the Creative Commons Attribution 4.0 License.
the Creative Commons Attribution 4.0 License.
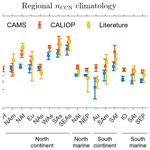
Pristine oceans are a significant source of uncertainty in quantifying global cloud condensation nuclei
Goutam Choudhury
Karoline Block
Mahnoosh Haghighatnasab
Johannes Quaas
Tom Goren
Matthias Tesche
Quantifying global cloud condensation nuclei (CCN) concentrations is crucial for reducing uncertainties in radiative forcing resulting from aerosol–cloud interactions. This study analyses two novel, independent, open-source global CCN datasets derived from spaceborne Cloud Aerosol Lidar with Orthogonal Polarization (CALIOP) measurements and Copernicus Atmosphere Monitoring Service (CAMS) reanalysis and examines the spatio-temporal variability of CCN concentrations pertinent to liquid clouds. The results reveal consistent large-scale patterns in both CALIOP and CAMS datasets, although CALIOP values are approximately 79 % higher than those from CAMS. Comparisons with the existing literature demonstrate that these datasets effectively bound regionally observed CCN concentrations, with CALIOP typically representing the upper bound and CAMS the lower bound. Monthly and annual variations in CCN concentrations obtained from the two datasets largely agree over the Northern Hemisphere and align with previously reported variations. However, inconsistencies emerge over pristine oceans, particularly in the Southern Hemisphere, where the datasets show not only opposing seasonal changes but also contrasting annual trends. Seasonal cycles in these regions are well represented in CAMS, consistent with previous in situ observations, while annual trends seems to be better captured by CALIOP. A comparative study of trends in CCN and cloud droplet concentrations suggests that dust-influenced and pristine maritime environments are primary regions that limit our current understanding of CCN–cloud droplet relationships. Long-term CCN observations in these regions are crucial for improving global datasets and advancing our understanding of aerosol–cloud interactions.
- Article
(5408 KB) - Full-text XML
-
Supplement
(2501 KB) - BibTeX
- EndNote
Aerosols act as cloud condensation nuclei (CCN) and through aerosol–cloud interactions (ACIs) induce a cooling effect on the climate, partially offsetting the warming due to greenhouse gases (Forster et al., 2021). The effective radiative forcing due to ACIs (ERFACI) is however highly uncertain, estimated to range between −1.7 and −0.3 W m−2 with moderate confidence (Forster et al., 2021).
A fundamental parameter for constraining ERFACI is the number concentration of CCN-forming aerosols (nCCN). Satellite-based studies of ERFACI rely on aerosol optical properties as proxies for nCCN. Part of the uncertainty in ERFACI arises from variations in estimates between different observation-based reports, particularly due to their choice of nCCN proxy (Forster et al., 2021; Gryspeerdt et al., 2017). The most common proxies are aerosol optical depth (AOD) and aerosol index (AI) (Quaas et al., 2020; Rosenfeld et al., 2023). AOD, being a column-integrated bulk property, poorly represents nCCN at cloud level. AI, calculated from AOD and the Ångström exponent, gives more weight to fine particles and offers an improvement over AOD. Using AI over AOD strengthens the negative radiative forcing by at least 30 % (Gryspeerdt et al., 2017). Nevertheless, because AI is derived from AOD, it inherits the limitations of AOD (Quaas et al., 2020; Rosenfeld et al., 2023). Incorporating additional polarimetric measurements enables retrievals of atmospheric-column-integrated aerosol number concentrations over oceans, which have been shown to yield significantly stronger negative forcing compared to AOD and AI (Hasekamp et al., 2019). Despite being a significant improvement over optical proxies, these concentrations are still column-integrated and may not represent the cloud-level values most relevant to ACIs. These studies illustrate that ERFACI significantly varies with the choice of nCCN proxy and highlight the critical need for a comprehensive, height-resolved global nCCN dataset as the next essential step for advancing ERFACI estimates.
Two recent efforts have addressed these limitations. Choudhury and Tesche (2023a) present a satellite-derived, vertically resolved, three-dimensional (3D) dataset of global nCCN. Their approach leverages the Cloud Aerosol Lidar with Orthogonal Polarization (CALIOP) retrievals and employs a validated CCN-retrieval algorithm (Choudhury and Tesche, 2022a) to retrieve nCCN from profiles of aerosol extinction coefficient. The retrieved nCCN values are then gridded onto a 2° by 5° latitude–longitude grid with a vertical resolution of 60 m to produce a monthly global nCCN dataset. The robustness of the retrieval algorithm is established through comparisons with in situ measurements from various land- and ocean-based platforms (Choudhury et al., 2022; Choudhury and Tesche, 2022b; Aravindhavel et al., 2023).
Complementing this effort, Block et al. (2024) present a 3D global nCCN dataset estimated from the Copernicus Atmosphere Monitoring Service (CAMS) aerosol reanalysis (Inness et al., 2019a). This dataset is based on a diagnostic box model built on a simplified κ-Köhler framework that estimates nCCN from CAMS-derived aerosol mass mixing ratios. It offers a high temporal resolution of 1 d, a horizontal resolution of 0.75°, and a hybrid sigma–pressure vertical grid with 60 levels. While the validation of this dataset is ongoing, a preliminary comparison to surface-based in situ observations gives promising results (Block et al., 2024).
The CAMS nCCN dataset with its high spatio-temporal resolution has great potential for better constraining ERFACI. However, its dependency on satellite-derived AOD (assimilated into CAMS) and the reliance on modelled aerosol inventories in its simulated component (Inness et al., 2019a) necessitate an extensive evaluation to assess the representativeness of this dataset. The CALIOP data's coarse monthly resolution complicates a direct integration into ERFACI estimation. Nevertheless, it was found to be representative of in situ-measured long-term variations in nCCN at multiple regional continental sites (Choudhury and Tesche, 2022b). Thus, the CALIOP nCCN dataset, currently the only satellite-based 3D global data available, presents a valuable tool for expanding the assessment of the CAMS dataset to a global scale, particularly in regions with limited in situ observations.
Here, we conduct a comparative study between the two independent novel nCCN datasets for a maximum supersaturation of 0.2 %, reconciling not only their variability across diverse spatio-temporal scales but also their co-variability with relevant cloud properties, such as cloud droplet number concentration (Nd). Furthermore, we augment their validation by comparing their regional concentrations with in situ measurements from the literature. The comparative analysis bridges the gap between the global datasets, providing insights for their future application and development. Ultimately, this work aims to establish a benchmark for applying and developing CCN-retrieval algorithms in the context of aerosol–cloud interactions.
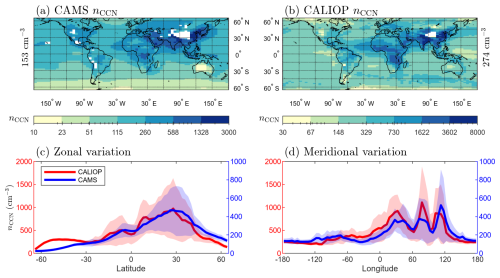
Figure 1Global climatology of cloud condensation nuclei (CCN) concentration (nCCN) at altitudes below 2 km. (a) Global climatology derived from CAMS reanalysis. (b) Global climatology derived from CALIOP spaceborne lidar. Median nCCN values are displayed on the lateral edges of panels (a) and (b). (c) Zonal variations of nCCN climatology. (d) Meridional variations of nCCN climatology. The semi-transparent patch in (c) and (d) represents 1 standard deviation. Note the different colour scales in the top row and the varying right and left y-axis limits in the bottom row. CALIOP and CAMS data from June 2006 through December 2021 are used to generate the climatology.
2.1 nCCN climatology in CAMS and CALIOP
We first compare the spatial variations in nCCN climatology for altitudes relevant to liquid clouds (< 2 km) in CALIOP and CAMS datasets (Fig. 1). CAMS nCCN ranges primarily between 28 and 619 cm−3 (5th and 95th percentiles), with a global median of 153 cm−3 (Fig. 1a). In contrast, CALIOP retrievals exhibit a broader range, varying from 107 to 1445 cm−3, with a global median of 274 cm−3 (Fig. 1b). Overall, CALIOP-derived nCCN values are approximately 79 % higher than those from CAMS. This difference is also reflected in the magnitudes of their zonal and meridional variations (Fig. 1c and d). Despite the discrepancies in magnitudes, the zonal and meridional patterns in both datasets are quite similar, with identical peaks and troughs across most regions except in the Southern Hemisphere (SH). The difference in the SH primarily originates from the retrievals over oceans, where CALIOP-derived concentrations are significantly higher than those from CAMS (by 208 %). This difference is particularly large for latitudes south of 45° S, where the median CAMS nCCN (33 cm−3) is roughly 7 times lower than that from CALIOP (263 cm−3).
Both datasets show higher nCCN in the Northern Hemisphere (NH) compared to the SH. However, this contrast is significantly stronger in CAMS (160 %) compared to CALIOP (20 %). This hemispheric difference in CAMS is particularly pronounced over oceans (121 %) compared to land (59%) and far exceeds the contrast observed in CALIOP (18 % over land and 10 % over oceans). Interestingly, the hemispheric contrast persists in CAMS, even over pristine oceans far from continental influence, where CALIOP exhibits homogeneous concentrations. Heterogeneity in CALIOP's oceanic nCCN is primarily confined to transatlantic dust transport in the tropics and the extra-tropical SH region of strong westerly winds. Since dust is not considered CCN-active in CAMS, the nCCN peak over the tropical Atlantic Ocean observed in CALIOP is less pronounced in CAMS. Furthermore, the CCN belt in the Southern Ocean (SO), though visible particularly in sea salt nCCN in CAMS (see Fig. S1 in the Supplement), does not appear in the total nCCN climatology due to low sea salt concentrations. When comparing the contrast between land and ocean nCCN, we find similar values for CAMS and CALIOP in the NH, with land values 65 % and 86 % higher than those over oceans, respectively. However, this difference in the SH is more pronounced in CAMS (130 %) than in CALIOP (73 %) due to substantially lower concentrations in CAMS over SH oceans. Refer to Table A1 for the median values used in these calculations.
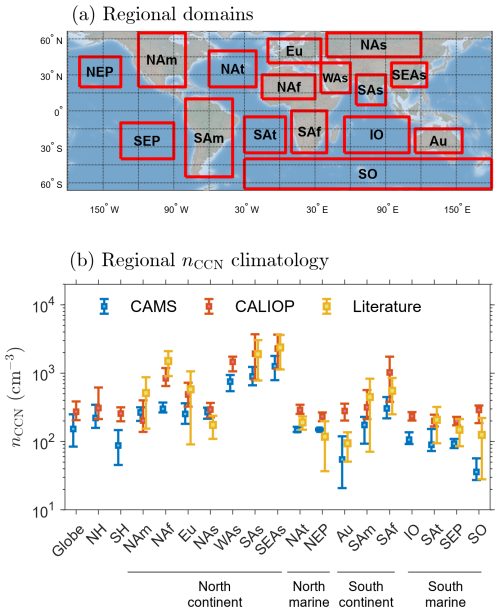
Figure 2Comparison of regional cloud condensation nuclei (CCN) concentrations nCCN with in situ measurements. (a) Geographical extent of regional domains considered in this study. (b) Comparison of median nCCN for various domains derived from CAMS reanalysis (blue), CALIOP (red), and in situ observations from the literature (yellow). Error bars for CAMS and CALIOP represent the geographic interquartile range of nCCN. Error bars for in situ observations represent the temporal nCCN variations at the specific measurement locations (refer to Table A1). CALIOP and CAMS data from June 2006 through December 2021 are used to produce the regional climatology. NH: Northern Hemisphere; SH: Southern Hemisphere; NAm: North America; NAf: Northern Africa; Eu: Europe; NAs: North Asia; WAs: West Asia; SAs: South Asia; SEAs: Southeast Asia; NAt: North Atlantic; NEP: Northeast Pacific; Au: Australia; SAm: South America; SAf: Southern Africa; IO: Indian Ocean; SAt: South Atlantic; SEP: Southeast Pacific.
2.1.1 Regional consistency with in situ observations
To evaluate the datasets, we compare the nCCN climatology from the global datasets with in situ observations (from the literature; refer to Table A1) for 16 regional domains encompassing major continents and ocean basins (geographical boundaries provided in Fig. 2a). Among all, Asia exhibits the highest overall nCCN (Fig. 2b), within which Southeast Asia shows the highest concentration, followed by South Asia and West Asia, consistently across CAMS, CALIOP, and in situ retrievals. Other continental and oceanic domains follow in decreasing order. Both datasets indicate cleaner SH oceanic regions (Southeast Pacific, South Atlantic, Indian Ocean, and Southern Ocean) compared to the NH oceans (Northeast Pacific and North Atlantic). However, this hemispheric order is opposite in the in situ measurements, where concentrations in the SH Atlantic and Pacific oceans exceed their respective NH counterparts. It is important to consider that while the regional domains over oceans in this study extend tens of degrees of longitude away from the coast, in situ observations for ocean environments may be limited in space (close to the coast) and time. For instance, the observations over the Southern Ocean (Humphries et al., 2023) are mostly obtained during the austral summer.
When comparing the magnitudes of nCCN, we observe that CALIOP-derived concentrations are consistently higher than those of CAMS across all regions except North America. These elevated values in the CALIOP data are expected because the retrieval in CALIOP assumes a fixed CCN activation radius, above which all aerosols are considered CCN-active regardless of their hygroscopicity. This assumption can lead to overestimation of nCCN in urban continental regions (Southeast and South Asia and Southern Africa) influenced by black carbon and regions downwind. CAMS, on the other hand, considers 80 % of black carbon aerosols to be hydrophobic (and thus not contributing to nCCN) (Block et al., 2024). Additionally, CAMS excludes dust as a potential CCN source, which is accounted for in CALIOP. These differences in the assumptions in CALIOP and CAMS in terms of aerosol hygroscopicity, activation size, and CCN activity may naturally lead to higher concentrations in CALIOP compared to CAMS. Other factors may also contribute to these differences. For example, CALIOP’s aerosol extinction coefficient may not correlate well with nCCN in complex aerosol mixtures with varying hygroscopicity (Choudhury and Tesche, 2022a). Additionally, inaccuracies in the representation of aerosol sources and sinks in CAMS may bias the derived nCCN (Moore et al., 2013). More details on the inherent differences between the global datasets are discussed in Sect. A1. Despite these discrepancies, this regional comparison with in situ measurements suggests that the global datasets adequately capture the observed variations in nCCN climatology for most regions. CALIOP appears to represent the upper bound, while CAMS represents the lower bound of nCCN, highlighting their potential for constraining nCCN, even in regions lacking in situ measurements.
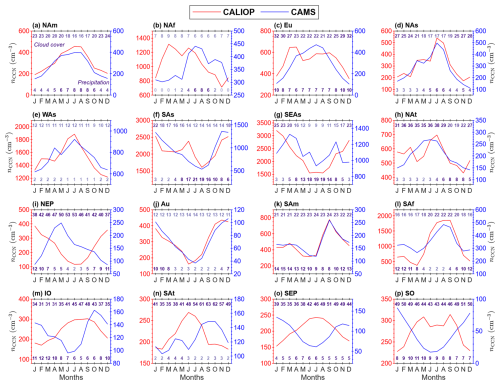
Figure 3Monthly variations in the cloud condensation nuclei concentration (nCCN) for various regions. Red lines represent nCCN derived from spaceborne CALIOP, and blue lines represent nCCN from CAMS reanalysis. Panels (a) to (i) correspond to Northern Hemisphere regions, while panels (j) to (p) represent Southern Hemisphere regions. Note the separate y axes for CALIOP (left) and CAMS (right). The numbers at the top and bottom of each panel represent the monthly climatology of low cloud cover (in %) from CERES and precipitation (in cm) from the GPCP product, respectively, with the opacity of the numbers proportional to their magnitude. Datasets from June 2006 through December 2021 are used to generate the monthly climatology.
2.2 Monthly nCCN variations
To understand how well the datasets capture the seasonal nCCN cycles, we analyse the average monthly variations in nCCN derived from CALIOP and CAMS for different regional domains (see Fig. 3). Both datasets exhibit a consistent pattern for most continental regions, with nCCN peaking in summer (boreal in the NH and austral in the SH) and reaching a minimum in winter. This pattern aligns with regional precipitation cycles (shown at the bottom of all panels of Fig. 3), where wet winters lead to precipitation scavenging of airborne particles, resulting in lower nCCN compared to dry summers. Exceptions include the monsoon-influenced South and Southeast Asia regions, which experience a summer minimum and winter maximum in nCCN due to prolonged summer rainfall. Both datasets adequately capture this seasonal pattern driven by the monsoon cycle.
However, the datasets show contrasting variations for all oceanic regions, except for the North Atlantic region. CALIOP exhibits a summer minimum and winter maximum in oceanic nCCN, while CAMS generally shows a spring–summer maximum and winter minimum. The variations in CALIOP align with the seasonal cycle of near-surface wind speeds over oceans (Yu et al., 2020). Higher wind speeds increase sea spray aerosol concentrations in marine environments by enhancing wave breaking and bubble bursting (Revell et al., 2019; Humphries et al., 2023), which may contribute to the observed CCN cycles in CALIOP. However, oceanic nCCN values are also influenced by factors beyond sea spray aerosols, such as biogenic emissions, which follow a seasonal pattern of summer maximum and winter minimum (Lana et al., 2011; Revell et al., 2019), more in line with CAMS. Studies in pristine oceans have shown that while sea salt aerosols primarily contribute to aerosol mass, sulfates from biogenic emissions dominate particle or CCN concentrations (Ayers and Gras, 1991; Gras and Keywood, 2017; Humphries et al., 2023). Consequently, in situ-derived nCCN variations in these regions closely follow biogenic emission patterns (Gras, 1990; Ayers and Gras, 1991; Gras and Keywood, 2017), exhibiting a spring–summer maximum and winter minimum. As a result, cloud droplet number concentration (Nd), a parameter sensitive to changes in nCCN, also displays a spring–summer maximum and winter minimum in the pristine SH oceans (McCoy et al., 2015; Mace and Avey, 2017; see also Fig. S2). These seasonal CCN cycles are well represented in CAMS but not in CALIOP. Additionally, the austral summer concentrations in CAMS for the Southern Ocean (Fig. 3p) are comparable to the in situ observations reported by Humphries et al. (2023), which were mostly obtained during the austral summer. This observation contrasts with the results inferred from climatological concentrations in Fig. 2, where CALIOP misleadingly appears to show better agreement.
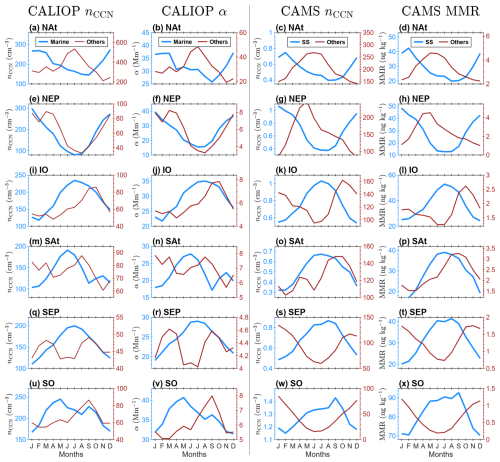
Figure 4Monthly variations in cloud condensation nuclei concentration (nCCN), extinction coefficient (α), and mass mixing ratio (MMR) for six oceanic domains. Blue lines represent marine aerosols in CALIOP and sea salt aerosols in CAMS, while brown lines represent contributions from other aerosol species. Panels in the first and second column depict the marine and non-marine nCCN and α derived from CALIOP, respectively. The third and fourth column show the sea salt and non-sea-salt nCCN and MMR derived from CAMS, respectively. Datasets from June 2006 through December 2021 are used to generate the monthly climatology.
Further investigation reveals that while the total nCCN seasonal cycles in most oceanic regions are opposite in CALIOP and CAMS, the marine nCCN in CALIOP aligns closely with CAMS's sea salt nCCN, with both exhibiting a summer minimum and winter maximum (first and third columns in Fig. 4). This similarity can be attributed to the similar seasonal cycles of CALIOP's marine extinction coefficients (αM; second column in Fig. 4) and CAMS's sea salt mass mixing ratio (MMRSS; fourth column in Fig. 4), the primary parameters from which their respective nCCN values are calculated (Choudhury and Tesche, 2022a; Block et al., 2024). Since aerosol mass in pristine oceans consists primarily of coarse-mode sea salt particles (Humphries et al., 2023; fourth column in Fig. A1), αM is expected to be proportional to MMRSS, as these coarse particles dominate light scattering. nCCN in CALIOP's retrieval algorithm is proportional to aerosol extinction coefficient (Shinozuka et al., 2015; Choudhury and Tesche, 2022a), so the seasonal nCCN cycles in CALIOP for pristine oceans follow the variations in sea salt aerosols. Given that sulfates are the primary contributors to nCCN in these regions (Ayers and Gras, 1991; Gras and Keywood, 2017; Humphries et al., 2023), the separation of marine extinction coefficients in CALIOP into contributions from sea salt and biogenic aerosols is crucial for accurately representing nCCN cycles over pristine oceans. This separation, however, requires precise quantification of their lidar ratios and depolarization properties (Tesche et al., 2009), which is currently lacking. On the other hand, CAMS, which can distinguish between different oceanic aerosol species such as sulfates, hydrophilic organic matter, and sea salt, better captures the overall nCCN variations in pristine marine environments.
Nevertheless, CAMS may significantly underestimate the contribution of sea salt aerosols to oceanic nCCN (third column of Figs. 4 and A1), which can be as high as 8 %–51 % of the total nCCN and may increase to 100 % at higher surface wind speeds (Fossum et al., 2018). This underestimation could stem from an underrepresentation of small-mode sea salt aerosol mass in CAMS (see fourth column of Fig. A1). Another plausible reason may be the size distribution assumed in CAMS's CCN-retrieval algorithm, which may not accurately represent small-mode sea salt aerosols. Such factors likely contribute to the observed low nCCN values in CAMS compared to in situ observations for SH oceanic domains (see Fig. 2b). Additionally, the inaccurate representation of CCN generated from new particle formation processes (McCoy et al., 2021; Mace et al., 2023, 2024) may further contribute to the underestimation of CCN in CAMS. However, due to limited in situ observations across different regions in the SH oceans, the contribution of these aerosol species to total oceanic nCCN, as well as their seasonal variations across different oceanic regions, remains uncertain. It is important to note that SH oceans are the primary contributor to global low-level cloud cover (see top of all panels in Figs. 3 and A2b). These inconsistencies observed in the global nCCN datasets in such cloud-rich regions demand further improvements in the underlying CALIOP and CAMS datasets, as well as in the associated CCN-retrieval algorithms, to better constrain aerosol–cloud interactions.
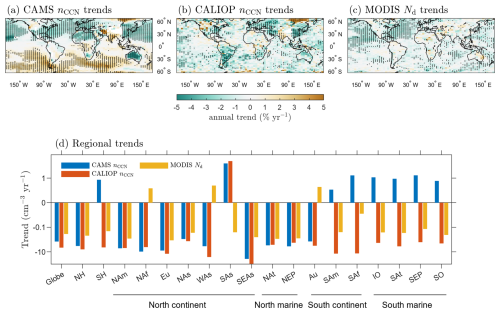
Figure 5Comparison of global and regional trends computed using annual time series. (a) Trends in the cloud condensation nuclei concentration (nCCN) derived from CAMS reanalysis. (b) Trends in nCCN from CALIOP. (c) Trends in cloud droplet number concentration (Nd) derived from MODIS. (d) Regional trends in nCCN derived from CAMS reanalysis (blue), nCCN from CALIOP (red), and Nd from MODIS (yellow) are compared. Dots in panels (a)–(c) indicate the grids where the trend is statistically significant. The absolute values of the trends in panels (a)–(c) are shown in Fig. S3. Trends in nCCN from CALIOP and CAMS are produced using data from 2007 to 2021, while data from 2007 to 2020 are used for Nd. Annual time series for the regional domains are provided in Fig. S4.
2.3 Reconciling trends in nCCN and Nd
Quantifying trends in nCCN is crucial for comprehending the present dynamics of radiative forcing due to ACIs and for projecting future changes. Recent decades have witnessed declining aerosol emission rates and aerosol loadings over land (Collaud Coen et al., 2020; Quaas et al., 2022) and oceans (IMO, 2019; Gryspeerdt et al., 2019) due to stricter emission policies. An exception is the South Asia region, where aerosol emissions have been increasing in the 21st century (Jin et al., 2023). These emission trends are also expected to be reflected in Nd because of their strong sensitivity to changes in nCCN (McCoy et al., 2018; Quaas et al., 2022). Therefore, we expect the annual trends in nCCN and Nd to be similar to the emission trends.
Over NH regions, the emission trends are reflected in both the nCCN datasets (Fig. 5a and b). As expected, all regions except South Asia exhibit a declining nCCN trend (see Fig. 5c and Table A1). The trends in Nd are also consistent with those in nCCN from both CALIOP and CAMS (Fig. 5c), with exceptions only observed over dust-influenced regions (Northern Africa and West Asia). This discrepancy may be attributed to the hydrophobic nature of fresh mineral dust, which may not readily act as CCN due to a lack of mixing or coating with water-soluble aerosols (Garimella et al., 2014).
Over SH regions, CALIOP shows declining nCCN trends across all domains. Nd trends are mostly negative as well consistent with CALIOP, except for dust-influenced Australia (Au) domain. Of particular interest are the spatially uniform and statistically significant increasing trends in CAMS-derived nCCN at altitudes below 2 km over most SH oceanic regions. This finding not only contradicts the negative trend observed in Nd and CALIOP-derived nCCN but also the expected decreasing trend inferred from previous ship emission reports (Quaas et al., 2022). The trend even exists in the mass mixing ratios in CAMS data (see Fig. S11), particularly corresponding to sulfate aerosol species. It is worth noting that the increasing SH nCCN trends in CAMS coincide with trends in AOD derived from MODIS (see Fig. A3). Since MODIS AOD is used to constrain the CAMS aerosol reanalysis (Inness et al., 2019a), a proportionality between AOD and CAMS-derived nCCN is inherent in homogeneous marine environments (Block et al., 2024) and may contribute to the observed increasing trends in CAMS. These inconsistencies over pristine oceans, where the trends in aerosol loadings differ between different spaceborne retrievals (Quaas et al., 2022), question the representativeness of the nCCN and Nd retrievals, making it challenging to derive their inter-relationship, a parameter key to quantifying ACIs.
The comparative study presented here shows good consistency between the independent CALIOP and CAMS global nCCN datasets in continental environments. However, significant discrepancies emerge over most pristine oceans, not only in nCCN climatology but also in their monthly and annual variations. While the seasonal cycles of oceanic nCCN derived from CAMS largely align with previous in situ observations (Gras, 1990; Ayers and Gras, 1991; Gras and Keywood, 2017; Humphries et al., 2023) and the variations in Nd, CAMS likely underestimates the contributions from sea salt and secondary biogenic nCCN. In contrast, the seasonal nCCN cycles in CALIOP are not representative, likely due to its inability to resolve marine nCCN into sea salt and sulfate (from biogenic emission) components.
The results, however, are completely opposite for annual trends in nCCN and Nd. While trends in CAMS and CALIOP generally agree across most NH regions, they diverge significantly in the SH. CALIOP consistently shows a declining nCCN trend in these regions, which aligns with previous reports (IMO, 2019; Gryspeerdt et al., 2019; Quaas et al., 2022) and the decreasing trend in Nd, while CAMS exhibits an anomalous increasing nCCN trend over SH oceans. This geographically limited disagreement, restricted to pristine oceans with limited in situ measurements, raises questions about the adequacy of aerosol inventories used by CAMS in SH oceans, a known issue in climate models (Moore et al., 2013). These discrepancies in cloud-rich pristine oceans are particularly concerning because cloud properties in these regions are highly sensitive to even small perturbations in aerosol concentrations (Moore et al., 2013; Gryspeerdt et al., 2023).
Caution should therefore be taken when using these nCCN datasets in the pristine oceans of the SH. Future research efforts should focus on first separating the sea salt and biogenic components of marine aerosols in CALIOP and, second, on accurately quantifying the sources and sinks of CCN and their long-term cycles in remote SH oceans for improving the representativeness of aerosol inventories in CAMS. An alternative approach could involve the further development of advanced data-driven techniques to derive global CCN dataset (Redemann and Gao, 2024). These efforts are crucial to refine the global nCCN datasets and ultimately to reduce the uncertainties in ERFACI. In conclusion, the aerosol-limited environments of SH oceans are identified as a significant source of uncertainty in the present effort to quantify a highly resolved global nCCN dataset.
A1 Global nCCN datasets
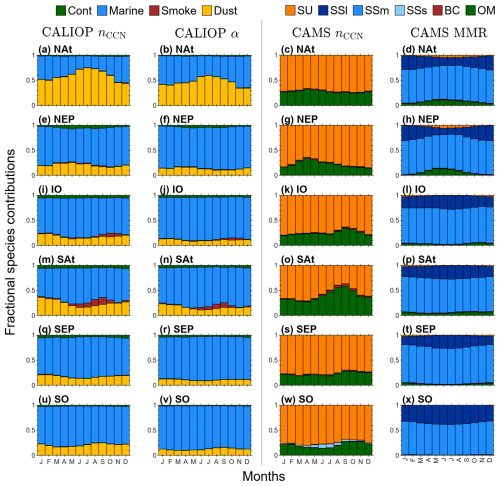
Figure A1Monthly variations in the fractional contributions of different aerosol species to cloud condensation nuclei concentration (nCCN), aerosol extinction coefficient (α), and mass mixing ratio (MMR) for six oceanic domains. Panels in the first and second column depict the fractional nCCN and α contributions of different aerosol species (continental, marine, smoke, and dust) in CALIOP. The third and fourth column show the fractional nCCN and MMR contributions of different aerosol species in CAMS (sulfate (SU), sea salt large (SSl), sea salt medium (SSm), sea salt small (SSs), black carbon (BC), and organic matter (OM)). Datasets from June 2006 to December 2021 are used to generate the monthly climatology. Fractional contributions for other regional domains are given in Figs. S5–S8.
The CALIOP dataset provides nCCN at a supersaturation of 0.20 %. It is available on a uniform latitude–longitude grid of resolution 2° by 5°, a vertical grid resolution of 60 m extending from mean sea level to a height of 8 km above mean sea level, and a temporal resolution of 1 month. The dataset is derived from more than 15 years of the CALIOP level 2 aerosol profile product from June 2006 to December 2021 (NASA/LARC/SD/ASDC, 2018). It is based on a CCN-retrieval algorithm (Choudhury and Tesche, 2022a) that integrates the CALIOP-derived height-resolved information on the aerosol-type-specific extinction coefficient and microphysical properties from CALIOP's aerosol model with the optical modelling capabilities of the MOPSMAP (Modelled Optical Properties of enseMbles of Aerosol Particles; Gasteiger and Wiegner, 2018) package. Essentially, the algorithm adjusts the normalized size distributions within the aerosol model to match the extinction coefficient. These adjusted size distributions are then used to estimate particle number concentrations relevant for CCN activation. Aerosol-type-specific CCN parameterizations are then applied to calculate nCCN at a supersaturation of 0.20 % for continental (comprising of clean, polluted, and smoke aerosols), dust, and marine aerosols. The algorithm accounts for hygroscopic growth of hydrophilic aerosols (continental and marine aerosols) under humid conditions using the κ parameterization within the MOPSMAP package. Evaluations of the algorithm have demonstrated good agreement with independent ground-based and airborne in situ measurements across diverse geographic locations, with a combined normalized mean bias of ≈ 22 % and a normalized absolute error of ≈ 61 % (Choudhury et al., 2022; Choudhury and Tesche, 2022b, 2023a; Aravindhavel et al., 2023). The resulting CALIOP-derived nCCN has also been utilized in quantifying the CCN activation ratio for liquid clouds (Alexandri et al., 2024).
CAMS nCCN dataset (Block et al., 2024) is derived from CAMS aerosol reanalysis of mass mixing ratios (Inness et al., 2019b) and provides nCCN at supersaturations ranging from 0.1 % to 1 %. The nCCN dataset retains the native resolution of CAMS reanalysis data and is available on a uniform horizontal grid of resolution 0.75° by 0.75° and a vertical grid with 60 hybrid sigma–pressure levels extending from the surface to 0.1 hPa. The CCN-retrieval algorithm in CAMS utilizes a box-model framework (O'Connor et al., 2014; West et al., 2014) to convert the mass mixing ratios of five aerosol species – sulfate, mineral dust, black carbon (hydrophobic and hydrophilic), organic matter (hydrophobic and hydrophilic), and sea salt – into total number concentrations. Subsequently, these concentrations are combined with normalized size distributions derived from the aerosol module of the European Centre for Medium-Range Weather Forecasts (ECMWF) Integrated Forecasting System (IFS) model (Benedetti et al., 2009) to estimate the actual aerosol size distribution. The size distributions of hydrophillic aerosols are then coupled with auxiliary meteorological parameters and used in modified Kappa-Köhler theory (Pöhlker et al., 2023) to calculate the activated nCCN at various supersaturations. Consistent with the CAMS model's assumption of completely hydrophobic dust with no consideration of internal mixing or external coating mechanisms, dust is excluded in the CCN calculations. Initial validation results using surface in situ CCN observations at continental and coastal Atmospheric Radiation Measurement (ARM) network sites have shown promising results, with an acceptable bias factor of 1.29 (Block et al., 2024).
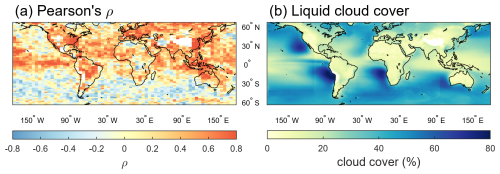
Figure A2Relating correlation between CALIOP and CAMS with global cloud cover. (a) Global map of Pearson’s correlation coefficient (ρ) between monthly mean cloud condensation nuclei concentration (nCCN) derived from spaceborne CALIOP and CAMS reanalysis datasets. (b) Low-level cloud cover climatology (in %) derived from CERES SYN product.
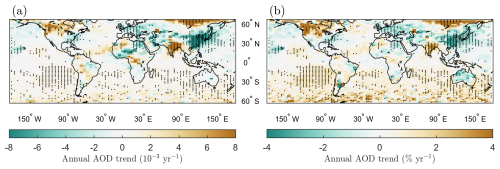
Figure A3Global map of annual trend in MODIS aerosol optical depth (AOD) derived using combined Dark Target and Deep Blue algorithms. Panel (a) shows the trend in 10−3 yr−1 and panel (b) in % yr−1. Dots in each panel indicate the grids where the trend is statistically significant. Data between 2007 and 2021 are used to estimate the trends.
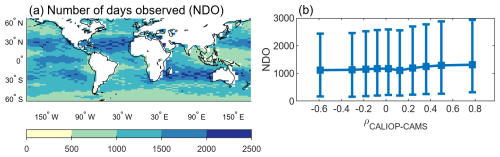
Figure A4Relationship between sampling frequency in CALIOP and correlation between the datasets. (a) Global map of number of days with a valid aerosol retrieval observed by CALIOP within period of June 2006 to December 2021. (b) Median number of valid CALIOP aerosol retrieval over oceans versus Pearson's correlation coefficient between CALIOP and CAMS (ρCALIOP−CAMS). Error bars denote the interquartile range. Each ρCALIOP−CAMS bin consists of 407 data points.
A1.1 Limitations of nCCN datasets
The CALIOP nCCN dataset is subject to uncertainties arising from errors in the underlying CALIOP products and approximations within the CCN-retrieval algorithm. Uncertainties in CALIOP extinction coefficients can reach 30 %. Assuming fixed aerosol-type-specific size distributions introduces additional uncertainty, estimated to be a factor of 1.5–2 (Choudhury and Tesche, 2022a). Further, the algorithm assumes an aerosol-species-dependent fixed CCN activation radius (50 nm for continental and marine aerosols and 100 nm for mineral dust at a supersaturation of 0.20 %). Using a fixed CCN activation size (assuming all larger particles are CCN active) may result in about a 20 % overestimation in the final CCN product (Choudhury and Tesche, 2022b). Accounting for all these limitations, the overall uncertainty associated with the CALIOP-derived CCN dataset is expected to be a factor of 2–3 (Choudhury and Tesche, 2023a). Moreover, the CALIOP dataset is produced using only cloud-free aerosol profiles. This can lead to sampling bias in regions with significant cloud cover, potentially leading to the differences observed between the CALIOP and CAMS datasets. However, there appears to be no clear relationship between the correlation of the CALIOP and CAMS nCCN datasets and the sampling frequency of CALIOP (Fig. A4).
Shen et al. (2019)Désalmand (1987)Paramonov et al. (2015)Asmi et al. (2016)Jayachandran et al. (2020)Shen et al. (2019)Wood et al. (2017)Brendecke et al. (2022)Humphries et al. (2023)Shen et al. (2019)Ross et al. (2003)Redemann et al. (2021)Allen et al. (2011)Humphries et al. (2023)Table A1Median cloud condensation nuclei (CCN) concentration (nCCN) at a supersaturation of 0.20 % in cm−3 with the interquartile range in parentheses and annual nCCN trend in cm−3 yr−1 for various regions. Trends in bold indicate statistically significant trends (p<0.05). In situ nCCN observations and their corresponding references are also provided. Abbreviations are explained in the footnote.
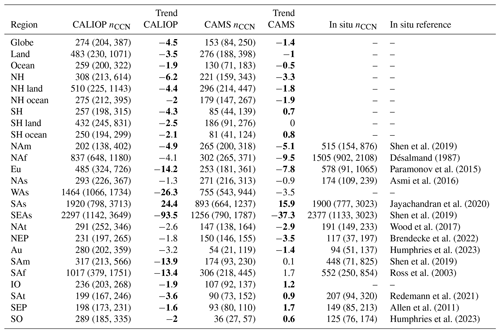
NAm: North America (20–65° N, 120–80° W); NAf: Northern Africa (10–30° N, 15° W–30° E); Eu: Europe (40–60° N, 10° W–35° E); NAs: North Asia (45–65° N, 40–120° E); WAs: West Asia (15–40° N, 35–60° E); SAs: South Asia (5–30° N, 65–90° E); SEAs: Southeast Asia (20–40° N, 95–125° E); NAt: North Atlantic (10–35° N, 60–20° W); NEP: Northeast Pacific (20–45° N, 170–135° W); Au: Australia (35–15° S, 115–155° E); SAm: South America (55–10° S, 80–40° W); SAf: Southern Africa (35–0° S, 10–40° E); IO: Indian Ocean (35–5° S, 55–110° E); SAt: South Atlantic (35–5° S, 30° W–5° E); SEP: Southeast Pacific (40–10° S, 135–90° W); SO: Southern Ocean (65–40° S, 30° W–180° E).
Similarly, uncertainties in the CAMS nCCN dataset may stem from the source CAMS aerosol reanalysis product and the CCN-estimation methodology. The CAMS aerosol product is constrained by satellite-derived AOD retrievals, particularly the MODIS Dark Target and Deep Blue AOD retrievals at 0.55 µm (Platnick et al., 2017b) and Advanced Along-Track Scanning Radiometer (AATSR)-retrieved AOD (Popp et al., 2016). Therefore, uncertainties in AOD retrievals can propagate into the CAMS reanalysis and ultimately the nCCN product. Additionally, missing aerosol sources in the CAMS emission inventory (Moore et al., 2013; Errera et al., 2021) can introduce uncertainties, especially in remote areas with sparse observations, limiting the effectiveness of emission parameterizations implemented in the aerosol model. Furthermore, unlike the approach in CALIOP, the CAMS-based retrieval excludes mineral dust. Studies have demonstrated that mineral dust may be a potential CCN source, particularly when coated or internally mixed with water-soluble hydrophilic aerosols (Kumar et al., 2009; Bègue et al., 2015). This exclusion may thus lead to an underestimation in the final nCCN product.
A2 Spaceborne cloud and precipitation data
Nd data for low-level liquid clouds are derived from the Moderate Resolution Imaging Spectroradiometer (MODIS) aboard the Aqua polar-orbiting satellite (Gryspeerdt et al., 2022). The dataset is available at a uniform spatial resolution of 1° by 1° with daily temporal resolution spanning July 2002–2020. Low-level cloud cover data are obtained from the Clouds and the Earth's Radiant Energy System (CERES) SYN Edition 4A monthly product (Doelling et al., 2013). This product merges retrievals from CERES, MODIS, and geostationary sensors to construct a global gridded dataset suitable for studying aerosol–cloud interactions. The dataset is operationally available at a latitude–longitude resolution of 1° by 1° starting from July 2002.
Precipitation data are derived from the Global Precipitation Climatology Project (GPCP) monthly product (Adler et al., 2003). This product integrates rainfall data obtained from several platforms, including satellites, in situ soundings, and rain gauges, to generate a global monthly precipitation dataset on a uniform horizontal resolution of 2.5° available from 1979.
A3 Data harmonization, trend estimation, and averaging methodologies
CCN, cloud, and precipitation parameters are considered between latitudes of 65° N and 65° S. Data at higher latitudes are not considered due to the uncertainties associated with MODIS observations at high solar zenith angles (Grosvenor and Wood, 2014; Grosvenor et al., 2018) and the lack of validation for CALIOP retrievals at these latitudes. Horizontal grids of all datasets are harmonized by transforming them to the coarser 2° by 5° latitude–longitude grid of CALIOP using bilinear interpolation. We exclude CAMS data in grids surrounding Mauna Loa and Altzomoni due to documented biases in CAMS aerosol emission datasets over these regions (Inness et al., 2019a).
To specifically focus on the liquid clouds, which are most relevant for aerosol–cloud interactions, average nCCN values between altitudes of 0–2 km are considered in this study. Additionally, a supersaturation of 0.20 % is selected because this value represents a characteristic supersaturation near the base of liquid clouds. Temporal averages of CALIOP data are weighted by the number of valid aerosol retrievals within each grid cell (Choudhury and Tesche, 2023a). Horizontal averages in CALIOP and CAMS are weighted by the area of the latitude–longitude grids. Trends in nCCN and Nd are estimated using the non-parametric Mann–Kendall trend test, as it does not require any assumptions about the distribution of the time series data and is more robust in handling outliers (Mann, 1945; Kendall, 1975). Annual trends computed using linear regression are shown in Fig. S4. Monthly and annual statistics are calculated using data between 2007 and 2021 for CALIOP- and CAMS-derived nCCN and between 2007 and 2020 for MODIS-derived Nd.
All datasets used in this work are open-source. The CALIPSO Level 2 Aerosol Profile product can be downloaded from https://doi.org/10.5067/CALIOP/CALIPSO/LID_L2_05KMAPRO-STANDARD-V4-20 (NASA/LARC/SD/ASDC, 2018). CALIOP CCN data can be accessed at https://doi.org/10.1594/PANGAEA.956215 (Choudhury and Tesche, 2023b). CAMS mass mixing ratios were acquired from the Copernicus Atmosphere Monitoring Service (CAMS) Atmosphere Data Store (ADS) (https://ads.atmosphere.copernicus.eu/datasets/cams-global-reanalysis-eac4-monthly?tab=overview, last access: 25 December 2024; Inness et al., 2019b). CAMS-derived CCN data can be downloaded from https://doi.org/10.26050/WDCC/QUAERERE_CCNCAMS_v1 (Block, 2023). The CERES SYN level 3 product can be downloaded from 10.5067/TERRA+AQUA/CERES/SYN1DE (NASA Langley Research Center, 2021). MODIS-derived cloud droplet number concentrations can be downloaded from https://doi.org/10.5285/864a46cc65054008857ee5bb772a2a2b (Gryspeerdt et al., 2022). The MODIS Aqua aerosol product (https://doi.org/10.5067/MODIS/MYD08_M3.061, Platnick et al., 2017a) is obtained from the Level-1 and Atmosphere Archive and Distribution System (LAADS) Distributed Active Archive Center (DAAC), located in the Goddard Space Flight Center in Greenbelt, Maryland (https://ladsweb.nascom.nasa.gov/, last access: 30 March 2025). Global Precipitation Climatology Project (GPCP) monthly precipitation data are available at https://doi.org/10.24381/cds.c14d9324 (Copernicus Climate Change Service, 2021).
The supplement related to this article is available online at https://doi.org/10.5194/acp-25-3841-2025-supplement.
MT conceptualized the initial research idea. GC processed the datasets, compiled the plots, and drafted the initial manuscript. KB, MH, and JQ assisted with the CAMS dataset. TG assisted with the cloud droplet dataset. All authors periodically contributed to the development of the research methodology and the revision of the manuscript.
At least one of the (co-)authors is a member of the editorial board of Atmospheric Chemistry and Physics. The peer-review process was guided by an independent editor, and the authors also have no other competing interests to declare.
Publisher’s note: Copernicus Publications remains neutral with regard to jurisdictional claims made in the text, published maps, institutional affiliations, or any other geographical representation in this paper. While Copernicus Publications makes every effort to include appropriate place names, the final responsibility lies with the authors.
The authors would like to acknowledge multiple research funding organizations for supporting this research. Goutam Choudhury was supported by the German Research Foundation (Deutsche Forschungsgemeinschaft, DFG; grant number 524386224). Tom Goren acknowledges funding from the Israel Science Foundation (grant number: 3171/24). Matthias Tesche and Goutam Choudhury (initially) were supported by the Franco-German Fellowship Program on Climate, Energy, and Earth System Research (Make Our Planet Great Again–German Research Initiative (MOPGA-GRI), grant number 57429422) of the German Academic Exchange Service (DAAD), funded by the German Ministry of Education and Research. Matthias Tesche has been supported by the Federal State of Saxony and the European Social Fund Plus (ESF; grant number 100649813). Karoline Block and Johannes Quaas received funding from the German Federal Ministry for Education and Research (BMBF) project “WarmWorld” (FKZ 01LK2202G). Johannes Quaas and MH acknowledge funding by the DFG project “VolCloud” (GZ QU 311/23-2). Johannes Quaas further acknowledges the EU Horizon Europe project CleanCloud (project number 101137639).
This research has been supported by the European Social Fund Plus (ESF; grant no. 100649813), the Deutsche Forschungsgemeinschaft (grant no. 524386224), and the Israel Science Foundation (grant no. 3171/24).
This paper was edited by Timothy Garrett and reviewed by Gerald Mace and Marc Daniel Mallet.
Adler, R. F., Huffman, G. J., Chang, A., Ferraro, R., Xie, P.-P., Janowiak, J., Rudolf, B., Schneider, U., Curtis, S., Bolvin, D., Gruber, A., Susskind, J., Arkin, P., and Nelkin, E.: The Version-2 Global Precipitation Climatology Project (GPCP) Monthly Precipitation Analysis (1979–Present), J. Hydrometeorol., 4, 1147–1167, https://doi.org/10.1175/1525-7541(2003)004<1147:TVGPCP>2.0.CO;2, 2003. a
Alexandri, F., Müller, F., Choudhury, G., Achtert, P., Seelig, T., and Tesche, M.: A cloud-by-cloud approach for studying aerosol–cloud interaction in satellite observations, Atmos. Meas. Tech., 17, 1739–1757, https://doi.org/10.5194/amt-17-1739-2024, 2024. a
Allen, G., Coe, H., Clarke, A., Bretherton, C., Wood, R., Abel, S. J., Barrett, P., Brown, P., George, R., Freitag, S., McNaughton, C., Howell, S., Shank, L., Kapustin, V., Brekhovskikh, V., Kleinman, L., Lee, Y.-N., Springston, S., Toniazzo, T., Krejci, R., Fochesatto, J., Shaw, G., Krecl, P., Brooks, B., McMeeking, G., Bower, K. N., Williams, P. I., Crosier, J., Crawford, I., Connolly, P., Allan, J. D., Covert, D., Bandy, A. R., Russell, L. M., Trembath, J., Bart, M., McQuaid, J. B., Wang, J., and Chand, D.: South East Pacific atmospheric composition and variability sampled along 20° S during VOCALS-REx, Atmos. Chem. Phys., 11, 5237–5262, https://doi.org/10.5194/acp-11-5237-2011, 2011. a
Aravindhavel, A., Choudhury, G., Prabhakaran, T., Murugavel, P., and Tesche, M.: Retrieval and validation of cloud condensation nuclei from satellite and airborne measurements over the Indian Monsoon region, Atmos. Res., 290, 106802, https://doi.org/10.1016/j.atmosres.2023.106802, 2023. a, b
Asmi, E., Kondratyev, V., Brus, D., Laurila, T., Lihavainen, H., Backman, J., Vakkari, V., Aurela, M., Hatakka, J., Viisanen, Y., Uttal, T., Ivakhov, V., and Makshtas, A.: Aerosol size distribution seasonal characteristics measured in Tiksi, Russian Arctic, Atmos. Chem. Phys., 16, 1271–1287, https://doi.org/10.5194/acp-16-1271-2016, 2016. a
Ayers, G. P. and Gras, J. L.: Seasonal relationship between cloud condensation nuclei and aerosol methanesulphonate in marine air, Nature, 353, 834–835, https://doi.org/10.1038/353834a0, 1991. a, b, c, d
Bègue, N., Tulet, P., Pelon, J., Aouizerats, B., Berger, A., and Schwarzenboeck, A.: Aerosol processing and CCN formation of an intense Saharan dust plume during the EUCAARI 2008 campaign, Atmos. Chem. Phys., 15, 3497–3516, https://doi.org/10.5194/acp-15-3497-2015, 2015. a
Benedetti, A., Morcrette, J.-J., Boucher, O., Dethof, A., Engelen, R. J., Fisher, M., Flentje, H., Huneeus, N., Jones, L., Kaiser, J. W., Kinne, S., Mangold, A., Razinger, M., Simmons, A. J., and Suttie, M.: Aerosol analysis and forecast in the European Centre for Medium-Range Weather Forecasts Integrated Forecast System: 2. Data assimilation, J. Geophys. Res.-Atmos., 114, D13205, https://doi.org/10.1029/2008JD011115, 2009. a
Block, K.: Cloud condensation nuclei (CCN) numbers derived from CAMS reanalysis EAC4 (Version 1), World Data Center for Climate [data set], https://doi.org/10.26050/WDCC/QUAERERE_CCNCAMS_v1, 2023. a
Block, K., Haghighatnasab, M., Partridge, D. G., Stier, P., and Quaas, J.: Cloud condensation nuclei concentrations derived from the CAMS reanalysis, Earth Syst. Sci. Data, 16, 443–470, https://doi.org/10.5194/essd-16-443-2024, 2024. a, b, c, d, e, f, g
Brendecke, J., Dong, X., Xi, B., and Zheng, X.: Maritime Aerosol and CCN Profiles Derived From Ship-Based Measurements Over Eastern North Pacific During MAGIC, Earth Space Sci., 9, e2022EA002319, https://doi.org/10.1029/2022EA002319, 2022. a
Choudhury, G. and Tesche, M.: Estimating cloud condensation nuclei concentrations from CALIPSO lidar measurements, Atmos. Meas. Tech., 15, 639–654, https://doi.org/10.5194/amt-15-639-2022, 2022a. a, b, c, d, e, f
Choudhury, G. and Tesche, M.: Assessment of CALIOP-Derived CCN Concentrations by In Situ Surface Measurements, Remote Sens., 14, 3342, https://doi.org/10.3390/rs14143342, 2022b. a, b, c, d
Choudhury, G. and Tesche, M.: A first global height-resolved cloud condensation nuclei data set derived from spaceborne lidar measurements, Earth Syst. Sci. Data, 15, 3747–3760, https://doi.org/10.5194/essd-15-3747-2023, 2023a. a, b, c, d
Choudhury, G. and Tesche, M.: Global multiyear 3D dataset of cloud condensation nuclei derived from spaceborne lidar measurements, Pangaea [data set], https://doi.org/10.1594/PANGAEA.956215, 2023b. a
Choudhury, G., Ansmann, A., and Tesche, M.: Evaluation of aerosol number concentrations from CALIPSO with ATom airborne in situ measurements, Atmos. Chem. Phys., 22, 7143–7161, https://doi.org/10.5194/acp-22-7143-2022, 2022. a, b
Collaud Coen, M., Andrews, E., Alastuey, A., Arsov, T. P., Backman, J., Brem, B. T., Bukowiecki, N., Couret, C., Eleftheriadis, K., Flentje, H., Fiebig, M., Gysel-Beer, M., Hand, J. L., Hoffer, A., Hooda, R., Hueglin, C., Joubert, W., Keywood, M., Kim, J. E., Kim, S.-W., Labuschagne, C., Lin, N.-H., Lin, Y., Lund Myhre, C., Luoma, K., Lyamani, H., Marinoni, A., Mayol-Bracero, O. L., Mihalopoulos, N., Pandolfi, M., Prats, N., Prenni, A. J., Putaud, J.-P., Ries, L., Reisen, F., Sellegri, K., Sharma, S., Sheridan, P., Sherman, J. P., Sun, J., Titos, G., Torres, E., Tuch, T., Weller, R., Wiedensohler, A., Zieger, P., and Laj, P.: Multidecadal trend analysis of in situ aerosol radiative properties around the world, Atmos. Chem. Phys., 20, 8867–8908, https://doi.org/10.5194/acp-20-8867-2020, 2020. a
Copernicus Climate Change Service: Precipitation monthly and daily gridded data from 1979 to present derived from satellite measurement, Copernicus Climate Change Service (C3S) Climate Data Store (CDS) [data set], https://doi.org/10.24381/cds.c14d9324, 2021. a
Doelling, D. R., Loeb, N. G., Keyes, D. F., Nordeen, M. L., Morstad, D., Nguyen, C., Wielicki, B. A., Young, D. F., and Sun, M.: Geostationary Enhanced Temporal Interpolation for CERES Flux Products, J. Atmos. Ocean. Technol., 30, 1072–1090, https://doi.org/10.1175/JTECH-D-12-00136.1, 2013. a
Désalmand, F.: Observations of CCN concentrations south of the Sahara during a Dust Haze, Atmos. Res., 21, 13–28, https://doi.org/10.1016/0169-8095(87)90014-7, 1987. a
Errera, Q., Bennouna, Y., Schulz, M., Eskes, H. J., Basart, S., Benedictow, A., Blechschmidt, A.-M., Chabrillat, S., Clark, H., Cuevas, E., Flentje, H., Hansen, K. M., Im, U., Kapsomenakis, J., Langerock, B., Petersen, K., Richter, A., Sudarchikova, N., Thouret, V., Wagner, A., Wang, Y., Warneke, T., and Zerefos, C.: Validation report of the CAMS global Reanalysis of aerosols and reactive gases, years 2003–2020, Copernicus Atmosphere Monitoring Service (CAMS) report, https://doi.org/10.24380/8gf9-k005, 2021. a
Forster, P., Storelvmo, T., Armour, K., Collins, W., Dufresne, J.-L., Frame, D., Lunt, D., Mauritsen, T., Palmer, M., Watanabe, M., Wild, M., and Zhang, H.: The Earth’s Energy Budget, Climate Feedbacks, and Climate Sensitivity, in: Climate Change 2021: The Physical Science Basis. Contribution of Working Group I to the Sixth Assessment Report of the Intergovernmental Panel on Climate Change, edited by: Masson-Delmotte, V., Zhai, P., Pirani, A., Connors, S., Péan, C., Berger, S., Caud, N., Chen, Y., Goldfarb, L., Gomis, M., Huang, M., Leitzell, K., Lonnoy, E., Matthews, J., Maycock, T., Waterfield, T., Yelekçi, O., Yu, R., and Zhou, B., 923–1054 pp., Cambridge University Press, Cambridge, United Kingdom and New York, NY, USA, https://doi.org/10.1017/9781009157896.009, 2021. a, b, c
Fossum, K. N., Ovadnevaite, J., Ceburnis, D., Dall’Osto, M., Marullo, S., Bellacicco, M., Simó, R., Liu, D., Flynn, M., Zuend, A., and O’Dowd, C.: Summertime Primary and Secondary Contributions to Southern Ocean Cloud Condensation Nuclei, Sci. Rep., 8, 13844, https://doi.org/10.1038/s41598-018-32047-4, 2018. a
Garimella, S., Huang, Y.-W., Seewald, J. S., and Cziczo, D. J.: Cloud condensation nucleus activity comparison of dry- and wet-generated mineral dust aerosol: the significance of soluble material, Atmos. Chem. Phys., 14, 6003–6019, https://doi.org/10.5194/acp-14-6003-2014, 2014. a
Gasteiger, J. and Wiegner, M.: MOPSMAP v1.0: a versatile tool for the modeling of aerosol optical properties, Geosci. Model Dev., 11, 2739–2762, https://doi.org/10.5194/gmd-11-2739-2018, 2018. a
Gras, J. L.: Cloud condensation nuclei over the Southern Ocean, Geophys. Res. Lett., 17, 1565–1567, https://doi.org/10.1029/GL017i010p01565, 1990. a, b
Gras, J. L. and Keywood, M.: Cloud condensation nuclei over the Southern Ocean: wind dependence and seasonal cycles, Atmos. Chem. Phys., 17, 4419–4432, https://doi.org/10.5194/acp-17-4419-2017, 2017. a, b, c, d
Grosvenor, D. P. and Wood, R.: The effect of solar zenith angle on MODIS cloud optical and microphysical retrievals within marine liquid water clouds, Atmos. Chem. Phys., 14, 7291–7321, https://doi.org/10.5194/acp-14-7291-2014, 2014. a
Grosvenor, D. P., Sourdeval, O., Zuidema, P., Ackerman, A., Alexandrov, M. D., Bennartz, R., Boers, R., Cairns, B., Chiu, J. C., Christensen, M., Deneke, H., Diamond, M., Feingold, G., Fridlind, A., Hünerbein, A., Knist, C., Kollias, P., Marshak, A., McCoy, D., Merk, D., Painemal, D., Rausch, J., Rosenfeld, D., Russchenberg, H., Seifert, P., Sinclair, K., Stier, P., van Diedenhoven, B., Wendisch, M., Werner, F., Wood, R., Zhang, Z., and Quaas, J.: Remote Sensing of Droplet Number Concentration in Warm Clouds: A Review of the Current State of Knowledge and Perspectives, Rev. Geophys., 56, 409–453, https://doi.org/10.1029/2017RG000593, 2018. a
Gryspeerdt, E., Quaas, J., Ferrachat, S., Gettelman, A., Ghan, S., Lohmann, U., Morrison, H., Neubauer, D., Partridge, D. G., Stier, P., Takemura, T., Wang, H., Wang, M., and Zhang, K.: Constraining the instantaneous aerosol influence on cloud albedo, P. Natl. Acad. Sci. USA, 114, 4899–4904, https://doi.org/10.1073/pnas.1617765114, 2017. a, b
Gryspeerdt, E., Smith, T. W. P., O'Keeffe, E., Christensen, M. W., and Goldsworth, F. W.: The Impact of Ship Emission Controls Recorded by Cloud Properties, Geophys. Res. Lett., 46, 12547–12555, https://doi.org/10.1029/2019GL084700, 2019. a, b
Gryspeerdt, E., McCoy, D., Crosbie, E., Moore, R. H., Nott, G. J., Painemal, D., Small-Griswold, J. D., Sorooshian, A., and Ziemba, L.: Cloud droplet number concentration, calculated from the MODIS (Moderate resolution imaging spectroradiometer) cloud optical properties retrieval and gridded using different sampling strategies, NERC EDS Centre for Environmental Data Analysis [data set], https://doi.org/10.5285/864a46cc65054008857ee5bb772a2a2b, 2022. a, b
Gryspeerdt, E., Povey, A. C., Grainger, R. G., Hasekamp, O., Hsu, N. C., Mulcahy, J. P., Sayer, A. M., and Sorooshian, A.: Uncertainty in aerosol–cloud radiative forcing is driven by clean conditions, Atmos. Chem. Phys., 23, 4115–4122, https://doi.org/10.5194/acp-23-4115-2023, 2023. a
Hasekamp, O. P., Gryspeerdt, E., and Quaas, J.: Analysis of polarimetric satellite measurements suggests stronger cooling due to aerosol-cloud interactions, Nat. Commun., 10, 5405, https://doi.org/10.1038/s41467-019-13372-2, 2019. a
Humphries, R. S., Keywood, M. D., Ward, J. P., Harnwell, J., Alexander, S. P., Klekociuk, A. R., Hara, K., McRobert, I. M., Protat, A., Alroe, J., Cravigan, L. T., Miljevic, B., Ristovski, Z. D., Schofield, R., Wilson, S. R., Flynn, C. J., Kulkarni, G. R., Mace, G. G., McFarquhar, G. M., Chambers, S. D., Williams, A. G., and Griffiths, A. D.: Measurement report: Understanding the seasonal cycle of Southern Ocean aerosols, Atmos. Chem. Phys., 23, 3749–3777, https://doi.org/10.5194/acp-23-3749-2023, 2023. a, b, c, d, e, f, g, h, i
IMO: IMO: Annex 15, Resolution MEPC.321(74) 2019 Guidelines for Port State Control Under MARPOL Annex VI Chap. 3, https://wwwcdn.imo.org/localresources/en/OurWork/Environment/Documents/MEPC.321(74).pdf (last access: 4 March 2022), 2019. a, b
Inness, A., Ades, M., Agustí-Panareda, A., Barré, J., Benedictow, A., Blechschmidt, A.-M., Dominguez, J. J., Engelen, R., Eskes, H., Flemming, J., Huijnen, V., Jones, L., Kipling, Z., Massart, S., Parrington, M., Peuch, V.-H., Razinger, M., Remy, S., Schulz, M., and Suttie, M.: The CAMS reanalysis of atmospheric composition, Atmos. Chem. Phys., 19, 3515–3556, https://doi.org/10.5194/acp-19-3515-2019, 2019a. a, b, c, d
Inness, A., Ades, M., Agustí-Panareda, A., Barré, J., Benedictow, A., Blechschmidt, A., Dominguez, J., Engelen, R., Eskes, H., Flemming, J., Huijnen, V., Jones, L., Kipling, Z., Massart, S., Parrington, M., Peuch, V.-H., Razinger, M., Remy, S., Schulz, M., and Suttie, M.: CAMS global reanalysis (EAC4) monthly averaged fields, copernicus Atmosphere Monitoring Service (CAMS) Atmosphere Data Store (ADS) [data set], https://ads.atmosphere.copernicus.eu/datasets/cams-global-reanalysis-eac4-monthly?tab=overview (last access: 20 October 2024), 2019b. a, b
Jayachandran, V. N., Varghese, M., Murugavel, P., Todekar, K. S., Bankar, S. P., Malap, N., Dinesh, G., Safai, P. D., Rao, J., Konwar, M., Dixit, S., and Prabha, T. V.: Cloud condensation nuclei characteristics during the Indian summer monsoon over a rain-shadow region, Atmos. Chem. Phys., 20, 7307–7334, https://doi.org/10.5194/acp-20-7307-2020, 2020. a
Jin, S., Ma, Y., Huang, Z., Huang, J., Gong, W., Liu, B., Wang, W., Fan, R., and Li, H.: A comprehensive reappraisal of long-term aerosol characteristics, trends, and variability in Asia, Atmos. Chem. Phys., 23, 8187–8210, https://doi.org/10.5194/acp-23-8187-2023, 2023. a
Kendall, M. G.: Rank Correlation Methods, Charles Griffin, London, 1975. a
Kumar, P., Nenes, A., and Sokolik, I. N.: Importance of adsorption for CCN activity and hygroscopic properties of mineral dust aerosol, Geophys. Res. Lett., 36, L24804, https://doi.org/10.1029/2009GL040827, 2009. a
Lana, A., Bell, T. G., Simó, R., Vallina, S. M., Ballabrera-Poy, J., Kettle, A. J., Dachs, J., Bopp, L., Saltzman, E. S., Stefels, J., Johnson, J. E., and Liss, P. S.: An updated climatology of surface dimethlysulfide concentrations and emission fluxes in the global ocean, Global Biogeochem. Cy., 25, GB1004, https://doi.org/10.1029/2010GB003850, 2011. a
Mace, G. G. and Avey, S.: Seasonal variability of warm boundary layer cloud and precipitation properties in the Southern Ocean as diagnosed from A-Train data, J. Geophys. Res.-Atmos., 122, 1015–1032, https://doi.org/10.1002/2016JD025348, 2017. a
Mace, G. G., Benson, S., Humphries, R., Gombert, P. M., and Sterner, E.: Natural marine cloud brightening in the Southern Ocean, Atmos. Chem. Phys., 23, 1677–1685, https://doi.org/10.5194/acp-23-1677-2023, 2023. a
Mace, G. G., Benson, S., Sterner, E., Protat, A., Humphries, R., and Hallar, A. G.: The Association Between Cloud Droplet Number over the Summer Southern Ocean and Air Mass History, J. Geophys. Res.-Atmos., 129, e2023JD040673, https://doi.org/10.1029/2023JD040673, 2024. a
Mann, H. B.: Nonparametric Tests Against Trend, Econometrica, 13, 245–259, https://doi.org/10.2307/1907187, 1945. a
McCoy, D. T., Burrows, S. M., Wood, R., Grosvenor, D. P., Elliott, S. M., Ma, P.-L., Rasch, P. J., and Hartmann, D. L.: Natural aerosols explain seasonal and spatial patterns of Southern Ocean cloud albedo, Sci. Adv., 1, e1500157, https://doi.org/10.1126/sciadv.1500157, 2015. a
McCoy, D. T., Bender, F. A.-M., Grosvenor, D. P., Mohrmann, J. K., Hartmann, D. L., Wood, R., and Field, P. R.: Predicting decadal trends in cloud droplet number concentration using reanalysis and satellite data, Atmos. Chem. Phys., 18, 2035–2047, https://doi.org/10.5194/acp-18-2035-2018, 2018. a
McCoy, I. L., Bretherton, C. S., Wood, R., Twohy, C. H., Gettelman, A., Bardeen, C. G., and Toohey, D. W.: Influences of Recent Particle Formation on Southern Ocean Aerosol Variability and Low Cloud Properties, J. Geophys. Res.-Atmos., 126, e2020JD033529, https://doi.org/10.1029/2020JD033529, 2021. a
Moore, R. H., Karydis, V. A., Capps, S. L., Lathem, T. L., and Nenes, A.: Droplet number uncertainties associated with CCN: an assessment using observations and a global model adjoint, Atmos. Chem. Phys., 13, 4235–4251, https://doi.org/10.5194/acp-13-4235-2013, 2013. a, b, c, d
NASA Langley Research Center: CERES SYN1deg Level 3 Data Product, Version 4.1., NASA Atmospheric Science Data Center (ASDC) [data set], https://doi.org/10.5067/Terra+Aqua/CERES/SYN1deg_L3.004A, 2021. a
NASA/LARC/SD/ASDC: CALIPSO Lidar Level 2 Aerosol Profile, V4-20, EarthData [data set], https://doi.org/10.5067/CALIOP/CALIPSO/LID_L2_05, 2018. a, b
O'Connor, F. M., Johnson, C. E., Morgenstern, O., Abraham, N. L., Braesicke, P., Dalvi, M., Folberth, G. A., Sanderson, M. G., Telford, P. J., Voulgarakis, A., Young, P. J., Zeng, G., Collins, W. J., and Pyle, J. A.: Evaluation of the new UKCA climate-composition model – Part 2: The Troposphere, Geosci. Model Dev., 7, 41–91, https://doi.org/10.5194/gmd-7-41-2014, 2014. a
Paramonov, M., Kerminen, V.-M., Gysel, M., Aalto, P. P., Andreae, M. O., Asmi, E., Baltensperger, U., Bougiatioti, A., Brus, D., Frank, G. P., Good, N., Gunthe, S. S., Hao, L., Irwin, M., Jaatinen, A., Jurányi, Z., King, S. M., Kortelainen, A., Kristensson, A., Lihavainen, H., Kulmala, M., Lohmann, U., Martin, S. T., McFiggans, G., Mihalopoulos, N., Nenes, A., O'Dowd, C. D., Ovadnevaite, J., Petäjä, T., Pöschl, U., Roberts, G. C., Rose, D., Svenningsson, B., Swietlicki, E., Weingartner, E., Whitehead, J., Wiedensohler, A., Wittbom, C., and Sierau, B.: A synthesis of cloud condensation nuclei counter (CCNC) measurements within the EUCAARI network, Atmos. Chem. Phys., 15, 12211–12229, https://doi.org/10.5194/acp-15-12211-2015, 2015. a
Platnick, S., King, M., and Hubanks, P.: MODIS Atmosphere L3 Monthly Product, NASA MODIS Adaptive Processing System, Goddard Space Flight Center [data set], https://doi.org/10.5067/MODIS/MYD08_M3.061, 2017a. a
Platnick, S., Meyer, K. G., King, M. D., Wind, G., Amarasinghe, N., Marchant, B., Arnold, G. T., Zhang, Z., Hubanks, P. A., Holz, R. E., Yang, P., Ridgway, W. L., and Riedi, J.: The MODIS Cloud Optical and Microphysical Products: Collection 6 Updates and Examples From Terra and Aqua, IEEE T. Geosci. Remote Sens., 55, 502–525, https://doi.org/10.1109/TGRS.2016.2610522, 2017b. a
Pöhlker, M. L., Pöhlker, C., Quaas, J., Mülmenstädt, J., Pozzer, A., Andreae, M. O., Artaxo, P., Block, K., Coe, H., Ervens, B., Gallimore, P., Gaston, C. J., Gunthe, S. S., Henning, S., Herrmann, H., Krüger, O. O., McFiggans, G., Poulain, L., Raj, S. S., Reyes-Villegas, E., Royer, H. M., Walter, D., Wang, Y., and Pöschl, U.: Global organic and inorganic aerosol hygroscopicity and its effect on radiative forcing, Nat. Commun., 14, 6139, https://doi.org/10.1038/s41467-023-41695-8, 2023. a
Popp, T., De Leeuw, G., Bingen, C., Brühl, C., Capelle, V., Chedin, A., Clarisse, L., Dubovik, O., Grainger, R., Griesfeller, J., Heckel, A., Kinne, S., Klüser, L., Kosmale, M., Kolmonen, P., Lelli, L., Litvinov, P., Mei, L., North, P., Pinnock, S., Povey, A., Robert, C., Schulz, M., Sogacheva, L., Stebel, K., Stein Zweers, D., Thomas, G., Tilstra, L. G., Vandenbussche, S., Veefkind, P., Vountas, M., and Xue, Y.: Development, Production and Evaluation of Aerosol Climate Data Records from European Satellite Observations (Aerosol_cci), Remote Sens., 8, 421, https://doi.org/10.3390/rs8050421, 2016. a
Quaas, J., Arola, A., Cairns, B., Christensen, M., Deneke, H., Ekman, A. M. L., Feingold, G., Fridlind, A., Gryspeerdt, E., Hasekamp, O., Li, Z., Lipponen, A., Ma, P.-L., Mülmenstädt, J., Nenes, A., Penner, J. E., Rosenfeld, D., Schrödner, R., Sinclair, K., Sourdeval, O., Stier, P., Tesche, M., van Diedenhoven, B., and Wendisch, M.: Constraining the Twomey effect from satellite observations: issues and perspectives, Atmos. Chem. Phys., 20, 15079–15099, https://doi.org/10.5194/acp-20-15079-2020, 2020. a, b
Quaas, J., Jia, H., Smith, C., Albright, A. L., Aas, W., Bellouin, N., Boucher, O., Doutriaux-Boucher, M., Forster, P. M., Grosvenor, D., Jenkins, S., Klimont, Z., Loeb, N. G., Ma, X., Naik, V., Paulot, F., Stier, P., Wild, M., Myhre, G., and Schulz, M.: Robust evidence for reversal of the trend in aerosol effective climate forcing, Atmos. Chem. Phys., 22, 12221–12239, https://doi.org/10.5194/acp-22-12221-2022, 2022. a, b, c, d, e
Redemann, J. and Gao, L.: A machine learning paradigm for necessary observations to reduce uncertainties in aerosol climate forcing, Nat. Commun., 15, 8343, https://doi.org/10.1038/s41467-024-52747-y, 2024. a
Redemann, J., Wood, R., Zuidema, P., Doherty, S. J., Luna, B., LeBlanc, S. E., Diamond, M. S., Shinozuka, Y., Chang, I. Y., Ueyama, R., Pfister, L., Ryoo, J.-M., Dobracki, A. N., da Silva, A. M., Longo, K. M., Kacenelenbogen, M. S., Flynn, C. J., Pistone, K., Knox, N. M., Piketh, S. J., Haywood, J. M., Formenti, P., Mallet, M., Stier, P., Ackerman, A. S., Bauer, S. E., Fridlind, A. M., Carmichael, G. R., Saide, P. E., Ferrada, G. A., Howell, S. G., Freitag, S., Cairns, B., Holben, B. N., Knobelspiesse, K. D., Tanelli, S., L'Ecuyer, T. S., Dzambo, A. M., Sy, O. O., McFarquhar, G. M., Poellot, M. R., Gupta, S., O'Brien, J. R., Nenes, A., Kacarab, M., Wong, J. P. S., Small-Griswold, J. D., Thornhill, K. L., Noone, D., Podolske, J. R., Schmidt, K. S., Pilewskie, P., Chen, H., Cochrane, S. P., Sedlacek, A. J., Lang, T. J., Stith, E., Segal-Rozenhaimer, M., Ferrare, R. A., Burton, S. P., Hostetler, C. A., Diner, D. J., Seidel, F. C., Platnick, S. E., Myers, J. S., Meyer, K. G., Spangenberg, D. A., Maring, H., and Gao, L.: An overview of the ORACLES (ObseRvations of Aerosols above CLouds and their intEractionS) project: aerosol–cloud–radiation interactions in the southeast Atlantic basin, Atmos. Chem. Phys., 21, 1507–1563, https://doi.org/10.5194/acp-21-1507-2021, 2021. a
Revell, L. E., Kremser, S., Hartery, S., Harvey, M., Mulcahy, J. P., Williams, J., Morgenstern, O., McDonald, A. J., Varma, V., Bird, L., and Schuddeboom, A.: The sensitivity of Southern Ocean aerosols and cloud microphysics to sea spray and sulfate aerosol production in the HadGEM3-GA7.1 chemistry–climate model, Atmos. Chem. Phys., 19, 15447–15466, https://doi.org/10.5194/acp-19-15447-2019, 2019. a, b
Rosenfeld, D., Kokhanovsky, A., Goren, T., Gryspeerdt, E., Hasekamp, O., Jia, H., Lopatin, A., Quaas, J., Pan, Z., and Sourdeval, O.: Frontiers in Satellite-Based Estimates of Cloud-Mediated Aerosol Forcing, Rev. Geophys., 61, e2022RG000799, https://doi.org/10.1029/2022RG000799, 2023. a, b
Ross, K. E., Piketh, S. J., Bruintjes, R. T., Burger, R. P., Swap, R. J., and Annegarn, H. J.: Spatial and seasonal variations in CCN distribution and the aerosol-CCN relationship over southern Africa, J. Geophys. Res.-Atmos., 108, 8481, https://doi.org/10.1029/2002JD002384, 2003. a
Shen, Y., Virkkula, A., Ding, A., Luoma, K., Keskinen, H., Aalto, P. P., Chi, X., Qi, X., Nie, W., Huang, X., Petäjä, T., Kulmala, M., and Kerminen, V.-M.: Estimating cloud condensation nuclei number concentrations using aerosol optical properties: role of particle number size distribution and parameterization, Atmos. Chem. Phys., 19, 15483–15502, https://doi.org/10.5194/acp-19-15483-2019, 2019. a, b, c
Shinozuka, Y., Clarke, A. D., Nenes, A., Jefferson, A., Wood, R., McNaughton, C. S., Ström, J., Tunved, P., Redemann, J., Thornhill, K. L., Moore, R. H., Lathem, T. L., Lin, J. J., and Yoon, Y. J.: The relationship between cloud condensation nuclei (CCN) concentration and light extinction of dried particles: indications of underlying aerosol processes and implications for satellite-based CCN estimates, Atmos. Chem. Phys., 15, 7585–7604, https://doi.org/10.5194/acp-15-7585-2015, 2015. a
Tesche, M., Ansmann, A., Müller, D., Althausen, D., Engelmann, R., Freudenthaler, V., and Groß, S.: Vertically resolved separation of dust and smoke over Cape Verde using multiwavelength Raman and polarization lidars during Saharan Mineral Dust Experiment 2008, J. Geophys. Res.-Atmos., 114, D13202, https://doi.org/10.1029/2009JD011862, 2009. a
West, R. E. L., Stier, P., Jones, A., Johnson, C. E., Mann, G. W., Bellouin, N., Partridge, D. G., and Kipling, Z.: The importance of vertical velocity variability for estimates of the indirect aerosol effects, Atmos. Chem. Phys., 14, 6369–6393, https://doi.org/10.5194/acp-14-6369-2014, 2014. a
Wood, R., Stemmler, J. D., Rémillard, J., and Jefferson, A.: Low-CCN concentration air masses over the eastern North Atlantic: Seasonality, meteorology, and drivers, J. Geophys. Res.-Atmos., 122, 1203–1223, https://doi.org/10.1002/2016JD025557, 2017. a
Yu, L., Zhong, S., and Sun, B.: The Climatology and Trend of Surface Wind Speed over Antarctica and the Southern Ocean and the Implication to Wind Energy Application, Atmosphere, 11, 108, https://doi.org/10.3390/atmos11010108, 2020. a