the Creative Commons Attribution 4.0 License.
the Creative Commons Attribution 4.0 License.
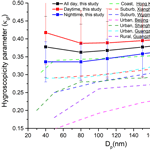
Characterization of aerosol hygroscopicity, mixing state, and CCN activity at a suburban site in the central North China Plain
Yuying Wang
Yingjie Zhang
Fang Zhang
Haobo Tan
Hanbing Xu
Tianyi Fan
Xiaoai Jin
Xinxin Fan
Zipeng Dong
Qiuyan Wang
This study investigates aerosol hygroscopicity, mixing state, and cloud condensation nucleation as part of the Atmosphere–Aerosol–Boundary Layer–Cloud Interaction Joint Experiment performed in the summer of 2016 at Xingtai (XT), a suburban site located in the center of the North China Plain (NCP). In general, the probability density function (PDF) of the hygroscopicity parameter (κ) for 40–200 nm particles had a unimodal distribution, and mean κ-PDF patterns for different sizes were similar, suggesting that the particles were highly aged and internally mixed because of strong photochemical reactions. The κ calculated from the hygroscopic growth factor in the daytime and at night suggests that photochemical reactions largely enhanced the aerosol hygroscopicity. This effect became weaker as the particle size increased. In addition, the aerosol hygroscopicity was much larger at XT than at other sites in the NCP. This is because new particle formation takes place much more frequently in the central NCP, which is heavily polluted from industrial activities, than elsewhere in the region. The evolution of the planetary boundary layer played a dominant role in dictating aerosol mass concentration. Particle size was the most important factor influencing the ability of aerosols to activate, whereas the effect of chemical composition was secondary, especially when supersaturation was high. Using a fixed value of κ=0.31 to calculate the cloud condensation nuclei number concentration in this region suffices.
- Article
(3257 KB) - Full-text XML
-
Supplement
(1287 KB) - BibTeX
- EndNote
Aerosols, defined as the mixture of solid and liquid particles suspended in air, are ubiquitous in the atmosphere because of direct emissions from biogenic and anthropogenic sources and the secondary transformation from gas precursors. Aerosol particles play an important role in climate change through direct and indirect effects (e.g., Ramanathan et al., 2001; Rosenfeld et al., 2008; Li et al., 2016). However, the impact of aerosols on climate change is difficult to simulate because of the highly variable physical and chemical properties of aerosols and complex aerosol–cloud interactions (IPCC, 2013; Lebo et al., 2017).
The hygroscopic growth and mixing state of aerosol particles are important for estimating the direct radiative effect of aerosols on Earth's climate. This is because the growth and mixing can change the particle size and optical properties of aerosol particles, which influences the solar radiation budget and atmospheric visibility. In addition, aerosol particles can be activated as cloud condensation nuclei (CCN) under supersaturation (SS) conditions. The variability in CCN number concentration (NCCN) can modify both cloud microphysical properties (Twomey, 1974; Albrecht, 1989) and morphology (Rosenfeld et al., 2008) and can lead to a broad impact on a wide range of meteorological variables including severe weather events (Li et al., 2017a).
Previous studies have addressed three main aerosol properties influencing the CCN activation, namely, particle size, chemical composition, and mixing state. However, their relative importance is different under different environmental conditions (e.g., Dusek et al., 2006; Ervens et al., 2007; Cubison et al., 2008; Deng et al., 2011; Zhang et al., 2014; Schmale et al., 2018).
Ambient aerosols are composed of different species, including inorganic ions, organic components, black carbon (BC), and mineral dust. Inorganics mainly contain sulfate, nitrate, and ammonium, while organic aerosols (OAs) consist of thousands of chemicals (Jacobson et al., 2000). The hygroscopicity and CCN activity of a single component can be characterized according to laboratory studies (e.g., Petters and Kreidenweis, 2007), but the properties of their mixtures are hard to estimate because of the different chemical species and mixing states of particles in the atmosphere. Therefore, aerosol hygroscopicity and CCN activity are very different in different regions due to different chemical compositions. Comprehensive field measurements of aerosol properties in different regions are thus necessary to improve models.
China, especially the North China Plain (NCP), has been suffering from severe air pollution over the last couple of decades due to rapid industrialization and urbanization. Diverse sources and aging processes make aerosol properties particularly diverse and complex in this part of the world. As such, the region has drawn much attention regarding the aerosol mixing state, hygroscopicity, and CCN activity (Deng et al., 2011; Liu et al., 2011; Zhang et al., 2014; F. Zhang et al., 2016; S. L. Zhang et al., 2016; Wu et al., 2016; Y. Wang et al., 2017). Liu et al. (2011) and Y. Wang et al. (2017) have suggested that ambient particles are mostly an external mixture with different hygroscopicities. Deng et al. (2011) have shown that the aerosol number size distribution is critical in the prediction of NCCN while Zhang et al. (2014, 2017) have highlighted the importance of chemical composition in determining particle activation properties. However, these studies were carried out using data from the northern part of the NCP. Few studies have focused on the central region of the NCP. Compared to the northern part of the NCP, the central part of the NCP is more affected by industrial emissions because a dense cluster of China's heavy industries is there (Fu et al., 2014). Measurements of aerosol properties in the central part of the NCP are thus critically needed to investigate the impact of air pollution on the environment and climate change.
Xingtai (XT), a city located in the center of the NCP, is considered one of the most polluted cities in China. Local industrial and domestic sources of pollution are the greatest contributors to severe haze events in that region (Wang et al., 2014). A field experiment called the Atmosphere–Aerosol–Boundary Layer–Cloud (A2BC) Interaction Joint Experiment was performed at a suburban site in XT in the summer of 2016. Differences in aerosol properties at this site and at sites in the northern part of the NCP were found.
The paper is organized as follows. Sections 2 and 3 describe the measurement method and data analysis theory. Section 4 presents and discusses the measurement results, which include data time series, aerosol mixing state, hygroscopicity, and CCN prediction and its sensitivity to chemical composition. A summary and conclusions are given in Sect. 5.
2.1 Sampling site and meteorology
The A2BC experiment was carried out at the National Meteorological Basic Station located in XT (37.18∘ N, 114.37∘ E, 180 m above sea level) from 1 May to 15 June 2016. This suburban site is situated ∼17 km northwest of the XT urban area in southern Heibei Province, which is located in the central part of the NCP and to the east of the Taihang Mountains (Fig. 1a). This region is heavily populated, urbanized, and industrialized. Major industrial manufacturers include coal-based power plants, steel and iron works, glassworks, and cement mills. Weak diffusion conditions and heavy industrial emissions lead to exceptionally high concentrations of particulate matter (PM) with diameters of less than 10 and 2.5 µm (PM2.5) as well as gas pollutants such as sulfur dioxide (SO2), volatile organic compounds (VOCs), and nitrogen oxides (NOx) during the frequently occurring haze episodes in this region (Wang et al., 2014; Fu et al., 2014). Figure 1b shows the mean distribution of SO2 concentrations from May 2012 to 2016, which confirms that the measurement site is located in one of the pollution centers in this region. A detailed analysis of gas precursors and aerosol chemical species shows that this station is a representative site in this region (Zhang et al., 2018).
Time series of meteorological variables measured at this meteorological station are shown in Fig. S1 in the Supplement. This site is strongly affected by mountain–valley winds. Southeasterly winds prevail during the day and at night northwesterly winds prevail (Figs. S1 and S2). There was almost no precipitation during the study period. The ambient temperature (T) and relative humidity (RH) time series show opposing trends. Campaign-mean values of T and RH are 21.9 ∘C and 51.6 %, respectively.
2.2 Instrumentation and operation
2.2.1 Aerosol hygroscopicity measurements
The custom-built hygroscopicity tandem differential mobility analyzer (H-TDMA) used in this study has been described in detail by others (Tan et al., 2013; Y. Wang et al., 2017). Briefly, ambient aerosols are first dried and neutralized using a Nafion dryer and a soft X-ray charger. A differential mobility analyzer (DMA1, model 3081L, TSI Inc.) is used to select monodispersed particles of a certain diameter. The monodispersed particles are then passed through a Nafion humidifier with a controlled higher RH and are humidified. A second DMA (DMA2, same model as the DMA1) and a water-based condensation particle counter (WCPC, model 3787, TSI Inc.) are used to measure the number size distribution of the humidified particles. The DMA1 and WCPC can also be connected directly to measure the 10–400 nm particle number size distribution (PNSD). In this study, the dry diameters selected by the DMA1 were 40, 80, 110, 150, and 200 nm, and the humidified RH was set to 85 %. The RH calibration with ammonium sulfate for the H-TDMA is shown in Fig. S3.
The hygroscopic growth factor (GF) is defined as the ratio of the humidified diameter at a given RH to the dry diameter:
where Dp(RH) is the particle diameter at the given RH and Dp0 is the dry diameter selected by the DMA1. The measured distribution function versus GF can be calculated with WCPC data downstream from the DMA1 and DMA2. The GF probability density function is then retrieved using the TDMAfit algorithm (Stolzenburg and McMurry, 1988, 2008).
2.2.2 Aerosol chemical composition measurements
An aerosol chemical speciation monitor (ACSM) was used to measure non-refractory submicron aerosol species (sulfate, nitrate, ammonium, chloride, and organics) in real time. A PM2.5 URG Corporation cyclone (model URG-2000-30ED) was installed in the front of the sampling inlet to remove coarse particles (>2.5 µm in diameter). Before sampling into the ACSM, aerosol particles were dried (below 40 % RH) with a silica gel diffusion dryer. The ACSM was calibrated routinely with pure ammonium nitrate to determine its ionization efficiency. More detailed descriptions about the ACSM are given by Ng et al. (2011) and Sun et al. (2012). A positive matrix factor analysis was used to analyze the organic spectral matrices according to Ulbrich et al. (2009). Three factors, i.e., hydrocarbon-like OA (HOA), cooking OA (COA), and oxygenated OA (OOA), are chosen as the ACSM dataset. HOA and COA are both primary organic aerosols (POAs) while OOA is the secondary organic aerosol (SOA).
The ACSM does not detect refractory material such as BC, so a seven-wavelength aethalometer (AE-33, Magee Scientific Corp.) with a cyclone with PM with diameters less than 1 µm (PM1) was used to measure the BC mass concentration of BC particles with diameters <1.0 µm. Mineral dust and sea salt are the other refractory species, but they typically exist in the coarse mode and contribute negligibly to PM1 (Jurányi et al., 2010; Meng et al., 2014).
2.2.3 Aerosol size distribution and CCN measurements
The aerosol particle number size distribution (15–685 nm) was measured with a scanning mobility particle sizer (SMPS) that was equipped with a long DMA (model 3081L, TSI Inc.) and a condensation particle counter (model 3775, TSI Inc.). A single-column continuous-flow thermal-gradient cloud condensation nuclei counter (model CCNC-100, DMT Inc.) was used to measure the bulk CCN number concentration. Five SS levels, i.e., 0.07 %, 0.1 %, 0.2 %, 0.4 %, and 0.8 %, were set in the CCNC and the running time was 10 min for each SS level. The SS levels in the CCNC were calibrated with pure ammonium sulfate (Rose et al., 2008) before and after the measurement campaign. The corrected SS levels were 0.11 %, 0.13 %, 0.22 %, 0.40 %, and 0.75 %.
The aerosol activation ratio (AR) at a certain SS is calculated as NCCN divided by the total particle number concentration in the 15–685 nm range (N15–685 nm), i.e., . The SMPS does not measure particle number concentrations below 15 nm. Since the activation critical diameter is always larger than 15 nm at these SS levels (Zhang et al., 2014), this does not affect the calculated NCCN. Aerosol particles with diameters greater than 685 nm are also not detected by the SMPS. These larger particles will always act as CCN due to their larger dry sizes. Note that the number concentration above 685 nm in the atmosphere is always negligible (Jurányi et al., 2010).
2.2.4 Other measurements
In this study, a micro-pulse lidar (MPL-4B, Sigma Space Corp.) was used to study the evolution of the planetary boundary layer (PBL), which plays a crucial role in modulating surface air quality (Li et al., 2017b). The pulse repetition rate of the MPL was 2.5 kHz at a visible wavelength of 532 nm. The peak value of the optical energy of the laser beam was 8 µJ. The pulse duration ranged from 10 to 100 ns, and the pulse interval was set to 200 ns, corresponding to a spatial resolution of 30 m. The MPL-retrieved PBL height is the altitude at which a sudden decrease in the scattering coefficient occurs (Brooks, 2003; Quan et al., 2013). Trace-gas analyzers (manufactured by Ecotech) were used to measure the gaseous species of ozone, SO2, NOx, NO, and carbon monoxide. More detailed descriptions of the analyzers are given by Zhu et al. (2016).
Two containers at ground level housed all sampling instruments. Two air conditioners maintained the temperature at 20–25 ∘C inside the containers. All stainless tube inlets were ∼1.5 m above the top of the containers.
3.1 Hygroscopicity parameter
To link hygroscopicity measurements below and above the water vapor saturation, the Köhler theory (Köhler, 1936) is parameterized using the hygroscopicity parameter κ (Petters and Kreidenweis, 2007). This is known as the κ-Köhler theory. According to the theory, the equilibrium equation for a solution droplet at a saturation ratio S(D) is
where D and Dd are the wet and dry droplet diameters, respectively, σs∕a is the surface tension coefficient, Mw is the mole mass of water, R is the universal gas constant, T is the temperature, and ρw is the density of water.
Below the water vapor saturation, S(D) is RH, D is Dp(RH), and Dd is Dp0 from Eq. (1). The κ parameter is then calculated using H-TDMA data according to Eqs. (1) and (2):
For a multicomponent particle, the Zdanovskii–Stokes–Robinson (ZSR) mixing rule (Stokes and Robinson, 1966) can also estimate κ using chemical composition data:
where εi and κi are the volume fraction and κ for the ith chemical component, respectively. The ACSM provides the mass concentrations of inorganic ions and organics. A simplified ion-pairing scheme such as that described by Gysel et al. (2007) is applied to convert ion mass concentrations to mass concentrations of their corresponding inorganic salts (see Table S1 in the Supplement). Table S1 also lists κ and the gravimetric density of each individual component under SS conditions. In the following discussions, κgf and κchem denote the hygroscopicity parameters derived from H-TDMA measurements and estimated using the ZSR mixing rule, respectively.
3.2 CCN estimation
The critical supersaturation (sc, ) for the Dd of a particle with hygroscopicity κ is calculated from the maximum of the κ-Köhler curve (Eq. 2; Petters and Kreidenweis, 2007). Dd is also the critical diameter corresponding to sc when κ is known. The sc–Dd relationship can thus be established. According to this relationship, the critical diameter (D0, crit) can be calculated using the estimated κchem (Eq. 4) at a given SS. All particles larger than D0, crit will activate as CCN, assuming that aerosols are internally mixed. Then the CCN number concentration can be estimated from the integral of the aerosol size distribution provided by the SMPS from D0, crit to the maximum measured size (Dmax):
NCCN(SS) can then be compared to the number of CCN at the same SS measured by the CCNC.
4.1 Overview
Figures 2 and 3 show time series of the main aerosol properties measured during the field campaign. The PNSD changes dramatically (Fig. 2a) and the aerosol number concentration in the 15–50 nm range (N15–50 nm) increases sharply in the morning almost every day (Fig. 2b). The time series of the mean diameter (Dm) of particles also shows that a growth process occurs after the sharp increase in N15–50 nm. All these phenomena suggest that new particle formation (NPF) events frequently occurred at XT during the field experiment (Kulmala et al., 2012; Y. Li et al., 2017). This is likely related to the high concentration of gas precursors from mainly local emissions. High emissions of SO2 and VOCs associated with the high oxidation capacity in a polluted atmosphere make NPF events occur more frequently in northern China (Z. Wang et al., 2017).
Figure 2c–d show time series of the probability density functions (PDFs) of κgf (κ-PDF) for 40 and 150 nm particles, respectively. In general, mono-modal κ-PDFs were observed. This is different from κ-PDFs at other sites in China where bi- and trimodal distributions dominate (Liu et al., 2011; Ye et al., 2013; Jiang et al., 2016; S. L. Zhang et al., 2016; Y. Wang et al., 2017). Differences in the aerosol mixing state explain this (see Sect. 4.2).
Figure 3a shows the bulk mass concentrations of organics, sulfate, nitrate, ammonium, and chloride measured by the ACSM and the BC mass concentration measured by the AE-33. Organics and sulfate were the dominant chemical species with mass fractions in PM1 of 39.1 % and 24.7 %, respectively. Figure 3b–c show the volume fractions of paired chemical compositions and κchem, respectively. The average volume fraction of inorganics () was similar to that of organics (POA + SOA), but their volume fractions changed diurnally. In general, the volume fraction of inorganics increased during daytime while the volume fraction of organics decreased. SOA was the dominant contributor to OA, accounting for ∼69 % of the organics volume. This shows that photochemical reactions were strong at XT during the field campaign (Huang et al., 2014). The mean κchem in Fig. 3c was 0.31, with values ranging from 0.20 to 0.40. The trend in κchem was similar to that of the volume fraction of inorganics. This suggests that inorganics played a key role in κchem. This is consistent with the study by Wu et al. (2016).
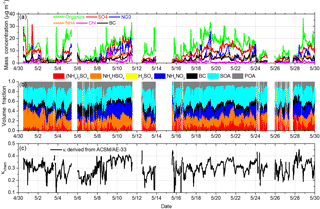
Figure 3Time series of (a) the bulk mass concentration of aerosol species in PM1, (b) the volume fractions of POA, SOA, BC, and inorganics with the simplified ion-pairing scheme, and (c) the hygroscopicity parameter derived from the chemical composition (κchem).
4.2 Aerosol mixing state and hygroscopicity
Figure 4 shows mean κ-PDFs for different particle sizes derived from H-TDMA data. For all particle sizes considered, κgf ranged from 0 to 0.8, and the κ-PDF patterns were similar. This suggests that hygroscopic compounds in different particle size modes were similar at XT. In general, κ-PDF patterns show only one hydrophilic mode with a weak hydrophobic mode occasionally appearing at night when photochemical reactions are weak (Fig. S4). The κ-PDF patterns always show bi- or trimodal distributions at other sites in China (Liu et al., 2011; Ye et al., 2013; Jiang et al., 2016; Zhang et al., 2016; Y. Wang et al., 2017). Based on previous studies (Liu et al., 2011; Y. Wang et al., 2017), ambient aerosols can be classified into three groups according to their κgf values:
- -
nearly hydrophobic (NH): κgf<0.1,
- -
less hygroscopic (LH): ,
- -
more hygroscopic (MH): 0.2≤κgf.
Table 1Number fractions of different hygroscopic groups for different particle sizes.
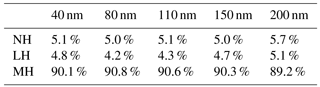
NH: nearly hydrophobic; LH: less hygroscopic; MH: more hygroscopic.
Table 1 gives the number fractions of each group for different particle sizes. The MH group dominated all particle sizes. The number fractions of the NH and LH groups were both less than 6.0 %. However, the volume fractions of hydrophobic BC and low-hygroscopicity organics (where κBC is approximately zero and κorganic is typically less than 0.1) were ∼10.1 % and 47.4 %, respectively, according to chemical composition measurements (Fig. 3b). This suggests that the particles were highly aged and internally mixed at XT during the field campaign. The coating of sulfates and secondary organics during the aging process changes the structure of BC and makes these particles grow, which can significantly enhance the hygroscopicities of particles (e.g., Zhang et al., 2008; Jimenez et al., 2009; Tritscher et al., 2011; Guo et al., 2016). The observed unimodal distribution of κ-PDF also suggests the internal mixing state of the particles (Swietlicki et al., 2008).
Figure 5 shows the average size-resolved κgf derived from H-TDMA data at XT and other sites in China. At XT, κgf values for different particle sizes were larger in the daytime than at night, and the difference between daytime and nighttime decreased with increasing particle size. This suggests that the impact of photochemical reactions on aerosol hygroscopicity is strong. The effect is weaker with increasing particle size because most of the larger particles are always well aged.
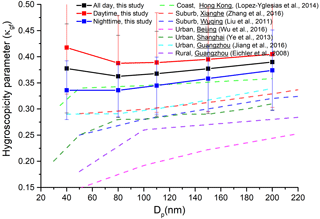
Figure 5Size-resolved aerosol hygroscopicity parameter derived from H-TDMA data at XT and at other sites in China.
The magnitude of κgf was larger at XT than at other sites in China. In particular, the magnitude of κgf was much larger at XT than at sites in the northern part of the NCP, i.e., Beijing, Wuqing, and Xianghe. The lower κgf in the Beijing urban area is likely related to the more severe traffic emissions there (Ye et al., 2013; Wu et al., 2016). Wuqing and Xianghe are located in the suburban area between the two megacities of Beijing and Tianjin and are simultaneously affected by traffic and industrial emissions. The magnitudes of κgf at these two sites are higher than at Beijing but lower than at XT. Although distant from these megacities, XT is situated in the industrial center of the NCP, so particles there are more internally mixed and highly aged due to the higher concentrations of precursors and strong photochemical reactions. This is why κgf at XT is larger than at other sites. This suggests that the hygroscopicities of particles from different emissions and chemical processes differ in the NCP. The 40 nm particles were always more hygroscopic than 80 nm particles at XT, especially in the daytime. This differed from other sites likely because the coating effect of sulfates and secondary organics is more significant for smaller particles (Tritscher et al., 2011; Guo et al., 2016). Furthermore, since the field measurements took place in a locality with heavy industrial activities, it is possible that amine contributed significantly to the hygroscopicity of 40 nm particles. Several studies have shown that amine compounds in the aerosol phase can be hygroscopic, sometimes even at low RH (e.g., Qiu and Zhang, 2012; Chu et al., 2015; Gomez-Hernandez et al., 2016).
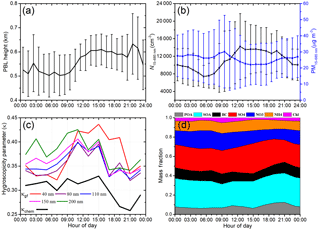
Figure 6Diurnal variations in (a) planetary boundary layer (PBL) height retrieved from micro-pulse lidar data; (b) aerosol number and mass concentrations in the 15–685 nm range (N15–685 nm and PM15–685 nm, respectively) derived from the SMPS (an aerosol density of 1.6 g cm−3 is assumed); (c) the hygroscopicity parameter derived from the hygroscopic growth factor (κgf) and predicted from the bulk chemical composition (κchem); and (d) the mass fractions of different species.
4.3 Diurnal variations in aerosol properties
4.3.1 Diurnal variations in aerosol number and mass concentrations
Figure 6a shows the diurnal variation in MPL-derived PBL height. The PBL height is the altitude at which a sudden decrease in the MPL-measured scattering coefficient occurs (Cohn and Angevine, 2000; Brooks, 2003). Note that the retrieved PBL height is only valid from 07:00 to 19:00 local time (LT) (Quan et al., 2013). The retrieved PBL height at night is not accurate because of the likely influence of residual aerosols within the nocturnal PBL. The evolution of PBL height from 07:00 to 19:00 LT is sufficient to analyze its link with the change in aerosol number and mass concentrations during the daytime. Figure 6b shows diurnal variations in aerosol number and mass concentrations in the 15–685 nm range (N15–685 nm and PM15–685 nm, respectively). Variations in N15–685 nm and PM15–685 nm trended opposite from each other. From 08:00 to 14:00 LT, the PBL height lifted from ∼0.5 to ∼0.6 km, while PM15–685 nm generally decreased from ∼24 to ∼19 µg m−3. This suggests the important effect of PBL evolution on PM15–685 nm. However, N15–685 nm sharply increased from ∼7600 cm−3 at 07:00 LT to ∼13 000 cm−3 at 13:00 LT. This is related to the sudden burst of small Aitken-mode particles (<50 nm) during NPF events. Newly formed fine particles contribute little to PM15–685 nm. In the evening, PM15–685 nm increased gradually while N15–685 nm decreased. The decline of the nocturnal PBL and particle coagulation and growth explains this. In other words, the evolution of the PBL influenced the aerosol mass concentration, while particle formation and growth had a greater influence on the variation in aerosol number concentration.
4.3.2 Diurnal variation in aerosol hygroscopicity
Figure 6c shows diurnal variations in κgf and κchem. Values of κgf for different particle sizes increased in the morning when the NPF event started. The increase was sharpest for 40 nm particles. The increase in κgf in the morning synchronized with the particle number concentration (N15–685 nm) but not with the PBL height, further suggesting the impact of photochemical reactions on aerosol hygroscopicity. The κgf for 40 nm particles increased from ∼0.32 at 07:00 LT to ∼0.44 at 15:00 LT and approached the κ value of pure ammonium sulfate. This also suggests that a large number of hygroscopic compounds were produced during NPF events. Figure S5 shows sharply increased concentrations of SO2 and VOCs in the morning and enhanced atmospheric oxidation capacity under high RH and low T conditions. The production of sulfate and SOAs resulted. This is why aerosol hygroscopicity and the occurrence of NPF events increased. Zhang et al. (2018) characterized the aerosol chemistry during NPF events in this field campaign. The diurnal pattern in κgf for 80–200 nm particles differs from that of 40 nm particles. The differences in κgf for 80–200 nm particles in the early morning were large but gradually decreased as the sun rose. The κgf for 80–200 nm particles was similar but lower than that for 40 nm particles after 11:00 LT. The condensation of sulfates and secondary organics likely caused the enhanced hygroscopicity of the 40–200 nm particles, especially of 40 nm particles (Fig. 6d).
Figure 6c also shows that the κchem for PM1 was lower than the κgf for 40–200 nm particles and had a weaker diurnal variation. This feature was stronger at noon when atmospheric oxidation and the aging process were more rapid. The simple ZSR mixing rule is responsible for this. During the daytime, the condensation of sulfuric acid on organics or BC greatly enhances their hygroscopicities (Zhang et al., 2008, 2017). The ZSR model cannot accurately represent this phenomenon. Cruz and Pandis (2000) have shown that the measured κgf of internally mixed (NH4)2SO4–OAs is larger than the predicted κchem based on the ZSR model.
In summary, the ample supply of SO2 and VOCs provided sufficient precursors for the strong photochemical reactions at XT during this field campaign, and the production and condensation of sulfate and SOAs greatly enhanced aerosol hygroscopicity, especially during the daytime. The oxidation of precursors likely induced the observed frequent NPF events.
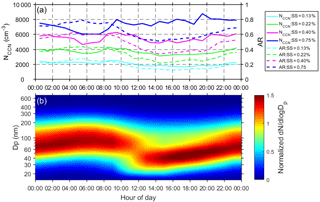
Figure 7Diurnal variations in (a) CCN number concentration (NCCN) and activation ratio (AR), and (b) the normalized aerosol size distribution in the 15–685 nm particle size range.
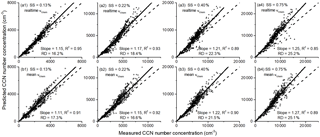
Figure 8Estimated versus measured cloud condensation nuclei (CCN) number concentrations (NCCN) for ambient aerosols at four different supersaturation (SS) levels. The NCCN is estimated based on κ-Köhler theory using the real-time κchem (a1–a4) and the mean κchem (b1–b4). The slope and coefficient of determination (R2) of the linear regression and the relative deviation (RD) of estimated NCCN (RD ) are shown in each panel. The regression line is overlaid on the measurements (solid line) and the dashed line is the 1 : 1 line.
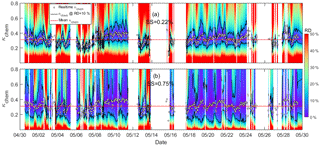
Figure 9Sensitivity of NCCN estimates to κchem as a function of time at (a) SS = 0.22% and (b) SS = 0.75 %. The color scale indicates the relative deviation (RD) of CCN estimates using the κchem value shown on the ordinate. In each panel, open circles show the real-time κchem. Note that RD is by definition zero at these points. The black line is κ at RD = 10 % and the red line is the mean value for κchem (0.31). Figure S8 shows the same plots but for SS = 0.13 % and 0.40 %.
4.3.3 Diurnal variation in CCN number concentration and activation ratio
Figure 7a shows the diurnal variations in NCCN and AR at different SS levels. In the morning, NCCN first decreased then increased, while AR showed the opposite trend. This is related to the evolution of the PBL and NPF events. At the initial stage of an NPF event, the newly formed particles were less than 15 nm in size, which was below the detection limit of the SMPS. As a result, N15–685 nm decreased (Fig. 6b) as the PBL lifted, and NCCN also decreased. However, the mixing of aged particles within the PBL made the particle size (Fig. 7b) and AR increase slightly. Condensation and the growth of new particles caused the number of fine particles detected by the SMPS to increase rapidly. However, because of their smaller sizes, some of these particles were not activated. Therefore, NCCN increased, but AR decreased from 08:00 to 14:00 LT. In the afternoon and evening, NCCN and AR increased slightly as particle sizes increased (Fig. 7b). These trends weakened as SS decreased because the critical diameter is larger at low SS values and the influence of aerosol size distribution on NCCN and AR is relatively weaker. Particle size was the most important factor influencing aerosol activation and CCN number concentrations, especially at larger SS values. Figure S6 shows the results from a sensitivity test of particle size in a CCN closure study similar to that performed by Dusek et al. (2006).
4.4 CCN estimation from chemical composition data
This section presents a CCN closure study and a discussion of the impact of chemical composition on NCCN. It is reasonable to assume that aerosols are internally mixed when estimating NCCN because H-TDMA data showed that this was the case at XT. Figure 8a shows estimated NCCN as a function of measured NCCN using real-time κchem. The estimated NCCN correlates well with measurements (R2≥0.85), but is generally overestimated. The slope of each linearly fitted line is greater than 1.10 and increases with increasing SS. The relative deviation (RD) increases from 16.2 % to 25.2 % as SS increases from 0.13 % to 0.75 %, suggesting that estimates become worse at larger SS values. The large measurement uncertainties of CCNC mainly cause the overestimation of NCCN: (1) the temperature or high flow rates in the CCNC may not allow enough time for particles to reach sizes large enough to be counted by the optical particle counter at the exit of the CCN chamber (Lance et al., 2006; Cubison et al., 2008) and (2) in high-particle-number-concentration environments, water depletion in the CCNC may reduce the counting rate of the CCNC (Deng et al., 2011). These uncertainties make measured NCCN lower than the actual NCCN. At larger SS values, activated aerosols in the cloud chamber of the CCNC are greater in number and smaller in size, so the impact of these uncertainties is greater. Figure S7 shows results from the NCCN closure study for separated NCCN. The CCN closure is reasonable when NCCN<5500 cm−3.
Figure 8b shows estimated NCCN using the mean value for κchem (κchem=0.31). Compared with results using real-time values for κchem, the fit parameters and RD change slightly, suggesting that the effect of chemical composition on NCCN is weaker relative to the particle size. Figure 9 shows the sensitivity of estimated NCCN to the variability in chemical composition. The variability in the equipotential lines of RD suggests that the sensitivity of NCCN is strongly time dependent. This is attributed to the variability in the shape of the aerosol size distribution (Jurányi et al., 2010), which further demonstrates the importance of particle size to NCCN. The sensitivity of NCCN to chemical composition (κchem) becomes weaker with increasing SS, suggesting that chemical composition becomes less important in NCCN estimates at larger SS. RD is always less than 10 % when estimating NCCN using the mean value of κchem. The value κ=0.31 is thus a good reference value to model NCCN in this region.
In summary, the particle size is the most important factor influencing aerosol activation at XT, especially at larger SS. The chemical composition was not as important when estimating NCCN because particles were highly aged and internally mixed at XT. Aerosol hygroscopicity was not sensitive to estimates of NCCN.
The Atmosphere–Aerosol–Boundary Layer–Cloud (A2BC) Interaction Joint Experiment was carried out at a suburban site (Xingtai, or XT) located in the central North China Plain (NCP) from 1 May to 15 June 2016. The study investigated aerosol hygroscopicity, the mixing state, and CCN activity at XT.
In general, the probability density function (PDF) of the hygroscopicity parameter κ (κ-PDF) for 40–200 nm particles was a unimodal distribution, which is different from distributions at other sites in China. Particles of all sizes covered a large range of κgf values (the hygroscopicity parameter derived from H-TDMA measurements; mostly from 0 to 0.8) and showed similar κ-PDF patterns, suggesting that the hygroscopic compounds in these particles from 40 to 200 nm were similar at XT. The κ-PDF patterns also suggest that particles were highly aged and internally mixed at XT during the field campaign. This is likely related to strong photochemical reactions.
The mean κgf for different particle sizes was larger in the daytime than at night. Daytime and nighttime κgf differences decreased with increasing particle size. The impact of photochemical reactions on aerosol hygroscopicity was strong, and the effect became weaker as particle size increased. The coating of sulfates or secondary organics likely enhanced the hygroscopicities of 40–200 nm particles. This effect was more significant for 40 nm particles. Compared with other sites in China, the aerosol hygroscopicity was much larger at XT because of the sufficient number of precursors and strong atmospheric oxidation. The comparison also shows that the hygroscopicities of particles from different emissions and chemical processes differed greatly.
New particle formation events occurred frequently at XT during this field campaign. The evolution of the planetary boundary layer influenced the aerosol mass concentration, while particle formation and growth had a greater influence on the variation in aerosol number concentration. Particle size was the most important factor influencing aerosol activation and the CCN number concentration (NCCN) at XT, especially at larger supersaturation (SS). Although estimated NCCN correlated well with measurements (R2≥0.85), NCCN was overestimated because of measurement uncertainties. The effect of chemical composition on NCCN was weaker relative to the particle size. Sensitivity tests show that the impact of chemical composition on NCCN became weaker as SS increased, suggesting that the effect of chemical composition on the estimation of NCCN is less important at larger SS values. The value κ=0.31 is a good proxy for NCCN in this region.
XT is located in the most polluted region in China. The multitude of factories in the region generate strong emissions. The plenitude of gas precursors and strong photochemical reactions at XT make aerosol properties there unique. More field measurements on gas–particle transformation and aerosol properties in this region are needed for studying haze formation mechanisms and climate effects.
Data used in the study are available from the first author upon request (wang.yuying@mail.bnu.edu.cn).
The supplement related to this article is available online at: https://doi.org/10.5194/acp-18-11739-2018-supplement.
ZL and YW designed the experiment; YW, YZ, and WD carried it out and analyzed the data; other co-authors participated in science discussions and suggested analyses. YW prepared the paper with contributions from all co-authors.
The authors declare that they have no conflict of interest.
This article is part of the special issue “Regional transport and transformation of air pollution in eastern China”. It is not associated with a conference.
This work was funded by National Key R&D Program of China
(2017YFC1501702), National
Natural Science Foundation of China (NSFC) research projects (grant no.
91544217, 41675141, 41705125), the National Basic Research Program of China
“973” (grant no. 2013CB955801), and the China Scholarship Council (award
no. 201706040194). We thank all participants in the field campaign for their
tireless work and cooperation.
Edited by: Yuan Wang
Reviewed by: two anonymous referees
Albrecht, B. A.: Aerosols, cloud microphysics, and fractional cloudiness, Science, 245, 1227–1230, https://doi.org/10.1126/science.245.4923.1227, 1989.
Brooks, I. M.: Finding boundary layer top: application of a wavelet covariance transform to lidar backscatter profiles, J. Atmos. Ocean. Tech., 20, 1092–1105, https://doi.org/10.1175/1520-0426(2003)020<1092:FBLTAO>2.0.CO;2, 2003.
Chu, Y., Sauerwein, M., and Chan, C. K.: Hygroscopic and phase transition properties of alkyl aminium sulfates at low relative humidities, Phys. Chem. Chem. Phys., 17, 19789–19796, https://doi.org/10.1039/c5cp02404h, 2015.
Cohn, S. A. and Angevine. W. M.: Boundary layer height and entrainment zone thickness measured by lidars and wind-profiling radars, J. Appl. Meteorol., 39, 1233–1247, https://doi.org/10.1175/1520-0450(2000)039<1233:BLHAEZ>2.0.CO;2, 2000.
Cruz, C. N. and Pandis, S. N.: Deliquescence and hygroscopic growth of mixed inorganic-organic atmospheric aerosol, Environ. Sci. Technol., 34, 4313–4319, https://doi.org/10.1021/es9907109, 2000.
Cubison, M. J., Ervens, B., Feingold, G., Docherty, K. S., Ulbrich, I. M., Shields, L., Prather, K., Hering, S., and Jimenez, J. L.: The influence of chemical composition and mixing state of Los Angeles urban aerosol on CCN number and cloud properties, Atmos. Chem. Phys., 8, 5649–5667, https://doi.org/10.5194/acp-8-5649-2008, 2008.
Deng, Z. Z., Zhao, C. S., Ma, N., Liu, P. F., Ran, L., Xu, W. Y., Chen, J., Liang, Z., Liang, S., Huang, M. Y., Ma, X. C., Zhang, Q., Quan, J. N., Yan, P., Henning, S., Mildenberger, K., Sommerhage, E., Schäfer, M., Stratmann, F., and Wiedensohler, A.: Size-resolved and bulk activation properties of aerosols in the North China Plain, Atmos. Chem. Phys., 11, 3835–3846, https://doi.org/10.5194/acp-11-3835-2011, 2011.
Dusek, U., Frank, G. P., Hildebrandt, L., Curtius, J., Schneider, J., Walter, S., Chand, D., Drewnick, F., Hings, S., and Jung D.: Size matters more than chemistry for cloud-nucleating ability of aerosol particles, Science, 312, 1375–1378, https://doi.org/10.1126/science.1125261, 2006.
Eichler, H., Cheng, Y. F., Birmili, W., Nowak, A., Wiedensohler, A., Brüggemann, E., Gnauk, T., Herrmann, H., Althausen, D., Ansmann, A., Engelmann, R., Tesche, M., Wendisch, M., Zhang, Y. H., Hu, M., Liu, S., and Zeng, L. M.: Hygroscopic properties and extinction of aerosol particles at ambient relative humidity in South-Eastern China, Atmos Environ, 42, 6321–6334, https://doi.org/10.1016/j.atmosenv.2008.05.007, 2008.
Ervens, B., Cubison, M., Andrews, E., Feingold, G., Ogren, J. A., Jimenez, J. L., DeCarlo, P., and Nenes, A.: Prediction of cloud condensation nucleus number concentration using measurements of aerosol size distributions and composition and light scattering enhancement due to humidity, J. Geophys. Res., 112, D10S32, https://doi.org/10.1029/2006JD007426, 2007.
Fu, G. Q., Xu, W. Y., Yang, R. F., Li, J. B., and Zhao, C. S.: The distribution and trends of fog and haze in the North China Plain over the past 30 years, Atmos. Chem. Phys., 14, 11949–11958, https://doi.org/10.5194/acp-14-11949-2014, 2014.
Gomez-Hernandez, M., McKeown, M., Secrest, J., Marrero-Ortiz, W., Lavi, A., Rudich, Y., Collins, D. R., and Zhang, R.: Hygroscopic characteristics of alkylaminium carboxylate aerosols, Environ. Sci. Technol., 50, 2292–2300, https://doi.org/10.1021/acs.est.5b04691, 2016.
Guo, S., Hu, M., Lin, Y., Gomez-Hernandez, M., Zamora, M. L., Peng, J., Collins, D. R., and Zhang, R.: OH-Initiated oxidation of m-xylene on black carbon aging, Environ. Sci. Technol., 50, 8605–8612, https://doi.org/10.1021/acs.est.6b01272, 2016.
Gysel, M., Crosier, J., Topping, D. O., Whitehead, J. D., Bower, K. N., Cubison, M. J., Williams, P. I., Flynn, M. J., McFiggans, G. B., and Coe, H.: Closure study between chemical composition and hygroscopic growth of aerosol particles during TORCH2, Atmos. Chem. Phys., 7, 6131–6144, https://doi.org/10.5194/acp-7-6131-2007, 2007.
Huang, R., Zhang, Y., Bozzetti, C., Ho, K., Cao, J., Han, Y., Daellenbach, K. R., Slowik, J. G., Platt, S. M., Canonaco, F., Zotter, P., Wolf, R., Pieber, S. M., Bruns, E. A., Crippa, M., Ciarelli, G., Piazzalunga, A., Schwikowski, M., Abbaszade, G., Schnelle-Kreis, J., Zimmermann, R., An, Z., Szidat, S., Baltensperger, U., Haddad, I. E., and Prévôt, A. S. H.: High secondary aerosol contribution to particulate pollution during haze events in China, Nature, 514, 218–222, https://doi.org/10.1038/nature13774, 2014.
IPCC: Climate change 2013: Scientific basis, Fifth assessment of the Inter-governmental Panel on Climate Change, Cambridge University Press, 2013.
Jacobson, M. C., Hansson, H. C., Noone, K. J., and Charlson, R. J.: Organic atmospheric aerosols: review and state of the science, Rev. Geophys., 38, 267–294, https://doi.org/10.1029/1998RG000045, 2000.
Jiang, R. X., Tan, H. B., Tang, L. L., Cai, M. F., Yin, Y., Li, F., Liu, L., Xu, H. B., Chan, P. W., Deng, X. J., and Wu, D.: Comparison of aerosol hygroscopicity and mixing state between winter and summer seasons in Pearl River Delta region, China, Atmos. Res., 169, 160–170, https://doi.org/10.1016/j.atmosres.2015.09.031, 2016.
Jimenez, J. L., Canagaratna, M. R., Donahue, N. M., et al.: Evolution of organic aerosols in the atmosphere, Science, 326, 1525–1529, https://doi.org/10.1126/science.1180353, 2009.
Jurányi, Z., Gysel, M., Weingartner, E., DeCarlo, P. F., Kammermann, L., and Baltensperger, U.: Measured and modelled cloud condensation nuclei number concentration at the high alpine site Jungfraujoch, Atmos. Chem. Phys., 10, 7891–7906, https://doi.org/10.5194/acp-10-7891-2010, 2010.
Köhler, H.: The nucleus in and the growth of hygroscopic droplets, T. Faraday Soc., 32, 1152–1161, https://doi.org/10.1039/TF9363201152, 1936.
Kulmala, M., Petäjä, T., Nieminen, T., Sipilä, M., Manninen, H. E., Lehtipalo, K., Dal Maso, M., Aalto, P. P., Junninen, H., Paasonen, P., Riipinen, I., Lehtinen, K. E. J., Laaksonen, A., and Kerminen, V.-M.: Measurement of the nucleation of atmospheric aerosol particles, Nat. Protoc., 7, 1651–1667, https://doi.org/10.1038/nprot.2012.091, 2012.
Lance, S., Nenes, A., Medina, J., and Smith, J. N.: Mapping the operation of the DMT continuous flow CCN counter, Aerosol Sci. Tech., 40, 242–254, https://doi.org/10.1080/02786820500543290, 2006.
Lebo, Z. J., Shipway, B. J., Fan, J., Geresdi, I., Hill, A., Miltenberger, A., Morrison, H., Rosenberg, P., Varble, A., and Xue, L.: Challenges for cloud modeling in the context of aerosol-cloud-precipitation interactions, B. Am. Meteorol. Soc., 98, 1749–1755, https://doi.org/10.1175/BAMS-D-16-0291.1, 2017.
Li, Y., Zhang, F., Li, Z., Sun, L., Wang, Z., Li, P., Sun, Y., Ren, J., Wang, Y., Cribb, M., and Yuan, C.: Influences of aerosol physiochemical properties and new particle formation on CCN activity from observation at a suburban site of China, Atmos. Res., 188, 80–89, https://doi.org/10.1016/j.atmosres.2017.01.009, 2017.
Li, Z., Lau, W. K.-M., Ramanathan, V., Wu, G., Ding, Y., Manoj, M. G., Liu, J., Qian, Y., Li, J., Zhou T., Fan, J., Rosenfeld, D., Ming, Y., Wang, Y., Huang, J., Wang, B., Xu, X., Lee, S.-S., Cribb, M., Zhang, F., Yang, X., Zhao, C., Takemura, T., Wang, K., Xia, X., Yin, Y., Zhang, H., Guo, J., Zhai, P. M., Sugimoto, N., Babu, S. S., and Brasseur, G. P.: Aerosol and monsoon climate interactions over Asia, Rev. Geophys., 54, 866–929, https://doi.org/10.1002/2015RG000500, 2016.
Li, Z., Daniel, R., and Fan, J. W.: Aerosols and their impact on radiation, clouds, precipitation, and severe weather events, Oxford Research Encyclopedias: Environmental Science, Interactive Factory, https://doi.org/10.1093/acrefore/9780199389414.013.126, 2017a.
Li, Z., Guo, J., Ding, A., Liao, H., Liu, J., Sun, Y., Wang, T., Xue, H., Zhang, H., and Zhu, B.: Aerosols and boundary-layer interactions and impact on air quality, Natl. Sci. Rev., 4, 810–833, https://doi.org/10.1093/nsr/nwx117, 2017b.
Liu, P. F., Zhao, C. S., Göbel, T., Hallbauer, E., Nowak, A., Ran, L., Xu, W. Y., Deng, Z. Z., Ma, N., Mildenberger, K., Henning, S., Stratmann, F., and Wiedensohler, A.: Hygroscopic properties of aerosol particles at high relative humidity and their diurnal variations in the North China Plain, Atmos. Chem. Phys., 11, 3479–3494, https://doi.org/10.5194/acp-11-3479-2011, 2011.
Lopez-Yglesias, X. F., Yeung, M. C., Dey, S. E., Brechtel, F. J., and Chan, C. K.: Performance evaluation of the Brechtel Mfg. Humidified Tandem Differential Mobility Analyzer (BMI HTDMA) for studying hygroscopic properties of aerosol particles, Aerosol Sci. Tech., 48, 969–980, https://doi.org/10.1080/02786826.2014.952366, 2014.
Meng, J. W., Yeung, M. C., Li, Y. J., Lee, B. Y. L., and Chan, C. K.: Size-resolved cloud condensation nuclei (CCN) activity and closure analysis at the HKUST Supersite in Hong Kong, Atmos. Chem. Phys., 14, 10267–10282, https://doi.org/10.5194/acp-14-10267-2014, 2014.
Ng, N. L., Herndon, S. C., Trimborn, A., Canagaratna, M. R., Croteau, P. L., Onasch, T. B., Sueper, D., Worsnop, D. R., Zhang, Q., Sun, Y. L., and Jayne, J. T.: An Aerosol Chemical Speciation Monitor (ACSM) for routine monitoring of the composition and mass concentrations of ambient aerosol, Aerosol Sci. Tech., 45, 780–794, https://doi.org/10.1080/02786826.2011.560211, 2011.
Petters, M. D. and Kreidenweis, S. M.: A single parameter representation of hygroscopic growth and cloud condensation nucleus activity, Atmos. Chem. Phys., 7, 1961–1971, https://doi.org/10.5194/acp-7-1961-2007, 2007.
Qiu, C. and Zhang, R.: Physiochemical properties of alkylaminium sulfates: hygroscopicity, thermostability, and density, Environ. Sci. Technol., 46, 4474–4480, https://doi.org/10.1021/es3004377, 2012.
Quan, J., Gao, Y., Zhang, Q., Tie, X., Cao, J., Han, S., Meng, J., Chen, P., and Zhao, D.: Evolution of planetary boundary layer under different weather conditions, and its impact on aerosol concentrations, Particuology, 11, 34–40, https://doi.org/10.1016/j.partic.2012.04.005, 2013.
Ramanathan, V., Crutzen, P. J., Kiehl, J. T., and Rosenfeld, D.: Aerosols, climate, and the hydrological cycle, Science, 294, 2119–2124, https://doi.org/10.1126/science.1064034, 2001.
Rose, D., Gunthe, S. S., Mikhailov, E., Frank, G. P., Dusek, U., Andreae, M. O., and Pöschl, U.: Calibration and measurement uncertainties of a continuous-flow cloud condensation nuclei counter (DMT-CCNC): CCN activation of ammonium sulfate and sodium chloride aerosol particles in theory and experiment, Atmos. Chem. Phys., 8, 1153–1179, https://doi.org/10.5194/acp-8-1153-2008, 2008.
Rosenfeld, D., Lohmann, U., Raga, G. B., O'Dowd, C. D., Kulmala, M., Fuzzi, S., Reissell, A., and Andreae, M. O.: Flood or drought: How do aerosols affect precipitation?, Science, 321, 1309—1313, https://doi.org/10.1126/science.1160606, 2008.
Schmale, J., Henning, S., Decesari, S., Henzing, B., Keskinen, H., Sellegri, K., Ovadnevaite, J., Pöhlker, M. L., Brito, J., Bougiatioti, A., Kristensson, A., Kalivitis, N., Stavroulas, I., Carbone, S., Jefferson, A., Park, M., Schlag, P., Iwamoto, Y., Aalto, P., Äijälä, M., Bukowiecki, N., Ehn, M., Frank, G., Fröhlich, R., Frumau, A., Herrmann, E., Herrmann, H., Holzinger, R., Kos, G., Kulmala, M., Mihalopoulos, N., Nenes, A., O'Dowd, C., Petäjä, T., Picard, D., Pöhlker, C., Pöschl, U., Poulain, L., Prévôt, A. S. H., Swietlicki, E., Andreae, M. O., Artaxo, P., Wiedensohler, A., Ogren, J., Matsuki, A., Yum, S. S., Stratmann, F., Baltensperger, U., and Gysel, M.: Long-term cloud condensation nuclei number concentration, particle number size distribution and chemical composition measurements at regionally representative observatories, Atmos. Chem. Phys., 18, 2853–2881, https://doi.org/10.5194/acp-18-2853-2018, 2018.
Stokes, R. H. and Robinson, R. A.: Interactions in aqueous nonelectrolyte solutions. I. Solute-solvent equilibria, J. Phys. Chem., 70, 2126–2131, https://doi.org/10.1021/j100879a010, 1966.
Stolzenburg, M. R. and McMurry, P. H.: TDMAFIT user's manual, Department of Mechanical Engineering, Particle Technology Laboratory, University of Minnesota, Minneapolis, 1–61, 1988.
Stolzenburg, M. R. and McMurry, P. H.: Equations governing single and tandem DMA configurations and a new lognormal approximation to the transfer function, Aerosol Sci. Tech., 42, 421–432, https://doi.org/10.1080/02786820802157823, 2008.
Sun, Y., Wang, Z., Dong, H., Yang, T., Li, J., Pan, X., Chen, P., and Jayne, J. T.: Characterization of summer organic and inorganic aerosols in Beijing, China with an Aerosol Chemical Speciation Monitor, Atmos. Environ., 51, 250–259, https://doi.org/10.1016/j.atmosenv.2012.01.013, 2012.
Swietlicki, E., Hansson, H. C., Hämeri, K., Svenningsson, B., Massling, A., McFiggans, G., McMurry, P. H., Petäjä, T., Tunved, P., Gysel, M., Topping, D., Weingartner, E., Baltensperger, U., Rissler, J., Wiedensohler, A., and Kulmala, M.: Hygroscopic properties of submicrometer atmospheric aerosol particles measured with H-TDMA instruments in various environments – a review, Tellus B, 60, 432–469, https://doi.org/10.1111/j.1600-0889.2008.00350.x, 2008.
Tan, H., Xu, H., Wan, Q., Li, F., Deng, X., Chan, P. W., Xia, D., and Yin, Y.: Design and application of an unattended multifunctional H-TDMA system, J. Atmos. Ocean. Tech., 30, 1136–1148, https://doi.org/10.1175/JTECH-D-12-00129.1, 2013.
Tritscher, T., Jurányi, Z., Martin, M., Chirico, R., Gysel, M., Heringa, M. F., DeCarlo, P. F., Sierau, B., Prévôt, A. S. H., Weingartner, E., and Baltensperger, U.: Changes of hygroscopicity and morphology during ageing of diesel soot, Environ. Res. Lett., 6, 034026, https://doi.org/10.1088/1748-9326/6/3/034026, 2011.
Twomey, S.: Pollution and the planetary albedo, Atmos. Environ., 8, 1251–1256, https://doi.org/10.1016/0004-6981(74)90004-3, 1974.
Ulbrich, I. M., Canagaratna, M. R., Zhang, Q., Worsnop, D. R., and Jimenez, J. L.: Interpretation of organic components from Positive Matrix Factorization of aerosol mass spectrometric data, Atmos. Chem. Phys., 9, 2891–2918, https://doi.org/10.5194/acp-9-2891-2009, 2009.
Wang, L. T., Wei, Z., Yang, J., Zhang, Y., Zhang, F. F., Su, J., Meng, C. C., and Zhang, Q.: The 2013 severe haze over southern Hebei, China: model evaluation, source apportionment, and policy implications, Atmos. Chem. Phys., 14, 3151–3173, https://doi.org/10.5194/acp-14-3151-2014, 2014.
Wang, Y., Zhang, F., Li, Z., Tan, H., Xu, H., Ren, J., Zhao, J., Du, W., and Sun, Y.: Enhanced hydrophobicity and volatility of submicron aerosols under severe emission control conditions in Beijing, Atmos. Chem. Phys., 17, 5239–5251, https://doi.org/10.5194/acp-17-5239-2017, 2017.
Wang, Z., Wu, Z., Yue, D., Shang, D., Guo, S., Sun, J., Ding, A., Wang, L., Jiang, J., Guo, H., Cheung, H. C., Morawska, L., Keywoodm, M., and Hu, M.: New particle formation in China: current knowledge and further directions, Sci. Total Environ., 577, 258–266, https://doi.org/10.1016/j.scitotenv.2016.10.177, 2017.
Wu, Z. J., Zheng, J., Shang, D. J., Du, Z. F., Wu, Y. S., Zeng, L. M., Wiedensohler, A., and Hu, M.: Particle hygroscopicity and its link to chemical composition in the urban atmosphere of Beijing, China, during summertime, Atmos. Chem. Phys., 16, 1123-138, https://doi.org/10.5194/acp-16-1123-2016, 2016.
Ye, X., Tang, C., Yin, Z., Chen, J., Ma, Z., Kong, L., Yang, X., Gao, W., and Geng, F.: Hygroscopic growth of urban aerosol particles during the 2009 Mirage-Shanghai Campaign, Atmos. Environ., 64, 263–269, https://doi.org/10.1016/j.atmosenv.2012.09.064, 2013.
Zhang, F., Li, Y., Li, Z., Sun, L., Li, R., Zhao, C., Wang, P., Sun, Y., Liu, X., Li, J., Li, P., Ren, G., and Fan, T.: Aerosol hygroscopicity and cloud condensation nuclei activity during the AC3Exp campaign: implications for cloud condensation nuclei parameterization, Atmos. Chem. Phys., 14, 13423–13437, https://doi.org/10.5194/acp-14-13423-2014, 2014.
Zhang, F., Li, Z., Li, Y., Sun, Y., Wang, Z., Li, P., Sun, L., Wang, P., Cribb, M., Zhao, C., Fan, T., Yang, X., and Wang, Q.: Impacts of organic aerosols and its oxidation level on CCN activity from measurement at a suburban site in China, Atmos. Chem. Phys., 16, 5413–5425, https://doi.org/10.5194/acp-16-5413-2016, 2016.
Zhang, F., Wang, Y., Peng, J., Ren, J., Collins, D., Zhang, R., Sun, Y., Yang, X., and Li, Z.: Uncertainty in predicting CCN activity of aged and primary aerosols, J. Geophys. Res.-Atmos., 122, 11723–11736, https://doi.org/10.1002/2017JD027058, 2017.
Zhang, R., Khalizov, A. F., Pagels, J., Zhang, D., Xue, H., and McMurry, P. H.: Variability in morphology, hygroscopicity, and optical properties of soot aerosols during atmospheric processing, P. Natl. Acad. Sci. USA, 105, 10291–10296, https://doi.org/10.1073/pnas.0804860105, 2008.
Zhang, S. L., Ma, N., Kecorius, S., Wang, P. C., Hu, M., Wang, Z. B., Größ, J., Wu, Z. J., and Wiedensohler, A.: Mixing state of atmospheric particles over the North China Plain, Atmos. Environ., 125, Part A, 152–164, https://doi.org/10.1016/j.atmosenv.2015.10.053, 2016.
Zhang, Y., Du, W., Wang, Y., Wang, Q., Wang, H., Zheng, H., Zhang, F., Shi, H., Bian, Y., Han, Y., Fu, P., Canonaco, F., Prévôt, A. S. H., Zhu, T., Wang, P., Li, Z., and Sun, Y.: Aerosol chemistry and particle growth events at an urban downwind site in the North China Plain, Atmos. Chem. Phys. Discuss., https://doi.org/10.5194/acp-2017-889, in review, 2018.
Zhu, Y., Zhang, J., Wang, J., Chen, W., Han, Y., Ye, C., Li, Y., Liu, J., Zeng, L., Wu, Y., Wang, X., Wang, W., Chen, J., and Zhu, T.: Distribution and sources of air pollutants in the North China Plain based on on-road mobile measurements, Atmos. Chem. Phys., 16, 12551–12565, https://doi.org/10.5194/acp-16-12551-2016, 2016.