the Creative Commons Attribution 4.0 License.
the Creative Commons Attribution 4.0 License.
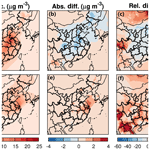
Impacts of water partitioning and polarity of organic compounds on secondary organic aerosol over eastern China
Jingyi Li
Haowen Zhang
Qi Ying
Zhijun Wu
Yanli Zhang
Xinming Wang
Xinghua Li
Yuanhang Zhang
Jianlin Hu
Secondary organic aerosol (SOA) is an important component of fine particular matter (PM2.5). Most air quality models use an equilibrium partitioning method along with the saturation vapor pressure (SVP) of semivolatile organic compounds (SVOCs) to predict SOA formation. However, the models typically assume that the organic particulate matter (OPM) is an ideal mixture and ignore the partitioning of water vapor to OPM. In this study, the Community Multiscale Air Quality model (CMAQ) is updated to investigate the impacts of water vapor partitioning and nonideality of the organic–water mixture on SOA formation during winter (January) and summer (July) of 2013 over eastern China. The updated model treats the partitioning of water vapor molecules into OPM and uses the universal functional activity coefficient (UNIFAC) model to estimate the activity coefficients of species in the organic–water mixture. The modified model can generally capture the observed surface organic carbon (OC) with a correlation coefficient R of 0.7 and the surface organic aerosol (OA) with the mean fractional bias (MFB) and mean fractional error (MFE) of −0.28 and 0.54, respectively. SOA concentration shows significant seasonal and spatial variations, with high concentrations in the North China Plain (NCP), central China, and the Sichuan Basin (SCB) regions during winter (up to 25 µg m−3) and in the Yangtze River Delta (YRD) during summer (up to 16 µg m−3). In winter, SOA decreases slightly in the updated model, with a monthly averaged relative change of 10 %–20 % in the highly concentrated areas, mainly due to organic–water interactions. The monthly averaged concentration of SOA increases greatly in summer, by 20 %–50 % at the surface and 30 %–60 % in the whole column. The increase in SOA is mainly due to the increase in biogenic SOA in inland areas and anthropogenic SOA in coastal areas. As a result, the averaged aerosol optical depth (AOD) is increased by up to 10 %, and the cooling effect of aerosol radiative forcing (ARF) is enhanced by up to 15 % over the YRD in summer. The aerosol liquid water content associated with OPM (ALWorg) at the surface is relatively high in inland areas in winter and over the ocean in summer, with a monthly averaged concentration of 0.5–3.0 and 5–7 µg m−3, respectively. The hygroscopicity parameter κ of OA based on the κ–Köhler theory is determined using the modeled ALWorg. The correlation of κ with the O:C ratio varies significantly across different cities and seasons. Analysis of two representative cities, Jinan (in the NCP) and Nanjing (in the YRD), shows that the impacts of water partitioning and nonideality of the organic–water mixture on SOA are sensitive to temperature, relative humidity (RH), and the SVP of SVOCs. The two processes exhibit opposite impacts on SOA in eastern China. Water uptake increases SOA by up to 80 % in the organic phase, while including nonunity activity coefficients decreases SOA by up to 50 %. Our results indicate that both water partitioning into OPM and the activity coefficients of the condensed organics should be considered in simulating SOA formation from gas–particle partitioning, especially in hot and humid environments.
- Article
(6781 KB) -
Supplement
(4887 KB) - BibTeX
- EndNote
Secondary organic aerosol (SOA) is formed via a complex interaction of volatile organic compounds (VOCs) with oxidants and primary particles emitted from anthropogenic and biogenic sources in the atmosphere. As an important component of fine particular matter (PM2.5), SOA can cause severe air pollution in urban and suburban areas (Huang et al., 2014) and exhibits adverse health effects (Atkinson et al., 2014). SOA also plays an important role in new particle formation and particle growth that further contribute to the enhancement of cloud condensation nuclei (CCNs; Wiedensohler et al., 2009; Ehn et al., 2014). This will, in turn, impact the atmospheric aerosol burden, precipitation and water circulation, solar radiation budget, and climate (Ramanathan et al., 2001). However, the extents of those influences are not well understood so far due to the high uncertainties associated with the formation as well as the physical and chemical properties of SOA (Shrivastava et al., 2017). Large gaps still exist in SOA mass loading and properties between models and observations (Gentner et al., 2017; Ervens et al., 2011; Hayes et al., 2015). Therefore, it is crucial to explore and resolve this issue to improve our knowledge of the roles of SOA in the environment, human health, and climate.
Gas–particle partitioning of semivolatile and low-volatile organic compounds (SVOCs and LVOCs) generated from VOC oxidation is an important pathway of SOA formation. In most current chemical transport models (CTMs), this process is treated as an equilibrium partitioning process that depends on the mass concentration of the organic particulate matter (OPM), ambient temperature (T), the mean molecular weight of the OPM, and the volatility of condensed organics (Pankow, 1994). The formation of condensed organic products is commonly represented by lumped surrogate SVOCs in a two-product model with volatilities and SVOC yields fitted to chamber experiments (Odum et al., 1996). To better represent the volatility of primary organic aerosol (POA) and the multigeneration oxidation of SVOCs to a wider range, Donahue et al. (2006) proposed the volatility basis set (VBS) model, in which the mass yields of SVOCs are fitted to a fixed number of volatility bins (usually 0.01–105 µg m−3). The VBS model has been adopted by several CTMs (WRF-Chem, GEOS-Chem, etc.).
Although the models can capture the general trend of SOA evolution and mass concentration to some extent (J. Li et al., 2017; Bergström et al., 2012; Woody et al., 2016), two key factors currently neglected in models may lead to biases: (1) the molecular structures and interactions of functional groups (−OH, −C = O, −COOH, etc.) of condensed organics (nonideality) and (2) partitioning of water vapor, the most abundant atmospheric constituent besides O2 and N2, to OPM. Nonideality alters the volatility of condensed organics and thus their contributions to the total SOA mass loading (Cappa et al., 2008). Water partitioning into OPM can reduce the partial pressure of organics due to the effect of Raoult's law (Prisle et al., 2010) and lead to increases in SOA mass. The amount of aerosol liquid water associated with organics (ALWorg) may vary for different precursors (Healy et al., 2009; Prisle et al., 2010). The above two aspects will not only affect the chemical composition of SOA but also the inorganic portion (Ansari and Pandis, 2000) and optical properties (Denjean et al., 2015) of aerosols.
Laboratory and field studies have observed water absorbed by SOA from a variety of precursor VOCs (Lambe et al., 2011; D. F. Zhao et al., 2016; Asa-Awuku et al., 2010; Varutbangkul et al., 2006). The hygroscopicity of SOA, quantitatively described by the hygroscopicity parameter κ is correlated with the oxygen-to-carbon ratio (O:C) and increases with more oxidized SOA during photochemical aging (Lambe et al., 2011; D. F. Zhao et al., 2016). The OPM-associated water can be estimated using the κ–Köhler theory under the Zdanovskii–Stokes–Robinson (ZSR) assumption of no interactions between any constituents in aerosols (Petters and Kreidenweis, 2007). The total water content is the summation of water associated with each solute at the same water activity. Guo et al. (2015) found that this simplified method, along with the ISORROPIA model, which is used to predict aerosol liquid water (ALW) associated with the inorganic portion of aerosols, could reproduce the observed total ALW in the ambient environment. Pye et al. (2017) found that the modeled organic aerosol (OA) improved significantly but biased high at nighttime when ALWorg is included in the calculation. However, as the interaction among organic species and between organics and water in the organic–water mixture has been shown to play an important role in SOA formation and water partitioning to OPM (Kim et al., 2019), the ALWorg estimated by the κ–Köhler theory and its impact on SOA might not be accurate. Using the universal functional activity coefficient (UNIFAC) method (Fredenslund et al., 1975) for calculating activity coefficients of the organic–water mixture, it was found that in the eastern US, where biogenic SOA dominated the OA, considering ALWorg leads to a significant increase in predicted SOA (Pankow et al., 2015; Jathar et al., 2016).
China has been suffering from severe PM2.5 pollution especially in the eastern region, with fast urbanization and economic development (Fu and Chen, 2017). SOA is a very significant component of PM2.5 in China, contributing about 20 %–50 % (Y. J. Li et al., 2017). The fraction of SOA in OA increases during haze events (Huang et al., 2014; Sun et al., 2019). Previous modeling studies in China indicate that SOA was underpredicted (Lin et al., 2016; Jiang et al., 2012), and the impacts of nonideality and water–OPM partitioning on modeled SOA have not been evaluated.
In this study, regional simulations of SOA during January and July of 2013 over eastern China were conducted to investigate the seasonal variation in SOA due to water partitioning into OPM. The model performance was evaluated against observed meteorological parameters (temperature and relative humidity, RH) as well as PM2.5, organic carbon (OC), and OA at ground monitoring sites. The regional and seasonal impacts on SOA, ALWorg, and properties of aerosols were quantified. Lastly, influences of the results by several factors including meteorological parameters, estimations of saturation vapor pressures (SVP) of condensed organics, and the individual impacts of ALWorg and nonideality of the organic–water mixture on SOA prediction were analyzed.
2.1 Model description
The Community Multiscale Air Quality model (CMAQ v5.0.1), coupled with a modified SAPRC-11, was used in this study. Model configurations were largely based on that used by Hu et al. (2016) as summarized below. Firstly, SAPRC-11 was expanded for a more detailed treatment of isoprene oxidation and for tracking dicarbonyl products (glyoxal and methylglyoxal) from different groups of major precursors (Ying et al., 2015). Secondly, SOA from isoprene epoxydiols (IEPOX), methacrylic acid epoxide (MAE), and dicarbonyls through surface-controlled irreversible reactive uptake was added (Hu et al., 2017; Li et al., 2015; Liu et al., 2020; Ying et al., 2015). Thirdly, the heterogeneous formation of secondary nitrate and sulfate from NO2 and SO2 reactions on the particle surfaces (Ying et al., 2014) was added, which is an important source of secondary inorganic aerosol (Zheng et al., 2015) and improves model estimates of nitrate and sulfate (Qiao et al., 2018; Shi et al., 2017). Fourthly, SOA yields were corrected for vapor wall loss (Zhang et al., 2014). Impacts of the above updates on model performances have been extensively discussed in the cited work and are not further investigated in the current study.
The SOA module mostly follows Pankow et al. (2015). Two types of SOA as traditionally treated in CMAQ were considered: the “semivolatile” (SV) portion that formed via equilibrium absorption partitioning of SVOCs and the “nonvolatile” (NV) portion that includes the oligomers and SOA formed via direct oxidation of aromatics at low NOx. SOA from dicarbonyls, IEPOX, and MAE was formed by irreversible reactive uptake and categorized as NV SOA in the current model as well. Some studies investigated SOA from glyoxal, methylglyoxal, and IEPOX using detailed reactions and reversible pathways in models or observed as reversible processes in chamber experiments, leading to a relatively lower SOA yield compared to the surface-controlled irreversible uptake (Lim et al., 2013; Knote et al., 2014; Galloway et al., 2009; El-Sayed et al., 2018; Budisulistiorini et al., 2017). The nonvolatile assumption used in this paper allows an upper-limit estimation of the importance of these additional SOA formation pathways. POA was treated as nonvolatile and nonreactive. The mass distribution of SVOCs between the gas phase and particle phase follows the equation
where Kp,i (m3 µg−1) is the gas–particle partitioning constant for compound i, Fi (µg m−3) is the concentration of species i in the particle phase, Ai (µg m−3) is the concentration of species i in the gas phase, and M (µg m−3) is the total mass concentration of the absorbing organic phase (i.e., OPM). The gas–particle partitioning constant Kp,i is dependent on the chemical composition of the OPM. Pankow (1994) derived Kp,i for SVOCs partitioning into an absorbing organic phase as
where (atm) is the SVP of the pure compound i at temperature T (K), ξi is the activity coefficient of species i in the absorbing organic phase, (g mol−1) is the average molecular weight of the OPM, R (8.314 J mol−1 K−1) is the gas constant, and 106 is used to convert the units to cubic meters per microgram.
There are 12 lumped SVOCs generated by oxidation of alkanes, alkenes, and aromatics under different NOx conditions and 8 NV organic products as listed in Tables S1 and S2 in the Supplement. More details about the lumped precursors such as formation conditions (“high” and “low” NOx), lumping species and method, and yields from parent VOCs can be found in Carlton et al. (2010) and are summarized in the Supplement. Activity coefficients of SVOCs were calculated based on the composition of the OPM using the UNIFAC method, with carbon number (nc), functional groups, and energy interaction parameters assigned to both SV and NV compounds (Pankow et al., 2015). The UNIFAC model is one of the most commonly used models with which activity coefficients of condensed organics and their interactions with water can be estimated. This method has been adopted to investigate the impacts of nonideality and water partitioning into OPM on SOA for different precursors in box models (Seinfeld et al., 2001; Bowman and Melton, 2004) and CTMs (Pankow et al., 2015; Kim et al., 2019). In the current model, POA was assumed to have a bulk composition of 10 categories of surrogate species (Table S3), as used by Li et al. (2015). POA is also involved in the calculation of activity coefficients for the organic–water mixture. Detailed information about the surrogate species, including their structures and properties, can be found in Li et al. (2015) and references therein.
In addition to organic compounds, water partitioning into OPM is enabled according to Eqs. (1) and (2). In such a case, the absorbing phase in Eq. (1) includes both organic aerosol and water associated with OPM. As water condenses in the absorbing organic phase, it will further alter the molar fraction of each composition, the activity coefficient of SVOCs, and the SV SOA mass concentrations as a result. In the current model, we assumed no interactions between the inorganic and organic phases.
2.2 Estimating the hygroscopicity of OA
Based on the κ–Köhler theory with linearly additive hygroscopic behavior of each component of the mixed particle, ALWorg is related to the hygroscopicity parameter for the organic mixture (κorg) by Eq. (3) (Petters and Kreidenweis, 2007):
where ρw is the density of water (assumed to be 1 g cm−1), Vorg is the volume concentration of organics, and aw is the water activity (assumed to be the same as RH). Since ALWorg in this study is calculated mechanistically using the partitioning theory, κorg can be estimated by rearranging Eq. (3):
Vorg can be estimated from the modeled mass concentration of OA, assuming the density of OA to be 1.2 g cm−3 (Li et al., 2019).
In many studies, κorg is assumed to increase linearly with the oxidation state of OA, expressed as the O:C ratio (Massoli et al., 2010; Duplissy et al., 2011; Lambe et al., 2011). The correlation of κorg and the O:C ratio in nine representative cities was evaluated during January and July of 2013 with the reduced major axis regression method (Ayers, 2001). The O:C ratio was calculated using Eq. (5):
where fi and (O:C)i are the molar fraction and O:C ratio of organic aerosol component i, respectively. For POA, a fixed molar fraction and composition were assumed following Li et al. (2015). For SOA, the O:C ratio was calculated by using the ratio of organic matter to organic carbon (OM:OC) following Simon and Bhave (2012):
The OM:OC ratio of each SOA component follows Pankow et al. (2015) as shown in Tables S1 and S2.
2.3 Model application
The simulation domain has a horizontal resolution of 36 km × 36 km (100×100 grids) and a vertical structure of 18 layers up to 21 km, which covers eastern China as shown in Fig. S1 in the Supplement. Anthropogenic emissions were generated from the Multi-resolution Emission Inventory for China (MEIC; Zhang et al., 2009) v1.0 at a resolution of 0.25∘ × 0.25∘ (http://www.meicmodel.org, last access: 1 March 2019) for China and from the Regional Emission inventory in ASia version 2 (REAS2; Kurokawa et al., 2013) at a resolution of 0.25∘ × 0.25∘ (http://www.nies.go.jp/REAS/, last access: 1 March 2019) for the rest of the domain. Biogenic emissions were generated by the Model for Emissions of Gases and Aerosols from Nature (MEGAN) v2.1, with the leaf area index (LAI) from the 8 d Moderate Resolution Imaging Spectroradiometer (MODIS) LAI product (MOD15A2) and the plant function types (PFTs) from the Global Community Land Model (CLM 3.0). Open biomass burning emissions were generated from the Fire INventory from the National Center for Atmospheric Research (FINN; Wiedinmyer et al., 2011). Dust and sea salt emissions were generated online during CMAQ simulations. The total emissions of major SOA precursors and their spatial distributions are shown in Table S4 and Fig. S2. Meteorological fields were generated using the Weather Research and Forecasting (WRF) model v3.6.1 with initial and boundary conditions from the National Centers for Environmental Prediction Final (NCEP FNL) Operational Model Global Tropospheric Analyses dataset. More details about the model application can be found in Hu et al. (2016).
Four scenarios are investigated in this study. The base case (BC) applies the default secondary organic aerosol module of CMAQ v5.0.1. In this case, no water partitioning into OPM is considered. Lumped semivolatile products from the oxidation of various precursors partition into a single organic phase, which is considered as an ideal mixture of POA and SOA with γorg=1. The water case (C1) includes water partitioning into OPM, which is again considered as an ideal solution (γorg=1 and ). The UNIFAC case (C2) considers the interaction between organic constituents with UNIFAC-calculated activity coefficients (γorg≠1) but does not allow water partitioning into OPM. The combined case (C3) allows both water partitioning and interactions between all constituents (including water and organics) using UNIFAC-calculated activity coefficients (γorg≠1 and ). The results of the BC and C3 are used to examine the overall impacts of water partitioning into OPM and polarity of organics on SOA and ALWorg, as shown in Sect. 3.1–3.4. The separate influences of those two processes on SOA from C1 and C2 are discussed in Sect. 3.5.
3.1 Model evaluation
The meteorological inputs and emissions have been used in several previous publications. Model performance on meteorological parameters (temperature and RH), gaseous species, and gas and aerosol concentrations have been extensively evaluated (Hu et al., 2016, 2017; Qiao et al., 2018; Shi et al., 2017). A summary of the model performance related to this study is provided below. Observed meteorological data were obtained from the National Climatic Data Center (ftp://ftp.ncdc.noaa.gov/pub/data/noaa, last access: 15 February 2019). Observations of OC at two urban locations, Beijing (Cao et al., 2014; Wang et al., 2015) and Guangzhou (Lai et al., 2016), and of OA in Beijing (Sun et al., 2013) during January of 2013 as well as surface PM2.5 observations from the China National Environmental Monitoring Center (http://113.108.142.147:20035/emcpublish/, last access: 10 May 2015) at several monitoring sites during July of 2013 were used to evaluate model estimates of aerosols. Details of measurement methodology and uncertainties of observations are listed in the corresponding references.
Temperature and RH are the two meteorological factors that affect SOA formation. Table 1 lists model statistics of mean observation (OBS), mean prediction (PRE), mean bias (MB), gross error (GE), and correlation coefficient (R) based on WRF and observations at monitoring sites located in eight subregions of the domain (Fig. S1) during January and July of 2013. The benchmarks for the MM5 model (another meteorology model) of 4–12 km horizontal resolution suggested by Emery et al. (2001) are also listed in the table. Details of monitoring sites in the eight subregions are listed in Table S5. Overall, WRF tends to underestimate both temperature and RH. The model shows better agreement with observed temperature as R is higher than that of RH. Both temperature and RH are well captured by the model in the YRD, the Pearl River Delta (PRD), and the central regions of China (the major regions of eastern China). In these regions, MB and GE of temperature are −1.2–0.7 and 1.8–2.6 K, respectively, which are −11.8 %–5.6 % and 9.2 %–16.8 % for RH, respectively.
Table 1Statistical analysis of modeled temperature (K) and relative humidity (%) of January and July of 2013 at the monitoring sites in different geographical areas as shown in Fig. S1. The cities are listed in Table S5.
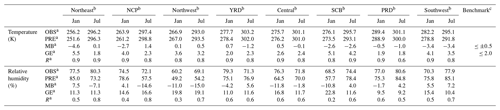
a OBS is mean observation, PRE is mean prediction, MB is mean bias, GE is gross error, and R is the correlation coefficient of predictions vs. observations. MB = ; GE = , where Cp and Co are the prediction and observation data, and N is the total number of data. b Northeast is northeastern China, NCP is the North China Plain, northwest is northwestern China, YRD is the Yangtze River Delta, central is central China, SCB is the Sichuan Basin, PRD is the Pearl River Delta, and southwest is southwestern China. c Benchmarks of MB and GE are for the MM5 model of 4–12 km horizontal resolution by Emery et al. (2001).
Model estimates of daily organic carbon (OC) from case C3 are compared with measurements at monitoring sites in Beijing and Guangzhou in January of 2013 (Fig. 1a). The factors used to convert SOA to OC (SOC) are listed in Tables S1 and S2. OC from POA (POC) is directly predicted by the model. Generally, the ratio between modeled and observed OC concentration falls in the range of 1:2 to 2:1, with an R value of 0.7. The model tends to underestimate OC on high-concentration days. Overall, the mean fractional bias (MFB) and mean fractional error (MFE) of OC are −0.20 and 0.27, which are within the criteria (MFB 0.6; MFE ≤ 0.75) suggested by the EPA (2007). The bias in OC might be due to underestimated POA emissions and underpredicted SOA in CMAQ from missing precursors (Hu et al., 2017; B. Zhao et al., 2016). No significant differences in OC are observed in C3 compared to BC (not shown), likely due to the low-biased SOA predicted in the current model that limits the impact of ALWorg on SOA formation.
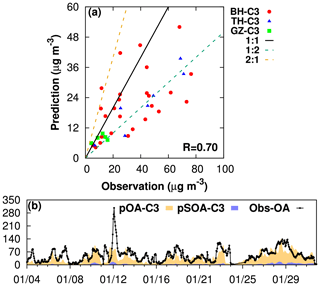
Figure 1Comparison of (a) observed and modeled organic carbon concentration at the University of Beihang (BH), Tsinghua University (TH), and Guangzhou (GZ); (b) observed organic aerosol (Obs-OA) at Beijing and predictions of total OA (pOA) and SOA (pSOA; µg m−3). The locations of the monitoring sites are shown in Fig. S1.
The underestimation of SOA can be seen from Fig. 1b as well. CMAQ can well capture the observed diurnal variation in OA in Beijing during wintertime except for the underestimates of peak values. The correlation coefficient of modeled to observed OA is 0.55. We find a 25 % underestimate of OA on average. Better agreement between the model and observations is shown on nonpolluted days (daily averaged concentration less than 75 µg m−3). The MFB and MFE of polluted days are −0.38 and 0.64, respectively, which are worse than those of nonpolluted days (−0.26 for MFB and 0.52 for MFE). The overall MFB and MFE of OA during January are −0.28 and 0.54, respectively, which are within the criteria (MFB 0.6; MFE ≤ 0.75) suggested by the EPA (2007). Again, no apparent changes in SOA or OA are observed between case C3 and BC (not shown) since POA is predicted to be the primary contributor to OA in Beijing in winter in the current model, with an averaged SOA∕POA ratio of 0.12. This ratio is much lower than the field observation of about 0.45–1.94 (Zhao et al., 2019; Sun et al., 2013, 2016). The bias might be due to the missing SOA converted by partitioning and aging of semivolatile POA as well as oxidation from intermediate volatile organic compounds (IVOCs) and VOC oxidation products. Those pathways are shown to be important for SOA formation by modeling, field, and chamber studies (Hodzic et al., 2010; Jimenez et al., 2009; Murphy et al., 2017; Robinson et al., 2007; Shrivastava et al., 2008; Tkacik et al., 2012; Zhao et al., 2014; B. Zhao et al., 2016).
A sensitivity test was performed by using the newest CMAQ model version 5.3.1, which includes all the above processes in the aerosol module. The SOA∕POA ratio in Beijing is improved greatly in winter, at 0.83. However, high uncertainties still exist in the emissions of the involved precursors and characterization of SOA formation through these processes, requiring further constraints by observations. Their influences on SOA due to water partitioning into OPM and nonideality of the organic–water mixture will be evaluated in a future study.
Due to the lack of observed OC and OA in July of 2013, model performances are evaluated by comparing predicted and observed PM2.5 at ground sites (Fig. S1) as an alternative, as shown in Fig. S3. Generally, the model can well reproduce the diurnal variation in PM2.5 in most regions. Predicted PM2.5 on highly concentrated days is biased low, especially in the North China Plain (NCP). The NCP has the highest PM2.5, ranging from 60 to 300 µg m−3. The bias in modeled PM2.5 is significant in cities in the northwest. This might be due to missing dust emissions in the current inventory (Hu et al., 2016). To further evaluate the model performance, the averaged MFB and MFE of modeled PM2.5 are plotted against observations of each site as shown in Fig. S4. The criteria of MFB and MFE followed recommendations by Boylan and Russell (2006). Our model performs well since most of the predictions meet the criteria, and a large fraction (>58 %) meets the goal. The averaged MFB and MFE across all the sites are −0.28 and 0.39, respectively, indicating slight underestimation of PM2.5 by the model.
3.2 Impacts on SOA and ALWorg
The spatial distribution of SOA varies greatly in the two seasons. In winter, SOA are relatively high in the eastern SCB and the central and eastern provinces of Shandong, Henan, Anhui, and Hubei (Figs. 2 and S5). Monthly averaged SOA concentrations in the above areas are up to 25 and 15–20 µg m−3, respectively. Anthropogenic emissions such as dicarbonyl products from the oxidation of xylene and toluene (Hu et al., 2017) are the major sources of SOA (Fig. S6). In summer, surface SOA is high in the northeast, the NCP, and the YRD. The highest SOA occurs in Shanghai and Jiangsu provinces as well as the coastal area of the Yellow Sea, with values of ∼9–16 µg m−3 at the surface and ∼20–25 mg m−2 in the column (col-SOA) of the atmosphere below 21 km (Fig. S5). In contrast to winter SOA, a significant fraction of summer SOA originates from biogenic emissions (Fig. S7). Anthropogenic SOA is highly concentrated in the coastal areas of the Yellow Sea and Bohai Bay.
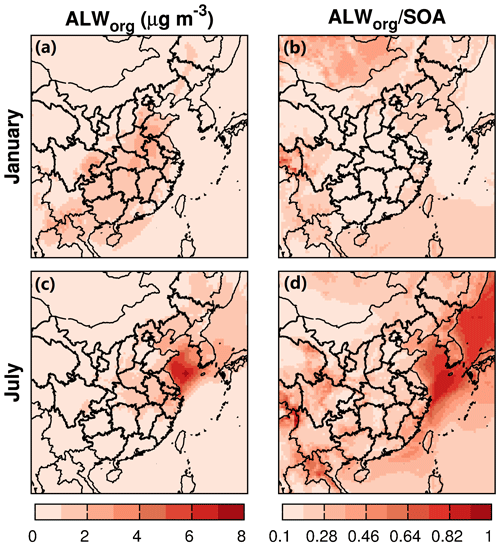
Figure 3Monthly averaged water partitioning into the organic phase (ALWorg, µg m−3) and the ratio to SOA (ALWorg∕SOA) during January and July of 2013.
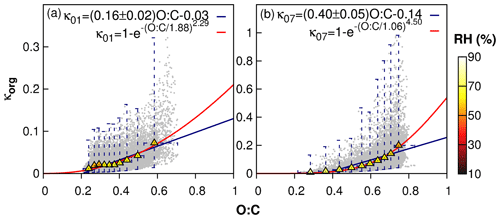
Figure 4The correlation of hygroscopicity of organic aerosol (κorg) and the O:C ratio in nine representative cities – Shenyang (SS), Beijing (BJ), Jinan (JN), Zhengzhou (ZZ), Xi'an (XA), Nanjing (NJ), Shanghai (SH), Chengdu (CD), and Guangzhou (GZ) – in January (a) and July (b) of 2013. O:C ratios are categorized into 10 bins. In each bin, the ranges of O:C and κorg are represented by bars. The mean values of O:C and κorg are represented by triangles colored by the averaged RH of each bin. The relationship between κorg and O:C is fitted by a linear function with reduced major-axis regression (blue lines) and an exponential function (red lines), respectively. κ01 and κ07 represent the fitted correlation for January and July, respectively.
The combined effects of water partitioning into OPM and nonideality on SOA formation also exhibit strong seasonal variation. In winter, SOA is slightly decreased by 1.5 µg m−3 (10 %–20 %) on average at the surface (Fig. 2) and less than ∼1 mg m−2 (20 %) in the column (Fig. S5) over high-SOA regions where anthropogenic sources dominate. We show later that the decrease in SOA is mainly due to the large activity coefficients of SVOCs, which decrease Kp,i. In summer, higher temperature and RH promote water partitioning and SOA formation so that SOA apparently increases over the entire domain, with the highest enhancement of 2–4 µg m−3 (20 %–50 %) at the surface (Fig. 2) and 4–6 mg m−2 (30 %–60 %) in the column (Fig. S5) over the YRD and the coastal area of the Yellow Sea. Anthropogenic SOA dominates the total change in winter as shown in Fig. S6. In summer, the increase in SOA is attributed to biogenic sources in inland areas and anthropogenic sources over the ocean (Fig. S7).
Regional distribution of ALWorg is similar to the change in SOA as shown in Fig. 3. In winter, a maximum averaged concentration of 3.0 µg m−3 for ALWorg occurs in the high-SOA region, where significant changes in SOA also occurs. In other areas, the averaged concentration of ALWorg is about 0.5–1.5 µg m−3. Overall, the average ratio of ALWorg to SOA is about 0.1–0.3 in winter. In summer, water partitioning into OPM mostly occurs in the eastern coastal area at the surface, where a significant increase in anthropogenic SOA (such as those from toluene and xylenes) is observed. This might be due to the high polarity of anthropogenic SVOCs (having more −COOH groups) that absorb more water. In the coastal area, the averaged concentration of ALWorg is about 5–7 µg m−3, with an ALWorg∕SOA ratio of 0.5–1.0. Over land, the averaged concentration of ALWorg is about 1–3 µg m−3 (ALWorg∕SOA ratio of 0.2–0.5) in northeastern and eastern China. Water partitioning is mostly associated with biogenic SOA originated from isoprene and monoterpene oxidation that produces SVOCs with abundant OH groups.
Based on the column concentrations of ALWorg and the ALWorg∕SOA ratio (Fig. S8), more ALWorg must have occurred in winter in the southern and southwestern regions at higher levels, where a significant increase in col-SOA occurs (Fig. S5). The averaged col-ALWorg∕col-SOA ratio in the high-SOA area is 0.1–0.3. In summer, the ALWorg must be high at higher altitudes over the central regions in China. The maximum col-ALWorg is about 7 mg m−2 over the YRD, with a col-ALWorg∕col-SOA ratio of about 0.3.
3.3 Impacts on aerosol properties
Since ALWorg is determined in C3, the values of κorg can be estimated from the modeled ALWorg, OA, and RH using Eq. (4). Nine representative cities were selected to investigate the relationship of κorg and O:C and its seasonal variation as shown in Figs. S9 and S10. The results of all the cities in winter and summer are merged and analyzed as shown in Fig. 4. Pairs of κorg and O:C data are grouped into 10 O:C bins, and the averaged κorg in each bin is then calculated. Overall, the estimated O:C ratio is within the range of 0.2–0.8. The averaged κorg in each O:C bin is less than 0.1 in winter, with the highest value in Guangzhou. As more ALWorg is formed in summer, the averaged κorg also increases greatly, with the highest value of 0.35 in Beijing. The linear correlation between κorg and O:C shows significant spatial and seasonal variations. For example, the slope of κorg–O:C is 70 %–90 % smaller in winter than in summer in northern cities such as Shenyang, Beijing, Zhengzhou, and Xi'an. However, in Guangzhou, the slope is 83% higher in winter than in summer. In Chengdu, the slope is quite similar in both seasons. Overall, the slope of κorg vs. O:C in the nine cities is 0.16 in winter and 0.40 in summer. Most of the fitted linear correlations of the individual cities fall outside of the range of 0.18–0.37 suggested in previous studies (Duplissy et al., 2011; Lambe et al., 2011; Massoli et al., 2010; Chang et al., 2010), indicating that the hygroscopicity of organic aerosol cannot be simply represented by a single parameter such as the O:C ratio (Rickards et al., 2013). In both seasons, κorg approaches zero and negative values as O:C decreases, which might be due to the linear regression of κorg and O:C. To avoid this, an exponential fitting of the two variables is performed so that κorg falls in the range of (0, 1) and is positively correlated with O:C. In this case, the fitted correlations are and for January and July of 2013, respectively.
The impacts on aerosol optical depth (AOD) and aerosol radiative forcing (ARF) are further investigated. Figure 5 shows the monthly averaged AOD at 550 nm in January and July of 2013. It is calculated by summarizing the product of the model-estimated extinction coefficient of fine particles (bext,i) multiplied by the thickness (HLi) in each layer:
where N is the number of layers. There are two methods to estimate the aerosol extinction coefficient in CMAQ v5.0.1. One is based on the Mie theory and the predicted aerosol component concentrations (bext,m), and the other is based on correlation with the IMPROVE monitoring network, which considers the impacts of hygroscopicity of different aerosol components (bext,r; Malm et al., 1994). AOD calculated with the two types of extinction coefficient are denoted as AODm and AODr, respectively.
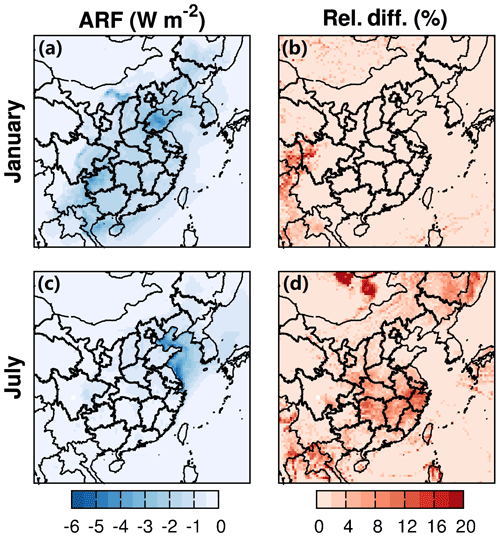
Figure 6Monthly averaged shortwave direct aerosol radiative forcing at the top of the atmosphere from C3 and the relative changes due to water partitioning into OPM and nonideality of the organic–water mixture during January and July of 2013. “Rel. Diff.” represents relative differences ((C3–BC)/BC; %).
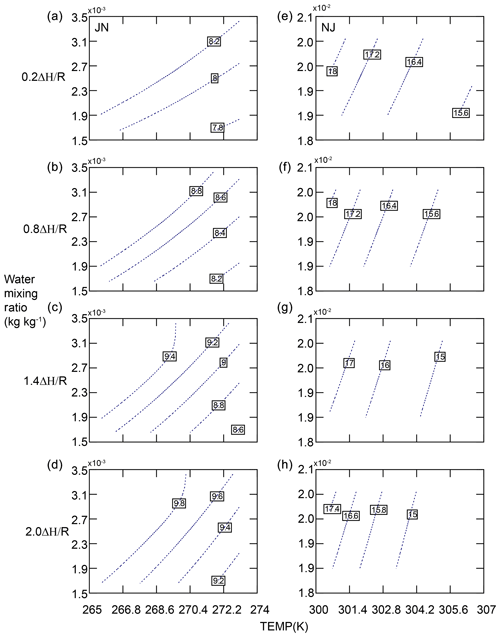
Figure 7The sensitivity of SOA formation to temperature (TEMP), water mixing ratio, and the temperature dependence parameter of SVP (ΔH∕R) at Jinan (JN, a–d) and Nanjing (NJ, e–h).
In Fig. 5, a clear pattern of high AODr in the SCB and the NCP and low AODr in western China is observed in both winter and summer, which is consistent with previous studies (He et al., 2016, 2019; Luo et al., 2014). An identical trend in AODm is shown in Fig. S11. The monthly averaged AODr ranges from 1.0 to 3.2 in January and from 0.3 to 0.9 in July. AODm is lower than AODr, falling within the range of 0.7–2.2 in January and 0.3–0.6 in July. The model significantly overestimates AOD in January but agrees better with observations from MODIS, where AOD is high in July (Fig. S12). The bias in the predicted AOD might be partially due to the empirical equation applied in the calculation of AOD in CMAQ (Wang et al., 2009; Liu et al., 2010) and partially due to the uncertainties of fine AOD over land from the MODIS data (Wang et al., 2009; Levy et al., 2010). The increase in AOD due to ALWorg shows a strong spatial and seasonal pattern. In winter, there are no significant changes in AODr across the whole domain due to insignificant changes in SOA. In summer, AODr increases significantly in the YRD and the adjacent areas by up to 10 %.
ARF represents the change in the radiative flux at the top of the atmosphere due to aerosols. An offline version of the Shortwave Radiative Transfer Model for GCMs (RRTMG_SW) was used to calculate the direct radiative effect of aerosols on shortwave radiation (Iacono et al., 2008). Generally, fine aerosols exhibit cooling effects on the shortwave radiation in both winter and summer over the entire domain as shown in Fig. 6. This impact is much stronger in the areas where AOD is high (Fig. 5). ARF is highest in Shandong in winter and in the coastal area near Jiangsu province in summer, amounting to about −5 and −6 W m−2, respectively. In winter, no significant changes in ARF are observed in eastern China (Fig. 6b). This is likely attributed to an insignificant contribution of SOA to PM2.5 in winter compared to other components with cooling effects, such as sulfate. In summer, SOA is an important component of PM2.5 (20 %–60 %), and the effects of water partitioning on shortwave radiation are relatively stronger. An enhancement in the cooling effects of ARF of up to 15 % occurs near the YRD region, where AOD significantly changes as well.
3.4 Sensitivity to T, RH, and SVP
Meteorological conditions and SOA precursors affect the impacts of water partitioning on SOA. To better illustrate the dependency of SOA on temperature, RH, and SVP of SVOCs, an offline calculation of SOA formation was performed in two representative cities (Jinan, in the NCP, during winter and Nanjing, in the YRD, during summer) when the daily maximum SOA increases occurred. We assume temperature (T) and water vapor mixing ratio (QV) to be within the range of , where and σ are the mean and standard deviation, respectively, calculated based on WRF predictions at each location. We choose 10 evenly distributed values for T and QV within the range of . The temperature dependence parameter of SVP (ΔH∕R) is also scaled separately by 0.2, 0.8, 1.4, and 2.0 for all the SVOCs. As shown in Fig. 7, SOA exhibits a negative correlation with T and a positive correlation with QV in both cities. SOA is more sensitive to QV under cold conditions (Jinan) and to temperature under hot conditions (Nanjing). When the temperature is fixed, the sensitivity of SOA to ΔH∕R is different in the two cities. We find more changes in SOA across ΔH∕R in Jinan. This is attributed to the temperature correction factor (ζcorr) of Kp in CMAQ as defined below:
where Tref is the reference temperature (298 K), and T is temperature. According to Fig. 7, the range of T is 265–274 K in Jinan and 300–307 K in Nanjing. The deviation of temperature from the reference value (298 K) is greater in Jinan than in Nanjing. Therefore, the unit change in ΔH∕R causes greater variations of ζcorr and thus Kp in Jinan. As a result, SOA is more sensitive to ΔH∕R. The impacts of SVP estimation on SOA are more significant in winter than in summer.
3.5 Separate impacts of water partitioning and polarity of condensed organics
The impacts of water partitioning into OPM and nonideality of the organic–water mixture on SOA are in opposite directions. Water partitioning increases SOA by ∼10 %–20 % in winter and ∼30 %–80 % in summer in most areas of the domain (Fig. 8). This is because the molecular weight of water is quite small and will reduce the mole-averaged molecular weight of the OPM () in Eq. (2) (Pankow et al., 2015). The reduced further increases Kp and promotes mass transfer of SVOCs from the gas phase to OPM. On the other hand, by considering nonideality of the organic–water mixture, activity coefficients of SVOCs are usually greater than 1.0 in this study, leading to a decrease in Kp. As a result, the total SOA concentration is reduced by ∼10 %–20 % in winter and ∼10 %–50 % in summer (Fig. 9). Overall, the final impacts are the combined consequences of the two processes. In winter, the increase in SOA caused by water partitioning is offset by the decrease in SOA due to the polarity of SVOCs in most areas of the domain, resulting in slight decreases in SOA. In summer, the effect of water partitioning overcomes that of SVOC polarity, so the total SOA loading increases. This further leads to an enhanced attenuation of shortwave solar radiation and cooling of the atmosphere.
The WRF–CMAQ model was used to investigate the impacts of water partitioning into OPM and nonideality of the organic–water mixture on SOA formation over eastern China during January and July of 2013. SOA is greatly enhanced in summer, especially in the YRD and over the Yellow Sea, by up to 50 % and 60 % at the surface and in the whole column, respectively. No significant impacts on SOA are observed in winter. This might be due to the underestimation of SOA in the current model. ALWorg is highly correlated with the change in SOA, with a ratio of ALWorg to SOA of 0.1–0.3 and 0.2–1.0 at the surface, where significant changes in SOA occur, in winter and summer, respectively. By using the modeled ALWorg, correlations between κorg and O:C are examined in nine representative cities, showing significant spatial and seasonal variations. The increases in SOA lead to an enhancement in the averaged AOD and the cooling effects of aerosols by up to 10 % and 15 %, respectively, in summer. The model-predicted SOA is sensitive to temperature and QV in both seasons, with higher sensitivity to QV during winter and to temperature during summer. Estimation of SVP also affects modeled SOA, especially in a cold environment. The effects of water partitioning into OPM and nonideality of the organic–water mixture on SOA are the opposite. Since the activity coefficients of SVOCs are mostly greater than 1.0 during the simulated episode, SOA concentration decreases when the nonideality effect is considered. The averaged SOA concentration decreases by up to 20 % in winter and 50 % in summer. Water partitioning alone increases SOA by 10 %–20 % in winter and 30 %–80 % in summer. It should be noticed that the results shown in this study are the lower limit as the current model tends to underestimate SOA. It is crucial to consider both effects in simulating SOA formation under hot and humid conditions in CTMs.
The data used in this paper can be provided upon request from the corresponding authors: Qi Ying (qying@civil.tamu.edu) and Jianlin Hu (jianlinhu@nuist.edu.cn).
The supplement related to this article is available online at: https://doi.org/10.5194/acp-20-7291-2020-supplement.
JL, QY, and JH designed the research and contributed to model development and configurations. YLZ, XW, XL, and YS provided the observations of OC and OA. JL, HZ, QY, and JH analyzed the data. JL prepared the manuscript and all coauthors helped improve the manuscript.
The authors declare that they have no conflict of interest.
The authors thank James F. Pankow for providing the SOA module code. Jingyi Li acknowledges the support from the Priority Academic Program Development of Jiangsu Higher Education Institutions (PAPD) and the Six Talent Peaks Project of Jiangsu Province.
This research has been supported by the National Key R & D Program of China ((grant no. 2018YFC0213802, Task #2), the National Science Foundation of China (grant nos. 41705102 and 41875149), the Major Research Plan of the National Social Science Foundation (grant no. 18ZDA052), and the Startup Foundation for Introducing Talent of NUIST (grant no. 2243141701014).
This paper was edited by Astrid Kiendler-Scharr and reviewed by two anonymous referees.
Ansari, A. S. and Pandis, S. N.: Water Absorption by Secondary Organic Aerosol and Its Effect on Inorganic Aerosol Behavior, Environ. Sci. Technol., 34, 71–77, https://doi.org/10.1021/es990717q, 2000.
Asa-Awuku, A., Nenes, A., Gao, S., Flagan, R. C., and Seinfeld, J. H.: Water-soluble SOA from Alkene ozonolysis: composition and droplet activation kinetics inferences from analysis of CCN activity, Atmos. Chem. Phys., 10, 1585–1597, https://doi.org/10.5194/acp-10-1585-2010, 2010.
Atkinson, R. W., Kang, S., Anderson, H. R., Mills, I. C., and Walton, H. A.: Epidemiological time series studies of PM2.5 and daily mortality and hospital admissions: a systematic review and meta-analysis, Thorax, 69, 660–665, https://doi.org/10.1136/thoraxjnl-2013-204492, 2014.
Ayers, G. P.: Comment on regression analysis of air quality data, Atmos. Environ., 35, 2423–2425, https://doi.org/10.1016/S1352-2310(00)00527-6, 2001.
Bergström, R., Denier van der Gon, H. A. C., Prévôt, A. S. H., Yttri, K. E., and Simpson, D.: Modelling of organic aerosols over Europe (2002–2007) using a volatility basis set (VBS) framework: application of different assumptions regarding the formation of secondary organic aerosol, Atmos. Chem. Phys., 12, 8499–8527, https://doi.org/10.5194/acp-12-8499-2012, 2012.
Bowman, F. M. and Melton, J. A.: Effect of activity coefficient models on predictions of secondary organic aerosol partitioning, J. Aerosol Sci., 35, 1415–1438, https://doi.org/10.1016/j.jaerosci.2004.07.001, 2004.
Boylan, J. W. and Russell, A. G.: PM and light extinction model performance metrics, goals, and criteria for three-dimensional air quality models, Atmos. Environ., 40, 4946–4959, https://doi.org/10.1016/j.atmosenv.2005.09.087, 2006.
Budisulistiorini, S. H., Nenes, A., Carlton, A. G., Surratt, J. D., McNeill, V. F., and Pye, H. O. T.: Simulating Aqueous-Phase Isoprene-Epoxydiol (IEPOX) Secondary Organic Aerosol Production During the 2013 Southern Oxidant and Aerosol Study (SOAS), Environ. Sci. Technol., 51, 5026–5034, https://doi.org/10.1021/acs.est.6b05750, 2017.
Cao, C., Jiang, W., Wang, B., Fang, J., Lang, J., Tian, G., Jiang, J., and Zhu, T. F.: Inhalable Microorganisms in Beijing's PM2.5 and PM10 Pollutants during a Severe Smog Event, Environ. Sci. Technol., 48, 1499–1507, https://doi.org/10.1021/es4048472, 2014.
Cappa, C. D., Lovejoy, E. R., and Ravishankara, A. R.: Evidence for liquid-like and nonideal behavior of a mixture of organic aerosol components, P. Natl. Acad. Sci. USA, 105, 18687–18691, https://doi.org/10.1073/pnas.0802144105, 2008.
Carlton, A. G., Bhave, P. V., Napelenok, S. L., Edney, E. O., Sarwar, G., Pinder, R. W., Pouliot, G. A., and Houyoux, M.: Model Representation of Secondary Organic Aerosol in CMAQv4.7, Environ. Sci. Technol., 44, 8553–8560, https://doi.org/10.1021/es100636q, 2010.
Chang, R. Y. W., Slowik, J. G., Shantz, N. C., Vlasenko, A., Liggio, J., Sjostedt, S. J., Leaitch, W. R., and Abbatt, J. P. D.: The hygroscopicity parameter (κ) of ambient organic aerosol at a field site subject to biogenic and anthropogenic influences: relationship to degree of aerosol oxidation, Atmos. Chem. Phys., 10, 5047–5064, https://doi.org/10.5194/acp-10-5047-2010, 2010.
Denjean, C., Formenti, P., Picquet-Varrault, B., Pangui, E., Zapf, P., Katrib, Y., Giorio, C., Tapparo, A., Monod, A., Temime-Roussel, B., Decorse, P., Mangeney, C., and Doussin, J. F.: Relating hygroscopicity and optical properties to chemical composition and structure of secondary organic aerosol particles generated from the ozonolysis of α-pinene, Atmos. Chem. Phys., 15, 3339–3358, https://doi.org/10.5194/acp-15-3339-2015, 2015.
Donahue, N. M., Robinson, A. L., Stanier, C. O., and Pandis, S. N.: Coupled partitioning, dilution, and chemical aging of semivolatile organics, Environ. Sci. Technol., 40, 02635–02643, https://doi.org/10.1021/es052297c, 2006.
Duplissy, J., DeCarlo, P. F., Dommen, J., Alfarra, M. R., Metzger, A., Barmpadimos, I., Prevot, A. S. H., Weingartner, E., Tritscher, T., Gysel, M., Aiken, A. C., Jimenez, J. L., Canagaratna, M. R., Worsnop, D. R., Collins, D. R., Tomlinson, J., and Baltensperger, U.: Relating hygroscopicity and composition of organic aerosol particulate matter, Atmos. Chem. Phys., 11, 1155–1165, https://doi.org/10.5194/acp-11-1155-2011, 2011.
Ehn, M., Thornton, J. A., Kleist, E., Sipilä, M., Junninen, H., Pullinen, I., Springer, M., Rubach, F., Tillmann, R., Lee, B., Lopez-Hilfiker, F., Andres, S., Acir, I.-H., Rissanen, M., Jokinen, T., Schobesberger, S., Kangasluoma, J., Kontkanen, J., Nieminen, T., Kurtén, T., Nielsen, L. B., Jørgensen, S., Kjaergaard, H. G., Canagaratna, M., Maso, M. D., Berndt, T., Petäjä, T., Wahner, A., Kerminen, V.-M., Kulmala, M., Worsnop, D. R., Wildt, J., and Mentel, T. F.: A large source of low-volatility secondary organic aerosol, Nature, 506, 476–479, https://doi.org/10.1038/nature13032, 2014.
El-Sayed, M. M. H., Ortiz-Montalvo, D. L., and Hennigan, C. J.: The effects of isoprene and NOx on secondary organic aerosols formed through reversible and irreversible uptake to aerosol water, Atmos. Chem. Phys., 18, 1171–1184, https://doi.org/10.5194/acp-18-1171-2018, 2018.
Emery, C., Tai, E., and Yarwood, G.: Enhanced meteorological modeling and performance evaluation for two texas episodes, Report to the Texas Natural Resources Conservation Commission, prepared by ENVIRON, International Corp., Novato, CA, available at: https://www.tceq.texas.gov/assets/public/implementation/air/am/contracts/reports/mm/EnhancedMetModelingAndPerformanceEvaluation.pdf (last access: 10 May 2019), 2001.
EPA: U.S.: Guidance on the Use of Models and Other Analyses for Demonstrating Attainment of Air Quality Goals for Ozone, PM2:5, and Regional Haze, EPA-454/B-07-002, available at: https://nepis.epa.gov/Exe/ZyPDF.cgi/P1009OL1.PDF?Dockey=P1009OL1.PDF (last access: 10 May 2019), 2007.
Ervens, B., Turpin, B. J., and Weber, R. J.: Secondary organic aerosol formation in cloud droplets and aqueous particles (aqSOA): a review of laboratory, field and model studies, Atmos. Chem. Phys., 11, 11069–11102, https://doi.org/10.5194/acp-11-11069-2011, 2011.
Fredenslund, A., Jones, R. L., and Prausnitz, J. M.: Group-contribution estimation of activity coefficients in nonideal liquid mixtures, AICHE J., 21, 1086–1099, https://doi.org/10.1002/aic.690210607, 1975.
Fu, H. and Chen, J.: Formation, features and controlling strategies of severe haze-fog pollutions in China, Sci. Total Environ., 578, 121–138, https://doi.org/10.1016/j.scitotenv.2016.10.201, 2017.
Galloway, M. M., Chhabra, P. S., Chan, A. W. H., Surratt, J. D., Flagan, R. C., Seinfeld, J. H., and Keutsch, F. N.: Glyoxal uptake on ammonium sulphate seed aerosol: reaction products and reversibility of uptake under dark and irradiated conditions, Atmos. Chem. Phys., 9, 3331–3345, https://doi.org/10.5194/acp-9-3331-2009, 2009.
Gentner, D. R., Jathar, S. H., Gordon, T. D., Bahreini, R., Day, D. A., El Haddad, I., Hayes, P. L., Pieber, S. M., Platt, S. M., de Gouw, J., Goldstein, A. H., Harley, R. A., Jimenez, J. L., Prévôt, A. S. H., and Robinson, A. L.: Review of Urban Secondary Organic Aerosol Formation from Gasoline and Diesel Motor Vehicle Emissions, Environ. Sci. Technol., 51, 1074–1093, https://doi.org/10.1021/acs.est.6b04509, 2017.
Guo, H., Xu, L., Bougiatioti, A., Cerully, K. M., Capps, S. L., Hite Jr., J. R., Carlton, A. G., Lee, S. H., Bergin, M. H., Ng, N. L., Nenes, A., and Weber, R. J.: Fine-particle water and pH in the southeastern United States, Atmos. Chem. Phys., 15, 5211–5228, https://doi.org/10.5194/acp-15-5211-2015, 2015.
Hayes, P. L., Carlton, A. G., Baker, K. R., Ahmadov, R., Washenfelder, R. A., Alvarez, S., Rappenglück, B., Gilman, J. B., Kuster, W. C., de Gouw, J. A., Zotter, P., Prévôt, A. S. H., Szidat, S., Kleindienst, T. E., Offenberg, J. H., Ma, P. K., and Jimenez, J. L.: Modeling the formation and aging of secondary organic aerosols in Los Angeles during CalNex 2010, Atmos. Chem. Phys., 15, 5773–5801, https://doi.org/10.5194/acp-15-5773-2015, 2015.
He, Q., Zhang, M., and Huang, B.: Spatio-temporal variation and impact factors analysis of satellite-based aerosol optical depth over China from 2002 to 2015, Atmos. Environ., 129, 79–90, https://doi.org/10.1016/j.atmosenv.2016.01.002, 2016.
He, Q., Gu, Y., and Zhang, M.: Spatiotemporal patterns of aerosol optical depth throughout China from 2003 to 2016, Sci. Total Environ., 653, 23–35, https://doi.org/10.1016/j.scitotenv.2018.10.307, 2019.
Healy, R. M., Temime, B., Kuprovskyte, K., and Wenger, J. C.: Effect of Relative Humidity on Gas/Particle Partitioning and Aerosol Mass Yield in the Photooxidation of p-Xylene, Environ. Sci. Technol., 43, 1884–1889, https://doi.org/10.1021/es802404z, 2009.
Hodzic, A., Jimenez, J. L., Madronich, S., Canagaratna, M. R., DeCarlo, P. F., Kleinman, L., and Fast, J.: Modeling organic aerosols in a megacity: potential contribution of semi-volatile and intermediate volatility primary organic compounds to secondary organic aerosol formation, Atmos. Chem. Phys., 10, 5491–5514, https://doi.org/10.5194/acp-10-5491-2010, 2010.
Hu, J., Chen, J., Ying, Q., and Zhang, H.: One-year simulation of ozone and particulate matter in China using WRF/CMAQ modeling system, Atmos. Chem. Phys., 16, 10333–10350, https://doi.org/10.5194/acp-16-10333-2016, 2016.
Hu, J., Wang, P., Ying, Q., Zhang, H., Chen, J., Ge, X., Li, X., Jiang, J., Wang, S., Zhang, J., Zhao, Y., and Zhang, Y.: Modeling biogenic and anthropogenic secondary organic aerosol in China, Atmos. Chem. Phys., 17, 77–92, https://doi.org/10.5194/acp-17-77-2017, 2017.
Huang, R.-J., Zhang, Y., Bozzetti, C., Ho, K.-F., Cao, J.-J., Han, Y., Daellenbach, K. R., Slowik, J. G., Platt, S. M., Canonaco, F., Zotter, P., Wolf, R., Pieber, S. M., Bruns, E. A., Crippa, M., Ciarelli, G., Piazzalunga, A., Schwikowski, M., Abbaszade, G., Schnelle-Kreis, J., Zimmermann, R., An, Z., Szidat, S., Baltensperger, U., Haddad, I. E., and Prévôt, A. S. H.: High secondary aerosol contribution to particulate pollution during haze events in China, Nature, 514, 218–222, https://doi.org/10.1038/nature13774, 2014.
Iacono, M. J., Delamere, J. S., Mlawer, E. J., Shephard, M. W., Clough, S. A., and Collins, W. D.: Radiative forcing by long-lived greenhouse gases: Calculations with the AER radiative transfer models, J. Geophys. Res., 113, D13103, https://doi.org/10.1029/2008jd009944, 2008.
Jathar, S. H., Mahmud, A., Barsanti, K. C., Asher, W. E., Pankow, J. F., and Kleeman, M. J.: Water uptake by organic aerosol and its influence on gas/particle partitioning of secondary organic aerosol in the United States, Atmos. Environ., 129, 142–154, https://doi.org/10.1016/j.atmosenv.2016.01.001, 2016.
Jiang, F., Liu, Q., Huang, X., Wang, T., Zhuang, B., and Xie, M.: Regional modeling of secondary organic aerosol over China using WRF/Chem, J. Aerosol Sci., 43, 57–73, https://doi.org/10.1016/j.jaerosci.2011.09.003, 2012.
Jimenez, J. L., Canagaratna, M. R., Donahue, N. M., Prevot, A. S. H., Zhang, Q., Kroll, J. H., DeCarlo, P. F., Allan, J. D., Coe, H., Ng, N. L., Aiken, A. C., Docherty, K. S., Ulbrich, I. M., Grieshop, A. P., Robinson, A. L., Duplissy, J., Smith, J. D., Wilson, K. R., Lanz, V. A., Hueglin, C., Sun, Y. L., Tian, J., Laaksonen, A., Raatikainen, T., Rautiainen, J., Vaattovaara, P., Ehn, M., Kulmala, M., Tomlinson, J. M., Collins, D. R., Cubison, M. J., Dunlea, J., Huffman, J. A., Onasch, T. B., Alfarra, M. R., Williams, P. I., Bower, K., Kondo, Y., Schneider, J., Drewnick, F., Borrmann, S., Weimer, S., Demerjian, K., Salcedo, D., Cottrell, L., Griffin, R., Takami, A., Miyoshi, T., Hatakeyama, S., Shimono, A., Sun, J. Y., Zhang, Y. M., Dzepina, K., Kimmel, J. R., Sueper, D., Jayne, J. T., Herndon, S. C., Trimborn, A. M., Williams, L. R., Wood, E. C., Middlebrook, A. M., Kolb, C. E., Baltensperger, U., and Worsnop, D. R.: Evolution of Organic Aerosols in the Atmosphere, Science, 326, 1525–1529, https://doi.org/10.1126/science.1180353, 2009.
Kim, Y., Sartelet, K., and Couvidat, F.: Modeling the effect of non-ideality, dynamic mass transfer and viscosity on SOA formation in a 3-D air quality model, Atmos. Chem. Phys., 19, 1241–1261, https://doi.org/10.5194/acp-19-1241-2019, 2019.
Knote, C., Hodzic, A., Jimenez, J. L., Volkamer, R., Orlando, J. J., Baidar, S., Brioude, J., Fast, J., Gentner, D. R., Goldstein, A. H., Hayes, P. L., Knighton, W. B., Oetjen, H., Setyan, A., Stark, H., Thalman, R., Tyndall, G., Washenfelder, R., Waxman, E., and Zhang, Q.: Simulation of semi-explicit mechanisms of SOA formation from glyoxal in aerosol in a 3-D model, Atmos. Chem. Phys., 14, 6213–6239, https://doi.org/10.5194/acp-14-6213-2014, 2014.
Kurokawa, J., Ohara, T., Morikawa, T., Hanayama, S., Janssens-Maenhout, G., Fukui, T., Kawashima, K., and Akimoto, H.: Emissions of air pollutants and greenhouse gases over Asian regions during 2000–2008: Regional Emission inventory in ASia (REAS) version 2, Atmos. Chem. Phys., 13, 11019–11058, https://doi.org/10.5194/acp-13-11019-2013, 2013.
Lai, S., Zhao, Y., Ding, A., Zhang, Y., Song, T., Zheng, J., Ho, K. F., Lee, S.-c., and Zhong, L.: Characterization of PM2.5 and the major chemical components during a 1-year campaign in rural Guangzhou, Southern China, Atmos. Res., 167, 208–215, https://doi.org/10.1016/j.atmosres.2015.08.007, 2016.
Lambe, A. T., Onasch, T. B., Massoli, P., Croasdale, D. R., Wright, J. P., Ahern, A. T., Williams, L. R., Worsnop, D. R., Brune, W. H., and Davidovits, P.: Laboratory studies of the chemical composition and cloud condensation nuclei (CCN) activity of secondary organic aerosol (SOA) and oxidized primary organic aerosol (OPOA), Atmos. Chem. Phys., 11, 8913–8928, https://doi.org/10.5194/acp-11-8913-2011, 2011.
Levy, R. C., Remer, L. A., Kleidman, R. G., Mattoo, S., Ichoku, C., Kahn, R., and Eck, T. F.: Global evaluation of the Collection 5 MODIS dark-target aerosol products over land, Atmos. Chem. Phys., 10, 10399–10420, https://doi.org/10.5194/acp-10-10399-2010, 2010.
Li, J., Cleveland, M., Ziemba, L. D., Griffin, R. J., Barsanti, K. C., Pankow, J. F., and Ying, Q.: Modeling regional secondary organic aerosol using the Master Chemical Mechanism, Atmos. Environ., 102, 52–61, https://doi.org/10.1016/j.atmosenv.2014.11.054, 2015.
Li, J., Zhang, M., Wu, F., Sun, Y., and Tang, G.: Assessment of the impacts of aromatic VOC emissions and yields of SOA on SOA concentrations with the air quality model RAMS-CMAQ, Atmos. Environ., 158, 105–115, https://doi.org/10.1016/j.atmosenv.2017.03.035, 2017.
Li, X., Song, S., Zhou, W., Hao, J., Worsnop, D. R., and Jiang, J.: Interactions between aerosol organic components and liquid water content during haze episodes in Beijing, Atmos. Chem. Phys., 19, 12163–12174, https://doi.org/10.5194/acp-19-12163-2019, 2019.
Li, Y. J., Sun, Y., Zhang, Q., Li, X., Li, M., Zhou, Z., and Chan, C. K.: Real-time chemical characterization of atmospheric particulate matter in China: A review, Atmos. Environ., 158, 270–304, https://doi.org/10.1016/j.atmosenv.2017.02.027, 2017.
Lim, Y. B., Tan, Y., and Turpin, B. J.: Chemical insights, explicit chemistry, and yields of secondary organic aerosol from OH radical oxidation of methylglyoxal and glyoxal in the aqueous phase, Atmos. Chem. Phys., 13, 8651–8667, https://doi.org/10.5194/acp-13-8651-2013, 2013.
Lin, J., An, J., Qu, Y., Chen, Y., Li, Y., Tang, Y., Wang, F., and Xiang, W.: Local and distant source contributions to secondary organic aerosol in the Beijing urban area in summer, Atmos. Environ., 124, 176–185, https://doi.org/10.1016/j.atmosenv.2015.08.098, 2016.
Liu, J., Shen, J., Cheng, Z., Wang, P., Ying, Q., Zhao, Q., Zhang, Y., Zhao, Y., and Fu, Q.: Source apportionment and regional transport of anthropogenic secondary organic aerosol during winter pollution periods in the Yangtze River Delta, China, Sci. Total Environ., 710, 135620, https://doi.org/10.1016/j.scitotenv.2019.135620, 2020.
Liu, X.-H., Zhang, Y., Cheng, S.-H., Xing, J., Zhang, Q., Streets, D. G., Jang, C., Wang, W.-X., and Hao, J.-M.: Understanding of regional air pollution over China using CMAQ, part I performance evaluation and seasonal variation, Atmos. Environ., 44, 2415–2426, https://doi.org/10.1016/j.atmosenv.2010.03.035, 2010.
Luo, Y. X., Zheng, X. B., Zhao, T. L., and Chen, J.: A climatology of aerosol optical depth over China from recent 10 years of MODIS remote sensing data, Int. J. Climatol., 34, 863–870, https://doi.org/10.1002/joc.3728, 2014.
Malm, W. C., Sisler, J. F., Huffman, D., Eldred, R. A., and Cahill, T. A.: Spatial and seasonal trends in particle concentration and optical extinction in the United States, J. Geophys. Res., 99, 1347–1370, https://doi.org/10.1029/93JD02916, 1994.
Massoli, P., Lambe, A. T., Ahern, A. T., Williams, L. R., Ehn, M., Mikkilä, J., Canagaratna, M. R., Brune, W. H., Onasch, T. B., Jayne, J. T., Petäjä, T., Kulmala, M., Laaksonen, A., Kolb, C. E., Davidovits, P., and Worsnop, D. R.: Relationship between aerosol oxidation level and hygroscopic properties of laboratory generated secondary organic aerosol (SOA) particles, Geophys. Res. Lett., 37, 1–5, https://doi.org/10.1029/2010GL045258, 2010.
Murphy, B. N., Woody, M. C., Jimenez, J. L., Carlton, A. M. G., Hayes, P. L., Liu, S., Ng, N. L., Russell, L. M., Setyan, A., Xu, L., Young, J., Zaveri, R. A., Zhang, Q., and Pye, H. O. T.: Semivolatile POA and parameterized total combustion SOA in CMAQv5.2: impacts on source strength and partitioning, Atmos. Chem. Phys., 17, 11107–11133, https://doi.org/10.5194/acp-17-11107-2017, 2017.
Odum, J. R., Hoffmann, T., Bowman, F., Collins, D., Flagan, R. C., and Seinfeld, J. H.: Gas/Particle Partitioning and Secondary Organic Aerosol Yields, Environ. Sci. Technol., 30, 2580–2585, https://doi.org/10.1021/es950943+, 1996.
Pankow, J. F.: An absorption model of gas/particle partitioning of organic compounds in the atmosphere, Atmos. Environ., 28, 185–188, https://doi.org/10.1016/1352-2310(94)90093-0, 1994.
Pankow, J. F., Marks, M. C., Barsanti, K. C., Mahmud, A., Asher, W. E., Li, J., Ying, Q., Jathar, S. H., and Kleeman, M. J.: Molecular view modeling of atmospheric organic particulate matter: Incorporating molecular structure and co-condensation of water, Atmos. Environ., 122, 400–408, https://doi.org/10.1016/j.atmosenv.2015.10.001, 2015.
Petters, M. D. and Kreidenweis, S. M.: A single parameter representation of hygroscopic growth and cloud condensation nucleus activity, Atmos. Chem. Phys., 7, 1961–1971, https://doi.org/10.5194/acp-7-1961-2007, 2007.
Prisle, N. L., Engelhart, G. J., Bilde, M., and Donahue, N. M.: Humidity influence on gas-particle phase partitioning of α-pinene + O3 secondary organic aerosol, Geophys. Res. Lett., 37, 1–5, https://doi.org/10.1029/2009gl041402, 2010.
Pye, H. O. T., Murphy, B. N., Xu, L., Ng, N. L., Carlton, A. G., Guo, H., Weber, R., Vasilakos, P., Appel, K. W., Budisulistiorini, S. H., Surratt, J. D., Nenes, A., Hu, W., Jimenez, J. L., Isaacman-VanWertz, G., Misztal, P. K., and Goldstein, A. H.: On the implications of aerosol liquid water and phase separation for organic aerosol mass, Atmos. Chem. Phys., 17, 343–369, https://doi.org/10.5194/acp-17-343-2017, 2017.
Qiao, X., Ying, Q., Li, X., Zhang, H., Hu, J., Tang, Y., and Chen, X.: Source apportionment of PM2.5 for 25 Chinese provincial capitals and municipalities using a source-oriented Community Multiscale Air Quality model, Sci. Total Environ., 612, 462–471, https://doi.org/10.1016/j.scitotenv.2017.08.272, 2018.
Ramanathan, V., Crutzen, P. J., Kiehl, J. T., and Rosenfeld, D.: Aerosols, Climate, and the Hydrological Cycle, Science, 294, 2119–2124, https://doi.org/10.1126/science.1064034, 2001.
Rickards, A. M. J., Miles, R. E. H., Davies, J. F., Marshall, F. H., and Reid, J. P.: Measurements of the Sensitivity of Aerosol Hygroscopicity and the κ Parameter to the O∕C Ratio, J. Phys. Chem. A, 117, 14120–14131, https://doi.org/10.1021/jp407991n, 2013.
Robinson, A. L., Donahue, N. M., Shrivastava, M. K., Weitkamp, E. A., Sage, A. M., Grieshop, A. P., Lane, T. E., Pierce, J. R., and Pandis, S. N.: Rethinking Organic Aerosols: Semivolatile Emissions and Photochemical Aging, Science, 315, 1259–1262, https://doi.org/10.1126/science.1133061, 2007.
Seinfeld, J. H., Erdakos, G. B., Asher, W. E., and Pankow, J. F.: Modeling the Formation of Secondary Organic Aerosol (SOA). 2. The Predicted Effects of Relative Humidity on Aerosol Formation in the α-Pinene-, β-Pinene-, Sabinene-, Δ3-Carene-, and Cyclohexene-Ozone Systems, Environ. Sci. Technol., 35, 1806–1817, https://doi.org/10.1021/es001765+, 2001.
Shi, Z., Li, J., Huang, L., Wang, P., Wu, L., Ying, Q., Zhang, H., Lu, L., Liu, X., Liao, H., and Hu, J.: Source apportionment of fine particulate matter in China in 2013 using a source-oriented chemical transport model, Sci. Total Environ., 601–602, 1476–1487, https://doi.org/10.1016/j.scitotenv.2017.06.019, 2017.
Shrivastava, M., Cappa, C. D., Fan, J., Goldstein, A. H., Guenther, A. B., Jimenez, J. L., Kuang, C., Laskin, A., Martin, S. T., Ng, N. L., Petaja, T., Pierce, J. R., Rasch, P. J., Roldin, P., Seinfeld, J. H., Shilling, J., Smith, J. N., Thornton, J. A., Volkamer, R., Wang, J., Worsnop, D. R., Zaveri, R. A., Zelenyuk, A., and Zhang, Q.: Recent advances in understanding secondary organic aerosol: Implications for global climate forcing, Rev. Geophys., 55, 509–559, https://doi.org/10.1002/2016RG000540, 2017.
Shrivastava, M. K., Lane, T. E., Donahue, N. M., Pandis, S. N., and Robinson, A. L.: Effects of gas particle partitioning and aging of primary emissions on urban and regional organic aerosol concentrations, J. Geophys. Res., 113, D18301, https://doi.org/10.1029/2007jd009735, 2008.
Simon, H. and Bhave, P. V.: Simulating the Degree of Oxidation in Atmospheric Organic Particles, Environ. Sci. Technol., 46, 331–339, https://doi.org/10.1021/es202361w, 2012.
Sun, J., Liang, M., Shi, Z., Shen, F., Li, J., Huang, L., Ge, X., Chen, Q., Sun, Y., Zhang, Y., Chang, Y., Ji, D., Ying, Q., Zhang, H., Kota, S. H., and Hu, J.: Investigating the PM2.5 mass concentration growth processes during 2013–2016 in Beijing and Shanghai, Chemosphere, 221, 452–463, https://doi.org/10.1016/j.chemosphere.2018.12.200, 2019.
Sun, Y., Du, W., Fu, P., Wang, Q., Li, J., Ge, X., Zhang, Q., Zhu, C., Ren, L., Xu, W., Zhao, J., Han, T., Worsnop, D. R., and Wang, Z.: Primary and secondary aerosols in Beijing in winter: sources, variations and processes, Atmos. Chem. Phys., 16, 8309–8329, https://doi.org/10.5194/acp-16-8309-2016, 2016.
Sun, Y. L., Wang, Z. F., Fu, P. Q., Yang, T., Jiang, Q., Dong, H. B., Li, J., and Jia, J. J.: Aerosol composition, sources and processes during wintertime in Beijing, China, Atmos. Chem. Phys., 13, 4577–4592, https://doi.org/10.5194/acp-13-4577-2013, 2013.
Tkacik, D. S., Presto, A. A., Donahue, N. M., and Robinson, A. L.: Secondary Organic Aerosol Formation from Intermediate-Volatility Organic Compounds: Cyclic, Linear, and Branched Alkanes, Environ. Sci. Technol., 46, 8773–8781, https://doi.org/10.1021/es301112c, 2012.
Varutbangkul, V., Brechtel, F. J., Bahreini, R., Ng, N. L., Keywood, M. D., Kroll, J. H., Flagan, R. C., Seinfeld, J. H., Lee, A., and Goldstein, A. H.: Hygroscopicity of secondary organic aerosols formed by oxidation of cycloalkenes, monoterpenes, sesquiterpenes, and related compounds, Atmos. Chem. Phys., 6, 2367–2388, https://doi.org/10.5194/acp-6-2367-2006, 2006.
Wang, H. B., Tian, M., Li, X. H., Chang, Q., Cao, J. J., Yang, F. M., Ma, Y. L., and He, K. B.: Chemical Composition and Light Extinction Contribution of PM2:5 in Urban Beijing for a 1-Year Period, Aerosol Air Qual. Res., 15, 2200–2211, https://doi.org/10.4209/aaqr.2015.04.0257, 2015.
Wang, K., Zhang, Y., Jang, C., Phillips, S., and Wang, B.: Modeling intercontinental air pollution transport over the trans-Pacific region in 2001 using the Community Multiscale Air Quality modeling system, J. Geophys. Res., 114, D04307, https://doi.org/10.1029/2008JD010807, 2009.
Wiedensohler, A., Cheng, Y. F., Nowak, A., Wehner, B., Achtert, P., Berghof, M., Birmili, W., Wu, Z. J., Hu, M., Zhu, T., Takegawa, N., Kita, K., Kondo, Y., Lou, S. R., Hofzumahaus, A., Holland, F., Wahner, A., Gunthe, S. S., Rose, D., Su, H., and Pöschl, U.: Rapid aerosol particle growth and increase of cloud condensation nucleus activity by secondary aerosol formation and condensation: A case study for regional air pollution in northeastern China, J. Geophys. Res., 114, D00G08, https://doi.org/10.1029/2008JD010884, 2009.
Wiedinmyer, C., Akagi, S. K., Yokelson, R. J., Emmons, L. K., Al-Saadi, J. A., Orlando, J. J., and Soja, A. J.: The Fire INventory from NCAR (FINN): a high resolution global model to estimate the emissions from open burning, Geosci. Model Dev., 4, 625–641, https://doi.org/10.5194/gmd-4-625-2011, 2011.
Woody, M. C., Baker, K. R., Hayes, P. L., Jimenez, J. L., Koo, B., and Pye, H. O. T.: Understanding sources of organic aerosol during CalNex-2010 using the CMAQ-VBS, Atmos. Chem. Phys., 16, 4081–4100, https://doi.org/10.5194/acp-16-4081-2016, 2016.
Ying, Q., Cureño, I. V., Chen, G., Ali, S., Zhang, H., Malloy, M., Bravo, H. A., and Sosa, R.: Impacts of Stabilized Criegee Intermediates, surface uptake processes and higher aromatic secondary organic aerosol yields on predicted PM2.5 concentrations in the Mexico City Metropolitan Zone, Atmos. Environ., 94, 438–447, https://doi.org/10.1016/j.atmosenv.2014.05.056, 2014.
Ying, Q., Li, J., and Kota, S. H.: Significant Contributions of Isoprene to Summertime Secondary Organic Aerosol in Eastern United States, Environ. Sci. Technol., 49, 7834–7842, https://doi.org/10.1021/acs.est.5b02514, 2015.
Zhang, H., Hu, J., Kleeman, M., and Ying, Q.: Source apportionment of sulfate and nitrate particulate matter in the Eastern United States and effectiveness of emission control programs, Sci. Total Environ., 490, 171–181, https://doi.org/10.1016/j.scitotenv.2014.04.064, 2014.
Zhang, Q., Streets, D. G., Carmichael, G. R., He, K. B., Huo, H., Kannari, A., Klimont, Z., Park, I. S., Reddy, S., Fu, J. S., Chen, D., Duan, L., Lei, Y., Wang, L. T., and Yao, Z. L.: Asian emissions in 2006 for the NASA INTEX-B mission, Atmos. Chem. Phys., 9, 5131–5153, https://doi.org/10.5194/acp-9-5131-2009, 2009.
Zhao, B., Wang, S., Donahue, N. M., Jathar, S. H., Huang, X., Wu, W., Hao, J., and Robinson, A. L.: Quantifying the effect of organic aerosol aging and intermediate-volatility emissions on regional-scale aerosol pollution in China, Sci. Rep., 6, 28815, https://doi.org/10.1038/srep28815, 2016.
Zhao, D. F., Buchholz, A., Kortner, B., Schlag, P., Rubach, F., Fuchs, H., Kiendler-Scharr, A., Tillmann, R., Wahner, A., Watne, Å. K., Hallquist, M., Flores, J. M., Rudich, Y., Kristensen, K., Hansen, A. M. K., Glasius, M., Kourtchev, I., Kalberer, M., and Mentel, T. F.: Cloud condensation nuclei activity, droplet growth kinetics, and hygroscopicity of biogenic and anthropogenic secondary organic aerosol (SOA), Atmos. Chem. Phys., 16, 1105–1121, https://doi.org/10.5194/acp-16-1105-2016, 2016.
Zhao, J., Qiu, Y., Zhou, W., Xu, W., Wang, J., Zhang, Y., Li, L., Xie, C., Wang, Q., Du, W., Worsnop, D. R., Canagaratna, M. R., Zhou, L., Ge, X., Fu, P., Li, J., Wang, Z., Donahue, N. M., and Sun, Y.: Organic Aerosol Processing During Winter Severe Haze Episodes in Beijing, J. Geophys. Res., 124, 10248–10263, https://doi.org/10.1029/2019jd030832, 2019.
Zhao, Y., Hennigan, C. J., May, A. A., Tkacik, D. S., de Gouw, J. A., Gilman, J. B., Kuster, W. C., Borbon, A., and Robinson, A. L.: Intermediate-Volatility Organic Compounds: A Large Source of Secondary Organic Aerosol, Environ. Sci. Technol., 48, 13743–13750, https://doi.org/10.1021/es5035188, 2014.
Zheng, B., Zhang, Q., Zhang, Y., He, K. B., Wang, K., Zheng, G. J., Duan, F. K., Ma, Y. L., and Kimoto, T.: Heterogeneous chemistry: a mechanism missing in current models to explain secondary inorganic aerosol formation during the January 2013 haze episode in North China, Atmos. Chem. Phys., 15, 2031–2049, https://doi.org/10.5194/acp-15-2031-2015, 2015.