the Creative Commons Attribution 4.0 License.
the Creative Commons Attribution 4.0 License.
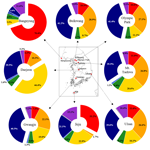
An investigation into atmospheric nitrous acid (HONO) processes in South Korea
Kiyeon Kim
Chul Han Song
Hyojun Lee
Ross Beardsley
Jinhyeok Yu
Greg Yarwood
Bonyoung Koo
Jasper Madalipay
Jung-Hun Woo
Seogju Cho
Nitrous acid (HONO) is a main precursor of hydroxyl radicals (OH), which contribute to the formation of numerous secondary air pollutants in the troposphere. Despite its importance in atmospheric chemistry, HONO chemistry has not been fully incorporated into many chemical transport models (CTMs). Due to the lack of atmospheric HONO processes, CTM simulations often tend to underestimate atmospheric mixing ratios of HONO. This study was undertaken because simulations with the current Community Multiscale Air Quality (CMAQ) model have a strong tendency to underestimate the HONO mixing ratio. In search of missing sources of atmospheric HONO, we attempted to sequentially incorporate the following potential HONO sources and processes into the CMAQ modeling framework: (i) gas-phase HONO reactions, (ii) traffic HONO emissions, (iii) soil HONO emissions, (iv) heterogeneous HONO production on the surfaces of aerosols, (v) heterogeneous HONO formation on tree leaf and building surfaces, and (vi) photolysis reactions of particulates and deposited HNO3/nitrates called “renoxification”. The simulation performances of the modified CMAQ models were then evaluated by comparing the modeled HONO mixing ratios with the HONO mixing ratios observed at the Olympic Park station in Seoul, South Korea. When HONO processes were fully added to the CMAQ model, average daily HONO mixing ratios increased from 0.06 to 1.18 ppb. The daytime HONO mixing ratios produced from the CMAQ model run with a full account of atmospheric HONO processes were found to be in better agreement with observations than those from the original CMAQ model (CMAQv5.2.1) runs with improved statistical metrics (e.g., the index of agreement (IOA) increased from 0.59 to 0.68, while the mean bias (MB) decreased dramatically from −0.57 to −0.34 ppb). In addition, we investigated the contributions of individual atmospheric HONO processes to HONO mixing ratios, as well as the impacts of HONO atmospheric processes on the concentrations of other atmospheric species in South Korea. All these issues are also discussed in this paper.
- Article
(3145 KB) - Full-text XML
-
Supplement
(1542 KB) - BibTeX
- EndNote
Hydroxyl radicals (OH) play a key role in atmospheric chemistry. OH radicals oxidize volatile organic compounds (VOCs), sulfur dioxide (SO2), and nitrogen dioxide (NO2), contributing to the formation of secondary organic and inorganic aerosols (Pathak et al., 2009). Therefore, accurate determination of the mixing ratio of OH radicals is crucial to understanding atmospheric photochemistry in both polluted and remote areas.
Nitrous acid (HNO2 or HONO) has been recognized as a main precursor of OH radicals via photo-dissociation (Reaction R1) (Harris et al., 1982; Alicke et al., 2003; Kleffmann et al., 2005):
Several studies have estimated that HONO photolysis reactions contribute 20 %–80 % of OH radicals and 30 %–87 % of HOx formation in polluted urban areas (Alicke et al., 2003; Ren et al., 2003; Kleffmann et al., 2005; Acker et al., 2006; Monks et al., 2009; Hendrick et al., 2014; Kim et al., 2014). However, it was also recognized that the HONO chemistry has not yet been fully understood.
Therefore, many field measurements have been carried out to characterize atmospheric HONO processes (Su et al., 2008; Li et al., 2012; Kim et al., 2014; Lee et al., 2016). These studies showed that the observed HONO mixing ratios were significantly higher than those predicted by atmospheric chemical transport model (CTM) simulations (Su et al., 2008; VandenBoer et al., 2013; Li et al., 2014; Lee et al., 2016). This indicates that there should be missing HONO sources or processes that are not considered in current atmospheric models (CTMs).
Recent studies have proposed incorporating several HONO production pathways into chemical transport models to explain the missing HONO processes. Suggested sources include (i) traffic HONO emissions (Kirchstetter et al., 1996; Kurtenbach et al., 2001; Rappenglück et al., 2013; Czader et al., 2015; Xu et al., 2015; Nakashima and Kajii, 2017; Li et al., 2018); (ii) soil HONO emissions (Nagai and Kubota, 1972; Oswald et al., 2013; Weber et al., 2015; Meusel et al., 2016); (iii) HONO emissions from biomass burning (Crutzen and Andreae, 1990; Cheng et al., 2014; Nie et al., 2015); (iv) indoor HONO emissions (Gligorovski, 2016; Zhang et al., 2019); and (v) heterogeneous conversion of NO2 to HONO on the surfaces of aerosols, grounds, and leaves (Svensson et al., 1987; Wiesen et al., 1995; Reisinger, 2000; Han et al., 2017).
Among these processes, traffic HONO emissions were reported to be the key factor influencing the HONO mixing ratio in the Beijing–Tianjin–Hebei (BTH) region at night (Zhang et al., 2019). Heterogeneous NO2 reactions on aerosol surfaces were an important source of HONO during severe haze periods in Beijing (Jia et al., 2020). On the other hand, Zhang et al. (2016) reported that heterogeneous reactions on ground surfaces could be the dominant source of atmospheric HONO, accounting for ∼42 % of the HONO mixing ratios in Hong Kong SAR suburban areas.
These findings may indicate that atmospheric HONO production and a potential cause of discrepancies between modeled and observed HONO mixing ratios may vary temporally and regionally. In addition, no research has been conducted on which sources of HONO control the levels of HONO in Seoul, South Korea. In this context, the aims of this study are threefold: (i) to determine which HONO sources or processes are significant in South Korea, (ii) to estimate the budget of the HONO mixing ratios from various HONO sources, and (iii) achieving objectives (i) and (ii) to develop a near-perfect CTM in terms of HONO mixing ratio. To accomplish these goals, we decided to improve the US EPA Community Multiscale Air Quality (CMAQ) v5.2.1 model by incorporating several HONO production pathways, including (i) homogeneous HONO reactions; (ii) direct HONO emissions from biomass burning, traffic/vehicles, and soil; (iii) heterogeneous HONO production on the surfaces of atmospheric aerosols, buildings, and tree leaves; and (iv) photolysis reactions of particulate and deposited HNO3/nitrate (renoxification).
We then tested the performances of the modified CMAQ models by comparing the modeled HONO mixing ratios with the HONO mixing ratios observed during the Korea–United States Air Quality (KORUS-AQ) campaign. After the comparison analysis, we evaluated the contributions of individual HONO processes to the HONO budget in South Korea and also investigated the effects of the HONO mixing ratios on the levels of other important atmospheric species.
In this study, we incorporated various HONO sources and reactions into the CMAQ model framework to accurately estimate HONO mixing ratios in the atmosphere. Then, the simulation results of the modified CMAQ models were analyzed, comparing the modeled outputs with observations during the KORUS-AQ campaign. Details of the modifications of CMAQ models, the HONO measurements, and potentially important HONO sources considered in this study are described in the following sections.
2.1 WRF-CMAQ model configuration
Simulation of the Community Multiscale Air Quality (CMAQ) v5.2.1 model (Byun and Schere, 2006) was carried out to estimate the HONO mixing ratios during the period of the KORUS-AQ campaign (9 May–12 June 2016). Figure 1 shows the horizontal domain (A1) for the CMAQ model simulation. The spatial domain has 273×204 grid cells with a horizontal resolution of 15 km×15 km and contains 15 vertical layers, with the first layer at ∼34 m above the ground.
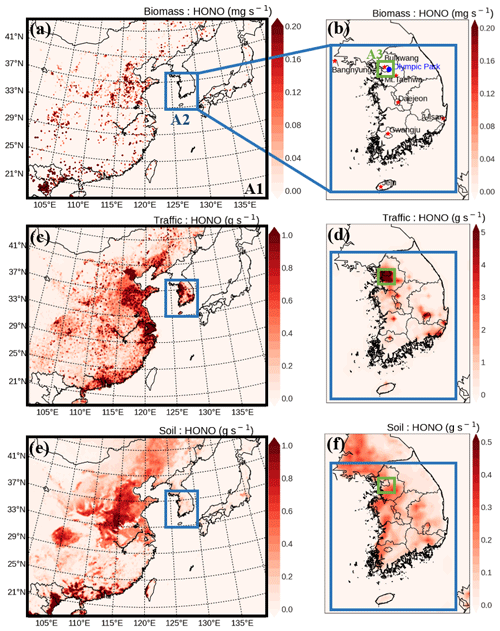
Figure 1Spatial distributions of HONO emission rates from biomass burning (a, b), from traffic (c, d), and from soil (e, f) over East Asia (A1), South Korea (A2), and the Seoul Metropolitan Area (A3). Several super-monitoring stations are located at Bangnyung-Do, Bulkwang-Dong, Olympic Park, Mt. Taehwa, Daejeon, Gwangju, Ulsan, and Jeju. The locations of these super-stations are shown in panel (b).
The photochemical mechanism used in the simulation of the CMAQ model was the Statewide Air Pollution Research Center-07 (SAPRC-07 TC) (Carter, 2010; Hutzell et al., 2012). The AERO6 module was used for aerosol calculations (Binkowski and Roselle, 2003). In particular, the heterogeneous reactions considered in this study were embedded into the SAPRC-07 TC via the Korean Flexible Chemistry (KFC) editor. The KFC editor is a chemical mechanism editor in a framework of a graphical user interface (GUI) developed to quickly implement the modifications of the chemical mechanisms of the CMAQ model. The details of the heterogeneous reactions we considered are discussed in Sect. 2.3.5 and 2.3.6.
The Weather Research and Forecasting (WRF) v3.8.1 model (Skamarock et al., 2008) was run to generate meteorological fields that drive the CMAQ model. The physical options used in the WRF run are as follows: (i) WRF Single-Moment 6-class Microphysics scheme (Hong and Lim, 2006), (ii) Rapid Radiative Transfer Model for GCMs (RRTMG) for longwave and shortwave radiation (Iacono et al., 2008), (iii) the Noah land surface scheme (Chen and Dudhia, 2001), (iv) Yonsei University (YSU) planetary boundary layer (PBL) scheme (Hong et al., 2006), (v) the MM5 surface layer scheme (Jiménez et al., 2012), and (vi) the Grell–Freitas ensemble scheme for cumulus physics (Grell and Freitas, 2014). Initial and boundary conditions for the WRF model runs were obtained from the National Center for Environmental Prediction Final Analysis (NCEP-FNL) every 6 h.
For anthropogenic emissions, this study used the KORUS v5.0 inventory processed by the Sparse Matrix Operator Kernel Emissions in Asia (SMOKE-Asia; Woo et al., 2012) (Woo et al., 2020). The KORUS v5.0 emissions were developed particularly for CTM runs as part of the KORUS-AQ campaign. Biogenic emissions were generated using the Model of Emissions of Gases and Aerosol from Nature (MEGAN) v2.10 (Guenther et al., 2012). Fire emissions were obtained from the Fire Inventory from NCAR (FINN) v1.5 emission inventory (https://www2.acom.ucar.edu/modeling/finn-fire-inventory-ncar, last access: 28 October 2024; Wiedinmyer et al., 2011). The various HONO emissions considered in this study are discussed in Sect. 2.3.2–2.3.4.
2.2 Measurements
During the KORUS-AQ campaign period, concentrations of NO2, O3, and particulate matter were measured at several locations such as Olympic Park (37.52 N, 127.12 E), Bangnyung (37.96 N, 124.64 E), Bulkwang (37.61 N, 126.93 E), Mt. Taewha (37.31 N, 127.31 E), Daejeon (36.35 N, 127.38 E), Gwangju (35.23 N, 126.84 E), Ulsan (35.53 N, 129.31 E), and Jeju (33.32 N, 126.40 E) (refer to Fig. 1b regarding the locations). In this study, we also used data observed at approximately 320 stations from the AIRKOREA network (https://m.airkorea.or.kr/main, last access: 28 October 2024), officially managed by the Korean Ministry of Environment in South Korea.
Surface data observed at the Olympic Park station in Seoul were used for direct comparisons between simulated and observed HONO mixing ratios. These HONO mixing ratios were measured using the Monitor for AeRosols and GAses in ambient air (MARGA ADI 2080) (Applikon-ECN, Netherlands) instrument with a time resolution of 1 h. This measurement is based on the wet denuder–ion chromatography (WD/IC) method. In the WD/IC system, HONO molecules absorbed by the solution in the denuder were converted to nitrite (), and then the nitrite concentrations were quantified by ion chromatography (Xu et al., 2019). The detection limit of the MARGA instrument for HONO is ∼0.02 ppb. At the Olympic Park station, NO2 and O3 were also measured using commercially available instruments, EC9841 and EC9810, respectively, manufactured by Ecotech. Their detection limits for both species are ∼0.5 ppb during the daytime. Details on the principles of EC9841 and EC9810 can be found in Keywood et al. (2019). PM2.5 at the Olympic Park station was measured continuously using a Thermo Scientific continuous particulate monitor, FH62C14, based on the beta attenuation method. The detection limit of the instrument is 4 µg m−3 in hourly measurements. Further information about instruments is provided in Table S1 in the Supplement.
Meteorological data on temperature, relative humidity, pressure, wind speeds, and wind directions were also measured by the Automated Synoptic Observing System (ASOS) at the Olympic Park station. In order to test the simulation performances of the WRF-CMAQ model, observed meteorological data were compared with the modeled outputs, which is shown in Fig. S1 and Table S2. In general, WRF model simulations tended to accurately predict meteorological fields.
2.3 HONO sources
In this study, we considered several possible missing HONO sources or processes in the CMAQ model simulations. The possible missing HONO sources include gas-phase HONO reactions, three HONO emission sources, three heterogeneous HONO reactions, and two photolytic reactions. The considered possible missing HONO sources are also contrasted to the current HONO processes embedded in the CMAQ v5.2.1 model in Table 1. The details of each HONO process are discussed below.
Table 1Comparison of parameterizations of HONO processes between CMAQ v5.2.1 and this study.
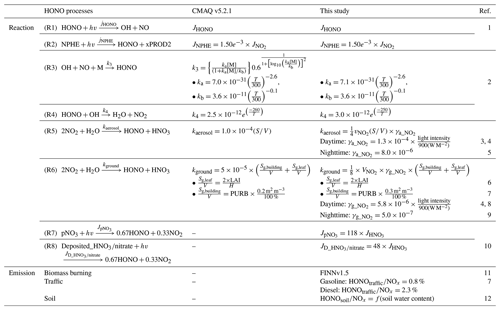
1 Burkholder et al. (2015). 2 Burkholder et al. (2020). 3 Xue et al. (2022). 4 Czader et al. (2012). 5 VandenBoer et al. (2013). 6 Sarwar et al. (2008). 7 Zhang et al. (2016). 8 Yu et al. (2021). 9 Yu et al. (2022). 10 Fu et al. (2019). 11 Wiedinmyer et al. (2011). 12 Meusel et al. (2018).
2.3.1 Gas-phase reactions (GAS)
We used the SAPRC-07 TC chemical mechanism as a base mechanism. A total of four gas-phase HONO-related reactions were considered for HONO formation and dissociation (Carter, 2010; Foley et al., 2010; Appel et al., 2017). HONO is produced by (i) photolysis of nitrophenol (NPHE) (Reaction R2) and (ii) reaction of NO with OH in the presence of the third body (M) (Reaction R3). Meanwhile, HONO is removed by reaction with OH radicals (Reaction R4) and photolytic dissociation (Reaction R1). All these reactions are shown below:
where JNPHE (Reaction R2) and JHONO (Reaction R1) are the photolysis rates constants of NPHE and HONO, respectively, which were adopted from the study of Stockwell et al. (1990). As shown in Table 1, JNPHE was calculated, based on (i.e., ), which was defined in Bejan et al. (2006). k3 and k4 are the reaction rate constants of Reactions (R3) and (R4) and were obtained from the National Aeronautics and Space Administration (NASA) Jet Propulsion Laboratory (JPL) Publication 19 (Burkholder et al., 2015). Among these reactions, the reaction rate constants of Reactions (R3) and (R4) were updated in our study (refer to Table 1). The effect of these gaseous reactions on HONO mixing ratios was tested in the EXP1 simulation (see GAS in Table 2).
Table 2Design for eight EXP simulations.
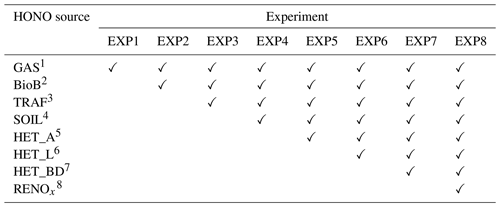
1 Gas-phase reactions. 2 Biomass burning emissions. 3 Traffic emissions. 4 Soil emissions. 5 Heterogeneous reactions on aerosol surfaces. 6 Heterogeneous reactions on the surfaces of leaves. 7 Heterogeneous reactions on the surfaces of buildings. 8 Renoxification.
2.3.2 Biomass burning emissions (BioB)
Biomass combustion includes three types of burning events: natural wildfires, agricultural fires, and wood burning (Wiedinmyer et al., 2011). In East Asia, agricultural fires typically occur in early summer and fall (Ryu et al., 2004; Tao et al., 2013; Zhang et al., 2013). The period of the KORUS-AQ campaign coincides with the period of the agricultural residue burning after barley and wheat harvest in East Asia. Biomass burning emissions, including agricultural fire emissions, were obtained from the Fire INventory from NCAR version 1.5 (FINN v1.5; Wiedinmyer et al., 2006, 2011). This was then considered in the EXP2 simulation (see BioB in Table 2). The spatial distributions of HONO emissions from the biomass burning events in the East Asia domain (A1), South Korea domain (A2), and Seoul Metropolitan Area domain (A3) are presented in Fig. 1a and b. However, we found that the HONO emission rates used in the EXP2 simulation were relatively small, compared to the total HONO emission rates presented in Fig. 1 and Table 3.
2.3.3 Traffic emissions (TRAF)
Traffic emissions are an important HONO source, particularly at night (Zhang et al., 2016). HONO is emitted directly from vehicle exhaust systems. In this study, to estimate the direct HONO emissions from traffic sources, we assumed that the HONO-to-NOx emission ratio is 0.8 % for gasoline vehicles and 2.3 % for diesel vehicles (Zhang et al., 2016). All off-road vehicles were treated as diesel vehicles in the calculations of HONO emissions (Gutzwiller et al., 2002). Table 3 presents the total emission rates for East Asia, South Korea, and the Seoul Metropolitan Area, which are 6.40, 0.32, and 0.1 Mg s−1, respectively. Moreover, as shown in Fig. 1c and d, HONO emissions from traffic sources are dominant, particularly in metropolitan areas such as Seoul, Beijing, Shanghai, and Hong Kong SAR. The contribution of traffic sources to total HONO emissions was estimated to be dominant in the Seoul Metropolitan Area. In the EXP3 simulation, the impact of traffic sources (see TRAF in Table 2) on the atmospheric HONO mixing ratios was investigated.
2.3.4 Soil emissions (SOIL)
Emissions from soil bacterial activity are important sources of HONO. The amount of their emissions depends on the soil type, land category, fertilization, temperature, soil water content (SWC in %), and soil pH (Meusel et al., 2018; Wu et al., 2020). In this study, HONO emissions were estimated based on the ratio of HONO to NOx emissions from soil (Oswald et al., 2013). SWC was used as a proxy for soil pH due to the technical limitations of direct measurement of the soil pH. The SWCs were set at 0 %–7.5 %, 7.5 %–15 %, 15 %–20 %, 20 %–30 %, and 30 %–40 % for HONO-to-NOx ratios of 1.0, 0.67, 0.75, 0.5, and 0.25, respectively, because the ratio of HONO to NOx is very sensitive to the water content in the soil. For this estimation, monthly soil NOx emissions were acquired from the MEGAN v2.10 model.
The HONO emission rate from soil was estimated at 0.06 Mg s−1 for South Korea, accounting for ∼16 % of the total HONO emission rate in South Korea (refer to Table 3). The spatial distributions of emission are presented in Fig. 1e and f. The impact of HONO soil emissions (see SOIL in Table 2) was examined in the EXP4 simulation.
2.3.5 Heterogeneous reaction of NO2 on atmospheric aerosol surfaces (HET_A)
In the EXP5 simulation, we added the heterogeneous reaction of NO2 on the surface of atmospheric aerosols via Reaction (R5) (see HET_A in Table 2), which has been reported to be a possible pathway for HONO formation (Svensson et al., 1987; Wiesen et al., 1995; Reisinger, 2000; Han et al., 2017; Lu et al., 2018).
We found a similar diurnal pattern of the concentration ratio to the HONO mixing ratio at the Olympic Park station. This indicates that the conversion of NO2 to HONO via Reaction (R5) may be a main process for HONO formation (Fig. S2). The ratios at the Olympic Park station in Seoul ranged from 1.9 % to 6.8 % during the KORUS-AQ campaign, which is also comparable to those observed in Taichung, Taiwan, and Shanghai, China (Tong et al., 2015; Hao et al., 2020).
The current AERO6 module in the CMAQv5.2.1 model already considers Reaction (R5) but does not take into account photo-enhancement. However, several previous studies suggested that the photo-enhanced reactions should produce more HONO molecules during the daytime (Li et al., 2010; Czader et al., 2012; Colussi et al., 2013; Levy et al., 2014; Fu et al., 2019). The potential photo-enhancement of the Reaction (R5) was taken into account by making kaerosol dependent on the magnitude of light intensity:
where , , and represent the mean molecular velocity of NO2 (m s−1), the aerosol surface density (m2 m−3), and the NO2 uptake coefficient on the surface of atmospheric aerosols, respectively. The values of were finally selected from the sensitivity tests. It should also be noted in Table 1 that the CMAQ v5.2.1 model simply uses a fixed reaction constant (i.e., ) for this heterogeneous reaction.
2.3.6 Heterogeneous reactions of NO2 on tree leaf and building surfaces (HET_L and HET_BD)
The heterogeneous reaction of NO2 can also take place on the ground surfaces (e.g., tree leaves and buildings). Several studies have reported that heterogeneous reactions on the surfaces of tree leaves and buildings via Reaction (R6) can contribute to the HONO mixing ratios in the atmosphere (An et al., 2013; Karamchandani et al., 2015; Hou et al., 2016; Zhang et al., 2016). Therefore, we also considered these photo-enhanced heterogeneous NO2 reactions.
In this study, kL&B was calculated using Eq. (2), with a modification of the equation:
where is the NO2 uptake coefficient on the ground surfaces. These values are also selected from sensitivity tests. Here, represents the ratios of the building surface area to the volume, which were calculated from Eq. (3):
where PURB represents the percentage of building area with a maximum value of 0.3 (Zhang et al., 2016). For vegetation areas, (the ratio of the leaf surface to volume) was estimated based on leaf area index (LAI) information, along with Eq. (4) proposed by Sarwar et al. (2008):
where H represents the height of the first layer of the model simulation (Sarwar et al., 2008; Yuan et al., 2011; Zhang et al., 2012). The LAI was obtained from improved Moderate Resolution Spectroradiometer (MODIS) land use data (Yuan et al., 2011).
2.3.7 Photolysis reactions (RENOx)
Several measurement studies have reported that the photolytic dissociation of particulate nitrate (pNO3) in the atmosphere (Reaction R7) may be able to explain the high HONO mixing ratios observed during the daytime (Ye et al., 2017; Romer et al., 2018). Other studies suggested that the photolysis reactions of HNO3 and nitrate deposited on tree canopies and artificial surfaces (Reaction R8) can also be significant sources of daytime HONO, particularly in rural areas (Zhou et al., 2011; Ye et al., 2016). All these heterogeneous reactions from N(V) to N(III) or N(IV) are called atmospheric “renoxification”. Some studies have also reported that these types of reduction reactions actually take place in the snow (Chen et al., 2019). In order to better estimate the daytime mixing ratios of HONO in the atmosphere, Reactions (R7) and (R8) were included in the EXP8 simulation (see RENOx in Table 2).
In the EXP8 simulation, we chose equations for both the photolysis rate constant of particulate (denoted by ) and the photolysis rate constant of HNO3/nitrate deposited on surfaces (denoted by ), following the methods proposed by Zhang et al. (2022) and Fu et al. (2019). These equations are presented below:
where is the reaction rate constant of gaseous HNO3 photo-dissociation, which is calculated by the photolysis rate preprocessor module (JPROC) in the CMAQ model.
In this section, we first evaluated the performances of the modified CMAQ models in terms of HONO mixing ratios by comparing the model outputs with ground-based observations from the Olympic Park station in South Korea. We then carried out sensitivity tests to estimate the contributions of the various atmospheric HONO processes to atmospheric HONO mixing ratios.
3.1 Observed vs. Simulated HONO mixing ratios
Figure 2 presents the hourly variations of the HONO mixing ratios at the Olympic Park station. Observations are marked with open black circles, and colored lines represent HONO mixing ratios calculated from the eight EXP simulations. When HONO sources were added sequentially to the experiments, the HONO mixing ratios averaged over the entire simulation period increased from 0.06 ppb (EXP1 simulation) to 1.18 ppb (EXP8 simulation). The average HONO mixing ratios in the EXP8 simulation, which took into account all the HONO processes, were almost comparable to those observed from the ground (1.35 ppb of HONO).
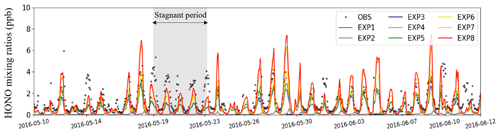
Figure 2Hourly variations of the HONO mixing ratios (unit: ppb) at the Olympic Park station in Seoul. The observations are marked with black circles, and the colored lines represent the HONO mixing ratios obtained from the eight experimental simulations.
The CMAQ-simulated HONO mixing ratios were particularly underestimated from 19 May to 23 May 2016 (refer to grey-shaded area in Fig. 2). This period was characterized by low wind speeds and poor mixing within the planetary boundary layer height (PBLH), which can lead to the accumulation of air pollutants (Crawford et al., 2021). On the other hand, the WRF model has a strong tendency to produce higher wind speeds than the actual ones, which may lead to underestimation of air pollutant concentrations (Jo et al., 2017). In particular, the modeled wind speed during the stagnant period is overestimated by 36.3 % compared to the observed wind speed, which is significantly higher than the overestimation of 23.4 % for the entire KORUS-AQ period. Therefore, the underestimation of the HONO mixing ratios may be caused by the overestimation of the wind speed on a given period. Despite all the discrepancies, the HONO mixing ratios agree relatively well with the observed HONO mixing ratios during the period of the KORUS-AQ campaign.
Figure 3 shows the diurnal variations of average HONO mixing ratios estimated from the eight EXP simulations, together with HONO observations at the Olympic Park station. For the analysis of Fig. 3, daytime and nighttime are defined as 06:00–18:00 and 18:00–06:00 LST (local standard time), respectively. The EXP1 simulation showed slightly elevated HONO mixing ratios during the daytime (purple line in Fig. 3) due to the net production of HONO in the gas phase. The peak mixing ratio of the simulated HONO is ∼0.14 ppb, which is significantly lower than the observed mixing ratio. The large differences between EXP1 results and observations suggest that there should be more unaccountable sources of HONO, which should be further taken into account in our model simulations.
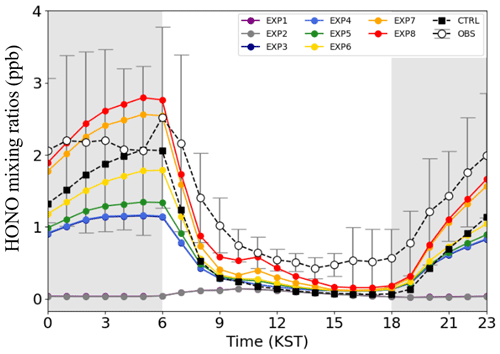
Figure 3Diurnal variations of HONO mixing ratios (unit: ppb) at the Olympic Park station averaged over the period of the KORUS-AQ campaign. Error bars and grey-shaded areas indicate 1 standard deviation and nighttime (18:00–06:00 LST, local standard time), respectively.
In the EXP2 simulation, HONO emissions from biomass burning were added. Several studies have reported that direct and indirect sources emitted from biomass burning events could contribute to the primary/secondary HONO formation (Gen et al., 2021; Wang et al., 2021; Jiang et al., 2023). However, the addition of these biomass burning emissions resulted in nearly negligible impact on the HONO mixing ratios because no major biomass burning events occurred in South Korea during the period of the KORUS-AQ campaign (refer to Fig. 1b). Thus, there are minimal differences between the EXP1 and EXP2 simulations (i.e., between the purple and grey lines in Fig. 3).
The EXP3 simulation was then carried out to examine the impact of traffic sources (TRAF) on HONO mixing ratios. The average HONO mixing ratio increases to ∼0.55 ppb. As previously discussed in Fig. 1c, HONO emissions from traffic sources can be significant, particularly in the Seoul Metropolitan Area. However, simulated levels of HONO are still much lower than observed levels of HONO.
HONO emissions from soil (SOIL) were further included in the EXP4 simulation. As discussed previously, several studies have reported that the consideration of soil emissions can lead to large increases in atmospheric HONO mixing ratios, particularly in East Asia (Fig. 1e). However, it was found that almost no significant changes had occurred in South Korea. This is because the low soil NOx levels in South Korea are linked to several factors: (i) geographical features mainly covered by forest and mountains areas, (ii) the use of low nitrogen fertilizers, (iii) the reduced availability of nitrogen in the soil caused by acidic deposition, and (iv) the relatively high soil water content (SWC) over the Korean Peninsula (Kim et al., 2008; An et al., 2023).
In the EXP5 simulation, the heterogeneous reactions of NO2 on the surfaces of atmospheric aerosols (HET_A) were further taken into account. The addition of these reactions was found to have only a minor effect on the HONO mixing ratios because used in Eq. (1) is too small to enhance the HONO mixing ratios in Reaction (R5). In our study, the heterogeneous reactions on the surface of atmospheric aerosols only contribute ∼0.06 ppb. The heterogeneous reactions can potentially be important in more polluted regions where larger aerosol surface areas are available (Zhang et al., 2019).
Conversely, the HONO mixing ratios can be greatly enhanced by NO2-to-HONO conversions on the surfaces of the tree leaves and buildings. These two processes were implemented in the EXP6 and EXP7 simulations (HET_L and HET_BD). In these two cases, there were significant increases in the HONO mixing ratios, particularly during the nighttime (i.e., on average, increases of 0.23 and 0.55 ppb in the HONO mixing ratios were found in the EXP6 and EXP7 simulations, respectively).
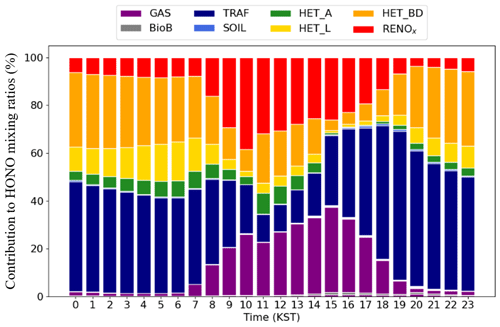
Figure 4Diurnal contributions of individual HONO processes to the HONO mixing ratios at the Olympic Park station during the period of the KORUS-AQ campaign.
Table 4Statistical analysis with modeled and observed HONO mixing ratios at the Olympic Park station, Seoul, South Korea.
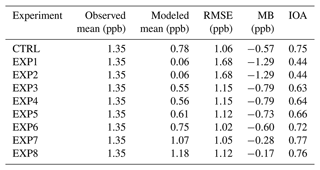
Finally, the photolytic renoxification of nitrate was added to the EXP8 simulation. In this EXP8 simulation, the average HONO mixing ratios increased by 0.11 ppb. The enhancement in the HONO mixing ratios was particularly large in the early morning (an increase of ∼0.23 ppb was found at 06:00 LT). Overall, the EXP8 simulation produced the best HONO mixing ratios (average value of 1.18 ppb), compared to observed HONO mixing ratio (1.35 ppb). Also, the estimated HONO mixing ratios were more comparable than those in the CTRL (original CMAQ v5.2.1) model simulation (represented by black squares in Fig. 3). Again, it is noted that our simulations incorporated “new” HONO processes, such as (i) the photo-enhanced HONO production pathway through Reactions (R5) and (R6), (ii) daytime HONO production from renoxification reactions through Reactions (R7) and (R8), and (iii) HONO emissions (refer to Table 1).
In addition to the graphical comparison in Fig. 3, several statistical metrics were also calculated to evaluate the performances of the eight EXP and CTRL simulations in Table 4. Significant improvements were found when the HONO processes were sequentially added from the EXP1 to the EXP8 simulations. For example, the index of agreement (IOA) increases from 0.44 to 0.76, and the mean bias (MB) decreases drastically from −1.29 to −0.17 ppb from the EXP1 to the EXP8 simulation. In particular, the EXP8 simulation showed the best performance, compared to the CTRL simulation during the daytime. For example, the IOA during the daytime increased from 0.59 to 0.68, while the MB decreased from −0.57 to −0.34, respectively. The root mean square error (RMSE) also decreased from 0.80 to 0.70 during the daytime.
Although the EXP8 simulation showed a notable enhancement in HONO production, the HONO mixing ratios were still underestimated during the daytime. Such underestimation of HONO mixing ratios during the daytime could be attributed to stronger HONO photo-dissociation than in real situations. This is possibly due to failure in predicting cloud shade fractions in meteorological modeling and/or due to additional sources that were not considered in this study (e.g., acid displacement for HNO3 and HCl, nitrate and Fe(II) in iron–organic complex under irradiation, and renoxification of nitrate in presence of carbonaceous aerosols) (VandenBoer et al., 2013; Gen et al., 2021; Wang et al., 2021). This certainly indicates that additional work is needed to further investigate HONO formation and removal during the daytime.
3.2 Relative contribution of HONO sources
Individual HONO processes affect the HONO mixing ratios in different ways. Figure 4 summarizes the relative contribution of HONO processes to the HONO mixing ratios. During the daytime, both GAS and RENOx contribute significantly to the production of atmospheric HONO molecules. In particular, the contribution of these two processes is the largest between 10:00 and 16:00 LT, when sunlight is strong. These two processes account for 29.1 % and 29.8 % of the daytime HONO production, respectively, but are almost negligible during the nighttime.
During the nighttime, TRAF (denoted by navy color in Fig. 4) contributes the large portion of 47.2 % of the total HONO production. However, there is a possibility that TRAF might have been somewhat overestimated during the nighttime since we applied constant diurnal anthropogenic NOx emissions, including those from traffic sources. In turn, HET_BD and HET_L exhibit substantial contributions of 28.5 % and 10.6 %, respectively, during the nighttime. The contributions of other processes such as biomass burning (BioB) and heterogeneous reactions to atmospheric aerosols (HET_A) are minimal. HET_A only contributes 4.3 % during the nighttime. In terms of the average 24 h contribution, TRAF (41.4 %), HET_BD (27.1 %), and HET_L (11.1 %) are the largest sources of atmospheric HONO at the Olympic Park station.
Using the same approach, we analyzed the HONO source contributions across South Korea during the period of the KORUS-AQ campaign. As shown in Fig. 5f and c, HET_L and TRAF were modeled to have the largest impacts on HONO production, contributing 0.15 ppb (41.5 %) and 0.08 ppb (18.1 %), respectively, across South Korea (also, refer to the incremental ratio in Fig. S3).
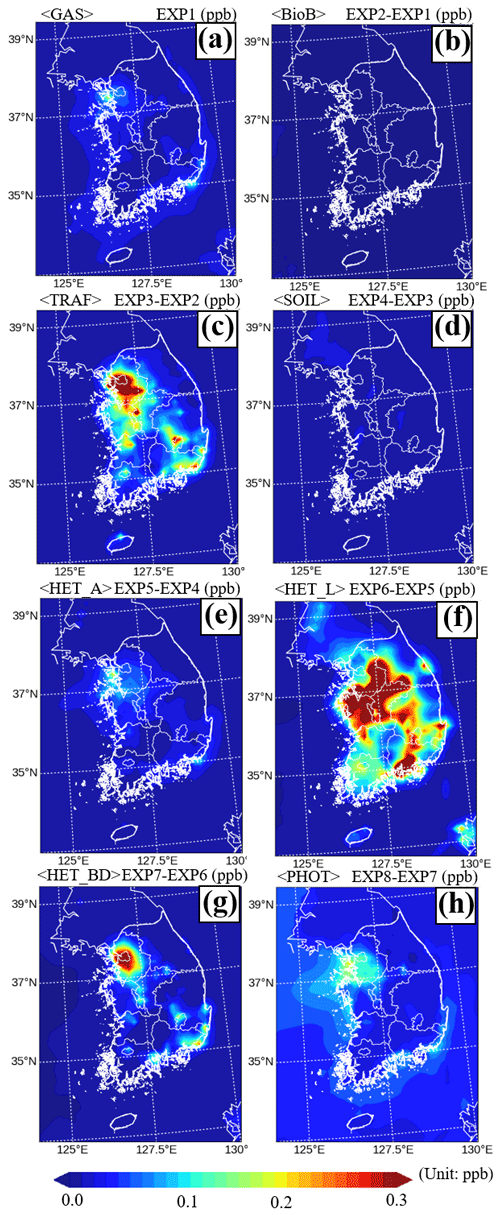
Figure 5Spatial impacts of (a) gas-phase reactions, (b) biomass burning emissions, (c) traffic emissions, (d) soil emissions, (e) heterogeneous reactions on the aerosol surfaces, (f) heterogeneous reactions on the leaf surfaces, (g) heterogeneous reactions on the building surfaces, and (h) renoxification on HONO mixing ratios, based on model simulations during the period of the KORUS-AQ campaign in South Korea.
Figure 6 shows the contributions of different sources to the HONO mixing ratios at eight super monitoring stations. As shown in Fig. 6, each station has different characteristics in terms of source contribution. In particular, the contribution of HET_L at the Daejeon is 44.4 %. Also, TRAF in Bulkwang, Olympic Park, Mt. Taehwa, Ulsan, and Gwangju has large contributions of 41.2 %, 41.4 %, 29.3 %, 29.6 %, and 40.5 %, respectively. As for TRAF and HET_BD, their contributions are only high in densely populated cities (refer to Fig. 5c and g). On the other hand, the contributions of BioB, SOIL, HET_A, and RENOx sources were insignificant, as shown in Fig. 5b, d, e, and h.
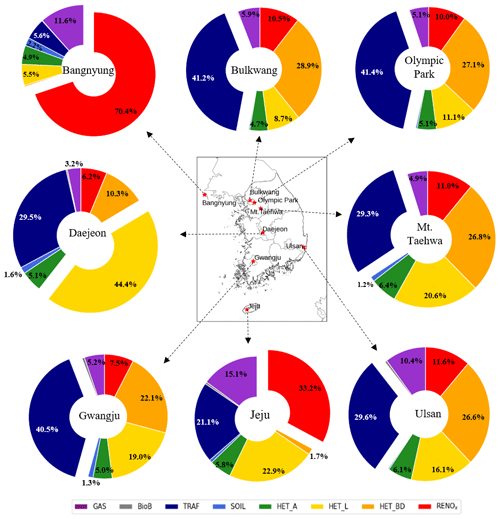
Figure 6Contributions of individual processes to the average HONO mixing ratios at eight monitoring stations during the period of the KORUS-AQ campaign.
Meanwhile, at the Bangnyung and Jeju stations, RENOx has the largest contribution of 70.4 % and 33.2 %, respectively. This is because the amounts of NO2 and HONO from direct emissions (BioB, TRAF, and SOIL) are relatively small. The Bangnyung and Jeju stations are located on remote and less populated islands.
3.3 Impact of HONO processes on atmospheric species
3.3.1 Impact on atmospheric species
We also investigated the effect of HONO processes on atmospheric levels of HOx (i.e., OH+HO2), HCHO, O3, NO, and PM2.5 at the Olympic Park station. Figure 7 presents the diurnal concentrations of these gaseous and particulate species at the Olympic Park station. The mixing ratios of OH and HO2 radicals in the EXP8 simulation increased by 0.02 ppt (35.2 %) and 0.23 ppt (39.2 %), respectively, compared to those in the CTRL simulation. This is certainly due to the enhancement in OH levels due to HONO photo-dissociation and then HO2 levels in the HOx cycle. As shown in Fig. 7a (and 7b), the OH (and HO2) mixing ratios increased from 0.21 to 0.29 ppt (1.71 to 2.28 ppt) at 13:00 local standard time. Subsequently, the HCHO mixing ratios were also enhanced by 0.18 ppb (8.8 %), due to increased VOC oxidation resulting from elevated levels of OH radicals (Fig. 7c). Conversely, the NO mixing ratios in the EXP8 simulation decreased by 2.13 ppb (20.1 %). This may be due to an increase in the mixing ratios of HO2 and RO2 radicals (organic peroxyl radicals) reacting with NO molecules (Fig. 7d). In other words, the reduced levels of NO indicate active NO-to-NO2 conversion via NO+HO2 and NO+RO2 reactions. Such active NO-to-NO2 conversion increases the mixing ratio of atmospheric ozone because these two reactions are rate-determining reactions for ozone production. This is presented in Fig. 7e. In Fig. 7e, the modeled ozone mixing ratios increased, approaching the observed ozone mixing ratios. This is another good result showing that the incorporation of atmospheric HONO processes may be able to enhance the accuracy of prediction of ozone mixing ratios. More details about ozone production are discussed in Sect. 3.3.2.
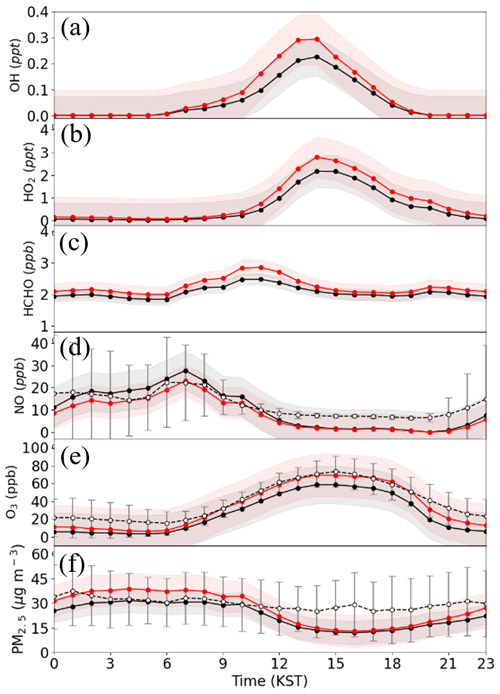
Figure 7Diurnal variations of the mixing ratios of (a) OH, (b) HO2, (c) HCHO, (d) NO, (e) O3, and (f) PM2.5 (black lines represent the mixing ratios from the CTRL simulation, and red lines represent those from the EXP8 simulation) and observations (marked with open white cycles) at the Olympic Park station during the period of the KORUS-AQ campaign. Shaded areas represent 1 standard deviation for each simulation.
Elevated levels of atmospheric O3 and HOx can change the rates of particulate nitrate and sulfate production. In particular, the formation of particulate nitrates and sulfates can also be enhanced by increasing the levels of HNO3, N2O5, and H2SO4. In addition, the nitrate concentration can also be enhanced by the HONO reaction (i.e., via , as accounted for by Reaction R6) during the nighttime. In total, the addition of HONO processes increased PM2.5 by 4.19 µg m−3 (18.6 %) at the Olympic Park station. However, PM2.5 in the EXP8 simulation was still underestimated by 3.16 µg m−3 at the Olympic Park station, as shown in Fig. 7f. There are several potential reasons for this underestimation, such as the underestimation of secondary organic aerosol (SOA) formation (e.g., Murphy et al., 2017). This issue may require further investigation in the future.
Figure S4 presents similar results for 320 AIRKOREA monitoring stations in South Korea. The impacts of HONO processes on atmospheric levels of OH, HO2, O3, and PM2.5 are also presented in Fig. S5. Overall, we found that incorporating HONO chemistry into the modeling system tends to enhance the mixing ratios of HOx, which in turn increases the mixing ratios of O3 and PM2.5.
3.3.2 Impact on net ozone production
The ozone mixing ratio is determined by the balance between ozone formation and destruction in the atmosphere. To better understand the impacts of HONO chemistry on ozone production, we quantitatively analyze the rate of net ozone production (P(O3)). The P(O3) is defined by Eq. (7):
where F(O3) and D(O3) represent the rate of ozone formation and destruction, respectively. F(O3) and D(O3) can be calculated from Eqs. (8) and (9), respectively (Song et al., 2003; Mazzuca et al., 2016).
where ki represents the reaction rate constants for each reaction i. In particular, khet denotes the heterogeneous reaction rate constants of N2O5 radicals.
Figure 8a shows the diurnal variations of F(O3), D(O3), and P(O3) from the CTRL and EXP8 simulations. Including HONO processes in the EXP8 simulation resulted in an average P(O3) that was 10.6 % higher than in the CTRL simulation. This is the primary reason for the ozone enhancement in Fig. 7e.
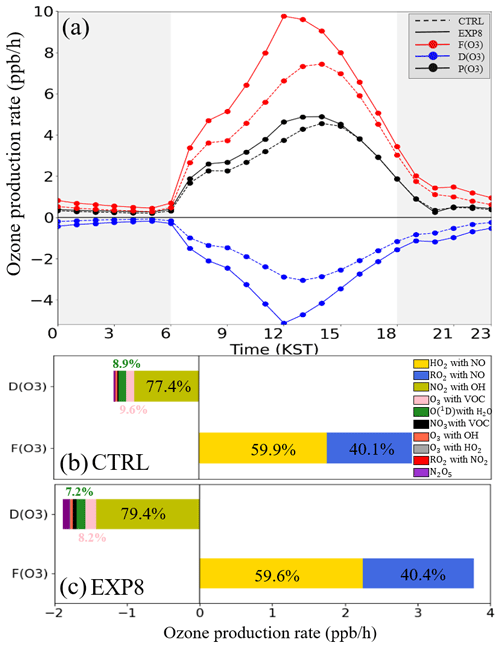
Figure 8Diurnal variations of (a) net ozone production rate (P(O3); black line), ozone formation rate (F(O3); red line), and ozone loss rate (D(O3); blue line). The dashed and solid lines represent the CTRL and EXP8 simulations, respectively. Cumulative bar chart for D(O3) and F(O3) in the case of (b) CTRL and (c) EXP8 simulations at the Olympic Park station during the period of the KORUS-AQ campaign.
Figure 8b and c provide more details about the budget of ozone production. The main increase in F(O3) occurred through the reactions of HO2+NO and RO2+NO. On the other hand, the increase in D(O3) was mainly controlled by the NO2+OH reaction at the Olympic Park station. The increases in the HO2+NO and RO2+NO reaction rate exceeded the increases in the reaction rate of NO2+OH, leading to the net positive ozone production (i.e., positive P(O3)) shown in Fig. 8a.
In this study, we successfully incorporated the following HONO processes into the CMAQ modeling framework to enhance the accuracy in the predictions of HONO mixing ratios: (i) gas-phase HONO reactions; (ii) HONO emission from biomass burning; (iii) HONO emission from traffic and soil; (iv) photo-induced heterogeneous reactions on the surfaces of atmospheric aerosols, tree leaves, and buildings; and (v) photolysis reactions of particulate nitrate and deposited HNO3/nitrate. The analysis showed that the incorporation of HONO processes into the CMAQ model framework increased the average HONO mixing ratios from 0.78 to 1.18 ppb compared to the CTRL simulation. Average mixing ratios of HONO and its diurnal patterns became much more comparable to observations, with large improvements in statistical parameters. Especially during the daytime, IOA increased from 0.59 to 0.68, while the MB decreased from −0.57 to −0.34 ppb, and RMSE dropped from 0.80 to 0.70 ppb, as HONO processes were fully incorporated into the CMAQ model.
Several findings also emerged from the sensitivity simulations. First, each HONO process had a different effect on the HONO mixing ratios during the daytime and the nighttime at the Olympic Park station. For example, the GAS (29.1 %) and RENOx processes (29.8 %) had major contributions to the mixing ratios of HONO during the daytime, while the TRAF (47.2 %) and HET_BD (28.5 %) processes had large contributions to the mixing ratios of HONO during the nighttime. During the period of the KORUS-AQ campaign, HONO mixing ratios estimated at the Olympic Park station were enhanced by an average of 41.4 % (TRAF), 27.1 % (HET_BD), and 11.1 % (HET_L).
In the experimental simulation including all the HONO processes (i.e., EXP8 simulation), the mixing ratios of OH, HO2, HCHO, O3, and PM2.5 at the Olympic Park station increased by 0.02 ppt (35.2 %), 0.23 ppt (39.2 %), 0.18 ppb (8.8 %), 7.86 ppb (30.8 %), and 4.19 µg m−3 (18.6 %), respectively, compared to those from the CTRL simulation. The net ozone production rate was enhanced by 0.19 ppb h−1 (10.6 %) with the EXP8 simulation. These increases in P(O3) were mainly caused by the increased reaction rates of HO2+NO.
In this study, we improved our understanding of atmospheric HONO processes in South Korea. Nevertheless, we believe that both further field studies and modeling investigations are necessary for many remaining HONO-related issues such as NO2 uptake coefficient, possible missing HONO sources, and daytime photochemical reaction pathways of HONO. Such studies will also help to further improve the performances of current CTMs.
For example, the Airborne and Satellite Investigation of Asian Air Quality (ASIA-AQ) field campaign organized by the National Institute of Environmental Research (NIER) in South Korea and the National Aeronautics and Space Administration (NASA) in the United States is planned in 2024 in South Korea. In this campaign, the HONO mixing ratios are scheduled to be measured in the aircraft and at the ground station. This joint campaign is thus expected to provide a valuable opportunity to expand our knowledge on atmospheric HONO processes and HONO photochemistry.
After user registration, the WRF model 3.8.1 (https://doi.org/10.5065/D6MK6B4K, WRF Users Page, 2024) and CMAQ v5.2.1 (https://doi.org/10.5281/zenodo.1079909, US EPA Office of Research and Development, 2015) are available online. The observation data we used can be accessed at https://doi.org/10.5067/Suborbital/KORUSAQ/DATA01 (NASA, 2019).
The supplement related to this article is available online at: https://doi.org/10.5194/acp-24-12575-2024-supplement.
KK designed experiments and led manuscript writing and conceptualization. KMH and CHS supervised this project and contributed to experimental design and manuscript writing. HL, RB, JY, GY, and BK performed research development. JM contributed to editing and writing review. JHW and SC provided useful datasets.
At least one of the (co-)authors is a member of the editorial board of Atmospheric Chemistry and Physics. The peer-review process was guided by an independent editor, and the authors also have no other competing interests to declare.
Publisher's note: Copernicus Publications remains neutral with regard to jurisdictional claims made in the text, published maps, institutional affiliations, or any other geographical representation in this paper. While Copernicus Publications makes every effort to include appropriate place names, the final responsibility lies with the authors.
All authors are grateful for the support of the FRIEND (Fine Particle Research Initiative in East Asia Considering National DIfferences) project and the National Research Foundation of Korea (NRF). We are also grateful for the reviewers' comments, which contributed to enhancing this article.
This research has been supported by the National Research Foundation of Korea (grant nos. 2020M3G1A1114617 and 2021R1A2C1006660).
This paper was edited by Anoop Mahajan and reviewed by two anonymous referees.
Acker, K., Möller, D., Wieprecht, W., Meixner, F. X., Bohn, B., Gilge, S., Plass-Dülmer, C., and Berresheim, H.: Strong daytime production of OH from HNO2 at a rural mountain site, Geophys. Res. Lett., 33, L02809, https://doi.org/10.1029/2005GL024643, 2006.
Alicke, B., Geyer, A., Hofzumahaus, A., Holland, F., Konrad, S., Pätz, H., Schäfer, J., Stutz, J., Volz-Thomas, A., and Platt, U.: OH formation by HONO photolysis during the BERLIOZ experiment, J. Geophys. Res.-Atmos., 108, PHO 3-1–PHO 3-17, https://doi.org/10.1029/2001JD000579, 2003.
An, J., Li, Y., Chen, Y., Li, J., Qu, Y., and Tang, Y.: Enhancements of major aerosol components due to additional HONO sources in the North China Plain and implications for visibility and haze, Adv. Atmos. Sci., 30, 57–66, https://doi.org/10.1007/s00376-012-2016-9, 2013.
An, Y., Shim, W., and Jeong, G.: High-Resolution Digital Soil Maps of Forest Soil Nitrogen across South Korea Using Three Machine Learning Algorithms, Forests, 14, 1141, https://doi.org/10.3390/f14061141, 2023.
Appel, K. W., Napelenok, S. L., Foley, K. M., Pye, H. O. T., Hogrefe, C., Luecken, D. J., Bash, J. O., Roselle, S. J., Pleim, J. E., Foroutan, H., Hutzell, W. T., Pouliot, G. A., Sarwar, G., Fahey, K. M., Gantt, B., Gilliam, R. C., Heath, N. K., Kang, D., Mathur, R., Schwede, D. B., Spero, T. L., Wong, D. C., and Young, J. O.: Description and evaluation of the Community Multiscale Air Quality (CMAQ) modeling system version 5.1, Geosci. Model Dev., 10, 1703–1732, https://doi.org/10.5194/gmd-10-1703-2017, 2017.
Bejan, I., Abd El Aal, Y., Barnes, I., Benter, T., Bohn, B., Wiesen, P., and Kleffmann, J.: The photolysis of ortho-nitrophenols: a new gas phase source of HONO, Phys. Chem. Chem. Phys., 8, 2028–2035, https://doi.org/10.1039/B516590C, 2006.
Binkowski, F. S. and Roselle, S. J.: Models-3 Community Multiscale Air Quality (CMAQ) model aerosol component 1. Model description, J. Geophys. Res.-Atmos., 108, 4183, https://doi.org/10.1029/2001JD001409, 2003.
Burkholder, J., Sander, S., Abbatt, J., Barker, J., Huie, R., Kolb, C., Kurylo, M., Orkin, V., Wilmouth, D., and Wine, P.: Chemical Kinetics and Photochemical Data for Use in Atmospheric Studies, Evaluation No. 18, JPL Publication 15-10, Jet Propulsion Laboratory, Pasadena, http://jpldataeval.jpl.nasa.gov (last access: 28 October 2024), 2015.
Burkholder, J. B., Sander, S. P., Abbatt, J. P. D., Barker, J. R., Huie, R. E., Kolb, C. E., Kurylo, M. J., Orkin, V. L., Wilmouth, D. M., and Wine, P. H.: Chemical kinetics and photochemical data for use in atmospheric studies – evaluation number 19, Nasa panel for data evaluation technical report, 19-5, https://jpldataeval.jpl.nasa.gov/pdf/NASA-JPL%20Evaluation%2019-5.pdf (last access: 28 October 2024), 2020.
Byun, D. and Schere, K. L.: Review of the Governing Equations, Computational Algorithms, and Other Components of the Models-3 Community Multiscale Air Quality (CMAQ) Modeling System, Appl. Mech. Rev., 59, 51–77, https://doi.org/10.1115/1.2128636, 2006.
Carter, W. P.: Development of the SAPRC-07 chemical mechanism, Atmos. Environ., 44, 5324–5335, https://doi.org/10.1016/j.atmosenv.2010.01.026, 2010.
Chen, F. and Dudhia, J.: Coupling an advanced land surface–hydrology model with the Penn State–NCAR MM5 modeling system. Part I: Model implementation and sensitivity, Mon. Weather Rev., 129, 569–585, https://doi.org/10.1175/1520-0493(2001)129<0569:CAALSH>2.0.CO;2, 2001.
Chen, Q., Edebeli, J., McNamara, S. M., Kulju, K. D., May, N. W., Bertman, S. B., Thanekar, S., Fuentes, J. D., and Pratt, K. A.: HONO, Particulate Nitrite, and Snow Nitrite at a Midlatitude Urban Site during Wintertime, ACS Earth and Space Chemistry, 3, 811–822, https://doi.org/10.1021/acsearthspacechem.9b00023, 2019.
Cheng, Z., Wang, S., Fu, X., Watson, J. G., Jiang, J., Fu, Q., Chen, C., Xu, B., Yu, J., Chow, J. C., and Hao, J.: Impact of biomass burning on haze pollution in the Yangtze River delta, China: a case study in summer 2011, Atmos. Chem. Phys., 14, 4573–4585, https://doi.org/10.5194/acp-14-4573-2014, 2014.
Colussi, A. J., Enami, S., Yabushita, A., Hoffmann, M. R., Liu, W.-G., Mishra, H., and Goddard III, W. A.: Tropospheric aerosol as a reactive intermediate, Faraday Discuss., 165, 407–420, https://doi.org/10.1039/C3FD00040K, 2013.
Crawford, J. H., Ahn, J.-Y., Al-Saadi, J., Chang, L., Emmons, L. K., Kim, J., Lee, G., Park, J.-H., Park, R. J., Woo, J. H., Song, C.-K., Hong, J.-H., Hong, Y.-D., Lefer, B. L., Lee, M., Lee, T., Kim, S., Min, K.-E., Yum, S. S., Shin, H. J., Kim, Y.-W., Choi, J.-S., Park, J.-S., Szykman, J. J., Long, R. W., Jordan, C. E., Simpson, I. J., Fried, A., Dibb, J. E., Cho, S., and Kim, Y. P.: The Korea–United States Air Quality (KORUS-AQ) field study, Elementa, 9, 00163, https://doi.org/10.1525/elementa.2020.00163, 2021.
Crutzen, P. J. and Andreae, M. O.: Biomass burning in the tropics: Impact on atmospheric chemistry and biogeochemical cycles, Science, 250, 1669–1678, https://doi.org/10.1126/science.250.4988.1669, 1990.
Czader, B. H., Rappenglück, B., Percell, P., Byun, D. W., Ngan, F., and Kim, S.: Modeling nitrous acid and its impact on ozone and hydroxyl radical during the Texas Air Quality Study 2006, Atmos. Chem. Phys., 12, 6939–6951, https://doi.org/10.5194/acp-12-6939-2012, 2012.
Czader, B. H., Choi, Y., Li, X., Alvarez, S., and Lefer, B.: Impact of updated traffic emissions on HONO mixing ratios simulated for urban site in Houston, Texas, Atmos. Chem. Phys., 15, 1253–1263, https://doi.org/10.5194/acp-15-1253-2015, 2015.
Foley, K. M., Roselle, S. J., Appel, K. W., Bhave, P. V., Pleim, J. E., Otte, T. L., Mathur, R., Sarwar, G., Young, J. O., Gilliam, R. C., Nolte, C. G., Kelly, J. T., Gilliland, A. B., and Bash, J. O.: Incremental testing of the Community Multiscale Air Quality (CMAQ) modeling system version 4.7, Geosci. Model Dev., 3, 205–226, https://doi.org/10.5194/gmd-3-205-2010, 2010.
Fu, X., Wang, T., Zhang, L., Li, Q., Wang, Z., Xia, M., Yun, H., Wang, W., Yu, C., Yue, D., Zhou, Y., Zheng, J., and Han, R.: The significant contribution of HONO to secondary pollutants during a severe winter pollution event in southern China, Atmos. Chem. Phys., 19, 1–14, https://doi.org/10.5194/acp-19-1-2019, 2019.
Gen, M., Zhang, R., and Chan, C. K.: Nitrite/nitrous acid generation from the reaction of nitrate and Fe(II) Promoted by photolysis of iron–organic complexes, Environ. Sci. Technol., 55, 15715–15723, https://doi.org/10.1021/acs.est.1c05641, 2021.
Gligorovski, S.: Nitrous acid (HONO): An emerging indoor pollutant, J. Photoch. Photobio. A, 314, 1–5, https://doi.org/10.1016/j.jphotochem.2015.06.008, 2016.
Grell, G. A. and Freitas, S. R.: A scale and aerosol aware stochastic convective parameterization for weather and air quality modeling, Atmos. Chem. Phys., 14, 5233–5250, https://doi.org/10.5194/acp-14-5233-2014, 2014.
Guenther, A. B., Jiang, X., Heald, C. L., Sakulyanontvittaya, T., Duhl, T., Emmons, L. K., and Wang, X.: The Model of Emissions of Gases and Aerosols from Nature version 2.1 (MEGAN2.1): an extended and updated framework for modeling biogenic emissions, Geosci. Model Dev., 5, 1471–1492, https://doi.org/10.5194/gmd-5-1471-2012, 2012.
Gutzwiller, L., Arens, F., Baltensperger, U., Gäggeler, H. W., and Ammann, M.: Significance of semivolatile diesel exhaust organics for secondary HONO formation, Environ. Sci. Technol., 36, 677–682, https://doi.org/10.1021/es015673b, 2002.
Han, C., Yang, W., Yang, H., and Xue, X.: Enhanced photochemical conversion of NO2 to HONO on humic acids in the presence of benzophenone, Environ. Pollut., 231, 979–986, https://doi.org/10.1016/j.envpol.2017.08.107, 2017.
Hao, Q., Jiang, N., Zhang, R., Yang, L., and Li, S.: Characteristics, sources, and reactions of nitrous acid during winter at an urban site in the Central Plains Economic Region in China, Atmos. Chem. Phys., 20, 7087–7102, https://doi.org/10.5194/acp-20-7087-2020, 2020.
Harris, G. W., Carter, W. P., Winer, A. M., Pitts, J. N., Platt, U., and Perner, D.: Observations of nitrous acid in the Los Angeles atmosphere and implications for predictions of ozone-precursor relationships, Environ. Sci. Technol., 16, 414–419, https://doi.org/10.1021/es00101a009, 1982.
Hendrick, F., Müller, J.-F., Clémer, K., Wang, P., De Mazière, M., Fayt, C., Gielen, C., Hermans, C., Ma, J. Z., Pinardi, G., Stavrakou, T., Vlemmix, T., and Van Roozendael, M.: Four years of ground-based MAX-DOAS observations of HONO and NO2 in the Beijing area, Atmos. Chem. Phys., 14, 765–781, https://doi.org/10.5194/acp-14-765-2014, 2014.
Hong, S.-Y. and Lim, J.-O. J.: The WRF single-moment 6-class microphysics scheme (WSM6), Asia-Pac. J. Atmos. Sci., 42, 129–151, 2006.
Hong, S.-Y., Noh, Y., and Dudhia, J.: A new vertical diffusion package with an explicit treatment of entrainment processes, Mon. Weather Rev., 134, 2318–2341, https://doi.org/10.1175/MWR3199.1, 2006.
Hou, S., Tong, S., Ge, M., and An, J.: Comparison of atmospheric nitrous acid during severe haze and clean periods in Beijing, China, Atmos. Environ., 124, 199–206, https://doi.org/10.1016/j.atmosenv.2015.06.023, 2016.
Hutzell, W., Luecken, D., Appel, K., and Carter, W.: Interpreting predictions from the SAPRC07 mechanism based on regional and continental simulations, Atmos. Environ., 46, 417–429, https://doi.org/10.1016/j.atmosenv.2011.09.030, 2012.
Iacono, M. J., Delamere, J. S., Mlawer, E. J., Shephard, M. W., Clough, S. A., and Collins, W. D.: Radiative forcing by long-lived greenhouse gases: Calculations with the AER radiative transfer models, J. Geophys. Res.-Atmos., 113, D13103, https://doi.org/10.1029/2008JD009944, 2008.
Jia, C., Tong, S., Zhang, W., Zhang, X., Li, W., Wang, Z., Wang, L., Liu, Z., Hu, B., and Zhao, P.: Pollution characteristics and potential sources of nitrous acid (HONO) in early autumn 2018 of Beijing, Sci. Total Environ., 735, 139317, https://doi.org/10.1016/j.scitotenv.2020.139317, 2020.
Jiang, H., Bao, F., Wang, J., Chen, J., Zhu, Y., Huang, D., Chen, C., and Zhao, J.: Direct formation of electronic excited NO2 contributes to the high yield of HONO during photosensitized renoxification, Environ. Sci. Technol., 57, 11144–11151, https://doi.org/10.1021/acs.est.3c01342, 2023.
Jiménez, P. A., Dudhia, J., González-Rouco, J. F., Navarro, J., Montávez, J. P., and García-Bustamante, E.: A revised scheme for the WRF surface layer formulation, Mon. Weather Rev., 140, 898–918, https://doi.org/10.1175/MWR-D-11-00056.1, 2012.
Jo, Y. J., Lee, H. J., Chang, L. S., and Kim, C. H.: Sensitivity study of the initial meteorological fields on the PM10 concentration predictions using CMAQ modeling, J. Korean Soc. Atmos. Environ., 33, 554–569, https://doi.org/10.5572/kosae.2017.33.6.554, 2017.
Karamchandani, P., Emery, C., Yarwood, G., Lefer, B., Stutz, J., Couzo, E., and Vizuete, W.: Implementation and refinement of a surface model for heterogeneous HONO formation in a 3-D chemical transport model, Atmos. Environ., 112, 356–368, https://doi.org/10.1016/j.atmosenv.2015.01.046, 2015.
Keywood, M., Selleck, P., Reisen, F., Cohen, D., Chambers, S., Cheng, M., Cope, M., Crumeyrolle, S., Dunne, E., Emmerson, K., Fedele, R., Galbally, I., Gillett, R., Griffiths, A., Guerette, E.-A., Harnwell, J., Humphries, R., Lawson, S., Miljevic, B., Molloy, S., Powell, J., Simmons, J., Ristovski, Z., and Ward, J.: Comprehensive aerosol and gas data set from the Sydney Particle Study, Earth Syst. Sci. Data, 11, 1883–1903, https://doi.org/10.5194/essd-11-1883-2019, 2019.
Kim, C.-H., Park, I.-S., Kim, S.-K., Son, H.-Y., Lee, J.-J., Lee, J.-B., Song, C.-K., and Shim, J.-M.: Estimation and mapping of nitrogen uptake by forest in South Korea, Water Air Soil Poll., 187, 315–325, https://doi.org/10.1007/s11270-007-9519-5, 2008.
Kim, S., VandenBoer, T. C., Young, C. J., Riedel, T. P., Thornton, J. A., Swarthout, B., Sive, B., Lerner, B., Gilman, J. B., and Warneke, C.: The primary and recycling sources of OH during the NACHTT-2011 campaign: HONO as an important OH primary source in the wintertime, J. Geophys. Res.-Atmos., 119, 6886–6896, https://doi.org/10.1002/2013JD019784, 2014.
Kirchstetter, T. W., Harley, R. A., and Littlejohn, D.: Measurement of nitrous acid in motor vehicle exhaust, Environ. Sci. Technol., 30, 2843–2849, https://doi.org/10.1021/es960135y, 1996.
Kleffmann, J., Gavriloaiei, T., Hofzumahaus, A., Holland, F., Koppmann, R., Rupp, L., Schlosser, E., Siese, M., and Wahner, A.: Daytime formation of nitrous acid: A major source of OH radicals in a forest, Geophys. Res. Lett., 32, L05818, https://doi.org/10.1029/2005GL022524, 2005.
Kurtenbach, R., Becker, K., Gomes, J., Kleffmann, J., Lörzer, J., Spittler, M., Wiesen, P., Ackermann, R., Geyer, A., and Platt, U.: Investigations of emissions and heterogeneous formation of HONO in a road traffic tunnel, Atmos. Environ., 35, 3385–3394, https://doi.org/10.1016/S1352-2310(01)00138-8, 2001.
Lee, J. D., Whalley, L. K., Heard, D. E., Stone, D., Dunmore, R. E., Hamilton, J. F., Young, D. E., Allan, J. D., Laufs, S., and Kleffmann, J.: Detailed budget analysis of HONO in central London reveals a missing daytime source, Atmos. Chem. Phys., 16, 2747–2764, https://doi.org/10.5194/acp-16-2747-2016, 2016.
Levy, M., Zhang, R., Zheng, J., Zhang, A. L., Xu, W., Gomez-Hernandez, M., Wang, Y., and Olaguer, E.: Measurements of nitrous acid (HONO) using ion drift-chemical ionization mass spectrometry during the 2009 SHARP field campaign, Atmos. Environ., 94, 231–240, https://doi.org/10.1016/j.atmosenv.2014.05.024, 2014.
Li, D., Xue, L., Wen, L., Wang, X., Chen, T., Mellouki, A., Chen, J., and Wang, W.: Characteristics and sources of nitrous acid in an urban atmosphere of northern China: Results from 1-yr continuous observations, Atmos. Environ., 182, 296–306, https://doi.org/10.1016/j.atmosenv.2018.03.033, 2018.
Li, G., Lei, W., Zavala, M., Volkamer, R., Dusanter, S., Stevens, P., and Molina, L. T.: Impacts of HONO sources on the photochemistry in Mexico City during the MCMA-2006/MILAGO Campaign, Atmos. Chem. Phys., 10, 6551–6567, https://doi.org/10.5194/acp-10-6551-2010, 2010.
Li, X., Brauers, T., Häseler, R., Bohn, B., Fuchs, H., Hofzumahaus, A., Holland, F., Lou, S., Lu, K. D., Rohrer, F., Hu, M., Zeng, L. M., Zhang, Y. H., Garland, R. M., Su, H., Nowak, A., Wiedensohler, A., Takegawa, N., Shao, M., and Wahner, A.: Exploring the atmospheric chemistry of nitrous acid (HONO) at a rural site in Southern China, Atmos. Chem. Phys., 12, 1497–1513, https://doi.org/10.5194/acp-12-1497-2012, 2012.
Li, X., Rohrer, F., Hofzumahaus, A., Brauers, T., Häseler, R., Bohn, B., Broch, S., Fuchs, H., Gomm, S., and Holland, F.: Missing gas-phase source of HONO inferred from Zeppelin measurements in the troposphere, Science, 344, 292–296, https://doi.org/10.1126/science.1248999, 2014.
Lu, X., Wang, Y., Li, J., Shen, L., and Fung, J. C.: Evidence of heterogeneous HONO formation from aerosols and the regional photochemical impact of this HONO source, Environ. Res. Lett., 13, 114002, https://doi.org/10.1088/1748-9326/aae492, 2018.
Mazzuca, G. M., Ren, X., Loughner, C. P., Estes, M., Crawford, J. H., Pickering, K. E., Weinheimer, A. J., and Dickerson, R. R.: Ozone production and its sensitivity to NOx and VOCs: results from the DISCOVER-AQ field experiment, Houston 2013, Atmos. Chem. Phys., 16, 14463–14474, https://doi.org/10.5194/acp-16-14463-2016, 2016.
Meusel, H., Kuhn, U., Reiffs, A., Mallik, C., Harder, H., Martinez, M., Schuladen, J., Bohn, B., Parchatka, U., Crowley, J. N., Fischer, H., Tomsche, L., Novelli, A., Hoffmann, T., Janssen, R. H. H., Hartogensis, O., Pikridas, M., Vrekoussis, M., Bourtsoukidis, E., Weber, B., Lelieveld, J., Williams, J., Pöschl, U., Cheng, Y., and Su, H.: Daytime formation of nitrous acid at a coastal remote site in Cyprus indicating a common ground source of atmospheric HONO and NO, Atmos. Chem. Phys., 16, 14475–14493, https://doi.org/10.5194/acp-16-14475-2016, 2016.
Meusel, H., Tamm, A., Kuhn, U., Wu, D., Leifke, A. L., Fiedler, S., Ruckteschler, N., Yordanova, P., Lang-Yona, N., Pöhlker, M., Lelieveld, J., Hoffmann, T., Pöschl, U., Su, H., Weber, B., and Cheng, Y.: Emission of nitrous acid from soil and biological soil crusts represents an important source of HONO in the remote atmosphere in Cyprus, Atmos. Chem. Phys., 18, 799–813, https://doi.org/10.5194/acp-18-799-2018, 2018.
Monks, P. S., Granier, C., Fuzzi, S., Stohl, A., Williams, M. L., Akimoto, H., Amann, M., Baklanov, A., Baltensperger, U., and Bey, I.: Atmospheric composition change–global and regional air quality, Atmos. Environ., 43, 5268–5350, https://doi.org/10.1016/j.atmosenv.2009.08.021, 2009.
Murphy, B. N., Woody, M. C., Jimenez, J. L., Carlton, A. M. G., Hayes, P. L., Liu, S., Ng, N. L., Russell, L. M., Setyan, A., Xu, L., Young, J., Zaveri, R. A., Zhang, Q., and Pye, H. O. T.: Semivolatile POA and parameterized total combustion SOA in CMAQv5.2: impacts on source strength and partitioning, Atmos. Chem. Phys., 17, 11107–11133, https://doi.org/10.5194/acp-17-11107-2017, 2017.
Nagai, K. and Kubato, M.: On the volatilization of nitrogen during nitrification in soil under vinyl covered culture (Part 5). On the effects of iron complex salts and treatments with nitrates or chloride on volatilization of nitrous acid, J. Sct. Soil Manure. Jpn., 43, 31–35, 1972.
Nakashima, Y. and Kajii, Y.: Determination of nitrous acid emission factors from a gasoline vehicle using a chassis dynamometer combined with incoherent broadband cavity-enhanced absorption spectroscopy, Sci. Total Environ., 575, 287–293, https://doi.org/10.1016/j.scitotenv.2016.10.050, 2017.
NASA: Korea United States Air Quality Study, NASA [data set], https://doi.org/10.5067/Suborbital/KORUSAQ/DATA01, 2019.
Nie, W., Ding, A. J., Xie, Y. N., Xu, Z., Mao, H., Kerminen, V.-M., Zheng, L. F., Qi, X. M., Huang, X., Yang, X.-Q., Sun, J. N., Herrmann, E., Petäjä, T., Kulmala, M., and Fu, C. B.: Influence of biomass burning plumes on HONO chemistry in eastern China, Atmos. Chem. Phys., 15, 1147–1159, https://doi.org/10.5194/acp-15-1147-2015, 2015.
Oswald, R., Behrendt, T., Ermel, M., Wu, D., Su, H., Cheng, Y., Breuninger, C., Moravek, A., Mougin, E., and Delon, C.: HONO emissions from soil bacteria as a major source of atmospheric reactive nitrogen, Science, 341, 1233–1235, https://doi.org/10.1126/science.1242266, 2013.
Pathak, R. K., Wu, W. S., and Wang, T.: Summertime PM2.5 ionic species in four major cities of China: nitrate formation in an ammonia-deficient atmosphere, Atmos. Chem. Phys., 9, 1711–1722, https://doi.org/10.5194/acp-9-1711-2009, 2009.
Rappenglück, B., Lubertino, G., Alvarez, S., Golovko, J., Czader, B., and Ackermann, L.: Radical precursors and related species from traffic as observed and modeled at an urban highway junction, J. Air Waste Manage., 63, 1270–1286, https://doi.org/10.1080/10962247.2013.822438, 2013.
Reisinger, A. R.: Observations of HNO2 in the polluted winter atmosphere: possible heterogeneous production on aerosols, Atmos. Environ., 34, 3865–3874, https://doi.org/10.1016/S1352-2310(00)00179-5, 2000.
Ren, X., Harder, H., Martinez, M., Lesher, R. L., Oliger, A., Simpas, J. B., Brune, W. H., Schwab, J. J., Demerjian, K. L., and He, Y.: OH and HO2 chemistry in the urban atmosphere of New York City, Atmos. Environ., 37, 3639–3651, https://doi.org/10.1016/S1352-2310(03)00459-X, 2003. .
Romer, P. S., Wooldridge, P. J., Crounse, J. D., Kim, M. J., Wennberg, P. O., Dibb, J. E., Scheuer, E., Blake, D. R., Meinardi, S., and Brosius, A. L.: Constraints on Aerosol Nitrate Photolysis as a Potential Source of HONO and NOx, Environ. Sci. Technol., 52, 13738–13746, https://doi.org/10.1021/acs.est.8b03861, 2018.
Ryu, S. Y., Kim, J. E., Zhuanshi, H., Kim, Y. J., and Kang, G. U.: Chemical composition of post-harvest biomass burning aerosols in Gwangju, Korea, J. Air Waste Manage., 54, 1124–1137, https://doi.org/10.1080/10473289.2004.10471018, 2004.
Sarwar, G., Roselle, S. J., Mathur, R., Appel, W., Dennis, R. L., and Vogel, B.: A comparison of CMAQ HONO predictions with observations from the Northeast Oxidant and Particle Study, Atmos. Environ., 42, 5760–5770, https://doi.org/10.1016/j.atmosenv.2007.12.065, 2008.
Skamarock, C., Klemp, B., Dudhia, J., Gill, O., Barker, D., Duda, G., Huang, X., Wang, W., and Powers, G.: A Description of the Advanced Research WRF Version 3 (No. NCAR/TN-475+STR), University Corporation for Atmospheric Research, https://doi.org/10.5065/D68S4MVH, 2008.
Song, C., Chen, G., Hanna, S., Crawford, J., and Davis, D.: Dispersion and chemical evolution of ship plumes in the marine boundary layer: Investigation of chemistry, J. Geophys. Res.-Atmos., 108, 4143, https://doi.org/10.1029/2002JD002216, 2003.
Stockwell, W. R., Middleton, P., Chang, J. S., and Tang, X.: The second generation regional acid deposition model chemical mechanism for regional air quality modeling, J. Geophys. Res.-Atmos., 95, 16343–16367, https://doi.org/10.1029/JD095iD10p16343, 1990.
Su, H., Cheng, Y. F., Cheng, P., Zhang, Y. H., Dong, S., Zeng, L. M., Wang, X., Slanina, J., Shao, M., and Wiedensohler, A.: Observation of nighttime nitrous acid (HONO) formation at a non-urban site during PRIDE-PRD2004 in China, Atmos. Environ., 42, 6219–6232, https://doi.org/10.1016/j.atmosenv.2008.04.006, 2008.
Svensson, R., Ljungström, E., and Lindqvist, O.: Kinetics of the reaction between nitrogen dioxide and water vapour, Atmos. Environ. (1967), 21, 1529–1539, https://doi.org/10.1016/0004-6981(87)90315-5, 1987.
Tao, M., Chen, L., Wang, Z., Tao, J., and Su, L.: Satellite observation of abnormal yellow haze clouds over East China during summer agricultural burning season, Atmos. Environ., 79, 632–640, https://doi.org/10.1016/j.atmosenv.2013.07.033, 2013.
Tong, S., Hou, S., Zhang, Y., Chu, B., Liu, Y., He, H., Zhao, P., and Ge, M.: Comparisons of measured nitrous acid (HONO) concentrations in a pollution period at urban and suburban Beijing, in autumn of 2014, Sci. China Chem., 58, 1393–1402, https://doi.org/10.1007/s11426-015-5454-2, 2015.
US EPA Office of Research and Development: CMAQv5.1, Zenodo [code], https://doi.org/10.5281/zenodo.1079909, 2015
VandenBoer, T. C., Brown, S. S., Murphy, J. G., Keene, W. C., Young, C. J., Pszenny, A. A. P., Kim, S., Warneke, C., de Gouw, J. A., Maben, J. R., Wagner, N. L., Riedel, T. P., Thornton, J. A., Wolfe, D. E., Dubé, W. P., Öztürk, F., Brock, C. A., Grossberg, N., Lefer, B., Lerner, B., Middlebrook, A. M., and Roberts, J. M.: Understanding the role of the ground surface in HONO vertical structure: High resolution vertical profiles during NACHTT-11, J. Geophys. Res.-Atmos., 118, 10155–10171, https://doi.org/10.1002/jgrd.50721, 2013.
Wang, Y., Huang, D. D., Huang, W., Liu, B., Chen, Q., Huang, R., Gen, M., Mabato, B. R. G., Chan, C. K., and Li, X.: Enhanced nitrite production from the aqueous photolysis of nitrate in the presence of vanillic acid and implications for the roles of light-absorbing organics, Environ. Sci. Technol., 55, 15694–15704, https://doi.org/10.1021/acs.est.1c04642, 2021.
Weber, B., Wu, D., Tamm, A., Ruckteschler, N., Rodriguez-Caballero, E., Steinkamp, J., Meusel, H., Elbert, W., Behrendt, T., and Soergel, M.: Biological soil crusts accelerate the nitrogen cycle through large NO and HONO emissions in drylands, P. Natl. Acad. Sci. USA, 112, 15384–15389, https://doi.org/10.1073/pnas.1515818112, 2015.
Wiedinmyer, C., Quayle, B., Geron, C., Belote, A., McKenzie, D., Zhang, X., O'Neill, S., and Wynne, K. K.: Estimating emissions from fires in North America for air quality modeling, Atmos. Environ., 40, 3419–3432, https://doi.org/10.1016/j.atmosenv.2006.02.010, 2006.
Wiedinmyer, C., Akagi, S. K., Yokelson, R. J., Emmons, L. K., Al-Saadi, J. A., Orlando, J. J., and Soja, A. J.: The Fire INventory from NCAR (FINN): a high resolution global model to estimate the emissions from open burning, Geosci. Model Dev., 4, 625–641, https://doi.org/10.5194/gmd-4-625-2011, 2011.
Wiesen, P., Kleffmann, J., Kurtenbach, R., and Becker, K. H.: Mechanistic study of the heterogeneous conversion of NO2 into HONO and N2O on acid surfaces, Faraday Discuss., 100, 121–127, https://doi.org/10.1039/FD9950000121, 1995.
Woo, J.-H., Choi, K.-C., Kim, H. K., Baek, B. H., Jang, M., Eum, J.-H., Song, C. H., Ma, Y.-I., Sunwoo, Y., and Chang, L.-S.: Development of an anthropogenic emissions processing system for Asia using SMOKE, Atmos. Environ., 58, 5–13, https://doi.org/10.1016/j.atmosenv.2011.10.042, 2012.
Woo, J.-H., Kim, Y., Kim, H.-K., Choi, K.-C., Eum, J.-H., Lee, J.-B., Lim, J.-H., Kim, J., and Seong, M.: Development of the CREATE Inventory in Support of Integrated Climate and Air Quality Modeling for Asia, Sustainability, 12, 7930, https://doi.org/10.3390/su12197930, 2020.
WRF Users Page: WRF Model Users’ Page, WRF Users Page [code], https://doi.org/10.5065/D6MK6B4K, 2024.
Wu, D., Deng, L., Liu, Y., Xi, D., Zou, H., Wang, R., Sha, Z., Pan, Y., Hou, L., and Liu, M.: Comparisons of the effects of different drying methods on soil nitrogen fractions: Insights into emissions of reactive nitrogen gases (HONO and NO), Atmospheric and Oceanic Science Letters, 13, 224–231, https://doi.org/10.1080/16742834.2020.1733388, 2020.
Xu, Z., Wang, T., Wu, J., Xue, L., Chan, J., Zha, Q., Zhou, S., Louie, P. K., and Luk, C. W.: Nitrous acid (HONO) in a polluted subtropical atmosphere: Seasonal variability, direct vehicle emissions and heterogeneous production at ground surface, Atmos. Environ., 106, 100–109, https://doi.org/10.1016/j.atmosenv.2015.01.061, 2015.
Xu, Z., Liu, Y., Nie, W., Sun, P., Chi, X., and Ding, A.: Evaluating the measurement interference of wet rotating-denuder–ion chromatography in measuring atmospheric HONO in a highly polluted area, Atmos. Meas. Tech., 12, 6737–6748, https://doi.org/10.5194/amt-12-6737-2019, 2019.
Xue, C., Ye, C., Kleffmann, J., Zhang, C., Catoire, V., Bao, F., Mellouki, A., Xue, L., Chen, J., Lu, K., Zhao, Y., Liu, H., Guo, Z., and Mu, Y.: Atmospheric measurements at Mt. Tai – Part I: HONO formation and its role in the oxidizing capacity of the upper boundary layer, Atmos. Chem. Phys., 22, 3149–3167, https://doi.org/10.5194/acp-22-3149-2022, 2022.
Ye, C., Gao, H., Zhang, N., and Zhou, X.: Photolysis of nitric acid and nitrate on natural and artificial surfaces, Environ. Sci. Technol., 50, 3530–3536, https://doi.org/10.1021/acs.est.5b05032, 2016.
Ye, C., Zhang, N., Gao, H., and Zhou, X.: Photolysis of Particulate Nitrate as a Source of HONO and NOx, Environ. Sci. Technol., 51, 6849–6856, https://doi.org/10.1021/acs.est.7b00387, 2017.
Yu, C., Wang, Z., Ma, Q., Xue, L., George, C., and Wang, T.: Measurement of heterogeneous uptake of NO2 on inorganic particles, sea water and urban grime, J. Environ. Sci., 106, 124–135, https://doi.org/10.1016/j.jes.2021.01.018, 2021.
Yu, C., Huang, L., Xue, L., Shen, H., Li, Z., Zhao, M., Yang, J., Zhang, Y., Li, H., and Mu, J.: Photoenhanced heterogeneous uptake of NO2 and HONO formation on authentic winter time urban grime, ACS Earth and Space Chemistry, 6, 1960–1968, https://doi.org/10.1021/acsearthspacechem.2c00054, 2022.
Yuan, H., Dai, Y., Xiao, Z., Ji, D., and Shangguan, W.: Reprocessing the MODIS Leaf Area Index products for land surface and climate modelling, Remote Sens. Environ., 115, 1171–1187, https://doi.org/10.1016/j.rse.2011.01.001, 2011.
Zhang, J., An, J., Qu, Y., Liu, X., and Chen, Y.: Impacts of potential HONO sources on the concentrations of oxidants and secondary organic aerosols in the Beijing-Tianjin-Hebei region of China, Sci. Total Environ., 647, 836–852, https://doi.org/10.1016/j.scitotenv.2018.08.030, 2019.
Zhang, J., Lian, C., Wang, W., Ge, M., Guo, Y., Ran, H., Zhang, Y., Zheng, F., Fan, X., Yan, C., Daellenbach, K. R., Liu, Y., Kulmala, M., and An, J.: Amplified role of potential HONO sources in O3 formation in North China Plain during autumn haze aggravating processes, Atmos. Chem. Phys., 22, 3275–3302, https://doi.org/10.5194/acp-22-3275-2022, 2022.
Zhang, L., Wang, T., Zhang, Q., Zheng, J., Xu, Z., and Lv, M.: Potential sources of nitrous acid (HONO) and their impacts on ozone: A WRF-Chem study in a polluted subtropical region, J. Geophys. Res.-Atmos., 121, 3645–3662, https://doi.org/10.1002/2015JD024468, 2016.
Zhang, R., Sarwar, G., Fung, J. C., Lau, A. K., and Zhang, Y.: Examining the impact of nitrous acid chemistry on ozone and PM over the Pearl River Delta Region, Adv. Meteorol., 2012, 140932, https://doi.org/10.1155/2012/140932, 2012.
Zhang, Y., Shao, M., Lin, Y., Luan, S., Mao, N., Chen, W., and Wang, M.: Emission inventory of carbonaceous pollutants from biomass burning in the Pearl River Delta Region, China, Atmos. Environ., 76, 189–199, https://doi.org/10.1016/j.atmosenv.2012.05.055, 2013.
Zhou, X., Zhang, N., TerAvest, M., Tang, D., Hou, J., Bertman, S., Alaghmand, M., Shepson, P. B., Carroll, M. A., and Griffith, S.: Nitric acid photolysis on forest canopy surface as a source for tropospheric nitrous acid, Nat. Geosci., 4, 440–443, https://doi.org/10.1038/ngeo1164, 2011.