the Creative Commons Attribution 4.0 License.
the Creative Commons Attribution 4.0 License.
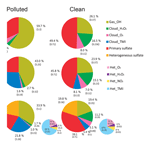
Heterogeneous sulfate aerosol formation mechanisms during wintertime Chinese haze events: air quality model assessment using observations of sulfate oxygen isotopes in Beijing
Jingyuan Shao
Qianjie Chen
Yuxuan Wang
Pengzhen He
Viral Shah
Randall V. Martin
Sajeev Philip
Shaojie Song
Zhouqing Xie
Lin Zhang
Air quality models have not been able to reproduce the magnitude of the observed concentrations of fine particulate matter (PM2.5) during wintertime Chinese haze events. The discrepancy has been at least partly attributed to low biases in modeled sulfate production rates, due to the lack of heterogeneous sulfate production on aerosols in the models. In this study, we explicitly implement four heterogeneous sulfate formation mechanisms into a regional chemical transport model, in addition to gas-phase and in-cloud sulfate production. We compare the model results with observations of sulfate concentrations and oxygen isotopes, Δ17O(), in the winter of 2014–2015, the latter of which is highly sensitive to the relative importance of different sulfate production mechanisms. Model results suggest that heterogeneous sulfate production on aerosols accounts for about 20 % of sulfate production in clean and polluted conditions, partially reducing the modeled low bias in sulfate concentrations. Model sensitivity studies in comparison with the Δ17O() observations suggest that heterogeneous sulfate formation is dominated by transition metal ion-catalyzed oxidation of SO2.
- Article
(2493 KB) -
Supplement
(3447 KB) - BibTeX
- EndNote
China has experienced rapid urbanization and industrialization in recent years, which has led to significant growth in concentration of PM2.5 (particulate matter with aerodynamic diameter less than 2.5 µm) in Chinese megacities, particularly in Beijing (the capital of China) and the surrounding area (Zhang et al., 2015, 2016; Wang et al., 2014). Extensive studies consistently show high PM2.5 levels in winter due to increased coal combustion for heating and a stable atmospheric boundary layer (Sun et al., 2014, 2016; Z. Liu et al., 2015; Ma et al., 2017). The frequency and concentration of PM2.5 pollution negatively impacts human health and atmospheric visibility and results in economic losses (Gao et al., 2015; Lelieveld, et al., 2015; Zhang et al., 2015). The Chinese government has implemented a series of policies to improve air quality, and, as a result, the annual average PM2.5 concentration in Beijing decreased by ∼20 % from 2013 to 2017 (Sun et al., 2016; Zheng et al., 2018; Beijing Environment Protection Agency, 2018; Chinese State Council, 2013). Despite these improvements, PM2.5 concentrations in Beijing still regularly exceed the Chinese National Ambient Air Quality Standards (CNAAQS, 35 µg m−3 annual average) (Sun et al., 2016; Zhang et al., 2016; Beijing Environment Protection Agency, 2018).
Sulfate is one of the most important components of PM2.5, representing 10 %–30 % of PM2.5 mass in eastern China (Huang et al., 2014; Zhang et al., 2013; Wang et al., 2014; Sun et al., 2016; Shi et al., 2017). Recent observations show that the sulfate mass fraction of PM2.5 increases during haze pollution periods, indicating that sulfate is a key driver for severe haze events (Wang et al., 2014, 2016; Cheng et al., 2016; G. Li et al., 2017). Previous simulations have shown that most models fail to predict severe haze pollution in Beijing, at least in part because of sulfate underestimation (Jiang et al., 2013; Park et al., 2014; Pozzer et al., 2012). Globally, sulfate production is dominated by the gas-phase oxidation of SO2 by OH and aqueous-phase oxidation of S(IV) (SO2 • H2O + +) by H2O2, O3, and O2 catalyzed by transition metal ions (TMIs) in cloud droplets (Calvert and Stockwell, 1983; Schwartz, 1987; Harris et al., 2013; Alexander et al., 2012; Chen et al., 2018). Heterogeneous sulfate production, which refers to aqueous-phase oxidation of S(IV) (SO2 • H2O + + ) on the surface of and/or within the bulk preexisting aerosols, is generally thought to be minor due to the low liquid water content of aerosols ( cm3 cm−3) compared to clouds (10−8 to 10−6 cm3 cm−3) (Jacob, 2000). However, recent studies have shown that traditional gas- and aqueous-phase chemistry in cloud droplets cannot explain rapid sulfate production observed during haze, suggesting missing sulfate formation mechanisms on aerosols in the models (Zheng et al., 2015; D. Chen et al., 2016; Zhang et al., 2015; Wang et al., 2014; Cheng et al., 2016; Huang et al., 2014). These missing sulfate formation mechanisms on aerosols include heterogeneous oxidation of SO2 by NO2 (Cheng et al., 2016; Wang et al., 2016, 2018; Gao et al., 2016; Zhang et al., 2015) and O2 catalyzed by TMIs (G. Li et al., 2017; Li et al., 2011) and via a free radical chain mechanism (Huie and Neta, 1984; Hung and Hoffman, 2015). The importance of these heterogeneous reactions remains highly uncertain, due in part to uncertainties regarding the aerosol liquid water content, pH, and ionic strength, all of which impact heterogeneous reaction rates (Herrmann et al., 2015; Cheng et al., 2016). In particular, ambient aerosol pH cannot be directly measured, and thus represents a large source of uncertainty (Hennigan et al., 2015).
Previous studies have calculated a large range of aerosol pH values (3.4–7.8) in Beijing using a thermodynamic model (ISORROPIA-II) (Fountoukis and Nenes, 2007). He et al. (2018) noted that the large differences in calculated aerosol pH depended on whether they assume aerosols exist in a stable (Wang et al., 2016; He et al., 2018) or metastable state (Liu et al., 2017; Guo et al., 2017; He et al., 2018). A stable-state assumption allows for the simultaneous existence of solid and aqueous phases, while the metastable-state assumption allows for the existence of aqueous phase only by assuming salts to be supersaturated in aerosols (Fountoukis and Nenes, 2007). Aerosol pH values simulated assuming a stable state are near neutral (pH of 7), much higher than when assuming a metastable state (pH of 4–5) during haze events in China (Guo et al., 2017; Liu et al., 2017; He et al., 2018). However, a recent study calculated an aerosol pH of around 4.6 during Chinese haze events for both the stable and metastable assumptions in ISORROPIA-II after fixing coding errors that impacted the stable-state assumption (Song et al., 2018). This casts doubt on the existence of neutral aerosol pH during Chinese haze events and thus the importance of the NO2 oxidation pathway, which is not important under acidic conditions. Other factors, such as an underestimate of NH3 emissions (Zhang et al., 2018) and Ca2+ concentrations in aerosols (Shen et al., 2016) and the lack of consideration of organic acids in aerosol pH calculations (Wang et al., 2018), add additional uncertainties in estimates of aerosol pH and thus sulfate production rates and mechanisms in Chinese haze events.
The oxygen isotopic composition Δ17O (≈ δ17O − 0.52 × δ18O) of secondary sulfate (Δ17O()) reflects the relative importance of different oxidation mechanisms in sulfate production because some of the oxidants transfer unique oxygen isotope signatures to the sulfate oxidation product (Savarino et al., 2000). Sulfate production via S(IV) oxidation by O3 and H2O2 leads to positive Δ17O () values of 9.8 ‰ and 0.7 ‰, respectively, while all other oxidants lead to Δ17O() at or near 0 ‰ (Table S1 in the Supplement) (Vicars and Savarino, 2014; Savarino and Thiemens, 1999; Lee and Schwartz, 1983; Holt et al., 1981; Dubey et al., 1997). Primary sulfate, both natural (dust and sea salt) and anthropogenic (coal and oil combustion), also has Δ17O() values equal to 0 ‰ (Dominguez et al., 2008; Lee et al., 2001). Once formed, sulfate in the atmosphere does not undergo further isotope exchange. Surface observations around the world show that Δ17O() ranges from 0 ‰ to 6 ‰ (Alexander et al., 2005, 2012; Q. Chen et al., 2016; Dominguez et al., 2008; Jenkins and Bao, 2006; Lee et al., 2001; Lee and Thiemens, 2001; McCabe et al., 2006; Patris et al., 2007; He et al., 2018; Ishino et al., 2017). Due to the large positive enrichment of sulfate formed from O3 oxidation (Δ17O() = 9.8 ‰) and the strong pH dependence of this aqueous-phase reaction, Δ17O() is highly sensitive to pH.
In this work, we implement four heterogeneous reactions for sulfate formation (via H2O2, O3, NO2, and TMIs) into a global chemical transport model (GEOS-Chem) and compare the model results with observations of sulfate and SO2 concentrations and Δ17O() in Beijing from October 2014 to January 2015. During this time period, Beijing held the Asia-Pacific Economic Cooperation (APEC) meeting from 5 to 11 November 2014. During and before APEC, SO2 emissions in Beijing and its surrounding regions decrease due to strict emission controls to improve air quality (Zhang et al., 2016; J. G. Liu et al., 2015). This paper is organized as follows. Section 2 describes the model simulations and the observations, and the method used to calculate heterogeneous sulfate production rates. Section 3 discusses model results with and without heterogeneous sulfate production considered in comparison with observed concentration and Δ17O(). Section 4 discusses and explains the differences between our results and observations. Section 5 summarizes the main conclusions.
2.1 GEOS-Chem model
We use the three-dimensional GEOS-Chem chemical transport model nested-grid version v10-01 (http://www.geos-chem.org/, last access: 1 May 2019) to investigate sulfate formation mechanisms in Beijing, China, between 18 October 2014 and 17 January 2015 (Wang et al., 2004, 2013, 2014; Zhang et al., 2015, 2016). The model has a horizontal resolution of 1∕4∘ latitude by 5∕16∘ longitude over East Asia (70–140∘ E, 15–55∘ N) and 47 vertical levels up to 0.01 hPa (≈81 km). In the boundary layer where most heterogeneous sulfate production occurs, the vertical layer thickness is 120–150 m for the first 12 model layers (below 1700 m altitude). The model is driven by assimilated meteorological data from the NASA Goddard Earth Observing System (GEOS-FP), which have a temporal resolution of 3 h (1 h for surface quantities and mixing depths).
The model utilizes the global anthropogenic emission inventory EDGAR v4.2 (EC-JRC/PBL, 2011, http://edgar.jrc.ec.europa.eu/, last access: 1 May 2019), overwritten by regional inventories such as the MIX Asian emission inventory over Asia (M. Li et al., 2017), EMEP over Europe (http://www.emep.int/index.html, last access: 1 May 2019), and NEI2011 over the US (https://www.epa.gov/air-emissions-inventories, last access: 1 May 2019). In particular, the MIX Asian emission inventory includes emissions of SO2, NOx, CO, NH3 and non-methane volatile organic compounds (NMVOCs) at a spatial resolution of 0.25∘ × 0.25∘ for the year 2010 (M. Li et al., 2017; Geng et al., 2017). Mineral dust aerosols are emitted in the model as described in Fairlie et al. (2007) and distributed in four size bins (radius of 0.1–1.0, 1.0–1.8, 1.8–3.0, and 3.0–6.0 µm). In addition to natural dust, our model includes anthropogenic dust (radius of 0.1–1.0 µm) released from anthropogenic activities such as road, residential, and commercial construction and combustion, following Phillip et al. (2017). Previous studies have suggested that anthropogenic dust accounts for about 25 % of the PM2.5 mass fraction in Beijing (Zhang et al., 2015; Phillip et al., 2017).
The sulfate–nitrate–ammonium aerosol system is fully coupled to oxidant chemistry (Park et al., 2004), with aerosol pH, ionic strength, and aerosol water content (AWC) calculated from the ISORROPIA-II thermodynamic equilibrium model (Fountoukis and Nenes, 2007) that was implemented into GEOS-Chem by Pye et al. (2009). In the standard model (Run_Std), sulfate is produced from gas-phase oxidation of SO2 by OH, aqueous-phase oxidation of S(IV) (= SO2 • H2O + + ) by H2O2 and O3 in cloud droplets, and heterogeneous oxidation on sea salt aerosols by O3 (Alexander et al., 2005). Primary anthropogenic emissions of sulfate constitute 3.1 % of total anthropogenic sulfur emissions in China and 1.5 %–3.5 % elsewhere. Sulfate is removed from the atmosphere via dry (Zhang et al., 2001) and wet (Liu et al., 2001) deposition, with a global lifetime of about 4 d (Alexander et al., 2005).
Table 1Description of model simulations.
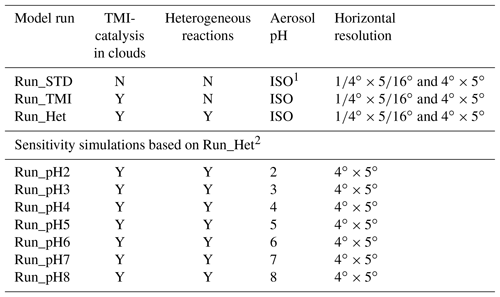
1 ISO means that the aerosol pH was calculated by ISORROPIA II in the model assuming a metastable state. 2 For the sensitivity simulations, aerosol pH was fixed to the stated prescribed value.
We performed three simulations at high horizontal resolution (1∕4∘ × 5∕16∘) and seven sensitivity simulations at low horizontal resolution (4∘ × 5∘) to investigate sulfate formation mechanisms in Beijing, as summarized in Table 1. In the model simulation Run_TMI, we implemented the in-cloud TMI-catalyzed aqueous-phase S(IV) oxidation by O2 chemistry into the model, which is thought to be one of the most important sulfate formation pathways during Northern Hemisphere winter (Huang et al., 2014; Harris et al., 2013; Alexander et al., 2009; Sofen et al., 2011). The parameterization of TMI-catalyzed S(IV) oxidation in cloud for GEOS-Chem follows Alexander et al. (2009) but with a reduced solubility of trace metals Fe and Mn derived from natural dust (from 1 % to 0.45 % for Fe and from 50 % to 5 % for Mn) to better match observations (Desboeufs et al., 2001, 2005; Chuang et al., 2005). In the model, Fe from natural dust ([Fe]nat) is 3.5 % of total dust mass and Mn from natural dust ([Mn]nat) is a factor of 50 lower than [Fe]nat (Alexander et al., 2009). Anthropogenic Mn ([Mn]ant) and Fe ([Fe]ant) are scaled to the abundance of primary anthropogenic sulfate due to common sources and atmospheric lifetimes. [Mn]ant is 1∕300 of primary sulfate concentration and [Fe]ant is 10 times that of [Mn]ant as described in Alexander et al. (2009). Only soluble Fe and Mn in the oxidation states Fe(III) and Mn(II) catalyze S(IV) oxidation. For Fe, we assume solubility of 10 % for [Fe]ant and 0.45 % of [Fe]nat in cloud water, respectively, with 10 % in the form of Fe(III) during daytime and 90 % at night. For Mn, we assume a solubility of 50 % for [Mn]ant and 5 % for [Mn]nat in cloud water, respectively, with 100 % in the form of Mn(II). After modification, the average aqueous-phase concentration of Fe(III) in cloud water during our studying period is 2.9 µM and Mn(II) is 1.3 µM in the model, which is consistent with previous work (Fe(III): 0.6–7.4 µM; Mn(II): 0.4–1.7 µM) (He et al., 2018; Shen et al., 2012; Guo et al., 2012; see Sect. S3 for more details).
In the model run Run_Het, we added four heterogeneous sulfate production mechanisms (via H2O2, O3, NO2, and TMIs) on aerosols into the model, in addition to TMI-catalyzed oxidation in clouds. Implementation of these reactions in the model is described in Sect. 2.2. In Run_Het, heterogeneous sulfate production on aerosols only occurs when relative humidity (RH) is greater than 50 %, effectively assuming that aerosol water content is too low for sufficient heterogeneous sulfate production at RH < 50 %. When RH < 50 %, aerosols are assumed to remain crystallized until reaching the deliquescence relative humidity (DRH). This is consistent with observations in previous studies showing that sulfate production rates in Chinese haze are positively correlated with RH (Sun et al., 2013; Zhang et al., 2015; Wang et al., 2016). Ca2+ and Mg2+ cations from dust (both natural and anthropogenic) are included in the aerosol thermodynamic calculations (aerosol pH, aerosol water content, ionic strength). We assume that Ca2+ and Mg2+ cations constitute 3.0 % and 0.6 % of the dust by mass, respectively, based on observations near East Asian dust source regions (Fairlie et al., 2010). In addition, we considered the impacts of acidity and ionic strength on TMI-catalyzed reaction rates following Cheng et al. (2016) (Table 2), since the ionic strength of aerosol liquid water can reach 20 M during polluted periods (He et al., 2018; Herrmann et al., 2015). We performed seven sensitivity studies based on Run_Het but with prescribed values of aerosol pH to examine the dependence of model results on aerosol pH alone (Table 1).
Table 2Aqueous-phase reaction rate expressions, rate constants (k), and the influence of ionic strength (Is) on the first-order aqueous-phase sulfate production in aerosol.
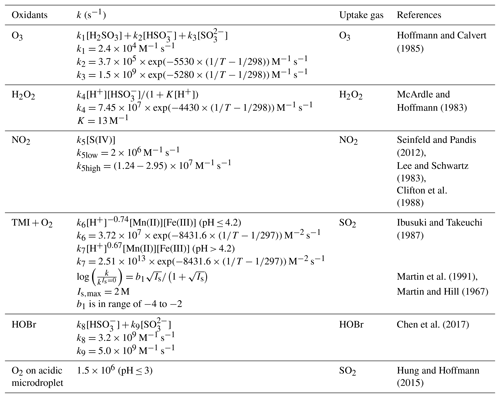
For all model simulations, sulfate produced from each oxidation pathway is labeled as a separate “tracer” in the model with a corresponding Δ17O() values (nine sulfate tracers in total, Table S1) as originally described in Alexander et al. (2005). Primary anthropogenic sulfate is also included as a separate tracer in the model with Δ17O = 0 ‰ (Lee et al., 2002). The details for calculating Δ17O() in the model are described in the Supplement (Text S1).
2.2 Heterogeneous sulfate production on aerosols
Heterogeneous S(IV) oxidation in the model occurs on all aerosol types, including sulfate–nitrate–ammonium, dust, black carbon, organic carbon, and sea salt aerosols. The heterogeneous sulfate production rate on aerosol (Phet) is calculated in the model assuming a first-order loss of SO2 or oxidant (depending on which is the rate limiting step) via uptake by the aerosol (Eq. 1).
The first-order loss rate constant (k, s−1) is calculated using the reaction probability formulation in Jacob (2000) (Eq. 2).
where ra is the radius of the specific type of aerosol (cm), A is the total aerosol surface area per unit volume of air for the specific type of aerosol (cm2 cm−3), v is the mean molecular speed of SO2 or the oxidant (cm s−1), Dg represents the gas-phase molecular diffusion coefficient of SO2 or the oxidant (cm2 s−1) calculated as follows:
where T is air temperature (K), ρair is air density (molecule m−3), and M represents the molar mass of SO2 or the oxidant (g mol−1). The reaction probability (γ) is defined as the probability that a molecule impacting the aerosol surface undergoes a chemical reaction (Ravishankara, 1997; Jacob, 2000). Due to limited understanding of sulfate formation on aerosols, chemical transport models typically calculate heterogeneous sulfate production rate on aerosols by assuming the bulk first-order uptake of SO2 and using a wide range of γ values (10−4–10−1) (Wang et al., 2014; Zhang et al., 2015). However, the relative contribution of different sulfate production mechanisms, which is important to inform air pollution mitigation efforts, cannot be determined with this simplified approach.
In this study, we use a more specific approach to calculate γ for each heterogeneous sulfate production mechanisms following Jacob (2000) and Ammann et al. (2013) (Eq. 4).
where α is the mass accommodation coefficient (unitless), kchem is the pseudo first-order aqueous-phase chemical rate constant between S(IV) and the oxidant (O3, H2O2, NO2 or O2) (s−1) (Table 2), Da is the aqueous-phase molecular diffusion coefficient of SO2 or the oxidant (cm2 s−1), K* is the effective Henry's law constant of SO2 or the oxidant (M atm−1), R is the universal gas constant (L atm mol−1 K−1), and fr is reacto-diffusive correction term that compares the radius of aerosols (ra) with the reacto-diffusive length scale of the reaction (l):
In the model, the heterogeneous sulfate production rate from the TMI-catalyzed reaction is calculated as first-order uptake in SO2. All other heterogeneous sulfate production pathways are calculated as first-order uptake in the oxidant (H2O2, O3, and NO2). This is based on whether the heterogeneous sulfate production on aerosol is limited by the availability of SO2 or the oxidant. For aerosol pH values less than 6, heterogeneous sulfate production rates calculated as a first-order loss in SO2 or the oxidant are similar (Fig. S1b in the Supplement). For aerosol pH values greater than 6, heterogeneous sulfate production rates calculated as a first-order loss in SO2 are higher than those calculated as a first-order loss in the oxidants O3 and NO2. The reaction rate for S(IV) oxidation by O3 and NO2 increases with increasing pH, and at high pH values γ is limited by the mass accommodation coefficient and becomes independent of pH. The mass accommodation coefficients for O3 () and NO2 () are much lower than for SO2 (0.23). The mass accommodation coefficient for H2O2 (0.11) is similar to SO2, and γ for the reaction of S(IV) with H2O2 was limited by the oxidant concentration. More details on first-order loss rates are described in Text S2.
In addition to the model simulations described in Table 1, we have also examined heterogeneous oxidation of SO2 by O2 on the surface of acidic microdroplets (Hung and Hoffman, 2015) and by HOBr (Q. Chen et al., 2016; 2017). The results are described in Text S2.
2.3 Observations of sulfate concentrations and oxygen isotopic composition
Between 17 October 2014 and 20 January 2015, PM2.5 samples were collected every 12 h for daytime (08:00–20:00 Beijing time) and nighttime (20:00–08:00 Beijing time) conditions at the campus of the University of the Chinese Academy of Sciences (40.41∘ N, 116.68∘ E, 20 m from the ground) in Beijing, around 60 km northeast of downtown. The oxygen-17 excess of sulfate (Δ17O()) of these PM2.5 samples were measured at University of Washington, Seattle. A detailed description of sampling and measurements of Δ17O() can be found in He et al. (2018). Observations of hourly sulfate concentrations were conducted at the Institute of Atmospheric Physics (IAP), Chinese Academy of Sciences (39.99∘ N, 116.37∘ E), an urban site in the north of Beijing, at ground level (∼4 m), as described by Sun et al. (2016). Sulfate concentrations in submicron aerosols (PM1) were measured by an Aerodyne High Resolution Aerosol Mass Spectrometer (HR-AMS). The difference of concentration between PM2.5 and PM1 is small because most sulfates exist in fine aerosols (Guo et al., 2014). A comparison of concentration in PM2.5 and PM1 during 22–29 November 2017 is shown in the Supplement (Fig. S2). Surface PM2.5, SO2, NO2, and O3 measurements (Figs. 1 and S6) are from the China National Environmental Monitoring Center (http://106.37.208.233:20035/, last access: 1 May 2019) with 12 sites in Beijing, including 8 urban and 4 suburban sites.
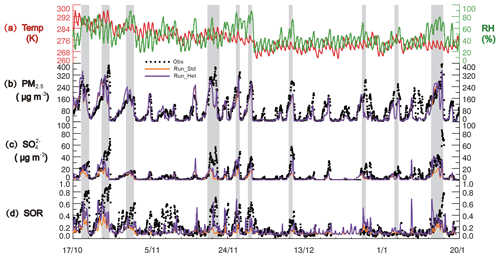
Figure 1Time series of (a) GEOS-FP temperature (red line) and relative humidity (RH; green line), (b) PM2.5 and (c) sulfate concentration, and (d) SOR at the surface in Beijing during the study period of 17 October 2014–20 January 2015. Hourly PM2.5, sulfate, and SOR observations (black dots) are compared with model results from Run_Std (orange line) and Run_Het (purple line). The gray-shaded bars represent 10 heavy pollution periods (HPPs) (PM2.5>150 µg m−3) as defined in the text.
3.1 Observed PM2.5 and sulfate concentrations and Δ17O()
Figure 1 shows the time series of observed concentrations of PM2.5 and sulfate from 17 October 2014 to 20 January 2015, along with temperature and relative humidity in Beijing from GEOS-FP. A previous study showed that the GEOS-FP meteorological data for temperature and relative humidity are in good agreement with the ground-based measurements in Beijing (R2>0.9) (Zhang et al., 2016). The CNAAQS defines the 24h average air quality levels as excellent (PM2.5=0–35 µg m−3), good (PM2.5=35–75 µg m−3), light (PM2.5=75–115 µg m−3), moderate (PM2.5=115–150 µg m−3), heavy (PM2.5=150–250 µg m−3), and severe (PM2.5>250 µg m−3). Using this metric, 10 time periods are categorized as heavy or severe (>150 µg m−3) during 17 October 2014–20 January 2015, and we refer to these as “heavy polluted period” (HPP). Another 10 time periods were in the excellent to good category and we refer to these days as “clean period” (CP). The average relative humidity (RH) from GEOS-FP during HPP was 60±11 %, much higher than the average RH during CP (42±10 %). Higher RH can accelerate the rates of conversion of SO2 and NO2 to and , respectively, contributing to increases in PM2.5 concentrations (Wang et al., 2016; Hua et al., 2015).
The observed concentrations show a similar variation as PM2.5, increasing from 2.1±1.8 µg m−3 in CP to 25.9±11.3 µg m−3 in HPP with a mean of 11.5±7.3 µg m−3 during the entire measurement period. The mass fraction of to PM2.5 ranged from 5 % to 19 %, varying from a mean of 8±2 % in CP to 13±2 % in HPP. Observed sulfate concentrations shown in Fig. 1 are 15 % lower on average during HPP than those reported in He et al. (2018) because the concentrations shown here represent PM1 instead of PM2.5, which is in good agreement with previous studies (Sun et al., 2013, 2014, 2016) (Fig. S2). Figure 1 also shows the observed sulfur oxidation ratio (SOR), defined as the molar ratio of sulfate over the sum of sulfate and SO2 (∕(SO2 + )) (Colbeck and Harrison, 1984). The observed SOR increases from CP (9±6 %) to HPP (32±18 %), consistent with increased sulfate production rates during HPP.
Figures 2 and S4 show the Δ17O() observations, 24 measurements represent HPP and 10 represent CP. The Δ17O() values are similar in HPP and CP, 0.9±0.1 ‰ and 0.9±0.5 ‰, respectively. A more detailed description of the Δ17O() observations can be found in He et al. (2018).
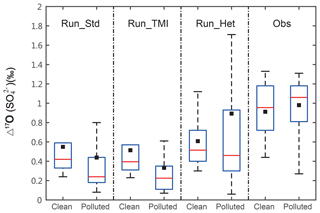
Figure 2The box charts show observed vs. modeled Δ17O() in Beijing for each model simulation, separated for heavy pollution and clean periods. The box line from bottom to top is, respectively, the percentile of 25 %, 50 %, and 75 %; the whisker from bottom to top is, respectively, the minimum and the maximum; and the black square is mean value.
3.2 Analyses of sulfate formation pathways
3.2.1 Sulfate formation in the standard model
Figure 1 compares the measured and modeled PM2.5 and sulfate concentration in Beijing. The standard model (Run_Std) generally captures the temporal variations in PM2.5 and observations during the entire sampling period but underestimates the PM2.5 and observations during HPP by 28 % and 64 %, respectively. Modeled sulfate concentrations increase from 1.5±1.2 µg m−3 in CP to 8.9±3.1 µg m−3 in HPP, which is a much smaller enhancement compared to observations (from 2.1±1.8 µg m−3 in CP to 25.9±11.3 µg m−3 in HPP). The model-simulated sulfate mass fractions in PM2.5 are 8±2 % in CP and 7±2 % in HPP. The model fails to reproduce increases in observed sulfate mass fraction from CP (8±2 %) to HPP (13±2 %). This is consistent with previous modeling studies, suggesting missing sulfate formation pathways (Wang et al., 2014; Zhang et al., 2015). The model also underestimates the sulfur oxidation ratio (SOR) observations during HPP by 53 %. This further suggests that the modeled SO2 oxidation rate is too slow.
Figure 2 compares observed and modeled Δ17O(). The modeled hourly Δ17O() values were averaged at 12 h intervals for comparison with the observations. The simulated Δ17O() values in Run_Std range from 0.02 ‰ to 1.5 ‰ with a mean of 0.5±0.1 ‰. Unlike the observations, modeled Δ17O() values in Run_Std during HPP (0.4±0.1 ‰) are lower than those during CP (0.6±0.1 ‰) due to higher fractional contribution of in-cloud H2O2 and O3 oxidation pathways during CP, as discussed below. Run_Std underestimates the Δ17O() observations (by 44 % on average), particularly during HPP (by 53 % on average).
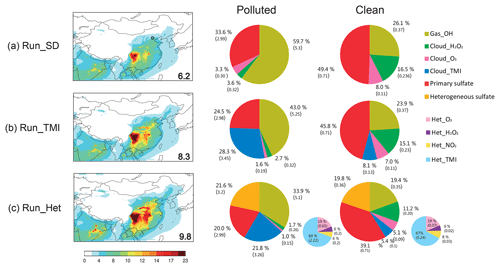
Figure 3Model-simulated sulfate aerosol concentrations (µg m−3) above the ground for (a) Run_Std, (b) Run_TMI, and (c) Run_Het. Panels on the left show the spatial distributions with the numbers in the inset representing simulated mean sulfate concentrations in Beijing (black circle in a) during the entire measurement period. The middle and right columns show the percent contributions of different sulfate formation pathways to sulfate aerosol concentration in Beijing as calculated by the different model runs during polluted (HPP) and clean (CP) periods, respectively. The smaller pie charts in (c) show the relative contributions of the four heterogeneous sulfate formation pathways implemented in the model. Numbers are percentage contributions (%) and the absolute sulfate concentrations (µg m−3) are in parentheses.
Figure 3 shows modeled spatial distribution of sulfate concentrations over China, and the fractional contribution of each sulfate formation pathway to total sulfate abundance in Beijing. The average simulated sulfate concentration in Beijing in Run_Std is around 6.2 µg m−3 (Fig. 3a), smaller than the observations (11.5 µg m−3) over the study period. Figure 3 also shows the model calculated average contribution of each sulfate production pathway to total sulfate concentration at the surface in Beijing during HPP and CP. In Run_Std, sulfate produced by gas-phase oxidation of SO2 by OH dominates the total sulfate abundance (59.7 %, 5.3 µg m−3) in HPP. Primary sulfate represents the second most important contributor (33.6 %, 3.0 µg m−3) despite the fact that primary sulfate is only 3 % of total anthropogenic sulfur emissions. The high fraction of primary anthropogenic sulfate reflects the relatively slow oxidation rate of SO2 in the model. In-cloud sulfate production contributes only 7 % of total sulfate abundance at the surface during HPP. For clean days, primary sulfate dominates surface sulfate concentrations (49.4 %, 0.7 µg m−3), suggesting an even slower SO2 oxidation rate in the model during CP compared to HPP. Gas-phase production via OH oxidation is the second most important contributor (26.1 %, 0.4 µg m−3) during CP. Gas-phase oxidation of SO2 by OH is more important in HPP than in CP because modeled OH concentrations during HPP are much higher than in CP, consistent with observations of high OH during polluted wintertime conditions in Beijing (Tan et al., 2018). Higher modeled OH in HPP compared to CP is due to higher nitrous acid (HONO) levels during HPP (Fig. S3) resulting from heterogeneous uptake of NO2 to produce HNO3 and HONO in the model. This is consistent with observation-based studies in Beijing showing that OH production from HONO photolysis is 10 times higher than that from O3 photolysis in winter (Hendrick et al., 2014) and that NO2(g) dissolution in acidic aerosol water is a source of HONO (Li et al., 2018). In-cloud sulfate production contributes 24.5 % of total sulfate abundance during CP, much higher than HPP (6.9 %) due to higher modeled H2O2 and O3 in CP.
3.2.2 Transition metal ion-catalyzed oxidation of S(IV) in clouds
The in-cloud concentration of soluble Fe3+ and Mn2+ determines the rate of sulfate formation via the TMI-catalyzed oxidation pathway, but large uncertainties exist in estimates of soluble Fe3+ and Mn2+ due to lack of observations. Adding aqueous-phase TMI-catalyzed S(IV) oxidation by O2 in cloud droplets in Run_TMI increases the average sulfate concentration in Beijing during the entire measurement period from 6.2 to 8.3 µg m−3 due to increases in the in-cloud sulfate production rate. However, the model still underestimates observations of PM2.5 (−35 %), sulfate (−48 %), and SOR (−40 %) during HPP. Sulfate from TMI-catalyzed oxidation dominates in-cloud sulfate production and accounts for up to 28.3 % of total sulfate abundance during HPP but only 8.1 % during CP in Beijing (Fig. 3b). The lower contribution of TMI catalysis during CP is due to lower concentrations of Fe and Mn in the model during CP. The largest enhancement in sulfate abundance after adding the in-cloud TMI pathway occurs in the Sichuan basin (around 6.5 µg m−3), where simulated anthropogenic Fe and Mn from coal fly ash (Fig. S4) and SO2 are high due to high SO2 emissions (Zhang et al., 2009) combined with stagnant air and high relative humidity all year (Huang et al., 2014). After adding the in-cloud TMI oxidation pathway, the average modeled Δ17O() decreased from 0.5 ‰ to 0.4 ‰ in Beijing because the TMI oxidation pathway leads to Δ17O() = 0 ‰ (Fig. 2), which makes the discrepancy between modeled and observed Δ17O() (0.9 ‰) even larger.
3.2.3 Heterogeneous sulfate formation on aerosols
Adding four heterogeneous S(IV) oxidation mechanisms by H2O2, O3, NO2 and TMI-catalyzed O2 on aerosols in Run_Het increases the average concentrations in Beijing (from 8.3 µg m−3 in Run_Std to 9.8 µg m−3 in Run_Het) (Fig. 1c). Modeled heterogeneous sulfate production represents 21.6 % of total surface sulfate concentrations in HPP and 19.8 % in CP (Fig. 3c). Modeled daily mean aerosol pH ranged from 3.0 to 5.4 with a mean of 4.3 over the entire time period in Beijing (Fig. 4), which is consistent with recent estimates (pH = 4.2–4.7) (Guo et al., 2017; Song et al., 2018; Liu et al., 2017). Heterogeneous sulfate production on aerosols is dominated by TMI-catalyzed O2 oxidation in both HPP (69 %) and CP (67 %) (Fig. 3c). S(IV) oxidation by O3 is the second most important heterogeneous oxidation pathway, accounting for 19 % of total heterogeneous sulfate formation in both HPP and CP. S(IV) oxidation by H2O2 (6 % in both HPP and CP) and NO2 (6 % in HPP and 8 % in CP) represent a minor heterogeneous sulfate production pathway. Previous studies suggested that oxidation of SO2 by NO2 in aerosol water dominates heterogeneous sulfate formation in Beijing during winter (Wang et al., 2016; Cheng et al., 2016) at neutral aerosol pH. However, subsequent studies showed that these high aerosol pH values were unlikely during wintertime Beijing haze events and they calculated aerosol pH values in the range of 4.2–4.7 (Guo et al., 2017; Song et al., 2018; Liu et al., 2017).
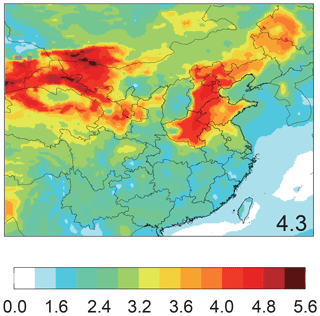
Figure 4Model-simulated mean aerosol pH values at the surface in China from 17 October 2014 to 20 January 2015 from Run_Het. Number in inset represents the mean calculated pH values in Beijing.
After adding the heterogeneous S(IV) oxidation pathways, the average modeled Δ17O() increased from 0.5±0.5 ‰ to 0.8±0.7 ‰ in Beijing, due to the increased importance of S(IV) oxidation by O3. Although the average modeled Δ17O() in Run_Het is similar to the observations (0.9±0.3 ‰), the modeled median (0.5 ‰) largely underestimates the observed median (1.0 ‰) (Fig. 2), and the majority of the modeled data underestimate the observed Δ17O() (Fig. S5).
Compared to observations in Beijing, mean model biases decrease from −28 % to −26 % for PM2.5, −45 % to −21 % for sulfate concentration, from −29 % to −11 % for SOR, and from −45 % to −15 % for Δ17O() for the entire measurement period for Run_Het relative to Run_Std. Model biases during HPP decrease from −38 % to −32 % for PM2.5, −65 % to −40 % for sulfate concentration, −53 % to −28 % for SOR, and from −50 % to −5 % for Δ17O(). The largest sulfate enhancements due to heterogeneous sulfate formation occur in megacities in eastern China and the Sichuan basin (Fig. 3), where both SO2 and aerosol abundances are highest. In addition, high anthropogenic emissions of Fe3+ and Mg2+ favor sulfate production catalyzed by TMIs. In Run_Het, the fractional contribution of each sulfate production mechanism in Beijing during HPP is 34 % for gas-phase oxidation of SO2 by OH, 22 % for TMI catalysis in cloud, 21 % for the four heterogeneous oxidation pathways, and 20 % for primary sulfate, respectively (Fig. 3c). The remaining 3 % of sulfate is formed via in-cloud sulfate production from H2O2 and O3. For clean days (CP), primary sulfate still dominates surface sulfate concentrations (39 %) in Run_Het, with gas-phase production via OH oxidation the second most important contributor (22 %) and the added heterogeneous sulfate formation pathways accounting for 22 %.
The model results demonstrate that implementation of heterogeneous sulfate formation pathways on aerosols reduces modeled low biases in both the concentration and oxygen isotopic signature of sulfate (Δ17O()) and suggests that TMI catalysis dominates heterogeneous sulfate production. However, the model is still biased low in both metrics, indicating that the model is still underestimating sulfate production rates. The modeled underestimate in Δ17O() reveals an underestimate in the O3 oxidation pathway. Since the relative contribution of heterogeneous sulfate formation pathways are sensitive to aerosol pH, we investigated the influence of aerosol pH using a series of sensitivity simulations with prescribed aerosol pH values between 2 and 8 (Table 1). Figure 5 shows modeled sulfate concentrations produced from different oxidation pathways and resulting Δ17O() in Beijing averaged over HPP assuming different aerosol pH values. Heterogeneous sulfate formation represents over 50 % of total sulfate formation when pH > 5 and when pH < 4. At aerosol pH < 4, heterogeneous sulfate formation is dominated by the TMI pathway because of the high solubility of Fe and Mn at low pH (Guieu et al., 1994; Mackie et al., 2005). At aerosol pH > 5, heterogeneous sulfate formation is dominated by the O3 pathway because the solubility of SO2 increases with increasing pH and because the S(IV) partitioning shifts to favor at higher pH. The aqueous-phase rate constant for + O3 is almost 4 orders of magnitude faster than for + O3 (Table 2). Heterogeneous sulfate formation by NO2 also increases with increasing pH due to the increased solubility of SO2 at high pH, and represents 15 %–30 % of total heterogeneous sulfate production between pH 6 and 8. In contrast to our results, previous results (Cheng et al., 2016; Wang et al., 2016) suggested that NO2 oxidation is more important than O3 oxidation at high pH values. The difference is due to assumed O3 concentrations used in the rate calculations. The aforementioned studies assumed an O3 concentration of 1 ppb relative to an NO2 concentration of 66 ppb. In our model simulations, average O3 and NO2 concentrations are 9 and 85 ppb, respectively. Modeled O3 concentrations are similar to the observations during the measurement period (Fig. S6). Heterogeneous sulfate production is lower (20 %) when pH 4–5 because of the low solubility of Fe and Mn and low concentrations of in this pH range. We note that while the pH-dependence of S(IV) partitioning is well known, the pH-dependency of metal solubility is more uncertain.
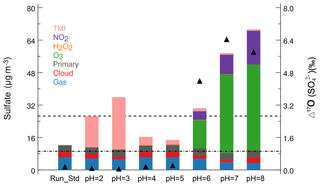
Figure 5Model-simulated mean sulfate concentration in Beijing averaged over heavy pollution periods (HPP, as defined in the text). Model results are from the standard simulation (Run_Std) and sensitivity simulations with prescribed aerosol pH ranging from 2 to 8. Different colors represent contributions from different sulfate formation pathways, including four heterogeneous reactions: oxidation by O2 (TMI), NO2, H2O2, O3; primary anthropogenic, aqueous-phase oxidation in clouds; and gas-phase oxidation. Also shown are the corresponding modeled Δ17O() values in Beijing (black triangles). The dashed line denotes observed mean sulfate concentration (25.9 µg m−3), and the dotted, dashed line denotes observed mean Δ17O() (0.9 ‰) during HPP in Beijing.
Anthropogenic SO2 emissions in China have been reduced sharply since 2009 due to the stringent pollution control measures implemented (Zheng et al., 2018; Van der A et al., 2017; Krotkov et al., 2016). Compared with 2010, anthropogenic SO2 emissions were reduced by about 50 % in 2015 (Krotkov et al., 2016; Zheng et al., 2018; Van der A et al., 2017). However, NH3 and NMVOC emissions in China remained stable during 2010–2017 due to the absence of effective mitigation measures in current policies (Zheng et al., 2018). The emission changes may affect the abundance of species that influence cloud and aerosol pH and further influence sulfate production rates and the contribution of each sulfate formation pathway. However, other studies using observations between 2014 and 2016 (Liu et al., 2017; Song et al., 2018) found a similar pH range to that calculated here, suggesting that a modeled low bias in aerosol pH is not likely to be the source of the modeled discrepancy in Δ17O().
Figure 5 also shows that model results with mean aerosol pH > 5 would result in a high bias in Δ17O() due to the increasing importance of the O3 oxidation pathway at higher pH values, effectively providing an observational constraint for typical aerosol pH < 5 in Beijing haze. However, modeled Δ17O() calculated at the aerosol pH of 4.2-4.7, as estimated by recent studies (Guo et al., 2017; Song et al., 2018) represents a low bias relative to the observations. A joint comparison of observations and model results with both sulfate concentrations and Δ17O() suggests an average aerosol pH between 5 and 6 in Beijing during the study period, which is higher than bulk aerosol pH calculations in our model calculations and in previous studies (Guo et al., 2017; Song et al., 2018).
Thermodynamic pH calculations in ISORROPIA II are based on the assumption of internally mixed aerosols and do not directly consider aerosol alkalinity (carbonate). Fresh dust is initially alkaline (calcium carbonates). This alkalinity is partially depleted (carbonate is converted to CO2) upon uptake of the acid gases HNO3, SO2, and H2SO4 (Fairlie et al., 2010; Usher et al., 2003). Due to the high pH of alkaline dust, uptake of SO2 would be followed by heterogeneous oxidation by O3 (Fairlie et al., 2010; Ullerstam et al., 2003) and NO2 (Zhao et al., 2018) if there is enough aerosol liquid water to promote aqueous-phase oxidation. A recent modeling study by Uno et al. (2017) using a previous version of GEOS-Chem explicitly includes uptake of HNO3, SO2, and H2SO4 on alkaline dust aerosols as described in Fairlie et al. (2010). Their model calculated that sulfate from uptake of SO2 and H2SO4 on alkaline dust in Beijing during our measurement period represents on average 3 % of total sulfate abundance. Most of this sulfate formation on alkaline dust was from uptake of H2SO4 (70 %–80 %, Fairlie et al., 2010), with the remaining fraction (20 %–30 %) from uptake of SO2, followed by oxidation to sulfate. On average, less than 1 % of sulfate in Beijing is formed on alkaline dust via the uptake and oxidation of SO2 in the model.
At pH values representative of alkaline dust (pH = 7–8), sulfate formed via oxidation of SO2 on alkaline dust would be dominated by O3, leading to a relatively high Δ17O() value of 6 ‰ (Fig. 5). In order to account for the difference in the median Δ17O() observed (1.0 ‰) and modeled (0.5 ‰), sulfate formation from the uptake and oxidation of SO2 on alkaline dust would need to account for an average of 9 % of total sulfate abundance during our measurement period. This fraction is higher than that calculated in Uno et al. (2017), which did not include anthropogenic dust. In our model, anthropogenic dust accounts for 28 % of total dust in Beijing (Fig. S7), and natural dust mostly originates from the Gobi Desert in southwestern Mongolia and the Badain Jaran Desert in northern China (Zhang et al., 2003, 2016). The anthropogenic dust is not abundant enough to explain the difference between the results of Uno et al. (2017) and amount of sulfate production on alkaline dust required to explain the observed Δ17O(). Due to uncertainties in processes such as the RH dependence of the uptake of SO2 on alkaline dust, the importance of this pathway could be underestimated in Uno et al. (2017). If this process does account for the difference in modeled and observed Δ17O(), then NO2 oxidation may be slightly more important than indicated in Fig. 3. Our calculations (Fig. 5) suggest that NO2 oxidation accounts for 23 % of heterogeneous sulfate formation at aerosol pH values between 7 and 8. If sulfate formation on alkaline dust accounts for 9 % of total sulfate formation and NO2 oxidation accounts for 23 % of this sulfate formation pathway, then NO2 oxidation can account for up to 2 % of total heterogeneous sulfate formation in Beijing, which still suggests NO2 oxidation is a minor pathway during wintertime Beijing haze events.
In addition to a model underestimate of the ozone oxidation pathway, a model underestimate of the H2O2 oxidation pathway may explain part of the modeled low bias in Δ17O(). The average modeled H2O2 concentrations during HPP and CP underestimate the observations (Ye et al., 2018) by up to an order of magnitude (Fig. S3c). Mao et al. (2013) proposed a HO2-Cu-Fe catalytic mechanism for H2O2 production in the aerosol phase. In their mechanism, the uptake of O2 and subsequent heterogeneous reactions with Cu and Fe will lead to production of H2O2, when the molar ratio of dissolved Cu to Fe was >0.1. Ye et al. (2018) found that the molar ratio of dissolved Cu to Fe is >0.1 during moderately polluted days, suggesting the uptake of HO2 radicals on particles might be an important source of H2O2 during the winter in Beijing. We note, however, that an underestimate of modeled H2O2 cannot explain all of the discrepancy in Δ17O(), as sulfate formed from H2O2 oxidation (0.7 ‰) is lower than the observed mean Δ17O() of 0.9 ‰. All other oxidation pathways yield Δ17O() = 0 ‰ and cannot explain the model's low bias in Δ17O().
An increase in sulfate abundance of 9 % from formation on alkaline dust is not enough to explain the remaining model underestimate in sulfate concentrations (−40 %) and SOR (−28 %) during HPP. Two recent studies suggested that hydroxymethane sulfonate (HMS) may be present in Chinese haze events and measured as sulfate via ion chromatography and HR-AMS (Moch et al., 2018; Song et al., 2019). HMS is formed via aqueous-phase oxidation via nucleophilic attack of and on HCHO. Moch et al. (2018) suggested that in-cloud formation of HMS could explain the low model bias in sulfate concentrations, while Song et al. (2019) suggested that heterogeneous formation of HMS on aerosol surfaces can explain up to one-third of the low model bias in their study. In the present study, as the organics were removed from the sample matrix prior to Δ17O() analysis (Geng et al., 2013), suggesting that measured Δ17O() does not have significant contributions from HMS. This will depend on the efficiency of HMS removal in He et al. (2018); previous work suggests that one of the two organic removal techniques employed (H2O2 oxidation) does not remove HMS under atmospheric conditions (Munger et al., 1984, 1986). However, the presence of HMS in the sample matrix, if any, would lower measured Δ17O(), and any correction for this would increase the observed Δ17O() and further increase the discrepancy between modeled and observed Δ17O().
We have used a combination of observations and modeling of sulfate and SO2 concentrations and Δ17O() to quantify sulfate production mechanisms in Beijing. We focus on the period of 17 October 2014–20 January 2015, when 10 heavy pollution periods (HPPs) were defined with observed PM2.5 concentrations > 150 µg m−3. The standard model simulation that only includes primary sulfate and sulfate formation from gas-phase oxidation by OH and in-cloud oxidation by H2O2 and O3 underestimates mean sulfate concentration by 65 % and Δ17O() by 50 % during HPPs. Adding in-cloud oxidation catalyzed by transition metal ions (TMIs) and heterogeneous oxidation by H2O2, O3, NO2, and TMIs on aerosols can improve the model simulation of sulfate abundance and Δ17O(), with the model biases decreasing from −65 % to −40 % for sulfate and from −50 % to −5 % for Δ17O() during HPP. Modeled heterogeneous sulfate production accounts for around 20 % of total sulfate production. The model predicts that the TMI-catalyzed oxidation dominates heterogeneous sulfate production under the calculated aerosol pH of ≤5; however, this reaction is highly uncertain due to limitations in our ability to assess modeled dissolved Fe(III) and Mn(II) concentrations. The modeled Δ17O() is still biased low compared to observations, suggesting an underestimate of sulfate production by O3 oxidation. We hypothesize that sulfate aerosol production by O3 on externally mixed alkaline dust aerosol can explain at least part of the remaining discrepancy in Δ17O(). The Δ17O() observations suggest that a fractional sulfate contribution of just 9 % originating from SO2 oxidation on alkaline dust aerosol can explain the model discrepancy in Δ17O(). We calculate that sulfate formation on alkaline dust is dominated by O3 oxidation (74 %), followed by NO2 oxidation (23 %). The Δ17O() observations combined with our model calculations indicate only a minor (2 %) role of heterogeneous sulfate formation via NO2 oxidation of SO2. Future studies will examine the impact of these heterogeneous S(IV) oxidation mechanisms on the regional and global sulfur budgets.
For the model results please contact Becky Alexander (beckya@uw.edu) and Lin Zhang (zhanglg@pku.edu.cn). For isotope measurements please contact Zhouqing Xie (zqxie@ustc.edu.cn).
The supplement related to this article is available online at: https://doi.org/10.5194/acp-19-6107-2019-supplement.
BA, LZ, JYS, and YXW designed the study. BA and LZ supervised the project. JYS performed model simulations and conducted analyses with the assistance of QJC, YXW, XL, VS, RVM, SP, SJS, and YZ. ZQX and PZH conducted the oxygen isotope measurements; YLS contributed the sulfate measurements. JYS, BA, and LZ wrote the paper. All authors contributed to the interpretation of the results and improvement of the paper.
The authors declare that they have no conflict of interest.
This work is supported by the National Key Research and Development Program of China (2017YFC0210102) and the National Natural Science Foundation of China (41475112). Jingyuan Shao acknowledges support from the China Scholarship Council (201606010049). Becky Alexander acknowledges support from NSF AGS 1644998 and Yuxuan Wang acknowledge support from NSF AGS 1645062. Zhouqing Xie acknowledges support from the National Natural Science Foundation of China (91544013). We thank Duncan Fairlie, Itushi Uno, Renyi Zhang, Havala O. T. Pye, Sakiko Ishino, Shohei Hattori, Youfan Chen, Jessica Haskins, Mi Zhou, Yuanhong Zhao for helpful discussions.
This paper was edited by Eliza Harris and reviewed by two anonymous referees.
Alexander, B., Park, R. J., Jacob, D. J., Li, Q. B., Yantosca, R. M., Savarino, J., Lee, C. C. W., and Thiemens, M. H.: Sulfate formation in sea-salt aerosols: Constraints from oxygen isotopes, J. Geophys. Res., 110, D10307, https://doi.org/10.1029/2004jd005659, 2005.
Alexander, B., Park, R. J., Jacob, D. J., and Gong, S.: Transition metal-catalyzed oxidation of atmospheric sulfur: Global implications for the sulfur budget, J. Geophys. Res., 114, D02309, https://doi.org/10.1029/2008jd010486, 2009.
Alexander, B., Allman, D. J., Amos, H. M., Fairlie, T. D., Dachs, J., Hegg, D. A., and Sletten, R. S.: Isotopic constraints on the formation pathways of sulfate aerosol in the marine boundary layer of the subtropical northeast Atlantic Ocean, J. Geophys. Res., 117, D06304, https://doi.org/10.1029/2011jd016773, 2012.
Ammann, M., Cox, R. A., Crowley, J. N., Jenkin, M. E., Mellouki, A., Rossi, M. J., Troe, J., and Wallington, T. J.: Evaluated kinetic and photochemical data for atmospheric chemistry: Volume VI – heterogeneous reactions with liquid substrates, Atmos. Chem. Phys., 13, 8045–8228, https://doi.org/10.5194/acp-13-8045-2013, 2013.
Beijing Environment Protection Agency: The report for 2017 air quality in Beijing, available at: http://sthjj.beijing.gov.cn (last access: 1 May 2019), 2018.
Calvert, J. G. and Stockwell, W. R.: Acid generation in the troposphere by gas-phase chemistry, Environ. Sci. Technol., 17, 428A–443A, https://doi.org/10.1021/es00115a727, 1983.
Chen, D., Liu, Z., Fast, J., and Ban, J.: Simulations of sulfate–nitrate–ammonium (SNA) aerosols during the extreme haze events over northern China in October 2014, Atmos. Chem. Phys., 16, 10707–10724, https://doi.org/10.5194/acp-16-10707-2016, 2016.
Chen, Q., Geng, L., Schmidt, J. A., Xie, Z., Kang, H., Dachs, J., Cole-Dai, J., Schauer, A. J., Camp, M. G., and Alexander, B.: Isotopic constraints on the role of hypohalous acids in sulfate aerosol formation in the remote marine boundary layer, Atmos. Chem. Phys., 16, 11433–11450, https://doi.org/10.5194/acp-16-11433-2016, 2016.
Chen, Q., Schmidt, J. A., Shah, V., Jaeglé, L., Sherwen, T., and Alexander, B.: Sulfate production by reactive bromine: Implications for the global sulfur and reactive bromine budgets, Geophys. Res. Lett., 44, 7069–7078, https://doi.org/10.1002/2017gl073812, 2017.
Chen, Q., Sherwen, T., Evans, M., and Alexander, B.: DMS oxidation and sulfur aerosol formation in the marine troposphere: a focus on reactive halogen and multiphase chemistry, Atmos. Chem. Phys., 18, 13617–13637, https://doi.org/10.5194/acp-18-13617-2018, 2018.
Cheng, Y., Zheng, G., Wei, C., Mu, Q., Zheng, B., Wang, Z., Gao, M., Zhang, Q., He, K., Carmichael, G., Poschl, U., and Su, H.: Reactive nitrogen chemistry in aerosol water as a source of sulfate during haze events in China, Sci. Adv., 2, e1601530, https://doi.org/10.1126/sciadv.1601530, 2016.
Chinese State Council: Atmospheric Pollution Prevention and Control Action Plan, available at: http://www.gov.cn/zwgk/2013-09/12/content_2486773.htm, last access: 12 January 2018.
Chuang, P. Y., Duvall, R. M., Shafer, M. M., and Schauer, J. J.: The origin of water soluble particulate iron in the Asian atmospheric outflow, Geophys. Res. Lett., 32, L07813, https://doi.org/10.1029/2004gl021946, 2005.
Clifton, C. L., Altstein, N., and Huie, R. E.: Rate constant for the reaction of nitrogen dioxide with sulfur (IV) over the pH range 5.3–13, Environ. Sci. Technol. 22, 586–589, https://doi.org/10.1021/es00170a018, 1988.
Colbeck, I. and Harrison, R.: Ozone–secondary aerosol–visibility relationships in North-West England, Sci. Total Environ., 34, 87–100, https://doi.org/10.1016/0048-9697(84)90043-3, 1984.
Desboeufs, K. V., Losno, R., and Colin, J. L.: Factors influencing aerosol solubility during cloud processes, Atmos. Environ., 35, 3529–3537, https://doi.org/10.1016/s1352-2310(00)00472-6, 2001.
Desboeufs, K. V., Sofikitis, A., Losno, R., Colin, J. L., and Ausset, P.: Dissolution and solubility of trace metals from natural and anthropogenic aerosol particulate matter, Chemosphere, 58, 195–203, https://doi.org/10.1016/j.chemosphere.2004.02.025, 2005.
Dominguez, G., Jackson, T., Brothers, L., Barnett, B., Nguyen, B., and Thiemens, M. H.: Discovery and measurement of an isotopically distinct source of sulfate in Earth's atmosphere, P. Natl. Acad. Sci. USA, 105, 12769–12773, https://doi.org/10.1073/pnas.0805255105, 2008.
Dubey, M. K., Mohrschladt, R., Donahue, N. M., and Anderson, J. G.: Isotope Specific Kinetics of Hydroxyl Radical (OH) with Water (H2O): Testing Models of Reactivity and Atmospheric Fractionation, J. Phys. Chem., 101, 1494–1500, https://doi.org/10.1021/jp962332p, 1997.
Fairlie, T. D., Jacob, D. J., and Park, R. J.: The impact of transpacific transport of mineral dust in the United States, Atmos. Environ., 41, 1251–1266, https://doi.org/10.1016/j.atmosenv.2006.09.048, 2007.
Fairlie, T. D., Jacob, D. J., Dibb, J. E., Alexander, B., Avery, M. A., van Donkelaar, A., and Zhang, L.: Impact of mineral dust on nitrate, sulfate, and ozone in transpacific Asian pollution plumes, Atmos. Chem. Phys., 10, 3999–4012, https://doi.org/10.5194/acp-10-3999-2010, 2010.
Fountoukis, C. and Nenes, A.: ISORROPIA II: a computationally efficient thermodynamic equilibrium model for K+-Ca2+-Mg2+--Na+---Cl−-H2O aerosols, Atmos. Chem. Phys., 7, 4639–4659, https://doi.org/10.5194/acp-7-4639-2007, 2007.
Gao, M., Guttikunda, S. K., Carmichael, G. R., Wang, Y., Liu, Z., Stanier, C. O., Saide, P. E., and Yu, M.: Health impacts and economic losses assessment of the 2013 severe haze event in Beijing area, Sci. Total Environ., 511, 553–561, https://doi.org/10.1016/j.scitotenv.2015.01.005, 2015.
Gao, M., Carmichael, G. R., Saide, P. E., Lu, Z., Yu, M., Streets, D. G., and Wang, Z.: Response of winter fine particulate matter concentrations to emission and meteorology changes in North China, Atmos. Chem. Phys., 16, 11837–11851, https://doi.org/10.5194/acp-16-11837-2016, 2016.
Geng, G., Zhang, Q., Martin, R. V., Lin, J., Huo, H., Zheng, B., Wang, S., and He, K.: Impact of spatial proxies on the representation of bottom-up emission inventories: A satellite-based analysis, Atmos. Chem. Phys., 17, 4131–4145, https://doi.org/10.5194/acp-17-4131-2017, 2017.
Geng, L., Schauer, A. J., Kunasek, S. A., Sofen, E. D., Erbland, J., Savarino, J., Allman, D. J., Sletten, R. S., and Alexander, B.: Analysis of oxygen-17 excess of nitrate and sulfate at sub-micromole levels using the pyrolysis method, Rapid Commun. Mass Spectrom., 27, 2411–2419, https://doi.org/10.1002/rcm.6703, 2013.
Guieu, C., Duce, R., and Arimoto, R.: Dissolved input of manganese to the ocean: Aerosol source, J. Geophys. Res., 99, 18789–18800, https://doi.org/10.1029/94jd01120, 1994.
Guo, H., Weber, R. J., and Nenes, A.: High levels of ammonia do not raise fine particle pH sufficiently to yield nitrogen oxide-dominated sulfate production, Sci. Rep., 7, 12109, https://doi.org/10.1038/s41598-017-11704-0, 2017.
Guo, J., Wang, Y., Shen, X., Wang, Z., Lee, T., Wang, X., Li, P., Sun, M., Collett, J. L., Wang, W., and Wang, T.: Characterization of cloud water chemistry at Mount Tai, China: Seasonal variation, anthropogenic impact, and cloud processing, Atmos. Environ., 60, 467–476, https://doi.org/10.1016/j.atmosenv.2012.07.016, 2012.
Guo, S., Hu, M., Zamora, M. L., Peng, J., Shang, D., Zheng, J., Du, Z., Wu, Z., Shao, M., Zeng, L., Molina, M. J., and Zhang, R.: Elucidating severe urban haze formation in China, P. Natl. Acad. Sci. USA, 111, 17373–17378, https://doi.org/10.1073/pnas.1419604111, 2014.
Harris, E., Sinha, B., van Pinxteren, D., Tilgner, A., Fomba, K. W., Schneider, J., Roth, A., Gnauk, T., Fahlbusch, B., Mertes, S., Lee, T., Collett, J., Foley, S., Borrmann, S., Hoppe, P., and Herrmann, H.: Enhanced Role of Transition Metal Ion Catalysis During In-Cloud Oxidation of SO2, Science, 340, 727–730, https://doi.org/10.1126/science.1230911, 2013.
He, P., Alexander, B., Geng, L., Chi, X., Fan, S., Zhan, H., Kang, H., Zheng, G., Cheng, Y., Su, H., Liu, C., and Xie, Z.: Isotopic constraints on heterogeneous sulfate production in Beijing haze, Atmos. Chem. Phys., 18, 5515–5528, https://doi.org/10.5194/acp-18-5515-2018, 2018.
Hendrick, F., Müller, J. F., Clémer, K., Wang, P., De Mazière, M., Fayt, C., Gielen, C., Hermans, C., Ma, J. Z., Pinardi, G., Stavrakou, T., Vlemmix, T., and Van Roozendael, M.: Four years of ground-based MAX-DOAS observations of HONO and NO2; in the Beijing area, Atmos. Chem. Phys., 14, 765–781, https://doi.org/10.5194/acp-14-765-2014, 2014.
Hennigan, C. J., Izumi, J., Sullivan, A. P., Weber, R. J., and Nenes, A.: A critical evaluation of proxy methods used to estimate the acidity of atmospheric particles, Atmos. Chem. Phys., 15, 2775–2790, https://doi.org/10.5194/acp-15-2775-2015, 2015.
Herrmann, H., Schaefer, T., Tilgner, A., Styler, S. A., Weller, C., Teich, M., and Otto, T.: Tropospheric aqueous-phase chemistry: kinetics, mechanisms, and its coupling to a changing gas phase, Chem. Rev., 115, 4259–4334, https://doi.org/10.1021/cr500447k, 2015.
Hoffmann, M. R. and Calvert, J. G.: Chemical Transformation Modules for Eulerian Acid Deposition Models: Volume II, the Aqueous-phase Chemistry, Atmospheric Sciences Research Laboratory, Office of Research and Development, US Environmental Protection Agency, Boulder, Colorado, USA, 1985.
Holt, B. D., Kumar, R., and Cunningham, P. T.: Oxygen-18 study of the aqueous-phase oxidation of sulfur dioxide, Atmos. Environ., 15, 557–566, https://doi.org/10.1016/0004-6981(81)90186-4, 1981.
Hua, Y., Cheng, Z., Wang, S., Jiang, J., Chen, D., Cai, S., Fu, X., Fu, Q., Chen, C., Xu, B., and Yu, J.: Characteristics and source apportionment of PM2.5 during a fall heavy haze episode in the Yangtze River Delta of China, Atmos. Environ., 123, 380–391, https://doi.org/10.1016/j.atmosenv.2015.03.046, 2015.
Huang, X., Song, Y., Zhao, C., Li, M., Zhu, T., Zhang, Q., and Zhang, X.: Pathways of sulfate enhancement by natural and anthropogenic mineral aerosols in China, J. Geophys. Res.-Atmos., 119, 14165–14179, https://doi.org/10.1002/2014jd022301, 2014.
Huie, R. E. and Neta, P.: Chemical behavior of and radicals in aqueous solutions, J. Phys. Chem., 88, 5665–5669, https://doi.org/10.1021/j150667a042, 1984.
Hung, H. M. and Hoffmann, M. R.: Oxidation of Gas-Phase SO2 on the Surfaces of Acidic Microdroplets: Implications for Sulfate and Sulfate Radical Anion Formation in the Atmospheric Liquid Phase, Environ. Sci. Technol., 49, 13768–13776, https://doi.org/10.1021/acs.est.5b01658, 2015.
Ibusuki, T. and Takeuchi, K.: Sulfur dioxide oxidation by oxygen catalyzed by mixtures of manganese(II) and iron(III) in aqueous solutions at environmental reaction conditions, Atmos. Environ., 21, 1555–1560, https://doi.org/10.1016/0004-6981(87)90317-9, 1987.
Ishino, S., Hattori, S., Savarino, J., Jourdain, B., Preunkert, S., Legrand, M., Caillon, N., Barbero, A., Kuribayashi, K., and Yoshida, N.: Seasonal variations of triple oxygen isotopic compositions of atmospheric sulfate, nitrate, and ozone at Dumont d'Urville, coastal Antarctica, Atmos. Chem. Phys., 17, 3713–3727, https://doi.org/10.5194/acp-17-3713-2017, 2017.
Jacob, D. J.: Heterogeneous chemistry and tropospheric ozone, Atmos. Environ., 34, 2131–2159, https://doi.org/10.1016/s1352-2310(99)00462-8, 2000.
Jenkins, K. A. and Bao, H.: Multiple oxygen and sulfur isotope compositions of atmospheric sulfate in Baton Rouge, Atmos. Environ., 40, 4528–4537, https://doi.org/10.1016/j.atmosenv.2006.04.010, 2006.
Jiang, H., Liao, H., Pye, H. O. T., Wu, S., Mickley, L. J., Seinfeld, J. H., and Zhang, X. Y.: Projected effect of 2000–2050 changes in climate and emissions on aerosol levels in China and associated transboundary transport, Atmos. Chem. Phys., 13, 7937–7960, https://doi.org/10.5194/acp-13-7937-2013, 2013.
Krotkov, N. A., McLinden, C. A., Li, C., Lamsal, L. N., Celarier, E. A., Marchenko, S. V., Swartz, W. H., Bucsela, E. J., Joiner, J., Duncan, B. N., Boersma, K. F., Veefkind, J. P., Levelt, P. F., Fioletov, V. E., Dickerson, R. R., He, H., Lu, Z., and Streets, D. G.: Aura OMI observations of regional SO2 and NO2 pollution changes from 2005 to 2015, Atmos. Chem. Phys., 16, 4605–4629, https://doi.org/10.5194/acp-16-4605-2016, 2016.
Lee, C. C. W. and Thiemens, M. H.: The δ17O and δ18O measurements of atmospheric sulfate from a coastal and high alpine region: A mass-independent isotopic anomaly, J. Geophys. Res.-Atmos., 106, 17359–17373, https://doi.org/10.1029/2000jd900805, 2001.
Lee, C. C. W., Savarino, J., and Thiemens, M. H.: Mass independent oxygen isotopic composition of atmospheric sulfate: Origin and implications for the present and past atmosphere of Earth and Mars, Geophys. Res. Lett., 28, 1783–1786, https://doi.org/10.1029/2000gl011826, 2001.
Lee, C. W., Savarino, J., Cachier, H., and Thiemens, M.: Sulfur (32S, 33S, 34S, 36S) and oxygen (16O, 17O, 18O) isotopic ratios of primary sulfate produced from combustion processes, Tellus B, 54, 193–200, https://doi.org/10.3402/tellusb.v54i3.16660, 2002.
Lee, Y. N. and Schwartz, S. E.: Kinetics of oxidation of aqueous sulfur (IV) by nitrogen dioxide, in: Kinetics of oxidation of aqueous sulfur (IV) by nitrogen dioxide, Precipitation scavenging, dry Deposition and resuspension, Proceedings of the Fourth International Conference, Santa Monica, California, 29 November–3 December 1982, vol. 1, edited by: Pruppacher, H. R. and Slinn, W. G. N., Elsevier, New York, 453–470, 1983.
Lelieveld, J., Evans, J. S., Fnais, M., Giannadaki, D., and Pozzer, A.: The contribution of outdoor air pollution sources to premature mortality on a global scale, Nature, 525, 367–371, https://doi.org/10.1038/nature15371, 2015.
Li, G., Bei, N., Cao, J., Huang, R., Wu, J., Feng, T., Wang, Y., Liu, S., Zhang, Q., Tie, X., and Molina, L. T.: A possible pathway for rapid growth of sulfate during haze days in China, Atmos. Chem. Phys., 17, 3301–3316, https://doi.org/10.5194/acp-17-3301-2017, 2017.
Li, L., Hoffmann, M. R., and Colussi, A. J.: Role of Nitrogen Dioxide in the Production of Sulfate during Chinese Haze-Aerosol Episodes, Environ. Sci. Technol., 52, 2686–2693, https://doi.org/10.1021/acs.est.7b05222, 2018.
Li, M., Zhang, Q., Kurokawa, J.-i., Woo, J.-H., He, K., Lu, Z., Ohara, T., Song, Y., Streets, D. G., Carmichael, G. R., Cheng, Y., Hong, C., Huo, H., Jiang, X., Kang, S., Liu, F., Su, H., and Zheng, B.: MIX: a mosaic Asian anthropogenic emission inventory under the international collaboration framework of the MICS-Asia and HTAP, Atmos. Chem. Phys., 17, 935–963, https://doi.org/10.5194/acp-17-935-2017, 2017.
Li, W., Zhou, S., Wang, X., Xu, Z., Yuan, C., Yu, Y., Zhang, Q., and Wang, W.: Integrated evaluation of aerosols from regional brown hazes over northern China in winter: Concentrations, sources, transformation, and mixing states, J. Geophys. Res., 116, D09301, https://doi.org/10.1029/2010jd015099, 2011.
Liu, H., Jacob, D. J., Bey, I., and Yantosca, R. M.: Constraints from 210Pb and 7Be on wet deposition and transport in a global three-dimensional chemical tracer model driven by assimilated meteorological fields, J. Geophys. Res.-Atmos., 106, 12109–12128, https://doi.org/10.1029/2000jd900839, 2001.
Liu, J. G., Xie, P., Wang, Y. S., Wang, Z. F., He, H., Liu, W.: Haze Observation and Control Measure Evaluation in Jing-Jin-Ji (Beijing, Tianjin, Hebei) Area during the Period of the Asia-Pacific Economic Cooperation (APEC) Meeting, Bull. Chin. Acad. Sci., 30, 368–377, https://doi.org/10.16418/j.issn.1000-3045.2015.03.011, 2015.
Liu, M., Song, Y., Zhou, T., Xu, Z., Yan, C., Zheng, M., Wu, Z., Hu, M., Wu, Y., and Zhu, T.: Fine particle pH during severe haze episodes in northern China, Geophys. Res. Lett., 44, 5213–5221, https://doi.org/10.1002/2017gl073210, 2017.
Liu, Z., Hu, B., Wang, L., Wu, F., Gao, W., and Wang, Y.: Seasonal and diurnal variation in particulate matter (PM10 and PM2.5) at an urban site of Beijing: analyses from a 9-year study, Environ. Sci. Pollut. Res. Int., 22, 627–642, https://doi.org/10.1007/s11356-014-3347-0, 2015.
Ma, Q., Cai, S., Wang, S., Zhao, B., Martin, R. V., Brauer, M., Cohen, A., Jiang, J., Zhou, W., Hao, J., Frostad, J., Forouzanfar, M. H., and Burnett, R. T.: Impacts of coal burning on ambient PM2.5 pollution in China, Atmos. Chem. Phys., 17, 4477–4491, https://doi.org/10.5194/acp-17-4477-2017, 2017.
Mackie, D. S., Boyd, P. W., Hunter, K. A., and McTainsh, G. H.: Simulating the cloud processing of iron in Australian dust: pH and dust concentration, Geophys. Res. Lett., 32, L06809, https://doi.org/10.1029/2004gl022122, 2005.
Mao, J., Fan, S., Jacob, D. J., and Travis, K. R.: Radical loss in the atmosphere from Cu–Fe redox coupling in aerosols, Atmos. Chem. Phys., 13, 509–519, https://doi.org/10.5194/acp-13-509-2013, 2013.
Martin, L. R. and Hill, M. W.: The iron catalyzed oxidation of sulfur: Reconciliation of the literature rates, Atmos. Environ., 21, 1487–1490, https://doi.org/10.1016/0004-6981(67)90100-x, 1967.
Martin, L. R., Hill, M. W., Tai, A. F., and Good, T. W.: The iron catalyzed oxidation of sulfur(IV) in aqueous solution: Differing effects of organics at high and low pH, J. Geophys. Res., 96, 3085, https://doi.org/10.1029/90jd02611, 1991.
McArdle, J. V. and Hoffmann, M. R.: Kinetics and mechanism of the oxidation of aquated sulfur dioxide by hydrogen peroxide at low pH, J. Phys. Chem., 87, 5425–5429, https://doi.org/10.1021/j150644a024, 1983.
McCabe, J. R., Savarino, J., Alexander, B., Gong, S., and Thiemens, M. H.: Isotopic constraints on non-photochemical sulfate production in the Arctic winter, Geophys. Res. Lett., 33, L05810, https://doi.org/10.1029/2005gl025164, 2006.
Moch, J. M., Dovrou, E., Mickley, L. J., Keutsch, F. N., Cheng, Y., Jacob, D. J., Jiang, J., Li, M., Munger, J. W., Qiao, X., and Zhang, Q.: Contribution of Hydroxymethane Sulfonate to Ambient Particulate Matter: A Potential Explanation for High Particulate Sulfur During Severe Winter Haze in Beijing, Geophys. Res. Lett., 45, 11969–11979, https://doi.org/10.1029/2018gl079309, 2018.
Munger, J. W., Jacob, D. J., and Hoffmann, M. R.: The occurrence of bisulfite-aldehyde addition products in fog- and cloud water, J. Atmos. Chem., 1, 335–350, https://doi.org/10.1007/BF00053799, 1984.
Munger, J. W., Tiller, C., and Hoffmann, M. R.: Identification of hydroxymethanesulfonate in fog water, Science, 231, 247–249, https://doi.org/10.1126/science.231.4735.247, 1986.
Park, R. J., Jacob, D. J., Field, B. D., Yantosca, R. M., and Chin, M.: Natural and transboundary pollution influences on sulfate–nitrate–ammonium aerosols in the United States: Implications for policy, J. Geophys. Res., 109, D15204, https://doi.org/10.1029/2003jd004473, 2004.
Park, R. S., Lee, S., Shin, S. K., and Song, C. H.: Contribution of ammonium nitrate to aerosol optical depth and direct radiative forcing by aerosols over East Asia, Atmos. Chem. Phys., 14, 2185–2201, https://doi.org/10.5194/acp-14-2185-2014, 2014.
Patris, N., Cliff, S. S., Quinn, P. K., Kasem, M., and Thiemens, M. H.: Isotopic analysis of aerosol sulfate and nitrate during ITCT-2k2: Determination of different formation pathways as a function of particle size, J. Geophys. Res., 112, D23301, https://doi.org/10.1029/2005jd006214, 2007.
Philip, S., Martin, R. V., Snider, G., Weagle, C. L., van Donkelaar, A., Brauer, M., Henze, D. K., Klimont, Z., Venkataraman, C., Guttikunda, S. K., and Zhang, Q.: Anthropogenic fugitive, combustion and industrial dust is a significant, underrepresented fine particulate matter source in global atmospheric models, Environ. Res. Lett., 12, 044018, https://doi.org/10.1088/1748-9326/aa65a4, 2017.
Pozzer, A., de Meij, A., Pringle, K. J., Tost, H., Doering, U. M., van Aardenne, J., and Lelieveld, J.: Distributions and regional budgets of aerosols and their precursors simulated with the EMAC chemistry-climate model, Atmos. Chem. Phys., 12, 961–987, https://doi.org/10.5194/acp-12-961-2012, 2012.
Pye, H. O. T., Liao, H., Wu, S., Mickley, L. J., Jacob, D. J., Henze, D. K., and Seinfeld, J. H.: Effect of changes in climate and emissions on future sulfate-nitrate-ammonium aerosol levels in the United States, J. Geophys. Res.-Atmos., 114, D01205, https://doi.org/10.1029/2008jd010701, 2009.
Ravishankara, A. R.: Heterogeneous and Multiphase Chemistry in the Troposphere, Science, 276, 1058–1065, https://doi.org/10.1126/science.276.5315.1058, 1997.
Savarino, J. and Thiemens, M. H.: Analytical procedure to determine both δ18O and δ17O of H2O2 in natural water and first measurements, Atmos. Environ., 33, 3683–3690, https://doi.org/10.1016/S1352-2310(99)00122-3, 1999.
Savarino, J., Lee, C. C. W., and Thiemens, M. H.: Laboratory oxygen isotopic study of sulfur (IV) oxidation: Origin of the mass-independent oxygen isotopic anomaly in atmospheric sulfates and sulfate mineral deposits on Earth, J. Geophys. Res.-Atmos., 105, 29079–29088, https://doi.org/10.1029/2000jd900456, 2000.
Schwartz, S. E.: Aqueous-phase reactions in cloud, American Chemical Society, Washington, D.C., 1987.
Seinfeld, J. H. and Pandis, S. N.: Atmospheric chemistry and physics: From air pollution to climate change, John Wiley & Sons, New Jersey, USA, 2012.
Shen, X. H., Lee, T. Y., Guo, J., Wang, X. F., Li, P. H., Xu, P. J., Wang, Y., Ren, Y., Wang, W., Wang, T., Li, Y., Cam, S. A., and Collett, J. L.: Aqueous phase sulfate production in clouds in eastern China, Atmos. Environ., 62, 502–511, https://doi.org/10.1016/j.atmosenv.2012.07.079, 2012.
Shen, Z., Sun, J., Cao, J., Zhang, L., Zhang, Q., Lei, Y., Gao, J., Huang, R.-J., Liu, S., Huang, Y., Zhu, C., Xu, H., Zheng, C., Liu, P., and Xue, Z.: Chemical profiles of urban fugitive dust PM2.5 samples in Northern Chinese cities, Sci. Total Environ., 569–570, 619–626, https://doi.org/10.1016/j.scitotenv.2016.06.156, 2016.
Shi, G., Xu, J., Peng, X., Xiao, Z., Chen, K., Tian, Y., Guan, X., Feng, Y., Yu, H., Nenes, A., and Russell, A. G.: pH of Aerosols in a Polluted Atmosphere: Source Contributions to Highly Acidic Aerosol, Environ. Sci. Technol., 51, 4289–4296, https://doi.org/10.1021/acs.est.6b05736, 2017.
Sofen, E. D., Alexander, B., and Kunasek, S. A.: The impact of anthropogenic emissions on atmospheric sulfate production pathways, oxidants, and ice core Δ17O (), Atmos. Chem. Phys., 11, 3565–3578, https://doi.org/10.5194/acp-11-3565-2011, 2011.
Song, S., Gao, M., Xu, W., Shao, J., Shi, G., Wang, S., Wang, Y., Sun, Y., and McElroy, M. B.: Fine-particle pH for Beijing winter haze as inferred from different thermodynamic equilibrium models, Atmos. Chem. Phys., 18, 7423–7438, https://doi.org/10.5194/acp-18-7423-2018, 2018.
Song, S., Gao, M., Xu, W., Sun, Y., Worsnop, D. R., Jayne, J. T., Zhang, Y., Zhu, L., Li, M., Zhou, Z., Cheng, C., Lv, Y., Wang, Y., Peng, W., Xu, X., Lin, N., Wang, Y., Wang, S., Munger, J. W., Jacob, D. J., and McElroy, M. B.: Possible heterogeneous chemistry of hydroxymethanesulfonate (HMS) in northern China winter haze, Atmos. Chem. Phys., 19, 1357–1371, https://doi.org/10.5194/acp-19-1357-2019, 2019.
Sun, Y., Wang, Z., Fu, P., Jiang, Q., Yang, T., Li, J., and Ge, X.: The impact of relative humidity on aerosol composition and evolution processes during wintertime in Beijing, China, Atmos. Environ., 77, 927–934, https://doi.org/10.1016/j.atmosenv.2013.06.019, 2013.
Sun, Y., Jiang, Q., Wang, Z., Fu, P., Li, J., Yang, T., and Yin, Y.: Investigation of the sources and evolution processes of severe haze pollution in Beijing in January 2013, J. Geophys. Res.-Atmos., 119, 4380–4398, https://doi.org/10.1002/2014jd021641, 2014.
Sun, Y., Du, W., Fu, P., Wang, Q., Li, J., Ge, X., Zhang, Q., Zhu, C., Ren, L., Xu, W., Zhao, J., Han, T., Worsnop, D. R., and Wang, Z.: Primary and secondary aerosols in Beijing in winter: sources, variations and processes, Atmos. Chem. Phys., 16, 8309–8329, https://doi.org/10.5194/acp-16-8309-2016, 2016.
Tan, Z., Lu, K., Jiang, M., Su, R., Dong, H., Zeng, L., Xie, S., Tan, Q., and Zhang, Y.: Exploring ozone pollution in Chengdu, southwestern China: A case study from radical chemistry to O3-VOC-NOx sensitivity, Sci. Total Environ., 636, 775–786, https://doi.org/10.1016/j.scitotenv.2018.04.286, 2018.
Ullerstam, M., Johnson, M. S., Vogt, R., and Ljungström, E.: DRIFTS and Knudsen cell study of the heterogeneous reactivity of SO2 and NO2 on mineral dust, Atmos. Chem. Phys., 3, 2043–2051, https://doi.org/10.5194/acp-3-2043-2003, 2003.
Uno, I., Osada, K., Yumimoto, K., Wang, Z., Itahashi, S., Pan, X., Hara, Y., Yamamoto, S., and Nishizawa, T.: Importance of Long-Range Nitrate Transport Based on Long-Term Observation and Modeling of Dust and Pollutants over East Asia, Aerosol Air Qual. Res., 17, 3052–3064, https://doi.org/10.4209/aaqr.2016.11.0494, 2017.
Usher, C. R., Michel, A. E., and Grassian, V. H.: Reactions on Mineral Dust, Chem. Rev., 103, 4883–4940, https://doi.org/10.1021/cr020657y, 2003.
Van Der A, R. J., Mijling, B., Ding, J., Koukouli, M. E., Liu, F., Li, Q., Mao, H., and Theys, N.: Cleaning up the air: effectiveness of air quality policy for SO2 and NOx emissions in China, Atmos. Chem. Phys., 17, 1775–1789, https://doi.org/10.5194/acp-17-1775-2017, 2017.
Vicars, W. C. and Savarino, J.: Quantitative constraints on the 17O-excess (Δ17O) signature of surface ozone: Ambient measurements from 50∘ N to 50∘ S using the nitrite-coated filter technique, Geochim. Cosmochim. Ac., 135, 270–287, https://doi.org/10.1016/j.gca.2014.03.023, 2014.
Wang, G., Zhang, R., Gomez, M. E., Yang, L., Levy Zamora, M., Hu, M., Lin, Y., Peng, J., Guo, S., Meng, J., Li, J., Cheng, C., Hu, T., Ren, Y., Wang, Y., Gao, J., Cao, J., An, Z., Zhou, W., Li, G., Wang, J., Tian, P., Marrero-Ortiz, W., Secrest, J., Du, Z., Zheng, J., Shang, D., Zeng, L., Shao, M., Wang, W., Huang, Y., Wang, Y., Zhu, Y., Li, Y., Hu, J., Pan, B., Cai, L., Cheng, Y., Ji, Y., Zhang, F., Rosenfeld, D., Liss, P. S., Duce, R. A., Kolb, C. E., and Molina, M. J.: Persistent sulfate formation from London Fog to Chinese haze, P. Natl. Acad. Sci. USA, 113, 13630–13635, https://doi.org/10.1073/pnas.1616540113, 2016.
Wang, G., Zhang, F., Peng, J., Duan, L., Ji, Y., Marrero-Ortiz, W., Wang, J., Li, J., Wu, C., Cao, C., Wang, Y., Zheng, J., Secrest, J., Li, Y., Wang, Y., Li, H., Li, N., and Zhang, R.: Particle acidity and sulfate production during severe haze events in China cannot be reliably inferred by assuming a mixture of inorganic salts, Atmos. Chem. Phys., 18, 10123–10132, https://doi.org/10.5194/acp-18-10123-2018, 2018.
Wang, Y., Zhang, Q. Q., He, K., Zhang, Q., and Chai, L.: Sulfate-nitrate-ammonium aerosols over China: response to 2000–2015 emission changes of sulfur dioxide, nitrogen oxides, and ammonia, Atmos. Chem. Phys., 13, 2635–2652, https://doi.org/10.5194/acp-13-2635-2013, 2013.
Wang, Y., Zhang, Q., Jiang, J., Zhou, W., Wang, B., He, K., Duan, F., Zhang, Q., Philip, S., and Xie, Y.: Enhanced sulfate formation during China's severe winter haze episode in January 2013 missing from current models, J. Geophys. Res.-Atmos., 119, 10425–10440, https://doi.org/10.1002/2013jd021426, 2014.
Wang, Y. X., McElroy, M. B., Jacob, D. J., and Yantosca, R. M.: A nested grid formulation for chemical transport over Asia: Applications to CO, J. Geophys. Res.-Atmos., 109, D22307, https://doi.org/10.1029/2004jd005237, 2004.
Ye, C., Liu, P., Ma, Z., Xue, C., Zhang, C., Zhang, Y., Liu, J., Liu, C., Sun, X., and Mu, Y.: High H2O2 Concentrations Observed during Haze Periods during the Winter in Beijing: Importance of H2O2 Oxidation in Sulfate Formation, Environ. Sci. Technol. Lett., 5, 757–763, https://doi.org/10.1021/acs.estlett.8b00579, 2018.
Zhang, L. M., Gong, S. L., Padro, J., and Barrie, L.: A size-segregated particle dry deposition scheme for an at- mospheric aerosol module, Atmos. Environ., 35, 549–560, https://doi.org/10.1016/s1352-2310(00)00326-5, 2001.
Zhang, L., Liu, L., Zhao, Y., Gong, S., Zhang, X., Henze, D. K., Capps, S. L., Fu, T.-M., Zhang, Q., and Wang, Y.: Source attribution of particulate matter pollution over North China with the adjoint method, Environ. Res. Lett., 10, 084011, https://doi.org/10.1088/1748-9326/10/8/084011, 2015.
Zhang, L., Shao, J., Lu, X., Zhao, Y., Hu, Y., Henze, D. K., Liao, H., Gong, S., and Zhang, Q.: Sources and Processes Affecting Fine Particulate Matter Pollution over North China: An Adjoint Analysis of the Beijing APEC Period, Environ. Sci. Technol., 50, 8731–8740, https://doi.org/10.1021/acs.est.6b03010, 2016.
Zhang, L., Chen, Y., Zhao, Y., Henze, D. K., Zhu, L., Song, Y., Paulot, F., Liu, X., Pan, Y., Lin, Y., and Huang, B.: Agricultural ammonia emissions in China: reconciling bottom-up and top-down estimates, Atmos. Chem. Phys., 18, 339–355, https://doi.org/10.5194/acp-18-339-2018, 2018.
Zhang, Q., Streets, D. G., Carmichael, G. R., He, K. B., Huo, H., Kannari, A., Klimont, Z., Park, I. S., Reddy, S., Fu, J. S., Chen, D., Duan, L., Lei, Y., Wang, L. T., and Yao, Z. L.: Asian emissions in 2006 for the NASA INTEX-B mission, Atmos. Chem. Phys., 9, 5131–5153, https://doi.org/10.5194/acp-9-5131-2009, 2009.
Zhang, R., Jing, J., Tao, J., Hsu, S. C., Wang, G., Cao, J., Lee, C. S. L., Zhu, L., Chen, Z., Zhao, Y., and Shen, Z.: Chemical characterization and source apportionment of PM2.5; in Beijing: seasonal perspective, Atmos. Chem. Phys., 13, 7053–7074, https://doi.org/10.5194/acp-13-7053-2013, 2013.
Zhang, X. Y., Gong, S. L., Shen, Z. X., Mei, F. M., Xi, X. X., Liu, L. C., Zhou, Z. J., Wang, D., Wang, Y. Q., and Cheng, Y.: Characterization of soil dust aerosol in China and its transport and distribution during 2001 ACE-Asia: 1. Network observations, J. Geophys. Res.-Atmos., 108, 4261–4274, https://doi.org/10.1029/2002jd002632, 2003.
Zhao, D., Song, X., Zhu, T., Zhang, Z., Liu, Y., and Shang, J.: Multiphase oxidation of SO2 by NO2 on CaCO3 particles, Atmos. Chem. Phys., 18, 2481–2493, https://doi.org/10.5194/acp-18-2481-2018, 2018.
Zheng, B., Zhang, Q., Zhang, Y., He, K. B., Wang, K., Zheng, G. J., Duan, F. K., Ma, Y. L., and Kimoto, T.: Heterogeneous chemistry: a mechanism missing in current models to explain secondary inorganic aerosol formation during the January 2013 haze episode in North China, Atmos. Chem. Phys., 15, 2031–2049, https://doi.org/10.5194/acp-15-2031-2015, 2015.
Zheng, B., Tong, D., Li, M., Liu, F., Hong, C., Geng, G., Li, H., Li, X., Peng, L., Qi, J., Yan, L., Zhang, Y., Zhao, H., Zheng, Y., He, K., and Zhang, Q.: Trends in China's anthropogenic emissions since 2010 as the consequence of clean air actions, Atmos. Chem. Phys., 18, 14095–14111, https://doi.org/10.5194/acp-18-14095-2018, 2018.