the Creative Commons Attribution 4.0 License.
the Creative Commons Attribution 4.0 License.
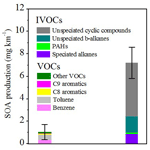
Measurement report: Emissions of intermediate-volatility organic compounds from vehicles under real-world driving conditions in an urban tunnel
Hua Fang
Xiaoqing Huang
Chenglei Pei
Zuzhao Huang
Yujun Wang
Yanning Chen
Jianhong Yan
Jianqiang Zeng
Shaoxuan Xiao
Shilu Luo
Sheng Li
Jun Wang
Ming Zhu
Xuewei Fu
Zhenfeng Wu
Runqi Zhang
Wei Song
Guohua Zhang
Weiwei Hu
Mingjin Tang
Xiang Ding
Xinhui Bi
Intermediate-volatility organic compounds (IVOCs) emitted from vehicles are important precursors to secondary organic aerosols (SOAs) in urban areas, yet vehicular emission of IVOCs, particularly from on-road fleets, is poorly understood. Here we initiated a field campaign to collect IVOCs with sorption tubes at both the inlet and the outlet in a busy urban tunnel (>30 000 vehicles per day) in south China for characterizing emissions of IVOCs from on-road vehicles. The average emission factor of IVOCs (EFIVOCs) was measured to be 16.77±0.89 mg km−1 (average ±95 % CI, confidence interval) for diesel and gasoline vehicles in the fleets, and based on linear regression, the average EFIVOCs was derived to be 62.79±18.37 mg km−1 for diesel vehicles and 13.95±1.13 mg km−1 for gasoline vehicles. The EFIVOCs for diesel vehicles from this study was comparable to that reported previously for non-road engines without after-treatment facilities, while the EFIVOCs for gasoline vehicles from this study was much higher than that recently tested for a China V gasoline vehicle. IVOCs from the on-road fleets did not show significant correlation with the primary organic aerosol (POA) or total non-methane hydrocarbons (NMHCs) as results from previous chassis dynamometer tests. Estimated SOA production from the vehicular IVOCs and VOCs surpassed the POA by a factor of ∼2.4, and IVOCs dominated over VOCs in estimated SOA production by a factor of ∼7, suggesting that controlling IVOCs is of greater importance to modulate traffic-related organic aerosol (OA) in urban areas. The results demonstrated that although on-road gasoline vehicles have much lower EFIVOCs, they contribute more IVOCs than on-road diesel vehicles due to its dominance in the on-road fleets. However, due to greater diesel than gasoline fuel consumption in China, emission of IVOCs from diesel engines would be much larger than that from gasoline engines, signaling the overwhelming contribution of IVOC emissions by non-road diesel engines in China.
- Article
(886 KB) -
Supplement
(541 KB) - BibTeX
- EndNote
Intermediate-volatility organic compounds (IVOCs) refer to organics with effective saturated concentrations ranging from 103 to 106 µg m−3, roughly corresponding to the volatility range of C12–C22 normal alkanes (n-alkanes) (Donahue et al., 2006; Zhao et al., 2014). Robinson et al. (2007) have demonstrated that IVOCs, as the missing secondary organic aerosol (SOA) precursors in many model studies, could efficiently narrow the gap between model-predicted and field-observed SOA. Smog chamber studies involving individual IVOC species, like higher n-alkanes and two-ring aromatics, have confirmed their significantly higher SOA formation potentials (Chan et al., 2009; Presto et al., 2010; Liu et al., 2015). In addition, recent model simulations including IVOCs as SOA precursors revealed that 30 %–80 % of ambient SOA could be explained by IVOCs (Ots et al., 2016; Zhao et al., 2016; Yang et al., 2019; Lu et al., 2020; Huang et al., 2020). However, due to a lack of direct measurements, these model simulations used the ratios of IVOCs to other species like primary organic aerosol (POA) or non-methane hydrocarbons (NMHCs) to estimate IVOC emissions.
Vehicular emission is an important anthropogenic source of IVOCs especially in urban environments (Tkacik et al., 2014; Jathar et al., 2014; Cross et al., 2015; Zhao et al., 2015, 2016; Ots et al., 2016). IVOCs could account for ∼60 % of non-methane hydrocarbons (NMHCs) from diesel vehicles and 4 %–17 % from gasoline vehicles, explaining a dominant portion of estimated SOA mass from diesel and gasoline exhaust (Zhao et al., 2015, 2016). Previous chamber simulations on SOA formation from vehicle exhaust revealed that traditional volatile organic compounds (VOCs) could not explain the formed SOA, and IVOCs instead might dominate the SOA production (Deng et al., 2020; Zhang et al., 2020). In megacities like London, diesel-emitted IVOCs alone could contribute ∼30 % SOA formed in ambient air (Ots et al., 2016). Therefore, for the control of fine particle pollution in urban areas, it is necessary to compile and upgrade emission inventories for IVOCs, and more work is needed to characterize their emissions from on-road vehicles.
Although previous chassis dynamometer tests used limited numbers of vehicles to characterize IVOC emissions (Zhao et al., 2015, 2016; Tang et al., 2021), the results obtained from the tests were widely applied to recent models and emission inventories (Liu et al., 2017; Lu et al., 2018; Wu et al., 2019; Huang et al., 2020). However, driving conditions significantly influence vehicular IVOC emissions (Drozd et al., 2018; Tang et al., 2021); therefore, emissions of IVOCs under real-world driving conditions may be quite different from that measured with chassis dynamometers. The tunnel test is a widely used method to characterize vehicle emissions in light of its advantage in capturing real-world emissions with a large number of driving vehicles. The emissions of PM2.5, carbonaceous aerosols, VOCs, NOx, and NH3 from on-road vehicles have been widely studied based on tunnel tests (Liu et al., 2014; Zhang et al., 2016, 2017, 2018). However, to the best of our knowledge, until present no reports are available about vehicular emission factors of IVOCs through tunnel tests.
In China, the number of on-road vehicles reached 348 million in 2019, which is more than double that in 2009 (http://www.mee.gov.cn/hjzl/sthjzk/ydyhjgl/, last access: 1 July 2021). However, emissions of IVOCs from mobile sources in China are very understudied. Only very recently, Tang et al. (2021) tested emissions of IVOCs from a China V light-duty gasoline vehicle. As IVOC emission factors derived from vehicle tests in the US have been used to update China's emission inventories with the inclusion of IVOCs (Liu et al., 2017), it is unknown whether the borrowed emission factors could well reflect the vehicular emissions of IVOCs in China. On the other hand, although China has made great achievements in combating air pollution in recent years, fine particle pollution is still an air quality problem in many of China's cities (Wang et al., 2020). As organic matter is often the most abundant component in PM2.5, and SOA pollution is increasingly standing out with the intensified primary emission control (Guo et al., 2020), understanding IVOC emissions from on-road vehicles is of great importance given that vehicle-emitted IVOCs contribute greatly to urban SOA formation (Gentner et al., 2012; Wu et al., 2019; Huang et al., 2020).
In this study, the emissions of IVOCs from on-road vehicles under real-world driving conditions were characterized through tests in an urban tunnel in Guangzhou, which is a megacity in southern China. The study aims to (1) investigate chemical compositions and volatility of IVOCs from on-road driving vehicles; (2) obtain average IVOC emission factors for the on-road fleet based on tests in the tunnel; (3) retrieve average IVOC emission factors for gasoline- and diesel-fueled vehicles by regression analysis, taking advantage of a large number of vehicles (>30 000 per day) passing the tunnel; and (4) compare the SOA formation potential of vehicle-emitted IVOCs to that of vehicle-emitted VOCs measured in the same campaign.
2.1 Field sampling
The sampling campaign was concurrently conducted at both the inlet and at the outlet of the Zhujiang tunnel (23∘6′ N, 113∘14′ E), which is located in urban Guangzhou, southern China (Fig. S1), on three weekdays (14–16 October 2019) and two weekend days (13 and 19 October 2019). A detailed description of the Zhujiang tunnel can be found in our previous studies (Liu et al., 2014; Zhang et al., 2016, 2017, 2018). IVOCs were collected by a sorption tube (Tenax TA/Carbograph 5TD, Marks International Ltd., UK) using an automatic sampler (JEC921, Jectec Science and Technology, Co., Ltd., Beijing, China). A Teflon filter was installed before the tube to remove particles in the air flow. The sampling flow rate was set at 0.6 L min−1, and hourly samples were collected from 05:00 to 24:00 LT on each sampling day. In order to compare SOA production from IVOCs and VOCs, hourly VOC samples were collected on 13 October 2019 with stainless-steel canisters at a flow rate of 66.7 mL min−1 using a model 910 pressurized canister sampler (Xonteck, Inc., CA, USA). Two-hour quartz filter samples were also collected by a high-volume PM2.5 sampler (Thermo Electron, Inc., USA) at the outlet and inlet sampling sites from 13 to 19 October. Trace gases were measured by online analyzers (CO, model 48i, Thermo Electron Inc., USA; NOx, model 42i, Thermo Electron Inc., USA). A video camera was installed at the inlet to record the vehicle flow during the campaign. After sampling, the video data were used to count the passing vehicles and classify vehicles into different fuel types.
2.2 Laboratory analysis
Sampled sorption tubes were analyzed by a thermal desorption (TD) system (TD-100, Markes International Ltd., UK) coupled to a gas chromatography and mass selective detector (GC/MSD; 7890 GC/5975 MSD, Agilent Technologies, USA) with a capillary column (HP-5MS, , Agilent Technologies, USA). Deuterated standards (C12-d26, C16-d34, C20-d42, naphthalene-d8, acenaphthene-d10, and phenanthrene-d10) were injected into the sorption tubes to determine their recoveries before analysis. The sampled sorption tubes and field blanks were thermally desorbed at 320 ∘C for 20 min, and the desorbed compounds were carried by high-purity helium into a cryogenic trap at −10 ∘C; then the trap was rapidly heated to transfer them into the GC/MSD system. The initial temperature of the GC oven was set at 65 ∘C, held for 2 min, then increased to 290 ∘C at 5 ∘C min−1, and kept at 290 ∘C for 20 min. The MSD was used in the SCAN mode with an electron impacting ionization at 70 eV.
Individual speciated IVOCs were quantified with the calibration curves by using authentic standards. The total IVOC mass was determined using the approach developed by Zhao et al. (2014, 2015, 2016), and a detailed description is provided in the Supplement (Sect. S1). Briefly, the total ion chromatogram (TIC) of IVOCs was divided into 11 bins based on the retention times of C12–C22 n-alkanes. Each bin centered on the retention time of an n-alkane. The start time and end times of the bin were determined by the average retention time of two successive n-alkanes. For example, the start time of bin 16 (B16) was calculated as the average retention time of n-C15 and n-C16, and the end time of B16 as the average retention time of n-C16 and n-C17. The IVOC mass in each bin was quantified by the response factor of n-alkane in the same bin. The total IVOC mass was the sum of IVOC masses determined in the 11 bins. The mass of unresolved complex mixtures of IVOCs (UCM-IVOCs) was determined by the difference between the total IVOCs and speciated IVOCs in each bin. The UCM-IVOCs were further classified into unspeciated branch alkanes (b-alkanes) and cyclic compounds (Zhao et al., 2014) (Sect. S1). The analysis of VOCs can be found elsewhere (Zhang et al., 2018). The POA emission was estimated as 1.2 times that of organic carbon that was measured in quartz filter samples (Zhao et al., 2015), which were analyzed by an OC/EC analyzer (DRI model 2015, Nevada, USA) (Li et al., 2018).
2.3 Quality assurance and quality control (QA/QC)
Before their use for field sampling, sorption tubes were conditioned at 320 ∘C for 2 h in an oxygen-free nitrogen flow and then sealed at both ends with brass storage caps fitted with PTFE ferrules. About 15 % of conditioned tubes were selected randomly to be analyzed in the same way as normal samples to check if any targeted species existed in the tubes. The batch of sorption tubes were certified as clean if speciated IVOCs were not found or presented in levels below the method detection limits (MDLs). Before and after sampling, the flow rates of samplers were calibrated by a soap-membrane flowmeter (Gilian Gilibrator-2, Sensidyne, USA). During the sampling, 10 field blanks (five at the inlet and five at the outlet) were collected by installing a sorption tube onto the sampler each day but with pump off at both the inlet and the outlet. The speciated IVOCs were not detected or presented in levels below their MDLs in the blanks. MDLs for all the speciated IVOCs, including n-alkanes and polycyclic aromatic hydrocarbons (PAHs), were below 8 ng m−3, such as 5.8 ng m−3 for n-C12, 5.9 ng m−3 for n-C16, and 4.7 ng m−3 for n-C22. To check if any breakthrough occurred during the sampling, prior to the field campaign two sorption tubes were connected in series to sample at the tunnel outlet station in the same way. IVOCs detected in the second tube only accounted for 2.6±1.4 % of the total in the two tubes, indicating negligible breakthrough during the sampling. To check the recoveries during thermodesorption, selected sampled sorption tubes were analyzed twice by the TD-GC/MS system, and the desorption recoveries, calculated as the percentage of IVOCs in first analysis, were 96.7±3.2 % on average. Duplicated samples revealed less than 15 % differences for all the speciated IVOCs.
2.4 Calculation of IVOC emission factors
The vehicular EF of IVOCs can be calculated by the following equation (Pierson and Brachaczek, 1983; Zhang et al., 2016, 2017, 2018):
where EF (mg km−1 veh.−1) is the fleet-average emission factor of a given species during the time interval T (1 h in this study); ΔC (mg m−3) is the inlet–outlet incremental concentration of IVOCs; Vair (m s−1) is wind speed parallel to the tunnel measured by a 3-D sonic anemometer (Campbell, Inc.); A (m2) is the cross-sectional area of the tunnel; N is the number of vehicles traveling through the tunnel during the time interval T; and l (km) is the distance between the outlet and the inlet.
3.1 Emission factors and compositions of IVOCs
Figure 1 shows diurnal variations of vehicle numbers and vehicular IVOC emission factors (EFIVOCs) during the campaign. Traffic flow in the tunnel varied between 571 and 2263 vehicles per hour during the campaign, and gasoline vehicles (GVs) dominated the vehicle fleets with a share of 76.3 % on average; diesel vehicles (DVs) only accounted for 4.0 %, and other types of vehicles, including liquefied petroleum gas vehicles (LPGVs) and electrical vehicles (EVs), had an average percentage of 18.7 % (Fig. S2). As LPGVs and EVs are considered to have no IVOC emissions (Stewart et al., 2021), only GVs and DVs are responsible for the inlet–outlet incremental concentrations of IVOCs. Based on the above Eq. (1), fleet-average EFIVOCs (GVs + DVs) ranged from 13.29±5.08 mg km−1 veh.−1 to 21.40±5.01 mg km−1 veh.−1, with an average of 16.77±0.89 mg km−1 veh.−1 (average ±95 % CI, confidence interval) (Fig. 1). The average EFIVOCs for DVs and GVs could be further derived through linear regression as below (Ho et al., 2007; Kramer et al., 2020):
where EFIVOCs represents the fleet-average emission factor measured during a time interval; EFDV and EFGV are the average EFIVOCs for DVs and GVs, respectively; and α is the fraction of DVs in the total IVOC-emitting DVs and GVs traveling through the tunnel. Based on the regression results (Fig. S3), the average EFIVOCs for DVs (62.79±18.37 mg km−1 veh.−1) was ∼4.5 times that for GVs (13.95±1.13 mg km−1 veh.−1).
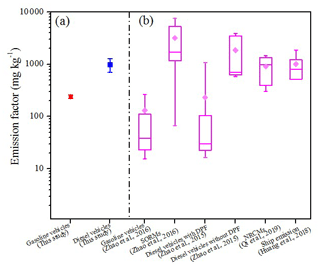
Figure 2Comparison of the EFIVOCs measured in this study with that previously measured for fossil fuel combustion sources. The error bars in (a) represent 95 % confidence interval. In (b), the boxes represent the 75th and 25th percentiles, the centerlines are the medians, and squares are the averages. The whiskers represent the 10th and 90th percentiles. SORMs refer to small off-road machines fueled with gasoline. NRCMs represent non-road construction machines fueled with diesel.
The mileage-based EF can be converted to fuel-based EF with the fuel density and fuel efficiency (Sect. S2) (Zhang et al., 2016). Thus, we could obtain an average fuel-based EFIVOCs of 239.5±19.5 mg kg−1 for GVs and 984.9±288.2 mg kg−1 for DVs. Zhao et al. (2015, 2016) measured IVOC emissions from DVs and GVs in the US by dynamometer tests. As shown in Fig. 2, the average EFIVOCs for DVs measured in our study was significantly lower than that for DVs without any diesel particulate filter (DPF) in the US but over 4 times higher than that with DPF. It is worth noting that the EFIVOCs for DVs from this study was comparable to that for ships and non-road construction machines (NRCMs) with diesel-fueled engines in China (Fig. 2) (Huang et al., 2018; Qi et al., 2019). As a matter of fact, China III or lower emission standard DVs accounted for ∼40 % of China's total in-use DVs in 2019 (http://www.mee.gov.cn/xxgk2018/xxgk/xxgk13/202012/t20201201_810776.html, last access: 1 July 2021), and like the non-road engines, they are not equipped with any after-treatment facilities. Although the after-treatment systems are installed in the China IV and China V DVs, their working performance might be not so satisfactory (Wu et al., 2017). This may explain why the DVs in this study had IVOC EFs comparable to non-road engines. The EFIVOCs for GVs from this study fell into the ranges of that for GVs in the US but was at the high end of the tested values (Fig. 2). A recent study revealed a significantly lower EFIVOCs of 83.7 mg kg−1 for a China V gasoline vehicle (Tang et al., 2021), implying that upgrading the emission standard could help reduce emissions of IVOCs from GVs, as China IV and China III GVs still share a much larger portion than the China V and China VI ones in China's on-road fleets (http://www.mee.gov.cn/hjzl/sthjzk/ydyhjgl/201909/P020190905586230826402.pdf, last access: 1 July 2021).
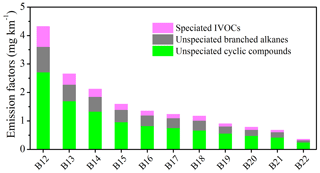
Figure 3The average emission factor of vehicular IVOCs in different bins measured during the campaign.
Figure 3 shows the EFs and compositions of the vehicular IVOCs in each retention-time-based bin (Table S1). Similar to previous studies (Zhao et al., 2015, 2016; Huang et al., 2018; Qi et al., 2019; Tang et al., 2021), the unspeciated cyclic compounds dominated the IVOCs, accounting for 59.07±1.06 %, followed by unspeciated b-alkanes (25.27±0.75 %) and speciated IVOCs (15.66±0.60 %). Among the speciated IVOCs (Table S1), naphthalene dominated the quantified PAHs, accounting for 56.82±1.21 % of total PAH emissions. The distribution of IVOCs in retention-time-based bins presented a significant decreasing trend with bin numbers. Previous studies have reported that more than 50 % of IVOCs concentrated in higher-volatility bins like B12, B13, and B14 in gasoline exhaust, while much broader volatility distributions were found in diesel exhaust (Zhao et al., 2015, 2016; Tang et al., 2021). The IVOCs in B12 measured in this study was also the most abundant as the GVs previously tested in the US (Fig. S4). This was reasonable since the GVs dominated the vehicle fleets during our tunnel experiments (Fig. S2). As shown in Fig. S5, the IVOCs determined in each volatility bin well correlated with those in the volatility bins close to them, and the total IVOCs have stronger correlations with IVOCs in the higher-volatility bins like B12, B13, and B14. In addition, the n-alkanes, as displayed in Table S2, were found to be significantly correlated to the total IVOCs that determined in the same volatility bins except for B20 and n-C20. The mass ratios of IVOCs to the n-alkane in the bins ranged from 9.0 to 15.8 (Table S2). As n-alkanes can be more easily and routinely quantified, the relationships of IVOCs and n-alkanes in each volatility bin might be used to estimate total IVOCs from on-road vehicles. However, vehicles types should be taken into consideration when using these ratios, as the results here were obtained for a fleet dominated by GVs.
3.2 Relationships between IVOCs and other species
Emissions of IVOCs from vehicles are often estimated by assuming a ratio of IVOCs to other species such as POA or NMHCs (Shrivastava et al., 2008; Pye and Seinfeld, 2010; Gentner et al., 2012; Murphy et al., 2017; Wu et al., 2019). However, these ratios might be highly variable with fuel types, operation conditions, and engine performance (Lu et al., 2018). As demonstrated in Fig. S6a and b, IVOCs correlated well with NOx (R=0.63, p<0.05) and CO (R=0.58, p<0.05), with an average IVOC-to-NOx ratio of 0.039±0.004 and an average IVOC-to-CO ratio of 0.033±0.015. The measured IVOC-to-POA ratio was 3.35±1.79 (Fig. S6c), which is comparable to that of 3.0±0.9 for GVs previously measured in dynamometer tests simulating arterial and freeway cycles but much higher than that of 1.5 previously used for estimating vehicle emissions in models (Robinson et al., 2007; Hodzic et al., 2010). As shown in Fig. S6d, the average IVOC-to-NMHC ratio measured in this study was 0.36±0.09, which is lower than that previously measured for diesel vehicle exhaust (0.6±0.1) (Zhao et al., 2015) but higher than that previously measured for gasoline vehicle exhaust (<0.2) (Zhao et al., 2016; Tang et al., 2021). It is worth noting that the IVOCs did not present significant correlations with POA or NMHCs from this study for on-road vehicle fleets (Fig. S6c and d). This would cast uncertainty over the emission estimates of IVOCs based on their ratios to POA or NMHCs.
3.3 Estimated SOA production from IVOCs
SOA formation potentials of IVOCs from the on-road vehicle fleet as measured in this tunnel study can be estimated as
where SOAFP is the SOA formation potential from the gaseous precursors; EFi represents the emission factor of precursor i; and Yi is the SOA yield of precursor i under high NOx at OA concentration of 20 µg m−3 (Zhao et al., 2015; Huang et al., 2018; Qi et al., 2019; Tang et al., 2021) (Table S3). As shown in Fig. 4, the SOA formation potentials from vehicular VOCs and IVOCs totaled 8.24±0.68 mg km−1. The SOA-to-POA ratio was 2.41±1.45, which was comparable to that of GVs tested in China (1.8–4.4) (Tang et al., 2021) and that of GVs (3.6) (Zhao et al., 2016) and high-speed DVs (3.2±1.7) without DPF in the US (Zhao et al., 2015). Our previous chamber studies simulating SOA formation from vehicle exhausts revealed the SOA-to-POA ratios of 2.0 for DVs and 3.8 for GVs when cruising at 40 km h−1 (Deng et al., 2020; Zhang et al., 2020), which is near the average driving speed of vehicles in the tunnel. Among the vehicle-emitted SOA precursors, similar to previous studies (Zhao et al., 2015, 2016; Huang et al., 2018; Qi et al., 2019; Tang et al., 2021), IVOCs produced significantly higher SOA (7.19±0.62 mg km−1), which is ∼7 times that from traditional VOCs (1.04±0.30 mg km−1). Previous smog chamber studies found that SOA formed during photochemical aging of vehicle exhaust could not be explained by traditional VOCs, especially for vehicles cruising at higher speeds (Robinson et al., 2007; Deng et al., 2020; Zhang et al., 2020). If this SOAIVOCs-to-SOAVOCs ratio of 7 from this study is used to re-estimate the SOA formation from exhaust for vehicles cruising at 40 km h−1 in our previous chamber studies (Deng et al., 2020; Zhang et al., 2020), the VOC plus IVOC precursors could explain 91 %–98 % SOA formed for GVs and 31.2 %–48.2 % SOA formed for DVs. Zhao et al. (2015, 2016) reported significantly higher SOAIVOCs-to-SOAVOCs ratios for diesel vehicle exhaust than gasoline vehicle exhaust. Furthermore, we also resolved the SOAIVOCs-to-SOAVOCs ratios for DVs and GVs via liner regression (Sect. S3). As shown in Fig. S7, although the correlation between SOAIVOCs-to-SOAVOCs ratios and DV fractions was not significant, the DVs did present a much higher average SOAIVOCs-to-SOAVOCs ratio (54.9) than that of GVs (6.82). Thus, SOAIVOCs-to-SOAVOCs ratio of 7 obtained from this study in a tunnel dominated by GVs would underestimate SOAIVOCs from DVs, consistent with higher NMHCs to IVOCs ratios in gasoline exhaust than in diesel exhaust (Zhao et al., 2015, 2016; Huang et al., 2018; Qi et al., 2019; Tang et al., 2021). Overall, the observed vehicular IVOCs as SOA precursors can help achieve mass closure between predicted and measured SOA.
Organic aerosol (OA), primary or secondary, accounts for a large fraction of particle matter (Zhang et al., 2007; Jimenez et al., 2009). On-road vehicles could be an important source of OA especially in urban environments (Gentner et al., 2017). Similar to previous smog chamber simulation results about SOA formed from photochemical aging of vehicle exhaust (Deng et al., 2020; Zhang et al., 2020), our tunnel test also demonstrated that estimated SOA surpassed the POA emission. In addition, IVOCs were found to dominate over traditional VOCs in SOA formation potentials by a factor of ∼7, implying that reducing vehicle-emitted IVOCs is of greater importance to modulate SOA for further reducing fine particle pollution particularly in urban areas. As for the ratios of IVOCs to other primary species, our tunnel tests for the on-road fleet revealed that, although the ratios of IVOC to POA and IVOC to NMHC were comparable to those from previous chassis dynamometer tests, no significant positive correlations were found between IVOCs and POA or NMHCs in our tunnel measurements. This implied that caution should be taken when applying the ratios from chassis dynamometer tests to estimate real-world traffic emissions or applying the ratios in the US to estimate the emissions in China or other regions. As IVOCs are not considered in normal vehicle emission tests, more field work characterizing real-world vehicular emissions of IVOCs is needed to further constrain these ratios.
EFIVOCs for the GV-dominated fleets from our tunnel test or EFIVOCs for GVs derived from regression was much higher than that from a recent chassis test for a China V gasoline vehicle (Tang et al., 2021), suggesting that stricter emission standards might help reduce emissions of IVOCs from GVs. Meanwhile, the EFIVOCs for on-road DVs was comparable to that for non-road engines without any after-treatment devices (Huang et al., 2018; Qi et al., 2019), suggesting that facilitating the installation of after-treatment devices with stricter emission standards or improving the performance of existing after-treatment devices is crucial to lower IVOC emissions from DVs, which have much higher EFIVOCs than GVs.
Based on the regression-derived average EFIVOCs for GVs and DVs and the camera-recorded fleet compositions, we could estimate that ∼81 % of IVOCs by vehicles traveling through the tunnel were coming from GVs and only ∼19 % were from DVs (Table S4). This is reasonable since DVs have higher EFIVOCs but much lower proportions in the fleets. These percentages may underestimate the contribution to IVOCs by on-road DVs at regional or national scales since DVs travel less in core urban areas due to traffic restriction rules in China. Differently, in an updated emission inventory of vehicular IVOCs in China (Liu et al., 2017) based on EFIVOCs tested in the US, emissions of IVOCs from DVs (145.07 Gg) were about 2.6 times that from GVs (55.30 Gg) in China in 2015. However, the ratio of DV EFIVOCs to GV EFIVOCs used in the study (Liu et al., 2017) on average was much higher than that of ∼4.5 from this study for on-road vehicles. Using the EFIVOCs from tests in the US might underestimate IVOC emissions from GVs but overestimate those from DVs in China. As an example, EFIVOCs of 83.7 mg kg−1 reported very recently for a China V gasoline vehicle (Tang et al., 2021) was still much higher than the maximum EFIVOCs (47.15 mg kg−1) they adopted for China V GVs, and the EFIVOCs used for China III and China IV DVs were however significantly larger than those from our tunnel tests (Fig. 2) for on-road DVs (mostly China III and China IV) (Liu et al., 2017). In 2019 the gasoline and diesel fuel consumptions in China were 1.20×102 and 1.50×102 Tg, respectively (http://www.mee.gov.cn/hjzl/sthjzk/ydyhjgl/, last access: 1 July 2021). Since gasoline is mostly used for on-road vehicles, diesel may be used for both on-road and non-road engines, and EFIVOCs for on-road diesel vehicles is comparable to that for non-road diesel engines (Huang et al., 2018; Qi et al., 2019), we could use the fuel-based EFIVOCs converted from our study to roughly estimate IVOCs from diesel and gasoline combustion. This way, the estimated emission of IVOCs from diesel engines (147.74 Gg) was about 5 times that from gasoline engines (28.74 Gg) in China in 2019 (Table S4). In comparison to a previous study (Liu et al., 2017), this result implies large uncertainties or even inconsistencies about China's vehicular IVOC emission estimates. Moreover, as diesel vehicles share less than 10 % among China's motor vehicles, and a substantial part of diesel fuel is consumed by non-road engines, the diesel-related IVOCs may largely come overwhelmingly from non-road engines instead of on-road DVs, signaling the increasingly important role of non-road engines as sources of IVOCs with the progress in on-road vehicular emission control.
The dataset for this paper is available upon request from the corresponding author Xinming Wang (wangxm@gig.ac.cn).
The supplement related to this article is available online at: https://doi.org/10.5194/acp-21-10005-2021-supplement.
XW and YZ designed the campaign and provided the funding supports. HF and XH analyzed the samples. HF wrote the paper. GZ, WH, MT, XD, and XB provided suggestions for this paper. XW revised and edited the paper. The others in author list conducted the field work.
The authors declare that they have no conflict of interest.
Publisher's note: Copernicus Publications remains neutral with regard to jurisdictional claims in published maps and institutional affiliations.
The authors gratefully acknowledge the Guangzhou Ecological and Environmental Monitoring Center of Guangdong Province and the Guangzhou Tunnel Development Company for their support during the tunnel experiment.
This work has been funded by the Natural Science Foundation of China (grant nos. 41961144029 and 42022023), the Chinese Academy of Sciences (grant nos. QYZDJ-SSW-DQC032, XDA23010303, and XDA23020301), the Youth Innovation Promotion Association, CAS (grant no. 2017406), the Hong Kong Theme-base project (grant no. T24-504/17-N), and the Department of Science and Technology of Guangdong Province (grant nos. 2020B1212060053, 2017BT01Z134, and 2019B121205006).
This paper was edited by Jianzhong Ma and reviewed by two anonymous referees.
Chan, A. W. H., Kautzman, K. E., Chhabra, P. S., Surratt, J. D., Chan, M. N., Crounse, J. D., Kürten, A., Wennberg, P. O., Flagan, R. C., and Seinfeld, J. H.: Secondary organic aerosol formation from photooxidation of naphthalene and alkylnaphthalenes: implications for oxidation of intermediate volatility organic compounds (IVOCs), Atmos. Chem. Phys., 9, 3049–3060, https://doi.org/10.5194/acp-9-3049-2009, 2009.
Cross, E. S., Sappok, A., Wong, V., and Kroll, J. H.: Load-dependent emission factors and chemical characteristics of IVOCs from a medium-duty diesel engine, Environ. Sci. Technol., 49, 13483–13491, https://doi.org/10.1021/acs.est.5b03954, 2015.
Deng, W., Fang, Z., Wang, Z. Y., Zhu, M., Zhang, Y. L., Tang, M. J., Song, W., Lowther, S., Huang, Z. H., Jones, K., Peng, P. A., and Wang, X. M.: Primary emissions and secondary organic aerosol formation from in-use diesel vehicle exhaust: comparison between idling and cruise mode, Sci. Total Environ., 699, 134357, https://doi.org/10.1016/j.scitotenv.2019.134357, 2020.
Donahue, N. M., Robinson, A. L., Stanier, C. O., and Pandis, S. N.: Coupled partitioning, dilution, and chemical aging of semivolatile organics, Environ. Sci. Technol., 40, 2635–2643, https://doi.org/10.1021/es052297c, 2006.
Drozd, G. T., Zhao, Y. L., Saliba, G., Frodin, B., Maddox, C., Oliver Chang, M. C., Maldonado, H., Sardar, S., Weber, R. J., Robinson, A. L., and Goldstein, A. H.: Detailed speciation of intermediate volatility and semivolatile organic compound emissions from gasoline vehicles: effects of cold-starts and implications for secondary organic aerosol formation, Environ. Sci. Technol., 53, 1706–1714, https://doi.org/10.1021/acs.est.8b05600, 2018.
Gentner, D. R., Isaacman, G., Worton, D. R., Chan, A. W. H., Dallmann, T. R., Davis, L., Liu, S., Day, D. A., Russell, L. M., Wilson, K. R., Weber, R., Guha, A., Harley, R. A., and Goldstein, A. H.: Elucidating secondary organic aerosol from diesel and gasoline vehicles through detailed characterization of organic carbon emissions, P. Natl. Acad. Sci. USA, 109, 18318–18323, https://doi.org/10.1073/pnas.1212272109, 2012.
Gentner, D. R., Jathar, S. H., Gordon, T, D., Bahreini, R., Day, D. A., EI Haddad, I., Hays, P. L., Pieber, S. M., Platt, S. M., de Gouw, J., Goldstein, A. H., Harley, R. A., Jimenez, J. L., Prévôt, A. S. H., and Robinson, A. L.: Review of urban secondary organic aerosol formation from gasoline and diesel motor vehicle emissions, Environ. Sci. Technol., 51, 1074–1093, https://doi.org/10.1021/acs.est.6b04509, 2017.
Guo, J., Zhou, S., Cai, M., Zhao, J., Song, W., Zhao, W., Hu, W., Sun, Y., He, Y., Yang, C., Xu, X., Zhang, Z., Cheng, P., Fan, Q., Hang, J., Fan, S., Wang, X., and Wang, X.: Characterization of submicron particles by time-of-flight aerosol chemical speciation monitor (ToF-ACSM) during wintertime: aerosol composition, sources, and chemical processes in Guangzhou, China, Atmos. Chem. Phys., 20, 7595–7615, https://doi.org/10.5194/acp-20-7595-2020, 2020.
Ho, K. F., Ho, S. S. H., Cheng, Y., Lee, S. C., and Yu, J. Z.: Real-world emission factors of fifteen carbonyl compounds measured in a Hong Kong tunnel, Atmos. Environ., 41, 1747–1758, https://doi.org/10.1016/j.atmosenv.2006.10.027, 2007.
Hodzic, A., Jimenez, J. L., Madronich, S., Canagaratna, M. R., DeCarlo, P. F., Kleinman, L., and Fast, J.: Modeling organic aerosols in a megacity: potential contribution of semi-volatile and intermediate volatility primary organic compounds to secondary organic aerosol formation, Atmos. Chem. Phys., 10, 5491–5514, https://doi.org/10.5194/acp-10-5491-2010, 2010.
Huang, C., Hu, Q. Y., Li, Y. J., Tian, J. J., Ma, Y. G., Zhao, Y. L., Feng, J. L., An, J. Y., Qiao, L. P., Wang, H. L., Jing, S. A., Huang, D. D., Lou, S. R., Zhou, M., Zhu, S. H., Tao, S. K., and Li, L.: Intermediate volatility organic compound emissions from a large cargo vessel operated under real-world conditions, Environ. Sci. Technol., 52, 12934–12942, https://doi.org/10.1021/acs.est.8b04418, 2018.
Huang, L., Wang, Q., Wang, Y. J., Emery, C., Zhu, A. S., Zhu, Y. H., Yin, S. J., Yarwood, G., Zhang, K., and Li, L.: Simulation of secondary organic aerosol over the Yangtze River Delta region: the impacts from the emissions of intermediate volatility organic compounds and the SOA modeling framework, Atmos. Environ., 246, 118079, https://doi.org/10.1016/j.atmosenv.2020.118079, 2020.
Jathar, S. H., Gordon, T. D., Hennigan, C. J., Pye, H. O., Pouliot, G., Adams, P. J., Donahue, N. M., and Robinson, A. L.: Unspeciated organic emissions from combustion sources and their influence on the secondary organic aerosol budget in the United States, P. Natl. Acad. Sci. USA, 111, 10473–10478, https://doi.org/10.1073/pnas.1323740111, 2014.
Jimenez, J. L., Canagaratna, M. R., Donahue, N. M., Prevot, A. S. H., Zhang, Q., Kroll, J. H., DeCarlo, P. F., Allan, J. D., Coe, H., Ng, N. L., Aiken, A. C., Docherty, K. S., Ulbrich, I. M., Grieshop, A. P., Robinson, A. L., Duplissy, J., Smith, J. D., Wilson, K. R., Lanz, V. A., Hueglin, C., Sun, Y. L., Tian, J., Laaksonen, A., Raatikainen, T., Rautiainen, J., Vaattovaara, P., Ehn, M., Kulmala, M., Tomlinson, J. M., Collins, D. R., Cubison, M. J., Dunlea, E. J., Huffman, J. A., Onasch, T. B., Alfarra, M. R., Williams, P. I., Bower, K., Kondo, Y., Schneider, J., Drewnick, F., Borrmann, S., Weimer, S., Demerjian, K., Salcedo, D., Cottrell, L., Griffin, R., Takami, A., Miyoshi, T., Hatakeyama, S., Shimono, A., Sun, J. Y., Zhang, Y. M., Dzepina, K., Kimmel, J. R., Sueper, D., Jayne, J. T., Herndon, S. C., Trimborn, A. M., Williams, L. R., Wood, E. C., Middlebrook, A. M., Kolb, C. E., Baltensperger, U., and Worsnop, D. R.: Evolution of organic aerosols in the atmosphere, Science, 326, 1525–1529, https://doi.org/10.1126/science.1180353, 2009.
Kramer, L. J., Crilley, L. R., Adams, T. J., Ball, S. M., Pope, F. D., and Bloss, W. J.: Nitrous acid (HONO) emissions under real-world driving conditions from vehicles in a UK road tunnel, Atmos. Chem. Phys., 20, 5231–5248, https://doi.org/10.5194/acp-20-5231-2020, 2020.
Li, S., Zhu, M., Yang, W. Q., Tang, M. J., Huang, X. L., Yu, Y. G., Fang, H., Yu, X., Yu, Q. Q., Fu, X. X.; Song, W., Zhang, Y. L., Bi, X. H., and Wang, X. M.: Filter-based measurement of light absorption by brown carbon in PM2.5 in a megacity in South China, Sci. Total Environ., 633, 1360–1369, https://doi.org/10.1016/j.scitotenv.2018.03.235, 2018.
Liu, H., Man, H., Cui, H., Wang, Y., Deng, F., Wang, Y., Yang, X., Xiao, Q., Zhang, Q., Ding, Y., and He, K.: An updated emission inventory of vehicular VOCs and IVOCs in China, Atmos. Chem. Phys., 17, 12709–12724, https://doi.org/10.5194/acp-17-12709-2017, 2017.
Liu, T., Wang, X., Deng, W., Hu, Q., Ding, X., Zhang, Y., He, Q., Zhang, Z., Lü, S., Bi, X., Chen, J., and Yu, J.: Secondary organic aerosol formation from photochemical aging of light-duty gasoline vehicle exhausts in a smog chamber, Atmos. Chem. Phys., 15, 9049–9062, https://doi.org/10.5194/acp-15-9049-2015, 2015.
Liu, T. Y., Wang, X. M., Wang, B. G., Ding, X., Deng, W., Lü, S. J., and Zhang, Y. L.: Emission factor of ammonia (NH3) from on-road vehicles in China: tunnel tests in urban Guangzhou, Environ. Res. Lett., 9, 064027, https://doi.org/10.1088/1748-9326/9/6/064027, 2014.
Lu, Q., Zhao, Y., and Robinson, A. L.: Comprehensive organic emission profiles for gasoline, diesel, and gas-turbine engines including intermediate and semi-volatile organic compound emissions, Atmos. Chem. Phys., 18, 17637–17654, https://doi.org/10.5194/acp-18-17637-2018, 2018.
Lu, Q., Murphy, B. N., Qin, M., Adams, P. J., Zhao, Y., Pye, H. O. T., Efstathiou, C., Allen, C., and Robinson, A. L.: Simulation of organic aerosol formation during the CalNex study: updated mobile emissions and secondary organic aerosol parameterization for intermediate-volatility organic compounds, Atmos. Chem. Phys., 20, 4313–4332, https://doi.org/10.5194/acp-20-4313-2020, 2020.
Murphy, B. N., Woody, M. C., Jimenez, J. L., Carlton, A. M. G., Hayes, P. L., Liu, S., Ng, N. L., Russell, L. M., Setyan, A., Xu, L., Young, J., Zaveri, R. A., Zhang, Q., and Pye, H. O. T.: Semivolatile POA and parameterized total combustion SOA in CMAQv5.2: impacts on source strength and partitioning, Atmos. Chem. Phys., 17, 11107–11133, https://doi.org/10.5194/acp-17-11107-2017, 2017.
Ots, R., Young, D. E., Vieno, M., Xu, L., Dunmore, R. E., Allan, J. D., Coe, H., Williams, L. R., Herndon, S. C., Ng, N. L., Hamilton, J. F., Bergström, R., Di Marco, C., Nemitz, E., Mackenzie, I. A., Kuenen, J. J. P., Green, D. C., Reis, S., and Heal, M. R.: Simulating secondary organic aerosol from missing diesel-related intermediate-volatility organic compound emissions during the Clean Air for London (ClearfLo) campaign, Atmos. Chem. Phys., 16, 6453–6473, https://doi.org/10.5194/acp-16-6453-2016, 2016.
Pierson, W. R. and Brachaczek, W. W.: Emissions of ammonia and amines from vehicles in the road, Environ. Sci. Technol., 17, 757–760, https://doi.org/10.1021/es00118a013, 1983.
Presto, A. A. and Miracolo, M. A.: Secondary organic aerosol formation from high-NOx photo-oxidation of low volatility precursors: n-alkanes, Environ. Sci. Technol., 44, 2029–2034, https://doi.org/10.1021/es903712r, 2010.
Pye, H. O. T. and Seinfeld, J. H.: A global perspective on aerosol from low-volatility organic compounds, Atmos. Chem. Phys., 10, 4377–4401, https://doi.org/10.5194/acp-10-4377-2010, 2010.
Qi, L. J., Liu, H., Shen, X. E., Fu, M. L., Huang, F. F., Man, H. Y., Deng, F. Y., Shaikh, A. A., Wang, X. T., Dong, R., Song, C., and He, K. B.: Intermediate-volatility organic compound emissions from nonroad construction machinery under different operation modes, Environ. Sci. Technol., 53, 13832–13840, https://doi.org/10.1021/acs.est.9b01316, 2019.
Robinson, A. L., Donahue, N. M., Shrivastava, M. K., Weitkamp, E. A., Sage, A. M., Grieshop, A. P., Lane, T. E., Pierce, J. R., and Pandis, S. N.: Rethinking organic aerosols: semivolatile emissions and photochemical aging, Science, 315, 1259–1262, https://doi.org/10.1126/science.1133061, 2007.
Shrivastava, M. K., Lane, T. E., Donahue, N. M., Pandis, S. N., and Robinson, A. L.: Effects of gas particle partitioning and aging of primary emissions on urban and regional organic aerosol concentrations, J. Geophys. Res.-Atmos., 113, D18301, https://doi.org/10.1029/2007jd009735, 2008.
Stewart, G. J., Nelson, B. S., Acton, W. J. F., Vaughan, A. R., Farren, N. J., Hopkins, J. R., Ward, M. W., Swift, S. J., Arya, R., Mondal, A., Jangirh, R., Ahlawat, S., Yadav, L., Sharma, S. K., Yunus, S. S. M., Hewitt, C. N., Nemitz, E., Mullinger, N., Gadi, R., Sahu, L. K., Tripathi, N., Rickard, A. R., Lee, J. D., Mandal, T. K., and Hamilton, J. F.: Emissions of intermediate-volatility and semi-volatile organic compounds from domestic fuels used in Delhi, India, Atmos. Chem. Phys., 21, 2407–2426, https://doi.org/10.5194/acp-21-2407-2021, 2021.
Tang, R., Lu, Q., Guo, S., Wang, H., Song, K., Yu, Y., Tan, R., Liu, K., Shen, R., Chen, S., Zeng, L., Jorga, S. D., Zhang, Z., Zhang, W., Shuai, S., and Robinson, A. L.: Measurement report: Distinct emissions and volatility distribution of intermediate-volatility organic compounds from on-road Chinese gasoline vehicles: implication of high secondary organic aerosol formation potential, Atmos. Chem. Phys., 21, 2569–2583, https://doi.org/10.5194/acp-21-2569-2021, 2021.
Tkacik, D. S., Lambe, A. T., Jathar, S., Li, X., Presto, A. A., Zhao, Y. L., Blake, D., Meinardi, S., Jayne, J. T., Croteau, P. L., and Robinson, A. L.: Secondary organic aerosol formation from in-use motor vehicle emissions using a potential aerosol mass reactor, Environ. Sci. Technol., 48, 11235–11242, https://doi.org/10.1021/es502239v, 2014.
Wang, Y. H., Gao, W. K., Wang, S., Song, T., Gong, Z. Y., Ji, D. S., Wang, L. L., Liu, Z. R., Tang, G. Q., Huo, Y. F., Tian, S. L., Li, J. Y., Li, M. G., Yang, Y., Chu, B. W., Petaja, T. K., Kerminen, V-M., He, H., Hao, J. M., Kulmala, M., Wang, Y. S., and Zhang, Y. H.: Contrasting trends of PM2.5 and surface-ozone concentrations in China from 2013 to 2017, Nat. Sci. Rev., 7, 1331–1339, https://doi.org/10.1093/nsr/nwaa032, 2020.
Wu, L., Wang, X., Lu, S., Shao, M., and Ling, Z.: Emission inventory of semi-volatile and intermediate-volatility organic compounds and their effects on secondary organic aerosol over the Pearl River Delta region, Atmos. Chem. Phys., 19, 8141–8161, https://doi.org/10.5194/acp-19-8141-2019, 2019.
Wu, Y., Zhang, S. J., Hao, J. M., Liu, H., Wu, X. M., Hu, J. N., Walsh, M. P., Wallington, T. J., Zhang, M. K., and Stevanovic, S.: On-road vehicle emissions and their control in China: a review and outlook, Sci. Total Environ., 574, 332–349, https://doi.org/10.1016/j.scitotenv.2016.09.040, 2017.
Yang, W. Y., Li, J., Wang, W. G., Li, J. L., Ge, M. F., Sun, Y. L., Chen, X. S., Ge, B. Z., Tong, S. R., Wang, Q. Q., and Wang, Z. F.: Investigating secondary organic aerosol formation pathways in China during 2014, Atmos. Environ., 213, 133–147, https://doi.org/10.1016/j.atmosenv.2019.05.057, 2019.
Zhang, Q., Jimenez, J. L., Canagaratna, M. R., Allan, J. D., Coe, H., Ulbrich, I., Alfarra, M. R., Takami, A., Middlebrook, A. M., and Sun, Y. L.: Ubiquity and dominance of oxygenated species in organic aerosols in anthropogenically-influenced northern hemisphere midlatitudes, Geophys. Res. Lett., 34, L13801, https://doi.org/10.1029/2007GL029979, 2007.
Zhang, Y. L., Wang, X. M., Wen, S., Herrmann, H., Yang, W. Q., Huang, X. Y., Zhang, Z., Huang, Z. H., He, Q. F., and George, C.: On-road vehicle emissions of glyoxal and methylglyoxal from tunnel tests in urban Guangzhou, China, Atmos. Environ., 127, 55–60, https://doi.org/10.1016/j.atmosenv.2015.12.017, 2016.
Zhang, Y. L., Yang, W. Q., Huang, Z. H., Liu, D., Simpson, I., Blake, D. R., George, C., and Wang, X. M.: Leakage rates of refrigerants CFC-12, HCFC-22, and HFC-134a from operating mobile air conditioning systems in Guangzhou, China: tests inside a busy urban tunnel under hot and humid weather conditions, Environ. Sci. Technol. Lett., 4, 481–486, https://doi.org/10.1021/acs.estlett.7b00445, 2017.
Zhang, Y. L., Yang, W. Q., Simpson, I., Huang, X. Y., Yu, J. Z., Huang, Z. H., Wang, Z. Y., Zhang, Z., Liu, D., Huang, Z. Z., Wang, Y. J., Pei, C. L.,Shao, M., Blake, D. R., Zheng, J. Y., Huang, Z. J., and Wang, X. M.: Decadal changes in emissions of volatile organic compounds (VOCs) from on-road vehicles with intensified automobile pollution control: case study in a busy urban tunnel in South China, Environ. Pollut., 233, 806–819, https://doi.org/10.1016/j.envpol.2017.10.133, 2018.
Zhang, Y. L., Deng, W., Hu, Q. H., Wu, Z. F., Yang, W. Q., Zhang, H. N., Wang, Z. Y., Fang, Z., Zhu, M., Li, S., Song, W., Ding, X., and Wang, X. M.: Comparison between idling and cruising gasoline vehicles in primary emissions and secondary organic aerosol formation during photochemical ageing, Sci. Total Environ., 772, 137934, https://doi.org/10.1016/j.scitotenv.2020.137934, 2020.
Zhao, Y. L., Hennigan, C. J., May, A. A., Tkacik, D. S., de Gouw, J. A., Gilman, J. B., Kuster, W. C., Borbon, A., and Robinson, A. L.: Intermediate-volatility organic compounds: a large source of secondary organic aerosol, Environ. Sci. Technol., 48, 13743–13750, https://doi.org/10.1021/es5035188, 2014.
Zhao, Y. L., Nguyen, N. T., Presto, A. A., Hennigan, C. J., May, A. A., and Robinson, A. L.: Intermediate volatility organic compound emissions from on-road diesel Vehicles: chemical composition, emission factors, and estimated secondary organic aerosol production, Environ. Sci. Technol., 49, 11516–11526, https://doi.org/10.1021/acs.est.5b02841, 2015.
Zhao, Y. L., Nguyen, N. T., Presto, A. A., Hennigan, C. J., May, A. A., and Robinson, A. L.: Intermediate volatility organic compound emissions from on-road gasoline vehicles and small off-road gasoline engines, Environ. Sci. Technol., 50, 4554–4563, https://doi.org/10.1021/acs.est.5b06247, 2016.