the Creative Commons Attribution 4.0 License.
the Creative Commons Attribution 4.0 License.
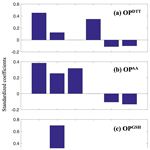
Characterization and comparison of PM2.5 oxidative potential assessed by two acellular assays
Dong Gao
Krystal J. Godri Pollitt
James A. Mulholland
Armistead G. Russell
The capability of ambient particles to generate in vivo reactive oxygen species (ROS), called oxidative potential (OP), is a potential metric for evaluating the health effects of particulate matter (PM) and is supported by several recent epidemiological investigations. Studies using various types of OP assays differ in their sensitivities to varying PM chemical components. In this study, we systematically compared two health-relevant acellular OP assays that track the depletion of antioxidants or reductant surrogates: (i) the synthetic respiratory-tract lining fluid (RTLF) assay that tracks the depletion of ascorbic acid (AA) and glutathione (GSH) and (ii) the dithiothreitol (DTT) assay that tracks the depletion of DTT. Yearlong daily samples were collected at an urban site in Atlanta, GA (Jefferson Street), during 2017, and both DTT and RTLF assays were performed to measure the OP of water-soluble PM2.5 components. PM2.5 mass and major chemical components, including metals, ions, and organic and elemental carbon were also analyzed. Correlation analysis found that OP as measured by the DTT and AA depletion (OPDTT and OPAA, respectively) were correlated with both organics and some water-soluble metal species, whereas that from the GSH depletion (OPGSH) was exclusively sensitive to water-soluble Cu. These OP assays were moderately correlated with each other due to the common contribution from metal ions. OPDTT and OPAA were moderately correlated with PM2.5 mass with Pearson's r=0.55 and 0.56, respectively, whereas OPGSH exhibited a lower correlation (r=0.24). There was little seasonal variation in the OP levels for all assays due to the weak seasonality of OP-associated species. Multivariate linear regression models were developed to predict OP measures from the particle composition data. Variability in OPDTT and OPAA were not only attributed to the concentrations of metal ions (mainly Fe and Cu) and organic compounds but also to antagonistic metal–organic and metal–metal interactions. OPGSH was sensitive to the change in water-soluble Cu and brown carbon (BrC), a proxy for ambient humic-like substances.
- Article
(2600 KB) - Full-text XML
-
Supplement
(910 KB) - BibTeX
- EndNote
Epidemiological studies have consistently reported associations between particulate matter (PM) and increased morbidity and mortality (Brunekreef and Holgate, 2002; Cohen et al., 2017; Lippmann, 2014; Norris et al., 1999; Pope et al., 2004; Samet et al., 2000; Sun et al., 2010; Thurston et al., 2017). The capacity of inhaled PM to elicit oxidative stress has emerged as a hypothesis to explain PM-induced adverse health effects. Inhaled PM can directly introduce PM-bound reactive oxygen species (ROS) to the surface of the lung, where they react with and deplete lung-lining fluid antioxidants, or introduce redox-active PM species, which can react with biological reductants and generate ROS in vivo (Lakey et al., 2016). The latter can occur in organs beyond the lungs by particles or chemical species being translocated from the lungs throughout the body. Oxidative stress arises when the presence and production of ROS overwhelms the antioxidant's defenses, and can lead to cell and tissue damage and induction of chronic and degenerative diseases (Das, 2016; Halliwell, 1994; Pizzino et al., 2017). The ability of PM to generate ROS in vivo, referred to as the oxidative potential (OP) of particles, has gained increasing attention as a possibly more integrative health-relevant measure of ambient PM toxicity than PM mass concentration, which may contain a mix of highly toxic (e.g., polycyclic aromatic hydrocarbons (PAHs), quinones, environmentally persistent free radicals, highly oxygenated organic molecules, and transition metals) to relatively benign (e.g., sulfate and ammonium nitrate) PM components (Frampton et al., 1999; Khachatryan et al., 2011; Lippmann, 2014; Tong et al., 2018, 2019).
A variety of acellular assays have been developed to assess PM OP (Ayres et al., 2008; Bates et al., 2019). In general, these assays involve the incubation of PM extracts or suspension with chemical reagents and probes, and the response is recorded over time or after incubation. The responses recorded include the depletion of reductant surrogate, such as the dithiothreitol (DTT) assay (Cho et al., 2005), and depletion of specific antioxidants in a composite solution, such as a synthetic respiratory-tract lining fluid (RTLF) model (Godri et al., 2011; Mudway et al., 2004; Zielinski et al., 1999). In contrast, other assays measure ROS generation (e.g., the dichlorofluorescein assay; Huang et al., 2016; Venkatachari et al., 2005) or hydroxyl radical formation in the presence of H2O2 (e.g., electron paramagnetic, EPR, or electron spin resonance, ESR; Shi et al., 2003a, b). The assays based on exposing PM species to antioxidants are currently more commonly used. The DTT assay (Cho et al., 2005) is a chemical system that mimics the in vivo PM-catalyzed electron transfer process. In this assay, DTT acts as a surrogate of the cellular reductant (NADH or NADPH), donating electrons to oxygen and producing ROS with the catalytic assistance of PM redox-active species. PM OP (i.e., OPDTT in this case) is determined by measuring the depletion of DTT over time, which is assumed to be proportional to the concentration of redox-active compounds in PM. For the RTLF assay, RTLF is constructed to simulate the aqueous environment that particles first encounter when inhaled into the lungs and deposited. The antioxidants in RTLF, specifically the major low-molecular-weight antioxidants, ascorbic acid (AA), uric acid (UA), and reduced glutathione (GSH), provide protective defenses against PM-induced oxidative damage. The extent to which they are depleted by PM over time reflects a direct measure of PM oxidative activity by this assay, expressed as OPAA, OPUA, and OPGSH (Kelly et al., 1996; Mudway et al., 2004; Zielinski et al., 1999). Some studies (DiStefano et al., 2009; Fang et al., 2016; Janssen et al., 2015; Mudway et al., 2005) have used a simplified alternative approach to AA analysis in RTLF, where only the single antioxidant AA is contained in the solution. If not explicitly stated, OPAA in this paper represents OPAA obtained from the RTLF model.
Recently, a growing number of epidemiological studies have used these OP assays to examine the link between particle OP and adverse health outcomes. OPDTT of ambient fine particles has been found to be more strongly associated with multiple cardiorespiratory outcomes, such as airway inflammation (Delfino et al., 2013; Janssen et al., 2015; Yang et al., 2016), asthma (Abrams et al., 2017; Bates et al., 2015; Fang et al., 2016; Yang et al., 2016), and congestive heart failure (Bates et al., 2015; Fang et al., 2016), than PM mass. Multiple population-scale studies employed the RTLF assay to assess OP of PM2.5 and found that OPGSH was associated with lung cancer, cardiometabolic mortality (Weichenthal et al., 2016a), emergency room visits for respiratory illness (Weichenthal et al., 2016c), myocardial infarction (Weichenthal et al., 2016b), and adverse birth outcomes (Lavigne et al., 2018). The association between airway inflammation in asthmatic children and OPGSH of PM2.5 personal exposure was also reported (Maikawa et al., 2016). In the study of Strak et al. (2012), no association was found between OPGSH and acute airway inflammation in healthy volunteers after 5 h of exposure. A population-scale study in London, UK, found no association between OPGSH and mortality and hospital admission (Atkinson et al., 2016). For the AA depletion, either in the composite RTLF model or in a simplified AA-only model, no association with adverse health end points has been found, including asthma, cause-specific mortality, and cardiorespiratory emergency department visits (Fang et al., 2016; Maikawa et al., 2016; Weichenthal et al., 2016a; Weichenthal et al., 2016b; Weichenthal et al., 2016c). Bates et al. (2019) provide a review of the relationships of various OP assays with adverse health effects.
The differences in observed health effects may be due to the different sensitivity of OP assays to various PM species. Past studies have shown that specific assays are correlated with different PM components. OPDTT has been found to be sensitive to transition metals (Charrier and Anastasio, 2012; Fang et al., 2016; Verma et al., 2015a) and organic species, especially more oxygenated aromatic organics, such as quinones and hydroxyquinones (Cho et al., 2005; Kumagai et al., 2002; McWhinney et al., 2011; Verma et al., 2015b). OPAA obtained from the simplified AA-only model are mostly responsive to the metal content of PM (Fang et al., 2016; Maikawa et al., 2016; Yang et al., 2014). Antioxidants (AA and GSH) within the synthetic RTLF are responsive to a slightly different group of metals. For example, OPAA responds to iron and OPGSH is related to aluminum (Godri et al., 2010). However, both OPAA and OPGSH are sensitive to copper (Ayres et al., 2008). Studies performed on real PM samples or standard solutions indicate that quinones also drive the oxidative losses of both antioxidants (Ayres et al., 2008; Calas et al., 2018; Kelly et al., 2011; Pietrogrande et al., 2019).
Since different assays capture different chemical fractions of the oxidative activity of PM, it is challenging to synthesize the findings from OP health studies. There remains a need to compare different assays on identical particle samples to advance our understanding of the effects of PM species on OP measures and in turn assess the results of the health studies that use OP. In this study, we used two acellular OP assays, DTT and RTLF, to measure the water-soluble OP of ambient PM2.5 collected from urban Atlanta, GA, over a 1-year period. These two assays were chosen since they are currently most commonly used and have shown significant associations with adverse health outcomes in some studies. A suite of chemical components was also measured on these samples and univariate and multivariate linear regression analyses were performed to identify and evaluate the contribution of major chemical components to each of these OP metrics.
2.1 Sampling
Yearlong sampling was conducted in 2017 from 1 January to 30 December at the Jefferson Street SEARCH (Southeastern Aerosol Research and Characterization) site (Edgerton et al., 2006, 2005). Jefferson Street is situated roughly 4.2 km northwest of downtown Atlanta and 2.3 km from a major interstate highway and is representative of the urban Atlanta region. The site has been extensively used in past studies characterizing urban Atlanta air quality (Hansen et al., 2006) and the data used in OP and epidemiological studies (Abrams et al., 2017; Bates et al., 2015; Fang et al., 2016; Sarnat, 2008; Verma et al., 2014).
Ambient PM2.5 was collected daily (from midnight to midnight, 24 h integrated samples) onto pre-baked 203×254 mm quartz filters (Pallflex Tissuquartz, Pall Life Sciences) using high-volume samplers (HiVol, Thermo Anderson, nominal flow rate 1.13 m3 min−1, PM2.5 impactor). A total of 349 filter samples were collected for analysis; missing days were due to instrumentation issues. The HiVol quartz filters were wrapped in pre-baked aluminum foil after collection and stored at −18 ∘C until analysis. PM2.5 mass concentration was monitored continuously by a tapered element oscillating microbalance (TEOM, Thermo Scientific TEOM 1400a), the sample stream dried at 30 ∘C using a Sample Equilibration System (Meyer et al., 2000). A Sunset semicontinuous OCEC analyzer (Sunset Laboratory) was used to provide in situ measurements of organic and elemental carbon (OC ∕ EC) content of fine PM. The data were obtained hourly by using 1 h cycles in which the instrument sampled ambient air through an activated carbon denuder for 45 min and analyzed the particles collected on the quartz filter for 15 min using the NIOSH 5040 analysis protocol (Birch and Cary, 1996).
2.2 Oxidative potential measurements
Two acellular assays, DTT and RTLF assays, were performed to measure the oxidative potential of water-soluble PM2.5. The DTT analysis was completed at Georgia Institute of Technology, and all filters were analyzed within 1 month after collection. The RTLF assay was conducted at Yale University during October 2018. Prior to the OP analyses, a fraction of each HiVol filter (5.1 cm2 for DTT and 4.5 cm2 for RTLF) was punched out, placed in a sterile polypropylene centrifuge vial (VWR International LLC, Suwanee, GA, USA), and then extracted in 5 mL of deionized water (DI, >18MΩ) via 30 min sonication. The water extract was filtered through a 0.45 µm PTFE syringe filter (Fisherbrand, Fisher Scientific) and then used for OP analysis.
2.2.1 DTT assay
The DTT assay was performed with a semiautomated system developed by Fang et al. (2015b), following the protocol described by Cho et al. (2005). In brief, the PM extract (3.5 mL; 40±15 µg mL−1 of PM) was incubated with DTT solution (0.5 mL; 1 mM) and potassium phosphate buffer (1 mL; pH ∼7.4, Chelex resin treated) at 37 ∘C. A small aliquot (100 µL) of the mixture was withdrawn at designated times (0, 4, 13, 23, 31 and 41 min) and mixed with trichloroacetic acid (TCA, 1 % w∕v) to quench the DTT reactions. After addition of Tris buffer (pH ∼8.9), the remaining DTT was reacted with 5,5′-dithiobis-(2-nitrobenzoic acid) (DTNB) to form a colored product that absorbs light at 412 nm. The final mixture was pushed through a 10 cm path length liquid waveguide capillary cell (LWCC; World Precision Instruments, Inc., FL, USA), and the light absorption was recorded by an online spectrometer, which included a UV-Vis light source (DT-mini-2, Ocean Optics, Inc., Dunedin, FL, USA) and a multiwavelength light detector (USB4000 Miniature Fiber Optic Spectrometer). The DTT consumption rate, used as a measure of OP, was determined from the slope of the linear regression of DTT residual vs. time. Good linearity was found for all samples with correlation coefficients (R2) larger than 0.98. In parallel with all sample batches, at least one field blank and one positive control (9,10-phenanthraquinone) was analyzed, and their OP values remained constant throughout the analysis. The limit of detection (LOD), defined as 3 times the standard deviation of OPDTT for blanks, was 0.31 nmol min−1. The PM OP measured by this assay (i.e., OPDTT) was blank-corrected and normalized by the air volume that passed through the extracted filter fraction, expressed as nmol DTT min−1 m−3. This approach did not involve the use of PM samples with constant mass, which is sometimes employed to limit nonlinear DTT response to certain metal ions (Charrier et al., 2016).
2.2.2 RTLF assay
The RTLF assay is based on the protocol adopted by Maikawa et al. (2016). PM water extracts (35±13 µg of PM mL−1) were transferred into a 96-well microplate with 180 µL of sample liquid in each well. A total of 20 µL of synthetic RTLF (pH ∼7.0) containing equimolar concentrations (2 mM) of AA, UA, and GSH was added into each well, resulting in a final starting concentration of 200 µM of antioxidants. The PM-RTLF mixture was incubated in a plate reader (SpectraMax190, Molecular Devices, LLC, San Jose, CA, USA) for 4 h at 37 ∘C with gentle mixing. Following incubation, the concentrations of AA and GSH were analyzed immediately. UA concentration was not measured since studies have consistently suggested that no depletion of UA was observed in the presence of PM (Kunzli et al., 2006; Mudway et al., 2004; Zielinski et al., 1999).
AA concentration was determined with the plate reader by measuring the light absorbance at 260 nm. The GSH concentration was indirectly quantified by measuring total glutathione (GSx) and oxidized glutathione (GSSG) concentrations, both compounds determined using the enzymatic recycling method (Baker et al., 1990). The incubated PM-RTLF mixture was diluted 49-fold with 100 mM sodium phosphate buffer (pH ∼7.5) containing ethylenediaminetetraacetic acid (EDTA). To measure the GSx concentration, 50 µL of each diluted sample was dispensed onto a microplate. A total of 100 µL of reaction mixture (0.15 mM DTNB, 0.2 mM NADPH, and 1 U glutathione reductase, GR) was added to each well. In the mixture, GSH reacted with DTNB, forming a yellow-colored product 5-thio-2-nitrobenzoic acid (TNB) and the mixed disulfide GS-TNB. In the presence of NAPDH and GR, GSSG and GS-TNB were reduced back to GSH, leading to more TNB production. The plate was analyzed on the plate reader for 2 min under constant mixing to continuously monitor the formation of TNB. The TNB formation rate, which is proportional to the GSH concentration, was measured at an absorbance of 405 nm. For GSSG measurement, 5 µL of 2-vinylpyridine was added to 130 µL of the diluted sample to conjugate GSH. The solution was incubated at room temperature for 1 h, followed by similar procedures performed for the GSx measurement. The GSH concentration was calculated by subtracting 2 times the GSSG concentration from the measured GSx concentration.
Field blanks and known controls (e.g., for positive controls H2O2 and Cu were used and Zn was used as a negative control) were run in parallel with all sample batches. All samples and controls were measured in triplicate. The percentage of AA and GSH depletion after 4 h incubation for each PM sample was calculated relative to the field blank. The LOD for AA and GSH depletion after 4 h incubation was 4.0 % and 4.5 %, respectively. PM OP obtained from this assay, i.e., OPAA and OPGSH, was determined by normalizing the percentage loss with the sampled air volume, in unit of percentage depletion per cubic meter.
2.3 Chemical analysis on PM filters
2.3.1 Elemental analysis
Both total and water-soluble trace metals were determined by inductively coupled plasma mass spectrometry (ICP-MS) (Agilent 7500a series, Agilent Technologies, Inc., CA, USA), including magnesium (Mg), aluminum (Al), potassium (K), calcium (Ca), chromium (Cr), manganese (Mn), iron (Fe), copper (Cu), and zinc (Zn). For the determination of concentrations of total metals, a 1.5 cm2 filter punch from the HiVol quartz filter was acid-digested for 20 min using aqua regia (HNO3 + 3HCl). The acid-digested sample was then diluted in DI water to 10 mL and filtered with a 0.45 µm PTFE syringe filter. For the analysis of water-soluble metals, no digestion was performed. In this case, one circular punch (5.1 cm2) was extracted in 5 mL of DI via 30 min sonication. The extract was filtered using a 0.45 µm PTFE syringe filter and then acid-preserved by adding concentrated nitric acid (70 %) to a final concentration of 2 % (v∕v).
2.3.2 Water-soluble organic carbon (WSOC) and brown carbon (BrC)
Two 1.5 cm2 filter punches from the HiVol filter were extracted in 15 mL DI in a pre-baked glass centrifuge vial (DWK Life Sciences, Rockwood, TN, USA) by 30 min sonication. The extracts filtered with 0.45 µm PTFE syringe filters were used to measure water-soluble organic carbon (WSOC) and its light absorption properties (BrC, used as a source tracer). A fraction (∼6 mL) of filter extract was injected by a syringe pump (Kloehn, Inc., NV, USA) into a 2.5 m path length LWCC (World Precision Instruments, Inc., FL, USA), with an internal volume of 500 µL . The absorbance at 365 nm wavelength (BrC) was measured by an online spectrophotometer. The remaining liquid extract was drawn into Sievers total organic carbon (TOC) analyzer (Model 900, GE Analytical Instruments, Boulder, CO, USA) for determination of WSOC concentration. The TOC was calibrated with a series of prepared sucrose standards.
2.3.3 Water-soluble ionic species
One 1.5 cm2 filter punch from the HiVol filter was extracted in 10 mL DI via sonication. The inorganic ions (, , and ) in the filtered water extracts were measured by ion exchange chromatography (IC) with conductivity detection. For anion measurements, eluent containing 3.2 mM Na2CO3 and 1.0 mM NaHCO3 was passed through an anion separation column (Metrosep A Supp 5-150/4.0) at a flow rate of 0.78 mL min−1. For cation measurements, eluent containing 1.7 mM HNO3 and 0.7 mM dipicolinic acid was passed through a cation separation column (Metrosep C4-150/4.0) at a flow rate of 0.9 mL min−1. An automated sampler (Dionex AS40, Thermo Fisher Scientific, Waltham, MA) was used to inject samples into IC.
2.4 Multivariate regression models
Multivariate linear regression models were developed to predict OPs based on PM speciation data and investigate the relative importance of species on different OPs. Prior to the regression analysis, boxplots were used to identify the outliers and test the normality of data. Extreme values (a total of ∼3 % of the OP measurements) were removed from the data set. Linear regression was performed between PM components and the various OPs. To simplify the analysis, PM components correlated with OP (r>0.4, p<0.05) were selected as the independent variables of the models. A stepwise regression was applied to the data set using MATLAB R2016a to form the multivariate regression models. To evaluate the performance of the final models, 5-fold cross-validation was employed and repeated 50 times. For each OP measure, the average mean-squared error over 50 iterations was within 25 % of the mean OP value (23.4 %, 17.9 %, and 12.2 % for OPDTT, OPAA, and OPGSH, respectively).
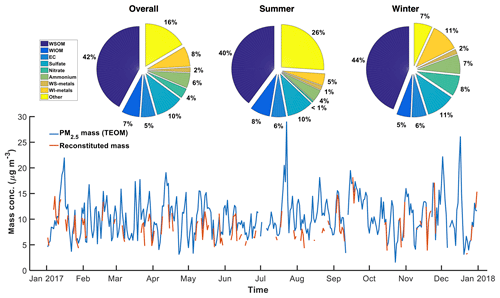
Figure 1Time series of PM2.5 mass concentration. The pie charts show the average aerosol composition based on PM2.5 mass measured by the tapered element oscillating microbalance (TEOM) during the whole sampling year, summer, and winter. WSOM is water-soluble organic matter (); WIOM is water-insoluble organic matter (); WS-metals is the sum of water-soluble metals, including Al, Mg, Ca, K, Fe, Cu, Mn, Zn; and WI-metals are water-insoluble metals (= total_metals–WS_metals). Summer is June–August, and winter is January–February and November–December.
3.1 Ambient PM composition
Figure 1 shows the time series of PM2.5 mass concentration and the averaged chemical composition of ambient particles collected at the site. A factor of 1.6 was applied to convert organic carbon to organic matter (Turpin and Lim, 2001; Weber, 2003). Reconstituted mass from measured chemical species agreed well with PM2.5 mass measured by the TEOM with Pearson's r=0.84, and accounted for more than 80 % of the PM2.5 mass. The missing mass may result from other species not measured, semi-volatile material lost from the filter, and the uncertainty in converting measured carbon mass to organic matter (factor of 1.6 used).
Fractions of various chemical components in PM2.5 are consistent with previous observations (Edgerton et al., 2005; Verma et al., 2014). In general, PM mass was dominated by organic compounds (WSOM + WIOM 50 %), followed by inorganic ions (10 % , 4 %–7 % , and 1 %–8 % ). Metals constituted 6 %–13 % of the PM mass, among which water-soluble metals were at trace amounts (1 %–2 %). EC accounted for a small fraction of the PM mass (5 %–6 %). , and , which are semi-volatile, accounted for a larger fraction of fine particle mass during the cold season. The metal fraction also increased in winter, whereas the fractions of other PM components did not vary significantly during the sampling period.
Although insoluble PM components also play an important role in OP (Gao et al., 2017; Verma et al., 2012), this study solely focuses on water-soluble OP measurements, and thus the water-soluble PM components are the primary focus in this study. The OP (DTT assay only) contribution from water-insoluble components was discussed in detail in another paper from this study (Gao et al., 2020).
3.2 Association of OP with PM components
Pearson's correlation coefficients for the linear regression between OP and select chemical components are shown in Table 1. The detailed correlation matrices for different seasonal periods are given in Tables S1–S3 in the Supplement. We defined the strength of the absolute correlation coefficient value as strong for values ≥0.65, moderate from 0.40 to 0.65, and weak for values <0.4. The OP assays were moderately intercorrelated over the sampling year. In all cases (whole year and summer or winter), OPAA and OPGSH had the highest correlations, which may in part be due to these measurements being conducted on the same sample extracts. As for OPDTT, the correlations with OPAA and OPGSH varied but were largely similar. The correlations between the OP measures and various PM components varied, highlighting the different sensitivities of OP assays to various PM components. Note these correlations do not imply that the compounds are responsible for the OP as some of them are not redox active compounds. However, the correlations may indicate the emission sources (as source tracers, e.g., vehicular emissions for EC, secondary processing for WSOC and , and biomass burning for BrC and K), which also probably emit the water-soluble species contributing to the measured OP.
As shown in Table 1, OPDTT was correlated with OC and WSOC, indicating a contribution from PM organic compounds. The correlations between OPDTT and certain water-soluble metals, such as Fe, Cu, and Mn, were also observed. A moderate correlation of EC with OPDTT (r=0.51) and somewhat with metals and OC (r=0.55, 0.43, and 0.83 for Fe, Mn, and OC, respectively; Table S1) suggests that incomplete combustion could be one of their common sources. The associations found in this study between OPDTT and PM composition are consistent with a number of previous studies (Fang et al., 2016, 2015b; Verma et al., 2014; Yang et al., 2014), though the correlations in our work were weaker (r>0.5, compared with r>0.65 in other studies).
Similar to OPDTT, OPAA was moderately correlated with OC, WSOC, and water-soluble metals, mainly Fe and Cu (r=0.47–0.55). The results are compared with a previous study conducted in the same Atlanta region by Fang et al. (2016), wherein a simplified AA assay was applied to assess water-soluble OP of PM2.5. The AA depletion in the AA-only model was found to be strongly correlated with water-soluble Cu with Pearson's r>0.65, and associations with WSOC (or BrC) and metals were also observed. The weaker sensitivity of AA to water-soluble Cu observed in our study is possibly due to the reactivity hierarchy existing within the antioxidant model with GSH > AA (Zielinski et al., 1999). This is further supported by other studies. In the study of Charrier et al. (2011), ligand speciation modeling indicated that GSH was a stronger ligand compared to AA and caused a dramatic shift in Cu speciation by forming Cu–GSH complexes. The experimental results in the study of Pietrogrande et al. (2019) showed that the response of the acellular AA assay was strongly dependent on the composition of synthetic RTLF used and the presence of GSH and UA would lower the sensitivity of AA response to Cu. The correlations of organics and EC, which comprised a large fraction of PM mass (Fig. 1), with OPDTT and OPAA, likely account for the OPDTT and OPAA correlations with PM mass.
In contrast to OPDTT and OPAA, OPGSH was found to be exclusively correlated with water-soluble Cu with Pearson's r>0.7. The consistently lower correlation of OPAA with water-soluble Cu than OPGSH with Cu, is consistent with GSH outcompeting AA in the RTLF in forming Cu complexes. The results are also consistent with other studies (Aliaga et al., 2010; Ayres et al., 2008; Godri et al., 2011).
The correlations differed by seasons. In winter, OPAA and OPDTT were more correlated with organic species, with stronger associations with WSOC, BrC, and K, indicating biomass burning as a common source of OPAA and OPDTT. In summer, all OP assays tended to be metal-driven. OPAA and OPDTT were more correlated with Cu, along with , suggesting possible influence of secondary processing on metal mobilization (Fang et al., 2017; Ghio et al., 1999) and resulting in a strong intercorrelation between different OP metrics.
3.3 Temporal variation
The time series of the monthly and seasonal averages of different OP measures are shown in Fig. 2. Significant seasonal variability in these OP measures was not evident; only a subtle seasonal variation observed for OPDTT and no variations for OPAA and OPGSH. OPDTT was slightly higher during the cold period (January–February and November–December) with an average level of 0.24±0.08 nmol min−1 m−3 compared to 0.20±0.04 nmol min−1 m−3 in the warm period (May–August) and a median OPDTT ratio between two periods of 1.20. However, OPAA and OPGSH had more similar levels across seasons, with median ratios between cold and warm periods of 1.10 and 0.97, respectively.
The seasonality in OP measures should result from the temporal variations in PM species driving the various OP. From the temporal variation in the OP-associated species shown in Fig. 3 (the seasonal averaged concentrations were given in Fig. S1 in the Supplement), BrC had an obvious seasonality, i.e., higher in winter and lower in summer, which is due to the stronger influence of biomass burning in winter. The variation in BrC may lead to the small variation in OPDTT, considering the good correlation between OPDTT and BrC in winter. Water-soluble Cu is slightly higher in midsummer (August) and water-soluble Fe is slightly higher in fall (September), but these trends are not seen in the various measures of OP.
3.4 Multivariate model
Given that one or more PM components contributed to these measures of OP, multivariate linear regression analysis was conducted to identify the main PM components that drive the variability in OP and provide a contrast between the assays. Water-soluble organic species (WSOC or BrC) and metals, mainly Fe, Cu, Mn, were selected as the independent variables to form multivariate linear regression models for OPDTT and OPAA, based on their high correlations, as noted above. EC, though also correlated with OPDTT and OPAA, was not chosen as one of the predictors due to its correlations with selected water-soluble species (e.g., WSOC, water-soluble Mn and Fe). For OPGSH, WSOC and BrC were used as input in addition to water-soluble Cu to include the possible influence of organic species on OPGSH. The resulting linear relationships between different OP measures and PM components are shown in Table 2. The time series of measured and predicted OPs and the contributions of model variables to each OP measure are given in Fig. S2. Overall, the multivariate models explained variability in OP measures reasonably well with the coefficients of determination between modeled and measured OPs (R2) greater than 0.4, with the models better capturing the OPAA and OPGSH variability. In the regression results for OPDTT and OPAA, components including water-soluble Fe, Cu, and BrC (or WSOC) and interaction terms between metal and organics and metal and metal were included, suggesting that the variability of OPDTT or OPAA is dependent upon not only bulk concentrations of PM components but also interactions between species. The regression model for OPGSH captured the contributions from Cu and BrC but had no interaction terms. The intercept in each regression model, though large and accounting for over 50 % of the mean of OP measures (Fig. S2), is practically meaningless because the regression models are applicable only when the PM components are at ambient concentrations.
Table 2Multivariate linear regression models for OP metrics.

All metals are water-soluble metals. The values represent the coefficients for variables. Cells are left blank when the corresponding variable is not included in the equation. As an example, the linear equation of OPDTT is as follows: OP. The concentrations of water-soluble metals are in units of ng m−3, whereas the units for WSOC and BrC are given in µg m−3 and Mm−1, respectively.
All models captured the contributions from organic species; however, the organic contributions in different models were represented by different measures of organics. In the OPDTT and OPGSH models, the organic contribution was denoted by BrC, whereas WSOC was used in the OPAA model. Although WSOC and BrC were correlated with each other (Tables S1–S3), there is a difference between these two parameters. The OP contribution from BrC, an optically defined measure, may not be straightforward; however, the optical properties of BrC are related to chemical properties. There is evidence showing that BrC components may directly contribute to OP (Chen et al., 2019). It has been found that BrC predominantly represents the hydrophobic organic fraction (i.e., the humic-like substances (HULIS) fraction) in PM (Verma et al., 2012) and is largely from incomplete combustion (mainly biomass burning) (Hecobian et al., 2010). For example, quinones, as a subset of the HULIS fraction (Verma et al., 2015b), can be estimated better with BrC than with WSOC. WSOC also includes organic compounds present in the hydrophilic fraction, e.g., levoglucosan (Lin and Yu, 2011), and low-molecular-weight organic acids (Sullivan and Weber, 2006) and thus is a more integrative measure of organic compounds compared to BrC. The difference between BrC and WSOC is also supported by the different seasonal variation observed in BrC and WSOC (Fig. 3).
For OPDTT results, the presence of Cu and BrC in the equation is as expected, since Cu and organic species have been found to be active in DTT oxidation (Charrier and Anastasio, 2012; Cho et al., 2005). However, Fe, which has a low intrinsic DTT activity (Charrier and Anastasio, 2012), was found to be predictive of OPDTT, likely suggesting that Fe represents surrogate measures of constituents with intrinsic redox active properties which were not quantified. This is also supported by the evidence that Fe had correlations with other PM constituents such as OC and EC (Tables S1–S3), which may suggest that Fe in the PM water extracts is solubilized by forming complexes with combustion-derived organic species. The interaction terms, along with their negative coefficients, suggest antagonistic interactions between Cu and organic compounds and between Cu and Fe. The interaction between metal and organics, though not taken into account when applying multivariate regression analysis in previous studies (Calas et al., 2018; Verma et al., 2015a), is consistent with experimental results (Yu et al., 2018), wherein antagonistic interactions between Cu and ambient HULIS were observed in the DTT consumption. However, the interaction between metals contrasts with experiments which showed additive effects for metal mixtures (Yu et al., 2018). However, it should be noted that the interactions among metals were usually tested with mixtures of individual species, which can only poorly represent the complex chemistry of ambient PM.
For RTLF assay, the variability of OPAA was attributed to the concentrations of Fe, Cu, and WSOC; antagonistic metal–organic interaction between Fe and organic compounds; and metal–metal competition between Fe and Cu. Even though the RTLF assay in previous studies was generally used to measure the OP of methanol-extracted PM suspension, the contributions from metals and organics observed in our water-soluble OP agree with previous results (Ayres et al., 2008; Kelly et al., 2011; Pietrogrande et al., 2019). The presence of interaction terms is novel and is supported by little empirical evidence. The antagonistic interaction between Fe and WSOC is reasonable. Fe in the water extracts has been found largely complexed with organic compounds (Wei et al., 2019). Since AA is not a strong ligand for Fe (Charrier and Anastasio, 2011), the complexation between Fe and organic compounds can prevent Fe from reacting with AA. For OPGSH, despite weak correlation of OPGSH with BrC, BrC still accounted for the variability in OPGSH, consistent with previous findings that OPGSH is responsive to quinones (Ayres et al., 2008; Calas et al., 2018).
It is noteworthy that the multivariate regression models do not account for the possible nonlinear behavior of species with OP responses. For example, nonlinear concentration–response curves have been found for DTT oxidation by dissolved Cu and Mn (Charrier and Anastasio, 2012), which may not be characterized in the multivariate regression model, potentially affecting the accuracy of the OPDTT model. We note that the variables in the models not only represent the contribution from individual species but also show a possible influence from co-emitted unquantified components. There are also interactions that exist among PM species, affecting the relationships between PM compounds and OP metrics.
To further investigate the sensitivity of different OP assays to PM species, standardized regression was applied to rescale the variables measured in different units and make the coefficients in the regression equations comparable. It is also an effective way to reduce collinearity induced by the intercorrelated nature of PM species and the existence of interaction terms. The standardized coefficient of a specific component indicates the estimated change in an OP measure for every one-unit increase in components. The higher the absolute value of the standardized coefficient, the stronger the effect of the PM species on the OP measure.
Figure 4 shows the relative importance of each PM component to OP metrics based on the calculation of standardized coefficients. As shown in Fig. 4, water soluble Fe was the most important variable in the model of OPDTT, followed by BrC, Cu, and antagonistic interactions. Even though OPDTT is not responsive to water-soluble Fe, Fe may be a surrogate measure of compounds co-emitted with Fe from brake and tire wear and secondary formation, which have been identified as two major sources of Fe in the southeastern US (Fang et al., 2015a). For OPAA, the strength of the effects of Fe, Cu, and WSOC on OPAA was similar. OPGSH was approximately 4 times more sensitive to Cu than to BrC, with standardized coefficients of 0.70 and 0.18 for Cu and BrC, respectively. These results show clear contrasts between the assays, where OPDTT and OPAA are more similar and both have significant contrasts to OPGSH.
In all of these cases, it must also be kept in mind that the measurements were performed on the PM water extracts, which are not the conditions that are found in the ambient aerosol. Thus, inferring associations between species in the extracts and applying to ambient conditions is not straightforward; however, this analysis is useful for interpreting and contrasting the possible causes for associations between these types of assays and any health effects.
Water-soluble Fe, as the most important determinant of OPDTT, has been estimated to have the strongest effect on cardiovascular outcomes in the Atlanta metropolitan region (Ye et al., 2018), which may account for associations between OPDTT and health outcomes observed in this region. OPGSH is strongly dependent on a limited number of PM components, and thus associations between OPGSH and health outcomes may vary more significantly by region than other assays do and associations could be expected if the PM toxicity in a region is mainly driven by specific species, such as water-soluble Cu. OPAA is affected by the composition of synthetic lung fluid, and thus the AA responses obtained from RTLF and AA-only model are not comparable and should be distinguished from each other. In the RTLF model, the response in the OPAA metric by PM is diminished due to the presence of GSH, possibly leading to weaker associations between OPAA and health endpoints.
In this study, a comparison was made between two of the most common techniques used for the assessment of PM oxidative potential based on antioxidant depletion from a complex synthetic RTLF (OPAA and OPGSH) and DTT oxidation (OPDTT). These two assays were used to quantify the water-soluble OP of ambient PM2.5 collected in urban Atlanta over a 1-year period based on daily filter samples. We observed moderate correlations among the OP assays, suggesting different sensitivities of OP measures to PM species. Univariate and multivariate regression analyses indicated that OPDTT and OPAA were correlated to organic species and water-soluble metals (Fe and Cu) and were negatively affected by the interactions among species. At a more detailed level, for organic components, OPDTT was associated specifically with HULIS and incomplete combustion products identified by BrC, whereas OPAA was associated to a more general measure of organic components, WSOC. OPGSH, though also affected by organic species, was predominantly sensitive to water-soluble Cu. Subtle temporal variation in OPDTT and no seasonal variations in OPAA and OPGSH were observed, which appears to be due to little seasonality in the combined PM constituents affecting each assay. A small OPDTT variation was associated with variation in BrC that was higher in the cold seasons.
This study suggests that all three OP metrics, OPAA and OPGSH in the RTLF assay, as well as OPDTT in the DTT assay, are associated with transition metal ions. However, OPDTT and OPAA are more chemically integrative OP measures compared to OPGSH and thus may be more informative and helpful in linking OP with health end points. The multivariate regression models for different OP measures indicate the degree to which OP variability in the PM water extracts is predicted by PM constituents.
We should note that the filter samples used in this study were averaged over 24 h, which may dampen variability in the emission sources that contribute to OP and obscure the impact of specific species (e.g., traffic-related metals) on redox activity of PM. Furthermore, all of these results were obtained from a specific location in Atlanta, GA, and should be interpreted and generalized with caution, as the chemical composition or sources of PM varies by region. There are marked differences in RTLF composition in different levels of the respiratory tract. The synthetic RTLF reflects select antioxidants in the lung and other key constituents are not represented in this simplified chemical model. The DTT assay is also subject to similar limitations, as DTT cannot fully represent biological complexities. However, these assays can be used as PM screening tools and provide rapid health-relevant assessment of PM.
All data are uploaded and available at: https://doi.pangaea.de/10.1594/PANGAEA.908043 (last access: 20 April 2020, Gao et al., 2019).
The supplement related to this article is available online at: https://doi.org/10.5194/acp-20-5197-2020-supplement.
DG collected and analyzed the data and drafted the manuscript. KJGP assisted with RTLF measurements. JAM and AGR helped with model development. The data were interpreted by DG and RJW. RJW conceived, designed, and oversaw the study. All authors discussed the results and contributed to the final manuscript.
The authors declare that they have no conflict of interest.
This publication was made possible by Georgia Tech EAS Jefferson St Fund, which was a generous gift from Georgia Power/Southern Company. We would like to thank Linghan Zeng, Gigi Pavur, Joseph Caggiano, and Allison Weber for assisting with the sampling campaigns and the lab work.
This research has been supported by Georgia Tech EAS Jefferson St Fund (GT project No. GTF 350000064).
This paper was edited by Alex Lee and reviewed by two anonymous referees.
Abrams, J. Y., Weber, R. J., Klein, M., Sarnat, S. E., Chang, H. H., Strickland, M. J., Verma, V., Fang, T., Bates, J. T., Mulholland, J. A., Russell, A. G., and Tolbert, P. E.: Associations between Ambient Fine Particulate Oxidative Potential and Cardiorespiratory Emergency Department Visits, Environ. Health Persp., 125, 107008, https://doi.org/10.1289/EHP1545, 2017.
Aliaga, M. E., Carrasco-Pozo, C., Lopez-Alarcon, C., and Speisky, H.: The Cu(I)-glutathione complex: factors affecting its formation and capacity to generate reactive oxygen species, Transit. Metal Chem., 35, 321–329, 2010.
Atkinson, R. W., Samoli, E., Analitis, A., Fuller, G. W., Green, D. C., Anderson, H. R., Purdie, E., Durister, C., Aitlhadj, L., Kelly, F. J., and Mudway, I. S.: Short-term associations between particle oxidative potential and daily mortality and hospital admissions in London, Int. J. Hyg. Envir. Heal. 219, 566–572, 2016.
Ayres, J. G., Borm, P., Cassee, F. R., Castranova, V., Donaldson, K., Ghio, A., Harrison, R. M., Hider, R., Kelly, F., Kooter, I. M., Marano, F., Maynard, R. L., Mudway, I., Nel, A., Sioutas, C., Smith, S., Baeza-Squiban, A., Cho, A., Duggan, S., and Froines, J.: Evaluating the toxicity of airborne particulate matter and nanoparticles by measuring oxidative stress potential – A workshop report and consensus statement, Inhal. Toxicol., 20, 75–99, 2008.
Baker, M. A., Cerniglia, G. J., and Zaman, A.: Microtiter Plate Assay for the Measurement of Glutathione and Glutathione Disulfide in Large Numbers of Biological Samples, Anal. Biochem., 190, 360–365, 1990.
Bates, J. T., Weber, R. J., Abrams, J., Verma, V., Fang, T., Klein, M., Strickland, M. J., Sarnat, S. E., Chang, H. H., Mulholland, J. A., Tolbert, P. E., and Russell, A. G.: Reactive Oxygen Species Generation Linked to Sources of Atmospheric Particulate Matter and Cardiorespiratory Effects, Environ. Sci. Technol., 49, 13605–13612, 2015.
Bates, J. T., Fang, T., Verma, V., Zeng, L. H., Weber, R. J., Tolbert, P. E., Abrams, J. Y., Sarnat, S. E., Klein, M., Mulholland, J. A., and Russell, A. G.: Review of Acellular Assays of Ambient Particulate Matter Oxidative Potential: Methods and Relationships with Composition, Sources, and Health Effects, Environ. Sci. Technol., 53, 4003–4019, 2019.
Birch, M. E. and Cary, R. A.: Elemental carbon-based method for monitoring occupational exposures to particulate diesel exhaust, Aerosol Sci. Tech., 25, 221–241, 1996.
Brunekreef, B. and Holgate, S. T.: Air pollution and health, Lancet, 360, 1233–1242, 2002.
Calas, A., Uzu, G., Kelly, F. J., Houdier, S., Martins, J. M. F., Thomas, F., Molton, F., Charron, A., Dunster, C., Oliete, A., Jacob, V., Besombes, J.-L., Chevrier, F., and Jaffrezo, J.-L.: Comparison between five acellular oxidative potential measurement assays performed with detailed chemistry on PM10 samples from the city of Chamonix (France), Atmos. Chem. Phys., 18, 7863–7875, https://doi.org/10.5194/acp-18-7863-2018, 2018.
Charrier, J. G. and Anastasio, C.: Impacts of antioxidants on hydroxyl radical production from individual and mixed transition metals in a surrogate lung fluid, Atmos. Environ., 45, 7555–7562, 2011.
Charrier, J. G. and Anastasio, C.: On dithiothreitol (DTT) as a measure of oxidative potential for ambient particles: evidence for the importance of soluble transition metals, Atmos. Chem. Phys., 12, 9321–9333, https://doi.org/10.5194/acp-12-9321-2012, 2012.
Charrier, J. G., McFall, A. S., Vu, K. K. T., Baroi, J., Olea, C., Hasson, A., and Anastasio, C.: A bias in the “mass-normalized” DTT response – An effect of non-linear concentration-response curves for copper and manganese, Atmos. Environ., 144, 325–334, 2016.
Chen, Q., Wang, M., Wang, Y., Zhang, L., Li, Y., and Han, Y.: Oxidative Potential of Water-Soluble Matter Associated with Chromophoric Substances in PM2.5 over Xi'an, China, Environ. Sci. Technol., 53, 8574–8584, 2019.
Cho, A. K., Sioutas, C., Miguel, A. H., Kumagai, Y., Schmitz, D. A., Singh, M., Eiguren-Fernandez, A., and Froines, J. R.: Redox activity of airborne particulate matter at different sites in the Los Angeles Basin, Environ. Res., 99, 40–47, 2005.
Cohen, A. J., Brauer, M., Burnett, R., Anderson, H. R., Frostad, J., Estep, K., Balakrishnan, K., Brunekreef, B., Dandona, L., Dandona, R., Feigin, V., Freedman, G., Hubbell, B., Jobling, A., Kan, H., Knibbs, L., Liu, Y., Martin, R., Morawska, L., Pope, C. A., Shin, H., Straif, K., Shaddick, G., Thomas, M., van Dingenen, R., van Donkelaar, A., Vos, T., Murray, C. J. L., and Forouzanfar, M. H.: Estimates and 25-year trends of the global burden of disease attributable to ambient air pollution: an analysis of data from the Global Burden of Diseases Study 2015, Lancet, 389, 1907–1918, 2017.
Das, S. K.: Special issue on “Free radicals and antioxidants in health and diseases” Preface, Indian J. Exp. Biol., 54, 681–683, 2016.
Delfino, R. J., Staimer, N., Tjoa, T., Gillen, D. L., Schauer, J. J., and Shafer, M. M.: Airway inflammation and oxidative potential of air pollutant particles in a pediatric asthma panel, J. Expo. Sci. Env. Epid., 23, 466–473, 2013.
DiStefano, E., Eiguren-Fernandez, A., Delfino, R. J., Sioutas, C., Froines, J. R., and Cho, A. K.: Determination of metal-based hydroxyl radical generating capacity of ambient and diesel exhaust particles, Inhal. Toxicol., 21, 731–738, 2009.
Edgerton, E. S., Hartsell, B. E., Saylor, R. D., Jansen, J. J., Hansen, D. A., and Hidy, G. M.: The southeastern aerosol research and characterization study: Part II, Filter-based measurements of fine and coarse particulate matter mass and composition, J. Air Waste Manage., 55, 1527–1542, 2005.
Edgerton, E. S., Hartsell, B. E., Saylor, R. D., Jansen, J. J., Hansen, D. A., and Hidy, G. M.: The Southeastern Aerosol Research and Characterization Study, Part 3: Continuous measurements of fine particulate matter mass and composition, J. Air Waste Manage., 56, 1325–1341, 2006.
Fang, T., Guo, H., Verma, V., Peltier, R. E., and Weber, R. J.: PM2.5 water-soluble elements in the southeastern United States: automated analytical method development, spatiotemporal distributions, source apportionment, and implications for heath studies, Atmos. Chem. Phys., 15, 11667–11682, https://doi.org/10.5194/acp-15-11667-2015, 2015a.
Fang, T., Verma, V., Guo, H., King, L. E., Edgerton, E. S., and Weber, R. J.: A semi-automated system for quantifying the oxidative potential of ambient particles in aqueous extracts using the dithiothreitol (DTT) assay: results from the Southeastern Center for Air Pollution and Epidemiology (SCAPE), Atmos. Meas. Tech., 8, 471–482, https://doi.org/10.5194/amt-8-471-2015, 2015b.
Fang, T., Verma, V., Bates, J. T., Abrams, J., Klein, M., Strickland, M. J., Sarnat, S. E., Chang, H. H., Mulholland, J. A., Tolbert, P. E., Russell, A. G., and Weber, R. J.: Oxidative potential of ambient water-soluble PM2.5 in the southeastern United States: contrasts in sources and health associations between ascorbic acid (AA) and dithiothreitol (DTT) assays, Atmos. Chem. Phys., 16, 3865–3879, https://doi.org/10.5194/acp-16-3865-2016, 2016.
Fang, T., Guo, H. Y., Zeng, L. H., Verma, V., Nenes, A., and Weber, R. J.: Highly Acidic Ambient Particles, Soluble Metals, and Oxidative Potential: A Link between Sulfate and Aerosol Toxicity, Environ. Sci. Technol., 51, 2611–2620, 2017.
Frampton, M. W., Ghio, A. J., Samet, J. M., Carson, J. L., Carter, J. D., and Devlin, R. B.: Effects of aqueous extracts of PM10 filters from the Utah Valley on human airway epithelial cells, Am. J. Physiol.-Lung C, 277, 960–967, 1999.
Gao, D.: Oxidative potential and PM2.5 species data for SEARCH site Jefferson Street, 2017, PANGAEA, available at: https://doi.pangaea.de/10.1594/PANGAEA.908043 (last access: 20 April 2020), 2019.
Gao, D., Fang, T., Verma, V., Zeng, L., and Weber, R. J.: A method for measuring total aerosol oxidative potential (OP) with the dithiothreitol (DTT) assay and comparisons between an urban and roadside site of water-soluble and total OP, Atmos. Meas. Tech., 10, 2821–2835, https://doi.org/10.5194/amt-10-2821-2017, 2017.
Gao, D., Mulholland, J. A., Russell, A. G., and Weber, R. J.: Characterization of water-insoluble oxidative potential of PM2.5 using the dithiothreitol assay, Atmos. Environ., 224, 117327, https://doi.org/10.1016/j.atmosenv.2020, 2020.
Ghio, A. J., Stoneheurner, J., McGee, J. K., and Kinsey, J. S.: Sulfate content correlates with iron concentrations in ambient air pollution particles, Inhal. Toxicol., 11, 293–307, 1999.
Godri, K. J., Duggan, S. T., Fuller, G. W., Baker, T., Green, D., Kelly, F. J., and Mudway, I. S.: Particulate Matter Oxidative Potential from Waste Transfer Station Activity, Environ. Health Persp., 118, 493–498, 2010.
Godri, K. J., Harrison, R. M., Evans, T., Baker, T., Dunster, C., Mudway, I. S., and Kelly, F. J.: Increased Oxidative Burden Associated with Traffic Component of Ambient Particulate Matter at Roadside and Urban Background Schools Sites in London, Plos One, 6, e21961, https://doi.org/10.1371/journal.pone.002196, 2011.
Halliwell, B.: Free-Radicals, Antioxidants, and Human-Disease – Curiosity, Cause, or Consequence, Lancet, 344, 721–724, 1994.
Hansen, D. A., Edgerton, E., Hartsell, B., Jansen, J., Burge, H., Koutrakis, P., Rogers, C., Suh, H., Chow, J., Zielinska, B., McMurry, P., Mulholland, J., Russell, A., and Rasmussen, R.: Air quality measurements for the aerosol research and inhalation epidemiology study, J. Air Waste Manage., 56, 1445–1458, 2006.
Hecobian, A., Zhang, X., Zheng, M., Frank, N., Edgerton, E. S., and Weber, R. J.: Water-Soluble Organic Aerosol material and the light-absorption characteristics of aqueous extracts measured over the Southeastern United States, Atmos. Chem. Phys., 10, 5965–5977, https://doi.org/10.5194/acp-10-5965-2010, 2010.
Huang, W., Zhang, Y. X., Zhang, Y., Fang, D. Q., and Schauer, J. J.: Optimization of the Measurement of Particle-Bound Reactive Oxygen Species with 2′, 7′-dichlorofluorescin (DCFH), Water Air Soil Poll., 227, 164, https://doi.org/10.1007/s11270-016-2860-9, 2016.
Janssen, N. A. H., Strak, M., Yang, A., Hellack, B., Kelly, F. J., Kuhlbusch, T. A. J., Harrison, R. M., Brunekreef, B., Cassee, F. R., Steenhof, M., and Hoek, G.: Associations between three specific a-cellular measures of the oxidative potential of particulate matter and markers of acute airway and nasal inflammation in healthy volunteers, Occup. Environ. Med., 72, 49–56, 2015.
Kelly, F. J., Cotgrove, M., and Mudway, I. S.: Respiratory tract lining fluid antioxidants: the first line of defence against gaseous pollutants, Cent. Eur. J. Pub. Heal., 4, 11–14, 1996.
Kelly, F., Anderson, H. R., Armstrong, B., Atkinson, R., Barratt, B., Beevers, S., Derwent, D., Green, D., Mudway, I., Wilkinson, P., and Committee, H. E. I. H. R.: The impact of the congestion charging scheme on air quality in London, Part 2. Analysis of the oxidative potential of particulate matter, Res. Rep. Health Eff. Inst., 2011, 73–144, 2011.
Khachatryan, L., Vejerano, E., Lomnicki, S., and Dellinger, B.: Environmentally persistent free radicals (EPFRs), 1. Generation of reactive oxygen species in aqueous solutions, Environ. Sci. Technol., 45, 8559–8566, 2011.
Kumagai, Y., Koide, S., Taguchi, K., Endo, A., Nakai, Y., Yoshikawa, T., and Shimojo, N.: Oxidation of proximal protein sulfhydryls by phenanthraquinone, a component of diesel exhaust particles, Chem. Res. Toxicol., 15, 483–489, 2002.
Kunzli, N., Mudway, I. S., Gotschi, T., Shi, T. M., Kelly, F. J., Cook, S., Burney, P., Forsberg, B., Gauderman, J. W., Hazenkamp, M. E., Heinrich, J., Jarvis, D., Norback, D., Payo-Losa, F., Poli, A., Sunyer, J., and Borm, P. J. A.: Comparison of oxidative properties, light absorbance, and total and elemental mass concentration of ambient PM2.5 collected at 20 European sites, Environ. Health Persp., 114, 684–690, 2006.
Lakey, P. S. J., Berkemeier, T., Tong, H. J., Arangio, A. M., Lucas, K., Poschl, U., and Shiraiwa, M.: Chemical exposure-response relationship between air pollutants and reactive oxygen species in the human respiratory tract, Sci. Rep.-UK, 6, 32916, https://doi.org/10.1038/srep32916, 2016.
Lavigne, É., Burnett, R.T., Stieb, D.M., Evans, G.J., Godri Pollitt, K. J., Chen, H., van Rijswijk, D. and Weichenthal, S.: Fine particulate air pollution and adverse birth outcomes: effect modification by regional nonvolatile oxidative potential, Environ. Health Persp., 126, 077012, https://doi.org/10.1289/EHP2535, 2018.
Lin, P. and Yu, J. Z.: Generation of Reactive Oxygen Species Mediated by Humic-like Substances in Atmospheric Aerosols, Environ. Sci. Technol., 45, 10362–10368, 2011.
Lippmann, M.: Toxicological and epidemiological studies of cardiovascular effects of ambient air fine particulate matter (PM2.5) and its chemical components: Coherence and public health implications, Crit. Rev. Toxicol., 44, 299–347, 2014.
Maikawa, C. L., Weichenthal, S., Wheeler, A. J., Dobbin, N. A., Smargiassi, A., Evans, G., Liu, L., Goldberg, M. S., and Pollitt, K. J. G.: Particulate Oxidative Burden as a Predictor of Exhaled Nitric Oxide in Children with Asthma, Environ. Health Persp., 124, 1616–1622, 2016.
McWhinney, R. D., Gao, S. S., Zhou, S. M., and Abbatt, J. P. D.: Evaluation of the Effects of Ozone Oxidation on Redox-Cycling Activity of Two-Stroke Engine Exhaust Particles, Environ. Sci. Technol., 45, 2131–2136, 2011.
Meyer, M. B., Patashnick, H., Ambs, J. L., and Rupprecht, E.: Development of a sample equilibration system for the TEOM continuous PM monitor, J. Air Waste Manage., 50, 1345–1349, 2000.
Mudway, I. S., Stenfors, N., Duggan, S. T., Roxborough, H., Zielinski, H., Marklund, S. L., Blomberg, A., Frew, A. J., Sandstrom, T., and Kelly, F. J.: An in vitro and in vivo investigation of the effects of diesel exhaust on human airway lining fluid antioxidants, Arch. Biochem. Biophys., 423, 200–212, 2004.
Mudway, I. S., Duggan, S. T., Venkataraman, C., Habib, G., Kelly, F. J., and Grigg, J.: Combustion of dried animal dung as biofuel results in the generation of highly redox active fine particulates, Part. Fibre Toxicol., 2, 6, https://doi.org/10.1186/1743-8977-2-6, 2005.
Norris, G., YoungPong, S. N., Koenig, J. Q., Larson, T. V., Sheppard, L., and Stout, J. W.: An association between fine particles and asthma emergency department visits for children in Seattle, Environ. Health Persp., 107, 489–493, 1999.
Pietrogrande, M. C., Bertoli, I., Manarini, F., and Russo, M.: Ascorbate assay as a measure of oxidative potential for ambient particles: Evidence for the importance of cell-free surrogate lung fluid composition, Atmos. Environ., 211, 103–112, 2019.
Pizzino, G., Irrera, N., Cucinotta, M., Pallio, G., Mannino, F., Arcoraci, V., Squadrito, F., Altavilla, D., and Bitto, A.: Oxidative Stress: Harms and Benefits for Human Health, Oxid. Med. Cell. Longev., 2017, 8416763, https://doi.org/10.1155/2017/8416763, 2017.
Pope, C. A., Burnett, R. T., Thurston, G. D., Thun, M. J., Calle, E. E., Krewski, D., and Godleski, J. J.: Cardiovascular mortality and long-term exposure to particulate air pollution – Epidemiological evidence of general pathophysiological pathways of disease, Circulation, 109, 71–77, 2004.
Samet, J. M., Dominici, F., Curriero, F. C., Coursac, I., and Zeger, S. L.: Fine particulate air pollution and mortality in 20 US Cities, 1987–1994, New Engl. J. Med., 343, 1742–1749, 2000.
Sarnat, J. A.: Fine Particle Sources and Cardiorespiratory Morbidity: An Application of Chemical Mass Balance and Factor Analytical Source Apportionment Methods, Epidemiology, 19, 459–466, 2008.
Shi, T., Knaapen, A. M., Begerow, J., Birmili, W., Borm, P. J. A., and Schins, R. P. F.: Temporal variation of hydroxyl radical generation and 8-hydroxy-2′-deoxyguanosine formation by coarse and fine particulate matter, Occup. Environ. Med., 60, 315–321, 2003a.
Shi, T. M., Schins, R. P. F., Knaapen, A. M., Kuhlbusch, T., Pitz, M., Heinrich, J., and Borm, P. J. A.: Hydroxyl radical generation by electron paramagnetic resonance as a new method to monitor ambient particulate matter composition, J. Environ. Monitor., 5, 550–556, 2003b.
Strak, M., Janssen, N. A. H., Godri, K. J., Gosens, I., Mudway, I. S., Cassee, F. R., Lebret, E., Kelly, F. J., Harrison, R. M., Brunekreef, B., Steenhof, M., and Hoek, G.: Respiratory Health Effects of Airborne Particulate Matter: The Role of Particle Size, Composition, and Oxidative Potential-The RAPTES Project, Environ. Health Persp., 120, 1183–1189, 2012.
Sullivan, A. P. and Weber, R. J.: Chemical characterization of the ambient organic aerosol soluble in water: 1. Isolation of hydrophobic and hydrophilic fractions with a XAD-8 resin, J. Geophys. Res.-Atmos., 111, https://doi.org/10.1029/2005JD006485, 2006.
Sun, Q. H., Hong, X. R., and Wold, L. E.: Cardiovascular Effects of Ambient Particulate Air Pollution Exposure, Circulation, 121, 2755–2765, 2010.
Thurston, G. D., Kipen, H., Annesi-Maesano, I., Balmes, J., Brook, R. D., Cromar, K., De Matteis, S., Forastiere, F., Forsberg, B., Frampton, M. W., Grigg, J., Heederik, D., Kelly, F. J., Kuenzli, N., Laumbach, R., Peters, A., Rajagopalan, S. T., Rich, D., Ritz, B., Samet, J. M., Sandstrom, T., Sigsgaard, T., Sunyer, J., and Brunekreef, B.: A joint ERS/ATS policy statement: what constitutes an adverse health effect of air pollution? An analytical framework, Eur. Respir. J., 49, https://doi.org/10.1183/13993003.00419-2016, 2017.
Tong, H., Lakey, P. S., Arangio, A. M., Socorro, J., Shen, F., Lucas, K., Brune, W. H., Pöschl, U., and Shiraiwa, M.: Reactive oxygen species formed by secondary organic aerosols in water and surrogate lung fluid, Environ. Sci. Technol., 52, 11642–11651, 2018.
Tong, H., Zhang, Y., Filippi, A., Wang, T., Li, C., Liu, F., Leppla, D., Kourtchev, I., Wang, K., Keskinen, H.M. and Levula, J.T.: Radical formation by fine particulate matter associated with highly oxygenated molecules, Environ. Sci. Technol., 53, 12506–12518, 2019.
Turpin, B. J. and Lim, H. J.: Species contributions to PM2.5 mass concentrations: Revisiting common assumptions for estimating organic mass, Aerosol. Sci. Tech., 35, 602–610, 2001.
Venkatachari, P., Hopke, P. K., Grover, B. D., and Eatough, D. J.: Measurement of particle-bound reactive oxygen species in rubidoux aerosols, J. Atmos. Chem., 52, 325–326, 2005.
Verma, V., Rico-Martinez, R., Kotra, N., King, L., Liu, J. M., Snell, T. W., and Weber, R. J.: Contribution of Water-Soluble and Insoluble Components and Their Hydrophobic/Hydrophilic Subfractions to the Reactive Oxygen Species-Generating Potential of Fine Ambient Aerosols, Environ. Sci. Technol., 46, 11384–11392, 2012.
Verma, V., Fang, T., Guo, H., King, L., Bates, J. T., Peltier, R. E., Edgerton, E., Russell, A. G., and Weber, R. J.: Reactive oxygen species associated with water-soluble PM2.5 in the southeastern United States: spatiotemporal trends and source apportionment, Atmos. Chem. Phys., 14, 12915–12930, https://doi.org/10.5194/acp-14-12915-2014, 2014.
Verma, V., Fang, T., Xu, L., Peltier, R. E., Russell, A. G., Ng, N. L., and Weber, R. J.: Organic Aerosols Associated with the Generation of Reactive Oxygen Species (ROS) by Water-Soluble PM2.5, Environ. Sci. Technol., 49, 4646–4656, 2015a.
Verma, V., Wang, Y., El-Afifi, R., Fang, T., Rowland, J., Russell, A. G., and Weber, R. J.: Fractionating ambient humic-like substances (HULIS) for their reactive oxygen species activity – Assessing the importance of quinones and atmospheric aging, Atmos. Environ., 120, 351–359, 2015b.
Weber, R.: Short-term temporal variation in PM2.5 mass and chemical composition during the Atlanta supersite experiment, 1999, J. Air Waste Manage., 53, 84–91, 2003.
Wei, J. L., Yu, H. R., Wang, Y. X., and Verma, V.: Complexation of Iron and Copper in Ambient Particulate Matter and Its Effect on the Oxidative Potential Measured in a Surrogate Lung Fluid, Environ. Sci. Technol., 53, 1661–1671, 2019.
Weichenthal, S., Crouse, D. L., Pinault, L., Godri-Pollitt, K., Lavigne, E., Evans, G., van Donkelaar, A., Martin, R. V., and Burnett, R. T.: Oxidative burden of fine particulate air pollution and risk of cause-specific mortality in the Canadian Census Health and Environment Cohort (CanCHEC), Environ. Res., 146, 92–99, 2016a.
Weichenthal, S., Lavigne, E., Evans, G., Pollitt, K., and Burnett, R. T.: Ambient PM2.5 and risk of emergency room visits for myocardial infarction: impact of regional PM2.5 oxidative potential: a case-crossover study, Environ. Health-Glob., 15, 46, https://doi.org/10.1186/s12940-016-0129-9, 2016b.
Weichenthal, S. A., Lavigne, E., Evans, G. J., Pollitt, K. J. G., and Burnett, R. T.: Fine Particulate Matter and Emergency Room Visits for Respiratory Illness Effect Modification by Oxidative Potential, Am. J. Resp. Crit. Care, 194, 577–586, 2016c.
Yang, A., Janssen, N. A. H., Brunekreef, B., Cassee, F. R., Hoek, G., and Gehring, U.: Children's respiratory health and oxidative potential of PM2.5: the PIAMA birth cohort study, Occup. Environ. Med., 73, 154–160, 2016.
Yang, A., Jedynska, A., Hellack, B., Kooter, I., Hoek, G., Brunekreef, B., Kuhlbusch, T. A. J., Cassee, F. R., and Janssen, N. A. H.: Measurement of the oxidative potential of PM2.5 and its constituents: The effect of extraction solvent and filter type, Atmos. Environ., 83, 35–42, 2014.
Ye, D. N., Klein, M., Mulholland, J. A., Russell, A. G., Weber, R., Edgerton, E. S., Chang, H. H., Sarnat, J. A., Tolbert, P. E., and Sarnat, S. E.: Estimating Acute Cardiovascular Effects of Ambient PM2.5 Metals, Environ. Health Persp., 126, 027007, https://doi.org/10.1289/EHP2182, 2018.
Yu, H. R., Wei, J. L., Cheng, Y. L., Subedi, K., and Verma, V.: Synergistic and Antagonistic Interactions among the Particulate Matter Components in Generating Reactive Oxygen Species Based on the Dithiothreitol Assay, Environ. Sci. Technol., 52, 2261–2270, 2018.
Zielinski, H., Mudway, I. S., Berube, K. A., Murphy, S., Richards, R., and Kelly, F. J.: Modeling the interactions of particulates with epithelial lining fluid antioxidants, Am. J. Physiol-Lung. C, 277, 719–726, 1999.