the Creative Commons Attribution 4.0 License.
the Creative Commons Attribution 4.0 License.
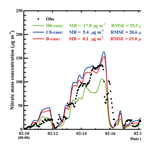
Effects of organic coating on the nitrate formation by suppressing the N2O5 heterogeneous hydrolysis: a case study during wintertime in Beijing–Tianjin–Hebei (BTH)
Lang Liu
Jiarui Wu
Suixin Liu
Xia Li
Jiamao Zhou
Tian Feng
Yang Qian
Junji Cao
Xuexi Tie
Guohui Li
Although stringent emission mitigation strategies have been carried out since 2013 in Beijing–Tianjin–Hebei (BTH), China, heavy haze with high levels of fine particulate matter (PM2.5) still frequently engulfs the region during wintertime and the nitrate contribution to PM2.5 mass has progressively increased. N2O5 heterogeneous hydrolysis is the most important pathway of nitrate formation at nighttime. In the present study, the WRF-Chem model is applied to simulate a heavy haze episode from 10 to 27 February 2014 in BTH to evaluate contributions of N2O5 heterogeneous hydrolysis to nitrate formation and effects of organic coating. The model generally performs reasonably well in simulating meteorological parameters, air pollutants, and aerosol species against observations in BTH. N2O5 heterogeneous hydrolysis with all the secondary organic aerosol assumed to be involved in coating considerably improves the nitrate simulations compared to the measurements in Beijing. On average, organic coating decreases nitrate concentrations by 8.4 % in BTH during an episode, and N2O5 heterogeneous hydrolysis with organic coating contributes about 30.1 % of nitrate concentrations. Additionally, the reaction also plays a considerable role in the heavy haze formation, with a PM2.5 contribution of about 11.6 % in BTH. Sensitivity studies also reveal that future studies need to be conducted to predict the organic aerosol hygroscopicity for accurately representing the organic coating effect on N2O5 heterogeneous hydrolysis.
- Article
(19382 KB) - Full-text XML
- BibTeX
- EndNote
Within recent decades, China has been suffering from pervasive and persistent haze pollution caused by elevated levels of fine particulate matters (PM2.5), particularly in Beijing–Tianjin–Hebei (BTH) (Guo et al., 2014; Gao et al., 2016; Wang et al., 2016). Numerous studies have revealed that the inorganic aerosols, including nitrate, sulfate, and ammonium, are the most abundant component of PM2.5 during haze pollution episodes in BTH, and that the evolution of the haze pollution is characterized by the formation of substantial amounts of sulfate and nitrate (Sun et al., 2013, 2015; Zhang et al., 2013; Zhao et al., 2013). Since 2013, several aggressive emission control strategies have been implemented in China, including desulfurization and dedusting for coal combustion, vehicle restriction and execution of stringent emission standards in key industries (Tao et al., 2017). However, the control of emissions of nitrate gaseous precursors does not seem to be effective since many observations have shown that the nitrate aerosol concentration has progressively increased in recent several years (Zhang et al., 2012, 2015; Sun et al., 2015; Tao et al., 2017).
In the atmosphere, nitrate aerosol is formed via nitrous acid (HNO3) to balance the inorganic cations in the aerosol phase. HNO3 is produced through four pathways (Kim et al., 2014): (1) the reaction of OH and NO2 (main gas-phase pathway and usually considered the daytime pathway because the OH radical is severely limited at night due to lack of O3 and peroxide photolysis), (2) NO3 radical reaction with hydrocarbons, (3) aqueous reaction of NO3 radical to form HNO3, and (4) NO3 conversion to N2O5 with subsequently heterogeneous chemical conversion to form HNO3. The last pathway is referred to as the most important pathway during nighttime since both NO3 and N2O5 are photolytically liable, or even under a heavy haze situation with weak sunlight and high relative humidity (RH) (Brown et al., 2016).
The heterogeneous hydrolysis of N2O5 on the surface of deliquescent aerosols to form HNO3 is quantified by the reaction probability () (Bertram and Thornton, 2009; Chen et al., 2018; Davis et al., 2008; Riemer et al., 2003). has been measured by previous laboratory experiments, dependent on particulate chemical composition, RH, temperature, aerosol surface area, and water content (Chang et al., 2011), and on the order of 10−2 (Zheng et al., 2015). In modeling studies, various parameterizations of are used to simulate the nitrate formation. Dentener and Crutzen (1993) first used 0.1 as the representative in a three-dimensional global model. Riemer et al. (2003) developed a parameterization on the surface of aerosols containing sulfate and nitrate (hereafter referred to as Riemer03), which has widely been used and further improved in air quality models. Davis et al. (2008) implemented a parameterization on the surface of particles containing sulfate, nitrate, and ammonium as a function of RH, temperature, and phase state to improve simulations of N2O5 hydrolysis. Bertram and Thornton (2009) developed a parameterization to consider the influence of chloride salts on as a function of RH.
The coating of particles by organic materials has been reported to inhibit N2O5 uptake (Anttila et al., 2006) and suggested as a possible explanation for field observations of suppressed N2O5 uptake (Brown et al., 2006). Evans and Jacob (2005) have incorporated a parameterization on surfaces of sulfate particles as a function of RH and temperature into the GEOS-CHEM model, including the effects of dust, sea salt, sulfate, elemental carbon, and organic carbon but ignoring nitrogen-containing species. Riemer et al. (2009) developed a N2O5 uptake parameterization (Riemer09) based on the laboratory results of Anttila et al. (2006), which combines nitrogen-containing and organic effects on N2O5 hydrolysis. The parameterization has been used to estimate the maximum effect of organic coating by assuming that all available secondary organic compounds (SOCs) contribute to the coating in a 3-D model. The results show that SOC could suppress N2O5 uptake significantly, reducing particulate nitrate concentrations by up to 90 %. Lowe et al. (2015) further combined the organic coating and chloride salt effects on in the WRF-Chem model. Most recently, Chen et al. (2018) developed a new parameterization with respect to RH, temperature, and aerosol composition, showing that organic coating effect on is not as important as expected over western and central Europe. However, there is still a lack of modeling studies focused on the effect of organic coating on and particulate nitrate formation in China. Wang et al. (2017) evaluated the potential particulate nitrate formation through the N2O5 hydrolysis reaction without considering the organic coating effect during a haze pollution episode in Beijing, and found that the observed nitrate concentration (20.6 µg m−3 on average) is lower than the assessment (57.0 µg m−3 on average). Considering the high organic aerosol concentration and increasing trend of particulate nitrate during haze days in BTH, it is imperative to assess the effect of organic coating on N2O5 hydrolysis and its consequent contribution to nitrate formation.
In the present study, based on Riemer09 parameterization, the contribution of the organic coating effect on N2O5 hydrolysis to nitrate formation is investigated using the WRF-Chem model. The model configuration and methodology are described in Sect. 2. Results and sensitivity studies are presented in Sect. 3. Discussion and summary are given in Sect. 4.
2.1 WRF-Chem model and configuration
A modified version of the WRF-Chem model (Grell et al., 2005) is used in this study, which is developed by Li et al. (2010, 2011a, b, 2012) at the Molina Center for Energy and the Environment. A new flexible gas-phase chemical module has been developed and implemented into the version of the WRF-Chem model, which can be utilized with different chemical mechanisms, including CBIV, RADM2, and SAPRC. The gas-phase chemistry is solved by an Eulerian backward Gauss–Seidel iterative technique with a number of iterations, inherited from NCAR-HANK (Hess et al., 2000). In the study, the SAPRC99 chemical mechanism is used based on the available emission inventory. For the aerosol simulations, the CMAQ/Models-3 aerosol module (AERO5) developed by U.S. EPA has incorporated into the model (Binkowski and Roselle, 2003). Briefly, wet deposition uses the method in the CMAQ module and dry deposition of chemical species is parameterized following Wesely (1989). The photolysis rates are calculated using the Fast Tropospheric Ultraviolet and Visible (FTUV) radiation model (Tie et al., 2003; Li et al., 2005), with the aerosol and cloud effects on the photochemistry (Li et al., 2011a).
ISORROPIA (version 1.7) is used to predict the thermodynamic equilibrium between the ammonia–sulfate–nitrate–chloride–water aerosols and their gas-phase precursors of H2SO4–HNO3–NH3–HCl–water vapor (Nenes et al., 1998). It is worth noting that the most recent extension of ISORROPIA, known as ISORROPIA II, has incorporated a larger number of aerosol species (Ca, Mn, K salts) and is designed to be a superset of ISORROPIA (Fountoukis and Nenes, 2007). Considering that crustal species are not considered in the study, ISORROPIA (version 1.7) is still used to calculate inorganic components and ISORROPIA II is imperative to be incorporated into the WRF-Chem model in future studies. In addition, a parameterization of sulfate heterogeneous formation involving aerosol liquid water (ALW) has been developed and implemented into the model, which has successfully reproduced the observed rapid sulfate formation during haze days (Li et al., 2017). The sulfate heterogeneous formation from SO2 is parameterized as a first-order irreversible uptake by ALW surfaces, with a reactive uptake coefficient of assuming that there is enough alkalinity to maintain the high iron-catalyzed reaction rate.
The organic aerosol (OA) module is based on the volatility basis set (VBS) approach with aging and detailed information can be found in Li et al. (2011b). The POA components from traffic-related combustion and biomass burning are represented by nine surrogate species with saturation concentrations (C∗) ranging from 10−2 to 106 µg m−3 at room temperature (Shrivastava et al., 2008), and assumed to be semi-volatile and photochemically reactive (Robinson et al., 2007). The secondary organic aerosol (SOA) formation from each anthropogenic or biogenic precursor is calculated using four semi-volatile organic compounds (VOCs) with effective saturation concentrations of 1, 10, 100, and 1000 µg m−3 at 298 K. The SOA formation via the heterogeneous reaction of glyoxal and methylglyoxal is parameterized as a first-order irreversible uptake by aerosol particles with an uptake coefficient of (Liggio et al., 2005; Zhao et al., 2006; Volkamer et al., 2007). The OA module has reasonably reproduced the POA and SOA concentration against measurements, and detailed model performance can be found in Li et al. (2011b), Feng et al. (2016), and Xing et al. (2019).
The anthropogenic emission inventory with a horizontal resolution of 6 km is developed by Zhang et al. (2009), with the base year of 2013, including industry, transportation, power plant, residential, and agriculture sources. The Model of Emissions of Gases and Aerosols from Nature (MEGAN) is used to calculate the biogenic emissions online (Guenther et al., 2006).
A heavy haze episode from 10 to 27 February 2014 in BTH is simulated in association with the field observation of air pollutants and secondary inorganic aerosols. Detailed model configuration can be found in Table 1 and the simulation domain is presented in Fig. 1.
2.2 Parameterization of the heterogeneous hydrolysis of N2O5
The reaction of N2O5 heterogeneous hydrolysis on the surface of deliquescent aerosols to form HNO3 can be represented as
This reaction is usually implemented into air quality transport models as a first-order loss:
[N2O5] represents the N2O5 concentration in the atmosphere. The loss rate constant, , is parameterized in the following way:
where is the average molecular velocity of N2O5, and S is the available aerosol surface area density.
In this study, the parameterization of follows Riemer03 and Riemer09. In the parameterization, primary emission compounds such as elemental carbon, insoluble organic matter (mostly part of POA), insoluble inorganic matter, and mineral dust particles are assumed to serve as a nucleus of aerosols. Condensation of soluble chemical components and further water vapor on the surface of the nucleus forms an aqueous layer. The nucleus and the aqueous layer are assumed to be a unified “core” (aqueous core) in the Riemer03 and Riemer09 parameterizations. In the Riemer03 parameterization, soluble inorganic components including sulfate and nitrate are taken into consideration for suppressing the N2O5 heterogeneous hydrolysis uptake in the aqueous core, and the parameterization of is defined as
with γ1=0.02 and γ2=0.002, and f is defined as
and are the aerosol mass concentrations of soluble sulfate and nitrate.
In the Riemer09 parameterization, unreactive organic layers are further considered for the suppression of N2O5 hydrolysis by covering the aqueous core. Organic layers may be formed by secondary organic aerosols, and such layers may consist of a single layer of molecules (monolayered coatings) or of several molecule layers (multilayered coatings) on the surface of the aqueous core. These organic layers are assumed to be an organic “coating” (shell) in the Riemer09 parameterization. The resistor scheme to calculate in the Riemer09 parameterization is parameterized as follows:
where is the reaction probability of the aqueous core, which is calculated using Eq. (4), and is the pseudo-reaction probability of the organic coating calculated by the following formulation:
where R is the universal gas constant, T is temperature, Horg is the Henry's law constant for N2O5 in the organic coating, and Dorg is the diffusion coefficient for N2O5 in the organic coating. Horg and Dorg depend on the physicochemical properties of the compounds comprising the organic coating. In the Riemer09 scheme, Horg⋅Dorg is defined as . Haq is the Henry's law constant of N2O5 for the aqueous phase (Haq=5000 M atm−1) and Daq is the diffusion coefficient of N2O5 in the aqueous phase ( m2 s−1). Rp, Rc, and l are the radius of the particle, radius of the inorganic core, and thickness of the coating, respectively. Rp, Rc, and l are calculated as follows:
where Vinorg and Vorg are the volume of inorganic and organic materials, respectively.
2.3 Statistical methods for model evaluation
In this study, the mean bias (MB), root-mean-square error (RMSE), the index of agreement (IOA), mean fractional bias (MFB), and mean fractional error (MFE) are used to evaluate the model performance in simulating air pollutants.
Here Pi and Oi are the simulated and observed variables, respectively. N is the total number of the simulations for comparisons, and donates the average of the observation. The IOA ranges from 0 to 1, with 1 showing a perfect agreement of the simulation with the observation.
2.4 Air pollutant observations
Simulations are compared to available meteorological and air pollutant observations to evaluate the model performance. The meteorological parameters including temperature, RH, wind speed, and direction are obtained from the website http://www.meteomanz.com (last access: 20 June 2019). The hourly observations of PM2.5, O3, SO2, NO2, and CO concentrations are released by the China National Environmental Monitoring Center and can be downloaded from the website http://106.37.208.233:20035 (last access: 20 June 2019).
Additionally, hourly organic carbon (OC) and elemental carbon (EC) concentrations are measured using a thermal–optical reflectance carbon analyzer (OCEC RT-4, Sunset Lab, USA) at the Chinese Research Academy of Environmental Sciences (CRAES, 40.04∘ N, 116.40∘ E) in Beijing (Wei et al., 2014; Liu et al., 2018). Hourly sulfate, nitrate, ammonium, and other inorganic ions are sampled and analyzed by ion chromatography (URG 9000S, Thermo Fisher Scientific, USA).
The OC ∕ EC ratio approach is used to derive the SOA mass concentrations from EC and OC filter measurements as follows (Strader, 1999; Cao et al., 2004):
where POC and SOC are the primary OC and secondary OC, respectively. In the present study, POC∕EC and SOA∕SOC are assumed to be 2.4 and 1.6, respectively, based on previous studies (Cao et al., 2007; Aiken et al., 2008; Yu et al., 2009), and detailed information about the approach can be found in Feng et al. (2016). It is worth noting that those assumed POC∕EC and SOA∕SOC could potentially affect the model–measurement comparisons.
3.1 Synoptic conditions during the wintertime of 2014
Based on the NCEP FNL reanalysis data (https://rda.ucar.edu/datasets/ds083.2, last access: 20 June 2019), we have initially performed the analysis of synoptic conditions using the wind, temperature, relative humidity, and geopotential height fields at 500 and 850 hPa averaged from 10 to 27 February 2014 over China (Fig. 2). At 500 hPa, flat westerly winds prevail over BTH and its surrounding area, indicating stagnant atmospheric circulation conditions (Fig. 2a). Moreover, the flat isotherm distribution is similar to that of the isobar at 500 hPa, showing that there is no obvious exchange of cold and warm air masses, which together with the flat westerly leads to weak turbulent mixing in the vertical direction and stable weather conditions (Fig. 2b). At 850 hPa, the southeast coastal areas of China are controlled by the anticyclone, whose center is located over the South China Sea (Fig. 2c). In eastern China influenced by the anticyclone, the weak southerly wind prevails over the BTH and its surrounding regions, providing a favorable condition for stagnant weather conditions and the formation of air pollution. With the prevailing southerly wind, the warm and humid air flow and the polluted air mass are subject to being transported from south to north, aggravating the air pollution in BTH. In addition, high-relative-humidity conditions facilitate heterogeneous reactions for secondary aerosol formation (Fig. 2d).
3.2 Model performance
In order to quantify effects of the N2O5 heterogeneous hydrolysis and organic coating on the nitrate formation, three experiments have been performed in the study. In the base case, the Riemer09 parameterization is used to take into consideration the organic coating effect on the N2O5 heterogeneous hydrolysis by assuming that all the SOA is involved in coating (hereafter referred to as the B-case). In the first sensitivity case, the contribution of N2O5 heterogeneous hydrolysis to nitrate formation is not considered (hereafter referred to as the H0-case). In the second sensitivity case, the organic coating effect is not considered in the Riemer09 parameterization (hereafter referred to as the C0-case). The simulation results in the B-case are compared to observations in BTH.
3.2.1 Meteorological parameter simulations in Beijing
Considering that the meteorological conditions play a crucial role in air pollution simulations, which determine accumulation or dispersion of pollutants, verifications are first performed for the simulations of meteorological fields. Figure 3 presents the temporal profile of the simulated and observed temperature, RH, wind speed, and wind direction averaged over 12 meteorological sites in Beijing from 10 to 27 February 2014. The WRF-Chem model reproduces the temporal variation in the surface temperature during the whole episode well. The MB and RMSE are −0.2 and 1.7 ∘ C, and the IOA reaches 0.94, indicating good agreement of the simulations with observations (Table 2). The simulated temporal RH variations are also consistent with observations, with a MB, RMSE, and IOA of 2.6 %, 10.9 %, and 0.89, respectively. In addition, the model tracks the temporal variations in the surface wind reasonably well, with IOAs of 0.73 and 0.66 for the wind speed and direction, respectively.
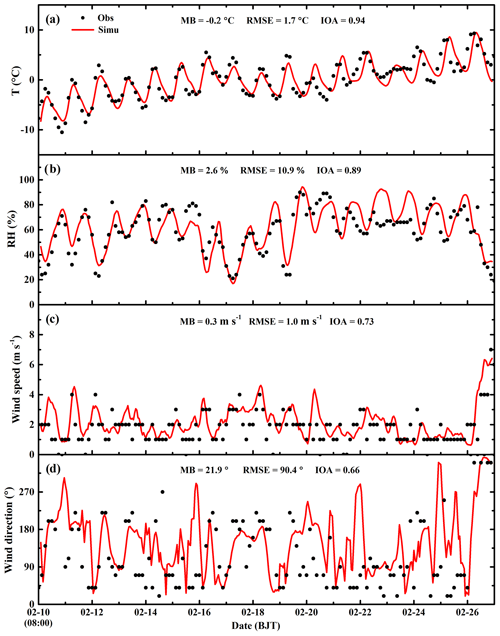
Figure 3Temporal variations in simulated (red line) and observed (black dots) meteorological parameters of near-surface (a) temperature, (b) relative humidity, (c) wind speed, and (d) wind direction averaged at 12 meteorological sites in Beijing from 10 to 27 February 2014.
3.2.2 Air pollutant simulations in BTH
Figure 4 shows the relationship between observed and simulated mass concentrations of PM2.5, O3, SO2, NO2, and CO in Beijing, Tianjin, and Hebei from 10 to 27 February 2014. The correlation coefficient (R) of PM2.5 mass concentrations between observations and simulations in Beijing, Tianjin, and Hebei is 0.83, 0.80, and 0.90, respectively, indicating a good performance of the WRF-Chem model in simulating the PM2.5 concentration in BTH. The correlation of O3 and NO2 mass concentrations between observations and simulations is not as good as that of PM2.5 concentrations in BTH, with an R between 0.6 and 0.8. Apparently, the R of SO2 simulations with observations shows that the WRF-Chem model still has difficulties in simulating SO2 concentrations in BTH well, particularly in Hebei. Except uncertainties from SO2 emissions, such as source intensities and distributions and diurnal profiles, the bias of simulated wind fields also substantially influences the SO2 simulation (Bei et al., 2017). In particular, SO2 is principally emitted by the point source, including the power plants and agglomerated industrial zones, so the SO2 simulations are more sensitive to the wind field simulation uncertainties. In terms of R, the SO2 simulations in Beijing and Tianjin are better than those in Hebei, indicating that the SO2 emissions in Beijing and Tianjin are generally determined by area sources, i.e., the residential living, but the point source dominates the SO2 concentration in Hebei. Considering the long lifetime of CO in the atmosphere, the CO simulation is decided by its emission and the meteorological fields. The R of CO simulations with observations in BTH ranges from around 0.6 to 0.7, showing that the CO emissions used in the study and simulated meteorological fields are generally reasonable.
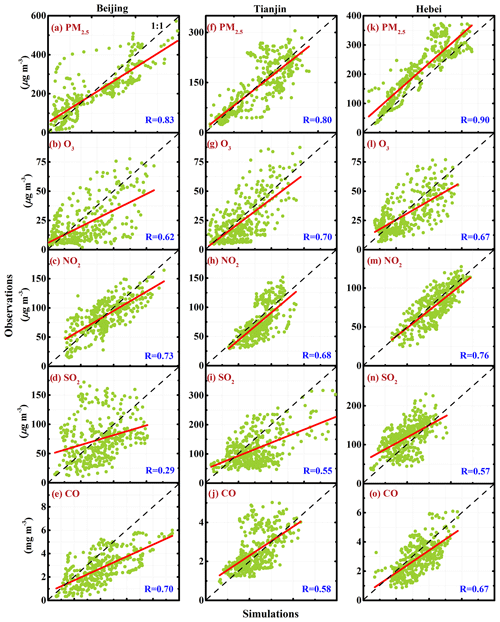
Figure 4Relationships between observed and simulated mass concentrations of PM2.5, O3, NO2, SO2, and CO in Beijing, Tianjin, and Hebei from 10 to 27 February 2014. The red line is the linear regression between observations and simulations, and the black dashed line presents the 1 : 1 line.
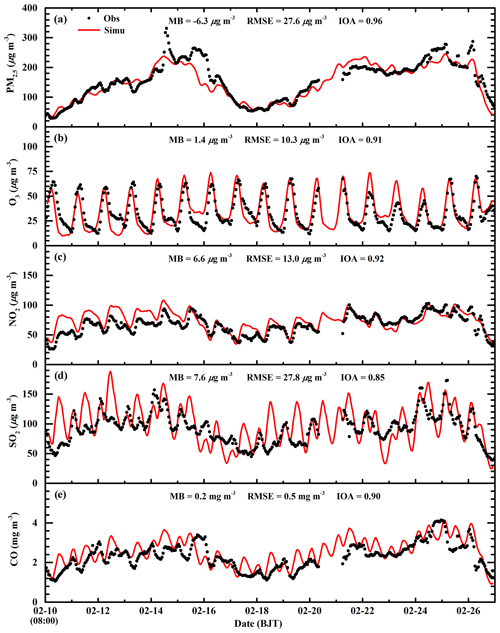
Figure 5Comparison of observed (black dots) and simulated (red line) diurnal profiles of near-surface hourly (a) PM2.5, (b) O3, (c) NO2, (d) SO2, and (e) CO averaged over all ambient monitoring stations in BTH from 10 to 27 February 2014.
Figure 5 presents the diurnal profiles of simulated and observed PM2.5, O3, NO2, SO2, and CO mass concentrations averaged over all ambient monitoring stations in BTH during the simulated episode. The WRF-Chem model reproduces the diurnal variations in the PM2.5 mass concentrations against observations in BTH well. The MB and RMSE are −6.3 and 27.6 µg m−3, respectively, and the IOA is 0.96. The model generally replicates the haze-developing stage well, but fails to capture the observed spikes of PM2.5 mass concentrations, which might be caused by the uncertainty of the simulated meteorological fields or irregular air pollutant emissions (Bei et al., 2017). The simulated O3 diurnal variations are in good agreement with observations, with a MB and IOA of 1.4 µg m−3 and 0.91, respectively. The model tracks the observed diurnal variations in NO2 mass concentrations with an IOA of 0.92 well, but it slightly overestimates NO2 concentrations compared to observations with a MB of 6.6 µg m−3. However, during nighttime, the model overestimation is considerable, which is perhaps due to the model biases in modeling nighttime planetary boundary layer (PBL). Although the model reasonably yields the variation trend of the observed SO2 concentration, with an IOA of 0.85, the dispersion of the simulated SO2 concentration is rather large, with a RMSE of 27.8 µg m−3. In addition, the model overestimates the SO2 concentration compared to observations, and the MB is 7.6 µg m−3, which might be mainly caused by the emission inventory that has undergone noticeable changes since implementation of emission control strategies in 2013 in BTH. The model performs well in simulating CO diurnal variations against observations, with a MB and IOA of 0.2 µg m−3 and 0.90, respectively.
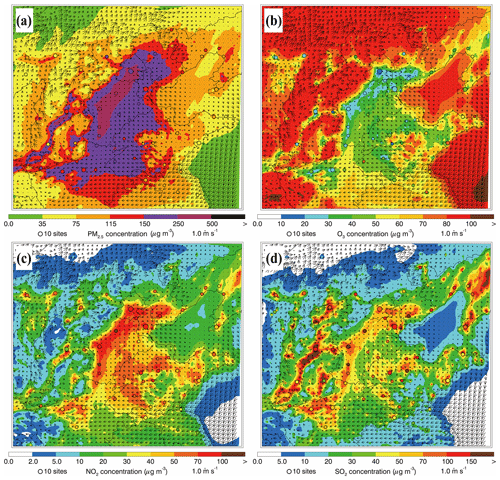
Figure 6Spatial distributions of average (a) PM2.5, (b) O3, (c) NO2, and (d) SO2 mass concentrations from 10 to 27 February 2014. Colored dots, colored contour, and black arrows are observations, simulations, and simulated surface winds, respectively.
Figure 6 presents the distributions of simulated and observed near-surface mass concentrations of PM2.5, O3, NO2, and SO2 along with the predicted wind fields averaged during the episode. Generally, the simulated wind in BTH is weak during the episode and the southerly wind prevails, corresponding to the synoptic situation at 850 and 500 hPa well, which is favorable for the accumulation of air pollutants. The observed PM2.5 concentrations are more than 115 µg m−3 on average, showing that BTH suffers from heavy haze pollution (Fig. 6a). The model generally reproduces the spatial distribution of PM2.5 concentrations against observations well, with the PM2.5 concentration exceeding 150 µg m−3 in the plain area of BTH. The simulated and observed O3 mass concentrations are less than 50 µg m−3 in the plain area of BTH, and in several megacities, including Beijing, Tianjin, Baoding, and Shijiazhuang, the O3 concentrations are less than 30 µg m−3 (Fig. 6b). The low O3 concentrations during the episode are generally caused by the weak insolation during wintertime, which is unfavorable for photochemical reactions, and the titration due to high NOx emissions in BTH (Fig. 6c). The simulated NO2 concentrations are generally more than 40 µg m−3, consistent with the observations at monitoring sites in BTH. The simulated and observed SO2 mass concentrations in cities or their surrounding areas are still rather high, exceeding 50 µg m−3 (Fig. 6d). Elevated SO2 concentrations in BTH during wintertime are to some degree contributed by the residential coal combustion (Li et al., 2018). High levels of NO2 and SO2 show that stringent emission mitigation strategies still need to be implemented in BTH.
3.2.3 Sulfate, ammonium, and SOA simulations in Beijing
The SOA and sulfate concentration directly influences the N2O5 heterogeneous hydrolysis in the Riemer09 parameterization, and the ammonium aerosol concentration substantially affects the nitrate aerosol formation. Therefore, Fig. 7 presents the temporal profiles of observed and calculated SOA, sulfate, and ammonium mass concentrations at the CRAES site in Beijing from 10 to 27 February 2014. The model reasonably tracks the diurnal variation in the SOA concentration compared to observations, with a MB and IOA of −1.2 µg m−3 and 0.83, respectively. The observed SOA concentration exhibits rather large fluctuations, which are not reproduced well by the model. The simulated sulfate trend is generally in agreement with observations with an IOA of 0.88, but there are considerable model biases. During the first pollution event, the model reasonably reproduces the sulfate increase during the haze-developing stage, but the early falloff of sulfate concentrations during the dissipation stage causes a substantial underestimation. However, during the second pollution event, the model considerably overestimates the sulfate concentration against the measurement from 22 to 26 February 2014. The ammonium simulation is slightly better than that of sulfate, with an IOA of 0.90.
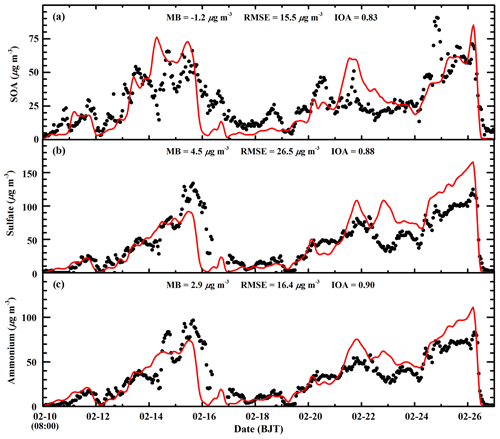
Figure 7Comparison of observed (black dots) and simulated (red line) diurnal profiles of hourly (a) SOA, (b) sulfate, and (c) ammonium concentrations at the CRAES site in Beijing from 10 to 27 February 2014.
Furthermore, the MFB and MFE between simulations and observations are also calculated to evaluate the model performance in simulating meteorological parameters and air pollutants (Table 2). Boylan and Russell (2006) proposed that MFB should be within ±60 % and MFE should be below 75 % for a satisfactory model performance. For the simulation in the B-case, MFB values are within 27 % and MFE values are below 55 %, indicating that the model performance is satisfactory.
In summary, the WRF-Chem model performs reasonably well in simulating air pollutants and aerosol species, showing that the simulated meteorological fields and emissions used in the study are generally reasonable.
3.3 Contributions of the N2O5 heterogeneous hydrolysis and organic coating to the nitrate formation
Figure 8 provides the nitrate temporal variations in the three cases against observations at CRAES site in Beijing from 10 to 27 February 2014. When the N2O5 heterogeneous hydrolysis is not considered in the H0-case, although the model tracks the observed nitrate variations well with an IOA of 0.91, it considerably underestimates nitrate concentration against the measurement, with a MB of −17.0 µg m−3. When the N2O5 heterogeneous hydrolysis is taken into consideration based on the Riemer09 parameterization without organic coating in the C0-case, the nitrate simulation is improved compared to that in the H0-case, with an IOA of 0.95. However, the model begins to overestimate the nitrate concentration compared to the measurement, with a MB of 5.4 µg m−3. In the B-case, when all the SOA is assumed to be involved in coating to suppress the N2O5 heterogeneous uptake on surfaces of deliquescent aerosols, the model performs best in simulating the nitrate variation compared to the measurement, with a MB and IOA of 0.1 µg m−3 and 0.96, respectively. The remarkable consistency of the simulated nitrate in the B-case with the measurement indicates that the organic coating plays an important role in improving the nitrate simulation. It is worth noting that the MB for nitrate aerosols at the CRAES site in the B-case is close to zero, but the RMSE is still rather large, reaching 19.0 µg m−3, showing considerable underestimation and overestimation, caused by uncertainties of meteorological fields and emissions. For example, the model overestimates nitrate concentrations on 11, 13, and 14 February and underestimates on 24 February against measurements. In addition, the early occurrence of intensified winds in the morning on 16 February in simulations causes rapid falloff of nitrate concentrations, leading to substantial model biases.
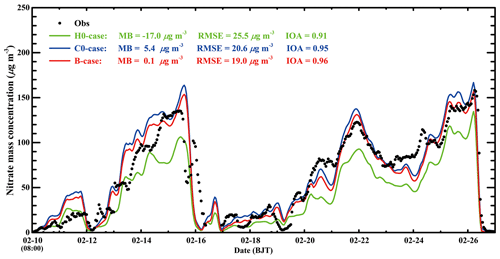
Figure 8Temporal variations in observed (black dot) and simulated (green line: H0-case; blue line: C0-case; red line: B-case) nitrate concentrations at the CRAES site in Beijing from 10 to 27 February 2014.
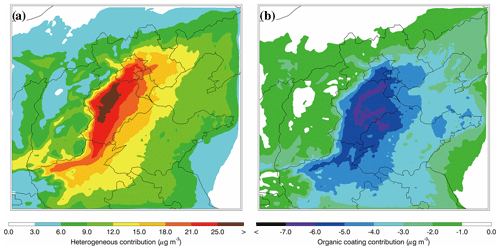
Figure 9Spatial distributions of average nitrate contributions of (a) the N2O5 heterogeneous hydrolysis and (b) organic coating in BTH from 10 to 27 February 2014.
Figure 9a presents the distribution of contributions of the N2O5 heterogeneous hydrolysis to the nitrate formation averaged during the episode by differentiating simulations in the B-case and H0-case. The contribution of the N2O5 heterogeneous hydrolysis to the nitrate formation is substantial in BTH, exceeding 15 µg m−3 in the plain area. Although the O3 concentration is fairly low in BTH during the episode (Fig. 6b), particularly during nighttime (Fig. 5b), the elevated NO2 level still facilitates the N2O5 formation to warrant occurrence of the N2O5 heterogeneous hydrolysis. Previous studies have revealed that N2O5 heterogeneous hydrolysis is vital in nitrate formation. For example, Wang et al. (2017) calculated the daily average nitrate formation potential from the N2O5 heterogeneous hydrolysis in Beijing, showing that the reaction accounts for 52 % of the total nitrate formation. Su et al. (2017) investigated the contribution of N2O5 heterogeneous hydrolysis to the nitrate formation in Beijing during autumn in 2015 and found that the reaction causes a 21.0 % enhancement of nitrate concentrations. In the present study, the nitrate contribution of the N2O5 heterogeneous hydrolysis is 29.4 % in Beijing during the episode on average, which is close to the result in Su et al. (2017) but much lower than that in Wang et al. (2017). The average nitrate contribution of the reaction in BTH is about 30.1 %, showing that the reaction constitutes an important nitrate source during the haze pollution episode. Additionally, the N2O5 heterogeneous hydrolysis contributes 11.6 % of the PM2.5 concentration on average, playing a considerable role in the haze formation in BTH.
However, it is worth noting that the brute force method (BFM) is used to quantify the contribution of the N2O5 heterogeneous hydrolysis to the nitrate formation (Dunker et al., 1996). The BFM is generally used to assess the importance of some source, but it lacks consideration of interactions of the complicated physical and chemical processes in the atmosphere (Zhang and Ying, 2011). Therefore, in the study, the contribution of the N2O5 heterogeneous hydrolysis to the nitrate formation might be underestimated, considering the competition of inorganic cations from HNO3 formed through gas-phase reactions and sulfate aerosols in the atmosphere. It is imperative to use the source-oriented base module to evaluate the nitrate contribution of the reaction.
Figure 9b shows the distribution of the average decrease in nitrate concentrations due to suppression of organic coating during the episode by differentiating simulations in the B-case and C0-case. The organic coating reduces the nitrate concentration by more than 5 µg m−3 in the plain area of BTH, and on average the decrease in nitrate aerosols is 4.7 µg m−3 or 8.4 % in BTH during the episode. Riemer et al. (2009) have shown that when the nitrate levels are high (above 15 µg m−3), the organic coating decreases nitrate concentrations by 10 %–15 % over Europe. However, Chen et al. (2018) have demonstrated that the suppression of organic coating is negligible over western and central Europe, with an influence on nitrate concentrations of less than 2 % on average and 20 % at the most significant moment. Apparently, except for N2O5 and water-soluble OA in the atmosphere, the effect of organic coating is also dependent on NH3, RH, and temperature. Hence, the inconsistency between the model results about the organic coating effect can be attributed to the variation in simulation conditions. For example, in order to obtain substantial effects of the organic coating, N2O5, SOA, and NH3 need to be present when RH is high and temperature is low. However, those conditions are rarely fulfilled simultaneously over western and central Europe, causing a negligible effect of organic coating (Chen et al., 2018). Additionally, Wang et al. (2017) have indicated that the evaluated nitrate level with the N2O5 heterogeneous hydrolysis in Beijing is much higher than the observation, which they have attributed to atmospheric dilution and deposition. It is worth noting that the organic coating effect might constitute one of the most possible reasons for the overestimation of nitrate concentrations, considering the elevated SOA level in Beijing, which suppresses the N2O5 heterogeneous hydrolysis and results in high observed N2O5 concentrations (Wu et al., 2017). Figure 10 presents the temporal variation in the simulated in Beijing during the episode. The simulated fluctuates between 0.009 and 0.02 when organic coating is included, with an average of 0.013. The estimated in Beijing by Wang et al. (2017) ranges from 0.025 to 0.072 without consideration of the suppression of organic coating, indicating that organic coating substantially hinders the N2O5 heterogeneous hydrolysis, likely causing the observed high level of N2O5 during nighttime.
It is worth noting that, in the study, the assumption of metastable aerosols is used or the water-soluble aerosol is assumed to be only in a liquid state in simulations. However, Wang et al. (2008) have highlighted the effect of the hysteresis of particle-phase transitions on the distribution of solid and aqueous aerosols. The aerosol phase is generally regulated by the hysteresis loop. Atmospheric particles containing inorganic salts remain solid until the RH reaches the DRH (deliquescence relative humidity). At the DRH, the solid particle spontaneously absorbs water to become a saturated aqueous solution. However, the liquid particle does not crystallize when the RH is below the DRH (Seinfeld and Pandis, 2006). Therefore, another possible pathway exists to suppress the N2O5 hydrolysis; i.e., the inorganic particles might be in solid phase without organic coating. Further studies need to be conducted to evaluate the hysteresis effect on the N2O5 hydrolysis and organic coating.
3.4 Studies of organic aerosol hygroscopicity sensitivity to nitrate formation
In Sect. 3.3, the WRF-Chem model considerably improves nitrate simulations when considering the N2O5 heterogeneous hydrolysis and organic coating effects. Organic aerosols (OAs) are broadly classified as primary OA (POA) directly emitted and SOA formed in the atmosphere, some of which are water soluble. In order to explore the effects of different OA coatings on the nitrate formation, an additional four sensitivity studies are conducted, in which half of SOA (C1-case), all SOA (C2-case), all SOA and half of POA (C3-case), and all SOA and POA (C4-case) are involved in coating.
Figure 11 shows the Taylor diagram (Taylor, 2001) to present the variance, bias, and correlation of the observed and simulated nitrate concentrations in the four sensitivity cases at the CRAES site during the episode. In the C1-case, when half of SOA is considered to be involved in coating, the simulated nitrate concentration is the most consistent with the observation, with a correlation coefficient of 0.96. In the C2-case with all SOA assumed to be engaged in coating, the correlation coefficient decreases to 0.95. The normalized standardized deviation (NSD) is 1.02 for the C1-case and C2-case, showing the model overestimation in these two cases. With half of POA involved in coating, the NSD is very close to 1.0 (0.99), indicating the simulated nitrate concentration in the C3-case is almost the same as the observation on average, but the correlation coefficient of 0.94 is less than those in the C1-case and C2-case. When all of OA is assumed as the coating, the bias between simulated and observed nitrate concentrations is the largest, and the effect of POA on suppressing the N2O5 heterogeneous hydrolysis might be overestimated in the C4-case.
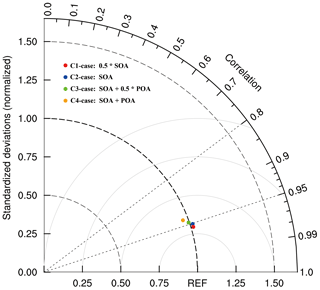
Figure 11Taylor diagram (Taylor, 2001) to present the variance, bias, and correlation of the observed and simulated nitrate concentrations at the CRAES site in Beijing from 10 to 27 February 2014.
Sensitivity results show that the effects of different organic compounds on suppressing the N2O5 heterogeneous hydrolysis to form nitrate vary, depending on the content of water-soluble OA. Laboratory and field measurements have revealed that OA becomes progressively oxidized and more hygroscopic during the aging process in the atmosphere (Jimenez et al., 2009). The OA hygroscopicity constitutes a necessary prerequisite for accurately representing the organic coating effect on the N2O5 heterogeneous hydrolysis. According to the simulations in the present study, in BTH, not all SOA can serve as the coating to suppress the nitrate formation, and the effect of POA on coating might be neglected. Xing et al. (2019) have shown that in BTH, the heterogeneous SOA formed by irreversible uptake of glyoxal and methylglyoxal on wet aerosol surfaces contributes about 30 % of the SOA mass during haze days. Considering the possible heterogeneous SOA contribution of other carbonyl compounds and the atmospheric aging of OA, about half of SOA should likely be hygroscopic and involved in coating.
Nitrate aerosol has constituted a main component of PM2.5 with implementation of aggressive emission control strategies since 2013 in BTH. In the study, the Riemer09 parameterization is implemented into the WRF-Chem model to simulate the nitrate formation from the N2O5 heterogeneous hydrolysis referred to as the most important pathway of the nitrate formation at nighttime. A heavy haze episode from 10 to 27 February 2014 in BTH is simulated using the WRF-Chem model to verify the effect of organic coating on the N2O5 heterogeneous hydrolysis and its consequent contribution to nitrate formation. Analyses of synoptic fields show a stagnant weather condition with the prevailing southerly wind in the low-level atmosphere in BTH and surrounding areas during the episode, facilitating accumulation of air pollutants and heavy haze formation.
The WRF-Chem model performs reasonably in predicting the temporal variations in the meteorological parameters compared to observations in Beijing. The model generally reproduces the temporal variations in and spatial distributions of air pollutants well against observations at monitoring sites in BTH. In addition, the simulated diurnal profiles of sulfate, ammonium, and SOA are also in good agreement with the measurements at the CRAES site in Beijing.
The Riemer09 parameterization with all the SOA assumed to be involved in coating considerably improves the nitrate simulations compared to the measurements at the CRAES site in Beijing. When organic coating is not considered in the Riemer09 parameterization, the model overestimates the nitrate concentration against the measurements. On average, organic coating decreases nitrate concentrations by 4.7 µg m−3 or 8.4 % in BTH during the episode. Furthermore, the N2O5 heterogeneous hydrolysis with organic coating contributes about 30.1 % of nitrate concentrations and 11.6 % of the PM2.5 concentration in BTH, playing a considerable role in the haze formation.
Sensitivity studies reveal that the OA hygroscopicity is a necessary prerequisite for accurately evaluating the organic coating effect on the N2O5 heterogeneous hydrolysis. In the present study, POA might not serve as coating and about half of SOA should be involved in coating to suppress the nitrate formation. Future studies still need to be conducted to further predict the OA hygroscopicity, in order to more precisely represent the organic coating effect on the N2O5 heterogeneous hydrolysis in chemical transport models.
The real-time PM2.5, O3, NO2, SO2, and CO are accessible for the public on the website http://106.37.208.233:20035 (China MEP, 2013a). One can also access the historic profile of observed ambient pollutants through visiting http://www.aqistudy.cn (China MEP, 2013b).
GL, as the contact author, provided the ideas and financial support, verified the conclusions, and revised the paper. LL conducted research, designed the experiments, carried the methodology out, performed the simulation, processed the data, prepared the data visualization, and prepared the paper with contributions from all authors. JW and XL provided the treatment of meteorological data, analyzed the study data, validated the model performance, and reviewed the paper. SL, YQ, TF, and JZ provided the observation data used in the study, synthesized the observation, and reviewed the paper. XT and JC provided critical reviews in the pre-publication stage.
The authors declare that they have no conflict of interest.
This article is part of the special issue “Regional transport and transformation of air pollution in eastern China”. It is not associated with a conference.
This work is financially supported by the National Key R&D Plan (Quantitative Relationship and Regulation Principle between Regional Oxidation Capacity of Atmospheric and Air Quality (2017YFC0210000)) and National Research Program for Key Issues in Air Pollution Control (DQGG0105).
This research has been supported by the National Key R&D Plan (grant no. 2017YFC0210000) and the National Research Program for Key Issues in Air Pollution Control (grant no. DQGG0105).
This paper was edited by Luisa Molina and reviewed by two anonymous referees.
Aiken, A. C., DeCarlo, P. F., Kroll, J. H., Worsnop, D. R., Huffman, J. A., Docherty, K. S., Ulbrich, I. M., Mohr, C., Kimmel, J. R., Sueper, D., Sun, Y., Zhang, Q., Trimborn, A., Northway, M., Ziemann, P. J., Canagaratna, M. R., Onasch, T. B., Alfarra, M. R., Prévôt, A. S. H., Dommen, J., Duplissy, J., Metzger, A., Baltensperger, U., and Jimenez, J. L.: O / C and OM / OC Ratios of Primary, Secondary, and Ambient Organic Aerosols with High-Resolution Time-of-Flight Aerosol Mass Spectrometry, Environ. Sci. Techn., 42, 4478–4485, https://doi.org/10.1021/es703009q, 2008.
Anttila, T., Kiendlerscharr, A., Tillmann, R., and Mentel, T. F.: On the reactive uptake of gaseous compounds by organic-coated aqueous aerosols: theoretical analysis and application to the heterogeneous hydrolysis of N2O5, J. Phys. Chem. A, 110, 10435–10443, https://doi.org/10.1021/jp062403c, 2006.
Bei, N., Wu, J., Elser, M., Feng, T., Cao, J., El-Haddad, I., Li, X., Huang, R., Li, Z., Long, X., Xing, L., Zhao, S., Tie, X., Prévôt, A. S. H., and Li, G.: Impacts of meteorological uncertainties on the haze formation in Beijing–Tianjin–Hebei (BTH) during wintertime: a case study, Atmos. Chem. Phys., 17, 14579–14591, https://doi.org/10.5194/acp-17-14579-2017, 2017.
Bertram, T. H. and Thornton, J. A.: Toward a general parameterization of N2O5 reactivity on aqueous particles: the competing effects of particle liquid water, nitrate and chloride, Atmos. Chem. Phys., 9, 8351–8363, https://doi.org/10.5194/acp-9-8351-2009, 2009.
Binkowski, F. S. and Roselle, S. J.: Models-3 Community Multiscale Air Quality (CMAQ) model aerosol component 1. Model description, J. Geophys. Res.-Atmos., 108, 335–346, https://doi.org/10.1029/2001JD001409, 2003.
Boylan, J. W. and Russell, A. G.: PM and light extinction model performance metrics, goals, and criteria for three-dimensional air quality models, Atmos. Environ., 40, 4946–4959, https://doi.org/10.1016/j.atmosenv.2005.09.087, 2006.
Brown, S. S., Ryerson, T. B., Wollny, A. G., Brock, C. A., Peltier, R., Sullivan, A. P., Weber, R. J., Dubé, W. P., Trainer, M., and Meagher, J. F.: Variability in Nocturnal Nitrogen Oxide Processing and Its Role in Regional Air Quality, Science, 311, 67–70, https://doi.org/10.1126/science.1120120, 2006.
Brown, S. S., Dubé, W. P., Tham, Y. J., Zha, Q., Xue, L., Poon, S., Wang, Z., Blake, D. R., Tsui, W., Parrish, D. D., and Wang, T.: Nighttime chemistry at a high altitude site above Hong Kong, J. Geophys. Res.-Atmos., 121, 2457–2475, https://doi.org/10.1002/2015JD024566, 2016.
Cao, J. J., Lee, S. C., Ho, K. F., Zou, S. C., Fung, K., Li, Y., Watson, J. G., and Chow, J. C.: Spatial and seasonal variations of atmospheric organic carbon and elemental carbon in Pearl River Delta Region, China, Atmos. Environ., 38, 4447–4456, https://doi.org/10.1016/j.atmosenv.2004.05.016, 2004.
Cao, J. J., Lee, S. C., Chow, J. C., Watson, J. G., Ho, K. F., Zhang, R. J., Jin, Z. D., Shen, Z. X., Chen, G. C., Kang, Y. M., Zou, S. C., Zhang, L. Z., Qi, S. H., Dai, M. H., Cheng, Y., and Hu, K.: Spatial and seasonal distributions of carbonaceous aerosols over China, J. Geophys. Res.-Atmos., 112, D22S11, https://doi.org/10.1029/2006JD008205, 2007.
Chang, W. L., Bhave, P. V., Brown, S. S., Riemer, N., Stutz, J., and Dabdub, D.: Heterogeneous Atmospheric Chemistry, Ambient Measurements, and Model Calculations of N2O5: A Review, Aerosol Sci. Technol., 45, 665–695, https://doi.org/10.1080/02786826.2010.551672, 2011.
Chen, F. and Dudhia, J.: Coupling an advanced land surface-hydrology model with the Penn State-NCAR MM5 modeling system. Part I: Model implementation and sensitivity, Mon. Weather Rev., 129, 569–585, https://doi.org/10.1175/1520-0493(2001)129<0569:CAALSH>2.0.CO;2, 2001.
Chen, Y., Wolke, R., Ran, L., Birmili, W., Spindler, G., Schröder, W., Su, H., Cheng, Y., Tegen, I., and Wiedensohler, A.: A parameterization of the heterogeneous hydrolysis of N2O5 for mass-based aerosol models: improvement of particulate nitrate prediction, Atmos. Chem. Phys., 18, 673–689, https://doi.org/10.5194/acp-18-673-2018, 2018.
Ministry of Environmental Protection, China (China MEP): Air Quality Observation Real-time Release Platform of MEP Data Center, available at: http://106.37.208.233:20035 (last access: 20 June 2019), 2013a.
Ministry of Environmental Protection, China (China MEP): Online Monitoring and Analysis Platform of China Air Quality, available at: http://www.aqistudy.cn (last access: 20 June 2019), 2013b.
Chou, M. D. and Suarez, M. J.: A solar radiation parameterization for atmospheric studies, edited by: Suarez, M. J., No. NASA/TM-1999-10460, NASA Technique Report, 1999.
Chou, M. D., Suarez, M. J., Liang, X. Z., Yan, M. H., and Cote, C.: A Thermal Infrared Radiation Parameterization for Atmospheric Studies, edited by: Suarez, M. J., No. NASA/TM-2001-104606, NASA Technique Report, 2001.
Davis, J. M., Bhave, P. V., and Foley, K. M.: Parameterization of N2O5 reaction probabilities on the surface of particles containing ammonium, sulfate, and nitrate, Atmos. Chem. Phys., 8, 5295–5311, https://doi.org/10.5194/acp-8-5295-2008, 2008.
Dentener, F. J. and Crutzen, P. J.: Reaction of N2O5 on tropospheric aerosols: Impact on the global distributions of NOx, O3, and OH, J. Geophys. Res.-Atmos., 98, 7149–7163, https://doi.org/10.1029/92JD02979, 1993.
Dunker, A. M., Morris, R. E., Pollack, A. K., Schleyer, C. H., and Yarwood, G: Photochemical modeling of the impact of fuels and vehicles on urban ozone using auto oil program data, Environ. Sci. Technol., 30, 787–801, https://doi.org/10.1021/es950175m, 1996.
Evans, M. J. and Jacob, D. J.: Impact of new laboratory studies of N2O5 hydrolysis on global model budgets of tropospheric nitrogen oxides, ozone, and OH, Geophys. Res. Lett., 32, 297–314, https://doi.org/10.1029/2005GL022469, 2005.
Feng, T., Li, G., Cao, J., Bei, N., Shen, Z., Zhou, W., Liu, S., Zhang, T., Wang, Y., Huang, R.-J., Tie, X., and Molina, L. T.: Simulations of organic aerosol concentrations during springtime in the Guanzhong Basin, China, Atmos. Chem. Phys., 16, 10045–10061, https://doi.org/10.5194/acp-16-10045-2016, 2016.
Fountoukis, C. and Nenes, A.: ISORROPIA II: a computationally efficient thermodynamic equilibrium model for aerosols, Atmos. Chem. Phys., 7, 4639–4659, https://doi.org/10.5194/acp-7-4639-2007, 2007.
Gao, M., Carmichael, G. R., Wang, Y., Saide, P. E., Yu, M., Xin, J., Liu, Z., and Wang, Z.: Modeling study of the 2010 regional haze event in the North China Plain, Atmos. Chem. Phys., 16, 1673–1691, https://doi.org/10.5194/acp-16-1673-2016, 2016.
Grell, G. A., Peckham, S. E., Schmitz, R., McKeen, S. A., Frost, G., Skamarock, W. C., and Eder, B.: Fully coupled “online” chemistry within the WRF model, Atmos. Environ., 39, 6957–6975, 2005.
Guenther, A., Karl, T., Harley, P., Wiedinmyer, C., Palmer, P. I., and Geron, C.: Estimates of global terrestrial isoprene emissions using MEGAN (Model of Emissions of Gases and Aerosols from Nature), Atmos. Chem. Phys., 6, 3181–3210, https://doi.org/10.5194/acp-6-3181-2006, 2006.
Guo, S., Hu, M., Zamora, M. L., Peng, J., Shang, D., Zheng, J., Du, Z., Wu, Z., Shao, M., and Zeng, L.: Elucidating severe urban haze formation in China, P. Natl. Acad. Sci. USA, 111, 17373–17378, https://doi.org/10.1073/pnas.1419604111, 2014.
Hess, P. G., Flocke, S., Lamarque, J. F., Barth, M. C., and Madronich, S.: Episodic modeling of the chemical structure of the troposphere as revealed during the spring MLOPEX 2 intensive, J. Geophys. Res.-Atmos., 105, 809–839, 2000.
Hong, S. Y. and Lim, J. O. J.: The WRF Single-Moment 6-Class Microphysics Scheme (WSM6), Asia-Pacif. J. Atmos. Sci., 42, 129–151, 2006.
Horowitz, L. W., Walters, S., Mauzerall, D. L., Emmons, L. K., Rasch, P. J., Granier, C., Tie, X., Lamarque, J. F., Schultz, M. G., Tyndall, G. S., Orlando, J. J., and Brasseur, G. P.: A global simulation of tropospheric ozone and related tracers: Description and evaluation of MOZART, version 2, J. Geophys. Res.-Atmos., 108, 4784, https://doi.org/10.1029/2002JD002853, 2003.
Janjić, Z. I.: Nonsingular Implementation of the Mellor-Yamada Level 2.5 Scheme in the NCEP Meso Model, NCEP Office Note 437, 2002.
Jimenez, J. L., Canagaratna, M. R., Donahue, N. M., Prevot, A. S. H., Zhang, Q., Kroll, J. H., DeCarlo, P. F., Allan, J. D., Coe, H., Ng, N. L., Aiken, A. C., Docherty, K. S., Ulbrich, I. M., Grieshop, A. P., Robinson, A. L., Duplissy, J., Smith, J. D., Wilson, K. R., Lanz, V. A., Hueglin, C., Sun, Y. L., Tian, J., Laaksonen, A., Raatikainen, T., Rautiainen, J., Vaattovaara, P., Ehn, M., Kulmala, M., Tomlinson, J. M., Collins, D. R., Cubison, M. J., Dunlea, E. J., Huffman, J. A., Onasch, T. B., Alfarra, M. R., Williams, P. I., Bower, K., Kondo, Y., Schneider, J., Drewnick, F., Borrnann, S., Weimer, S., Demerjian, K., Salcedo, D., Cottrell, L., Griffin, R., Takami, A., Miyoshi, T., Hatakeyama, S., Shimono, A., Sun, J. Y., Zhang, M. Y., Dzepina, K., Kimmel, J. R., Sueper, D., Jayne, J. T., Herndon, S. C., Trimborn, A. M., Williams, L. R., Wood, E. C., Middlebrook, A. M., Kolb, C. E., Baltensperger, U., and Worsnop, D. R.: Evolution of organic aerosols in the atmosphere, Science, 326, 1525–1529, https://doi.org/10.1126/science.1180353, 2009.
Kim, Y. J., Spak, S. N., Carmichael, G. R., Riemer, N., and Stanier, C. O.: Modeled aerosol nitrate formation pathways during wintertime in the Great Lakes region of North America, J. Geophys. Res.-Atmos., 119, 12420–12445, https://doi.org/10.1002/2014JD022320, 2014.
Li, G., Zhang, R., Fan, J., and Tie, X.: Impacts of black carbon aerosol on photolysis and ozone, J. Geophys. Res.-Atmos., 110, D23206, https://doi.org/10.1029/2005JD005898, 2005.
Li, G., Lei, W., Zavala, M., Volkamer, R., Dusanter, S., Stevens, P., and Molina, L. T.: Impacts of HONO sources on the photochemistry in Mexico City during the MCMA-2006/MILAGO Campaign, Atmos. Chem. Phys., 10, 6551–6567, https://doi.org/10.5194/acp-10-6551-2010, 2010.
Li, G., Bei, N., Tie, X., and Molina, L. T.: Aerosol effects on the photochemistry in Mexico City during MCMA-2006/MILAGRO campaign, Atmos. Chem. Phys., 11, 5169–5182, https://doi.org/10.5194/acp-11-5169-2011, 2011a.
Li, G., Zavala, M., Lei, W., Tsimpidi, A. P., Karydis, V. A., Pandis, S. N., Canagaratna, M. R., and Molina, L. T.: Simulations of organic aerosol concentrations in Mexico City using the WRF-CHEM model during the MCMA-2006/MILAGRO campaign, Atmos. Chem. Phys., 11, 3789–3809, https://doi.org/10.5194/acp-11-3789-2011, 2011b.
Li, G., Lei, W., Bei, N., and Molina, L. T.: Contribution of garbage burning to chloride and PM2.5 in Mexico City, Atmos. Chem. Phys., 12, 8751–8761, https://doi.org/10.5194/acp-12-8751-2012, 2012.
Li, G., Bei, N., Cao, J., Huang, R., Wu, J., Feng, T., Wang, Y., Liu, S., Zhang, Q., Tie, X., and Molina, L. T.: A possible pathway for rapid growth of sulfate during haze days in China, Atmos. Chem. Phys., 17, 3301–3316, https://doi.org/10.5194/acp-17-3301-2017, 2017.
Li, X., Wu, J., Elser, M., Feng, T., Cao, J., El-Haddad, I., Huang, R., Tie, X., Prévôt, A. S. H., and Li, G.: Contributions of residential coal combustion to the air quality in Beijing–Tianjin–Hebei (BTH), China: a case study, Atmos. Chem. Phys., 18, 10675–10691, https://doi.org/10.5194/acp-18-10675-2018, 2018.
Liggio, J., Li, S. M., and McLaren, R.: Reactive uptake of glyoxal by particulate matter, J. Geophys. Res.-Atmos., 110, D10304, https://doi.org/10.1029/2004jd005113, 2005.
Liu, W., Shen, G., Chen, Y., Shen, H., Huang, Y., Li, T., Wang, Y., Fu, X., Tao, S., Liu, W., Huang-Fu, Y., Zhang, W., Xue, C., Liu, G., Wu, F., and Wong, M.: Air pollution and inhalation exposure to particulate matter of different sizes in rural households using improved stoves in central China, J. Environ. Sci.-China, 63, 87–95, https://doi.org/10.1016/j.jes.2017.06.019, 2018.
Lowe, D., Archer-Nicholls, S., Morgan, W., Allan, J., Utembe, S., Ouyang, B., Aruffo, E., Le Breton, M., Zaveri, R. A., Di Carlo, P., Percival, C., Coe, H., Jones, R., and McFiggans, G.: WRF-Chem model predictions of the regional impacts of N2O5 heterogeneous processes on night-time chemistry over north-western Europe, Atmos. Chem. Phys., 15, 1385–1409, https://doi.org/10.5194/acp-15-1385-2015, 2015.
Nenes, A., Pandis, S. N., and Pilinis, C.: ISORROPIA: A new thermodynamic equilibrium model for multiphase multicomponent inorganic aerosols, Aquat. Geochem., 4, 123–152, https://doi.org/10.1023/a:1009604003981, 1998.
Riemer, N., Vogel, H., Vogel, B., Schell, B., Ackermann, I., Kessler, C., and Hass, H.: Impact of the heterogeneous hydrolysis of N2O5 on chemistry and nitrate aerosol formation in the lower troposphere under photosmog conditions, J. Geophys. Res.-Atmos., D4, 4144, https://doi.org/10.1029/2002JD002436, 2003.
Riemer, N., Vogel, H., Vogel, B., Anttila, T., Kiendler-Scharr, A., and Mentel, T.F.: Relative importance of organic coatings for the heterogeneous hydrolysis of N2O5 during summer in Europe, J. Geophys. Res.-Atmos., 114, D17307, https://doi.org/10.1029/2008JD011369, 2009.
Robinson, A. L., Donahue, N. M., Shrivastava, M. K.,Weitkamp, E. A., Sage, A. M., Grieshop, A. P., Lane, T. E., Pandis, S. N., and Pierce, J. R.: Rethinking organic aerosols: semivolatile emissions and photochemical aging, Science, 315, 1259–1262, 2007.
Seinfeld, J. H. and Pandis, S. N.: Atmospheric Chemistry and Physics: From Air Pollution to Climate Change, 2nd Edn., John Wiley & Sons Inc., New York, USA, 2006.
Shrivastava, M. K., Lane, T. E., Donahue, N. M., Pandis, S. N., and Robinson, A. L.: Effects of gas particle partitioning and aging of primary emissions on urban and regional organic aerosol concentrations, J. Geophys. Res.-Atmos., 113, D18301, https://doi.org/10.1029/2007jd009735, 2008.
Strader, R.: Evaluation of secondary organic aerosol formation in winter, Atmos. Environ., 33, 4849–4863, https://doi.org/10.1016/s1352-2310(99)00310-6, 1999.
Su, X., Tie, X., Li, G., Cao, J., Huang, R., Feng, T., Long, X., and Xu, R.: Effect of hydrolysis of N2O5 on nitrate and ammonium formation in Beijing China: WRF-Chem model simulation, Sci. Total Environ., 579, 221–229, 2017.
Sun, Y. L., Wang, Z. F., Fu, P. Q., Yang, T., Jiang, Q., Dong, H. B., Li, J., and Jia, J. J.: Aerosol composition, sources and processes during wintertime in Beijing, China, Atmos. Chem. Phys., 13, 4577–4592, https://doi.org/10.5194/acp-13-4577-2013, 2013.
Sun, Y. L., Wang, Z. F., Du, W., Zhang, Q., Wang, Q. Q., Fu, P. Q., Pan, X. L., Li, J., Jayne, J., and Worsnop, D. R.: Long-term real-time measurements of aerosol particle composition in Beijing, China: seasonal variations, meteorological effects, and source analysis, Atmos. Chem. Phys., 15, 10149–10165, https://doi.org/10.5194/acp-15-10149-2015, 2015.
Tao, J., Zhang, L., Cao, J., and Zhang, R.: A review of current knowledge concerning PM2.5 chemical composition, aerosol optical properties and their relationships across China, Atmos. Chem. Phys., 17, 9485–9518, https://doi.org/10.5194/acp-17-9485-2017, 2017.
Taylor, K. E.: Summarizing multiple aspects of model performance in a single diagram, J. Geophys. Res.-Atmos., 106, 7183–7192, https://doi.org/10.1029/2000JD900719, 2001.
Tie, X., Madronich, S., Walters, S., Zhang, R., Rasch, P., and Collins, W.: Effect of clouds on photolysis and oxidants in the troposphere, J. Geophys. Res.-Atmos., 108, 4642, https://doi.org/10.1029/2003JD003659, 2003.
Volkamer, R., Martini, F. S., Molina, L. T., Salcedo, D., Jimenez, J. L., and Molina, M. J.: A missing sink for gas-phase glyoxal in Mexico City: Formation of secondary organic aerosol, Geophys. Res. Lett., 34, L19807, https://doi.org/10.1029/2007gl030752, 2007.
Wang, G., Zhang, R., Gomez, M. E., Yang, L., Levy Zamora, M., Hu, M., Lin, Y., Peng, J., Guo, S., Meng, J., Li, J., Cheng, C., Hu, T., Ren, Y., Wang, Y., Gao, J., Cao, J., An, Z., Zhou, W., Li, G., Wang, J., Tian, P., Marrero-Ortiz, W., Secrest, J., Du, Z., Zheng, J., Shang, D., Zeng, L., Shao, M., Wang, W., Huang, Y., Wang, Y., Zhu, Y., Li, Y., Hu, J., Pan, B., Cai, L., Cheng, Y., Ji, Y., Zhang, F., Rosenfeld, D., Liss, P. S., Duce, R. A., Kolb, C. E., and Molina, M. J.: Persistent sulfate formation from London Fog to Chinese haze, P. Natl. Acad. Sci. USA, 113, 13630–13635, https://doi.org/10.1073/pnas.1616540113, 2016.
Wang, H., Lu, K., Chen, X., Zhu, Q., Chen, Q., Guo, S., Jiang, M., Li, X., Shang, D., Tan, Z., Wu, Y., Wu, Z., Zou, Q., Zheng, Y., Zeng, L., Zhu, T., Hu, M., and Zhang, Y.: High N2O5 concentrations observed in urban Beijing: Implications of a large nitrate formation Pathway, Environ. Sci. Tech. Lett., 4, 416–420, https://doi.org/10.1021/acs.estlett.7b00341, 2017.
Wang, J., Hoffmann, A. A., Park, R. J., Jacob, D. J., and Martin, S. T.: Global distribution of solid and aqueous sulfate aerosols: Effect of the hysteresis of particle phase transitions, J. Geophys. Res.-Atmos., 113, D11206, https://doi.org/10.1029/2007JD009367, 2008.
Wei, S., Shen, G., Zhang, Y., Xue, M., Xie, H., Lin, P., Chen, Y., Wang, X. and Tao, S.: Field measurement on the emissions of PM, OC, EC and PAHs from indoor crop straw burning in rural China, Environ. Pollut., 184, 18–24, https://doi.org/10.1016/j.envpol.2013.07.036, 2014.
Wesely, M. L.: Parameterization of surface resistances to gaseous dry deposition in regional-scale numerical models, Atmos. Environ., 23, 1293–1304, https://doi.org/10.1016/0004-6981(89)90153-4, 1989.
Wu, J., Li, G., Cao, J., Bei, N., Wang, Y., Feng, T., Huang, R., Liu, S., Zhang, Q., and Tie, X.: Contributions of trans-boundary transport to summertime air quality in Beijing, China, Atmos. Chem. Phys., 17, 2035–2051, https://doi.org/10.5194/acp-17-2035-2017, 2017.
Xing, L., Wu, J., Elser, M., Tong, S., Liu, S., Li, X., Liu, L., Cao, J., Zhou, J., El-Haddad, I., Huang, R., Ge, M., Tie, X., Prévôt, A. S. H., and Li, G.: Wintertime secondary organic aerosol formation in Beijing–Tianjin–Hebei (BTH): contributions of HONO sources and heterogeneous reactions, Atmos. Chem. Phys., 19, 2343–2359, https://doi.org/10.5194/acp-19-2343-2019, 2019.
Yu, X.-Y., Cary, R. A., and Laulainen, N. S.: Primary and secondary organic carbon downwind of Mexico City, Atmos. Chem. Phys., 9, 6793–6814, https://doi.org/10.5194/acp-9-6793-2009, 2009.
Zhang, H. and Ying, Q.: Secondary organic aerosol formation and source apportionment in Southeast Texas, Atmos. Environ., 45, 3217–3227, https://doi.org/10.1016/j.atmosenv.2011.03.046, 2011.
Zhang, Q., Streets, D. G., Carmichael, G. R., He, K. B., Huo, H., Kannari, A., Klimont, Z., Park, I. S., Reddy, S., Fu, J. S., Chen, D., Duan, L., Lei, Y., Wang, L. T., and Yao, Z. L.: Asian emissions in 2006 for the NASA INTEX-B mission, Atmos. Chem. Phys., 9, 5131–5153, https://doi.org/10.5194/acp-9-5131-2009, 2009.
Zhang, R., Jing, J., Tao, J., Hsu, S.-C., Wang, G., Cao, J., Lee, C. S. L., Zhu, L., Chen, Z., Zhao, Y., and Shen, Z.: Chemical characterization and source apportionment of PM2.5 in Beijing: seasonal perspective, Atmos. Chem. Phys., 13, 7053–7074, https://doi.org/10.5194/acp-13-7053-2013, 2013.
Zhang, X. Y., Wang, Y. Q., Niu, T., Zhang, X. C., Gong, S. L., Zhang, Y. M., and Sun, J. Y.: Atmospheric aerosol compositions in China: spatial/temporal variability, chemical signature, regional haze distribution and comparisons with global aerosols, Atmos. Chem. Phys., 12, 779–799, https://doi.org/10.5194/acp-12-779-2012, 2012.
Zhang, X. Y., Wang, J. Z., Wang, Y. Q., Liu, H. L., Sun, J. Y., and Zhang, Y. M.: Changes in chemical components of aerosol particles in different haze regions in China from 2006 to 2013 and contribution of meteorological factors, Atmos. Chem. Phys., 15, 12935–12952, https://doi.org/10.5194/acp-15-12935-2015, 2015.
Zhao, J., Levitt, N. P., Zhang, R., and Chen, J.: Heterogeneous reactions of methylglyoxal in acidic media: Implications for secondary organic aerosol formation, Environ. Sci. Technol., 40, 7682–7687, https://doi.org/10.1021/es060610k, 2006.
Zhao, P. S., Dong, F., He, D., Zhao, X. J., Zhang, X. L., Zhang, W. Z., Yao, Q., and Liu, H. Y.: Characteristics of concentrations and chemical compositions for PM2.5 in the region of Beijing, Tianjin, and Hebei, China, Atmos. Chem. Phys., 13, 4631–4644, https://doi.org/10.5194/acp-13-4631-2013, 2013.
Zheng, B., Zhang, Q., Zhang, Y., He, K. B., Wang, K., Zheng, G. J., Duan, F. K., Ma, Y. L., and Kimoto, T.: Heterogeneous chemistry: a mechanism missing in current models to explain secondary inorganic aerosol formation during the January 2013 haze episode in North China, Atmos. Chem. Phys., 15, 2031–2049, https://doi.org/10.5194/acp-15-2031-2015, 2015.