Y. L., Song, W., Yang, W., Sun, X. C., Tong, Y. D., Wang, X. M., Liu, C. Q., Bai, Z. P., and Liu, X. Y.: Influences of Atmospheric Pollution on the Contributions of Major Oxidation Pathways to PM2.5 Nitrate Formation in Beijing, J. Geophys. Res.-Atmos., 124, 4174–4185, 2019.
Wen, L., Xue, L., Wang, X., Xu, C., Chen, T., Yang, L., Wang, T., Zhang, Q., and Wang, W.: Summertime fine particulate nitrate pollution in the North China Plain: increasing trends, formation mechanisms and implications for control policy, Atmos. Chem. Phys., 18, 11261–11275, https://doi.org/10.5194/acp-18-11261-2018, 2018.
Wen, Z., Xu, W., Pan, X. Y., Han, M. J., Wang, C., Benedict, K., Tang, A. H., Collet, J. L., and Liu, X. J.: Effects of reactive nitrogen gases on the aerosol formation in Beijing from late autumn to early spring, Environ. Res. Lett., 16, 025005, https://doi.org/10.1088/1748-9326/abd973, 2021.
Womack, C. C., McDuffie, E. E., Edwards, P. M., Bares, R., de Gouw, J. A., Docherty, K. S., Dube, W. P., Fibiger, D. L., Franchin, A., Gilman, J. B., Goldberger, L., Lee, B. H., Lin, J. C., Lone, R., Middlebrook, A. M., Millet, D. B., Moravek, A., Murphy, J. G., Quinn, P. K., Riedel, T. P., Roberts, J. M., Thornton, J. A., Valin, L. C., Veres, P. R., Whitehill, A. R., Wild, R. J., Warneke, C., Yuan, B., Baasandorj, M., and Brown, S. S.: An Odd Oxygen Framework for Wintertime Ammonium Nitrate Aerosol Pollution in Urban Areas: NOx and VOC Control as Mitigation Strategies, Geophys. Res. Lett., 46, 4971–4979, 2019.
Xie, M., Zhu, K. G., Wang, T. J., Yang, H. M., Zhuang, B. L., Li, S., Li, M. G., Zhu, X. S., and Ouyang, Y.: Application of photochemical indicators to evaluate ozone nonlinear chemistry and pollution control countermeasure in China, Atmos. Environ., 99, 466–473, 2014.
Xue, J., Yuan, Z. B., Lau, A. K. H., and Yu, J. Z.: Insights into factors affecting nitrate in PM2.5 in a polluted high NOx environment through hourly observations and size distribution measurements, J. Geophys. Res.-Atmos., 119, 4888–4902, 2014.
Xue, J., Yuan, Z. B., Griffith, S. M., Yu, X., Lau, A. K. H., and Yu, J. Z.: Sulfate Formation Enhanced by a Cocktail of High NOx, SO2, Particulate Matter, and Droplet pH during Haze-Fog Events in Megacities in China: An Observation-Based Modeling Investigation, Environ. Sci. Technol., 50, 7325–7334, 2016.
Zaveri, R. A. and Peters, L. K.: A new lumped structure photochemical mechanism for large-scale applications, J. Geophys. Res.-Atmos., 104, 30387–30415, 1999.
Zaveri, R. A., Easter, R. C., Fast, J. D., and Peters, L. K.: Model for Simulating Aerosol Interactions and Chemistry (MOSAIC), J. Geophys. Res.-Atmos., 113, D13204, https://doi.org/10.1029/2007jd008782, 2008.
Zhai, S., Jacob, D. J., Wang, X., Shen, L., Li, K., Zhang, Y., Gui, K., Zhao, T., and Liao, H.: Fine particulate matter (PM2.5) trends in China, 2013–2018: separating contributions from anthropogenic emissions and meteorology, Atmos. Chem. Phys., 19, 11031–11041, https://doi.org/10.5194/acp-19-11031-2019, 2019.
Zhang, W. Q., Tong, S. R., Ge, M. F., An, J. L., Shi, Z. B., Hou, S. Q., Xia, K. H., Qu, Y., Zhang, H. X., Chu, B. W., Sun, Y. L., and He, H.: Variations and sources of nitrous acid (HONO) during a severe pollution episode in Beijing in winter 2016, Sci. Total Environ., 648, 253–262, 2019.
Zhang, Y. M., Wang, Y. Q., Zhang, X. Y., Shen, X. J., Sun, J. Y., Wu, L. Y., Zhang, Z. X., and Che, H. C.: Chemical Components, Variation, and Source Identification of PM1 during the Heavy Air Pollution Episodes in Beijing in December 2016, J. Meteorol. Res.-PRC, 32, 1–13, 2018.
Zhao, M. F., Xiu, G. L., Qiao, T., Li, Y. L., and Yu, J. Z.: Characteristics of Haze Pollution Episodes and Analysis of a Typical Winter Haze Process in Shanghai, Aerosol Air Qual. Res., 16, 1625–1637, 2016.
Zhao, P. S., Dong, F., He, D., Zhao, X. J., Zhang, X. L., Zhang, W. Z., Yao, Q., and Liu, H. Y.: Characteristics of concentrations and chemical compositions for PM2.5 in the region of Beijing, Tianjin, and Hebei, China, Atmos. Chem. Phys., 13, 4631–4644, https://doi.org/10.5194/acp-13-4631-2013, 2013.
Zheng, B., Tong, D., Li, M., Liu, F., Hong, C., Geng, G., Li, H., Li, X., Peng, L., Qi, J., Yan, L., Zhang, Y., Zhao, H., Zheng, Y., He, K., and Zhang, Q.: Trends in China's anthropogenic emissions since 2010 as the consequence of clean air actions, Atmos. Chem. Phys., 18, 14095–14111, https://doi.org/10.5194/acp-18-14095-2018, 2018.