the Creative Commons Attribution 4.0 License.
the Creative Commons Attribution 4.0 License.
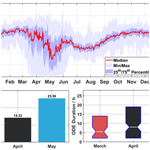
On the dynamics of ozone depletion events at Villum Research Station in the High Arctic
Jens Liengaard Hjorth
Lise Lotte Sørensen
Ozone depletion events (ODEs) occur every spring in the Arctic and have implications for the region's atmospheric oxidizing capacity, radiative balance, and mercury oxidation. Here, we comprehensively analyze ozone, ODEs, and their connection to meteorological and air mass history variables through statistical analyses, back trajectories, and machine learning (ML) at Villum Research Station, Station Nord, Greenland, from 1996 to 2019.
We show that the ODE frequency and duration peak in May, followed by April and March, which is likely related to air masses spending more time over sea ice and increases in radiation from March to May. Back trajectories indicate that, as spring progresses, ODE air masses spend more time within the mixed layer, and the geographic origins move closer to Villum. Positive trends in ODE frequency and duration are observed during May (low confidence) and April (high confidence), respectively. Our analysis revealed that ODEs are favorable under sunny, calm conditions, with air masses arriving from northerly wind directions with sea ice contact.
The ML model was able to reproduce the ODE occurrence and illuminated that radiation, time over sea ice, and temperature were important variables for modeling ODEs during March, April, and May, respectively. Several variables displayed threshold ranges for contributing to the positive prediction of ODEs vs. non-ODEs, notably temperature, radiation, wind direction, time spent over sea ice, and snow on land. Our ML methodology provides a framework for investigating and comparing the environmental drivers of ODEs between different Arctic sites and can be applied to other atmospheric phenomena (e.g., atmospheric-mercury depletion events).
- Article
(7358 KB) - Full-text XML
-
Supplement
(8899 KB) - BibTeX
- EndNote
Globally, ozone is an important constituent of the stratosphere, but it also plays a central role in the tropospheric chemistry. Due to ozone's radiative properties, such as absorption in both the ultraviolet (UV) and infrared (IR) regions, it serves as an important short-lived climate forcer (SLCF). The absorption of UV light by ozone also leads to the formation of an O1D atom, which reacts with water vapor to form hydroxyl (OH) radicals, the most crucial oxidant in the troposphere. Tropospheric-ozone sources include in situ photochemical formation from the catalytic reactions involving nitrogen oxides (NOx) and volatile organic compounds (VOCs), which are initiated by OH but are dependent on the ratio between NOx and VOCs (Seinfeld and Pandis, 2016). Stratosphere–troposphere exchange (STE) represents another significant ozone source (Monks et al., 2015). Sinks of ozone include dry deposition and reactions with NOx, hydrocarbons, and halogens, as well as photolysis-driven loss.
During winter and spring in the Arctic, long-range transport from the mid-latitudes and STE are the major sources of ozone (Helmig et al., 2007a; Hirdman et al., 2010; Stohl, 2006). In the summertime Arctic, low absolute humidity suppresses the formation of OH radicals, and, coupled with low primary emissions of precursor species (VOCs and NOx), in situ formation of ozone is limited (Ianniello et al., 2021; Morin et al., 2008; Pernov et al., 2021). Dry deposition, photolysis, and reactions with halogens are the dominant sinks, while wet deposition is of less importance in the Arctic because of the low humidity and the limited removal efficiency of ozone by precipitating snow and/or ice (Barten et al., 2021).
A phenomenon of the springtime Arctic, known as ozone depletion episodes (ODEs), involves the rapid depletion of ozone due to catalytic reaction with halogen species (X or Y, representing Br, Cl, or I) (Barrie et al., 1988; Simpson et al., 2007b, 2015; Skov et al., 2004). This is shown in Reactions (R1)–(R6).
While ozone is catalytically destroyed by Reactions (R1) to (R3), the number of available halogen atoms is not increased. Multiphase reactions like the halogen explosion sequence (Reactions R1, R2, R4, R5, and R6) accelerate halogen production, leading to high concentrations of ultra-reactive halogen species and causing observed ODEs. These reactions require the presence of a frozen, heterogenous surface aided by high acidity (Sander et al., 2006; Simpson et al., 2007b, 2015).
Moreover, ODEs occur simultaneously with atmospheric-mercury depletion episodes (AMDEs) (Schroeder et al., 1998), and the relative rate principle suggests that ODEs and AMDEs can be explained by competing reactions of ozone and elemental mercury with Br atoms (Skov et al., 2004, 2020), which has recently been demonstrated by direct measurements (Wang et al., 2019). The relative importance of ozone removal through reactions with Br and I atoms in spring is unclear (AMAP, 2015; Benavent et al., 2022; Wang et al., 2019; Whaley et al., 2023). Recently, it was found that Br is the dominant oxidant during spring, whereas I chemistry was active during the entire sunlight period (March to October) (Benavent et al., 2022).
The sources for atmospheric halogens include sea spray aerosols, brine migration through sea ice and the snowpack, blowing snow, and frost flowers (Simpson et al., 2007b, 2015), and the relative importance of the halogen sources depends on the location and time. Sea ice surfaces, aerosol, and frost flowers gained significant interest as halogen sources in earlier investigations. Later studies indicate that frost flowers are of minor importance (Abbatt et al., 2012; Simpson et al., 2007a). Frieß et al. (2004) showed, using trajectory analysis, that areas of first-year sea ice are correlated with high BrO levels, in agreement with later satellite observations for the Arctic (Bougoudis et al., 2020). First-year sea ice is saltier than multi-year ice and is therefore expected to be a greater source of halogens to the atmosphere; however, studies have shown that both first- and multi-year ice are sources of halogens and ODEs (Bognar et al., 2020; Peterson et al., 2019). The recycling of halogens on frozen heterogenous surfaces such as sea salt aerosols and snowpack is also an important source of halogens in polar regions (Custard et al., 2017; Frieß et al., 2023; Peterson et al., 2017, 2018; Pratt et al., 2013; Raso et al., 2017).
Meteorologically, ODEs have usually been associated with sunny conditions and cold temperatures (Simpson et al., 2015). High and low wind speeds have also been connected to ODEs, where high wind speeds generate blowing snow (which is a source of halogens) (Blechschmidt et al., 2016; Bougoudis et al., 2020; Choi et al., 2012; Frieß et al., 2011; Seo et al., 2020; Zhao et al., 2016) and low wind speeds are associated with a stably stratified boundary layer, confining reactants and oxidants to the lowermost atmosphere (Jones et al., 2009). High wind speeds can induce vertical mixing, thus bringing ozone-rich air masses to the surface and terminating ODEs and AMDEs (Moore et al., 2014). Halogen explosion events and ODEs have also been shown to be temperature dependent (Koo et al., 2012; Tarasick and Bottenheim, 2002). This is likely connected to the need for an acidic, frozen heterogeneous surface (sea ice, snowpack, blowing snow, and aerosols) required for halogen propagation (Burd et al., 2017; Jeong et al., 2022), although other studies have not found such evidence (Halfacre et al., 2014; Jacobi et al., 2010).
Despite numerous studies and significant progress in understanding Arctic tropospheric ozone, the dynamics of O3 are still not yet fully understood (Simpson et al., 2015; Whaley et al., 2023), and significant questions remain, including the following: what is the contribution of different halogen sources to ODEs such as sea ice surfaces (multi- vs. first-year ice), snowpack emissions, or recycling on aerosol particles? What are the conducive meteorological conditions for ODEs? What is the contribution of halogen activation aloft vs. in the boundary layer? What is the relative importance of Br and I atoms to ODEs during spring?
The lack of a full understanding of halogen dynamics and the connection to ODEs makes it very important to address the external variables that influence and determine the observed ozone concentrations, especially during ODEs. In the present paper, the connection to meteorological and air mass history variables is studied to cast light on the variables that control ODEs. This is achieved through statistical analyses, back trajectories, and machine learning (ML) applied to ODEs observed at Villum Research Station, Station Nord, northeastern Greenland, from 1996 to 2019.
2.1 Site description
Villum Research Station (Villum) is located on a small peninsula in northeastern Greenland (Fig. 1). The station is located at the Danish military outpost Station Nord (81°36′ N, 16°40′ W; 24 m a.s.l.). Ozone measurements were conducted at Flyger's Hut from 1995 to 2014 and at the Air Observatory from 2014 to present. They are located a few hundred meters apart and 2 km south of the central complex of Station Nord, as well as being upwind of the station the majority of the time (> 95 %). No significant differences in ozone levels were observed when moving measurement locations.
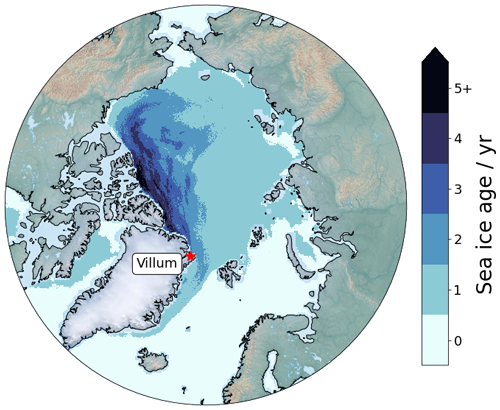
Figure 1Location of Villum Research Station (Villum). Mean sea ice ages for March, April, and May 2007–2019 were taken from the National Sea and Ice Data Center (https://doi.org/10.5067/UTAV7490FEPB, Tschudi et al., 2019). The map background was made with data from Cross Blended Hypso (https://naturalearthdata.com, last access: 1 January 2024). The mean sea ice age for individual spring months closely resembled the spring mean; therefore, the spring mean is displayed for clarity.
2.2 Atmospheric measurements
Sample air was drawn into a 20 cm inner diameter (i.d.) electro-polished stainless-steel sampling line with a protective inlet cap connected to a blower, where the ozone monitors sampled 0.8 L min−1 air. The setup is constructed to avoid ice formation in the sample tube. Ozone is measured based on its absorption of UV light at 254 nm. The original data were averaged to half-hourly mean values and were later reported to EBAS (https://ebas.nilu.no/, last access: 1 July 2022). Here, we use 1 h mean mixing ratios averaged from the native time resolution (15 min). The stability of the instruments is ensured by the addition of known concentrations of ozone from an internal ozone generator traceable to a primary standard; in this way, although different instruments have been employed, all use the same measurement and calibration methods, and, thus, the measurements uncertainties are estimated to remain unchanged. The Department of Environmental Science at Aarhus University is accredited (EN 17025) to measure ozone, but at Villum, it is not possible to maintain the accreditation as visits to the station are not possible frequently enough. However, the instruments are operated as closely as possible to the accreditation procedures. To compensate for the deviations, two monitors are operated in parallel. The uncertainty at a 95 % confidence level (CL) is < 7 % for mixing ratios above 20 and 1.4 ppbv for mixing ratios below 20 ppbv (Skov et al., 2004, 2020).
To quantify the frequency and the duration of ODEs, the parameter “ozone depletion hour” was defined as an hour during which the average ozone mixing ratio was below 10 ppbv, following the definition used by other studies (Halfacre et al., 2014; Koo et al., 2012; Tarasick and Bottenheim, 2002; Yang et al., 2020). In total, 6605 ODE hours were detected. To account for ozone mixing ratios exceeding 10 ppbv during a single hour which was part of a larger depletion event, hours that were below 15 ppbv and the previous and subsequent hours that were below 10 ppbv were also classified as ODEs. This resulted in 57 additional hours being classified as ODEs, which brings the total number of ODEs to 6662, although this additional criteria did not affect the results of this study.
2.3 Meteorological variables
Meteorological data were collected at or near the ozone measurement sites. From 1996 to 2014, measurements of temperature, relative humidity, wind speed, and wind direction were obtained through the Danish Meteorological Institute's weather station located within Station Nord (Jensen, 2022). From 2014 to 2020, measurements of temperature, relative humidity (RH), wind speed, wind direction, and solar radiation were obtained from an automatic weather station located ∼ 44 m from the Air Observatory.
Observations of solar radiation only started in 2014, and input data for ML models require no missing data. To overcome this absence of measurements before 2014 and to extend the input dataset for the ML model to 2007, we supplemented observations with ERA5 reanalysis data (Hersbach et al., 2020). The ERA5 output of “shortwave solar radiation downwards” was used, which is the amount of shortwave downwelling solar radiation (including both direct and diffuse radiation) that reaches the Earth's surface on a horizontal plane. This is the ERA5 equivalent of the output of a pyranometer with a radiation spectrum of 0.2–4 µm (Hogan, 2015). ERA5 originally provided data as an accumulated value in J m−2, but this was converted to W m−2 by dividing the original values by 1 h in seconds (3600 s). Data are at a 0.25° × 0.25° spatial resolution and an hourly temporal resolution. These data were only used to substitute missing data after 2014 and as a replacement for the absence of measurements before 2014 and were not included in the evaluation of the statistical analysis of ODEs and meteorological variables. This approach was only implemented for the machine learning model and not for the statistical analysis of meteorological variables. A comparison of solar radiation measured at Villum and ERA5 data after 2014 is shown in Fig. S8 in the Supplement. Overall, ERA5 agrees quite well with observations, with a Spearman rank correlation coefficient of 0.974, although ERA5 shows a slight underestimation, with a slope of 0.881 (Fig. S8), which is common for ERA5 in the Arctic (Pernov et al., 2024a). ERA5 data were corrected using the slope of the observation–model comparison to avoid change points in the time series, which could affect the results of the machine learning model.
2.4 Back-trajectory analysis
Air mass back trajectories were calculated via the HYSPLIT trajectory model (Draxler and Hess, 1998; Rolph et al., 2017; Stein et al., 2015). Trajectories of 168 h length were calculated, arriving at 50 m above ground level, for every hour from 2007 to 2019. The trajectory starting height of 50 m was selected as a compromise between capturing air masses that are representative of our sampling site due to very low boundary layers in the Arctic (Gryning et al., 2023) and avoiding trajectories intercepting the surface, which can produce unrepresentative trajectories (Stohl, 1998). The trajectory length was chosen to avoid the uncertainty associated with extremely long trajectory calculations while capturing the entire geographic extent of ODE air masses. This trajectory length of 1 week roughly corresponds to the longest observed ODE at Villum during the study period (∼ 6.5 d, Sect. 3.1) and is shorter than the longest observed ODE at a land-based station (9 d at Alert by Strong et al., 2002). Previous studies have shown that ODE air masses can extend over great distances in the Arctic (Halfacre et al., 2014; Peterson et al., 2017); therefore, we selected a trajectory length of 1 week to fully investigate the air mass history of ODEs. Other studies have used trajectory lengths shorter (Bognar et al., 2020; Frieß et al., 2023) or longer (Bottenheim and Chan, 2006; Begoin et al., 2010; Simpson et al., 2018) than 1 week. Trajectories were calculated based on meteorological files from the NCEP/NCAR reanalysis data, which have a resolution of 2.5° in latitude and longitude (Kalnay et al., 1996). The mixed-layer height for each step of each trajectory was output by the HYSPLIT model. Only trajectories corresponding temporally to available ozone measurements were used in this study. To analyze the geographic origins of ODEs, a concentric grid centered around the location of Villum, consisting of 2° × 4° (latitude × longitude) grid cells, was constructed. The normalized trajectory frequency for each grid cell was calculated by counting the number of trajectory steps that were below the mixed-layer height and intersecting each grid cell. This was normalized by the total number of trajectory steps that were below the mixed layer over all grid cells and multiplied by 100 %. This methodology has been utilized by previous studies to systematically analyze the geographic origins of air masses (Dall'Osto et al., 2017, 2018; Frieß et al., 2023; Heslin-Rees et al., 2020; Pernov et al., 2022).
For each trajectory, a surface-type footprint analysis was performed. The underlying surface types used for the surface footprint type analysis were produced by the National Oceanic and Atmospheric Association/National Environmental Satellite, Data, and Information Service (NOAA/NESDIS) Interactive Multisensor Snow and Ice Mapping System (IMS) developed under the direction of the Interactive Processing Branch (IPB) of the Satellite Services Division (SSD). The altitude at each step along the trajectory was compared to the height of the mixed layer. Steps were classified as being above the mixed layer (AML) if the trajectory altitude was above this height. If the trajectory altitude was below this height then the underlying surface type (land without snow, sea, sea ice, or snow on land) was recorded using a polar stereographic map of the Northern Hemisphere classified into 1024 × 1024 24 km grid cells. It is important to note that grid cells classified as sea ice likely contain snow on the surface, although the satellite products used in this study do not differentiate between bare sea ice and snow-covered sea ice, likely due to the similar spectral signatures between sea ice and snow (U.S. National Ice Center, 2008). We opted to keep the original labels from the satellite product for this analysis as we cannot make any definitive statements about the presence of snow on top of sea ice. The reader should keep this in mind when interpreting the results. The time spent over different surfaces is expressed as a percentage of the total trajectory length.
2.5 Trend analysis
A trend analysis of the ODE frequency and duration and of the start, end, and range of ODE days for March, April, and May was performed. The Mann–Kendall test was used to determine the presence of a statistically significant (SS) trend (Kendall, 1948; Mann, 1945), and the Theil–Sen slope estimator was used to calculate the magnitude of the trend slope (Sen, 1968; Theil, 1950) via the 3PW algorithm from Collaud Coen et al. (2020). The 3PW algorithm tests for autocorrelation in the time series as this can affect the results of the Mann–Kendall test; however, no SS autocorrelation was detected. Therefore, these data were not prewhitened.
2.6 Machine learning modeling
In this study, we utilize a supervised, binary classification form of machine learning (ML) to investigate the dynamics of ODEs. The target variable used was the binary label of ODE or non-ODE, defined as ozone mixing ratios above or below 10 ppbv, respectively. The explanatory variables used in the ML model were the meteorological and air mass history variables (RH, wind direction, wind speed, temperature, radiation, pressure, time air masses spent over snow on land, time air masses spent over sea ice, and time air masses spent above the mixed layer). Below, we describe the missing data imputation, the machine learning model, the hyperparameter tuning, the ML explainability approach employed, and the model evaluation metrics.
Before being input into the ML model, missing data were imputed since ML models require no missing data in the input files. We imputed missing data using the median value for the hour of the day for that day of the year. For instance, if a value is missing for hour 12 on the 90th day of the year then this value was imputed using the median of all values from hour 12 on the 90th day of the year from the entire dataset. This imputation approach allows us to account for changes occurring from early to late spring, as well as for diurnal changes, which would otherwise be overlooked if only using a single median for the spring months. This is especially important for variables that drastically change over this short period (e.g., temperature, RH, solar radiation). Table S1 in the Supplement lists the percentage of missing data before imputation for each variable. Wind speed and direction exhibited the highest percentage of missing data, with both missing ∼ 21 %; therefore, data imputation should not adversely affect the results of the ML model. No feature engineering (standardization or normalization) was applied prior to modeling since the initial evaluation metrics were deemed to be sufficiently accurate. No temporal information (Julian day, day of year, hour of day) was included in the input variables.
The XGBoost model was selected as the model used in this study due to its accuracy, computational efficiency, and ability to handle collinearity amongst the input variables, which is important for meteorological variables. XGBoost is an ensemble machine learning algorithm that uses the gradient-boosting methodology on individual decision trees (which are weak learners) and then builds multiple decision trees that are sequentially added (Chen and Guestrin, 2016). This allows for the previous tree's errors to be learned by the next tree, therefore reducing the loss function while obtaining the best prediction. A regularized model formalization is used in the XGBoost model to improve computational efficiency and to prevent overfitting. The xgboost package (v1.6.2) was used, and all ML modeling was implemented in a Python environment (v3.10.2).
Hyperparameter tuning is an essential part of ML which ensures optimal model performance. We utilized a Bayesian approach for exploring the optimum hyperparameter configuration, implemented though the Optuna (Akiba et al., 2019) library (v3.0.3). The hyperparameters, including the range of values explored, and the optimum values are listed in Table S2. This study employed a stratified 70 30 train test split ratio, meaning the test set contained the same proportion of positive labels (i.e., ODEs) as the entire dataset. The purpose of the training set is for the model to learn how to model the data, and the test set is used to evaluate the model's performance on unseen data. The objective of the hyperparameter tuning procedure is to maximize the mean recall score using 10-fold cross-validation. Cross-validation involves splitting the training data into 10 equally sized folds (or groups), training the model using 9 folds and testing the model using the remaining fold. This was repeated 10 times to use each fold as a test set once. The final evaluation metrics were averaged using the arithmetic mean to select the optimal hyperparameters and to make an overall evaluation of the model performance. Tuning was performed for 1000 trials, and the best hyperparameters were selected. Hyperparameter values were sampled using the tree-structured Parzen estimator (TPE) algorithm (Bergstra et al., 2011), and trials were pruned using the Hyperband pruner (Li et al., 2018). The final set of hyperparameters was selected based on the compromise between overall performance (high recall scores) and agreement between the training and test set evaluation metrics using 10-fold cross-validation (prevention of overfitting).
We employed SHapley Additive exPlanations (SHAP) values (Lundberg and Lee, 2017), which are based on Shapely values (Shapley, 1953), to assess the effect of the input variables on the model output. The SHAP approach is a model-agnostic methodology designed to assess input variable importance based on coalitional game theory (Molnar, 2022), where input variables are treated as “players” in a “game” (model framework), with SHAP aiming to assess the players' contributions to the “payout” (model output). For each observation, the SHAP value represents an input variable's marginal contribution over the mean model output when considering all possible combinations of the input variables. SHAP values can be positive or negative, with positive values indicating that a variable is more likely to contribute to an observation being predicted as an ODE, while negative values mean a variable is more likely to contribute to an observation being labeled as a non-ODE. It is important to note that SHAP values do not represent how well the input variables explain the behavior of our target variable in the natural environment but rather how well these variables explain the behavior of our target variable in our model; therefore, SHAP values represent purely statistical relationships. SHAP can produce both local and global explanations, contrarily to other commonly used input variable importance methods (e.g., split count, gain, permutation importance) that only produce an estimate of global importance (Lundberg et al., 2019). The global importance for each feature is calculated as the mean of the absolute SHAP values for said input variable, which gives an overview of the most important variables; however, this does not account for the relationship between the SHAP and the input value (positive or negative relationship, linear or non-linear). Therefore, we assessed the relationship between the SHAP and ambient values by discretizing the ambient values into 15 equally spaced bins and calculated the median and 25th and 75th percentiles for each bin. These two approaches allow for the evaluation of the overall global importance as well as the relationship between ambient and SHAP values for each input variable. The SHAP approach was applied via the shap package (v0.41.0).
The ML model was evaluated using common metrics for a classification model, namely accuracy, recall, and area-under-curve receiver operating characteristics (AUC ROC). The accuracy is the fraction of correctly labeled data, both positive (ODEs) and negative (non-ODEs), compared to the total number of data points (sum of ODEs and non-ODEs) and ranges from 0 to 1. In other words, accuracy is the fraction of correctly predicted observations regardless of the label (ODE vs. non-ODE). The recall (also defined as the true-positive rate or sensitivity) is the fraction of correctly identified positive labels (ODEs identified by the ML model) compared to the total number of positive labels (total number of ODEs) and ranges from 0 to 1. In other words, recall is the fraction of ODEs correctly predicted. The ROC curve displays the performance of a classification model across different decision thresholds and is represented by a plot of the true-positive rate versus the false-positive rate. The AUC ROC is the area underneath the ROC curve and evaluates how well a model can discriminate between positive and negative labels across all decision thresholds (0.5 is the default threshold used in this study). The AUC ROC ranges from 0 to 1, with 0.5 representing random chance and 1 representing a perfect model. The accuracy gives an overview of the model performance for both labels (ODEs vs. non-ODEs), recall gives the model performance only for positive labels (ODEs), and AUC ROC evaluates the model performance over different decision thresholds; together, these three metrics give a comprehensive view of the model's performance. These metrics were implemented using the scikit-learn package (v1.0.2).
3.1 Overview of ozone and ozone depletion events
The seasonal cycle of ozone mixing ratios with the daily median, minimum and maximum, and interquartile range for each day of the year is shown in Fig. 2a. During winter (December–February), ozone mixing ratios are elevated and increase slightly from January to March, displaying maximum daily median ozone values in February. During spring (March–May), ozone mixing ratios are highly variable, with daily minimum values reaching 0 ppbv and with maximum values being observed in April. During summer (June–August), ozone mixing ratios begin to decrease in late June, remain low during July, and begin to increase in August. During autumn (September–November), ozone mixing ratios continue to increase and begin to return to wintertime values in October. A seasonal histogram of ozone mixing ratios is displayed in Fig. 2b. For winter, autumn, and summer, ozone values are normally distributed, with the highest averages being experienced in the order of winter > autumn > summer. Spring experiences a non-parametric distribution and the highest and lowest observed values, as explained above.
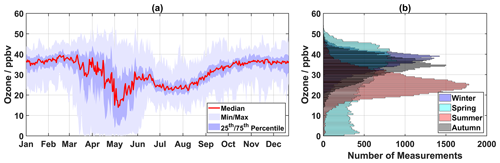
Figure 2Overview of the seasonal cycle and seasonal distribution. (a) Seasonal ozone cycle of the daily median (red line), minimum and maximum (light-blue shading), and interquartile range (blue shading) and (b) histograms of ozone by season (winter in blue: December–February, spring in cyan: March–May, summer in red: June–August, and autumn in gray: September–November).
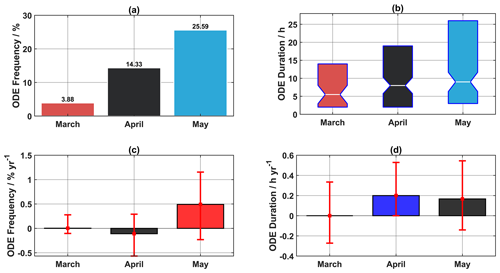
Figure 3Overview of ozone depletion events including (a) bar plots of the frequency of ODEs color-coded by month; (b) boxplots of ODE duration (the white line represents the median, and the colored boxes represent the interquartile range, while the medians of boxes whose notches do not overlap differ with 95 % confidence); (c) trends in ODE frequency; and (d) trends in ODE duration for March, April, and May. The blue, red, and black bars in panels (c) and (d) represent trends that are significant at the > 95th, > 85th, and < 85th % CLs, respectively. The red error bars represent the 95th % confidence intervals (CIs) of the slope. The p values for ODE frequency in March, April, and May are 0.54, 0.75, and 0.14, respectively. The p values for ODE duration in March, April, and May are 0.85, 0.04, and 0.41, respectively.
An overview regarding the frequency and duration of ODEs at Villum is shown in Fig. 3a and b, respectively. ODEs were formally defined in this study as an hourly mean observation with an ozone mixing ratio below 10 ppbv (Halfacre et al., 2014; Koo et al., 2012; Tarasick and Bottenheim, 2002; Yang et al., 2020). The frequency is calculated as the percentage of ODE hours relative to the number of available hourly observations during a month over the study period. The ODE duration is defined as the number of consecutive hours that were classified as ODEs. ODEs are most frequently observed during May, followed by April and March (Fig. 3a). The increase in the ODE frequency from March to April (10.45 %) is similar to the increase from April to May (11.26 %). The distribution (median and interquartile range) of the ODE duration for the spring months is shown in Fig. 3b. The most common duration of ODEs is 1–2 h, with longer ODEs occurring more often in May. The longest ODE occurred during May and lasted 155 h (∼ 6.5 d). For comparison, the longest ODE observed at a ground-based Arctic station was at Alert, Canada, and lasted for 9 d (Strong et al., 2002). Over the central Arctic Ocean, Bottenheim et al. (2009) observed an ODE lasting from 21 April to 23 May 2007. ODEs lasting less than 8 h occurred ∼ 50 % of the time. ODEs lasting more than 1 d (2 d) occurred 21 % (9 %) of the time, respectively. Interestingly, the median of ODE duration between any of the spring months is not significantly different (Fig. 3b). The median ODE duration increases from March (5.5 h) to April (8 h) to May (9 h), while the interquartile range increases more drastically from March to May (Fig. 3b). The diurnal ODE frequencies for each spring month are displayed in Fig. S2; only minor variability is displayed, which is most evident during April.
To investigate changes in the frequency and duration of ODEs, a temporal trend analysis was performed for 1996–2019. Temporal trends of ODE frequency and duration for each month are displayed in Fig. 3c and d, respectively. The slopes of the trends are displayed as boxes (colored by p-value range), with the 95th % confidence intervals (CI) represented by the red error bars. For ODE frequency, no SS trends at the 95th % CL were detected, although May is SS at the 85th % CL (p = 0.14), with a slope [lower CI, upper CI] of 0.49 [−0.23, 1.2] % yr−1. The only SS trend for ODE duration at the 95th % CL (p = 0.039) is during April, with a positive trend of 0.2 [0, 0.53] h yr−1. Temporal trends in the start, end, and range of ODE days for each year were also examined to investigate any changes in the “ODE season”. The first ODE was defined as the first day of the year with an ozone measurement < 10 ppbv, the last ODE day was defined as the last day of the year with an ozone measurement < 10 ppbv, and the range of the ODE days was defined as the difference between the last ODE day of the year and the first ODE day of the year. The results are shown in Fig. S3, and no SS trends at the 95th % CL were found.
3.2 Statistical relationships of ODEs with meteorological and air mass history variables
The relationships between the ODEs, ozone mixing ratios, and meteorological and air mass history variables were investigated. This was accomplished by grouping the meteorological variables into bins and summing the number of ODE hours for each bin, which were normalized by the total number of hours within the same bin, and the median ozone mixing ratio for each bin was calculated for each month separately. The results are shown in Fig. 4, the distributions (median and interquartile range) of these variables for ODEs and non-ODEs are displayed in Fig. 5, and wind roses for ODEs and non-ODEs for the spring months are displayed in Fig. S5. It should be noted that this analysis simply considers the statistical relationship between a given meteorological variable and ozone/ODEs and not the causal relationship. All available data for a given meteorological parameter collocated with ozone measurements were used in this analysis. It should be kept in mind that the air mass history variable time spent over sea ice does not give information about the presence of snow cover; rather, it only gives information about whether or not the underlying surface was classified as sea ice.
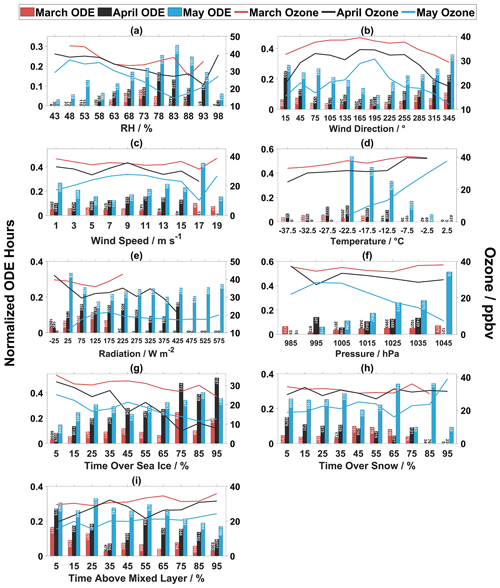
Figure 4Median ozone and normalized ODE hours binned in predefined intervals of (a) RH; (b) wind direction; (c) wind speed; (d) temperature; (e) radiation; (f) pressure; and time air masses spent (g) over sea ice, (h) over snow on land, and (i) above the mixed layer for March, April, and May. The number associated with each bar represents the number of total observations in that bin. All available data for each variable collocated with ozone measurements were used, resulting in different years used for each variable, with the minimum number of years included being 5 for radiation.
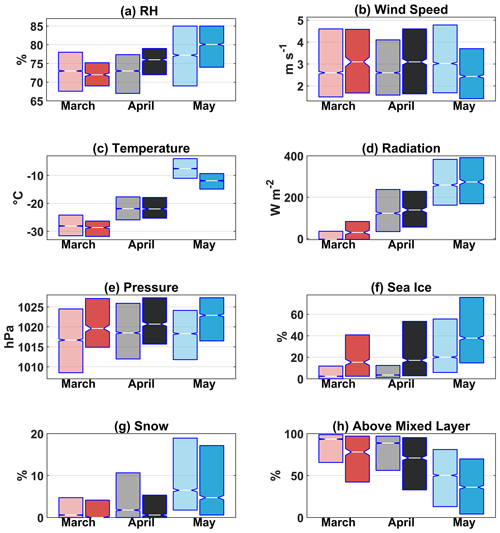
Figure 5Distribution of meteorological and air mass history variables during the spring months for ODEs (dark colors) and non-ODEs (light colors), including (a) RH, (b) wind speed, (c) temperature, (d) radiation, (e) pressure, (f) time over sea ice, (g) time over snow on land, and (h) time above the mixed layer. The line in the middle of the box represents the median, and the boxes represent the interquartile range; the medians of boxes whose notches do not overlap differ with 95 % confidence. For a description of how the time spent over different surface types is calculated, see the “Methods and materials” section. All available data for each variable collocated with ozone measurements were used, resulting in different years being used for each variable, with the minimum number of years included being 5 years for radiation.
For RH, during March, the lowest median ozone mixing ratio and the highest normalized ODE hours are mainly confined to the 65 %–90 % range (midpoints of 68 %–88 %) (Fig. 4a), while lower median ozone mixing ratios occur at higher RH values, which are infrequent. During April and May, lower median ozone mixing and higher normalized ODE hours are observed at higher RH values (75 %–90 %, midpoints of 78 %–88 %) (Fig. 4a). There is little difference between the distributions for RH when comparing ODEs and non-ODEs during March, while for April and May, a consistently higher RH is observed during ODEs (Fig. 5a).
For wind direction, there is a clear effect of northerly wind directions during all spring months, with the lowest median ozone mixing ratios and the highest normalized ODE hours occurring in the 315–45° sector (Fig. 4b). Wind roses for each spring month show a lack of northerly winds for non-ODE periods and wind that arrives more frequently from the north and northwest during ODE periods (Fig. S5).
For wind speed, during March, there is little effect on ozone mixing ratios, and the normalized ODE hours display no discernable pattern across the range of wind speeds (Fig. 4c). The distribution of wind speeds shows a higher median during ODEs compared to non-ODEs (Fig. 5b). During April, the median ozone mixing ratios show little variation with wind speed, although the normalized ODE hours show a tendency for ODEs to occur more often at higher wind speeds (midpoints of 9–15 m s−1); however, these values seldomly occur (Fig. 4c). The distribution of wind speeds during ODEs in April is shifted towards higher values compared to non-ODEs (Fig. 5b). During May, a clearer picture of the effect of wind speed is presented; median ozone mixing ratios and normalized ODE hours show two modes, one at low wind speeds and one at high wind speeds, although it should be noted that the mode at higher wind speeds (midpoints of 15–18 m s−1) seldom occurs (Fig. 4c). Interestingly, during May, the distribution of wind speeds was lower for ODEs compared to non-ODEs (Fig. 5b).
For temperature, median ozone mixing ratios show a slight decreasing pattern for colder temperatures during March and April. The normalized ODE hours showed a slight increase with colder temperatures during March, although, for April, values increased from freezing, peaked in the −25 to −20 °C range (midpoint of −22.5 °C), and decreased thereafter (Fig. 4d). During May, median ozone shows a stark decrease with colder temperatures, and the normalized ODE hours increase sharply with decreasing temperatures. The −25 to −20 °C bin (midpoint of −22.5 °C) displayed the lowest median ozone mixing ratios and the largest normalized ODE hours during May (Fig. 4d). The distribution of temperatures is similar for ODEs compared to non-ODEs during March and April, while ODEs in May experience substantially colder temperatures compared to non-ODEs (Fig. 5c).
For solar radiation, there are large differences in the magnitude between different spring months. During March, median ozone mixing ratios (normalized ODE hours) experienced a minimum (maximum) in the 100 to 150 W m−2 range (midpoint of 125 W m−2). The distribution of solar radiation values is substantially higher during ODEs in March compared to non-ODEs, and the medians are significantly different at the 95th % CL (Fig. 5d). During April, median ozone mixing ratios display a decrease from the lowest bin to the 50 to 100 W m−2 bin (midpoint of 75 W m−2); afterwards, they plateau until the 300 to 350 W m−2 bin (midpoint of 325 W m−2) and finally decrease afterwards, and the normalized ODE hours displayed a similar, yet opposite, pattern (Fig. 4e). During May, median ozone mixing ratios are consistently < 22 ppbv across the range of solar radiation values (Fig. 4e). The normalized ODE hours display a maximum in the 0 to 50 W m−2 bin (midpoint of 25 W m−2), although these values seldom occur, and display similar values afterward.
For pressure, during March and April, there is little variation in the median ozone mixing ratios and normalized ODE hours; however, during May, there is a clear dependency of lower (higher) median ozone mixing ratios (normalized ODE hours) with higher values of atmospheric pressure (Fig. 4f). Interestingly, the distribution of pressure during ODEs is substantially higher compared to during non-ODEs for each spring month, with median values being significantly different at the 95th % CL (Fig. 5e).
For time spent over sea ice, every spring month displays a decreasing (increasing) pattern in terms of median ozone mixing ratios (normalized ODE hours) with increasing time spent over sea ice (Fig. 4g), which supports the results shown earlier for ODEs corresponding to northerly wind directions (Figs. 4b and S5). Trajectories during all spring months consistently spent more time over sea ice during ODEs compared to during non-ODEs (Fig. 5f).
For the time air masses spent over snow on land, no clear impact on median ozone mixing ratios is observed for March and April, while May displays higher ozone mixing ratios for 90 %–100 % of the time spent over snow on land (Fig. 4h). During each spring month, the normalized ODE hours display no discernable pattern over the range of time spent over snow on land (Fig. 4h). Interestingly, the distribution of time spent over snow on land during ODEs is consistently lower compared to non-ODEs for each spring month, and the median is significantly different at the 95th % CL (Fig. 5g).
For time spent above the mixed layer (i.e., free troposphere), each spring month displays a similar pattern, with a general tendency of decreasing (increasing) ozone mixing ratios (normalized ODE hours) with less time spent above the mixed layer (Fig. 4i). The distribution of time spent above the mixed layer for ODEs is consistently lower than for non-ODEs, and the median is significantly different at the 95th % CL (Fig. 5h).
3.3 Air mass history of ODEs
To understand the air mass origin of ODEs and non-ODEs, source regions were investigated through trajectory frequency maps (see “Methods and materials” section for details). Figure 6 displays the trajectory frequency only for steps below the mixed layer for ODE hours (Fig. 6a–c), non-ODE hours (Fig. 6d–f), and the difference between ODE and Non-ODE hours (Fig. 6g–i) for each spring month. Air masses arriving at Villum have been shown to predominantly reside above the mixed layer (∼ 75 %) during March and April, while, during May, this value decreases to ∼ 50 % (Pernov et al., 2022), hence the smaller air mass footprint for March and April. During March, the main source regions for ODE air masses appear to be the Chukchi Sea, while, for non-ODE air masses, the main source region is Greenland, with a minor contribution from the central Arctic Ocean (Fig. 6a and d). The difference between these trajectory frequency maps during March reveals that ODE air masses are spending relatively more time over the Chukchi Sea and the Canadian Archipelago and less time over Greenland (Fig. 6g). During April, ODE air masses originate from the central Arctic Ocean and, especially, the Beaufort and Chukchi seas, while non-ODE air masses arrive from the central Arctic Ocean and Greenland (Fig. 6b and e). The difference between ODEs and non-ODE air masses during April shows that the ODEs are preferentially coming from the central Arctic Ocean (Beaufort and Chukchi seas) and are spending comparatively less time over Greenland (Fig. 6h). During May, ODE air masses experience the most time over the central Arctic Ocean, with a minor contribution from the west coast of Greenland, which is similar to the source regions of non-ODE air masses, although with an increased contribution from Greenland (Fig. 6c and f). The difference between May ODE and non-ODE trajectory frequencies shows that the central Arctic Ocean is the main source region for ODE air masses and that non-ODE air masses are related to more southerly regions (Fig. 6i).
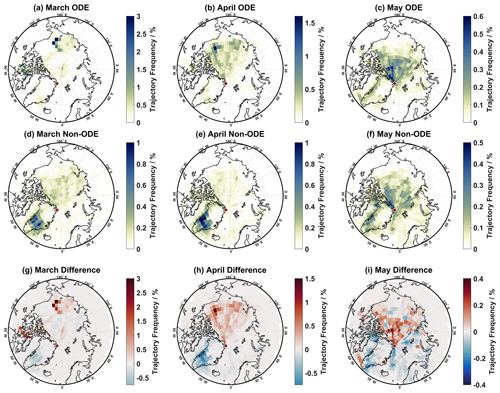
Figure 6Trajectory frequency maps for trajectory steps below the mixed layer for (a–c) March, April, and May ODEs and (d–f) March, April, and May non-ODEs and (g–i) difference between ODE and non-ODE trajectory frequencies during March, April, and May at Villum (indicated by the red and white circle).
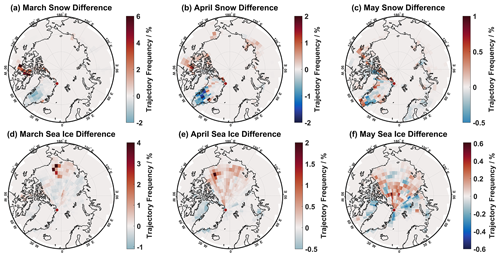
Figure 7Difference between ODE and non-ODE trajectory frequencies for (a–c) trajectory steps below the mixed layer and over snow on land during March, April, and May and for (d–f) trajectory steps below the mixed layer and over sea ice during March, April, and May at Villum (indicated by the red and white circle).
To investigate the geographic extent of the different surface types experienced during ODEs and non-ODEs, the trajectory frequencies for steps below the mixed layer and over sea ice and snow on land during ODEs and non-ODEs were also calculated; the frequencies are displayed in Figs. S6 and S7, respectively, while the difference is displayed in Fig. 7. For brevity, only the difference between ODE and non-ODE trajectory frequencies for each spring month will be discussed. The air mass history variable time spent over sea ice does not give information about the presence of snow cover; rather, it only gives information about whether or not the underlying surface was classified as sea ice.
During March, ODE trajectory steps over snow on land preferentially arrive from the Canadian Archipelago, while they arrive less often from Greenland compared to non-ODEs (Fig. 7a). Trajectory steps over sea ice during ODEs in March arise from the Chukchi Sea and arrive less often from the central Arctic Ocean compared to non-ODEs (Fig. 7d). During April, ODE trajectory steps over snow on land display a similar pattern to March (Canadian Archipelago), although now with minor contributions from other continental regions (Greenland, Alaska, and Siberia) compared to non-ODE air masses (Fig. 7b). Trajectory steps over sea ice during ODEs in April preferentially arrive from the Beaufort and Chukchi seas and less often from Baffin Bay compared to non-ODEs (Fig. 7e). During May, ODE trajectory steps over snow on land preferentially arrive from the Canadian Archipelago, similarly to March and April but now with increased contributions from Greenland compared to non-ODEs (Fig. 7c). Trajectory steps over sea ice during May ODEs arrive more often from the central Arctic Ocean and less often from more southerly areas (Baffin Bay, Greenland Sea, and Barents Sea) compared to non-ODEs (Fig. 7f). Interestingly, certain areas of the central Arctic Ocean experience more trajectory steps over sea ice during non-ODEs compared to ODEs (Fig. 7f); this is likely due to the central Arctic Ocean being a common source area for air masses below the mixed layer during May (Fig. S7). However, the results point to the central Arctic Ocean overall being a major source region for ODEs during May.
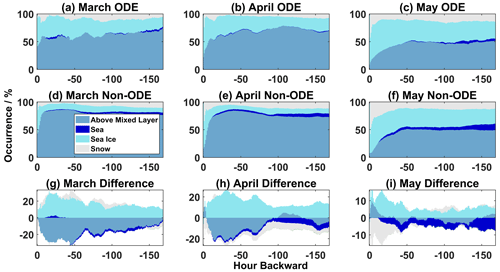
Figure 8The occurrence of each surface type experienced in the previous 168 h backward for (a–c) ODEs, (d–f) non-ODEs, and (g–i) the difference between ODEs and non-ODEs for March, April, and May. Note the differences in the y-axis scale for (g)–(i).
The above analysis investigated the geographic extents and surface types experienced by ODE and non-ODE air masses, although it does not give any temporal information. To further investigate the temporal relationships between ODEs and air mass history, the relative occurrence of each surface type (sea, sea ice, or snow on land) and the time spent above the mixed layer for each hourly step backward along the trajectories were calculated. Figure 8 shows the results of this analysis for ODEs on the top (Fig. 8a–c) and for non-ODEs in the middle (Fig. 8d–f), as well as the difference between ODEs and non-ODEs in the bottom row (Fig. 8g–i).
For ODEs during March and April, air masses spend a similar amount of time above the mixed layer and over sea ice. However, during March, trajectories experience slightly more time spent over snow on land and the sea, and, during April, they begin their descent later along the trajectory compared to March (Fig. 8a and b). During May, ODE trajectories spend less time above the mixed layer and more time over sea ice, sea, and snow on land compared to March and April (Fig. 8c). For non-ODEs during March and April, a similar picture is presented, whereby air masses spent a majority of their time above the mixed layer, followed by sea ice, snow on land, and sea, and the occurrence of each surface type is relatively constant throughout the length of the trajectory until they begin their descent into the boundary layer (Fig. 8d and e). For non-ODEs, during May, different air mass history conditions are presented compared to March and April. Air masses no longer spend a majority of their time above the mixed layer overall (45 % on average) and start to descend later along the trajectory compared to March and April (Fig. 8f). Instead, air masses experience increased time below the mixed layer and over sea ice and snow on land, with minor increases in the time spent over the sea. The time air masses spend over snow on land is relatively constant throughout the trajectory length until air masses start to descend. This pattern for non-ODEs largely reflects the typical air mass history for the spring months observed at Villum (Pernov et al., 2022). The difference in the occurrence of each surface type between ODEs and non-ODEs reveals that ODE air masses experience more time over sea ice and less time above the mixed layer during March and April (Fig. 8g and h). Air masses experience more time over snow on land during ODEs compared to non-ODEs when contrasting March and April, while less time over the sea is experienced during April compared to March (Fig. 8g and h). During May, the main differences between ODEs and non-ODEs are more time over sea ice and less time over the sea and snow on land; interestingly, there is little difference between time spent above the mixed layer, except for several hours before arrival at Villum, when ODE air masses experience more time above the mixed layer (Fig. 8i).
3.4 Machine learning modeling of ODEs
The statistical analysis of ODEs, meteorological variables, and air mass history variables examines the relationships between ozone and ODEs and each variable individually and does not consider interactions between them or give any information about which variables are most important for ODEs. To address this shortcoming and quantitatively investigate the most important variables for ODEs and how they affect ODEs, we utilized an ML model in our analysis (see the “Methods and materials” section for further details).
Table 1Evaluation metrics of the ML model for the spring months, together and individually. AUC ROC stands for area-under-curve receiver operating characteristics. For each metric, the top value represents the mean of the 10-fold cross-validation score, and the value below in parentheses represents the standard deviation (see Sect. 2.6 for a description of cross-validation).The accuracy gives an overview of the model performance for both labels (ODEs vs. non-ODEs), recall gives the model performance only for positive labels (ODEs), and AUC ROC evaluates the model performance over different decision thresholds; together, these three metrics give a comprehensive view of the model's performance. The three metrics range from 0 (worst) to 1 (best).

The evaluation metrics of the ML for all spring months combined and individual months are displayed in Table 1. We use three common metrics for evaluating a binary-classification ML model: accuracy, recall, and AUC ROC (area-under-curve receiver operating characteristics). Briefly, accuracy is the fraction of correctly predicted observations regardless of label (ODE vs. non-ODE), recall is the fraction of ODEs correctly predicted, and AUC ROC evaluates how well a model can discriminate between positive and negative labels across all decision thresholds for binary classification (see Sect. 2.6 for a detailed description of the evaluation metrics). In general, the ML model can accurately reproduce ODEs over all spring months combined, as evidenced by how all three metrics are close to unity (their maximum value). However, when evaluating the results on an individual monthly basis, there is an increase in the recall metric and a decrease in the accuracy and AUC ROC from March to May (Table 1), which is likely connected to the increasing occurrence of ODEs from March to May. With increased ODE occurrence, the recall metrics would increase as positive labels (ODEs) are more likely to be identified when they occur more often, and the accuracy and AUC ROC metrics would decrease with the increased occurrence of positive labels due to a concurrent increase in the number of incorrectly labeled ODEs. The ML model is also free from overfitting given the close agreement between the train and test sets. Overall, this ML model is sufficiently accurate, robust, and suitable for the investigation of ODEs.
The most important variables in the ML model are explored using SHAP values (Lundberg and Lee, 2017). The SHAP approach is designed to estimate the importance of each input variable to the model output based on coalitional game theory (Molnar, 2022) (see Sect. 2.6 for a more detailed description). SHAP values represent the marginal contribution of each input variable to the model output or, in other words, how each observation for each variable affects the model's prediction. SHAP values can be positive or negative, with positive values indicating that a variable is more likely to contribute to an observation being predicted as an ODE, while negative values mean a variable is more likely to contribute to an observation being labeled as a non-ODE. The SHAP methodology can produce both local and global explanations. The global importance gives an overview of the most important variables to the model output. The local importance of each observation can give information about the relationship between the SHAP and input values (positive or negative relationship, linear or non-linear) or, in other words, how the model output varies over the range of input values.
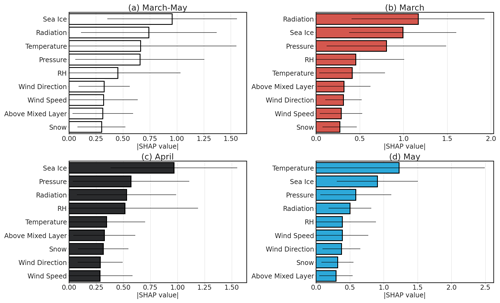
Figure 9Overall importance of each feature in the ML model during (a) all spring months combined, (b) March, (c) April, and (d) May. The bars represent the mean of the absolute SHAP values, while the lines represent the standard deviation.
The mean (± standard deviation) SHAP values for each variable during all spring months and individual months is displayed in Fig. 9. The most important variables overall are time spent over sea ice, radiation, temperature, pressure, and RH, which are the top variables during all spring months combined and each month individually, although the order differs slightly, while wind direction, wind speed, time spent above the mixed layer, and time spent over snow on land are consistently ranked near the bottom (Fig. 9a–d). During March, the most important variables are radiation, time spent over sea ice, and pressure (Fig. 9b). During April, time spent over sea ice, pressure, radiation, and RH are indicated as the most important variables (Fig. 9c). During May, the most important variables are temperature, time spent over sea ice, pressure, and radiation (Fig. 9d).
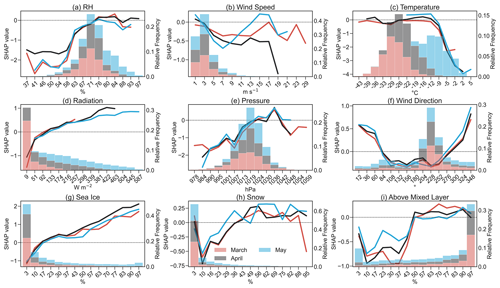
Figure 10The relationships between SHAP and ambient values for (a) RH, (b) wind speed, (c) temperature, (d) radiation, (e) pressure, (f) wind direction, (g) time air masses spent over sea ice, (h) time spent over snow on land, and (i) time spent above the mixed layer for each month. A total of 15 equally spaced bins were calculated for each variable, and the median of the SHAP values was computed for each bin, as represented by the colored lines. The value listed on the x axis is the midpoint of each bin. The colored bars represent a histogram of the ambient values for each month. The relative frequency of each histogram bin for each variable is displayed on the right axis. The legend is the same for the colored lines and bars.
While the overall importance of each variable in the ML model gives information about which variable has the largest influence on the model output, it does not give information about the nature of the relationship between the SHAP and ambient values for each variable (i.e., how the model outputs (SHAP values) vary over the range of input values). Here, ambient values refer to the observed values of each variable, i.e., the input data for the ML model. We binned the ambient values of each variable into 15 equally spaced bins and calculated the median SHAP value for each bin, as displayed in Fig. 10. A similar figure is presented in Fig. S9, which shows each month as its own subpanel, with the 25th and 75th percentiles included, and Fig. S10 shows all spring months combined, with the 25th and 75th percentiles included. Overall, the results largely agree with the results of the statistical analysis but reveal unique information about each variable during each month and how it affects the model prediction of ODEs. Notably, we observe the presence of certain threshold ranges where the relationship between ambient and SHAP values differs above and below this range. The ranges reported here indicate the lower and upper bin limits for one or more bins.
Ambient values of RH are normally distributed in each month, and the median SHAP values are negative for RH values below the mode of the distribution and are near zero for above-average RH values (Fig. 10a). This indicates that, when RH is below average, it has a negative effect on the model prediction of ODEs (i.e., the model is more likely to predict a non-ODE), and above-average RH values have little effect on the model output.
Ambient values of wind speed are usually low at Villum (< 5 m s−1), with values rarely exceeding 11 m s−1, and median SHAP values are only positive for the lowest bin during April and May (Fig. 10b). With higher values of wind speed, the median SHAP values are mostly negative except for the 13–19 m s−1 range during May and only the 17 m s−1 bin during March, although these high speeds rarely occur.
For temperature, the ambient values are normally distributed in each month, and, interestingly, a threshold value for temperature is observed during all months, with negative median SHAP values observed in the −10 to −13 °C bin (midpoint of −12 °C) and values centered around zero towards lower temperatures (Fig. 10c).
The distribution of radiation during each month is skewed towards lower values, and a threshold value for positive median SHAP values is also displayed for this variable. At values below the 112 to 153 W m−2 bin range (midpoint of 133 W m−2), radiation makes a negative contribution to the model output, and at values above this bin range, it contributes positively, and the relationship appears to be nearly linear (Fig. 10d).
For pressure, the ambient values are all normally distributed in each month. Similarly to RH, the relationship between ambient and SHAP values is negative for below-average ambient values, although, for above-average ambient values, the median SHAP value is only positive for the next several bins and becomes negative at very high values of pressure (though this rarely occurs) (Fig. 10e).
The most common wind direction at Villum is from the southeast, as observed in previous studies (Nguyen et al., 2016), although only northerly wind directions (288 to 72° bins) exhibit positive median SHAP values (Fig. 10f). This observation is congruent with the statistical analysis of wind direction (Fig. 4b) and with the origin of ODEs being the central Arctic Ocean (Figs. 6 and 7).
The distribution of time air masses spend over sea ice is skewed towards lower values for all 3 spring months, and only during May do values above 50 % occur regularly. The relationship between ambient and SHAP values for time spent over sea ice is almost linear, with higher values of time spent over sea ice increasing the likelihood of an ODE occurring (Fig. 10g). A threshold value for average positive SHAP values for time spent over sea ice is observed in the 13 % to 19 % bin range (midpoint of 17 %), and, interestingly, only after 30 % of the time spent over sea ice does the average relationship begin to differ for each month, although this still follows a linear pattern, indicating a slightly different sensitivity to exposure to sea ice and ODEs.
For time spent over snow on land, the distribution is more skewed towards lower values when compared to time spent over sea ice. The relationship between ambient and SHAP values for time spent over snow on land is complex and non-linear (Fig. 10h). The mode of time spent over snow on land is also the lowest value and appears to have little effect on the model output; however, the second most occurring bin for time spent over snow on land shows a strongly negative effect. After the third bin, SHAP values increase almost linearly and, on average, become positive in the 32 %–39 % bin range (midpoint of 36 %) during March and April and in the 26 % to 32 % bin range (midpoint of 29 %) during May. During all spring months, the SHAP values reach a plateau around 56 % of the time spent over snow on land, after which increasing time spent over snow on land has little effect on the model prediction of ODEs (Fig. 10h).
The relationship between ambient and SHAP values for time spent above the mixed layer shows negative contributions until a threshold range of 46 % to 53 % (midpoint of 50 %) is reached, after which a slightly positive contribution is observed (Fig. 10i).
4.1 Overview of ozone and ozone depletion events
Overall, the seasonal cycle of ozone at Villum displays a similar pattern to those observed at other coastal High Arctic sites that experience ODEs (Barrie et al., 1988; Eneroth et al., 2007; Law et al., 2023; Schroeder et al., 1998; Whaley et al., 2023), with elevated values during winter, highly variable and minimum values during spring, low values during summer, and increasing values during the autumn. The elevated values during winter are due to the efficient transport of anthropogenic pollution from the mid-latitudes (Stohl, 2006), descending air masses bringing ozone-rich air into the boundary layer (Hirdman et al., 2010), and inefficient removal mechanisms (absence of sunlight, reduced dry deposition due to a stably stratified atmosphere, snow coverage, and minimal wet scavenging). The minimum values observed during spring are due to ozone depletion events (ODEs) (Helmig et al., 2007a; Simpson et al., 2007b) caused by reactions with halogen species (Simpson et al., 2015; Yang et al., 2020). The maximum ozone values occurring in April are likely due to the maximum transport efficiency of anthropogenic pollution from the mid-latitudes during this period (Stohl, 2006), as well as stratospheric intrusions of dry, ozone-rich air (Helmig et al., 2007b; Liang et al., 2009).
The ODE frequency and duration display an increasing pattern from March to May, which is likely due to air masses spending more time within the mixed layer and over sea ice coupled with increased amounts of radiation as these variables are all important for ODEs (Fig. 9) and show a similar seasonal progression from March to May (Fig. 5). The geographic origin of ODEs within the mixed layer also shows a seasonal progression from March to May, with sources being more distant during March and progressively moving closer to Villum during April and May (Figs. 6 and 7). The ODE frequency at Zeppelin follows a similar seasonal progression, with ODEs occurring more often in late spring compared to early spring (Solberg et al., 1996; Zilker et al., 2023).
The ODE frequency and duration trends are positive during May (0.49 [−0.23, 1.2] % yr−1, > 85th % CL) and April (0.2 [0, 0.53] h yr−1, > 95th % CL), respectively (Fig. 3). There appears to be no SS trends in the start, end, or range of ODE days for any spring month (Fig. S3). SS positive trends in ODE frequency of 0.54 [± 0.26] (slope [± 95 % CI]) have been observed at Utqiaġvik (formerly known as Barrow), Alaska, only during March over the period 1973–2010 (Oltmans et al., 2012). A tendency for positive ODE frequency trends throughout the lowest level of ozonesonde measurements has also been observed at Canadian Arctic sites at Alert (0.19 [± 0.53] % yr−1, 1987–2020), Eureka (0.79 [± 0.83] % yr−1, 1991–2020), and Resolute (0.60 [± 0.30] % yr−1, 1966–2020 ) (Law et al., 2023; Tarasick and Bottenheim, 2002), which are similar in magnitude to the positive trend observed in this study. These positive trends in ODE frequencies around the Arctic and the trends in ODE frequency and duration at Villum could be connected to multiple causes: an increase in springtime tropospheric BrO in the Arctic, as observed by satellites (Bougoudis et al., 2020); the increase in Arctic sea salt aerosol due to multi-year ice being replaced with first-year ice (Confer et al., 2023); changing transport patterns (Heslin-Rees et al., 2020; Koo et al., 2014); increasing frequency of re-freezing leads (Yang et al., 2020); or increasing salinity of surface snow, which release halogen compounds to the atmosphere (Peterson et al., 2018; Pratt et al., 2013; Simpson et al., 2005). Further research is required to elucidate the underlying causes of these trends as well as positive trends in ozone mixing ratios observed at Villum (Law et al., 2023).
4.2 Dynamics of ODEs in relation to meteorological variables and air mass history
Our investigation into the dynamics of ODEs at Villum using a statistical analysis and ML modeling approach indicates that ODEs are connected to clear (high amounts of radiation), calm conditions (cold temperatures, high pressures, and low wind speeds), with air masses arriving from a northerly direction having experienced surface contact with sea ice (northerly wind directions and air masses experiencing a high amount of time over sea ice in the central Arctic Ocean). Our ML model revealed that the most important variables are similar throughout each month (time air masses spent over sea ice, radiation, temperature, and pressure) but exhibit different orders (Fig. 9). This indicates that the ML model can discern the overall conditions leading to ODEs but can also reveal distinct circumstances in each month. For instance, the time air masses spent over sea ice was consistently among the top variables for each month, which likely indicates that the release of halogen species from sea ice (or snow on top of sea ice) is a key condition for the observation of ODEs at Villum. During March, the most important variable is radiation, while, during May, it is temperature. Interestingly, these two variables (radiation and temperature) are often limited during these months (March and May), with low values of radiation during March and temperatures closer to 0 °C during May (Fig. 5d and c, respectively). In the following paragraphs, we discuss each variable's relation to ODEs for each spring month through our statistical analysis, ML modeling, and back-trajectory source regions.
Solar radiation is required for the photolysis of molecular halogen species (Peterson et al., 2018; Pratt et al., 2013; Raso et al., 2017; Wang et al., 2019). The results presented in Fig. 4e show that ODEs can occur across all values of radiation during April and May, while March shows a clearer dependency. Only during March were solar radiation medians significantly different during ODEs and non-ODEs (Fig. 5d), with solar radiation appearing to be a limiting factor. During April and May, sunlight is omnipresent; therefore, a clear lack of dependency of ozone mixing ratios and normalized ODE hours on radiation is not unexpected. This is supported by the high importance of radiation in the ML model during March compared to April and May (Fig. 9b). The results from the statistical analysis suggest that, while the presence of solar radiation is required, the intensity is not a limiting factor for the occurrence of ODEs. However, the relationships between ambient and SHAP values of radiation indicate there is a near-linear relationship (Fig. 10d), which highlights the added value of ML modeling. Alternatively, this could be due to ODEs resulting from the advection of previously depleted air masses, and in situ solar radiation measurements are not indicative of conditions along the trajectory path (although solar radiation exhibits a high degree of autocorrelation over all relevant lags) or in regions where depletion is occurring (Bottenheim and Chan, 2006; Halfacre et al., 2014). It should be noted that solar radiation measurements started in the autumn of 2014; thus, only 5 years of data are included in the statistical analysis, while the ML model was supplemented with radiation from ERA5 (see “Methods and materials” section); this could also contribute to the discrepancy between analysis methods.
Cold temperatures have been shown to be an important factor influencing ODEs (Simpson et al., 2007b, 2015); indeed, reactions on acidic, frozen heterogeneous surfaces can lead to the release of bromine, which is known from studies using reanalysis products (Seo et al., 2020; Zilker et al., 2023), laboratory experiments (Abbatt et al., 2012; Halfacre et al., 2019), and mesocosm and field studies (Gao et al., 2022; Pöhler et al., 2010; Pratt et al., 2013; Swanson et al., 2020). Cold temperatures also facilitate calcium carbonate precipitation from sea ice, which acidifies and lowers the buffering capacity of the salty sea ice surface, thus promoting halogen release (Sander et al., 2006). Observational evidence has shown that halogen activation ceases at above-freezing temperatures (Burd et al., 2017; Jeong et al., 2022). While several studies have reported a temperature dependency of ODEs (Koo et al., 2012; Pöhler et al., 2010; Tarasick and Bottenheim, 2002; Zeng et al., 2006), other studies have not (Halfacre et al., 2014; Jacobi et al., 2010; Neuman et al., 2010; Solberg et al., 1996). Any relationship between ODEs and temperature is likely a result of air masses having surface contact with the cold Arctic Ocean before arriving at Villum, where cold temperatures aid in the re-freezing of leads, as well as in the formation of sea ice and frost flowers (Kaleschke et al., 2004; Yang et al., 2020), all of which are known halogen sources. Cold temperatures could also indicate the presence of a temperature inversion, which traps oxidants and ozone near the surface and inhibits vertical mixing, which replenishes ozone and terminates ODEs (Moore et al., 2014). Temperature has the greatest influence on ODEs during May (Fig. 9d), which is the only month which regularly experiences temperatures above the threshold range of −10 to −13 °C found through our ML model analysis (Figs. 4, 5, and 10). Similarly to radiation, the temperature used in this analysis does not necessarily represent the temperature where ozone depletion occurred, although temperature is usually highly correlated to previous days' measurements and therefore gives a good indication of the temperature upwind of Villum. Therefore, this temperature threshold range should not be interpreted as absolute but rather as an indication of the existence of a threshold below which temperature has little effect and above which temperature makes a negative contribution to ODEs. This observation could help explain the contradictory evidence about the temperature dependence of ODEs. Depending on the local conditions of the measurement site, ODEs might be observed at temperatures below this threshold range (which would indicate no relationship) or above this threshold range (where ODEs show a negative relationship with temperature). This threshold range would be site specific and emphasizes the need for pan-Arctic assessments of the temperature dependency of ODEs.
Above-average values of RH are revealed to be conducive to ODEs through our statistical and ML model analysis (Figs. 4, 5, and 10). A relationship between RH and ODEs in the Arctic has not been reported in the literature before (to the authors' knowledge), and the physical mechanism behind this observation remains unclear. However, the relationship between RH and ozone has been explored in Antarctica by Frieß et al. (2023), who showed negative correlations at Neumayer and Arrival Heights, supporting observations made in this study. We hypothesize that the higher normalized ODE hours (Fig. 4a) and positive SHAP values (Fig. 10a) for above-average RH values during ODEs are likely connected to air masses spending time over the central Arctic Ocean, where RH would be higher due to the cold temperatures and the escape of water vapor through open leads and polynya (Bintanja and Selten, 2014; Boisvert et al., 2015). The lower values of normalized ODE hours (Fig. 4a) and negative SHAP values (Fig. 10a) for below-average RH could also be related to drier air masses having experienced higher altitudes, which are ozone-rich and less influenced by the surface, during transport to Villum (Moore et al., 2014).
Northerly wind directions are more common during ODEs compared to during non-ODEs (Fig. S6), corresponding to low ozone values, high normalized ODE hours, and positive SHAP values (Figs. 4b and 10f). A similar observation was made at Utqiaġvik (Barrow), Alaska, for low ozone mixing ratios showing a clear minimum when wind arrived from northerly directions (Helmig et al., 2012). Halfarce et al. (2014) used buoy measurements of air mass direction and ozone from the Beaufort Sea to show that northerly directions were dominating but that easterly and westerly directions also made a contribution, showing that, in the central Arctic Ocean, wind direction has less of an influence due to the omnidirectional presence of sea ice. These observations are directly related to the presence of sea ice in a northerly direction relative to these land-based stations (Fig. 1).
Wind speed can have dual effects on ozone variability, with low wind speeds corresponding to a stable boundary layer where reactants are confined to a small volume and with high wind speeds generating blowing snow, which acts as a source of reactive halogen species in addition to favoring the advection of air masses previously depleted in ozone (Jones et al., 2009; Swanson et al., 2020). The distributions of wind speeds during March and April were consistently higher for ODEs compared to for non-ODEs; this relationship is reversed for May (Fig. 5b), but in all months, relatively low wind speeds prevailed (≲ 3 m s−1). Our statistical analysis revealed no relationship between wind speeds and ozone mixing ratios and normalized ODE hours during March, a tendency for high normalized ODE hours with higher wind speeds during April (although there is little effect on ozone mixing ratios), and two modes during May (one at low and one at high wind speeds) (Fig. 4c). The ML model also showed a similar relationship during May (positive SHAP values at low and high wind speeds), although these high wind speeds did not occur very often. Overall, wind speeds are usually low at Villum (Figs. 4c, 5b, and 10b; Nguyen et al., 2016). Low ozone mixing ratios concurrent with low wind speeds have also been observed at Utqiaġvik (Barrow), Alaska; at Zeppelin Observatory on Svalbard; and from buoy measurements in the Arctic Ocean (Bottenheim et al., 2009; Halfacre et al., 2014; Helmig et al., 2012; Solberg et al., 1996). Conversely, enhanced BrO events at Zeppelin, Eureka, and Alert, as well as for the Arctic region, have been connected to high wind speeds, mostly likely related to stormy conditions that generate blowing snow (Seo et al., 2020; Swanson et al., 2020; Zhao et al., 2016; Zilker et al., 2023). In the Antarctic, positive correlations between wind speed and surface ozone were observed during spring at Arrival Heights but not at Neumayer, likely due to Arrival Heights being more influenced by local topography effects (Frieß et al., 2023). The results of our statistical and ML model analysis suggest that ODEs at Villum occur mainly under stable conditions with low wind speeds and are likely not connected to the generation of halogen species through blowing snow and Arctic cyclones. High wind speeds can also enhance vertical mixing of ozone-enriched air masses from aloft, which could mask the contribution of halogen activation from blowing snow. Only during May do high wind speeds regularly make a positive contribution to the model output, and the magnitude of this contribution is small (Fig. 10b). Overall, the rare occurrence of high wind speeds (Fig. S4b) hinders any definitive conclusions about their effect on ODEs.
Distributions of pressure are consistently higher for ODEs compared to for non-ODEs during each spring month (Fig. 5e), and above-average pressure is related to the occurrence of ODEs, as shown through our statistical analysis (Fig. 4f) and our ML model (Fig. 10e). High-pressure systems could indicate the presence of a stably stratified lower troposphere, and low-pressure systems could signal the passage of frontal systems which are conducive for strong vertical mixing (which brings ozone rich down from aloft) and a break up of inversion layers (Hopper et al., 1998; Jacobi et al., 2010; Simpson et al., 2015). Ozone and atmospheric pressure have been shown to be anti-correlated during spring in the Arctic Ocean (Jacobi et al., 2010). Conversely, low pressures have been associated with ODEs at Zeppelin (Zilker et al., 2023) and BrO enhancement events over the Arctic region (Blechschmidt et al., 2016; Seo et al., 2020) and at Eureka, Canada (Zhao et al., 2016), where they were related to polar storms and blowing-snow generation of reactive halogens. The pressure dependence of ODEs found at Villum is congruent with the relationship for wind speed (Fig. 10b) and further suggests that Arctic cyclones and blowing snow do not have an important effect on ODEs at Villum. Furthermore, very high values of pressure are likely associated with descending air masses from aloft, which are often enriched in ozone and contain few sources of halogen species (Simpson et al., 2007b; Peterson et al., 2015; Swanson et al., 2020), which could explain the negative SHAP values at high values of pressure, although it should be noted that these values do not occur often (Fig. 10e).
Heterogeneous, photochemical reactions on the snowpack have been demonstrated to be a source of reactive halogen species (Pratt et al., 2013; Raso et al., 2017; Peterson et al., 2018; McNamara et al., 2020; Custard et al., 2017), along with the generation of blowing snow at high wind speeds and the subsequent release of reactive halogens (Jones et al., 2009; Marelle et al., 2021; Chen et al., 2022; Swanson et al., 2022; Zilker et al., 2023; Frieß et al., 2023). Air masses spend little time over snow on land during each spring month (Fig. S4g), and, on average, ODEs actually experience less time over snow on land compared to non-ODEs (Fig. 5h). Non-ODEs experiencing more time over snow on land is likely tied to the different regions of contact with snow on land for non-ODEs (southern half of Greenland) (Fig. S6d–f), while source regions of air mass contact with snow on land during ODEs are consistently in the Canadian Archipelago and on Greenlandic coasts during the spring months (Fig. S6a–e). The Canadian Archipelago has been demonstrated to be a hotspot for BrO enhancements (Bognar et al., 2020; Bougoudis et al., 2020; Seo et al., 2020), which has been connected to low pressure and high wind speeds, suggesting blowing snow to be a source of halogen species in this region. Contributions from other continental regions (Alaska and Siberia) to snowpack exposure only appear in April (Fig. 7b), which could reflect the greater extent of the polar dome during this month (Stohl, 2006). Snowpack located on the west coast of Greenland only appears to contribute to ODEs during May (Fig. 7c); this could be related to air masses spending more time below the mixed layer during May compared to during other months (Fig. 5h). Our statistical analysis suggests that there is no clear dependency of ozone mixing ratios and normalized ODE hours on varying amounts of time spent over snow on land (Fig. 4h). Our ML model revealed that low values of time spent over snow on land contribute negatively, whereas, after a threshold range of 26 %–39 % (depending on the month), time spent over snow on land makes a small positive contribution to the model output that varies little with increasing values (Fig. 10). This is supported by the back-trajectory analysis, which showed that ODE air masses are not preferentially experiencing more time over snow on land during any particular point along the trajectory length compared to non-ODEs (Fig. 8g–i). High amounts of time spent over snow on land are uncommon during each spring month; therefore, it is difficult to assess the importance of snowpack mechanisms to ozone depletion at Villum. The generation of halogen species in the Canadian Archipelago, either through snowpack emissions or blowing snow at higher wind speeds, appears to consistently have a minor influence on ODEs during each spring month.
Sea ice sourced halogens have been indicated to be responsible for the halogen generation necessary for ozone depletion in the Arctic (Simpson et al., 2007b; Halfacre et al., 2014; Simpson et al., 2015; Burd et al., 2017; Yang et al., 2020; Marelle et al., 2021; Brockway et al., 2024) and Antarctic (Frieß et al., 2023). It should be noted that the snowpack on top of sea ice is the likely source of these halogens, given that the surface of sea ice is not conducive for halogen activation (Abbatt et al., 2012), although the satellite product used in this study cannot differentiate between snow-covered sea ice and bare sea ice (see “Methods and materials” section). The amount of time spent over sea ice increases from early to late spring (Fig. S4f), and ODE air masses experience higher values of time over sea ice during each spring month compared to non-ODEs (Fig. 5f). Our statistical analysis displays increased (decreased) normalized ODE hours (ozone mixing ratios) with higher values of time spent over sea ice (Fig. 4g), which is congruent with the ML model showing higher SHAP values for more time spent over sea ice. This relationship is linearly positive and, on average, becomes positive after the 13 % to 19 % threshold range (Fig. 10g). This indicates that air masses need to spend only a fraction of time over sea ice for it to increase the probability of observing an ODE at Villum. The back-trajectory analysis shows that ODE air masses experience more time over sea ice closer to the measurement site compared to non-ODEs (Fig. 8g–i). It has been found that ODEs can be the result of the transport of previously depleted air masses, where ozone depletion was occurring relatively far (several hundred kilometers) from the observation point (Halfacre et al., 2014; Tarasick and Bottenheim, 2002; Yang et al., 2020). As the spring progresses from March to May, it appears that the main ODE geographic source regions for sea ice contact move closer to Villum each month (Fig. 7d–f). During March, ODEs are initiated over the Chukchi Sea, which is usually covered by first-year sea ice (FYI) (Fig. 1). During April, ODE air mass source regions are located over the Beaufort and Chukchi seas but also over the central Arctic Ocean, which represents a mix of FYI and multi-year sea ice (MYI). During May, ODE air mass source regions are in closer proximity to Villum, mainly arriving from the central Arctic Ocean, which contains the highest concentration of MYI. This source region analysis is supported by the wind sector/speed analysis, which displays a northerly wind direction dependency for ODEs during each spring month (Figs. 4b, S5, and 10f). During March and April, wind speeds during ODEs are consistently higher compared to during non-ODEs, while, during May, wind speeds are lower (Fig. 5b). This could indicate that, in March, ODEs likely result from the transport of ozone-depleted air masses from FYI regions; that April experiences a mixture of transport-related ODEs and ODEs occurring closer to Villum from FYI and MYI regions; and that May ODEs occur in proximity to the measurement site, arriving mainly from regions with MYI but also with influences from FYI in the central Arctic Ocean. This is supported by Herrmann et al. (2022), who suggested that MYI makes important contributions to ozone depletion at Villum, and by Marelle et al. (2021), who showed that both snowpack emissions and blowing snow can contribute to ozone depletion, although sea ice surfaces were responsible for regional ozone depletion and halogen activation. It should be noted that this analysis is based on trajectory frequency maps and average sea ice age over the observation period, and a more detailed investigation of sea ice age would help elucidate the exact contribution of FYI and MYI to ODEs.
While this and previous work point towards ODEs being a surface-related process through the generation of reactive halogen species from sea ice and snowpack mechanisms, the activation of halogen species on aerosol particles aloft has also been demonstrated in the Arctic (Bognar et al., 2020; Peterson et al., 2017; Seabrook and Whiteway, 2016; Solberg et al., 1996). In the Antarctic, strong, positive correlations between aerosol extinction and BrO mixing rations have been observed during spring (Frieß et al., 2023). A general feature of the distributions for ODEs and non-ODEs when progressing from March to May is that trajectories spend increasingly less time above the mixed layer (Fig. 5h). Our statistical analysis indicates that, in general, ODEs are more likely to occur and ozone mixing ratios are more likely to be lower when air masses spend more time near the surface (Fig. 4i). Although ODE trajectories spend less time above the mixed layer compared to non-ODE trajectories (Figs. 5h and 8g–i), they are still spending a considerable amount of time aloft as the median time spent above the mixed layer only drops below 50 % during May (Fig. 5h). The recycling of halogen species on lofted aerosol particles could explain the ODEs experiencing a significant amount of time above the mixed layer. This would be especially relevant for the earlier spring months (March and April) given that the burden of acidic, tropospheric aerosols (i.e., Arctic haze) is greatest during these months (Flyger et al., 1980; Heidam et al., 1999, 2004; Nguyen et al., 2013, 2016) and the increased amount of time air masses spend above the mixed layer during these months. Our ML model revealed, on average, a positive contribution within the > 46 % to 53 % threshold range of time spent above the mixed layer (Fig. 10i). A physical explanation for our ML results for SHAP values of the time above the mixed layer could be that ozone is initially depleted within the boundary layer followed by lifting above the boundary layer and remains depleted either through inhibited mixing with ozone-rich air (Moore et al., 2014), decreased mixed-layer height with frequently occurring surface temperature inversions (Pilz et al., 2024), or halogen recycling on acidic aerosol particles aloft (Peterson et al., 2017). This could also be due to the time spent over the mixed layer being calculated over the entire trajectory length and therefore is not time resolved. It is also important to note that SHAP values represent how well these variables explain the behavior of our target variable in our ML model and not how well the input variables explain the behavior of our target variable in the natural environment.
To understand the conditions leading to a correct model prediction for the input variables and to investigate the cause of the relationship between ambient and SHAP values for time spent above the mixed layer, we calculated the distribution of ambient and SHAP values for correctly and incorrectly labeled observations of ODEs and non-ODEs for all spring months combined and for each month individually. The results for the ambient and SHAP value distributions are displayed in Fig. S11 and S12, respectively. The variables with the largest differences in the distribution of correct vs. incorrect ODEs are time spent above the mixed layer, time spent over sea ice, and radiation, whilst RH, time spent over snow on land, wind direction, and wind speed showed few differences (Fig. S11). The variables with the largest differences are also indicated to be the most important variables, and variables with little differences were shown to be the least important (Fig. 10), except for time above the mixed layer. Temperature displays a large difference between correct and incorrectly labeled ODEs when evaluating all spring months combined, but when analyzing individual spring months, this difference is diminished, which is likely a result of the seasonal progress of warmer temperatures later in the spring (Fig. 5c). The distributions for SHAP values between correctly and incorrectly labeled ODEs shows that SHAP values of time spent over sea ice experienced the largest difference for all spring months combined and for each individual month (Fig. S12). Other variables showing large differences in the distribution of SHAP values include pressure, temperature, radiation, and wind direction. Time spent above the mixed layer did not show large differences between correctly and incorrectly labeled ODEs, likely as a result of the small magnitude of the SHAP values for time spent above the mixed layer, indicating that this variable does not contribute largely to the model output (Fig. 9); therefore, while this relationship is counterintuitive, it does not affect the accurate prediction of ODEs in our ML model. The large differences between the distribution of time spent above the mixed layer for correctly vs. incorrectly labeled ODEs could be the underlying cause of the counterintuitive relationship between ambient and SHAP values for this variable, as displayed in Fig. 10; this could also be a result of ODE trajectories experiencing a majority of time above the mixed layer further back along the trajectory length (Fig. 8a–c). Other factors that could contribute to this relationship include the length of the back trajectory (trajectories experience comparatively more time above the mixed layer further backward), the misrepresentation of the mixed-layer height by the HYSPLIT model (too low of a mixed-layer height would result in a larger fraction of air masses above this altitude), the uncertainty of HYSPLIT increasing proportionately with the trajectory length, and the starting altitude of the back trajectories being too high (a higher starting altitude would result in a larger fraction of air masses residing above the mixed layer). Proper representation of air mass history is therefore an important aspect of evaluating ODEs and other atmospheric phenomena, and future studies should evaluate this in more detail, including the effects of varying trajectory lengths, the accuracy of the mixed-layer height from HYSPLIT, and the starting altitude at the receptor location. Overall, this shows the ability of ML to identify the appropriateness of input variables for modeling atmospheric phenomena and suggests that the importance of time spent above the mixed layer and time spent over sea ice might be over- and underestimated, respectively, as the ML model mis-characterizes their effect on ODEs.
ODEs occur every spring, with an increasing frequency from early to late spring. This seasonal pattern is the result of higher amounts of radiation, air masses spending more time within the mixed layer and over sea ice, and source regions for air mass contact with sea ice (and, thus, ozone depletion) moving closer to Villum from March to May. ODE duration and frequency displayed positive trends during April and May, respectively; however, we have low confidence in the frequency trend. Positive trends in ODE frequency at other Arctic sites suggest that this is a pan-Arctic phenomenon. Possible causes for the positive trends in the duration and frequency of ODEs include more FYI, BrO, saltier snowpack, changing transport patterns, and increased occurrence of refreezing leads.
ODEs are likely to occur during clear (high amounts of radiation), calm (cold temperatures, high pressure, low wind speeds) conditions with air masses arriving from northerly wind directions with sea ice contact (high time over sea ice, high RH). Time spent over sea ice, radiation, temperature, and pressure are shown to be the most important factors affecting ODEs. The most important variable affecting ODEs changes as spring progresses are radiation during March, sea ice during April, and temperature during May. During March and May, radiation and temperature are often the limiting factors, with smaller amounts of radiation observed during March and warmer temperatures observed during May. The source regions for ozone depletion also change as spring progresses. During March, sea ice (likely FYI) in the Chukchi Sea is the main source region for ODE air masses. During April, a mix of FYI and MYI in the Chukchi and Beaufort seas and the central Arctic Ocean are the main source regions for ODEs. During May, sea ice (likely a mix of FYI and MYI) in the central Arctic Ocean is the main ODE source region. Air masses experiencing snowpack contact within the mixed layer from the Canadian Archipelago make a consistent yet minor contribution during each spring month. The back-trajectory and wind speed analysis indicate that ozone depletion occurs upwind of Villum during early spring and moves progressively closer towards Villum during late spring.
We show that ODEs can be accurately predicted using ML modeling, with physically interpretable results. We also show that ML can be a useful tool for investigating atmospheric phenomena by quantifying the importance of each variable, identifying threshold ranges for positive contributions, and investigating the appropriateness of input variables. Of the sources leading to halogen emission (sea ice or snow on top of sea ice, snowpack on land, and recycling on aerosol particles aloft), our results suggest that emissions from sea ice regions are the most important.
While this work has made progress in understanding the dynamics of ozone depletion in the Arctic, further investigation is warranted. Recent research has shown that ozone mixing ratios are increasing around the Arctic (Christiansen et al., 2022, 2017; Cooper et al., 2020; Law et al., 2023), coupled with the positive trend in pan-Arctic ODE frequencies and the positive trend in ODE duration observed in this study, suggesting that the factors controlling ozone variability are being altered and that a detailed investigation into the underlying causes is warranted. Recently, iodine has been shown to be as important as bromine to ozone destruction in the central Arctic Ocean (Benavent et al., 2022); further studies investigating this discovery at pan-Arctic stations are needed to evaluate iodine's role in ozone depletion over the entire Arctic region. ML could aid in this task. Future studies investigating ozone and ODE dynamics would benefit from the incorporation of direct measurements of halogen species to investigate different chemical regimes of ozone destruction, which will help predict the response of springtime ozone dynamics in a future climate. Direct halogen measurements will also help elucidate the cause of ODE initiation, duration, and termination, as well as determine if ODEs are the result of the transport of already depleted air masses or if ODEs are occurring locally at Villum. Incorporating time-resolved air mass history variables and air mass exposure to first- and multi-year-ice sea ice concentrations would help clarify the role of different cryosphere environments in ozone destruction. Analyzing meteorological conditions along the trajectory path (e.g., temperature and radiation) would help extrapolate the observations from individual stations to the larger Arctic region. Future studies should also consider the vertical structure of the lower atmosphere (i.e., the mixed-layer height and its variability) when initializing trajectory calculation as this can have an effect on the air mass history, although this can be computationally challenging for a multi-decadal dataset. While this and many other studies investigate ozone at the surface, the radiative forcing of ozone is largely determined by its vertical distribution (Lacis et al., 1990; Stevenson et al., 2013); therefore, studies investigating the vertical and the horizontal distributions are needed. This could be accomplished through the use of tethered balloons deployed at ground-based stations or directly on the sea ice (Pilz et al., 2022; Pohorsky et al., 2024).
The added value of ML modeling over classical statistical analysis is highlighted by identifying variable importance, quantitative relationships, threshold ranges, and input variable deficiencies. While a statistical analysis can qualitatively identify relationships, ML can identify synergistic efforts regarding interactions between variables, indicating that the right mix of conditions is necessary for ODEs to occur: high sea ice contact, high amounts of radiation, cold temperatures, and high pressure. The ML methodology could be applied to other Arctic stations, either individually or utilizing multi-station (e.g., ground-based, ship-based, buoys) merging techniques for pan-Arctic modeling of ODEs, where the environmental drivers of ODEs could be investigated from a geographic perspective. This would be especially pertinent for measurements performed over sea ice, where the actual ozone destruction is likely occurring. ML modeling could also be used to investigate other atmospheric phenomena such as AMDEs and BrO enhancement events and for bias-correcting chemical transport models.
The results from our ML model largely agree with our statistical analysis and are physically meaningful/interpretable but also reveal threshold ranges for certain variables that are not evident otherwise and can help predict the response of ODEs in a future climate. Rising temperatures in the Arctic (Rantanen et al., 2022) could affect ODEs through earlier onset of melt days by ceasing halogen emissions. The temperature relationship displayed in this study (Fig. 10c) indicates that rising temperatures would have the biggest effect in May and would not start to negatively affect ODEs until they rise above the threshold range of −10 to −13 °C. Arctic sea ice is rapidly diminishing (Kwok, 2018; Stroeve and Notz, 2018), and the Arctic Ocean is projected to be completely ice-free during summer in the coming decades (Kim et al., 2023; Notz and Community, 2020), which will have profound effects on ODEs (Simpson et al., 2007b, 2015). Retreating sea ice would have a major effect on ODEs when sea ice loss is propagated into the springtime, and these effects would be most profound in May. Conversely, retreating sea ice would also increase sea salt aerosol emission through increased areas of open water, which is a source of bromine emission and recycling, therefore the competing effects of sea ice retreat require further investigation through coupled cryosphere–atmosphere modeling approaches. Changes in cloud cover, especially low-level liquid-containing clouds, would affect the amount of solar radiation reaching the surface. Previous studies have presented evidence for positive and negative trends in low cloud cover for the Arctic region (Boccolari and Parmiggiani, 2018; Jenkins and Dai, 2022; Lelli et al., 2023; Sviashchennikov and Drugorub, 2022; Wang et al., 2021). Increases in cloud cover would affect the amount of radiation received at the surface, which would mainly affect ODEs in March, when radiation is lower compared to the later spring months. How the Arctic and the nature of ODEs evolve with climate change remains an open question and should be the focus of future research endeavors.
The data used in this study are available at https://doi.org/10.5281/zenodo.11669155 (Pernov et al., 2024b). The original data sources are EBAS (https://doi.org/10.48597/YK7P-T42P, Keller and Kemp, 2023) for ozone, DMI Report 22-08 (https://www.dmi.dk/fileadmin/Rapporter/2022/DMIRep22-08.pdf, Jensen, 2022) for meteorological data, and ERDA (https://erda.au.dk/, login required, The MiG Project, 2024) for meteorological data. All code used in this study is available upon reasonable request from the corresponding authors. Sea ice age data are available from https://doi.org/10.5067/UTAV7490FEPB (Tschudi et al., 2019). Surface type maps used for the trajectory analysis are available from the National Snow and Ice Data Center (https://doi.org/10.7265/N52R3PMC, U.S. National Ice Center, 2008).
The supplement related to this article is available online at: https://doi.org/10.5194/acp-24-13603-2024-supplement.
JBP: conceptualization, methodology, software, validation, formal analysis, investigation, resources, data curation, writing (original draft preparation), writing (review and editing), visualization, supervision, project administration. JLH: conceptualization, methodology, software, validation, formal analysis, investigation, resources, data curation, writing (original draft preparation), writing (review and editing), visualization, supervision, project administration. LLS: funding acquisition, resources, data curation, writing (review and editing). HS: conceptualization, methodology, validation, formal analysis, investigation, resources, data curation, writing – original draft preparation, funding acquisition, writing (review and editing), supervision, project administration.
The contact author has declared that none of the authors has any competing interests.
Publisher's note: Copernicus Publications remains neutral with regard to jurisdictional claims made in the text, published maps, institutional affiliations, or any other geographical representation in this paper. While Copernicus Publications makes every effort to include appropriate place names, the final responsibility lies with the authors.
The Villum Foundation is gratefully acknowledged for financing the establishment of Villum Research Station. Thanks are given to the Royal Danish Air Force and the Arctic Command for providing logistical support to the project. Christel Christoffersen, Bjarne Jensen, Martin Ole Bjært Sørensen, Claus Nordstrøm, and Keld Mortensen are gratefully acknowledged for their technical support. The Danish Meteorological Institute (DMI) is acknowledged for the measurements from Station Nord (Jensen, 2022). Michele Volpi from the Swiss Data Science Center (SDSC) is acknowledged for the helpful discussions about machine learning modeling. Andrea Baccarini from EPFL is acknowledged for helping produce the map in Fig. 1. David Beddows from the University of Birmingham is acknowledged for the help with the trajectory analysis.
This research has been financially supported by the Danish Environmental Protection Agency and the Danish Energy Agency, with continuous funding over the years from the “Danish Program for Arctic Research” and ERA-PLANET (the European Network for observing our changing Planet) projects, as well as from iGOSP and iCUPE and, finally, from the Graduate School of Science and Technology, Aarhus University. Jakob Boyd Pernov received funding from the Swiss Data Science Center (project no. C20-01 – Arctic climate change: exploring the Natural Aerosol baseline for improved model Predictions (ArcticNAP)). This project received additional funding from the Ingvar Kamprad Chair funded by Ferring Pharmaceuticals and held by Julia Schmale from École Polytechnique Fédérale de Lausanne (EPFL).
This research has been supported by Miljøstyrelsen (Danish Program for Arctic Research), Energistyrelsen (Danish Program for Arctic Research), EU Horizon 2020 (iGOSP and iCUPE), the Aarhus Universitet (PhD stipend), Ferring Pharmaceuticals (Ingvar Kamprad Chair), and Swiss Data Science Center (project no. C20-01 – Arctic climate change: exploring the Natural Aerosol baseline for improved model Predictions (ArcticNAP)).
This paper was edited by Anoop Mahajan and reviewed by Peter Peterson and two anonymous referees.
Abbatt, J. P. D., Thomas, J. L., Abrahamsson, K., Boxe, C., Granfors, A., Jones, A. E., King, M. D., Saiz-Lopez, A., Shepson, P. B., Sodeau, J., Toohey, D. W., Toubin, C., von Glasow, R., Wren, S. N., and Yang, X.: Halogen activation via interactions with environmental ice and snow in the polar lower troposphere and other regions, Atmos. Chem. Phys., 12, 6237–6271, https://doi.org/10.5194/acp-12-6237-2012, 2012.
Akiba, T., Sano, S., Yanase, T., Ohta, T., and Koyama, M.: Optuna: A Next-Generation Hyperparameter Optimization Framework, in: Proceedings of the 25th ACM SIGKDD International Conference on Knowledge Discovery & Data Mining, Anchorage, AK, USA, 4–8 August 2019, Association for Computing Machinery, New York, NY, USA, 2623–2631, https://doi.org/10.1145/3292500.3330701, 2019.
AMAP: AMAP Assessment 2015: Black carbon and ozone as Arctic climate forcers, Arctic Monitoring and Assessment Programme (AMAP), p. 116, ISBN 978-82-7971-092-9, 2015.
Barrie, L. A., Bottenheim, J. W., Schnell, R. C., Crutzen, P. J., and Rasmussen, R. A.: Ozone destruction and photochemical reactions at polar sunrise in the lower Arctic atmosphere, Nature, 334, 138–141, https://doi.org/10.1038/334138a0, 1988.
Barten, J. G. M., Ganzeveld, L. N., Steeneveld, G.-J., and Krol, M. C.: Role of oceanic ozone deposition in explaining temporal variability in surface ozone at High Arctic sites, Atmos. Chem. Phys., 21, 10229–10248, https://doi.org/10.5194/acp-21-10229-2021, 2021.
Begoin, M., Richter, A., Weber, M., Kaleschke, L., Tian-Kunze, X., Stohl, A., Theys, N., and Burrows, J. P.: Satellite observations of long range transport of a large BrO plume in the Arctic, Atmos. Chem. Phys., 10, 6515–6526, https://doi.org/10.5194/acp-10-6515-2010, 2010.
Benavent, N., Mahajan, A. S., Li, Q., Cuevas, C. A., Schmale, J., Angot, H., Jokinen, T., Quéléver, L. L. J., Blechschmidt, A.-M., Zilker, B., Richter, A., Serna, J. A., Garcia-Nieto, D., Fernandez, R. P., Skov, H., Dumitrascu, A., Simões Pereira, P., Abrahamsson, K., Bucci, S., Duetsch, M., Stohl, A., Beck, I., Laurila, T., Blomquist, B., Howard, D., Archer, S. D., Bariteau, L., Helmig, D., Hueber, J., Jacobi, H.-W., Posman, K., Dada, L., Daellenbach, K. R., and Saiz-Lopez, A.: Substantial contribution of iodine to Arctic ozone destruction, Nat. Geosci., 15, 770–773, https://doi.org/10.1038/s41561-022-01018-w, 2022.
Bergstra, J., Bardenet, R., Bengio, Y., and Kégl, B.: Algorithms for Hyper-Parameter Optimization, in: Advances in Neural Information Processing Systems, edited by: Shawe-Taylor, J., Zemel, R., Bartlett, P., Pereira, F., and Weinberger, K. Q., Curran Associates, Inc., https://papers.nips.cc/paper_files/paper/2011/hash/86e8f7ab32cfd12577bc2619bc635690-Abstract.html (last access: 1 July 2022), 2011.
Bintanja, R. and Selten, F. M.: Future increases in Arctic precipitation linked to local evaporation and sea-ice retreat, Nature, 509, 479–482, https://doi.org/10.1038/nature13259, 2014.
Blechschmidt, A.-M., Richter, A., Burrows, J. P., Kaleschke, L., Strong, K., Theys, N., Weber, M., Zhao, X., and Zien, A.: An exemplary case of a bromine explosion event linked to cyclone development in the Arctic, Atmos. Chem. Phys., 16, 1773–1788, https://doi.org/10.5194/acp-16-1773-2016, 2016.
Boccolari, M. and Parmiggiani, F.: Trends and variability of cloud fraction cover in the Arctic, 1982–2009, Theor. Appl. Climatol., 132, 739–749, https://doi.org/10.1007/s00704-017-2125-6, 2018.
Bognar, K., Zhao, X., Strong, K., Chang, R. Y.-W., Frieß, U., Hayes, P. L., McClure-Begley, A., Morris, S., Tremblay, S., and Vicente-Luis, A.: Measurements of Tropospheric Bromine Monoxide Over Four Halogen Activation Seasons in the Canadian High Arctic, J. Geophys. Res.-Atmos., 125, e2020JD033015, https://doi.org/10.1029/2020jd033015, 2020.
Boisvert, L. N., Wu, D. L., and Shie, C.-L.: Increasing evaporation amounts seen in the Arctic between 2003 and 2013 from AIRS data, J. Geophys. Res.-Atmos., 120, 6865–6881, https://doi.org/10.1002/2015JD023258, 2015.
Bottenheim, J. W. and Chan, E.: A trajectory study into the origin of spring time Arctic boundary layer ozone depletion, J. Geophys. Res.-Atmos., 111, D19301, https://doi.org/10.1029/2006JD007055, 2006.
Bottenheim, J. W., Netcheva, S., Morin, S., and Nghiem, S. V.: Ozone in the boundary layer air over the Arctic Ocean: measurements during the TARA transpolar drift 2006–2008, Atmos. Chem. Phys., 9, 4545–4557, https://doi.org/10.5194/acp-9-4545-2009, 2009.
Bougoudis, I., Blechschmidt, A.-M., Richter, A., Seo, S., Burrows, J. P., Theys, N., and Rinke, A.: Long-term time series of Arctic tropospheric BrO derived from UV–VIS satellite remote sensing and its relation to first-year sea ice, Atmos. Chem. Phys., 20, 11869–11892, https://doi.org/10.5194/acp-20-11869-2020, 2020.
Brockway, N., Peterson, P. K., Bigge, K., Hajny, K. D., Shepson, P. B., Pratt, K. A., Fuentes, J. D., Starn, T., Kaeser, R., Stirm, B. H., and Simpson, W. R.: Tropospheric bromine monoxide vertical profiles retrieved across the Alaskan Arctic in springtime, Atmos. Chem. Phys., 24, 23–40, https://doi.org/10.5194/acp-24-23-2024, 2024.
Burd, J. A., Peterson, P. K., Nghiem, S. V., Perovich, D. K., and Simpson, W. R.: Snowmelt onset hinders bromine monoxide heterogeneous recycling in the Arctic, J. Geophys. Res.-Atmos., 122, 8297–8309, https://doi.org/10.1002/2017jd026906, 2017.
Chen, D., Luo, Y., Yang, X., Si, F., Dou, K., Zhou, H., Qian, Y., Hu, C., Liu, J., and Liu, W.: Study of an Arctic blowing snow-induced bromine explosion event in Ny-Ålesund, Svalbard, Sci. Total Environ., 839, 156335, https://doi.org/10.1016/j.scitotenv.2022.156335, 2022.
Chen, T. and Guestrin, C.: XGBoost: A Scalable Tree Boosting System, in: Proceedings of the 22nd ACM SIGKDD International Conference on Knowledge Discovery and Data Mining, San Francisco, California, USA, 13–17 August 2016, Association for Computing Machinery, New York, NY, USA, 785–794, https://doi.org/10.1145/2939672.2939785, 2016.
Choi, S., Wang, Y., Salawitch, R. J., Canty, T., Joiner, J., Zeng, T., Kurosu, T. P., Chance, K., Richter, A., Huey, L. G., Liao, J., Neuman, J. A., Nowak, J. B., Dibb, J. E., Weinheimer, A. J., Diskin, G., Ryerson, T. B., da Silva, A., Curry, J., Kinnison, D., Tilmes, S., and Levelt, P. F.: Analysis of satellite-derived Arctic tropospheric BrO columns in conjunction with aircraft measurements during ARCTAS and ARCPAC, Atmos. Chem. Phys., 12, 1255–1285, https://doi.org/10.5194/acp-12-1255-2012, 2012.
Christiansen, A., Mickley, L. J., Liu, J., Oman, L. D., and Hu, L.: Multidecadal increases in global tropospheric ozone derived from ozonesonde and surface site observations: can models reproduce ozone trends?, Atmos. Chem. Phys., 22, 14751–14782, https://doi.org/10.5194/acp-22-14751-2022, 2022.
Christiansen, B., Jepsen, N., Kivi, R., Hansen, G., Larsen, N., and Korsholm, U. S.: Trends and annual cycles in soundings of Arctic tropospheric ozone, Atmos. Chem. Phys., 17, 9347–9364, https://doi.org/10.5194/acp-17-9347-2017, 2017.
Collaud Coen, M., Andrews, E., Alastuey, A., Arsov, T. P., Backman, J., Brem, B. T., Bukowiecki, N., Couret, C., Eleftheriadis, K., Flentje, H., Fiebig, M., Gysel-Beer, M., Hand, J. L., Hoffer, A., Hooda, R., Hueglin, C., Joubert, W., Keywood, M., Kim, J. E., Kim, S.-W., Labuschagne, C., Lin, N.-H., Lin, Y., Lund Myhre, C., Luoma, K., Lyamani, H., Marinoni, A., Mayol-Bracero, O. L., Mihalopoulos, N., Pandolfi, M., Prats, N., Prenni, A. J., Putaud, J.-P., Ries, L., Reisen, F., Sellegri, K., Sharma, S., Sheridan, P., Sherman, J. P., Sun, J., Titos, G., Torres, E., Tuch, T., Weller, R., Wiedensohler, A., Zieger, P., and Laj, P.: Multidecadal trend analysis of in situ aerosol radiative properties around the world, Atmos. Chem. Phys., 20, 8867–8908, https://doi.org/10.5194/acp-20-8867-2020, 2020.
Confer, K. L., Jaeglé, L., Liston, G. E., Sharma, S., Nandan, V., Yackel, J., Ewert, M., and Horowitz, H. M.: Impact of Changing Arctic Sea Ice Extent, Sea Ice Age, and Snow Depth on Sea Salt Aerosol From Blowing Snow and the Open Ocean for 1980–2017, J. Geophys. Res.-Atmos., 128, e2022JD037667, https://doi.org/10.1029/2022JD037667, 2023.
Cooper, O. R., Schultz, M. G., Schröder, S., Chang, K.-L., Gaudel, A., Benítez, G. C., Cuevas, E., Fröhlich, M., Galbally, I. E., Molloy, S., Kubistin, D., Lu, X., McClure-Begley, A., Nédélec, P., O'Brien, J., Oltmans, S. J., Petropavlovskikh, I., Ries, L., Senik, I., Sjöberg, K., Solberg, S., Spain, G. T., Spangl, W., Steinbacher, M., Tarasick, D., Thouret, V., and Xu, X.: Multi-decadal surface ozone trends at globally distributed remote locations, Elem. Sci. Anth., 8, 23, https://doi.org/10.1525/elementa.420, 2020.
Custard, K. D., Raso, A. R. W., Shepson, P. B., Staebler, R. M., and Pratt, K. A.: Production and Release of Molecular Bromine and Chlorine from the Arctic Coastal Snowpack, ACS Earth Space Chem., 1, 142–151, https://doi.org/10.1021/acsearthspacechem.7b00014, 2017.
Dall'Osto, M., Beddows, D. C. S., Tunved, P., Krejci, R., Ström, J., Hansson, H. C., Yoon, Y. J., Park, K.-T., Becagli, S., Udisti, R., Onasch, T., O'Dowd, C. D., Simó, R., and Harrison, R. M.: Arctic sea ice melt leads to atmospheric new particle formation, Sci. Rep., 7, 3318, https://doi.org/10.1038/s41598-017-03328-1, 2017.
Dall'Osto, M., Geels, C., Beddows, D. C. S., Boertmann, D., Lange, R., Nojgaard, J. K., Harrison, R. M., Simo, R., Skov, H., and Massling, A.: Regions of open water and melting sea ice drive new particle formation in North East Greenland, Sci. Rep., 8, 6109, https://doi.org/10.1038/s41598-018-24426-8, 2018.
Draxler, R. R. and Hess, G. D.: An overview of the HYSPLIT_4 modelling system for trajectories, dispersion and deposition, Aust. Meteorol. Mag., 47, 295–308, 1998.
Eneroth, K., Holmén, K., Berg, T., Schmidbauer, N., and Solberg, S.: Springtime depletion of tropospheric ozone, gaseous elemental mercury and non-methane hydrocarbons in the European Arctic, and its relation to atmospheric transport, Atmos. Environ., 41, 8511–8526, https://doi.org/10.1016/j.atmosenv.2007.07.008, 2007.
Flyger, H., Heidam, N. Z., Hansen, K. A., Rasmussen, L., and Megaw, W. J.: The background levels of the summer tropospheric aerosol and trace gases in Greenland, J. Aerosol Sci., 11, 95–110, https://doi.org/10.1016/0021-8502(80)90149-4, 1980.
Frieß, U., Hollwedel, J., König-Langlo, G., Wagner, T., and Platt, U.: Dynamics and chemistry of tropospheric bromine explosion events in the Antarctic coastal region, J. Geophys. Res.-Atmos., 109, D06305, https://doi.org/10.1029/2003JD004133, 2004.
Frieß, U., Sihler, H., Sander, R., Pöhler, D., Yilmaz, S., and Platt, U.: The vertical distribution of BrO and aerosols in the Arctic: Measurements by active and passive differential optical absorption spectroscopy, J. Geophys. Res.-Atmos., 116, D00R04, https://doi.org/10.1029/2011JD015938, 2011.
Frieß, U., Kreher, K., Querel, R., Schmithüsen, H., Smale, D., Weller, R., and Platt, U.: Source mechanisms and transport patterns of tropospheric bromine monoxide: findings from long-term multi-axis differential optical absorption spectroscopy measurements at two Antarctic stations, Atmos. Chem. Phys., 23, 3207–3232, https://doi.org/10.5194/acp-23-3207-2023, 2023.
Gao, Z., Geilfus, N.-X., Saiz-Lopez, A., and Wang, F.: Reproducing Arctic springtime tropospheric ozone and mercury depletion events in an outdoor mesocosm sea ice facility, Atmos. Chem. Phys., 22, 1811–1824, https://doi.org/10.5194/acp-22-1811-2022, 2022.
Gryning, S.-E., Batchvarova, E., Floors, R., Münkel, C., Sørensen, L. L., and Skov, H.: Observed aerosol-layer depth at Station Nord in the high Arctic, Int. J. Climatol., 43, 3247–3263, https://doi.org/10.1002/joc.8027, 2023.
Halfacre, J. W., Knepp, T. N., Shepson, P. B., Thompson, C. R., Pratt, K. A., Li, B., Peterson, P. K., Walsh, S. J., Simpson, W. R., Matrai, P. A., Bottenheim, J. W., Netcheva, S., Perovich, D. K., and Richter, A.: Temporal and spatial characteristics of ozone depletion events from measurements in the Arctic, Atmos. Chem. Phys., 14, 4875–4894, https://doi.org/10.5194/acp-14-4875-2014, 2014.
Halfacre, J. W., Shepson, P. B., and Pratt, K. A.: pH-dependent production of molecular chlorine, bromine, and iodine from frozen saline surfaces, Atmos. Chem. Phys., 19, 4917–4931, https://doi.org/10.5194/acp-19-4917-2019, 2019.
Heidam, N. Z., Wåhlin, P., and Christensen, J. H.: Tropospheric Gases and Aerosols in Northeast Greenland, Journal of the Atmospheric Sciences, 56, 261–278, https://doi.org/10.1175/1520-0469(1999)056<0261:Tgaain>2.0.Co;2, 1999.
Heidam, N. Z., Christensen, J., Wahlin, P., and Skov, H.: Arctic atmospheric contaminants in NE Greenland: levels, variations, origins, transport, transformations and trends 1990–2001, Sci. Total Environ., 331, 5–28, https://doi.org/10.1016/j.scitotenv.2004.03.033, 2004.
Helmig, D., Oltmans, S. J., Carlson, D., Lamarque, J.-F., Jones, A., Labuschagne, C., Anlauf, K., and Hayden, K.: A review of surface ozone in the polar regions, Atmos. Environ., 41, 5138–5161, https://doi.org/10.1016/j.atmosenv.2006.09.053, 2007a.
Helmig, D., Oltmans, S. J., Morse, T. O., and Dibb, J. E.: What is causing high ozone at Summit, Greenland?, Atmos. Environ., 41, 5031–5043, https://doi.org/10.1016/j.atmosenv.2006.05.084, 2007b.
Helmig, D., Boylan, P., Johnson, B., Oltmans, S., Fairall, C., Staebler, R., Weinheimer, A., Orlando, J., Knapp, D. J., Montzka, D. D., Flocke, F., Frieß, U., Sihler, H., and Shepson, P. B.: Ozone dynamics and snow-atmosphere exchanges during ozone depletion events at Barrow, Alaska, J. Geophys. Res.-Atmos., 117, D20303, https://doi.org/10.1029/2012JD017531, 2012.
Herrmann, M., Schöne, M., Borger, C., Warnach, S., Wagner, T., Platt, U., and Gutheil, E.: Ozone depletion events in the Arctic spring of 2019: a new modeling approach to bromine emissions, Atmos. Chem. Phys., 22, 13495–13526, https://doi.org/10.5194/acp-22-13495-2022, 2022.
Hersbach, H., Bell, B., Berrisford, P., Hirahara, S., Horányi, A., Muñoz-Sabater, J., Nicolas, J., Peubey, C., Radu, R., Schepers, D., Simmons, A., Soci, C., Abdalla, S., Abellan, X., Balsamo, G., Bechtold, P., Biavati, G., Bidlot, J., Bonavita, M., Chiara, G., Dahlgren, P., Dee, D., Diamantakis, M., Dragani, R., Flemming, J., Forbes, R., Fuentes, M., Geer, A., Haimberger, L., Healy, S., Hogan, R. J., Hólm, E., Janisková, M., Keeley, S., Laloyaux, P., Lopez, P., Lupu, C., Radnoti, G., Rosnay, P., Rozum, I., Vamborg, F., Villaume, S., and Thépaut, J.: The ERA5 global reanalysis, Q. J. Roy. Meteor. Soc., 146, 1999–2049, https://doi.org/10.1002/qj.3803, 2020.
Heslin-Rees, D., Burgos, M., Hansson, H.-C., Krejci, R., Ström, J., Tunved, P., and Zieger, P.: From a polar to a marine environment: has the changing Arctic led to a shift in aerosol light scattering properties?, Atmos. Chem. Phys., 20, 13671–13686, https://doi.org/10.5194/acp-20-13671-2020, 2020.
Hirdman, D., Sodemann, H., Eckhardt, S., Burkhart, J. F., Jefferson, A., Mefford, T., Quinn, P. K., Sharma, S., Ström, J., and Stohl, A.: Source identification of short-lived air pollutants in the Arctic using statistical analysis of measurement data and particle dispersion model output, Atmos. Chem. Phys., 10, 669–693, https://doi.org/10.5194/acp-10-669-2010, 2010.
Hogan, R.: Radiation Quantities in the ECMWF model and MARS, ECMWF, Reading, UK, https://www.ecmwf.int/en/elibrary/80755-radiation-quantities-ecmwf-model-and-mars (last access: 1 July 2022), 2015.
Hopper, J. F., Barrie, L. A., Silis, A., Hart, W., Gallant, A. J., and Dryfhout, H.: Ozone and meteorology during the 1994 Polar Sunrise Experiment, J. Geophys. Res.-Atmos., 103, 1481–1492, https://doi.org/10.1029/97JD02888, 1998.
Ianniello, A., Salzano, R., Salvatori, R., Esposito, G., Spataro, F., Montagnoli, M., Mabilia, R., and Pasini, A.: Nitrogen Oxides (NOx) in the Arctic Troposphere at Ny-Ålesund (Svalbard Islands): Effects of Anthropogenic Pollution Sources, Atmosphere, 12, 901, https://doi.org/10.3390/atmos12070901, 2021.
Jacobi, H.-W., Morin, S., and Bottenheim, J. W.: Observation of widespread depletion of ozone in the springtime boundary layer of the central Arctic linked to mesoscale synoptic conditions, J. Geophys. Res.-Atmos., 115, D17302, https://doi.org/10.1029/2010JD013940, 2010.
Jenkins, M. T. and Dai, A.: Arctic Climate Feedbacks in ERA5 Reanalysis: Seasonal and Spatial Variations and the Impact of Sea-Ice Loss, Geophys. Res. Lett., 49, e2022GL099263, https://doi.org/10.1029/2022GL099263, 2022.
Jensen, C. D.: Weather Observations from Greenland 1958–2022, DMI Report 22-08, Danish Meteorological Institute [data set], https://www.dmi.dk/fileadmin/Rapporter/2022/DMIRep22-08.pdf (last access: 1 January 2024), 2022.
Jeong, D., McNamara, S. M., Barget, A. J., Raso, A. R. W., Upchurch, L. M., Thanekar, S., Quinn, P. K., Simpson, W. R., Fuentes, J. D., Shepson, P. B., and Pratt, K. A.: Multiphase Reactive Bromine Chemistry during Late Spring in the Arctic: Measurements of Gases, Particles, and Snow, ACS Earth Space Chem., 6, 2877–2887, https://doi.org/10.1021/acsearthspacechem.2c00189, 2022.
Jones, A. E., Anderson, P. S., Begoin, M., Brough, N., Hutterli, M. A., Marshall, G. J., Richter, A., Roscoe, H. K., and Wolff, E. W.: BrO, blizzards, and drivers of polar tropospheric ozone depletion events, Atmos. Chem. Phys., 9, 4639–4652, https://doi.org/10.5194/acp-9-4639-2009, 2009.
Kaleschke, L., Richter, A., Burrows, J., Afe, O., Heygster, G., Notholt, J., Rankin, A. M., Roscoe, H. K., Hollwedel, J., Wagner, T., and Jacobi, H. W.: Frost flowers on sea ice as a source of sea salt and their influence on tropospheric halogen chemistry, Geophys. Res. Lett., 31, L16114, https://doi.org/10.1029/2004gl020655, 2004.
Kalnay, E., Kanamitsu, M., Kistler, R., Collins, W., Deaven, D., Gandin, L., Iredell, M., Saha, S., White, G., Woollen, J., Zhu, Y., Chelliah, M., Ebisuzaki, W., Higgins, W., Janowiak, J., Mo, K. C., Ropelewski, C., Wang, J., Leetmaa, A., Reynolds, R., Jenne, R., and Joseph, D.: The NCEP/NCAR 40-Year Reanalysis Project, B. Am. Meteorol. Soc., 77, 437–472, https://doi.org/10.1175/1520-0477(1996)077<0437:TNYRP>2.0.CO;2, 1996.
Keller, R. and Kemp, K.: Ozone at Villum Research Station, Station Nord, AMAP, EMEP, GAW-WDCRG, 2001–2023, data hosted by EBAS at NILU [data set], https://doi.org/10.48597/YK7P-T42P, 2023.
Kendall, M. G.: Rank correlation methods, Griffin, Oxford, UK, 1948.
Kim, Y.-H., Min, S.-K., Gillett, N. P., Notz, D., and Malinina, E.: Observationally-constrained projections of an ice-free Arctic even under a low emission scenario, Nat. Commun., 14, 3139, https://doi.org/10.1038/s41467-023-38511-8, 2023.
Koo, J.-H., Wang, Y., Kurosu, T. P., Chance, K., Rozanov, A., Richter, A., Oltmans, S. J., Thompson, A. M., Hair, J. W., Fenn, M. A., Weinheimer, A. J., Ryerson, T. B., Solberg, S., Huey, L. G., Liao, J., Dibb, J. E., Neuman, J. A., Nowak, J. B., Pierce, R. B., Natarajan, M., and Al-Saadi, J.: Characteristics of tropospheric ozone depletion events in the Arctic spring: analysis of the ARCTAS, ARCPAC, and ARCIONS measurements and satellite BrO observations, Atmos. Chem. Phys., 12, 9909–9922, https://doi.org/10.5194/acp-12-9909-2012, 2012.
Koo, J.-H., Wang, Y., Jiang, T., Deng, Y., Oltmans, S. J., and Solberg, S.: Influence of climate variability on near-surface ozone depletion events in the Arctic spring, Geophys. Res. Lett., 41, 2582–2589, https://doi.org/10.1002/2014GL059275, 2014.
Kwok, R.: Arctic sea ice thickness, volume, and multiyear ice coverage: losses and coupled variability (1958–2018), Environ. Res. Lett., 13, 105005, https://doi.org/10.1088/1748-9326/aae3ec, 2018.
Lacis, A. A., Wuebbles, D. J., and Logan, J. A.: Radiative forcing of climate by changes in the vertical distribution of ozone, J. Geophys. Res.-Atmos., 95, 9971–9981, https://doi.org/10.1029/JD095iD07p09971, 1990.
Law, K. S., Hjorth, J. L., Pernov, J. B., Whaley, C. H., Skov, H., Collaud Coen, M., Langner, J., Arnold, S. R., Tarasick, D., Christensen, J., Deushi, M., Effertz, P., Faluvegi, G., Gauss, M., Im, U., Oshima, N., Petropavlovskikh, I., Plummer, D., Tsigaridis, K., Tsyro, S., Solberg, S., and Turnock, S.: Arctic Tropospheric Ozone Trends, Geophys. Res. Lett., 50, e2023GL103096, https://doi.org/10.1029/2023GL103096, 2023.
Lelli, L., Vountas, M., Khosravi, N., and Burrows, J. P.: Satellite remote sensing of regional and seasonal Arctic cooling showing a multi-decadal trend towards brighter and more liquid clouds, Atmos. Chem. Phys., 23, 2579–2611, https://doi.org/10.5194/acp-23-2579-2023, 2023.
Li, L., Jamieson, K., DeSalvo, G., Rostamizadeh, A., and Talwalkar, A.: Hyperband: A Novel Bandit-Based Approach to Hyperparameter Optimization, J. Mach. Learn. Res., 18, 1–52, 2018.
Liang, Q., Douglass, A. R., Duncan, B. N., Stolarski, R. S., and Witte, J. C.: The governing processes and timescales of stratosphere-to-troposphere transport and its contribution to ozone in the Arctic troposphere, Atmos. Chem. Phys., 9, 3011–3025, https://doi.org/10.5194/acp-9-3011-2009, 2009.
Lundberg, S. M. and Lee, S.-I.: A Unified Approach to Interpreting Model Predictions, in: Adv Neural Inf Process Syst, arXiv [preprint], https://doi.org/10.48550/arXiv.1705.07874, 22 May 2017.
Lundberg, S. M., Erion, G. G., and Lee, S.-I.: Consistent Individualized Feature Attribution for Tree Ensembles, CoRR, arXiv [preprint], https://doi.org/10.48550/arXiv.1802.03888, 7 March 2019.
Mann, H. B.: Nonparametric Tests Against Trend, Econometrica, 13, 245–259, https://doi.org/10.2307/1907187, 1945.
Marelle, L., Thomas, J. L., Ahmed, S., Tuite, K., Stutz, J., Dommergue, A., Simpson, W. R., Frey, M. M., and Baladima, F.: Implementation and Impacts of Surface and Blowing Snow Sources of Arctic Bromine Activation Within WRF-Chem 4.1.1, J. Adv. Model. Earth Sys., 13, e2020MS002391, https://doi.org/10.1029/2020MS002391, 2021.
McNamara, S. M., Garner, N. M., Wang, S., Raso, A. R. W., Thanekar, S., Barget, A. J., Fuentes, J. D., Shepson, P. B., and Pratt, K. A.: Bromine Chloride in the Coastal Arctic: Diel Patterns and Production Mechanisms, ACS Earth Space Chem., 4, 620–630, https://doi.org/10.1021/acsearthspacechem.0c00021, 2020.
Molnar, C.: Interpretable Machine Learning: A Guide for Making Black Box Models Explainable, 2nd edn., Independently published, ISBN 979-8411463330, 2022.
Monks, P. S., Archibald, A. T., Colette, A., Cooper, O., Coyle, M., Derwent, R., Fowler, D., Granier, C., Law, K. S., Mills, G. E., Stevenson, D. S., Tarasova, O., Thouret, V., von Schneidemesser, E., Sommariva, R., Wild, O., and Williams, M. L.: Tropospheric ozone and its precursors from the urban to the global scale from air quality to short-lived climate forcer, Atmos. Chem. Phys., 15, 8889–8973, https://doi.org/10.5194/acp-15-8889-2015, 2015.
Moore, C. W., Obrist, D., Steffen, A., Staebler, R. M., Douglas, T. A., Richter, A., and Nghiem, S. V.: Convective forcing of mercury and ozone in the Arctic boundary layer induced by leads in sea ice, Nature, 506, 81–4, https://doi.org/10.1038/nature12924, 2014.
Morin, S., Savarino, J., Frey, M. M., Yan, N., Bekki, S., Bottenheim, J. W., and Martins, J. M. F.: Tracing the Origin and Fate of NOx in the Arctic Atmosphere Using Stable Isotopes in Nitrate, Science, 322, 730–732, https://doi.org/10.1126/science.1161910, 2008.
Neuman, J. A., Nowak, J. B., Huey, L. G., Burkholder, J. B., Dibb, J. E., Holloway, J. S., Liao, J., Peischl, J., Roberts, J. M., Ryerson, T. B., Scheuer, E., Stark, H., Stickel, R. E., Tanner, D. J., and Weinheimer, A.: Bromine measurements in ozone depleted air over the Arctic Ocean, Atmos. Chem. Phys., 10, 6503–6514, https://doi.org/10.5194/acp-10-6503-2010, 2010.
Nguyen, Q. T., Skov, H., Sørensen, L. L., Jensen, B. J., Grube, A. G., Massling, A., Glasius, M., and Nøjgaard, J. K.: Source apportionment of particles at Station Nord, North East Greenland during 2008–2010 using COPREM and PMF analysis, Atmos. Chem. Phys., 13, 35–49, https://doi.org/10.5194/acp-13-35-2013, 2013.
Nguyen, Q. T., Glasius, M., Sørensen, L. L., Jensen, B., Skov, H., Birmili, W., Wiedensohler, A., Kristensson, A., Nøjgaard, J. K., and Massling, A.: Seasonal variation of atmospheric particle number concentrations, new particle formation and atmospheric oxidation capacity at the high Arctic site Villum Research Station, Station Nord, Atmos. Chem. Phys., 16, 11319–11336, https://doi.org/10.5194/acp-16-11319-2016, 2016.
Notz, D. and Community, S.: Arctic Sea Ice in CMIP6, Geophys. Res. Lett., 47, e2019GL086749, https://doi.org/10.1029/2019GL086749, 2020.
Oltmans, S. J., Johnson, B. J., and Harris, J. M.: Springtime boundary layer ozone depletion at Barrow, Alaska: Meteorological influence, year-to-year variation, and long-term change, J. Geophys. Res.-Atmos., 117, D00R18, https://doi.org/10.1029/2011JD016889, 2012.
Pernov, J. B., Bossi, R., Lebourgeois, T., Nøjgaard, J. K., Holzinger, R., Hjorth, J. L., and Skov, H.: Atmospheric VOC measurements at a High Arctic site: characteristics and source apportionment, Atmos. Chem. Phys., 21, 2895–2916, https://doi.org/10.5194/acp-21-2895-2021, 2021.
Pernov, J. B., Beddows, D., Thomas, D. C., Dall'Osto, M., Harrison, R. M., Schmale, J., Skov, H., and Massling, A.: Increased aerosol concentrations in the High Arctic attributable to changing atmospheric transport patterns, npj Clim. Atmos. Sci., 5, 1–13, https://doi.org/10.1038/s41612-022-00286-y, 2022.
Pernov, J. B., Gros-Daillon, J., and Schmale, J.: Comparison of selected surface level ERA5 variables against in situ observations in the continental Arctic, Q. J. Roy. Meteor. Soc., 150, 2123–2146, https://doi.org/10.1002/qj.4700, 2024a.
Pernov, J., Skov, H., Hjorth, J., and Sørensen, L. L.: Dataset for “On the dynamics of ozone depletion events at Villum Research Station in the High Arctic” Pernov et al., Zenodo [data set], https://doi.org/10.5281/zenodo.11669155, 2024b.
Peterson, P. K., Simpson, W. R., Pratt, K. A., Shepson, P. B., Frieß, U., Zielcke, J., Platt, U., Walsh, S. J., and Nghiem, S. V.: Dependence of the vertical distribution of bromine monoxide in the lower troposphere on meteorological factors such as wind speed and stability, Atmos. Chem. Phys., 15, 2119–2137, https://doi.org/10.5194/acp-15-2119-2015, 2015.
Peterson, P. K., Pöhler, D., Sihler, H., Zielcke, J., General, S., Frieß, U., Platt, U., Simpson, W. R., Nghiem, S. V., Shepson, P. B., Stirm, B. H., Dhaniyala, S., Wagner, T., Caulton, D. R., Fuentes, J. D., and Pratt, K. A.: Observations of bromine monoxide transport in the Arctic sustained on aerosol particles, Atmos. Chem. Phys., 17, 7567–7579, https://doi.org/10.5194/acp-17-7567-2017, 2017.
Peterson, P. K., Pöhler, D., Zielcke, J., General, S., Frieß, U., Platt, U., Simpson, W. R., Nghiem, S. V., Shepson, P. B., Stirm, B. H., and Pratt, K. A.: Springtime Bromine Activation over Coastal and Inland Arctic Snowpacks, ACS Earth Space Chem., 2, 1075–1086, https://doi.org/10.1021/acsearthspacechem.8b00083, 2018.
Peterson, P. K., Hartwig, M., May, N. W., Schwartz, E., Rigor, I., Ermold, W., Steele, M., Morison, J. H., Nghiem, S. V., and Pratt, K. A.: Snowpack measurements suggest role for multi-year sea ice regions in Arctic atmospheric bromine and chlorine chemistry, Elem. Sci. Anth., 7, 14, https://doi.org/10.1525/elementa.352, 2019.
Pilz, C., Düsing, S., Wehner, B., Müller, T., Siebert, H., Voigtländer, J., and Lonardi, M.: CAMP: an instrumented platform for balloon-borne aerosol particle studies in the lower atmosphere, Atmos. Meas. Tech., 15, 6889–6905, https://doi.org/10.5194/amt-15-6889-2022, 2022.
Pilz, C., Cassano, J. J., de Boer, G., Kirbus, B., Lonardi, M., Pöhlker, M., Shupe, M. D., Siebert, H., Wendisch, M., and Wehner, B.: Tethered balloon measurements reveal enhanced aerosol occurrence aloft interacting with Arctic low-level clouds, Elem. Sci. Anth., 12, 00120, https://doi.org/10.1525/elementa.2023.00120, 2024.
Pöhler, D., Vogel, L., Frieß, U., and Platt, U.: Observation of halogen species in the Amundsen Gulf, Arctic, by active long-path differential optical absorption spectroscopy, P. Natl. Acad. Sci. USA, 107, 6582–6587, https://doi.org/10.1073/pnas.0912231107, 2010.
Pohorsky, R., Baccarini, A., Tolu, J., Winkel, L. H. E., and Schmale, J.: Modular Multiplatform Compatible Air Measurement System (MoMuCAMS): a new modular platform for boundary layer aerosol and trace gas vertical measurements in extreme environments, Atmos. Meas. Tech., 17, 731–754, https://doi.org/10.5194/amt-17-731-2024, 2024.
Pratt, K. A., Custard, K. D., Shepson, P. B., Douglas, T. A., Pöhler, D., General, S., Zielcke, J., Simpson, W. R., Platt, U., Tanner, D. J., Gregory Huey, L., Carlsen, M., and Stirm, B. H.: Photochemical production of molecular bromine in Arctic surface snowpacks, Nat. Geosci., 6, 351–356, https://doi.org/10.1038/ngeo1779, 2013.
Rantanen, M., Karpechko, A. Yu., Lipponen, A., Nordling, K., Hyvärinen, O., Ruosteenoja, K., Vihma, T., and Laaksonen, A.: The Arctic has warmed nearly four times faster than the globe since 1979, Communications Earth & Environment, 3, 168, https://doi.org/10.1038/s43247-022-00498-3, 2022.
Raso, A. R. W., Custard, K. D., May, N. W., Tanner, D., Newburn, M. K., Walker, L., Moore, R. J., Huey, L. G., Alexander, L., Shepson, P. B., and Pratt, K. A.: Active molecular iodine photochemistry in the Arctic, P. Natl. Acad. Sci. USA, 114, 10053–10058, https://doi.org/10.1073/pnas.1702803114, 2017.
Rolph, G., Stein, A., and Stunder, B.: Real-time Environmental Applications and Display sYstem: READY, Environ. Model. Softw., 95, 210–228, https://doi.org/10.1016/j.envsoft.2017.06.025, 2017.
Sander, R., Burrows, J., and Kaleschke, L.: Carbonate precipitation in brine – a potential trigger for tropospheric ozone depletion events, Atmos. Chem. Phys., 6, 4653–4658, https://doi.org/10.5194/acp-6-4653-2006, 2006.
Schroeder, W. H., Anlauf, K. G., Barrie, L. A., Lu, J. Y., Steffen, A., Schneeberger, D. R., and Berg, T.: Arctic springtime depletion of mercury, Nature, 394, 331–332, https://doi.org/10.1038/28530, 1998.
Seabrook, J. and Whiteway, J.: Influence of mountains on Arctic tropospheric ozone, J. Geophys. Res.-Atmos., 121, 1935–1942, https://doi.org/10.1002/2015JD024114, 2016.
Seinfeld, J. H. and Pandis, S. N.: Atmospheric Chemistry and Physics: From Air Pollution to Climate Change, 3rd edn., John Wiley & Sons, 1152 pp., ISBN 978-1-118-94740-1, 2016.
Sen, P. K.: Estimates of the Regression Coefficient Based on Kendall's Tau, J. Am. Stat. Assoc., 63, 1379–1389, https://doi.org/10.1080/01621459.1968.10480934, 1968.
Seo, S., Richter, A., Blechschmidt, A.-M., Bougoudis, I., and Burrows, J. P.: Spatial distribution of enhanced BrO and its relation to meteorological parameters in Arctic and Antarctic sea ice regions, Atmos. Chem. Phys., 20, 12285–12312, https://doi.org/10.5194/acp-20-12285-2020, 2020.
Shapley, L. S.: 17. A Value for n-Person Games, in: Contributions to the Theory of Games (AM-28), Volume II, edited by: Kuhn, H. W. and Tucker, A. W., Princeton University Press, Princeton, 307–318, https://doi.org/10.1515/9781400881970-018, 1953.
Simpson, W. R., Alvarez-Aviles, L., Douglas, T. A., Sturm, M., and Domine, F.: Halogens in the coastal snow pack near Barrow, Alaska: Evidence for active bromine air-snow chemistry during springtime, Geophys. Res. Lett., 32, L04811, https://doi.org/10.1029/2004GL021748, 2005.
Simpson, W. R., Carlson, D., Hönninger, G., Douglas, T. A., Sturm, M., Perovich, D., and Platt, U.: First-year sea-ice contact predicts bromine monoxide (BrO) levels at Barrow, Alaska better than potential frost flower contact, Atmos. Chem. Phys., 7, 621–627, https://doi.org/10.5194/acp-7-621-2007, 2007a.
Simpson, W. R., von Glasow, R., Riedel, K., Anderson, P., Ariya, P., Bottenheim, J., Burrows, J., Carpenter, L. J., Frieß, U., Goodsite, M. E., Heard, D., Hutterli, M., Jacobi, H.-W., Kaleschke, L., Neff, B., Plane, J., Platt, U., Richter, A., Roscoe, H., Sander, R., Shepson, P., Sodeau, J., Steffen, A., Wagner, T., and Wolff, E.: Halogens and their role in polar boundary-layer ozone depletion, Atmos. Chem. Phys., 7, 4375–4418, https://doi.org/10.5194/acp-7-4375-2007, 2007b.
Simpson, W. R., Brown, S. S., Saiz-Lopez, A., Thornton, J. A., and Glasow, R.: Tropospheric halogen chemistry: sources, cycling, and impacts, Chem. Rev., 115, 4035–62, https://doi.org/10.1021/cr5006638, 2015.
Simpson, W. R., Frieß, U., Thomas, J. L., Lampel, J., and Platt, U.: Polar Nighttime Chemistry Produces Intense Reactive Bromine Events, Geophys. Res. Lett., 45, 9987–9994, https://doi.org/10.1029/2018GL079444, 2018.
Skov, H., Christensen, J. H., Goodsite, M. E., Heidam, N. Z., Jensen, B., Wahlin, P., and Geernaert, G.: Fate of elemental mercury in the arctic during atmospheric mercury depletion episodes and the load of atmospheric mercury to the arctic, Environ. Sci. Technol., 38, 2373–2382, https://doi.org/10.1021/es030080h, 2004.
Skov, H., Hjorth, J., Nordstrøm, C., Jensen, B., Christoffersen, C., Bech Poulsen, M., Baldtzer Liisberg, J., Beddows, D., Dall'Osto, M., and Christensen, J. H.: Variability in gaseous elemental mercury at Villum Research Station, Station Nord, in North Greenland from 1999 to 2017, Atmos. Chem. Phys., 20, 13253–13265, https://doi.org/10.5194/acp-20-13253-2020, 2020.
Solberg, S., Schmidbauer, N., Semb, A., Stordal, F., and Hov, Ø.: Boundary-layer ozone depletion as seen in the Norwegian Arctic in spring, J. Atmos. Chem., 23, 301–332, https://doi.org/10.1007/BF00055158, 1996.
Stein, A. F., Draxler, R. R., Rolph, G. D., Stunder, B. J. B., Cohen, M. D., and Ngan, F.: NOAA's HYSPLIT Atmospheric Transport and Dispersion Modeling System, B, Am, Meteorol, Soc,, 96, 2059–2077, https://doi.org/10.1175/bams-d-14-00110.1, 2015.
Stevenson, D. S., Young, P. J., Naik, V., Lamarque, J.-F., Shindell, D. T., Voulgarakis, A., Skeie, R. B., Dalsoren, S. B., Myhre, G., Berntsen, T. K., Folberth, G. A., Rumbold, S. T., Collins, W. J., MacKenzie, I. A., Doherty, R. M., Zeng, G., van Noije, T. P. C., Strunk, A., Bergmann, D., Cameron-Smith, P., Plummer, D. A., Strode, S. A., Horowitz, L., Lee, Y. H., Szopa, S., Sudo, K., Nagashima, T., Josse, B., Cionni, I., Righi, M., Eyring, V., Conley, A., Bowman, K. W., Wild, O., and Archibald, A.: Tropospheric ozone changes, radiative forcing and attribution to emissions in the Atmospheric Chemistry and Climate Model Intercomparison Project (ACCMIP), Atmos. Chem. Phys., 13, 3063–3085, https://doi.org/10.5194/acp-13-3063-2013, 2013.
Stohl, A.: Computation, accuracy and applications of trajectories – A review and bibliography, Atmos. Environ., 32, 947–966, https://doi.org/10.1016/s1352-2310(97)00457-3, 1998.
Stohl, A.: Characteristics of atmospheric transport into the Arctic troposphere, J. Geophys. Res., 111, D11306, https://doi.org/10.1029/2005jd006888, 2006.
Stroeve, J. and Notz, D.: Changing state of Arctic sea ice across all seasons, Environ. Res. Lett., 13, 103001, https://doi.org/10.1088/1748-9326/aade56, 2018.
Strong, C., Fuentes, J. D., Davis, R. E., and Bottenheim, J. W.: Thermodynamic attributes of Arctic boundary layer ozone depletion, Atmos. Environ., 36, 2641–2652, https://doi.org/10.1016/S1352-2310(02)00114-0, 2002.
Sviashchennikov, P. and Drugorub, A.: Long-term trends in total cloud cover in the Arctic based on surface observations in 1985–2020, Bulletin of Geography. Physical Geography Series, 22, 33–43, https://doi.org/10.12775/bgeo-2022-0003, 2022.
Swanson, W. F., Graham, K. A., Halfacre, J. W., Holmes, C. D., Shepson, P. B., and Simpson, W. R.: Arctic Reactive Bromine Events Occur in Two Distinct Sets of Environmental Conditions: A Statistical Analysis of 6 Years of Observations, J. Geophys. Res.-Atmos., 125, e2019JD032139, https://doi.org/10.1029/2019JD032139, 2020.
Swanson, W. F., Holmes, C. D., Simpson, W. R., Confer, K., Marelle, L., Thomas, J. L., Jaeglé, L., Alexander, B., Zhai, S., Chen, Q., Wang, X., and Sherwen, T.: Comparison of model and ground observations finds snowpack and blowing snow aerosols both contribute to Arctic tropospheric reactive bromine, Atmos. Chem. Phys., 22, 14467–14488, https://doi.org/10.5194/acp-22-14467-2022, 2022.
Tarasick, D. W. and Bottenheim, J. W.: Surface ozone depletion episodes in the Arctic and Antarctic from historical ozonesonde records, Atmos. Chem. Phys., 2, 197–205, https://doi.org/10.5194/acp-2-197-2002, 2002.
Theil, H.: A rank-invariant method of linear and polynomial regression analysis, Nederl. Akad. Wetensch., Proc., 53, 386–392, 1950.
The MiG Project: ERDA, The MiG Project, https://erda.au.dk/, last access: 1 July 2024.
Tschudi, M., Meier, W. N., Stewart, J. S., Fowler, C., and Maslanik, J.: EASE-Grid Sea Ice Age, Version 4, NASA National Snow and Ice Data Center Distributed Active Archive Center [data set], https://doi.org/10.5067/UTAV7490FEPB, 2019.
U.S. National Ice Center: IMS Daily Northern Hemisphere Snow and Ice Analysis at 1 km, 4 km, and 24 km Resolutions (G02156, Version 1), National Snow and Ice Data Center, Boulder, Colorado USA [data set], https://doi.org/10.7265/N52R3PMC, 2008.
Wang, S., McNamara, S. M., Moore, C. W., Obrist, D., Steffen, A., Shepson, P. B., Staebler, R. M., Raso, A. R. W., and Pratt, K. A.: Direct detection of atmospheric atomic bromine leading to mercury and ozone depletion, P. Natl. Acad. Sci. USA, 116, 14479–14484, https://doi.org/10.1073/pnas.1900613116, 2019.
Wang, X., Liu, J., Yang, B., Bao, Y., Petropoulos, G. P., Liu, H., and Hu, B.: Seasonal Trends in Clouds and Radiation over the Arctic Seas from Satellite Observations during 1982 to 2019, Remote Sens., 13, 3201, https://doi.org/10.3390/rs13163201, 2021.
Whaley, C. H., Law, K. S., Hjorth, J. L., Skov, H., Arnold, S. R., Langner, J., Pernov, J. B., Bergeron, G., Bourgeois, I., Christensen, J. H., Chien, R.-Y., Deushi, M., Dong, X., Effertz, P., Faluvegi, G., Flanner, M., Fu, J. S., Gauss, M., Huey, G., Im, U., Kivi, R., Marelle, L., Onishi, T., Oshima, N., Petropavlovskikh, I., Peischl, J., Plummer, D. A., Pozzoli, L., Raut, J.-C., Ryerson, T., Skeie, R., Solberg, S., Thomas, M. A., Thompson, C., Tsigaridis, K., Tsyro, S., Turnock, S. T., von Salzen, K., and Tarasick, D. W.: Arctic tropospheric ozone: assessment of current knowledge and model performance, Atmos. Chem. Phys., 23, 637–661, https://doi.org/10.5194/acp-23-637-2023, 2023.
Yang, X., Blechschmidt, A.-M., Bognar, K., McClure-Begley, A., Morris, S., Petropavlovskikh, I., Richter, A., Skov, H., Strong, K., Tarasick, D. W., Uttal, T., Vestenius, M., and Zhao, X.: Pan-Arctic surface ozone: modelling vs. measurements, Atmos. Chem. Phys., 20, 15937–15967, https://doi.org/10.5194/acp-20-15937-2020, 2020.
Zeng, T., Wang, Y., Chance, K., Blake, N., Blake, D., and Ridley, B.: Halogen-driven low-altitude O3 and hydrocarbon losses in spring at northern high latitudes, J. Geophys. Res.-Atmos., 111, D17313, https://doi.org/10.1029/2005JD006706, 2006.
Zhao, X., Strong, K., Adams, C., Schofield, R., Yang, X., Richter, A., Friess, U., Blechschmidt, A.-M., and Koo, J.-H.: A case study of a transported bromine explosion event in the Canadian high arctic, J. Geophys. Res.-Atmos., 121, 457–477, https://doi.org/10.1002/2015JD023711, 2016.
Zilker, B., Richter, A., Blechschmidt, A.-M., von der Gathen, P., Bougoudis, I., Seo, S., Bösch, T., and Burrows, J. P.: Investigation of meteorological conditions and BrO during ozone depletion events in Ny-Ålesund between 2010 and 2021, Atmos. Chem. Phys., 23, 9787–9814, https://doi.org/10.5194/acp-23-9787-2023, 2023.