the Creative Commons Attribution 4.0 License.
the Creative Commons Attribution 4.0 License.
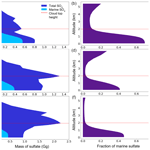
Quantifying the impacts of marine aerosols over the southeast Atlantic Ocean using a chemical transport model: implications for aerosol–cloud interactions
Rebecca M. Garland
The southeast Atlantic region, characterized by persistent stratocumulus clouds, has one of the highest uncertainties in aerosol radiative forcing and significant variability across climate models. In this study, we analyze the seasonally varying role of marine aerosol sources and identify key uncertainties in aerosol composition at cloud-relevant altitudes over the southeast Atlantic using the GEOS-Chem chemical transport model. We evaluate simulated aerosol optical depth (AOD) and speciated aerosol concentrations against those collected from ground observations and aircraft campaigns such as LASIC, ORACLES, and CLARIFY, conducted during 2017. The model consistently underestimates AOD relative to AERONET, particularly at remote locations like Ascension Island. However, when compared with aerosol mass concentrations from aircraft campaigns during the biomass burning period, it performs adequately at cloud-relevant altitudes, with a normalized mean bias (NMB) between −3.5 % (CLARIFY) and −7.5 % (ORACLES). At these altitudes, in the model, organic aerosols (63 %) dominate during the biomass burning period, while sulfate (41 %) prevails during austral summer, when dimethylsulfide (DMS) emissions peak in the model. Our findings indicate that marine sulfate can account for up to 69 % of total sulfate during the high-DMS period. Sensitivity analyses indicate that refining DMS emissions and oxidation chemistry may increase sulfate aerosol produced from marine sources, highlighting that there remains large uncertainty as to the role of DMS emissions in the marine boundary layer. Additionally, we find marine primary organic aerosol emissions may substantially increase total organic aerosol concentrations, particularly during austral summer. This study underscores the imperative need to refine marine emissions and their chemical transformations, as aerosols from marine sources are a major component of total aerosols at cloud-relevant altitudes and may impact uncertainties in aerosol radiative forcing over the southeast Atlantic.
- Article
(4623 KB) - Full-text XML
- BibTeX
- EndNote
Marine aerosols are a primary contributor to natural atmospheric aerosols and consequently influence the Earth's radiative balance (Spracklen et al., 2008; Vignati et al., 2001). Aerosols in the marine boundary layer have a significant impact on the properties of low-altitude marine clouds, particularly on their ability to reflect solar radiation and cool the climate (Seinfeld and Pandis, 2016; Wood, 2012; Chen et al., 2014; Quinn et al., 2017). The southeast Atlantic (SEA) is marked by a persistent deck of low-level stratocumulus (Sc) clouds. However, this region exhibits the highest uncertainty in aerosol radiative forcings in the Aerosol Comparisons between Observations and Models (AeroCom) intercomparison across Coupled Model Intercomparison Project Phase 5 (CMIP5) general circulation models (GCMs) and chemical transport models (Stier et al., 2013). This uncertainty is primarily driven by challenges in accurately representing cloud fraction, aerosol–cloud properties, and vertical structure, both in the presence and absence of smoke (Stier et al., 2013; Doherty et al., 2022). In this study, we investigate the role of marine aerosols and sources of uncertainty affecting aerosol composition within the boundary layer, particularly in this critical region of aerosol–cloud interactions over the SEA.
The SEA region encompasses the Benguela upwelling system (BUS), renowned for its high primary production of marine phytoplankton and fish populations (Shannon and Nelson, 1996; Jarre et al., 2015). This elevated phytoplankton activity serves as the main natural source of the volatile organic compound dimethylsulfide (DMS), thereby influencing the global tropospheric sulfur budget (Andreae, 1990; Bates et al., 1992). Once released into the atmosphere through air–sea exchange, DMS undergoes complex chemical transformations. In the gas phase, it is oxidized to form H2SO4 and methanesulfonic acid (MSA), which has implications for new particle formation (Chen et al., 2015), while in the aqueous phase, it leads to the production of MSA and sulfate aerosols, impacting cloud microphysical properties (Kaufman and Tanré, 1994). Although DMS is a critical source of natural aerosols, contributing over 50 % of natural gas-phase sulfur emissions (Chin et al., 1996; Kilgour et al., 2022), the exact mechanisms of DMS oxidation and subsequent formation of sulfate and MSA aerosol remain inadequately understood (Ravishankara et al., 1997; Barnes et al., 2006; Hoffmann et al., 2016). This gap in understanding contributes to substantial uncertainty in aerosol radiative forcing, which is highly sensitive to uncertainties in natural aerosols (Carslaw et al., 2013; Fung et al., 2022). Additionally, marine aerosols comprise primary aerosols such as sea spray aerosols, which consist of salts, sulfate, and organic matter, released into the atmosphere primarily by the bubble-bursting process (O'Dowd and De Leeuw, 2007; Russell et al., 2010; Prather et al., 2013; Brooks and Thornton, 2018; Russell et al., 2023). Investigating the uncertainties related to marine emissions and chemistry is crucial to refine our understanding of the impacts of marine aerosols on climate.
The SEA lies at the confluence of not only marine aerosols, but also other natural and anthropogenic aerosols from local and distant origin (Andreae et al., 1995; Swap et al., 1996; Formenti et al., 1999; Swap et al., 2003; Tournadre, 2014). During the austral spring (August to October), seasonal fires in the neighboring southern African region contribute nearly one-third of global total biomass burning emissions (van der Werf et al., 2010). This seasonal influx of biomass burning aerosols aloft interacts with the underlying Sc deck, introducing considerable variability into aerosol forcing assessments in the SEA region (Lindesay et al., 1996; Swap et al., 2003). To address these uncertainties, several international field campaigns were conducted between 1992 and 2018 during the peak-biomass-burning season (Swap et al., 2003; Formenti et al., 2019; Haywood et al., 2021; Redemann et al., 2021). Despite the region being a prolific source of marine aerosols throughout the year, the potential impact of aerosols on regional climate dynamics through interactions with the persistent low-level marine clouds outside of the biomass burning season has been largely overlooked.
Here, we use the GEOS-Chem global chemical transport model to analyze high-resolution, seasonally varying aerosol composition at the altitudes of persistent stratocumulus clouds over the SEA. We specifically focus on the role of marine aerosols, analyzing their contributions to sulfate and organic aerosol concentrations. We evaluate simulated aerosol optical depth (AOD) and speciated aerosol concentrations against observational data from the Aerosol Robotic Network (AERONET) and the Layered Atlantic Smoke Interactions with Clouds (LASIC; Zuidema et al., 2018), ObseRvations of Aerosols above CLouds and their intEractionS (ORACLES; Redemann et al., 2021), and CLoud–Aerosol–Radiation Interaction and Forcing (CLARIFY; Haywood et al., 2021) field campaigns during the year 2017. We assess the sensitivity of our results to uncertainty in DMS oxidation mechanisms and emissions of DMS, SO2, and marine primary organics. Our findings aim to enhance the understanding of the seasonally varying role of marine aerosols in aerosol–cloud interactions in the SEA by a comprehensive evaluation of aerosol composition at cloud altitudes.
2.1 Model description
Here, we use the GEOS-Chem 3D atmospheric chemical transport model version 13.3.3 with detailed gas- and aerosol-phase tropospheric chemistry (https://doi.org/10.5281/zenodo.5748260, The International GEOS-Chem User Community, 2021). The model is driven by meteorology from the Modern-Era Retrospective analysis for Research and Applications, Version 2 (MERRA2) reanalysis, from the NASA Global Modeling Assimilation Office (GMAO) (Gelaro et al., 2017). We perform nested grid simulations over the southwestern coast of Africa (40° W–20° E, 0–40° S) with a horizontal resolution of 0.5° by 0.625° and extending over 47 vertical layers from the surface to 0.01 hPa. A chemical time step of 20 min and transport time step of 10 min are applied, as recommended by Philip et al. (2016). Prior to the target year, 2017, we conduct a 6-month spin-up simulation. Boundary conditions are obtained from global simulations performed at 4° latitude × 5° longitude horizontal resolution for the same year after a 6-month initialization.
In GEOS-Chem, carbonaceous aerosol includes organic aerosols (OAs) and black carbon (BC). Organic aerosol is simulated using the “simple” scheme, which treats primary organic aerosol (POA) as non-volatile and includes irreversible direct yield of secondary organic aerosol (SOA) from precursors (Pai et al., 2020). The BC simulation follows the methodologies of Park et al. (2003) and Wang et al. (2014). Sulfate (Alexander et al., 2009), nitrate (Jaeglé et al., 2018), and ammonium (Fountoukis and Nenes, 2007) thermodynamic partitioning is estimated using ISORROPIA II, a widely used aerosol thermodynamic model (Fountoukis and Nenes, 2007). Monthly anthropogenic emissions follow the Community Emissions Data System (CEDSv2) inventory (Hoesly et al., 2018). Biomass burning emissions are calculated using the Global Fire Emissions Database (GFED4.1s) at 0.25° × 0.25° spatial resolution, with fractional daily and 3-hourly scaling factors applied to the cumulative monthly data (van der Werf et al., 2017). DMS emissions in the standard model use the Lana et al. (2011) climatology, which compiles DMS concentrations using data from the Global Surface Seawater DMS Database (https://saga.pmel.noaa.gov/dms_data, last access: 2 May 2024) collected from 1972 to 2009, incorporated with additional observations from the South Pacific (Lee et al., 2010). The standard DMS oxidation mechanism in the model includes only three gas-phase DMS reactions, which directly yield SO2 and MSA according to the reaction mechanism outlined by Chin et al. (1996), and incorporates updated reaction rate coefficients from Burkholder et al. (2015). Sea-salt aerosol (SSA) emissions from the open ocean are both wind speed dependent (Gong, 2003) and sea surface temperature dependent (Jaeglé et al., 2011). Dust emissions include natural dust (Duncan Fairlie et al., 2007) and anthropogenic dust from the Anthropogenic Fugitive, Combustion, and Industrial Dust (AFCID) inventory (Philip et al., 2017).
In this study, we carry out multiple simulations to explore the sensitivity of marine aerosols to various emission sources. To quantify the impact of marine sources on sulfate aerosols within the stratocumulus cloud layer, we perform a high-resolution (0.5° × 0.625°) marine-emissions-only sensitivity simulation where SO2 and SO4 emissions from anthropogenic sources, biomass burning, volcanic activity, ships, and aviation were turned off. Additionally, to investigate the sensitivity of DMS emission fluxes to surface ocean DMS concentrations, we perform an additional simulation with DMS concentrations from Galí et al. (2018). In this dataset, DMS concentrations are estimated through a remote-sensing algorithm that integrates satellite-derived estimates of chlorophyll and light penetration, along with climatological mixed layer depth (Galí et al., 2018). Furthermore, we assess the impact of adding marine POA, co-emitted with sea-salt aerosols (Gantt et al., 2015), to the overall organic aerosol burden, which is not included in the standard model configuration. Finally, to evaluate how uncertainty in biomass burning SO2 emissions affects the relative importance of marine emissions to sulfate aerosol, we conduct two sets of sensitivity simulations using the Quick Fire Emissions Dataset (QFED) (Darmenov and da Silva, 2013; Das et al., 2017) and the Global Fire Assimilation System (GFAS) (Kaiser et al., 2012; Su et al., 2023). Each of these inventories differ in data sources, methodology, temporal resolution, and plume injection height. These sensitivity analyses were conducted for the year 2017, following a 6-month spin-up period. Details regarding the spatial resolution used in each sensitivity analysis are provided in Table A1.
2.2 Ground-based measurements
We evaluate simulated aerosol optical depth (AOD) against AOD retrieved from the ground-based Aerosol Robotic Network (AERONET) of sun photometers with direct sun measurements every 15 min (Holben et al., 1998). We use level 2.0, version 3 data that have improved cloud-screening algorithms (Giles et al., 2019). We strategically select nine sites in the study domain along coastal and oceanic regions, as shown in Fig. 1. Site information, including the coordinates, number of months with available data, and the monthly average AOD for three distinct time periods, is summarized in Table A2. The AERONET monthly average AOD is computed from daily averages for sites with at least 3 months of observations during the model simulation period (year 2017) and months with at least 15 d of measurements. These are then compared with the monthly mean AOD from the GEOS-Chem model.
The modeled AOD is sampled at each AERONET site location and computed at 550 nm wavelength by vertically integrating scattering and absorption coefficients based on the properties of various aerosol components, such as size distributions, hygroscopicity, refractive indices, and densities (Latimer and Martin, 2019). For comparison with modeled monthly AOD, daily measurements at each site at 440 nm are first interpolated to the standard wavelength of 550 nm using the local Ångström exponent between 440 and 870 nm channels, following the Ångström power law (Eq. 1; Martínez-Lozano et al., 1998). These interpolated values are then averaged to calculate the observed mean monthly AOD. The interpolation formula used is
In addition, we evaluate the model's relative aerosol composition against measurements from the Atmospheric Radiation Measurement (ARM) facility on Ascension Island during the LASIC campaign, conducted from January to November 2017. LASIC employed an Aerodyne aerosol chemical speciation monitor (ACSM) to provide quantitative measurement of the chemical composition of non-refractory aerosol components including sulfate, nitrate, ammonium, and organics. For comparative analysis, we use aerosol concentrations corrected for composition-dependent collection efficiency (CDCE) obtained from the ARM Data Archive. Barrett et al. (2022) reported that aerosol mass concentrations of individual components observed by the LASIC ACSM were 2 to 4.5 times lower than those measured by the aerosol mass spectrometer (AMS) aboard the CLARIFY campaign aircraft. Hence, we evaluate the relative rather than absolute aerosol speciation in GEOS-Chem against the LASIC ACSM.
2.3 Aircraft measurements
We evaluate simulated aerosol composition against airborne measurements from two campaigns, NASA ORACLES (Redemann et al., 2021; Ryoo et al., 2021) and UK CLARIFY (Haywood et al., 2021). The ORACLES field campaign used the NASA P-3 aircraft to make measurements based out of São Tomé and Príncipe, while CLARIFY used the FAAM BAe-146 aircraft around Ascension Island for data collection. The ORACLES aircraft primarily conducted morning sampling, between 08:00–13:00 UTC, while the CLARIFY aircraft often sampled extended hours, typically from 07:00–18:00 UTC. Both campaigns occurred during the austral winter and spring (August–September), corresponding with peak-biomass-burning events in southern Africa (Adebiyi et al., 2015). Figure 2 shows the flight tracks for these campaigns. The primary instruments and references for each campaign are listed in Table 1.
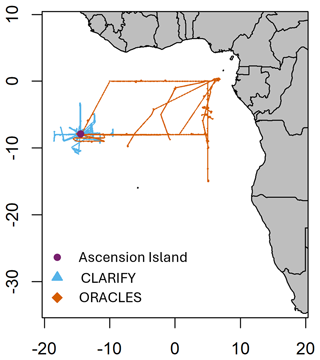
Figure 2Flight tracks from the two aircraft campaigns, CLARIFY (in blue) and ORACLES (in orange), used to evaluate the model, conducted during August–September 2017 over the southeast Atlantic region. Ascension Island is marked by the purple dot.
Table 1Aircraft campaigns in the southeast Atlantic used for model evaluation during the biomass burning season.

* Compact time-of-flight aerosol mass spectrometer (C-ToF-AMS) and high-resolution time-of-flight aerosol mass spectrometer (HR-ToF-AMS).
To facilitate comparison between airborne measurements and the GEOS-Chem model, we sampled the model to the nearest grid box, both temporally and spatially, along the flight tracks. Observations from both campaigns are reported at 1 min averaging intervals, while the model operates at a 10 min temporal resolution (see Sect. 2.1). Aerosol concentrations from the campaigns are reported as mass concentrations at standard temperature and pressure (STP: 273 K, 1 atm). The modeled concentrations are thus also standardized to STP conditions.
3.1 Model evaluation
3.1.1 Seasonal variation of AOD
The spatial distribution of seasonal mean AOD from GEOS-Chem for the year 2017 is presented in Fig. 3. Three distinct seasonal periods reflect dominant atmospheric and oceanic processes. These include the high-DMS-emission period in the SEA, during the months of January, February, November, and December (JFND); the peak-biomass-burning season in southern Africa, spanning from July to October (JASO); and the transitional season, encompassing March, April, May, and June (MAMJ).
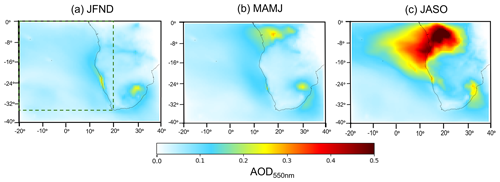
Figure 3Spatial distribution of seasonal mean modeled AOD at 550 nm for 2017. Seasons are as follows: (a) the peak-DMS-emission period (JFND), (b) the transitional period (MAMJ), and (c) the peak-biomass-burning period (JASO). The sub-domain (0–35° S, 20° E–20° W) is highlighted with a dotted green rectangle in panel (a) for reference.
The simulated DMS emissions, based on Lana climatology (2011), indicate that emissions in the BUS region peak in January, leading to elevated concentrations of sulfate aerosols. This increased sulfate (∼ 20 %), combined with dust (59 %) emissions from the Namib desert, contributes to an AOD hotspot as depicted in Fig. 3a on the southwestern coast. In the JASO period (Fig. 3c), modeled AOD increases due to biomass burning aerosols, originating from savannah fires in central and southern Africa and transported westward towards the SEA region by the southern African easterly jet (Adebiyi and Zuidema, 2016). The spatial distribution of mean transitional period AOD (Fig. 3b) features hotspots in Congo and Angola, which coincide with the onset of biomass burning in central Africa. Additionally, a year-round AOD hotspot is observed in northeastern South Africa (Gauteng province; Fig. 3), which is associated with elevated aerosol concentrations due to industrial and mining activities, as well as domestic fuel burning (Arowosegbe et al., 2021; Zhang et al., 2021).
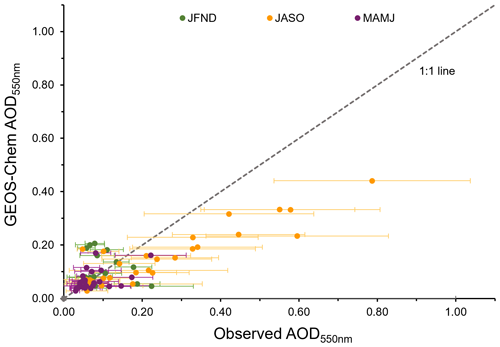
Figure 4Modeled AOD550 nm (y axis) with respect to AERONET AOD550 nm (x axis). Each data point represents the monthly mean values for each station color coded by season (green, DMS period; yellow, biomass burning period; purple, transitional period). Error bars indicate the standard deviation of the AERONET AOD550 nm values, and the dotted line depicts the one-to-one relationship.
Figure 4 shows the correlation of monthly average AERONET and GEOS-Chem AOD across the nine selected sites (see Sect. 2.1 and Fig. 1), with the three seasonal periods distinguished by color: green for the peak-DMS-emission season (JFND), yellow for the biomass burning season (JASO), and purple for the transition period (MAMJ). Each data point corresponds to the monthly mean AOD values at distinct AERONET sites. The error bars in Fig. 4 represent the ±1 standard deviation in monthly AOD measurements at these sites, with higher deviations observed during the biomass burning months (up to ±0.25 at the Namibe site). The comparison of monthly mean AOD across individual sites (see Table A2) shows that, with the exception of Ascension Island, Gobabeb, and Upington, the mean AOD at the remaining sites during the biomass burning season (JASO) is at least 1 standard deviation higher than the mean AOD in other seasons (JFND and MAMJ).
Table 2 compiles the performance of monthly mean GEOS-Chem AOD with respect to AERONET AOD by season. JASO exhibits the strongest correlation (R= 0.901), which is statistically significant (p<0.05). The transitional period (MAMJ) shows a moderate correlation (R= 0.48) with a normalized mean bias (NMB) of 4.5 %. A negligible negative correlation coefficient (R= −0.058) with a positive bias (29.8 %) is seen during the summer period (JFND), predominantly due to anomalies at two sites. This period witnesses a considerable underestimation of AOD at Ascension Island, alongside an overestimation of dust aerosol at Gobabeb. Excluding these two sites improves the model's correlation to 0.67 (p= 0.55) and reduces the NMB to 4.7 %. This underestimate of AOD at Ascension Island (Fig. A1) during summer (JFND) suggests potential model limitations in accurately simulating natural aerosol emissions such as sea-salt and marine biogenic emissions. Meanwhile, the AOD discrepancy at Ascension Island in the biomass burning season may be due to the underestimate of transatlantic transport of light-absorbing carbon aerosols (Das et al., 2017) and deviations in its spatial distribution from typical zonal patterns over the Atlantic (Adebiyi et al., 2023). The sources of these model biases are discussed in further detail in Sect. 3.1.2.
Table 2Statistical parameters of monthly mean modeled AOD with respect to observed AOD at the AERONET sites by season.
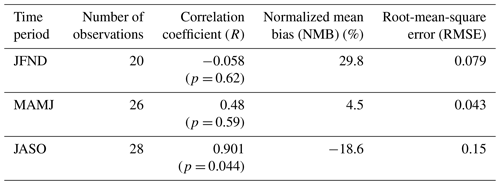
We evaluate the relative aerosol speciation simulated at Ascension Island against monthly mean ACSM observations during the LASIC campaign (see Sect. 2.2) available for January–November 2017 (Fig. A2). The seasonality of the relative contributions of organic aerosols and sulfate is consistent between the model and observations. However, the model underestimates the relative contribution of sulfate during most months, while generally overestimating the proportion of organics. An increase in the transport of biomass burning organic aerosols would further worsen the model underestimate of sulfate. A slight overestimate in the modeled relative contribution of sulfate is observed in February and November, when simulated DMS emissions in the region are high (Lana et al., 2011), largely due to enhanced underestimations of organics and nitrates.
3.1.2 Vertical profiles of aerosol composition
Figure 5 depicts the mean vertical profiles of speciated aerosol mass concentrations observed during the ORACLES and CLARIFY aircraft campaigns in August–September 2017 (the biomass burning season) compared to GEOS-Chem (see Sect. 2.2 and Table 1). The cloud top height in the SEA region generally falls between 0 and 2 km (Redemann et al., 2021). Findings from Diamond et al. (2018) indicate that aerosols below clouds in this lower atmospheric layer can also substantially impact cloud microphysics. At these altitudes (0–2 km), GEOS-Chem performs well against AMS measurements of total aerosol mass, which includes sulfate, nitrate, ammonium, and organics from these campaigns, with a NMB between −3.5 % (CLARIFY) and −7.5 % (ORACLES). At mid-altitudes (2–4 km), the model is biased low, with NMB values spanning −19 % (ORACLES) to −57 % (CLARIFY). However, the model demonstrates a pronounced bias at higher altitudes (4–7 km), where NMB values drop to −92 % (ORACLES) and −93.5 % (CLARIFY), underscoring challenges in accurately modeling aerosol concentrations at these elevations. These significant low biases in aerosol concentrations at higher altitude likely contribute to the model's underestimation of AOD during the biomass burning period (see Sect. 3.1.1). This underestimation may also be affected by the model's bulk aerosol scheme, which inadequately captures the optical properties of aerosols and is compounded by a low relative humidity bias (Zhai et al., 2021). The bulk scheme also assumes all aerosols are externally mixed, which contrasts with the variable degree of particle mixing states in the atmosphere (Yu et al., 2012; Dang et al., 2022). Moreover, studies like Hodzic et al. (2020) using NASA ATom aircraft data indicate that GEOS-Chem substantially underestimates oxidation levels of organic aerosols in remote areas, which could affect estimates of their burden and optical properties. Pai et al. (2020) further suggests that the model underestimation of organic aerosol loading at mid-tropospheric heights is linked to the surface injection treatment of fire emissions in GFED4.1s. Recent studies by Wizenberg et al. (2023) and Marvin et al. (2024) concur that the fire injection scheme is a critical source of model uncertainty, emphasizing the potential importance of accurate fire injection modeling in the free troposphere. Nonetheless, our study focuses on aerosol composition within cloud-relevant altitudes to improve our understanding of aerosol–cloud interactions and their climate implications. The observed vertical distribution of aerosol mass concentrations (left panels of Fig. 5) indicates that 18 % and 36 % of the aerosol mass for the ORACLES and CLARIFY campaigns, respectively, is located below 2 km, within columns extending up to flight altitudes of 7 km and 8 km. However, the model simulates elevated aerosol mass at these lower altitudes, 24 % and 50 % of the column for ORACLES and CLARIFY, respectively.
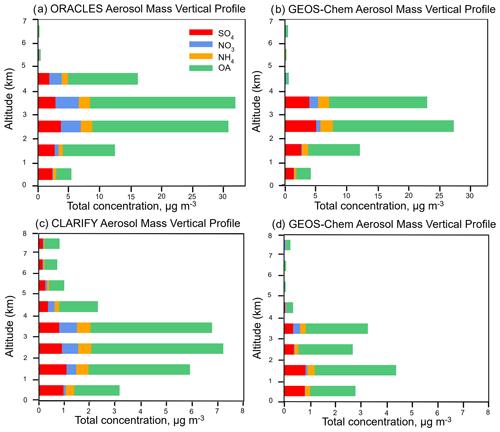
Figure 5Average vertical profiles of simulated and observed aerosol mass during August–September 2017 (peak-biomass-burning season) from aircraft campaigns. Panels (a) and (c) present the vertical distribution of aerosols observed during the ORACLES flight campaign (panel a) and the CLARIFY flight campaign (panel c) at STP (see Sect. 2.3). Panels (b) and (d) display the GEOS-Chem model simulations along the respective flight tracks of each campaign. All data are averaged over 1 km vertical bins.
At altitudes where clouds persist in the domain (0 to 2 km), sulfate and organic aerosols are the dominant aerosol types. Here, the model effectively captures the mass concentration of organic aerosols, with a NMB ranging from −0.40 % for ORACLES to −14 % for CLARIFY. However, it underestimates sulfate aerosol concentrations by 19 % at cloud altitudes for both campaigns. For other aerosol types and altitudes, the model consistently underestimates concentrations, except for sulfate and ammonium aerosols between 2 and 4 km during the ORACLES campaign, which the model overestimates by 40 % and 4.6 %, respectively. The model captures the total aerosol mass from 0 through 7 km for sulfate and ammonium aerosols during the ORACLES campaign, with only minimal underestimations of 1.5 % and 0.7 %, respectively. This indicates a potential discrepancy in the vertical distribution of these aerosols rather than in total mass.
3.2 Seasonal variation in aerosol composition and sources at cloud altitudes
Figure 6 presents the simulated seasonal mean aerosol fractional composition within cloud-relevant altitudes (0–2 km), averaged over the ocean only across the sub-domain (0–35° S, 20° E–20° W) (see the map shown in Fig. 7). This area is strategically selected to coincide with the persistent Sc cloud deck and enhance our analysis of aerosol–cloud interactions. Organic aerosols, an indicator of biomass burning, predominate during both the biomass burning (JASO) and transitional (MAMJ) periods. In contrast, sulfate aerosols dominate during austral summer, likely influenced by the high primary production from coastal upwelling that leads to DMS emissions. We investigate the model representation of sulfate and these processes further in subsequent sections. An increase in the accumulation-mode sea-salt aerosols (radius 0.01–0.5 µm) contribution (total mass of 6.7 Gg) is observed in summer (Fig. 6a) as well, compared to other seasons (5.2 Gg during MAMJ and 5.8 Gg during JASO), owing to the peak wind speeds in the southern Benguela region in this season (Hutchings et al., 2009). Black carbon, ammonium, and nitrate aerosols make minor contributions to simulated aerosol mass at cloud height throughout the year.
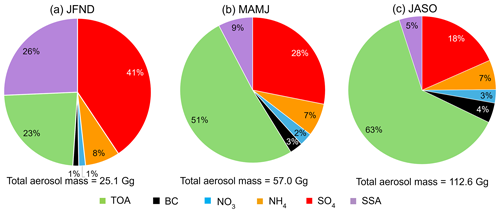
Figure 6Simulated mean fractional aerosol composition at cloud heights (0–2 km) over the ocean in the stratocumulus sub-domain (0–35° S, 20° E–20° W) by season: (a) JFND, (b) MAMJ, and (c) JASO. Here SO4, NH4, NO3, BC, TOA, and SSA represent sulfate, ammonium, nitrate, black carbon, total organic aerosol, and accumulation-mode sea-salt aerosols, respectively.
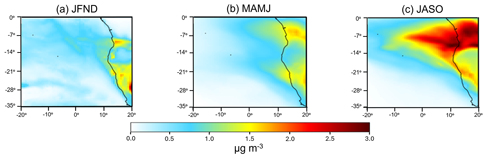
Figure 7Spatial distribution of simulated mean sulfate aerosol concentrations averaged over cloud altitudes (0–2 km) in the sub-domain (0–35° S, 20° E–20° W) by season in 2017: (a) peak-DMS-emission season (JFND), (b) transitional phase (MAMJ), and (c) biomass burning season (JASO).
3.2.1 Drivers of sulfate aerosol and importance of marine precursor emissions
Sulfate aerosols are the most or second most important aerosol component in cloud heights over the SEA (Fig. 6). We examine the sources of sulfur emissions within the model in Fig. 8. Within the broader domain (0–40° S, 40° E–20° W), anthropogenic activities are the largest source of sulfur emissions throughout the year (Fig. 8). However, the model default CEDS inventory (Hoesly et al., 2018) fails to capture the seasonality of these emissions due to absence of regional inventories and reliance on the global datasets such as the International Energy Agency (IEA) energy statistics. The anthropogenic emissions are followed by DMS emissions from the ocean, which become more pronounced during the austral summer, peaking in January. Additionally, biomass burning contributes to SO2 emissions seasonally, becoming the third most important source of total sulfur emissions during July–September (Fig. 8). In contrast, sulfur contributions from volcanic, shipping, and aircraft emissions remain minimal and constant year-round, reflecting assumptions of static fuel burned and emission levels across inventories.
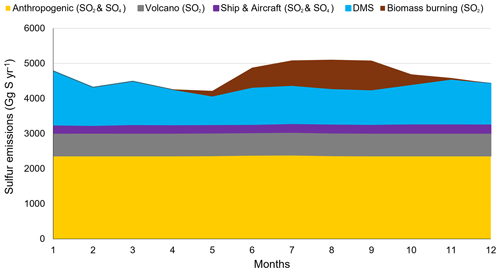
Figure 8Stacked area chart of monthly total sulfur emissions by source for 2017 across the study domain (0–40° S, 40° E–20° W) in gigagrams of sulfur per year (Gg S yr−1). Sources are indicated by color and encompass anthropogenic activities, volcanic activity, ship and aircraft emissions, biomass burning, and natural emissions of dimethyl sulfide (DMS).
To improve the understanding of the processes driving sulfate aerosol concentrations in the region, we examine sulfate aerosol's simulated spatial distribution averaged by season over the cloud height (0–2 km) in Fig. 7. Elevated concentrations of DMS, resulting from higher rates of primary production (Lana et al., 2011; Galí et al., 2018), lead to an increase in sulfate concentrations along the coastline of the Benguela region and the inner shelf of Namibia during JNFD (Fig. 7a), aligning with the AOD hotspot observed in Fig. 3a. This is consistent with the simulated dominance of sulfate aerosols at cloud-relevant altitudes during JFND (Fig. 6a). During the biomass burning months (JASO), while their relative contribution decreases (Fig. 6c), sulfate aerosols display a pronounced increase in absolute concentration (Fig. 7c) as a consequence of savanna fire emissions from southwestern Africa (van der Werf et al., 2010; Das et al., 2017). As outlined in the AOD evaluation (Sect. 3.1.1), the model underestimates the transport of emissions to remote sites (Fig. A1), resulting in a steep gradient in sulfate concentrations from the eastern landmass towards the western open ocean.
To quantitatively estimate the contribution of marine precursor emissions to sulfate aerosols, we compare the sulfate mass between the standard and marine-emissions-only sensitivity simulations (Sect. 2.1). Figure 9 shows seasonally averaged vertical profiles over the ocean region of the Sc sub-domain (0–35° S, 20° E–20° W). The figure presents the marine-only sulfate mass and the total sulfate mass from the standard simulation (left panels) and the ratio of marine sulfate to total sulfate (right panels). Vertical profiles were computed by summing the sulfate mass within each grid box, scaled by the grid box ocean fraction, across latitude and longitude within each vertical layer of the model, and then averaged temporally across each season.
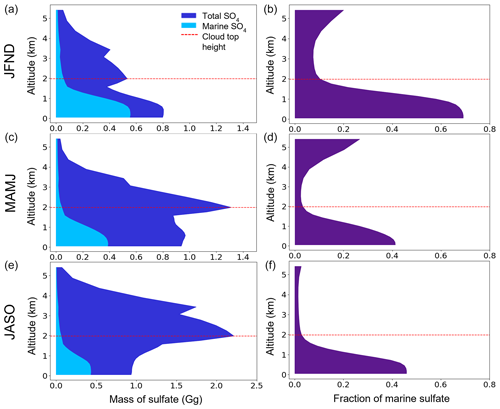
Figure 9Simulated vertical profiles of sulfate aerosol mass over oceanic regions within the sub-domain (0–35° S, 20° E–20° W) by season. Panels (a), (c), and (e) show the mass of total and marine sulfate aerosols, and panels (b), (d), and (f) indicate the sulfate fraction from marine sources. Panels (a) and (b) correspond to the peak-DMS-emission period (JFND), panels (c) and (d) correspond to the transitional period (MAMJ), and panels (e) and (f) correspond to the peak-biomass-burning period (JASO) (note that panel e displays a higher x-axis scale). The dashed red line denotes the typical maximum cloud top height (Redemann et al., 2021).
Our analysis highlights the substantial influence of marine sulfur sources on sulfate during JFND, as evidenced in the top-left panel of Fig. 9. During this period the proportion of marine sulfate reaches up to 69.1 % within the cloud (from surface to 2 km). The contribution of marine sulfate within the cloud in the subsequent periods is reduced (ranging between 2.7 %–45.9 %; Fig. 9). We find that marine-sourced sulfate mass remains fairly consistent throughout the year (Fig. 9a, c, e), with variations in the marine sulfate fraction (Fig. 9b, d, f) mainly due to changes in land-based sulfate sources. Total sulfate mass during seasons influenced by biomass burning (MAMJ and JASO) peaks at 2 km, with greater mass above 2 km during peak biomass burning (JASO), in contrast to the JFND period, in which mass peaks within clouds (0–2 km).
Table 3 summarizes the monthly mean percent contribution of marine sulfate averaged across cloud altitudes (0–2 km). The annual average total sulfate mass and marine sulfate mass are 16.2 and 3.5 Gg, respectively. The within-cloud marine sulfate contribution peaks in January (57.7 %) and is smallest in September (10.3 %). Thus, our analysis suggests that DMS emissions influence sulfate aerosol formation and their interactions with clouds in the region throughout most of the year, excepting only the peak-biomass-burning season. This emphasizes that constraining marine sulfur sources and chemistry in chemical transport and climate models may improve representation of aerosol–climate dynamics in the SEA region. Limited available observations suggest the model is biased low in AOD throughout most of the year (Sect. 3.1.1) and underestimates sulfate aerosol concentrations in August and September at cloud altitudes (Sect. 3.1.2, Fig. 5). We explore related uncertainties and their implications in the following sections.
3.3 Uncertainties
3.3.1 Assessing variations in DMS emission rates and oxidation mechanism on sulfate aerosol formation
The Benguela region has substantial uncertainties in DMS concentrations in surface seawater (Asher et al., 2011; Tortell et al., 2011) and the corresponding emission fluxes owing to the limited availability of biogenic sulfur measurements. To investigate the sensitivity of DMS emission fluxes to changes in surface seawater DMS concentrations, we conducted two simulations with DMS concentrations from Lana et al. (2011) and Galí et al. (2018) (see Sect. 2.1). The standard results presented thus far were conducted using the Lana dataset.
In the southern Benguela region, south of approximately 27° S, marked upwelling during the austral summer (Shannon and Nelson, 1996; Hutchings et al., 2009) promotes phytoplankton growth and elevates DMS emissions. Figure 10 indicates that the Lana dataset aligns with this phenomenon, displaying peak-DMS-emission fluxes over the Sc sub-domain in January. However, it lacks clear seasonality for the remaining months. In contrast, satellite-based DMS estimates from Galí show pronounced emissions throughout the austral summer (JFND), as shown in Fig. 10. Both datasets concur in magnitude for January and February, a period with better data coverage in the Lana et al. (2011) climatological dataset over the domain. However, the Lana dataset DMS emissions are up to 38 % lower during December, while they are 51 % higher in July relative to the Galí dataset. This suggests the marine contribution to sulfate in our standard simulation using the Lana dataset may be underestimated from October through December (encompassing 2 months of the peak DMS season) and overestimated from March through August (Fig. 10).
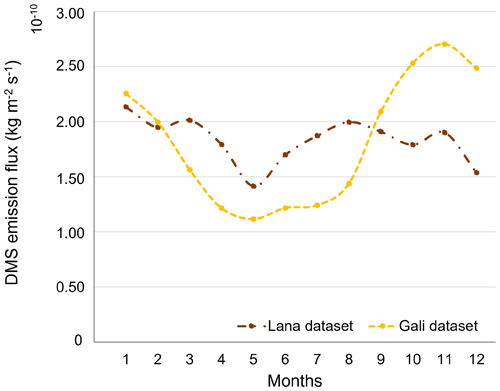
Figure 10Monthly DMS emissions over the stratocumulus sub-domain (0–35° S, 20° E–20° W) using two distinct datasets for surface seawater DMS concentrations. The dashed brown line presents emissions calculated using the Lana et al. (2011) climatology, which compiles data across 1972–2009 from multiple sources. In contrast, the dashed yellow line depicts emissions based on satellite-derived estimates of surface seawater DMS concentrations (Galí et al., 2018).
The ongoing discovery of complexities within DMS oxidation mechanisms, along with the incomplete incorporation of these mechanisms into atmospheric chemistry models, further contributes to uncertainties in predicting the impact of DMS emissions on aerosols and climate (Faloona, 2009; Quinn and Bates, 2011; Carslaw et al., 2013). Chen et al. (2018) highlighted the impacts of changes to DMS chemistry in the GEOS-Chem model, integrating a series of multiphase sulfur oxidation mechanisms and two DMS intermediates, which led to a decrease in the global DMS burden, thereby decreasing SO2 and sulfate levels. On the other hand, Novak et al. (2021) found that the cloud uptake of hydroperoxymethyl thioformate (HPMTF), a newly identified oxidation product of DMS (Wu et al., 2015; Veres et al., 2020), lowers near-surface SO2 concentration while elevating sulfate concentration in the model. Most recently, Tashmim et al. (2024) implemented an advanced DMS oxidation mechanism in GEOS-Chem that incorporates the latest developments in DMS chemistry, including those previously mentioned, which led to a lower SO2 mixing ratio (∼ 70 %) and a higher SO4 mixing ratio (∼ 35 %) over the SEA during austral summer. Thus, an improved representation of DMS emissions and oxidation chemistry in the model could enhance the sulfate aerosol estimations during the peak DMS season. This refinement may address model underestimates of aerosol concentrations during this period (Sect. 3.1.1).
3.3.2 Exploring the impact of marine organic aerosol emissions on organic aerosol concentrations
Beyond marine sulfate and sea-salt aerosols, organic matter also makes a significant contribution to marine aerosol mass (Middlebrook et al., 1998; Oppo et al., 1999; Russell et al., 2010). Notably, substantial concentrations of organic carbon aerosols have been observed in marine regions, particularly during periods of intense biological activity (O'Dowd et al., 2004). These aerosols can also increase CCN, affecting cloud properties and radiative balance (Arnold et al., 2009; Gantt and Meskhidze, 2013). However, the standard GEOS-Chem model does not account for these marine organic aerosol emissions. Here, we analyzed the impact of marine POA on cloud-altitude aerosols over the SEA by incorporating marine POA emissions based on satellite-derived chlorophyll a concentrations (Gantt et al., 2015; See Sect. 2.1) in the model.
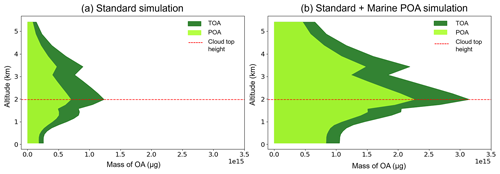
Figure 11Vertical distribution of organic aerosol mass during November 2017, the month of maximum discrepancy between the standard and MPOA simulations, over the Sc sub-domain (0–35° S, 20° E–20° W). Mass profile for total organic aerosols (TOA) and primary organic aerosols (POA) (a) under standard simulation conditions (standard) and (b) when marine primary organic aerosol (MPOA) emissions are included (standard + MPOA). The dashed red line indicates the typical maximum cloud top height.
We find that the inclusion of MPOA emissions consistently resulted in higher organic aerosol mass, with the greatest increase in November. Figure 11 shows the vertical distribution of total organic aerosol (TOA) mass and POA mass (including MPOA and other POA sources) with and without MPOA emissions during this month. Similar to our earlier vertical profile analysis (refer to Sect. 3.2.2), we find that the maximum organic aerosol mass occurred at the highest cloud top height (2 km). The standard + MPOA-simulated peak total organic aerosol mass was approximately 3 times higher than that in the standard simulation, highlighting the potential contribution of marine sources to total organic aerosol mass concentrations. However, during the biomass burning season, the sensitivity simulation showed only a minimal increase, indicating that it does not adequately address the model's underestimation (refer to Fig. 5). Gantt et al. (2015) demonstrated that including MPOA emissions in GEOS-Chem reduced the normalized mean bias (NMB) of surface organic aerosol concentrations at coastal sites by 67 %. Additionally, Pai et al. (2020) noted that without a marine POA source, the model fails to accurately reproduce lower-tropospheric concentrations over oceans, although the marine POA scheme might be biased high. Despite the limitations of a chlorophyll-based parameterization like the one used here in providing a mechanistic understanding of the seasonal and geographical variability of organic matter emissions from sea spray (Burrows et al., 2022), our findings suggest that MPOA may play a role in aerosol–cloud interactions outside of the biomass burning season, in addition to marine-derived sulfate from DMS (Sect. 3.2).
3.3.3 Impacts of uncertainties in biomass burning emissions of SO2
To assess the impact of uncertainty in biomass burning emissions of SO2 on the relative contribution of marine vs. land sources to aerosol, we performed a sensitivity analysis using two alternative inventories, QFED and GFAS (see Sect. 2.1 and Fig. A3). The standard simulations, as detailed in Sect. 2.3, use the default biomass burning inventory in GEOS-Chem, GFED. The GFAS inventory SO2 and CO emissions over the domain are constant in time, aligning with QFED during the non-biomass-burning months (Fig. A3). We find that CO emissions from GFED and QFED align closely; however, there is a notable difference in SO2 emissions between the two inventories (Fig. A3). These discrepancies likely originate from variations in SO2 emission factors employed by each inventory. In July, which exhibits the largest difference between the two inventories, peak SO2 emissions in QFED are almost 5-fold higher than those in GFED. This discrepancy leads to a 25 % increase in sulfate aerosol concentrations at cloud altitudes relative to the standard results using GFED (not shown). Consequently, the contribution of marine sulfate to total sulfate (see Sect. 3.2.1) may further decrease during the peak-biomass-burning season if QFED is used, highlighting the sensitivity of aerosol source attributions to the selected biomass burning inventory.
In this study, monthly marine sulfate constitutes between 10.3 % and 57.7 % of total sulfate within the cloud height, peaking during the high-DMS-emission period. However, the default Lana et al. (2011) climatology largely underestimates DMS emissions during the austral summer (November and December) by up to 38 %, compared to the satellite-derived estimates from Galí et al. (2018). Moreover, improvement of DMS chemistry in the model by incorporating new oxidation mechanisms and intermediate products could shift the balance towards increased sulfate aerosol production (with Tashmim et al., 2024, suggesting an increase of up to 35 % over the SEA). Marine primary organic aerosol emissions may also contribute substantially to the organic aerosol mass during the peak-primary-production period (JNFD), highlighting the importance of marine contributions to overall aerosol concentrations. Meanwhile, discrepancies in SO2 emissions from biomass burning can increase sulfate aerosol from biomass burning by up to 25 %. These changes would improve the model underestimate of AOD relative to AERONET observations; however, observations of aerosol composition outside of August–September are very limited, and this is a large gap. Our results suggest marine-sourced sulfate and organics significantly influence aerosol loading and composition in the SEA, particularly during the non-biomass-burning period. Accurately characterizing the seasonal dynamics of aerosols within cloud heights is imperative for quantifying aerosol–cloud interactions and understanding the dynamics of marine aerosols in the SEA region, where uncertainties in aerosol radiative forcing are most pronounced. This understanding is essential for improving the reliability of climate models in areas critical to both regional and global climate dynamics.
Aerosols over the southeast Atlantic strongly influence global climate dynamics due to the presence of persistent stratocumulus clouds and large uncertainties in aerosol–cloud interactions. However, precisely representing these interactions in global climate models remains challenging, in part due to sparse available observations, especially outside of the biomass burning season. In this study, we employ the GEOS-Chem chemical transport model to assess the aerosol composition at cloud-relevant altitudes (0–2 km) and identify the sensitivities to marine emissions and chemistry in the southeast Atlantic. This analysis aims to enhance our understanding of the role of marine aerosols and the associated uncertainties affecting aerosol–cloud interactions within this climate-sensitive region.
We performed nested grid simulations with a 0.5° × 0.625° horizontal resolution and evaluated the model against ground-based and aircraft campaign observations throughout 2017. We analyzed results for three seasonal periods with distinct dominant processes including (a) the high-DMS-emission season (JFND), (b) the peak-biomass-burning season (JASO), and (c) the transitional season (MAMJ). Our analysis showed that simulated monthly average aerosol optical depth (AOD) exhibits the strongest correlation (R= 0.901) with the AERONET AOD observations during the JASO season. However, the model generally underestimates AOD throughout the year, except in the JFND period, in which an overestimate at the Gobabeb site offsets underestimations at other sites. Moreover, a comparison of aerosol speciation measured at Ascension Island during the LASIC campaign indicates that the model consistently underestimates sulfate aerosols. We further evaluated the simulated vertical profile of aerosol mass concentrations and composition against measurements from the ORACLES and CLARIFY campaigns. These comparisons showed that sulfate aerosols were underestimated by 19 % at cloud-relevant altitudes of 0–2 km by both campaigns. The underestimate of sulfate aerosols at lower altitudes (0–2 km), coupled with an underestimate of other aerosols at higher altitudes (4–8 km), likely contributes to the overall low bias in modeled AOD. The misrepresentation of natural aerosol emissions and transatlantic aerosol transport may be responsible for these underestimates. Nevertheless, discrepancies increase with altitude, reflecting challenges in accurately modeling high-altitude aerosol concentrations.
Analysis of seasonal mean aerosol composition at cloud height showed that organic aerosols predominate during JASO (63 %) and MAMJ (51 %), while sulfate aerosols are most prevalent (41 %) during the austral summer (JFND). Given the prominence of sulfate as a marine-sourced aerosol in remote oceanic environments, we investigated the processes influencing the sulfate aerosol concentrations in our domain. Throughout the year, anthropogenic sources and oceanic DMS emissions are the primary atmospheric sulfur contributors. Spatial mapping across the sub-domain (0–35° S, 20° E–20° W) showed high sulfate concentrations (up to 3 µg m−3) at cloud height during the peak-biomass-burning season (JASO), primarily from savannah fires in southern Africa. Despite this, sulfate aerosols only account for 18 % of the total aerosol mass in JASO.
Sulfate, primarily from marine sources, is the dominant aerosol at cloud-relevant altitudes during JFND in the model (up to 69 % marine contribution); however, significant uncertainties regarding the treatment of DMS that may affect this finding persist. To assess the impact of these uncertainties on sulfate aerosols, we compared DMS emission fluxes from Lana et al. (2011) climatological data, which have limited spatial and temporal coverage in our domain, with those from Galí et al. (2018), which are based on satellite-based estimates of surface seawater DMS concentrations. We find that, within our domain, the Lana dataset emission estimates are 51 % higher in July and 38 % lower in December compared to the Galí dataset. Moreover, improvement of DMS chemistry in the model by incorporating new oxidation mechanisms and intermediate products could shift the balance towards increased sulfate aerosol production (with Tashmim et al., 2024, suggesting an increase of up to 35 % over the SEA). Additionally, emissions of marine primary organic aerosols during the peak-primary-production period (JNFD) may substantially contribute to the mass of organic aerosols, which can also act as CCN. This emphasizes the critical role of marine sources in influencing aerosol concentrations, even in oceanic regions impacted by large seasonal biomass burning. Variations in SO2 emissions from biomass burning could potentially increase sulfate aerosol concentrations at cloud altitudes by up to 25 %. Addressing these discrepancies is essential for improving the model's underestimation of AOD and aerosol concentrations compared to observations.
This study highlights the importance of constraining marine emissions and their chemical transformations by incorporating satellite-retrieved datasets and extending field campaign efforts during non-biomass-burning periods. Such initiatives are essential to accurately characterize seasonal aerosol dynamics at cloud heights and to improve our understanding of aerosol–cloud interactions in regions with persistent low-altitude clouds. These advancements could substantially minimize uncertainties in model estimates of radiative forcing and enhance the reliability of climate model projections in the southeast Atlantic region.
Table A2AERONET site information and the monthly average AOD550 value (±1 standard deviation) for three distinct time periods per site are shown. NA: not available
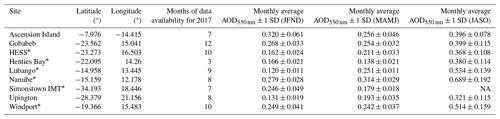
* An asterisk (first column) indicates that the mean AOD during the biomass burning season (JASO) at these sites is more than 1 standard deviation higher than the mean AOD in other season(s) (JFND and MAMJ).
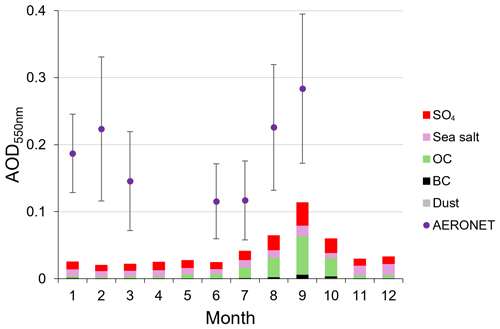
Figure A1Comparative analysis of aerosol optical depth at 550 nm (AOD550 nm) for Ascension Island in 2017. The purple dots present the measured mean monthly AOD values, with vertical error bars illustrating the range of AOD550 nm measurements captured by the AERONET ground station. The stacked bars represent the GEOS-Chem model's simulated AOD values, with each layer corresponding to the major aerosol components, such as sulfate (SO4), sea salt, organic carbon (OC), black carbon (BC), and dust, providing insight into the model's aerosol composition representation.
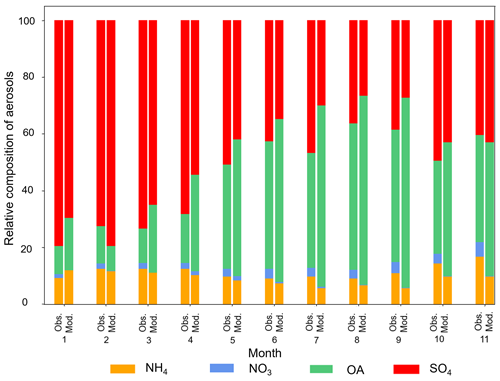
Figure A2Comparative analysis of the relative aerosol composition at Ascension Island in 2017. The stacked bars on the left depict observations of chemical composition taken during the LASIC campaign at the ARM facility on Ascension Island, utilizing an aerosol chemical speciation monitor (ACSM) at 341 m. The bars on the right illustrate the GEOS-Chem simulated aerosol composition at the same coordinates. Each segment of the stack represents different aerosol components: ammonium (NH4), nitrates (NO3), organic aerosols (OAs), and sulfate (SO4).
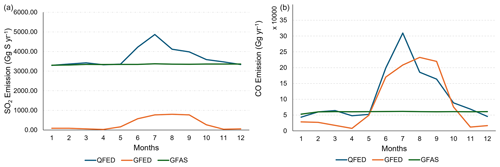
Figure A3Comparison of biomass burning emissions across various inventories, namely GFED, QFED, and GFAS across the domain (0–40° S, 40° E–20° W). The panels depict the interannual variability of biomass burning emissions, with panel (a) illustrating sulfur dioxide (SO2) emissions and panel (b) displaying carbon monoxide (CO) emissions. Both GFED and QFED indicate similar emission trends; however, GFED exhibits lower SO2 emission magnitudes compared to QFED. GFAS presents emission magnitudes similar to QFED during the non-biomass-burning period.
The GEOS-Chem model used here is publicly available at https://doi.org/10.5281/zenodo.5748260 (The International GEOS-Chem User Community, 2021). The ORACLES campaign data from 2017 are available at https://doi.org/10.5067/Suborbital/ORACLES/P3/2017_V2 (ORACLES Science Team, 2020). The CLARIFY campaign data are available at http://data.ceda.ac.uk/badc/faam/data/2017/c056-sep-09 (last access: 22 June 2024, CEDA, 2021). The ACSM dataset from LASIC campaign is available at https://doi.org/10.5439/1763029 (Atmospheric Radiation Measurement (ARM) user facility, 2016).
MH, HMH, and RMG designed the research. MH conducted the model simulations, analysis, and visualization, with expert advice from HMH. MH drafted the manuscript, which was then revised by all co-authors.
At least one of the (co-)authors is a member of the editorial board of Atmospheric Chemistry and Physics. The peer-review process was guided by an independent editor, and the authors also have no other competing interests to declare.
Publisher’s note: Copernicus Publications remains neutral with regard to jurisdictional claims made in the text, published maps, institutional affiliations, or any other geographical representation in this paper. While Copernicus Publications makes every effort to include appropriate place names, the final responsibility lies with the authors.
This article is part of the special issue “New observations and related modelling studies of the aerosol–cloud–climate system in the Southeast Atlantic and southern Africa regions (ACP/AMT inter-journal SI)”. It is not associated with a conference.
Mashiat Hossain and Hannah M. Horowitz gratefully acknowledge Michael Diamond for discussions on cloud-relevant altitudes over the southeast Atlantic region.
This paper was edited by Lynn M. Russell and reviewed by two anonymous referees.
Adebiyi, A. A. and Zuidema, P.: The role of the southern African easterly jet in modifying the southeast Atlantic aerosol and cloud environments, Q. J. Roy., 142, 1574–1589, https://doi.org/10.1002/qj.2765, 2016.
Adebiyi, A. A., Zuidema, P., and Abel, S. J.: The convolution of dynamics and moisture with the presence of shortwave absorbing aerosols over the southeast Atlantic, J. Climate, 28, 1997–2024, https://doi.org/10.1175/JCLI-D-14-00352.1, 2015.
Adebiyi, A. A., Akinsanola, A. A., and Ajoku, O. F.: The Misrepresentation of the Southern African Easterly Jet in Models and Its Implications for Aerosol, Clouds, and Precipitation Distributions, J. Climate, 36, 7785–7809, https://doi.org/10.1175/JCLI-D-23-0083.1, 2023.
Alexander, B., Park, R. J., Jacob, D. J., and Gong, S.: Transition metal-catalyzed oxidation of atmospheric sulfur: Global implications for the sulfur budget. J. Geophys. Res., 114, D02309, https://doi.org/10.1029/2008JD010486, 2009.
Andreae, M. O.: Ocean-atmosphere interactions in the global biogeochemical sulfur cycle, Mar. Chem., 30, 1–29, https://doi.org/10.1016/0304-4203(90)90059-L, 1990.
Andreae, M. O., Elbert, W., and De Mora, S. J.: Biogenic sulfur emissions and aerosols over the tropical South Atlantic. 3. Atmospheric dimethylsulfide, aerosols and cloud condensation nuclei, J. Geophys. Res., 100, 11335–11356, https://doi.org/10.1029/94jd02828, 1995.
Arnold, S. R., Spracklen, D. V., Williams, J., Yassaa, N., Sciare, J., Bonsang, B., Gros, V., Peeken, I., Lewis, A. C., Alvain, S., and Moulin, C.: Evaluation of the global oceanic isoprene source and its impacts on marine organic carbon aerosol, Atmos. Chem. Phys., 9, 1253–1262, https://doi.org/10.5194/acp-9-1253-2009, 2009.
Arowosegbe, O. O., Röösli, M., Adebayo-Ojo, T. C., Dalvie, M. A., and de Hoogh, K.: Spatial and Temporal Variations in PM10 Concentrations between 2010–2017 in South Africa, Int. J. Env. Res. Pub. He., 18, 1–12, https://doi.org/10.1016/j.envpol.2022.119883, 2021.
Asher, E.C., Merzouk, A., and Tortell, P. D.: Fine-scale spatial and temporal variability of surface water dimethylsufide (DMS) concentrations and sea–air fluxes in the NE Subarctic Pacific, Mar. Chem., 126, 63–75, https://doi.org/10.1016/j.marchem.2011.03.009, 2011.
Atmospheric Radiation Measurement (ARM) user facility: ACSM, corrected for composition-dependent collection efficiency (ACSMCDCE), ARM Data Center [data set], https://doi.org/10.5439/1763029, 2016.
Barnes, I., Hjorth, J., and Mihalapoulos, N.: Dimethyl sulfide and dimethyl sulfoxide and their oxidation in the atmosphere, Chem. Rev., 106, 940–975, https://doi.org/10.1021/cr020529, 2006.
Barrett, P. A., Abel, S. J., Coe, H., Crawford, I., Dobracki, A., Haywood, J., Howell, S., Jones, A., Langridge, J., McFarquhar, G. M., Nott, G. J., Price, H., Redemann, J., Shinozuka, Y., Szpek, K., Taylor, J. W., Wood, R., Wu, H., Zuidema, P., Bauguitte, S., Bennett, R., Bower, K., Chen, H., Cochrane, S., Cotterell, M., Davies, N., Delene, D., Flynn, C., Freedman, A., Freitag, S., Gupta, S., Noone, D., Onasch, T. B., Podolske, J., Poellot, M. R., Schmidt, S., Springston, S., Sedlacek III, A. J., Trembath, J., Vance, A., Zawadowicz, M. A., and Zhang, J.: Intercomparison of airborne and surface-based measurements during the CLARIFY, ORACLES and LASIC field experiments, Atmos. Meas. Tech., 15, 6329–6371, https://doi.org/10.5194/amt-15-6329-2022, 2022.
Bates, T. S., Lamb, B. K., Guenther, A., Dignon, J., and Stoiber, R. E.: Sulfur emissions to the atmosphere from natural sources, J. Atmos. Chem., 14, 1–4, https://doi.org/10.1007/BF00115242, 1992.
Brooks, S. D. and Thornton, D. C. O.: Marine aerosols and clouds, Annu. Rev. Mar. Sci., 10, 289–313, https://doi.org/10.1146/annurev-marine-121916-063148, 2018.
Burkholder, J. B., Sander, S. P., Abbatt, J., Barker, J. R., Huie, R. E., Kolb, C. E., Kurylo, M. J., Orkin, V. L., Wilmouth, D. M., and Wine, P. H.: Chemical Kinetics and Photochemical Data for Use in Atmospheric Studies, Evaluation No. 18, JPL Publication 15-10, Jet Propulsion Laboratory, Pasadena, http://jpldataeval.jpl.nasa.gov (last access: 10 May 2024), 2015.
Burrows, S. M., Easter, R. C., Liu, X., Ma, P.-L., Wang, H., Elliott, S. M., Singh, B., Zhang, K., and Rasch, P. J.: OCEANFILMS (Organic Compounds from Ecosystems to Aerosols: Natural Films and Interfaces via Langmuir Molecular Surfactants) sea spray organic aerosol emissions – implementation in a global climate model and impacts on clouds, Atmos. Chem. Phys., 22, 5223–5251, https://doi.org/10.5194/acp-22-5223-2022, 2022.
Carslaw, K. S., Lee, L. A., Reddington, C. L., Pringle, K. J., Rap, A., Forster, P. M., Mann, G. W., Spracklen, D. V., Woodhouse, M. T., Regayre, L. A., and Pierce, J. R.: Large contribution of natural aerosols to uncertainty in indirect forcing, Nature, 503, 67–71, https://doi.org/10.1038/nature12674, 2013.
Chen, H., Ezell, M. J., Arquero, K. D., Varner, M. E., Dawson, M. L., Gerber, R. B., and Finlayson-Pitts, B. J.: New particle formation and growth from methanesulfonic acid, trimethylamine and water, Phys. Chem. Chem. Phys., 17, 13699–13709, https://doi.org/10.1039/c5cp00838g , 2015.
Chen, Q., Sherwen, T., Evans, M., and Alexander, B.: DMS oxidation and sulfur aerosol formation in the marine troposphere: a focus on reactive halogen and multiphase chemistry, Atmos. Chem. Phys., 18, 13617–13637, https://doi.org/10.5194/acp-18-13617-2018, 2018.
Chen, Y. C., Christensen, M. W., Stephens, G. L., and Seinfeld, J. H.: Satellite-based estimate of global aerosol-cloud radiative forcing by marine warm clouds, Nat. Geosci., 7, 643–646, https://doi.org/10.1038/ngeo2214, 2014.
CEDA: FAAM C056 CLARIFY flight: Airborne atmospheric measurements from core instrument suite on board the BAE-146 aircraft, CEDA [data set], http://data.ceda.ac.uk/badc/faam/data/2017/c056-sep-09 (last access: 22 June 2024), 2021.
Chin, M., Jacob, D. J., Gardner, G. M., Foreman-Fowler, M. S., Spiro, P. A., and Savoie, D. L.: A global three-dimensional model of tropospheric sulfate, J. Geophys. Res., 101, 18667–18690, https://doi.org/10.1029/96jd01221, 1996.
Dang, C., Segal-Rozenhaimer, M., Che, H., Zhang, L., Formenti, P., Taylor, J., Dobracki, A., Purdue, S., Wong, P.-S., Nenes, A., Sedlacek III, A., Coe, H., Redemann, J., Zuidema, P., Howell, S., and Haywood, J.: Biomass burning and marine aerosol processing over the southeast Atlantic Ocean: a TEM single-particle analysis, Atmos. Chem. Phys., 22, 9389–9412, https://doi.org/10.5194/acp-22-9389-2022, 2022.
Darmenov, A, and da Silva, A. M.: The Quick Fire Emissions Dataset (QFED) – Documentation of versions 2.1, 2.2 and 2.4, NASA Tech. Rep. Ser. Glob. Model. Data Assim. NASA TM-2013-104606, 32, 183 pp., https://ntrs.nasa.gov/citations/20180005253 (last access: 21 May 2024), 2013.
Das, S., Harshvardhan, H., Bian, H., Chin, M., Curci, G., Protonotariou, A. P., Mielonen, T., Zhang, K., Wang, H., and Liu, X.: Biomass burning aerosol transport and vertical distribution over the South African-Atlantic region, J. Geophys. Res., 122, 6391–6415, https://doi.org/10.1002/2016JD026421, 2017.
Diamond, M. S., Dobracki, A., Freitag, S., Small Griswold, J. D., Heikkila, A., Howell, S. G., Kacarab, M. E., Podolske, J. R., Saide, P. E., and Wood, R.: Time-dependent entrainment of smoke presents an observational challenge for assessing aerosol–cloud interactions over the southeast Atlantic Ocean, Atmos. Chem. Phys., 18, 14623–14636, https://doi.org/10.5194/acp-18-14623-2018, 2018.
Doherty, S. J., Saide, P. E., Zuidema, P., Shinozuka, Y., Ferrada, G. A., Gordon, H., Mallet, M., Meyer, K., Painemal, D., Howell, S. G., Freitag, S., Dobracki, A., Podolske, J. R., Burton, S. P., Ferrare, R. A., Howes, C., Nabat, P., Carmichael, G. R., da Silva, A., Pistone, K., Chang, I., Gao, L., Wood, R., and Redemann, J.: Modeled and observed properties related to the direct aerosol radiative effect of biomass burning aerosol over the southeastern Atlantic, Atmos. Chem. Phys., 22, 1–46, https://doi.org/10.5194/acp-22-1-2022, 2022.
Fairlie, T. D., Jacob, D. J., and Park, R. J.: The impact of transpacific transport of mineral dust in the United States, Atmos. Environ., 41, 1251–1266, https://doi.org/10.1016/j.atmosenv.2006.09.048, 2007.
Faloona, I.: Sulfur processing in the marine atmospheric boundary layer: A review and critical assessment of modeling uncertainties, Atmos. Environ., 43, 2841–2854, https://doi.org/10.1016/j.atmosenv.2009.02.043, 2009.
Formenti, P., Piketh, S. J., and Annegarn, H. J.: Detection of non-sea salt sulphate aerosol at a remote coastal site in South Africa: a PIXE study, Nucl. Instrum. Meth. B, 150, 332–338, https://doi.org/10.1016/S0168-583X(98)01041-6, 1999.
Formenti, P., D'Anna, B., Flamant, C., Mallet, M., Piketh, S. J., Schepanski, K., Waquet, F., Auriol, F., Brogniez, G., Burnet, F., Chaboureau, J., Chauvigné, A., Chazette, P., Denjean, C., Desboeufs, K., Doussin, J., Elguindi, N., Feuerstein, S., Gaetani, M., Giorio, C., Klopper, D., Mallet, M. D., Nabat, P., Monod, A., Solmon, F., Namwoonde, A., Chikwililwa, C., Mushi, R., Welton, E. J., and Holben, B.: The aerosols, radiation and clouds in southern Africa field campaign in Namibia overview, illustrative observations, and way forward, B. Am. Meteorol. Soc., 100, 1277–1298, https://doi.org/10.1175/BAMS-D-17-0278.1, 2019.
Fountoukis, C. and Nenes, A.: ISORROPIA II: a computationally efficient thermodynamic equilibrium model for K+–Ca2+–Mg2+–NH–Na+–SO–NO–Cl−–H2O aerosols, Atmos. Chem. Phys., 7, 4639–4659, https://doi.org/10.5194/acp-7-4639-2007, 2007.
Fung, K. M., Heald, C. L., Kroll, J. H., Wang, S., Jo, D. S., Gettelman, A., Lu, Z., Liu, X., Zaveri, R. A., Apel, E. C., Blake, D. R., Jimenez, J.-L., Campuzano-Jost, P., Veres, P. R., Bates, T. S., Shilling, J. E., and Zawadowicz, M.: Exploring dimethyl sulfide (DMS) oxidation and implications for global aerosol radiative forcing, Atmos. Chem. Phys., 22, 1549–1573, https://doi.org/10.5194/acp-22-1549-2022, 2022.
Galí, M., Levasseur, M., Devred, E., Simó, R., and Babin, M.: Sea-surface dimethylsulfide (DMS) concentration from satellite data at global and regional scales, Biogeosciences, 15, 3497–3519, https://doi.org/10.5194/bg-15-3497-2018, 2018.
Gantt, B. and Meskhidze, N.: The physical and chemical characteristics of marine primary organic aerosol: a review, Atmos. Chem. Phys., 13, 3979–3996, https://doi.org/10.5194/acp-13-3979-2013, 2013.
Gantt, B., Johnson, M. S., Crippa, M., Prévôt, A. S. H., and Meskhidze, N.: Implementing marine organic aerosols into the GEOS-Chem model, Geosci. Model Dev., 8, 619–629, https://doi.org/10.5194/gmd-8-619-2015, 2015.
Gelaro, R., McCarty, W., Suarez, M. J., Todling, R., Molod, A., Takacs, L., Randles, C., Darmenov, A., Bosilovich, M. G., Reichle, R., Wargan, K., Coy, L., Cullather, R., Draper, C., Akella, S., Buchard, V., Conaty, A., da Silva, A., Gu, W., Kim, G. K., Koster, R., Lucchesi, R., Merkova, D., Nielsen, J. E., Partyka, G., Pawson, S., Putman, W., Rienecker, M., Schubert, S. D., Sienkiewicz, M., and Zhao, B.: The modern-era retrospective analysis for research and applications, version 2 (MERRA-2), J. Climate, 30, 5419–5454, https://doi.org/10.1175/JCLI-D-16-0758.1, 2017.
Giles, D. M., Sinyuk, A., Sorokin, M. G., Schafer, J. S., Smirnov, A., Slutsker, I., Eck, T. F., Holben, B. N., Lewis, J. R., Campbell, J. R., Welton, E. J., Korkin, S. V., and Lyapustin, A. I.: Advancements in the Aerosol Robotic Network (AERONET) Version 3 database – automated near-real-time quality control algorithm with improved cloud screening for Sun photometer aerosol optical depth (AOD) measurements, Atmos. Meas. Tech., 12, 169–209, https://doi.org/10.5194/amt-12-169-2019, 2019.
Gong, S. L.: A Parameterization of Sea-salt Aerosol Source Function for Sub- and Super-micron Particles, Global Biogeochem. Cy., 17, 1097, https://doi.org/10.1029/2003GB002079, 2003.
Haywood, J. M., Abel, S. J., Barrett, P. A., Bellouin, N., Blyth, A., Bower, K. N., Brooks, M., Carslaw, K., Che, H., Coe, H., Cotterell, M. I., Crawford, I., Cui, Z., Davies, N., Dingley, B., Field, P., Formenti, P., Gordon, H., de Graaf, M., Herbert, R., Johnson, B., Jones, A. C., Langridge, J. M., Malavelle, F., Partridge, D. G., Peers, F., Redemann, J., Stier, P., Szpek, K., Taylor, J. W., Watson-Parris, D., Wood, R., Wu, H., and Zuidema, P.: The CLoud–Aerosol–Radiation Interaction and Forcing: Year 2017 (CLARIFY-2017) measurement campaign, Atmos. Chem. Phys., 21, 1049–1084, https://doi.org/10.5194/acp-21-1049-2021, 2021.
Hodzic, A., Campuzano-Jost, P., Bian, H., Chin, M., Colarco, P. R., Day, D. A., Froyd, K. D., Heinold, B., Jo, D. S., Katich, J. M., Kodros, J. K., Nault, B. A., Pierce, J. R., Ray, E., Schacht, J., Schill, G. P., Schroder, J. C., Schwarz, J. P., Sueper, D. T., Tegen, I., Tilmes, S., Tsigaridis, K., Yu, P., and Jimenez, J. L.: Characterization of organic aerosol across the global remote troposphere: a comparison of ATom measurements and global chemistry models, Atmos. Chem. Phys., 20, 4607–4635, https://doi.org/10.5194/acp-20-4607-2020, 2020.
Hoesly, R. M., Smith, S. J., Feng, L., Klimont, Z., Janssens-Maenhout, G., Pitkanen, T., Seibert, J. J., Vu, L., Andres, R. J., Bolt, R. M., Bond, T. C., Dawidowski, L., Kholod, N., Kurokawa, J.-I., Li, M., Liu, L., Lu, Z., Moura, M. C. P., O'Rourke, P. R., and Zhang, Q.: Historical (1750–2014) anthropogenic emissions of reactive gases and aerosols from the Community Emissions Data System (CEDS), Geosci. Model Dev., 11, 369–408, https://doi.org/10.5194/gmd-11-369-2018, 2018.
Hoffmann, E. H., Tilgner, A., Schrödner, R., Bräuer, P., Wolke, R., and Herrmann, H.: An advanced modeling study on the impacts and atmospheric implications of multiphase dimethyl sulfide chemistry, P. Natl. Acad. Sci. USA, 113, 11776–11781, https://doi.org/10.1073/pnas.1606320113, 2016.
Holben, B. N., Eck, T. F., Slutsker, I., Tanré, D., Buis, J. P., Setzer, A., Vermote, E., Reagan, J. A., Kaufman, Y. J., Nakajima, T., Lavenu, F., Jankowiak, I., and Smirnov, A.: AERONET - A federated instrument network and data archive for aerosol characterization, Remote Sens. Environ., 66, 1–16, https://doi.org/10.1016/S0034-4257(98)00031-5, 1998.
Hutchings, L., van der Lingen, C. D., Shannon, L. J., Crawford, R. J. M., Verheye, H. M. S., Bartholomae, C. H., van der Plas, A. K., Louw, D., Kreiner, A., Ostrowski, M., Fidel, Q., Barlow, R. G., Lamont, T., Coetzee, J., Shillington, F., Veitch, J., Currie, J. C., and Monteiro, P. M. S.: The Benguela Current: An ecosystem of four components, Prog. Oceanogr., 83, 15–32, https://doi.org/10.1016/j.pocean.2009.07.046, 2009.
Jaeglé, L., Quinn, P. K., Bates, T. S., Alexander, B., and Lin, J.-T.: Global distribution of sea salt aerosols: new constraints from in situ and remote sensing observations, Atmos. Chem. Phys., 11, 3137–3157, https://doi.org/10.5194/acp-11-3137-2011, 2011.
Jaeglé, L., Shah, V., Thornton, J. A., Lopez-Hilfiker, F. D., Lee, B. H., McDuffie, E. E., Fibiger, D., Brown, S. S., Veres, P., Sparks, T. L., Ebben, C. J., Wooldridge, P. J., Kenagy, H. S., Cohen, R. C., Weinheimer, A. J., Campos, T. L., Montzka, D. D., Digangi, J. P., Wolfe, G. M., Hanisco, T., Schroder, J. C., Campuzano-Jost, P., Day, D. A., Jimenez, J. L., Sullivan, A. P., Guo, H., and Weber, R. J.: Nitrogen Oxides Emissions, Chemistry, Deposition, and Export Over the Northeast United States During the WINTER Aircraft Campaign, J. Geophys. Res.-Atmos., 123, 12368–12393, https://doi.org/10.1029/2018JD029133, 2018.
Jarre, A., Hutchings, L., Kirkman, S. P., Kreiner, A., Tchipalanga, P. C. M., Kainge, P., Uanivi, U., van der Plas, A. K., Blamey, L. K., Coetzee, J. C., Lamont, T., Samaai, T., Verheye, H. M., Yemane, D. G., Axelsen, B. E., Ostrowski, M., Stenevik, E. K., and Loeng, H.: Synthesis: Climate effects on biodiversity, abundance and distribution of marine organisms in the Benguela, Fish. Oceanogr., 24, 122–149, https://doi.org/10.1111/fog.12086, 2015.
Kaiser, J. W., Heil, A., Andreae, M. O., Benedetti, A., Chubarova, N., Jones, L., Morcrette, J.-J., Razinger, M., Schultz, M. G., Suttie, M., and van der Werf, G. R.: Biomass burning emissions estimated with a global fire assimilation system based on observed fire radiative power, Biogeosciences, 9, 527–554, https://doi.org/10.5194/bg-9-527-2012, 2012.
Kaufman, Y. J. and Tanré, D.: Effect of variations in super-saturation on the formation of cloud condensation nuclei, Nature, 369, 45–48, https://doi.org/10.1038/369045a0, 1994.
Kilgour, D. B., Novak, G. A., Sauer, J. S., Moore, A. N., Dinasquet, J., Amiri, S., Franklin, E. B., Mayer, K., Winter, M., Morris, C. K., Price, T., Malfatti, F., Crocker, D. R., Lee, C., Cappa, C. D., Goldstein, A. H., Prather, K. A., and Bertram, T. H.: Marine gas-phase sulfur emissions during an induced phytoplankton bloom, Atmos. Chem. Phys., 22, 1601–1613, https://doi.org/10.5194/acp-22-1601-2022, 2022.
Lana, A., Bell, T. G., Simó, R., Vallina, S. M., Ballabrera-Poy, J., Kettle, A. J., Dachs, J., Bopp, L., Saltzman, E. S., Stefels, J., Johnson, J. E., and Liss, P. S.: An updated climatology of surface dimethlysulfide concentrations and emission fluxes in the global ocean, Global Biogeochem. Cy., 25, GB1004, https://doi.org/10.1029/2010GB003850, 2011.
Latimer, R. N. C. and Martin, R. V.: Interpretation of measured aerosol mass scattering efficiency over North America using a chemical transport model, Atmos. Chem. Phys., 19, 2635–2653, https://doi.org/10.5194/acp-19-2635-2019, 2019.
Lee, G., Park, J., Jang, Y., Lee, M., Kim, K. R., Oh, J. R., Kim, D., Yi, H. Il, and Kim, T. Y.: Vertical variability of seawater DMS in the South Pacific Ocean and its implication for atmospheric and surface seawater DMS, Chemosphere, 78, 1063–1070, https://doi.org/10.1016/j.chemosphere.2009.10.054, 2010.
Lindesay, J. A., Andreae, M. O., Goldammer, J. G., Harris, G., Annegarn, H. J., Garstang, M., Scholes, R. J., and Van Wilgen, B. W.: International geosphere-biosphere programme/international global atmospheric chemistry SAFARI-92 field experiment: Background and overview, J. Geophys. Res., 101, 23521–23530, https://doi.org/10.1029/96jd01512, 1996.
Martínez-Lozano, J. A., Utrillas, M. P., Tena, F., and Cachorro, V. E.: The parameterisation of the atmospheric aerosol optical depth using the Ångström power law, Sol. Energy, 63, 303–311, https://doi.org/10.1016/S0038-092X(98)00077-2, 1998.
Marvin, M. R., Palmer, P. I., Yao, F., Latif, M. T., and Khan, M. F.: Uncertainties from biomass burning aerosols in air quality models obscure public health impacts in Southeast Asia, Atmos. Chem. Phys., 24, 3699–3715, https://doi.org/10.5194/acp-24-3699-2024, 2024.
Middlebrook, A. M., Murphy, D. M., and Thomson, D. S.: Observations of organic material in individual marine particles at Cape Grim during the First Aerosol Characterization Experiment (ACE 1), J. Geophys. Res., 103, 16475–16483, https://doi.org/10.1029/97JD03719, 1998.
Novak, G. A., Fite, C. H., Holmes, C. D., Veres, P. R., Neuman, J. A., Faloona, I., Thornton, J. A., Wolfe, G. M., Vermeuel, M. P., Jernigan, C. M., Peischl, J., Ryerson, T. B., Thompson, C. R., Bourgeois, I., Warneke, C., Gkatzelis, G. I., Coggon, M. M., Sekimoto, K., Bui, T. P., Dean-Day, J., Diskin, G. S., DiGangi, J. P., Nowak, J. B., Moore, R. H., Wiggins, E. B., Winstead, E. L., Robinson, C., Thornhill, K. L., Sanchez, K. J., Hall, S. R., Ullmann, K., Dollner, M., Weinzierl, B., Blake, D. R., and Bertram, T. H.: Rapid cloud removal of dimethyl sulfide oxidation products limits SO2 and cloud condensation nuclei production in the marine atmosphere, P. Natl. Acad. Sci. USA, 118, e2110472118, https://doi.org/10.1073/pnas.2110472118, 2021.
O'Dowd, C. D. and De Leeuw, G.: Marine aerosol production: A review of the current knowledge, Philos. T. R. Soc. A., 365, 1753–1774, https://doi.org/10.1098/rsta.2007.2043, 2007.
O'Dowd, C. D., Facchini, M. C., Cavalli, F., Ceburnis, D., Mircea, M., Decesari, S., Fuzzi, S., Young, J. Y., and Putaud, J. P.: Biogenically driven organic contribution to marine aerosol, Nature, 431, 676–680, https://doi.org/10.1038/nature02959, 2004.
Oppo, C., Bellandi, S., Degli Innocenti, N., Stortini, A. M., Loglio, G., Schiavuta, E., and Cini, R.: Surfactant components of marine organic matter as agents for biogeochemical fractionation and pollutant transport via marine aerosols, Mar. Chem., 63, 235–253, https://doi.org/10.1016/S0304-4203(98)00065-6, 1999.
ORACLES Science Team: Suite of Aerosol, Cloud, and Related Data Acquired Aboard P3 During ORACLES 2017, Version 2, Moffett Field, CA, NASA Ames Earth Science Project Office (ESPO) [data set], https://doi.org/10.5067/Suborbital/ORACLES/P3/2017_V2, 2020.
Pai, S. J., Heald, C. L., Pierce, J. R., Farina, S. C., Marais, E. A., Jimenez, J. L., Campuzano-Jost, P., Nault, B. A., Middlebrook, A. M., Coe, H., Shilling, J. E., Bahreini, R., Dingle, J. H., and Vu, K.: An evaluation of global organic aerosol schemes using airborne observations, Atmos. Chem. Phys., 20, 2637–2665, https://doi.org/10.5194/acp-20-2637-2020, 2020.
Park, R. J., Jacob, D. J., Chin, M., and Martin, R. V.: Sources of carbonaceous aerosols over the United States and implications for natural visibility, J. Geophys. Res., 108, 4355, https://doi.org/10.1029/2002jd003190, 2003.
Philip, S., Martin, R. V., and Keller, C. A.: Sensitivity of chemistry-transport model simulations to the duration of chemical and transport operators: a case study with GEOS-Chem v10-01, Geosci. Model Dev., 9, 1683–1695, https://doi.org/10.5194/gmd-9-1683-2016, 2016.
Philip, S., Martin, R. V., Snider, G., Weagle, C. L., Van Donkelaar, A., Brauer, M., Henze, D. K., Klimont, Z., Venkataraman, C., Guttikunda, S. K., and Zhang, Q.: Anthropogenic fugitive, combustion and industrial dust is a significant, underrepresented fine particulate matter source in global atmospheric models, Environ. Res. Lett., 12, 044018, https://doi.org/10.1088/1748-9326/aa65a4, 2017.
Prather, K. A., Bertram, T. H., Grassian, V. H., Deane, G. B., Stokes, M. D., DeMott, P. J., Aluwihare, L. I., Palenik, B. P., Azam, F., Seinfeld, J. H., Moffet, R. C., Molina, M. J., Cappa, C. D., Geiger, F. M., Roberts, G. C., Russell, L. M., Ault, A. P., Baltrusaitis, J., Collins, D. B., Corrigan, C. E., Cuadra-Rodriguez, L. A., Ebben, C. J., Forestieri, S. D., Guasco, T. L., Hersey, S. P., Kim, M. J., Lambert, W. F., Modini, R. L., Mui, W., Pedler, B. E., Ruppel, M. J., Ryder, O. S., Schoepp, N. G., Sullivan, R. C., and Zhao, D.: Bringing the ocean into the laboratory to probe the chemical complexity of sea spray aerosol, P. Natl. Acad. Sci. USA, 110, 7550–7555, https://doi.org/10.1073/pnas.1300262110, 2013.
Quinn, P. K. and Bates, T. S.: The case against climate regulation via oceanic phytoplankton sulphur emissions, Nature, 480, 51–56, https://doi.org/10.1038/nature10580, 2011.
Quinn, P. K., Coffman, D. J., Johnson, J. E., Upchurch, L. M., and Bates, T. S.: Small fraction of marine cloud condensation nuclei made up of sea spray aerosol, Nat. Geosci., 10, 674–679, https://doi.org/10.1038/ngeo3003, 2017.
Ravishankara, A. R., Rudich, Y., Talukdar, R., and Barone, S. B.: Oxidation of atmospheric reduced sulphur compounds: Perspective from laboratory studies, Philos. T. R. Soc. Lon. B., 352, 171–182, https://doi.org/10.1098/rstb.1997.0012, 1997.
Redemann, J., Wood, R., Zuidema, P., Doherty, S. J., Luna, B., LeBlanc, S. E., Diamond, M. S., Shinozuka, Y., Chang, I. Y., Ueyama, R., Pfister, L., Ryoo, J.-M., Dobracki, A. N., da Silva, A. M., Longo, K. M., Kacenelenbogen, M. S., Flynn, C. J., Pistone, K., Knox, N. M., Piketh, S. J., Haywood, J. M., Formenti, P., Mallet, M., Stier, P., Ackerman, A. S., Bauer, S. E., Fridlind, A. M., Carmichael, G. R., Saide, P. E., Ferrada, G. A., Howell, S. G., Freitag, S., Cairns, B., Holben, B. N., Knobelspiesse, K. D., Tanelli, S., L'Ecuyer, T. S., Dzambo, A. M., Sy, O. O., McFarquhar, G. M., Poellot, M. R., Gupta, S., O'Brien, J. R., Nenes, A., Kacarab, M., Wong, J. P. S., Small-Griswold, J. D., Thornhill, K. L., Noone, D., Podolske, J. R., Schmidt, K. S., Pilewskie, P., Chen, H., Cochrane, S. P., Sedlacek, A. J., Lang, T. J., Stith, E., Segal-Rozenhaimer, M., Ferrare, R. A., Burton, S. P., Hostetler, C. A., Diner, D. J., Seidel, F. C., Platnick, S. E., Myers, J. S., Meyer, K. G., Spangenberg, D. A., Maring, H., and Gao, L.: An overview of the ORACLES (ObseRvations of Aerosols above CLouds and their intEractionS) project: aerosol–cloud–radiation interactions in the southeast Atlantic basin, Atmos. Chem. Phys., 21, 1507–1563, https://doi.org/10.5194/acp-21-1507-2021, 2021.
Russell, L. M., Hawkins, L. N., Frossard, A. A., Quinn, P. K., and Bates, T. S.: Carbohydrate-like composition of submicron atmospheric particles and their production from ocean bubble bursting, P. Natl. Acad. Sci. USA, 107, 6652–6657, https://doi.org/10.1073/pnas.0908905107, 2010.
Russell, L. M., Moore, R. H., Burrows, S. M., and Quinn, P. K.: Ocean flux of salt, sulfate, and organic components to atmospheric aerosol, Earth-Sci. Rev., 239, 104364, https://doi.org/10.1016/j.earscirev.2023.104364, 2023.
Ryoo, J.-M., Pfister, L., Ueyama, R., Zuidema, P., Wood, R., Chang, I., and Redemann, J.: A meteorological overview of the ORACLES (ObseRvations of Aerosols above CLouds and their intEractionS) campaign over the southeastern Atlantic during 2016–2018: Part 1 – Climatology, Atmos. Chem. Phys., 21, 16689–16707, https://doi.org/10.5194/acp-21-16689-2021, 2021.
Seinfeld, J. H. and Pandis, S. N.: Atmos. Chem. Phys.: From Air Pollution to Climate Change. John Wiley and Sons, Hoboken, ISBN 9781118591505, 2016.
Shannon, L. V. and Nelson, G.: The Benguela: Large Scale Features and Processes and System Variability, in: The South Atlantic, Springer, Berlin, Heidelberg, Germany, https://doi.org/10.1007/978-3-642-80353-6_9, 1996.
Spracklen, D. V., Carslaw, K. S., Kulmala, M., Kerminen, V. M., Sihto, S. L., Riipinen, I., Merikanto, J., Mann, G. W., Chipperfield, M. P., Wiedensohler, A., Birmili, W., and Lihavainen, H.: Contribution of particle formation to global cloud condensation nuclei concentrations, Geophys. Res. Lett., 35, L06808, https://doi.org/10.1029/2007GL033038, 2008.
Stier, P., Schutgens, N. A. J., Bellouin, N., Bian, H., Boucher, O., Chin, M., Ghan, S., Huneeus, N., Kinne, S., Lin, G., Ma, X., Myhre, G., Penner, J. E., Randles, C. A., Samset, B., Schulz, M., Takemura, T., Yu, F., Yu, H., and Zhou, C.: Host model uncertainties in aerosol radiative forcing estimates: results from the AeroCom Prescribed intercomparison study, Atmos. Chem. Phys., 13, 3245–3270, https://doi.org/10.5194/acp-13-3245-2013, 2013.
Su, M., Shi, Y., Yang, Y., and Guo, W.: Impacts of different biomass burning emission inventories: Simulations of atmospheric CO2 concentrations based on GEOS-Chem, Sci. Total Environ., 876, 162825, https://doi.org/10.1016/j.scitotenv.2023.162825, 2023.
Swap, R., Garstang, M., Macko, S. A., Tyson, P. D., Maenhaut, W., Artaxo, P., Kållberg, P., and Talbot, R.: The long-range transport of southern African aerosols to the tropical South Atlantic, J. Geophys. Res., 101, 23777–23791, https://doi.org/10.1029/95jd01049, 1996.
Swap, R. J., Annegarn, H. J., Suttles, J. T., King, M. D., Platnick, S., Privette, J. L., and Scholes, R. J.: Africa burning: A thematic analysis of the Southern African Regional Science Initiative (SAFARI 2000), J. Geophys. Res.-Atmos., 108, 8465, https://doi.org/10.1029/2003jd003747, 2003.
Tashmim, L., Porter, W. C., Chen, Q., Alexander, B., Fite, C. H., Holmes, C. D., Pierce, J. R., Croft, B., and Ishino, S.: Contribution of expanded marine sulfur chemistry to the seasonal variability of dimethyl sulfide oxidation products and size-resolved sulfate aerosol, Atmos. Chem. Phys., 24, 3379–3403, https://doi.org/10.5194/acp-24-3379-2024, 2024.
The International GEOS-Chem User Community: geoschem/GCClassic: GEOS-Chem 13.3.3, Zenodo [code], https://doi.org/10.5281/zenodo.5748260, 2021.
Tortell, P. D., Guéguen, C., Long, M. C., Payne, C. D., Lee, P., and DiTullio, G. R.: Spatial variability and temporal dynamics of surface water pCO2, ΔO and dimethylsulfide in the Ross Sea, Antarctica, Deep-Sea Res. Pt. I, 58, 241-259, https://doi.org/10.1016/j.dsr.2010.12.006, 2011.
Tournadre, J.: Anthropogenic pressure on the open ocean: The growth of ship traffic revealed by altimeter data analysis, Geophys. Res. Lett., 41, 7924–7932, https://doi.org/10.1002/2014GL061786, 2014.
van der Werf, G. R., Randerson, J. T., Giglio, L., Collatz, G. J., Mu, M., Kasibhatla, P. S., Morton, D. C., DeFries, R. S., Jin, Y., and van Leeuwen, T. T.: Global fire emissions and the contribution of deforestation, savanna, forest, agricultural, and peat fires (1997–2009), Atmos. Chem. Phys., 10, 11707–11735, https://doi.org/10.5194/acp-10-11707-2010, 2010.
van der Werf, G. R., Randerson, J. T., Giglio, L., van Leeuwen, T. T., Chen, Y., Rogers, B. M., Mu, M., van Marle, M. J. E., Morton, D. C., Collatz, G. J., Yokelson, R. J., and Kasibhatla, P. S.: Global fire emissions estimates during 1997–2016, Earth Syst. Sci. Data, 9, 697–720, https://doi.org/10.5194/essd-9-697-2017, 2017.
Veres, P. R., Andrew Neuman, J., Bertram, T. H., Assaf, E., Wolfe, G. M., Williamson, C. J., Weinzierl, B., Tilmes, S., Thompson, C. R., Thames, A. B., Schroder, J. C., Saiz-Lopez, A., Rollins, A. W., Roberts, J. M., Price, D., Peischl, J., Nault, B. A., Møller, K. H., Miller, D. O., Meinardi, S., Li, Q., Lamarque, J. F., Kupc, A., Kjaergaard, H. G., Kinnison, D., Jimenez, J. L., Jernigan, C. M., Hornbrook, R. S., Hills, A., Dollner, M., Day, D. A., Cuevas, C. A., Campuzano-Jost, P., Burkholder, J., Paul Bui, T., Brune, W. H., Brown, S. S., Brock, C. A., Bourgeois, I., Blake, D. R., Apel, E. C., and Ryerson, T. B.: Global airborne sampling reveals a previously unobserved dimethyl sulfide oxidation mechanism in the marine atmosphere, P. Natl. Acad. Sci. USA, 117, 4505–4510, https://doi.org/10.1073/pnas.1919344117, 2020.
Vignati, E., De Leeuw, G., and Berkowicz, R.: Modeling coastal aerosol transport and effects of surf-produced aerosols on processes in the marine atmospheric boundary layer, J. Geophys. Res., 106, 20225–20238, https://doi.org/10.1029/2000JD000025, 2001.
Wang, X., Heald, C. L., Ridley, D. A., Schwarz, J. P., Spackman, J. R., Perring, A. E., Coe, H., Liu, D., and Clarke, A. D.: Exploiting simultaneous observational constraints on mass and absorption to estimate the global direct radiative forcing of black carbon and brown carbon, Atmos. Chem. Phys., 14, 10989–11010, https://doi.org/10.5194/acp-14-10989-2014, 2014.
Wizenberg, T., Strong, K., Jones, D. B. A., Lutsch, E., Mahieu, E., Franco, B., and Clarisse, L.: Exceptional wildfire enhancements of PAN, C2H4, CH3OH, and HCOOH over the Canadian high Arctic during August 2017, J. Geophys. Res.-Atmos., 128, e2022JD038052, https://doi.org/10.1029/2022JD038052, 2023.
Wood, R.: Stratocumulus clouds, Mon. Weather Rev., 140, 2373–2423, https://doi.org/10.1175/MWR-D-11-00121.1, 2012.
Wu, R., Wang, S., and Wang, L.: New mechanism for the atmospheric oxidation of dimethyl sulfide. The importance of intramolecular hydrogen shift in a CH3SCH2OO radical, J. Phys. Chem. A, 119, 112–117, https://doi.org/10.1021/jp511616j, 2015.
Yu, F., Luo, G., and Ma, X.: Regional and global modeling of aerosol optical properties with a size, composition, and mixing state resolved particle microphysics model, Atmos. Chem. Phys., 12, 5719–5736, https://doi.org/10.5194/acp-12-5719-2012, 2012.
Zhai, S., Jacob, D. J., Brewer, J. F., Li, K., Moch, J. M., Kim, J., Lee, S., Lim, H., Lee, H. C., Kuk, S. K., Park, R. J., Jeong, J. I., Wang, X., Liu, P., Luo, G., Yu, F., Meng, J., Martin, R. V., Travis, K. R., Hair, J. W., Anderson, B. E., Dibb, J. E., Jimenez, J. L., Campuzano-Jost, P., Nault, B. A., Woo, J.-H., Kim, Y., Zhang, Q., and Liao, H.: Relating geostationary satellite measurements of aerosol optical depth (AOD) over East Asia to fine particulate matter (PM2.5): insights from the KORUS-AQ aircraft campaign and GEOS-Chem model simulations, Atmos. Chem. Phys., 21, 16775–16791, https://doi.org/10.5194/acp-21-16775-2021, 2021.
Zhang, D., Du, L., Wang, W., Zhu, Q., Bi, J., Scovronick, N., Naidoo, M., Garland, R.M. and Liu, Y.: A machine learning model to estimate ambient PM2.5 concentrations in industrialized highveld region of South Africa, Remote Sens. Environ., 266, 112713, https://doi.org/10.1016/j.rse.2021.112713, 2021.
Zuidema, P., Alvarado, M., Chiu, C., de Szoeke, S., Fairall, C., Feingold, G., Freedman, A., Ghan, S., Haywood, J., Kollias, P., Lewis, E., McFarquhar, G., McComiskey, A., Mechem, D., Onasch, T., Redemann, J., Romps, D., Turner, D., Wang, H., Wood, R., Yuter, S., and Zhu, P.: Layered Atlantic Smoke Interactions with Clouds (LASIC) Field Campaign Report, United States, https://www.osti.gov/servlets/purl/1437446 (last access: 7 July 2024), 2018.