the Creative Commons Attribution 4.0 License.
the Creative Commons Attribution 4.0 License.
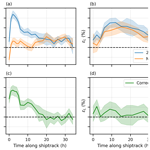
Weak liquid water path response in ship tracks
Edward Gryspeerdt
Peter Manshausen
Philip Stier
Tristan W. P. Smith
The assessment of aerosol–cloud interactions remains a major source of uncertainty in understanding climate change, partly due to the difficulty in making accurate observations of aerosol impacts on clouds. Ships can release large numbers of aerosols that serve as cloud condensation nuclei, which can create artificially brightened clouds known as ship tracks. These aerosol emissions offer a “natural”, or “opportunistic”, experiment to explore aerosol effects on clouds, while also disentangling meteorological influences. Utilizing ship positions and reanalysis wind fields, we predict ship track locations, colocating them with satellite data to depict the temporal evolution of cloud properties after an aerosol perturbation. Repeating our analysis for a null experiment does not necessarily recover zero signal as expected; instead, it reveals subtleties between different null-experiment methodologies. This study uncovers a systematic bias in prior ship track research, due to the assumption that background gradients will, on average, be linear. We correct for this bias, which is linked to the correlation between wind fields and cloud properties, to reveal the true ship track response.
We find that, once this bias is corrected for, the liquid water path (LWP) response after an aerosol perturbation is weak on average. This has important implications for estimates of radiative forcings due to LWP adjustments, as previous responses in unstable cases were overestimated. A noticeable LWP response is only recovered in specific cases, such as marine stratocumulus clouds, where a positive LWP response is found in precipitating or clean clouds. This work highlights subtleties in the analysis of isolated opportunistic experiments, reconciling differences in the LWP response to aerosols reported in previous studies.
- Article
(1345 KB) - Full-text XML
-
Supplement
(6537 KB) - BibTeX
- EndNote
A significant uncertainty in quantifying the effective radiative forcing (ERF) due to anthropogenic activity stems from the uncertainty in cloud responses to aerosol perturbations, known as aerosol–cloud interactions (Forster et al., 2020). The primary way in which aerosols can influence clouds is by acting as cloud condensation nuclei (CCN), thereby increasing the cloud droplet number concentration (Nd) over very short timescales (Twomey, 1974). In the near-instantaneous case, the water content of the cloud remains constant; therefore, droplets become smaller on average (known as the Twomey effect; Twomey, 1977) and more reflective to incoming shortwave radiation, leading to a negative forcing on the climate's energy balance (a cooling effect; Forster et al., 2021).
However, over longer timescales, the water content of the cloud may change. Albrecht (1989) hypothesized that smaller droplets take longer to coalesce into rain droplets, implying that an aerosol perturbation would reduce the precipitation efficiency in a cloud (Rosenfeld, 2000). Consequently, this suppression of precipitation would enable a cloud to persist for a longer duration (the “lifetime effect”) and result in an increase in the liquid water path (LWP) of the cloud. This increased water content, in turn, elevates the cloud albedo, leading to a negative ERF. Nevertheless, reduced droplet size can also promote the entrainment of dry air above the cloud, causing cloud desiccation, a decreased LWP, and a warming effect (Ackerman et al., 2004; Bretherton et al., 2007). These are inherently time-dependent processes, and attempts have been made to quantify the timescales over which these competing adjustments to clouds occur (Glassmeier et al., 2021; Gryspeerdt et al., 2021).
Previous studies have found a range of potential LWP responses to aerosols. Some studies, such as Small et al. (2009), Chen et al. (2012, 2014), Sato et al. (2018), and Wall et al. (2022), suggest that the LWP will decrease following an aerosol perturbation. Others, such as Quaas et al. (2009), Koren et al. (2014), Grosvenor et al. (2017), Neubauer et al. (2017), McCoy et al. (2018), Rosenfeld et al. (2019), Gryspeerdt et al. (2021), Zipfel et al. (2022), and Manshausen et al. (2022), argue that aerosols cause an increase in the LWP under some conditions. Some studies, however, suggest that the LWP response will be weak (Malavelle et al., 2017) or bidirectional (Ackerman et al., 2004; Michibata et al., 2016; Toll et al., 2017, 2019; Gryspeerdt et al., 2019a; Possner et al., 2020; Glassmeier et al., 2021; Zhang et al., 2022; Fons et al., 2023). Typically, modelling studies suggest a uniform increase in the LWP (Quaas et al., 2009; Michibata et al., 2016; Sato et al., 2018; Gryspeerdt et al., 2020), whereas observational studies are much more varied: large-scale studies typically find a LWP decrease (e.g. Chen et al., 2014); studies looking at the impact of effusive volcanic eruptions typically find no change in the LWP (e.g. Malavelle et al., 2017; Toll et al., 2017; Gryspeerdt et al., 2019a); and other natural experiments, such as ship track studies, find both a decrease and an increases in the LWP (e.g Christensen and Stephens, 2011; Toll et al., 2019; Christensen et al., 2022), depending on the situation.
The meteorological context in which the aerosol perturbation occurs is an important control on the sign of the LWP response, where it is typically suggested that the LWP will likely increase in clouds that are clean and precipitating or decrease in clouds that are polluted and non-precipitating (Ackerman et al., 2004; Toll et al., 2017; Gryspeerdt et al., 2019a; Possner et al., 2020). The regional dependence of the LWP response, and therefore the dependence on cloud regime, must also be considered when comparing the LWP responses between studies. Studies that investigate LWP responses to aerosols can often occur in different cloud regimes (marine stratocumulus, trade cumulus, etc.) which can have opposing responses (Lebo and Feingold, 2014). Any conclusion regarding the LWP response to an aerosol perturbation must be given in the context of the cloud regime and meteorology in which the study took place.
Reports on the LWP response to an aerosol perturbation are varied, with different methods typically obtaining different effects. In order to reduce the uncertainty in our understanding of aerosol–cloud interactions and any potential warming or cooling effects, it is important to reconcile these differences in the LWP response to aerosol perturbations. This will be vital for the assessment of the potential impacts of geoengineering (Feingold et al., 2024), as the conditions under which cooling could be induced remain a topic of uncertainty.
In this study, we investigate the LWP response to an aerosol perturbation, using ship tracks as our “natural experiment” to disentangle the meteorological covariance. Ship tracks refer to linear cloud formations often observed in the wake of ships, resulting from the release of aerosol particles into the cloud due to burnt fuel. By comparing the polluted cloud within ship tracks to the adjacent unpolluted clouds outside the tracks, one can isolate the aerosol effect on clouds (Conover, 1966; Durkee et al., 2000). A review of the use of ship tracks as natural experiments can be found in Christensen et al. (2022). Moreover, ship tracks can be regarded as linear formations of independently perturbed clouds, as no information is transmitted along their length (Kabatas et al., 2013). This characteristic allows us to consider the distance along the ship track as a time axis, through which the cloud adjustment evolution after a perturbation can be determined (as in Gryspeerdt et al., 2021, and Manshausen et al., 2022). This previous work has demonstrated that the time evolution of the cloud response to aerosol is important to consider when investigating the sign and magnitude of the response (Glassmeier et al., 2021).
Many ship track studies utilize hand-logged track positions or employ automated track detection algorithms to identify polluted pixels in satellite imagery for analysis based on their appearance as quasi-linear albedo perturbations, either manually (Segrin et al., 2007; Christensen et al., 2009; Christensen and Stephens, 2011, 2012) or using machine learning (Watson-Parris et al., 2022; Yuan et al., 2022). Manshausen et al. (2022) address the potential selection bias that these studies may have, as only the cloud response in visible tracks is considered. Recent work (Gryspeerdt et al., 2021; Manshausen et al., 2022) predicts ship track locations by advecting historical ship positions in reanalysis wind fields, thereby allowing a much greater number of tracks to be analysed.
The majority of these ship track studies split the cloud scene into clouds that are polluted (inside the ship track) and unpolluted (outside the ship track) by the ship emissions. They then investigate the relative anomalies of cloud properties inside and outside the ship track in order to separate the aerosol effect from the covarying background meteorology. However, in doing so, these studies assume that the background gradients in the cloud properties will be linear, on average. This relies on the assumption that ship tracks are randomly oriented with respect to background gradients in cloud properties and, therefore, that the “average” ship track will have a linear background gradient. This assumption is investigated in this work.
In this study, we establish the temporal development of the Nd and LWP in ship tracks in the Atlantic Ocean. As in Gryspeerdt et al. (2021), we use ship positions from transponder data (Smith et al., 2015), which are advected in 3D with ERA5 reanalysis wind fields (Hersbach et al., 2020) to predict ship track locations. Following Manshausen et al. (2022), we place no conditions on the ship tracks being visible in the satellite data; instead, we look at the combined effect of all visible and “invisible” tracks. We colocate these ship track locations with Moderate Resolution Imaging Spectroradiometer (MODIS) Aqua and Terra satellite overpasses (Platnick et al., 2017) to build up a composite image of the time evolution of cloud properties in ship tracks. To assess the impact of background cloud variation, we conduct a null experiment using ship locations from one year and cloud and wind data from a different year, effectively “sailing” the ships through the wrong year of wind and satellite data. We investigate any false signals seen in the null-experiment composite; moreover, by considering an alternative null-experiment methodology, we isolate the cause of the false signal, revealing the importance of considering the background gradients in the cloud properties when analysing ship tracks. Using our correct null experiment to account for the natural covariability in clouds and wind fields, we isolate the causal aerosol impact on the Nd and LWP across the Atlantic. We investigate the conditions controlling the sign and magnitude of the response and use our corrected Nd and LWP responses to place an estimate on the radiative forcing from LWP adjustments to changes in Nd.
2.1 Ship track location prediction
This work predicts ship track locations using a similar method to that of Gryspeerdt et al. (2019b, 2021), utilizing over 35 000 ships from automatic identification system (AIS) transponder data in 2018, filtered to include specific ship types (large container vessels, bulk carriers, oil tankers, cruise ships, and general cargo ships; Smith et al., 2015). The region of interest for this study is chosen to be the same as in Manshausen et al. (2022, 2023), to enable direct comparison of results. This region in the Atlantic Ocean is bounded by 50° S–50° N and 90° W–20° E and contains both stratocumulus and trade cumulus regimes.
We advect these ship locations forward in time for 36 h using ERA5 reanalysis wind fields (Hersbach et al., 2020). This provides us with not only the predicted ship track location but also information about the time since that position of the ship track experienced the ship aerosol perturbation. Any errors in the interpolation of ship location data from AIS will lead to incorrect ship track locations; therefore, the resultant ship tracks are filtered to exclude cases in which ships were moving unrealistically fast (with an apparent ship velocity of more than 40 knots). There will be some small additional uncertainty in this “time since aerosol perturbation” if there is no relative motion between the ships and the clouds; however, we estimate this to occur in a small number of cases.
Vertical advection of ship plume
As a modification to the methods of previous studies that predict ship track locations, we impose vertical motion of the ship plume within our advection scheme. In the work of Gryspeerdt et al. (2021), the ship emission locations are advected using 1000 hPa wind fields, thereby making an assumption of a constant plume height. Manshausen et al. (2022) aims to incorporate vertical motion by employing the HYSPLIT model (Stein et al., 2015), which relies on advection in the ERA5 vertical wind fields (Hersbach et al., 2020). However, these vertical wind fields are often close to zero, particularly in stratocumulus regions (due to low model resolution in ERA5), leading to minimal vertical rise in the resulting trajectories. In contrast, this research introduces plume rise to the advection scheme, ensuring that the emission positions are advected at increasing heights along the length of the track. The plume rise equation used in this study is given by Briggs (1965):
Here, H(t) is the height of the plume, t is the time along ship track, F0 is the buoyancy flux (840 m4 s−3), β is the entrainment rate (0.3), k is the added mass coefficient (1), and U0 is the relative wind speed (using a representative value of 10 m s−1). Furthermore, the vertical motion of the ship track is capped at the boundary layer height from ERA5, ensuring that the plume is advected with the boundary layer, rather than higher-level wind fields.
2.2 Data
Ship positions are advected in ERA5 reanalysis wind fields at a 0.25° resolution and 3 h intervals between the surface and the boundary layer top, which is also obtained from ERA5 (Hersbach et al., 2020). Cloud property data utilized in this study were acquired from NASA's Aqua and Terra satellites, equipped with MODIS. We locate our ship tracks in MODIS Aqua and Terra satellite imagery, leaving us with roughly 52 000 MODIS granules containing approximately 4 000 000 tracks. Cloud properties were extracted from the Level-2 Collection 6.1 dataset (MYD06L2 and MOD06L2, corresponding to Aqua and Terra, respectively; Platnick et al., 2017). To ensure data quality, a filtering process was applied based on the “Cloud_Multi_Layer Flag”, allowing only clear or single-layer cloud scenes, and restrictions on solar and sensor zenith angles (solar zenith angle < 65° and sensor zenith angle < 55°) were imposed to minimize potential retrieval biases (Grosvenor and Wood, 2014). To minimize the impact of the bow-tie effect on pixel geolocation (Sayer et al., 2015), we regrid the MODIS data to a 5 km resolution. Additionally, we filtered our data to include only low-level clouds with cloud tops below 700 hPa.
The Nd and LWP are calculated using MODIS effective radius and cloud optical thickness retrievals, following Quaas et al. (2006) and Grosvenor et al. (2018). Estimated inversion strength (EIS) was calculated from the potential temperature at 700 hPa and the potential temperature at the surface (Wood and Bretherton, 2006), which were obtained from ERA5 reanalysis data. These data are only used to filter our ship tracks into different stability scenes in Sect. 3.2.2. The resolution of the ERA5 data is coarser than our central ship track region (roughly 25 km); however, as we only consider the EIS values in regions outside of the track, this should not be an issue.
2.3 Quantifying ship impacts on cloud
For each ship track, we investigate how cloud properties vary with perpendicular distance away from the centre of track (in a similar method to that of Segrin et al., 2007). We define the distance left of the ship track (with respect to the direction of travel of the ship at the head of the track) as negative, whereas the distance right of the ship track is defined as positive. Additionally, we use the associated time along the ship track to grid our MODIS data into 2D space – binning our cloud properties in time along and with distance away from each ship track. These data are combined for all tracks to produce a “composite” ship track.
We define the polluted region inside the composite ship track as the area within 5 km of the centre of the track, whereas the clean outside region is defined as the area 30–60 km away from the centre of the track. This clean outside region is assumed to be representative of the cloud properties at the track location if there was no ship track present. This means that we can isolate the aerosol impact on the clouds in the ship track, separating it from any changes in the surrounding meteorology.
We calculate the enhancement of cloud properties inside the track as the percentage difference between these polluted and clean regions. We define our enhancements in the Nd and LWP as ϵN and ϵL, respectively. We calculate the enhancement from the composite ship track, rather than compositing individual enhancements in order to avoid errors (as the operations of calculating the mean of a distribution and calculating the ratio of two distributions are non-commutable; Manshausen et al., 2022). Errors in the enhancements are calculated using a bootstrapped method with 1000 samples (Efron, 1979).
There are subtleties in the method used to combine all ship tracks into a composite ship track that can significantly impact the calculated track enhancements. We summarize these subtleties in the following paragraphs.
Firstly, compositing every ship track means combining any background gradients in the cloud scene for each ship track. If the cross-track gradients are linear (i.e. the gradient in the cloud property in the direction perpendicular to the ship track) when compositing all of the ship tracks together, the composite ship track background will also be linear, allowing us to consider either side of the track equivalently. This is demonstrated in Fig. 1a, where combining linear trends results in a linear composite. However, if the cross-track gradients are non-linear, compositing all of the ship tracks together will create a non-linear composite background (Fig. 1b) if the ship tracks are not randomly oriented with respect to the gradient.
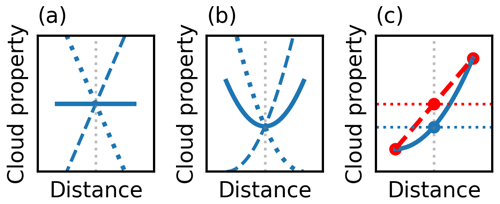
Figure 1Subtleties in compositing cross-track background gradients in cloud properties. (a) Linear gradients will combine to form a linear composite background. (b) Non-linear gradients will combine to form non-linear composite backgrounds. (c) Any non-linearity in the composite background gradient will lead to false positive or negative enhancements in the composite ship track. In panels (a) and (b), dotted or dashed blue lines represent the background gradients from individual ship tracks, whereas the solid blue line represents the composite background gradient when these gradients are combined. In panel (c), the solid blue line represents the composite background gradient, whereas the red dashed line represents the average outside track value if a liner fit is assumed.
Secondly, any non-linearity in the cross-track background gradient will lead to false positive/negative enhancements in the composite ship track (Fig. 1c). If the background gradient is concave (convex), the average value outside of the track will be greater (less) than the average value inside the track, even when there is no ship track present. This will lead to a false positive (negative) enhancement in the composite ship track.
2.4 Null experiments
In order to ensure that any signal we see is due to the ship emissions and not a background effect, we repeat the same analysis for a null experiment. We require three components to conduct this ship track analysis: ship locations, reanalysis wind fields (to advect the ship locations and predict the track locations), and satellite data (from the advected ship track locations). When considering a null experiment, the correlations between these three components are important to consider, as subtle differences can bias results.
The null experiment chosen for this study uses the same ship locations as the real case (from 2018), but it employs the wind fields and MODIS data from 2019. The resultant null-experiment ship tracks predicted will most likely be incorrect and will not fall in the same locations as any actual ship tracks, revealing any potential effects from our ship track orientations and the background gradients in the cloud properties and testing the assumption that ship tracks will be randomly oriented. Assuming that the ship routes are only weakly constrained by weather conditions, this is equivalent to sailing our ships through a completely different year; therefore, the predicted ship tracks are very unlikely to align with any real tracks. There is the possibility of a small localized impact in any shipping corridors, although only when ship directions and wind fields are closely aligned. We expect this effect to be small in comparison to the total number of tracks.
We also investigate the sensitivity of our results due to the choice of year used for the null experiment by repeating our null experiment with 2017 data (see Fig. S5 in the Supplement). We find that, whilst there is some interannual variability, it does not significantly impact the results of this study.
Manshausen et al., 2023Table 1Sources of data for the cases analysed in this study. The 2018 ship tracks use ship locations, wind fields, and MODIS data from 2018. The null experiment of this study, referred to as 2019, uses ship locations from 2018 but wind fields and MODIS data from 2019. The alternative uncorrelated null experiment uses ship locations from 2018, but it employs wind fields from 2018 to predict the track locations and MODIS data from 2019.
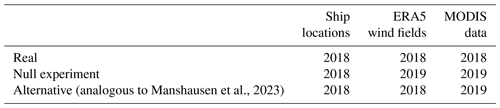
The null-experiment methodology in this study differs from Manshausen et al. (2023), who consider a null experiment that uses ship locations and wind fields from a certain day to predict their ship track locations but employs satellite data from the day before (therefore wind fields and satellite data will be uncorrelated). In this study, we retain the correlation between the wind fields and satellite data, as this correlation will be present in the true ship track case. Table 1 summarizes the sources of data for the cases analysed in this study.
We calculate our corrected ship track response by calculating the difference between our real ship track case and the null experiment. This will remove any false enhancements due to background effects and isolate the response of the cloud to the aerosol perturbation.
3.1 Impact of null-experiment choices
3.1.1 Microphysical response
The time evolution in the Nd and LWP enhancements is produced for up to 36 h after the aerosol perturbation, for both the ship track case and the null experiment (see Fig. 2a and b). We bin the Nd data into 1 h bins for the first 5 h and then into 2 h bins for the remaining time along the track. Due to the noise in LWP data, we use 2 h bins for the first 5 h and then 3 h bins for the remaining time.
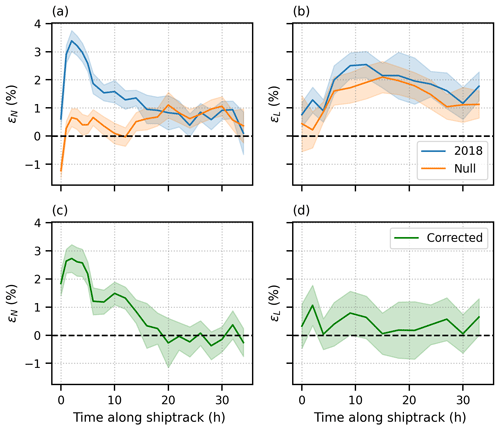
Figure 2(a) Nd and (b) LWP anomalies within ship tracks in 2018 as a function of time since aerosol perturbation (shown in blue) and the background trend found in the 2019 null experiment (shown in orange). The 2019 null experiment uses ship locations from 2018 but wind fields and cloud data from 2019. The LWP response in the true ship track case and null experiment is found to be very similar with respect to magnitude and time dependence. Corrected (c) Nd and (d) LWP anomalies within ship tracks in 2018 as a function of time since aerosol perturbation. Responses are corrected by taking away the background signal, which is calculated from the 2019 null experiment.
The Nd evolution is similar to that found in previous studies (Gryspeerdt et al., 2021; Manshausen et al., 2022, 2023), with a large increase in the droplet number inside the ship track within the first 2–3 h and a subsequent decay back to the background state over the following 20 h. The null experiment shows a constant enhancement in Nd of roughly 0.5 %, rather than recovering the null signal expected from the absence of any ship tracks. We observe a positive LWP anomaly that increases in magnitude for roughly 20 h along the length of the ship track before decreasing. Surprisingly, we observe a very similar LWP response in the null experiment, with very similar evolution in time along the track, despite the absence of any significant aerosol perturbation (solely any small effect from shipping corridors).
The appearance of both an Nd and a LWP enhancement inside the ship track region in the null experiment, despite the lack of any aerosol emissions, highlights a potential bias in previous work. Previous studies depend on the assumption that the clean background cloud state can be identified by a linear average of the cloud conditions either side of the track when compositing millions of ship tracks. The presence of an enhancement in the null experiment suggests that this assumption may not be valid.
As previous studies (Manshausen et al., 2023) used a similar null-experiment method to account for this effect and found no Nd or LWP response, this discrepancy suggests that the source of the bias lies in the method by which the null experiment is calculated. We isolate this bias, as well as its subtleties, in the following sections.
3.1.2 Background gradients
The method by which enhancements are calculated involves taking an average of the cloud properties in the regions 30–60 km away from the centre of the composite ship track (on either side of the track) and calculating the percentage difference from the central 10 km region. The purpose of the outside region is to estimate what the cloud properties at the track location would have been if there was no ship track present.
However, if there is any non-linearity in the background gradient, this will introduce an overestimation or underestimation of the actual value at the centre, which will over- or underestimate the signal in the centre of the track, via the proposed mechanism shown in Fig. 1c. In essence, our estimate of what the cloud properties would have been at the track location if there was no ship track present will be incorrect and, therefore, the enhancement calculated will be biased.
Figure 3 demonstrates this for the null experiment (the case in which no ship tracks are present) in this study. As no ship tracks would be present in this experiment, we are solely seeing the impacts of the non-linear background gradients. In Fig. 3a and b, the Nd gradient in the composite is plotted at early times along the track (between 0 and 5 h) and at later times along the track (between 15 and 20 h). Figure 3c and d show the same but for the LWP.
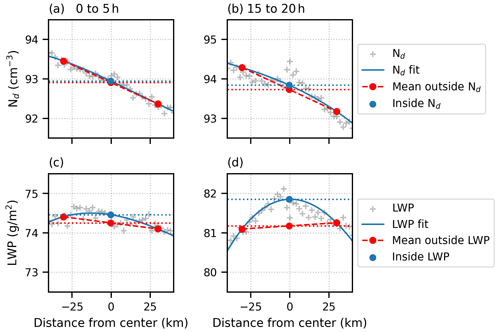
Figure 3For our composite null experiment (with incorrect ship locations), we take a slice at early times along the track (0–5 h; panels a and c) and later times along the track (15-20 h; panels b and d); we then plot the observed Nd and LWP as a function of distance from the centre of the track (grey crosses). As shown by the solid blue lines, we plot a polynomial fit (order 3) to the data to demonstrate the non-linearity of the background gradients. As displayed using the dashed red lines, we plot a linear fit calculated from the average outside track values (at 30 km from the centre of the track). The difference between the dotted blue and red horizontal lines represents the overestimation in the centre of the track due to the non-linearity in the background gradient and, thus, a false positive enhancement. This false positive enhancement is relatively constant with time along the track for the Nd, but it increases in magnitude for later times along the track for the LWP.
The trends in the Nd and LWP enhancements in the null experiment (orange lines in Fig. 2a and b) can be explained by how the non-linearity in the composite background gradient changes with time along the track. The Nd gradient is non-linear, thereby producing a small false positive enhancement in the centre of the track (Fig. 3a). The non-linearity of this gradient increases slightly but does not change significantly with time along the track; therefore, the false positive enhancement also only increases slightly with time along the track (Fig. 3b), as seen in Fig. 2a. There is a small peak in the Nd in the centre of the null-experiment track, which could possibly be attributed to the presence of shipping corridors.
The LWP gradient, however, is slightly non-linear at early times along the track, also producing a false enhancement (Fig. 3c), but becomes increasingly non-linear with time along the track (Fig. 3d), causing the magnitude of the LWP enhancement to increase with time (Fig. 2b). This null experiment reveals that the enhancement seen in the LWP response is actually a measure of the non-linearity of the background LWP gradient, not an aerosol effect.
The surprising similarity between the LWP response in the null-experiment and real ship track case suggests that previous conclusions regarding the LWP response in ship tracks may be due to this false signal, rather than a true response to the aerosol perturbation. The increasing LWP in ship tracks observed in Gryspeerdt et al. (2021) and Manshausen et al. (2022, 2023) up to 20 h post-perturbation is similar to the LWP responses observed in the null-experiment and ship track cases in this work, suggesting that these previous studies may suffer from this bias. However, it is likely that any ship track study that calculates relative anomalies in a way similar to this study will suffer from this bias.
3.1.3 Correlations between the LWP and wind fields
We attribute the source of this bias to the assumption that ship tracks are randomly oriented with respect to the background gradients in cloud properties and, therefore, that the composite ship track background gradient will be linear. This assumption will not be valid if there is a correlation between the ship track locations and the cloud property data retrieved. We investigate this by considering the correlation between the wind fields and the local maxima in the LWP.
Figure 4 shows the regional distribution of the LWP enhancement in the null experiment (where there should be no enhancements) and the correlation between the ERA5 wind fields and the second derivative in the LWP (local maxima) in the Atlantic region. The metric for correlation is calculated by multiplying the second latitude (longitude) derivative by the wind speed orthogonal to the derivative direction, i.e. the zonal (meridional) wind speed component. We then take the length of the resulting two-component vector as the measure of correlation. For differentiation, we use the second-order accurate central differences method implemented in numpy.gradient.
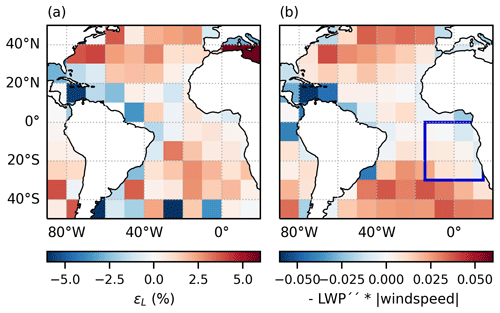
Figure 4(a) Regional enhancements in the null-experiment ship tracks, averaged over the 36 h length of the track and the central track location binned to 10°. (b) Correlation between the second derivative in the LWP (local maxima) and wind speed (from ERA5). The regional distribution of the LWP enhancement matches very closely to that of the correlation between the maxima in the LWP and wind fields, suggesting that this is the reason for the non-linear background gradients in the composite. The navy box indicates the south-east Pacific stratocumulus region investigated in Sect. 3.2.
The regional distribution of the LWP enhancement in the null experiment matches very closely to the correlation between the wind fields and the local maxima in LWP, suggesting that this is the reason for the non-linear background gradients in the composite. We see the greatest false enhancements in the locations where the correlation between the wind fields and clouds is strongest and, therefore, the composite background gradients are the most non-linear.
This result invalidates the assumption that averaging many ship tracks will produce a linear background gradient. Ship track locations are inherently a function of the wind fields in which they are advected and, therefore, will be correlated with the clouds in which they are found.
Manshausen et al. (2023) do not observe LWP enhancements in their null experiment. In this null experiment, the ship positions and wind fields are from the same day, but the satellite data are from the day before. This means that there will be little correlation between the wind fields used to predict the track locations and the cloud properties retrieved; therefore, when compositing all the ship tracks, the cross-track gradients do average out to zero. This is in contrast with the null experiment used in this study, where the ship locations are from 2018 but the wind fields and satellite data are from 2019. We retain the correlation between the wind fields and the cloud properties in our null experiment and, therefore, reveal the bias due to the non-linear background gradients.
Repeating an analogous null experiment to Manshausen et al. (2023) (details can be found in Table 1), we find a very weak LWP response (Fig. S1), further suggesting that the correlation between wind fields and cloud properties on a given day is the source of the bias. Additionally, we find very little correlation when we consider the mean wind fields and mean LWP maxima, highlighting the importance of considering daily correlations (see Fig. S2a) and individual weather systems.
This demonstrates the importance of correlations between cloud properties and wind fields, as all ship track studies will suffer from this bias when calculating enhancements inside the track compared with unpolluted regions on either side of the track, regardless of the method used to predict the track locations or whether the time dependence of the response is investigated. We only begin to see the significance of this effect when exploring the time evolution. At longer times along the track, the ship track position is a greater function of the wind fields in which it is advected and is less dependent on the initial ship position. Thus, the correlation between the cloud properties and the wind field becomes more significant. This can be seen in Fig. 2, where the LWP enhancement increases with time.
Whilst this effect will be present in all ship track studies that assume a linear background gradient, it will be much more significant in studies that consider all ship tracks, not just those that are visible. When considering all tracks, the ship track signal will be much smaller, and the dominant effect will be due to the non-linear background gradients.
It is worth noting that we would still see this false signal if we conducted our analysis by considering the enhancements in Nd and LWP for each individual ship track and then averaged these to obtain the composite (rather than compositing each ship track and then calculating the enhancement). This is because each individual ship track would still have a non-linear background gradient; therefore, the individual enhancements, whilst noisier, would still contain this bias.
3.2 Isolating the aerosol effect
We make the assumption that the non-linear background gradients in our null experiment are representative of this bias; thus, we subtract the null-experiment enhancement from the 2018 ship track enhancement to isolate the response of the cloud to the aerosol perturbation. The corrected Nd and LWP responses can be found in Fig. 2c and d.
Comparing the 2018 responses and the corrected response, we see that the Nd response remains largely similar in shape, with only a 3 % enhancement in the droplet number concentration after 2–3 h. The LWP response, however, remains weak (roughly 0.5 %) for all times and shows very little evolution over time, as opposed to the strong positive LWP response seen in the uncorrected case.
Whilst the LWP response shows small changes over time, the sensitivity due to changes in the droplet number will show some time dependence due to the time evolution of the droplet number perturbation, which is consistent with Glassmeier et al. (2021).
To investigate if we can observe a stronger LWP response, we filter our ship tracks into those that occur in polluted/clean backgrounds, stable/unstable environments, and precipitating/non-precipitating environments. We find that there is little impact of these factors on the LWP when averaging across the entire Atlantic region, with the LWP response remaining noisy and close to zero for all times (see Fig. S3). This suggests that, when averaging over all clouds in this large region, there is no control on the LWP response because so many clouds are insensitive to the aerosol perturbation.
However, when we consider a smaller subregion of the Atlantic, we recover a LWP response under certain conditions. We select a region bounded by 30° S–0° S and 15° W–15° E, which contains a large number of ship tracks in the marine stratocumulus deck in the South Atlantic (see box in Fig. 4b). This region is chosen as it contains a large number of ship tracks in a single cloud regime; therefore, we can investigate the controls on the LWP response in this regime. The results are presented in the following subsections and in Fig. 5.
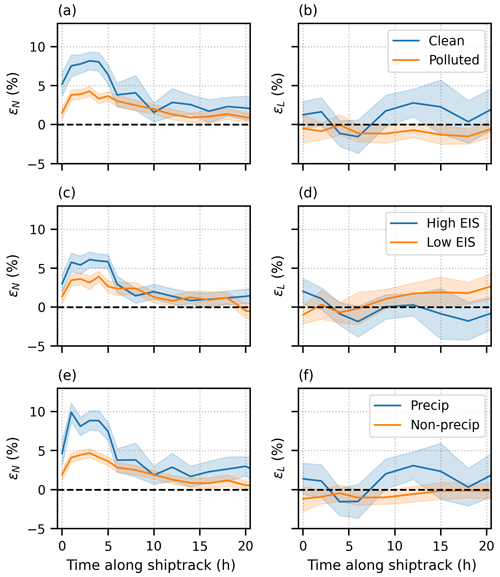
Figure 5Time evolution of the Nd and LWP responses in (a, b) polluted and clean, (c, d) stable (high-EIS) and unstable (low-EIS), and (e, f) precipitating and non-precipitating background environments, for the marine stratocumulus subregion in the South Atlantic.
3.2.1 Background Nd
We subset our ship tracks into those that occur in polluted and clean backgrounds. We define the outside unpolluted region of each ship track as the distance between 30 and 60 km away from the ship track and then calculate the average Nd in this region. Following this, we filter each ship track based in this background Nd. We consider those with a background Nd > 100 cm−3 as polluted and those with an Nd < 50 cm−3 as clean.
Figure 5a and b show the time evolution of the Nd and LWP responses in polluted and clean background environments in a marine stratocumulus subregion. When considering this marine stratocumulus region, we find much greater enhancements in Nd than seen in the entire Atlantic composite. We see greater maximum enhancement in Nd under clean conditions (roughly 8 %) compared with polluted conditions (roughly 4 %).
We find a non-zero LWP response in the marine stratocumulus region, with clean background clouds experiencing an increase in the liquid water content and polluted clouds experiencing a slight decrease in the liquid water content. This is consistent with there being a greater enhancement of entrainment in polluted regions, whereas the precipitation suppression mechanism is more dominant in clean regions, where there is more frequent drizzle to suppress due to smaller droplet number concentrations but greater droplet effective radii.
3.2.2 Inversion strength
Previous studies have suggested that boundary layer stability could potentially be a control on the strength of the cloud response to an aerosol perturbation (Toll et al., 2019; Possner et al., 2020; Manshausen et al., 2022). Using the estimated inversion strength (EIS) as a measure of atmospheric stability, we separate the ship tracks into those that occur in high-EIS (> 3.5 K, stable) and low-EIS (< 3.5 K, unstable) backgrounds, with Fig. 5c and d showing the time evolution of the Nd and LWP responses in these different backgrounds. We use the same definition of outside track region as in Sect. 3.2.1.
We find that there is a weakly negative LWP enhancement in stable environments and a weakly positive LWP enhancement in unstable environments. In both cases, however, the LWP response is weak and difficult to distinguish from the noise. Manshausen et al. (2022) found that there is a negative LWP anomaly in high-EIS environments and a roughly zero LWP anomaly in unstable environments, whereas Toll et al. (2017) and Possner et al. (2020) found negative LWP responses in deeper boundary layers, which are commonly associated with lower EIS. We also see similar results to Manshausen et al. (2022) in the Nd response, with a greater enhancement in the droplet number concentration in stable environments compared with unstable environments. This is consistent with stronger inversions occurring in shallower boundary layers and cleaner environments.
3.2.3 Precipitation
We define a precipitating background as one with an average cloud effective radius (CER) greater than 15 µm, whereas a non-precipitating background is defined as one with a CER of less than 15 µm (as in Toll et al., 2017). Figure 5e and f show the time evolution of the Nd and LWP responses in these different backgrounds. Manshausen et al. (2023) require both inside and outside tracks to have a CER > 15 µm to define precipitating clouds, as cutting off the lower CER region of the distribution will lead to a bias in calculating the enhancements. We address this issue through the subtraction of the background signal from our null experiment, which would contain a similar bias; therefore, the difference between the 2018 data and the null experiment should leave an unbiased signal.
We find that the Nd response is greater in precipitating cases, with a 9 % enhancement in Nd after 2–3 h, compared with a 5 % enhancement in non-precipitating cases. The LWP response is positive in precipitating backgrounds and weakly negative in non-precipitating backgrounds. This is consistent with the precipitation suppression mechanism – when background clouds are precipitating, this will be suppressed by smaller droplets on average being smaller (Albrecht, 1989) and causes an increase in the LWP. The timescale for this onset appears to be roughly 6 h. Wang and Feingold (2009a) observe an enhancement in the LWP in clean clouds that begins roughly 5 h after the perturbation and is consistent with the LWP response of clean and precipitating clouds in this study. Gryspeerdt et al. (2021) find a faster LWP response to the Nd perturbation (on the order of 2 h); however, it is likely that their study suffers from the non-linear background gradient bias identified in this work and, therefore, that the timescales of this response are potentially inaccurate.
When background clouds are non-precipitating, there is no precipitation to suppress; this may drive the slight decrease in the LWP due to the enhancement in entrainment, as is consistent with the negative LWP in polluted regions (Fig. 5b). Manshausen et al. (2023) find similar results, with a positive LWP response in precipitating clouds and roughly zero LWP anomalies for non-precipitating clouds.
3.3 Radiative forcing
Following the method of Manshausen et al. (2022), we calculate the sensitivity of the LWP to Nd for four equally sized EIS bins (defined in Table S1). We do not see the EIS having a strong control on the LWP response as was seen in Manshausen et al. (2022), yet we elect to use the same method for the sake of consistency. We use our enhancements in the LWP and Nd that have been corrected for the background effect, by subtracting the null-experiment response for each EIS bin.
We use all ship track observations from our region of interest (50° N–50° S and 90° W–20° E) to calculate these sensitivities, only utilizing the cloudy ship track scenes of this study.
We calculate the sensitivities using , where ϵL and ϵN are the corrected enhancements in the LWP and Nd, respectively. As in Manshausen et al. (2022), we calculate the LWP enhancement after 5 h and the Nd enhancement before 5 h to provide an upper constraint on the potential cooling from the LWP response. The Nd response is largest in the first 5 h, and in Manshausen et al. (2022), the response plateaued after 5 h. This means that, by using ϵN from the first 5 h and ϵL from after 5 h to calculate the sensitivities, we calculate an upper estimate on the forcing. Using data from all times, the estimate of the forcing would become more negative; therefore, this current method provides an upper limit.
We extrapolate these sensitivities globally to calculate an estimate of the global radiative forcing due to rapid adjustments in the LWP, following the method of Manshausen et al. (2022) and Bellouin et al. (2020). The global distribution of the sensitivity is found by considering the regional liquid cloud fraction (from MODIS), EIS (from ERA5), and our sensitivity of the LWP to Nd in each EIS bin. We use the estimation of Nd changes due to aerosols from Bellouin et al. (2020). More detailed information on the forcing calculation can be found in Manshausen et al. (2022) and Bellouin et al. (2020).
In order to investigate if there is any control on the magnitude of the forcing, we repeat this analysis with 2 and 12 equally sized EIS bins. This provides an estimate of the uncertainty in the forcing due to the choice of binning. We also investigate the sensitivity of the forcing due to the choice of year used for the null experiment (see Fig. S5) and find that the choice of year has little impact on the forcing estimate.
We obtain an estimate of the forcing (upper and lower bounds) of −0.16 (−0.29, −0.07) W m−2, which is weaker than the estimate of −0.76 (−1.03, −0.49) W m−2 found in Manshausen et al. (2022). This is consistent with the false background enhancement contributing to an overestimation of the LWP response in ship tracks and, therefore, also to the sensitivity of certain clouds to aerosol perturbations. Once we correct for this effect, we obtain much weaker LWP responses and, therefore, weaker radiative forcing estimates. However, this result still suggests a cooling effect from the LWP response to aerosol perturbations, in contrast to the estimate of +0.2 (0.0, +0.4) W m−2 from the latest Intergovernmental Panel on Climate Change report (Forster et al., 2021).
This work provides a better constraint on the response of clouds to an aerosol perturbation, in particular the liquid water path (LWP) response and its effective radiative forcing. Following methodology similar to Gryspeerdt et al. (2021) and Manshausen et al. (2022), we use ship positions data and reanalysis wind fields to predict over 4 000 000 ship track locations in the Atlantic in 2018. From these, we investigate the time evolution of the Nd and LWP in clouds after an aerosol perturbation.
Through the analysis of a null experiment, in which we sail our ships through the wind fields and satellite data of a different year, we identify a bias in ship track studies that causes an overestimation of the LWP enhancement in ship tracks. We suggest that the large positive LWP enhancements seen in trade cumulus ship tracks in Manshausen et al. (2022) are likely due to this bias and that the LWP response to aerosol in these cases is much weaker.
This effect can be attributed to the fact that non-linear cross-track background gradients in the LWP do not average out to zero when compositing many ship tracks, as they are not randomly oriented compared to the cloud field. We argue that the correlation between clouds and wind fields is the source of this bias. When considering an alternative null experiment that removes the correlations between ship track locations and cloud properties (analogous to the null experiment of Manshausen et al., 2023), we see that this LWP response disappears. This suggests that the correlation between wind fields and clouds is the source of this bias.
The subtle bias identified in this work will be prevalent in any ship track study that considers the relative anomaly of cloud properties inside the track compared with the unpolluted region on either side of the track. Despite this, in cases with a smaller number of visually verified tracks, the anomalies inside the tracks are likely to be much larger than the impact of this background effect and, therefore, are unlikely to cause a change in the sign of the response. Additionally, this bias is found to have a regional distribution, as seen in Fig. 4. The stratocumulus regions tend to have a much weaker bias compared with the cumulus regions; therefore, this bias is likely to be much less significant in studies that focus on stratocumulus regions.
This study predicts ship track locations with no requirement for tracks to be visible and includes track locations that are a strong function of the wind field. This is also the case in Gryspeerdt et al. (2021) and Manshausen et al. (2022, 2023). In studies such as these, this bias becomes non-negligible due to the much weaker signal, the relative importance of weak tracks, and the significant correlation between cloud properties and the wind field in these locations. By correcting for this bias, we find that the LWP response is close to zero in a composite of all tracks in the Atlantic region. This is in much closer agreement with LWP responses to the 2014 Holuhraun effusive eruption (Malavelle et al., 2017) and studies based on visible ship tracks (Toll et al., 2019). Chen et al. (2024) see slight decreases in the LWP in a volcanic plume under various meteorological conditions in a trade cumulus regime, suggesting again that the LWP response to aerosol perturbations is weak, rather than extremely positive as suggested by Manshausen et al. (2022).
We do find a LWP response when considering a subset of tracks in the Namibian stratocumulus deck. This suggests that cloud regime is an important control on the LWP response. It appears that the stratocumulus decks are much more sensitive to aerosol loading than shallow cumulus. Possner et al. (2020) suggests that the differences in LWP adjustments between shallow cumulus and stratocumulus are due to the lateral entrainment effects predominant in shallow cumulus, compared with the strong control on vertical moisture gradients and stability in stratocumulus.
We find an increase in the LWP to aerosol in ship tracks that occur in clean, precipitating scenes, whereas we note negative LWP responses under polluted, non-precipitating conditions, in agreement with Ackerman et al. (2004), Gryspeerdt et al. (2019a), and Toll et al. (2019). These results are consistent with the precipitation suppression mechanism in cleaner, precipitating clouds, in which there is precipitation to suppress via the decrease in droplet size. This enhancement through the precipitation suppression mechanism is seen 5-6 h after the aerosol perturbation, which is consistent with Wang and Feingold (2009a). These results also support the idea that entrainment is enhanced more in polluted, non-precipitating clouds. The stability (EIS) is not found to have as strong a control on the Nd or LWP response, with stable environments experiencing a weakly negative LWP enhancement and unstable environments experiencing a weakly positive LWP enhancement, while Nd enhancements are greater for more stable environments.
The results of this study are aligned with the findings of high-resolution simulations of ship tracks. Wang and Feingold (2009b) simulate ship tracks in a high-resolution model with a double-moment bulk cloud microphysics scheme and see very small changes in the LWP under non-precipitating conditions. They do observe secondary circulation effects induced by the ship aerosol perturbation; however, the composite nature of satellite observations in this study and the use of predicted ship track locations means that we would be unlikely to observe this behaviour. Possner et al. (2015) also simulate ship tracks in a drizzling stratocumulus deck and find that the liquid water content was increased in some ship tracks. This is in line with our results in precipitating Namibian stratocumulus.
Using our corrected LWP and Nd responses, we extrapolate globally to calculate an estimate of the radiative forcing from LWP adjustments. We find a weak negative forcing of −0.16 (−0.29, −0.07) W m−2 globally. This is much weaker than previously reported negative forcing estimates from ship tracks (Manshausen et al., 2022) and suggests that the LWP response to aerosol perturbations is closer to that determined from other lines of evidence (Malavelle et al., 2017; Toll et al., 2019).
Glassmeier et al. (2021) find that LWP adjustments in ship track studies can overestimate the cooling effect of aerosol perturbations when generalized to the global scale. This study avoids the issues suggested by Glassmeier et al. (2021) by considering ship tracks that are 20 h long (on the order of the adjustment equilibrium timescale) and placing no requirement on tracks to be visible. However, the results of this paper do suggest an alternative way in which ship track studies can overestimate the LWP response to aerosol perturbations and, therefore, their potential cooling impact, which must be taken into account when using ship tracks to investigate aerosol–cloud interactions.
The implications of these results are significant for the field of geoengineering. Marine cloud brightening (MCB) is often proposed as a method to mitigate the effects of climate change, by increasing the albedo of marine stratocumulus clouds through the injection of sea salt aerosol (Latham et al., 2012; Diamond et al., 2022). The sign of the LWP response (and hence the warming or cooling that an aerosol perturbation could induce) is vitally important to know with certainty in order to assess the effectiveness of MCB. Previous ship track studies (Manshausen et al., 2022), which suggest aerosol-induced increases in the LWP in ship tracks in shallow cumulus regimes, must be re-evaluated when considering the feasibility of MCB (Diamond et al., 2022; Hansen et al., 2023), as they will suffer from the bias identified in this study. This study hopes to emphasize the importance of the regional dependence of the LWP response as well as the need for more studies in different cloud regimes in different meteorological contexts to fully understand the implications of MCB.
Although the magnitude and time dependence of these responses remain more uncertain, this study (1) demonstrates the importance of the background environment with respect to controlling the LWP response to aerosol perturbations and (2) emphasizes the importance of considering non-linearities in the background gradients when interpreting enhancements from a background state. Once we consider these background effects, we find that the LWP response is very weak in a composite of all ship tracks in the Atlantic ocean in 2018 and that the marine stratocumulus deck LWP is much more sensitive to aerosol loading than shallow cumulus clouds. This reconciles the results of previous work and provides a constraint on the radiative forcing due to LWP adjustments in clouds.
MODIS data used in this work were acquired from the Level-1 and Atmosphere Archive and Distribution System (LAADS) Distributed Active Archive Center (DAAC) (https://ladsweb.modaps.eosdis.nasa.gov, LAADS DAAC, 2021; Platnick et al., 2017). The ERA5 data are from the Copernicus Climate Change Service (C3S) Climate Data Store (CDS) (https://doi.org/10.24381/cds.adbb2d47, Copernicus Climate Change Service, 2023; Hersbach et al., 2020). Ship AIS data were obtained from exactEarth. exactEarth data are not publicly available; however, they can be accessed via a paid subscription: https://www.marinetraffic.org/exactearth (last access: 29 November 2024).
The supplement related to this article is available online at: https://doi.org/10.5194/acp-24-13269-2024-supplement.
AT and EG designed the study. AT performed the analysis, and PM contributed Fig. 4b. PM, EG, and PS assisted with the interpretation of the results. TWPS provided the ship location data. AT drafted the manuscript, and PM, PS, and EG provided comments and suggestions.
At least one of the (co-)authors is a member of the editorial board of Atmospheric Chemistry and Physics. The peer-review process was guided by an independent editor, and the authors also have no other competing interests to declare.
Publisher's note: Copernicus Publications remains neutral with regard to jurisdictional claims made in the text, published maps, institutional affiliations, or any other geographical representation in this paper. While Copernicus Publications makes every effort to include appropriate place names, the final responsibility lies with the authors.
Anna Tippett and Edward Gryspeerdt acknowledge funding from the Horizon Europe programme (project CERTAINTY – Cloud–aERosol inTeractions & their impActs IN The earth sYstem; grant agreement no. 101137680) and a Royal Society University Research Fellowship (grant no. URF/R1/191602).
Peter Manshausen acknowledges funding from the European Union's Horizon 2020 Research and Innovation programme (under a Marie Skłodowska-Curie iMIRACLI grant; grant agreement no. 860100) and from the German Academic Scholarship Foundation (Studienstiftung des deutschen Volkes).
Philip Stier acknowledges support from the ACRUISE UK Natural Environment Research Council project (grant no. NE/S005099/1), the European Union's Horizon 2020 FORCeS project (grant agreement no. 821205), and the European Union's Horizon Europe CleanCloud project (grant agreement no. 101137639) and its UKRI underwrite.
This research has been supported by Horizon Europe Climate, Energy and Mobility (grant nos. 101137680 and 101137639), the Royal Society (grant no. URF/R1/191602), UK Research and Innovation (grant no. NE/S005099/1), the Horizon Europe Marie Skłodowska-Curie Actions (grant no. 860100), and the European Union's Horizon 2020 FORCeS project (grant agreement no. 821205).
This paper was edited by Matthias Tesche and reviewed by two anonymous referees.
Ackerman, A. S., Kirkpatrick, M. P., Stevens, D. E., and Toon, O. B.: The impact of humidity above stratiform clouds on indirect aerosol climate forcing, Nature, 432, 1014–1017, https://doi.org/10.1038/nature03174, 2004. a, b, c, d
Albrecht, B. A.: Aerosols, Cloud Microphysics, and Fractional Cloudiness, Science, 245, 1227–1230, https://doi.org/10.1126/science.245.4923.1227, 1989. a, b
Bellouin, N., Quaas, J., Gryspeerdt, E., Kinne, S., Stier, P., Watson-Parris, D., Boucher, O., Carslaw, K. S., Christensen, M., Daniau, A.-L., Dufresne, J.-L., Feingold, G., Fiedler, S., Forster, P., Gettelman, A., Haywood, J. M., Lohmann, U., Malavelle, F., Mauritsen, T., McCoy, D. T., Myhre, G., Mülmenstädt, J., Neubauer, D., Possner, A., Rugenstein, M., Sato, Y., Schulz, M., Schwartz, S. E., Sourdeval, O., Storelvmo, T., Toll, V., Winker, D., and Stevens, B.: Bounding Global Aerosol Radiative Forcing of Climate Change, Rev. Geophys., 58, e2019RG000660, https://doi.org/10.1029/2019RG000660, 2020. a, b, c
Bretherton, C. S., Blossey, P. N., and Uchida, J.: Cloud droplet sedimentation, entrainment efficiency, and subtropical stratocumulus albedo, Geophys. Res. Lett., 34, L03813, https://doi.org/10.1029/2006GL027648, 2007. a
Briggs, G. A.: A Plume Rise Model Compared with Observations, JAPCA J. Air Waste Ma., 15, 433–438, https://doi.org/10.1080/00022470.1965.10468404, 1965. a
Chen, Y., Haywood, J., Wang, Y., Malavelle, F., Jordan, G., Peace, A., Partridge, D. G., Cho, N., Oreopoulos, L., Grosvenor, D., Field, P., Allan, R. P., and Lohmann, U.: Substantial cooling effect from aerosol-induced increase in tropical marine cloud cover, Nat. Geosci., 17, 404–410, https://doi.org/10.1038/s41561-024-01427-z, 2024. a
Chen, Y.-C., Christensen, M. W., Xue, L., Sorooshian, A., Stephens, G. L., Rasmussen, R. M., and Seinfeld, J. H.: Occurrence of lower cloud albedo in ship tracks, Atmos. Chem. Phys., 12, 8223–8235, https://doi.org/10.5194/acp-12-8223-2012, 2012. a
Chen, Y.-C., Christensen, M. W., Stephens, G. L., and Seinfeld, J. H.: Satellite-based estimate of global aerosol–cloud radiative forcing by marine warm clouds, Nat. Geosci., 7, 643–646, https://doi.org/10.1038/ngeo2214, 2014. a, b
Christensen, M. W. and Stephens, G. L.: Microphysical and macrophysical responses of marine stratocumulus polluted by underlying ships: Evidence of cloud deepening, J. Geophys. Res.-Atmos., 116, D03201, https://doi.org/10.1029/2010JD014638, 2011. a, b
Christensen, M. W. and Stephens, G. L.: Microphysical and macrophysical responses of marine stratocumulus polluted by underlying ships: 2. Impacts of haze on precipitating clouds, J. Geophys. Res.-Atmos., 117, D11203, https://doi.org/10.1029/2011JD017125, 2012. a
Christensen, M. W., Coakley, J. A., and Tahnk, W. R.: Morning-to-Afternoon Evolution of Marine Stratus Polluted by Underlying Ships: Implications for the Relative Lifetimes of Polluted and Unpolluted Clouds, J. Atmos. Sci., 66, 2097–2106, https://doi.org/10.1175/2009JAS2951.1, 2009. a
Christensen, M. W., Gettelman, A., Cermak, J., Dagan, G., Diamond, M., Douglas, A., Feingold, G., Glassmeier, F., Goren, T., Grosvenor, D. P., Gryspeerdt, E., Kahn, R., Li, Z., Ma, P.-L., Malavelle, F., McCoy, I. L., McCoy, D. T., McFarquhar, G., Mülmenstädt, J., Pal, S., Possner, A., Povey, A., Quaas, J., Rosenfeld, D., Schmidt, A., Schrödner, R., Sorooshian, A., Stier, P., Toll, V., Watson-Parris, D., Wood, R., Yang, M., and Yuan, T.: Opportunistic experiments to constrain aerosol effective radiative forcing, Atmos. Chem. Phys., 22, 641–674, https://doi.org/10.5194/acp-22-641-2022, 2022. a, b
Conover, J. H.: Anomalous Cloud Lines, J. Atmos. Sci., 23, 778–785, https://doi.org/10.1175/1520-0469(1966)023<0778:ACL>2.0.CO;2, 1966. a
Copernicus Climate Change Service, Climate Data Store: ERA5 hourly data on single levels from 1940 to present, Copernicus Climate Change Service (C3S) Climate Data Store (CDS) [data set], https://doi.org/10.24381/cds.adbb2d47, 2023. a
Diamond, M. S., Gettelman, A., Lebsock, M. D., McComiskey, A., Russell, L. M., Wood, R., and Feingold, G.: To assess marine cloud brightening's technical feasibility, we need to know what to study – and when to stop, P. Natl. Acad. Sci. USA, 119, e2118379119, https://doi.org/10.1073/pnas.2118379119, 2022. a, b
Durkee, P. A., Chartier, R. E., Brown, A., Trehubenko, E. J., Rogerson, S. D., Skupniewicz, C., Nielsen, K. E., Platnick, S., and King, M. D.: Composite Ship Track Characteristics, J. Atmos. Sci., 57, 2542–2553, https://doi.org/10.1175/1520-0469(2000)057<2542:CSTC>2.0.CO;2, 2000. a
Efron, B.: Bootstrap Methods: Another Look at the Jackknife, Ann. Stat., 7, 1–26, https://doi.org/10.1214/aos/1176344552, 1979. a
Feingold, G., Ghate, V. P., Russell, L. M., Blossey, P., Cantrell, W., Christensen, M. W., Diamond, M. S., Gettelman, A., Glassmeier, F., Gryspeerdt, E., Haywood, J., Hoffmann, F., Kaul, C. M., Lebsock, M., McComiskey, A. C., McCoy, D. T., Ming, Y., Mülmenstädt, J., Possner, A., Prabhakaran, P., Quinn, P. K., Schmidt, K. S., Shaw, R. A., Singer, C. E., Sorooshian, A., Toll, V., Wan, J. S., Wood, R., Yang, F., Zhang, J., and Zheng, X.: Physical science research needed to evaluate the viability and risks of marine cloud brightening, Sci. Adv., 10, eadi8594, https://doi.org/10.1126/sciadv.adi8594, 2024. a
Fons, E., Runge, J., Neubauer, D., and Lohmann, U.: Stratocumulus adjustments to aerosol perturbations disentangled with a causal approach, npj Climate and Atmospheric Science, 6, 1–10, https://doi.org/10.1038/s41612-023-00452-w, 2023. a
Forster, P., Storelvmo, T., Armour, K., Collins, W., Dufresne, J.-L., Frame, D., Lunt, D., Mauritsen, T., Palmer, M., Watanabe, M., Wild, M., and Zhang, H.: The Earth's Energy Budget, Climate Feedbacks, and Climate Sensitivity, Climate Change 2021 – The Physical Science Basis: Working Group I Contribution to the Sixth Assessment Report of the Intergovernmental Panel on Climate Change, Cambridge University Press, https://doi.org/10.1017/9781009157896.009, 2021. a, b
Forster, P. M., Forster, H. I., Evans, M. J., Gidden, M. J., Jones, C. D., Keller, C. A., Lamboll, R. D., Quéré, C. L., Rogelj, J., Rosen, D., Schleussner, C.-F., Richardson, T. B., Smith, C. J., and Turnock, S. T.: Current and future global climate impacts resulting from COVID-19, Nat. Clim. Change, 10, 913–919, https://doi.org/10.1038/s41558-020-0883-0, 2020. a
Glassmeier, F., Hoffmann, F., Johnson, J. S., Yamaguchi, T., Carslaw, K. S., and Feingold, G.: Aerosol-cloud-climate cooling overestimated by ship-track data, Science, 371, 485–489, https://doi.org/10.1126/science.abd3980, 2021. a, b, c, d, e, f
Grosvenor, D. P. and Wood, R.: The effect of solar zenith angle on MODIS cloud optical and microphysical retrievals within marine liquid water clouds, Atmos. Chem. Phys., 14, 7291–7321, https://doi.org/10.5194/acp-14-7291-2014, 2014. a
Grosvenor, D. P., Field, P. R., Hill, A. A., and Shipway, B. J.: The relative importance of macrophysical and cloud albedo changes for aerosol-induced radiative effects in closed-cell stratocumulus: insight from the modelling of a case study, Atmos. Chem. Phys., 17, 5155–5183, https://doi.org/10.5194/acp-17-5155-2017, 2017. a
Grosvenor, D. P., Sourdeval, O., Zuidema, P., Ackerman, A., Alexandrov, M. D., Bennartz, R., Boers, R., Cairns, B., Chiu, J. C., Christensen, M., Deneke, H., Diamond, M., Feingold, G., Fridlind, A., Hünerbein, A., Knist, C., Kollias, P., Marshak, A., McCoy, D., Merk, D., Painemal, D., Rausch, J., Rosenfeld, D., Russchenberg, H., Seifert, P., Sinclair, K., Stier, P., van Diedenhoven, B., Wendisch, M., Werner, F., Wood, R., Zhang, Z., and Quaas, J.: Remote Sensing of Droplet Number Concentration in Warm Clouds: A Review of the Current State of Knowledge and Perspectives, Rev. Geophys., 56, 409–453, https://doi.org/10.1029/2017RG000593, 2018. a
Gryspeerdt, E., Goren, T., Sourdeval, O., Quaas, J., Mülmenstädt, J., Dipu, S., Unglaub, C., Gettelman, A., and Christensen, M.: Constraining the aerosol influence on cloud liquid water path, Atmos. Chem. Phys., 19, 5331–5347, https://doi.org/10.5194/acp-19-5331-2019, 2019a. a, b, c, d
Gryspeerdt, E., Smith, T. W. P., O'Keeffe, E., Christensen, M. W., and Goldsworth, F. W.: The Impact of Ship Emission Controls Recorded by Cloud Properties, Geophys. Res. Lett., 46, 12547–12555, https://doi.org/10.1029/2019GL084700, 2019b. a
Gryspeerdt, E., Mülmenstädt, J., Gettelman, A., Malavelle, F. F., Morrison, H., Neubauer, D., Partridge, D. G., Stier, P., Takemura, T., Wang, H., Wang, M., and Zhang, K.: Surprising similarities in model and observational aerosol radiative forcing estimates, Atmos. Chem. Phys., 20, 613–623, https://doi.org/10.5194/acp-20-613-2020, 2020. a
Gryspeerdt, E., Goren, T., and Smith, T. W. P.: Observing the timescales of aerosol–cloud interactions in snapshot satellite images, Atmos. Chem. Phys., 21, 6093–6109, https://doi.org/10.5194/acp-21-6093-2021, 2021. a, b, c, d, e, f, g, h, i, j, k, l
Hansen, J. E., Sato, M., Simons, L., Nazarenko, L. S., Sangha, I., Kharecha, P., Zachos, J. C., von Schuckmann, K., Loeb, N. G., Osman, M. B., Jin, Q., Tselioudis, G., Jeong, E., Lacis, A., Ruedy, R., Russell, G., Cao, J., and Li, J.: Global warming in the pipeline, Oxford Open Climate Change, 3, kgad008, https://doi.org/10.1093/oxfclm/kgad008, 2023. a
Hersbach, H., Bell, B., Berrisford, P., Hirahara, S., Horányi, A., Muñoz-Sabater, J., Nicolas, J., Peubey, C., Radu, R., Schepers, D., Simmons, A., Soci, C., Abdalla, S., Abellan, X., Balsamo, G., Bechtold, P., Biavati, G., Bidlot, J., Bonavita, M., De Chiara, G., Dahlgren, P., Dee, D., Diamantakis, M., Dragani, R., Flemming, J., Forbes, R., Fuentes, M., Geer, A., Haimberger, L., Healy, S., Hogan, R. J., Hólm, E., Janisková, M., Keeley, S., Laloyaux, P., Lopez, P., Lupu, C., Radnoti, G., de Rosnay, P., Rozum, I., Vamborg, F., Villaume, S., and Thépaut, J.-N.: The ERA5 global reanalysis, Q. J. Royal Meteor. Soc., 146, 1999–2049, https://doi.org/10.1002/qj.3803, 2020. a, b, c, d, e
Kabatas, B., Menzel, W. P., Bilgili, A., and Gumley, L. E.: Comparing Ship-Track Droplet Sizes Inferred from Terra and Aqua MODIS Data, J. Appl. Meteorol. Clim., 52, 230–241, https://doi.org/10.1175/JAMC-D-11-0232.1, 2013. a
Koren, I., Dagan, G., and Altaratz, O.: From aerosol-limited to invigoration of warm convective clouds, Science, 344, 1143–1146, https://doi.org/10.1126/science.1252595, 2014. a
Latham, J., Bower, K., Choularton, T., Coe, H., Connolly, P., Cooper, G., Craft, T., Foster, J., Gadian, A., Galbraith, L., Iacovides, H., Johnston, D., Launder, B., Leslie, B., Meyer, J., Neukermans, A., Ormond, B., Parkes, B., Rasch, P., Rush, J., Salter, S., Stevenson, T., Wang, H., Wang, Q., and Wood, R.: Marine cloud brightening, Philos. T. Roy. Soc. A, 370, 4217–4262, https://doi.org/10.1098/rsta.2012.0086, 2012. a
Lebo, Z. J. and Feingold, G.: On the relationship between responses in cloud water and precipitation to changes in aerosol, Atmos. Chem. Phys., 14, 11817–11831, https://doi.org/10.5194/acp-14-11817-2014, 2014. a
Level-1 and Atmosphere Archive and Distribution System (LAADS) Distributed Active Archive Center (DAAC): MODIS data, Goddard Space Flight Center in Greenbelt, Maryland, USA, https://ladsweb.modaps.eosdis.nasa.gov, last access: 28 November 2021. a
Malavelle, F. F., Haywood, J. M., Jones, A., Gettelman, A., Clarisse, L., Bauduin, S., Allan, R. P., Karset, I. H. H., Kristjánsson, J. E., Oreopoulos, L., Cho, N., Lee, D., Bellouin, N., Boucher, O., Grosvenor, D. P., Carslaw, K. S., Dhomse, S., Mann, G. W., Schmidt, A., Coe, H., Hartley, M. E., Dalvi, M., Hill, A. A., Johnson, B. T., Johnson, C. E., Knight, J. R., O'Connor, F. M., Partridge, D. G., Stier, P., Myhre, G., Platnick, S., Stephens, G. L., Takahashi, H., and Thordarson, T.: Strong constraints on aerosol–cloud interactions from volcanic eruptions, Nature, 546, 485–491, https://doi.org/10.1038/nature22974, 2017. a, b, c, d
Manshausen, P., Watson-Parris, D., Christensen, M. W., Jalkanen, J.-P., and Stier, P.: Invisible ship tracks show large cloud sensitivity to aerosol, Nature, 610, 7930, https://doi.org/10.1038/s41586-022-05122-0, 2022. a, b, c, d, e, f, g, h, i, j, k, l, m, n, o, p, q, r, s, t, u, v, w, x, y, z
Manshausen, P., Watson-Parris, D., Christensen, M. W., Jalkanen, J.-P., and Stier, P.: Rapid saturation of cloud water adjustments to shipping emissions, Atmos. Chem. Phys., 23, 12545–12555, https://doi.org/10.5194/acp-23-12545-2023, 2023. a, b, c, d, e, f, g, h, i, j, k, l
McCoy, D. T., Field, P. R., Schmidt, A., Grosvenor, D. P., Bender, F. A.-M., Shipway, B. J., Hill, A. A., Wilkinson, J. M., and Elsaesser, G. S.: Aerosol midlatitude cyclone indirect effects in observations and high-resolution simulations, Atmos. Chem. Phys., 18, 5821–5846, https://doi.org/10.5194/acp-18-5821-2018, 2018. a
Michibata, T., Suzuki, K., Sato, Y., and Takemura, T.: The source of discrepancies in aerosol–cloud–precipitation interactions between GCM and A-Train retrievals, Atmos. Chem. Phys., 16, 15413–15424, https://doi.org/10.5194/acp-16-15413-2016, 2016. a, b
Neubauer, D., Christensen, M. W., Poulsen, C. A., and Lohmann, U.: Unveiling aerosol–cloud interactions – Part 2: Minimising the effects of aerosol swelling and wet scavenging in ECHAM6-HAM2 for comparison to satellite data, Atmos. Chem. Phys., 17, 13165–13185, https://doi.org/10.5194/acp-17-13165-2017, 2017. a
Platnick, S., Meyer, K. G., King, M. D., Wind, G., Amarasinghe, N., Marchant, B., Arnold, G. T., Zhang, Z., Hubanks, P. A., Holz, R. E., Yang, P., Ridgway, W. L., and Riedi, J.: The MODIS Cloud Optical and Microphysical Products: Collection 6 Updates and Examples From Terra and Aqua, IEEE T. Geosci. Remote, 55, 502–525, https://doi.org/10.1109/TGRS.2016.2610522, 2017. a, b, c
Possner, A., Zubler, E., Lohmann, U., and Schär, C.: Real-case simulations of aerosol–cloud interactions in ship tracks over the Bay of Biscay, Atmos. Chem. Phys., 15, 2185–2201, https://doi.org/10.5194/acp-15-2185-2015, 2015. a
Possner, A., Eastman, R., Bender, F., and Glassmeier, F.: Deconvolution of boundary layer depth and aerosol constraints on cloud water path in subtropical stratocumulus decks, Atmos. Chem. Phys., 20, 3609–3621, https://doi.org/10.5194/acp-20-3609-2020, 2020. a, b, c, d, e
Quaas, J., Boucher, O., and Lohmann, U.: Constraining the total aerosol indirect effect in the LMDZ and ECHAM4 GCMs using MODIS satellite data, Atmos. Chem. Phys., 6, 947–955, https://doi.org/10.5194/acp-6-947-2006, 2006. a
Quaas, J., Ming, Y., Menon, S., Takemura, T., Wang, M., Penner, J. E., Gettelman, A., Lohmann, U., Bellouin, N., Boucher, O., Sayer, A. M., Thomas, G. E., McComiskey, A., Feingold, G., Hoose, C., Kristjánsson, J. E., Liu, X., Balkanski, Y., Donner, L. J., Ginoux, P. A., Stier, P., Grandey, B., Feichter, J., Sednev, I., Bauer, S. E., Koch, D., Grainger, R. G., Kirkevåg, A., Iversen, T., Seland, Ø., Easter, R., Ghan, S. J., Rasch, P. J., Morrison, H., Lamarque, J.-F., Iacono, M. J., Kinne, S., and Schulz, M.: Aerosol indirect effects – general circulation model intercomparison and evaluation with satellite data, Atmos. Chem. Phys., 9, 8697–8717, https://doi.org/10.5194/acp-9-8697-2009, 2009. a, b
Rosenfeld, D.: Suppression of Rain and Snow by Urban and Industrial Air Pollution, Science, 287, 1793–1796, https://doi.org/10.1126/science.287.5459.1793, 2000. a
Rosenfeld, D., Zhu, Y., Wang, M., Zheng, Y., Goren, T., and Yu, S.: Aerosol-driven droplet concentrations dominate coverage and water of oceanic low-level clouds, Science, 363, eaav0566, https://doi.org/10.1126/science.aav0566, 2019. a
Sato, Y., Goto, D., Michibata, T., Suzuki, K., Takemura, T., Tomita, H., and Nakajima, T.: Aerosol effects on cloud water amounts were successfully simulated by a global cloud-system resolving model, Nat. Commun., 9, 985, https://doi.org/10.1038/s41467-018-03379-6, 2018. a, b
Sayer, A. M., Hsu, N. C., and Bettenhausen, C.: Implications of MODIS bow-tie distortion on aerosol optical depth retrievals, and techniques for mitigation, Atmos. Meas. Tech., 8, 5277–5288, https://doi.org/10.5194/amt-8-5277-2015, 2015. a
Segrin, M. S., Coakley, J. A., and Tahnk, W. R.: MODIS Observations of Ship Tracks in Summertime Stratus off the West Coast of the United States, J. Atmos. Sci., 64, 4330–4345, https://doi.org/10.1175/2007JAS2308.1, 2007. a, b
Small, J. D., Chuang, P. Y., Feingold, G., and Jiang, H.: Can aerosol decrease cloud lifetime?, Geophys. Res. Lett., 36, 2009GL038888, https://doi.org/10.1029/2009GL038888, 2009. a
Smith, T., Jalkanen, J., Anderson, B., Corbett, J., Faber, J., Hanayama, S., O'Keeffe, E., Parker, S., Johansson, L., Aldous, L., Raucci, C., Traut, M., Ettinger, S., Nelissen, D., Lee, D., Ng, S., Agrawal, A., Winebrake, J., Hoen, M., Chesworth, S., and Pandey, A.: Third IMO Greenhouse Gas Study 2014, International Maritime Organization, United Kingdom, MEPC 67/INF.3, 2015. a, b
Stein, A. F., Draxler, R. R., Rolph, G. D., Stunder, B. J. B., Cohen, M. D., and Ngan, F.: NOAA's HYSPLIT Atmospheric Transport and Dispersion Modeling System, B. Am. Meteorol. Soc., 96, 2059–2077, https://doi.org/10.1175/BAMS-D-14-00110.1, 2015. a
Toll, V., Christensen, M., Gassó, S., and Bellouin, N.: Volcano and Ship Tracks Indicate Excessive Aerosol‐Induced Cloud Water Increases in a Climate Model, Geophys. Res. Lett., 44, 12492–12500, https://doi.org/10.1002/2017GL075280, 2017. a, b, c, d, e
Toll, V., Christensen, M., Quaas, J., and Bellouin, N.: Weak average liquid-cloud-water response to anthropogenic aerosols, Nature, 572, 51–55, https://doi.org/10.1038/s41586-019-1423-9, 2019. a, b, c, d, e, f
Twomey, S.: Pollution and the planetary albedo, Atmos. Environ., 8, 1251–1256, https://doi.org/10.1016/0004-6981(74)90004-3, 1974. a
Twomey, S.: The Influence of Pollution on the Shortwave Albedo of Clouds, J. Atmos. Sci., 34, 1149–1152, https://doi.org/10.1175/1520-0469(1977)034<1149:TIOPOT>2.0.CO;2, 1977. a
Wall, C. J., Norris, J. R., Possner, A., McCoy, D. T., McCoy, I. L., and Lutsko, N. J.: Assessing effective radiative forcing from aerosol–cloud interactions over the global ocean, P. Natl. Acad. Sci. USA, 119, e2210481119, https://doi.org/10.1073/pnas.2210481119, 2022. a
Wang, H. and Feingold, G.: Modeling Mesoscale Cellular Structures and Drizzle in Marine Stratocumulus. Part I: Impact of Drizzle on the Formation and Evolution of Open Cells, J. Atmos. Sci., 66, 3237–3256, https://doi.org/10.1175/2009JAS3022.1, 2009a. a, b
Wang, H. and Feingold, G.: Modeling Mesoscale Cellular Structures and Drizzle in Marine Stratocumulus. Part II: The Microphysics and Dynamics of the Boundary Region between Open and Closed Cells, J. Atmos. Sci., 66, 3257–3275, https://doi.org/10.1175/2009JAS3120.1, 2009b. a
Watson-Parris, D., Christensen, M. W., Laurenson, A., Clewley, D., Gryspeerdt, E., and Stier, P.: Shipping regulations lead to large reduction in cloud perturbations, P. Natl. Acad. Sci. USA, 119, https://doi.org/10.1073/pnas.2206885119, 2022. a
Wood, R. and Bretherton, C. S.: On the Relationship between Stratiform Low Cloud Cover and Lower-Tropospheric Stability, J. Climate, 19, 6425–6432, https://doi.org/10.1175/JCLI3988.1, 2006. a
Yuan, T., Song, H., Wood, R., Wang, C., Oreopoulos, L., Platnick, S. E., von Hippel, S., Meyer, K., Light, S., and Wilcox, E.: Global reduction in ship-tracks from sulfur regulations for shipping fuel, Science Advances, 8, eabn7988, https://doi.org/10.1126/sciadv.abn7988, 2022. a
Zhang, J., Zhou, X., Goren, T., and Feingold, G.: Albedo susceptibility of northeastern Pacific stratocumulus: the role of covarying meteorological conditions, Atmos. Chem. Phys., 22, 861–880, https://doi.org/10.5194/acp-22-861-2022, 2022. a
Zipfel, L., Andersen, H., and Cermak, J.: Machine-Learning Based Analysis of Liquid Water Path Adjustments to Aerosol Perturbations in Marine Boundary Layer Clouds Using Satellite Observations, Atmosphere, 13, 586, https://doi.org/10.3390/atmos13040586, 2022. a