the Creative Commons Attribution 4.0 License.
the Creative Commons Attribution 4.0 License.
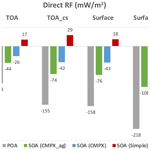
Anthropogenic amplification of biogenic secondary organic aerosol production
Yiqi Zheng
Larry W. Horowitz
Raymond Menzel
David J. Paynter
Vaishali Naik
Jingyi Li
Biogenic secondary organic aerosols (SOAs) contribute to a large fraction of fine aerosols globally, impacting air quality and climate. The formation of biogenic SOA depends on not only emissions of biogenic volatile organic compounds (BVOCs) but also anthropogenic pollutants including primary organic aerosol, sulfur dioxide (SO2), and nitrogen oxides (NOx). However, the anthropogenic impact on biogenic SOA production (AIBS) remains unclear. Here we use the decadal trend and variability in observed organic aerosol (OA) in the southeast US, combined with a global chemistry–climate model, to better constrain AIBS. We show that the reduction in SO2 emissions can only explain 40 % of the decreasing decadal trend of OA in this region, constrained by the low summertime month-to-month variability in surface OA. We hypothesize that the rest of the OA decreasing trend is largely due to a reduction in NOx emissions. By implementing a scheme for monoterpene SOA with enhanced sensitivity to NOx, our model can reproduce the decadal trend and variability in OA in this region. Extending to a centennial scale, our model shows that global SOA production increases by 36 % despite BVOC reductions from the preindustrial period to the present day, largely amplified by AIBS. Our work suggests a strong coupling between anthropogenic and biogenic emissions in biogenic SOA production that is missing from current climate models.
- Article
(2747 KB) - Full-text XML
-
Supplement
(12691 KB) - BibTeX
- EndNote
Terrestrial vegetation emits more than 1 Pg yr−1 of biogenic volatile organic compounds (BVOCs; Guenther et al., 2012), leading to a major source of secondary organic aerosol (SOA) in the atmosphere (Goldstein and Galbally, 2007). SOA exerts significant impacts on climate, air quality, and human welfare (Shrivastava et al., 2017; Pye et al., 2021) but is not well represented in climate models. Global climate models differ largely in simulated SOA burden, variability, and radiative effects (Tsigaridis et al., 2014) due to complexity associated with the emission of precursors, multiphase chemical and physical processes, aging, radiative properties, and other processes (Shrivastava et al., 2017). Many climate models simply scale SOA yield with BVOC precursors (Horowitz et al., 2020; Carslaw et al., 2013; Koch et al., 2011).
The current understanding of biogenic SOA formation has advanced far beyond this simple scaling of BVOC emissions. SOA formation from BVOC oxidation is largely dependent on its oxidants (), and the yields show nonlinear behavior under different NOx conditions (Ng et al., 2017; Presto et al., 2005). One advanced scheme is the volatility basis set (VBS), in which intermediate semivolatile products from the oxidation of BVOCs are grouped into volatility bins and can reversibly condense onto pre-existing organic aerosols (Donahue et al., 2006; Pye et al., 2010). VBS accounts for the dependence of SOA formation on atmospheric oxidants, NOx-dependent chemical regimes, primary organic aerosol (POA) and temperature. Some studies showed that VBS schemes underestimated observations and that photochemical aging schemes with varying complexity may improve simulation results in different regions and seasons (Zheng et al., 2015; Robinson et al., 2007; Oak et al., 2022). Another pathway is through the reactive uptake of smaller molecules onto aqueous aerosols. Several isoprene oxidation products, such as epoxides (IEPOX) (Paulot et al., 2009) and glyoxal (Liggio et al., 2005; Li et al., 2016), though often not directly condensable due to their high equilibrium vapor pressure, can undergo aqueous-phase reactions and oligomerize in the condensed phase. The detailed mechanism is complicated by aerosol acidity, composition, and coating (Shrivastava et al., 2017). These advancements highlight the role of anthropogenic emissions modulating biogenic SOA formation through nitrogen oxides (NOx), SO2, and POA.
One major uncertainty is to what extent anthropogenic emissions modulate biogenic SOA formation. In the southeast US (SEUS), a region largely covered by natural vegetation and also heavily populated, organic aerosol has shown a decreasing trend in the last two decades (Kim et al., 2015; Attwood et al., 2014), likely due to reductions in POA (Blanchard et al., 2016; Ridley et al., 2018; Liu et al., 2023), sulfate and aerosol water (Christiansen et al., 2020; Ridley et al., 2018; Marais et al., 2017; Malm et al., 2017; Blanchard et al., 2016; Liu et al., 2023), and NOx (Zheng et al., 2015; Xu et al., 2015; Pye et al., 2019). Several studies suggest that SO2 largely modulates SOA through the reactive uptake of IEPOX (Pye et al., 2013; Marais et al., 2017), but the acidity-catalyzed sulfate uptake mechanism appears to overestimate the trend of organic aerosol (OA) reduction in the SEUS (Zheng et al., 2020). The role of NOx remains unclear. While SOA yield generally decreases with NOx level due to the fragmentation of large molecules (Kroll and Seinfeld, 2008), recent studies show that NOx can in fact increase SOA production (Zheng et al., 2015; Xu et al., 2015; Pye et al., 2019; Pullinen et al., 2020). The combined effect of NOx, SO2, and POA can be significant (Carlton et al., 2010; Hoyle et al., 2011) but remain unconstrained by ambient observations.
Here we use the decadal trend and variability in observed OA in the southeast US, combined with other observational datasets and a global chemistry–climate model (GFDL AM4.1) (Horowitz et al., 2020), to better constrain the anthropogenic impact on biogenic SOA production (AIBS). We use three schemes (summarized in Table 1 and detailed in Methods) to investigate the AIBS from decadal to centennial timescales.
Table 1Comparison of the SOA schemes used in this study. Further details and discussions are included in Methods.
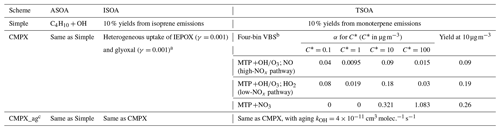
a γ represents uptake coefficients of IEPOX or glyoxal onto aqueous sulfate aerosol. b In the four-bin VBS, monoterpene (MTP) is oxidized by OH, O3, or NO3 to generate four semivolatile surrogate products, which can reversibly partition into pre-existing organic aerosol. C* represents saturation concentration of each semivolatile product and determines the partitioning of these products between the gas and aerosol phase. The mass-based stoichiometric yield coefficients, α, for each parent hydrocarbon and oxidant system are fit with a VBS using C* of 0.1, 1, 10, and 100 µg m−3 (Pye et al., 2010). c In the aging scheme, at every time step, each semivolatile product except for the lowest volatility bin (C* = 0.1 µg m−3) is assumed to be further oxidized by OH with a rate constant of , which reduces its volatility by an order of magnitude.
2.1 GFDL AM4.1
The Geophysical Fluid Dynamics Laboratory's (GFDL) Atmospheric Model version 4.1 (AM4.1) (Horowitz et al., 2020) is a three-dimensional global chemistry–climate model that includes interactive simulation of stratospheric chemistry and tropospheric O3–NOx–CO–VOCs and bulk aerosol chemistry, allowing explicit treatment of aerosol reactive uptake of IEPOX and glyoxal (Li et al., 2016, 2018; Mao et al., 2013). AM4.1 has 49 vertical levels from the surface to 1 Pa (∼ 80 km). We conduct AM4.1 simulations at a horizontal resolution of 1∘ × 1.25∘ latitude by longitude and a main dynamical atmosphere time step of 30 min. Annual varying historical anthropogenic emissions from the preindustrial era to the present day (1849 to 2016) are from the Community Emissions Data System (CEDS) (Hoesly et al., 2018) and the dataset of van Marle et al. (2017), which are developed in support of the Coupled Model Intercomparison Project Phase 6 (CMIP6). Global fire emissions are based on Global Fire Emissions Database version 4 (GFED4), the Fire Modeling Intercomparison Project (FireMIP), visibility observations, and Global Charcoal Database (GCD). Biogenic isoprene and monoterpene emissions are calculated online by the Model of Emissions of Gases and Aerosols from Nature version 2.1 (MEGAN2.1), using empirical functions of plant functional type (PFT)-specific emission basal factors, leaf area index (LAI), temperature, and light. Dependence of soil moisture, O3, and CO2 are neglected due to large uncertainties. LAI values follow an annual cycle of the year 1992, and PFTs are prescribed at the 1992 level. The gas-phase and aerosol chemistry is detailed in Horowitz et al. (2020), in which heterogenous reactive uptakes of HO2, HO2, NO2, N2O5, NO3, SO2, IEPOX, and glyoxal onto aerosol surfaces are included. Dry and wet depositions of gases are described in Paulot et al. (2016). More details can be found in Horowitz et al. (2020) and Dunne et al. (2020). Radiative effects of SOA are calculated assuming SOA is externally mixed from other aerosols (Horowitz et al., 2020), although isoprene SOA (ISOA) is formed through sulfate uptake in the chemistry module.
We perform simulations for the years 1998–2016 for the present day (PD) and 1870–1888 for preindustrial (PI) periods. In each simulation, the first 2 years are discarded as spin-up. The remaining 17 years are used for analysis. The PD simulations are nudged with reanalysis winds from NCEP-DOE Reanalysis 2. The PI simulations are free running with no nudging. All simulations are driven by observed or reconstructed sea surface temperature and sea ice (Horowitz et al., 2020). In the two PI simulations, we scale up the isoprene and monoterpene emission basal factors by 35 % to account for the higher natural vegetation cover in the preindustrial period than today, equivalent to a 26 % reduction in natural vegetation cover from PI to PD (Unger, 2014). We apply this single scaling factor to BVOC emission basal factors as an idealized study instead of using reconstructed land cover type and LAI to avoid uncertainties in historical vegetation reconstructions.
2.2 Modeling of SOA formation
In GFDL AM4.1, SOA is composed of anthropogenic SOA (ASOA), ISOA, and monoterpene SOA (TSOA). ASOA is formed through the oxidation of C4H10 by OH in all simulations. In the default “Simple” scheme, ISOA and TSOA are assumed to be produced with a pseudo-emission equivalent to a 10 % per carbon yield of the interactively calculated isoprene and monoterpene emissions, respectively. This 10 % yield in the Simple scheme is consistent with previous model versions GFDL AM3 and AM4.0 and within the range of estimates suggested by other studies. For example, a chemical transport model GEOS-Chem assumed a 3 % yield for isoprene and a 10 % yield for monoterpene emissions (Pye et al., 2010; Pai et al., 2020). However, a study using a more complex scheme suggested a SOA yield from isoprene of 13 % per carbon (Bates and Jacob, 2019).
We implement a complex (CMPX) SOA scheme in GFDL AM4.1, in which isoprene and monoterpenes are oxidized by OH, O3, and NO3 to form ISOA and TSOA. ISOA is computed through the aqueous-phase uptake of IEPOX and glyoxal onto sulfate aerosol. The uptake coefficients for IEPOX and glyoxal are set to 0.001, different than previous studies using higher or acidity-dependent uptake coefficients (Marais et al., 2016; Lin et al., 2014a). This is supported by the OA month-to-month variability (MMV) in summer and its decadal trend over SEUS, as a previous model with acidity-dependent uptake coefficients shows MMV that is too high and too much OA in the early 2000s (Zheng et al., 2020). The uptake rate coefficients can be even lower due to the effect of aerosol-phase state (Y. Zhang et al., 2018). To avoid uncertainties associated with aerosol acidity, relative humidity, and coating effect, we here apply an uptake coefficient of 0.001 for both IEPOX and glyoxal. This leads to good agreement between our model and observations in SEUS on both OA magnitude and summertime MMV (Figs. 1 and S1 and S2 in the Supplement).
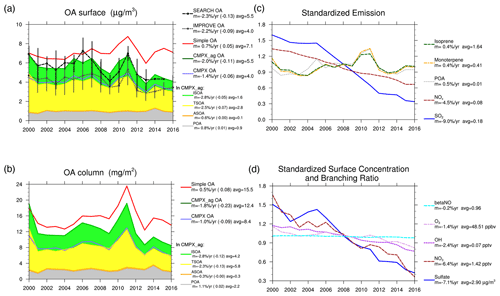
Figure 1Summertime (June–July–August) values averaged in the southeast US (29–37∘ N, 74–96∘ W) in 2000–2016. (a) Surface concentrations of OA from the two measurement networks, IMPROVE and SEARCH, and the Simple, CMPX, and CMPX_ag simulations. (b) Column concentrations of OA. In panels (a) and (b), color shades represent OA components from the CMPX_ag scheme. (c) Standardized emissions of isoprene, monoterpenes, POA, NOx, and SO2. (d) Standardized surface concentrations of gases O3, OH, NO3, sulfate aerosol, and branching ratio. In panels (c) and (d), each variable has been divided by its 17-year average for standardization. In attached text, “m” represents the 2000–2016 relative trend (with units of % yr−1), numbers in parentheses in panels (a) and (b) represent trends (with units of or ), and “avg” represents the 17-year average (with units of µg m−3 in panel a, mg m−2 in panel b, in panel c, and different units shown in panel d). ISOA, TSOA, and ASOA refer to isoprene, monoterpene, and anthropogenic SOA, respectively.
In the updated CMPX scheme, TSOA is calculated by a four-product volatility basis set (VBS) summarized in Table 1. Organic peroxy radicals (RO2) formed from OH- and O3-initiated oxidation of monoterpene can react with NO under high-NOx conditions and with HO2 under low-NOx conditions. The low-NOx pathway (RO2 + HO2) has higher yields for SOA than the high-NOx pathway (RO2 + NO) (Pye et al., 2010; Zheng et al., 2015). The branching ratio between the low- versus high-NOx pathways is defined as
where and represent the reaction rate coefficients of RO2 + NO and RO2 + HO2, respectively. At nighttime, the NO3-initiated oxidation of monoterpenes has a high yield of organic nitrates and contributes a significant amount of SOA (Ng et al., 2017). The surrogate TSOA products are implemented in AM4.1, and the implementation does not count reductions in OH, O3, and NO3 in addition to the original gas-phase monoterpene oxidation chemistry. There is little difference in the concentration of these gases between the CMPX and Simple simulations. The gas-phase chemistry has been validated in Horowitz et al. (2020) and in Fig. S3, in which we show that summertime surface O3 and NO2 in SEUS reproduce their observed decreasing trend well.
Such semi-empirical partitioning-based VBS schemes have been widely used in chemistry–climate models and Earth system models (e.g., Zheng et al., 2015; Tilmes et al., 2019). Recent research has shown that these schemes may underestimate SOA formation without considering further aging processes, such as oligomerization in the organic-phase and aqueous-phase reactions (Hu et al., 2013; Yu et al., 2021; Oak et al., 2022 and references therein). One major recently identified explicit mechanism is the formation of monoterpene-derived highly oxygenated molecules (HOMs) through the auto-oxidation of peroxy radicals (Crounse et al., 2013; Ehn et al., 2014; Pye et al., 2019). Mechanistic schemes of monoterpene-derived SOA have been developed with varying complexity at a cost of more tracers and reactions (Pye et al., 2019; Berkemeier et al., 2020; Pullinen et al., 2020; Yu et al., 2021), which may not be ready yet for a global climate model as part of an Earth system model considering large uncertainties associated with multi-phase processes and increased computational cost. In this study, in addition to the semi-empirical VBS scheme, we implement a simplified photochemical aging parameterization to the semivolatile oxidation products of terpenes in the CMPX scheme (CMPX_ag) (Zheng et al., 2015) to account for the decrease in volatility as a result of OH oxidation (Donahue et al., 2012). We apply a rate constant of kOH = 4 × 10−11 (Robinson et al., 2007), in line with recent estimates of 2–4 × 10−11 for terpene SOA (Donahue et al., 2012; Isaacman-VanWertz et al., 2018). Including the aging scheme in CMPX does not increase computational cost notably. This simplified aging scheme does not explicitly represent up-to-date knowledge of SOA chemistry but similarly increases the SOA burden, as well as the sensitivity of SOA to NOx, improving the model underestimate of SOA by the VBS scheme. The details of the Simple, CMPX, and CMPX_ag schemes are summarized in Table 1.
2.3 Observational datasets
For model evaluation we use long-term measurements of organic aerosol (OA) or organic carbon (OC). We do not use explicit SOA tracers in this study because it is not suitable to use short-term observations to validate simulated results from a chemistry–climate model like AM4.1, in which meteorology is not specified offline by reanalysis data but is free running in the dynamic core. However, the long-term (covering at least months to years) measurement of explicit SOA species is not available. We use filter measurements of organic carbon from two surface aerosol measurement networks in the US: IMPROVE (Interagency Monitoring of Protected Visual Environments) (Solomon et al., 2014) and SEARCH (Southeastern Aerosol Research and Characterization) (Edgerton et al., 2005). IMPROVE and SEARCH report daily average organic carbon measurements every 3 d. We focus on SEUS which is both heavily vegetated and populated. We select 20 IMPROVE sites and 3 rural SEARCH sites within the SEUS region (29–37∘ N, 74–96∘ W) and calculate monthly averages of OA across these sites for each network (see site locations in Fig. S4). We apply a seasonal-dependent ratio to convert organic carbon to OA mass: 2.2 in June–July–August, 1.8 in December–January–February, and 1.9 in other months (Philip et al., 2014). In Sect. 3.1, we calculate the absolute trend of a variable as the slope of the regression line of the variable's value versus time, and we calculate the relative trend (represented by “m” in Fig. 1) as the absolute trend divided by the variable's 2000–2016 average.
We also compare modeling results to OA measurements by the Aerosol Chemical Speciation Monitor (ACSM). We select three European sites from the ACTRIS (the Aerosol, Clouds, and Trace Gases Research Infrastructure) network (Crenn et al., 2015) – Hyytiälä (Finland), Puy de Dôme (France), and Birkenes II (Norway) – and two sites from the ARM (Atmospheric Radiation Measurement) network (Uin et al., 2019) – Southern Great Plains (US) and Manacapuru, Amazonia (Brazil). These sites are covered by natural vegetation and have more than a year's worth of data available. We average the original hourly OA measurements to monthly mean data for these sites to compare with modeling results.
3.1 Decadal trend of summertime OA in SEUS and its variability
The SEUS is a region heavily influenced by both biogenic and anthropogenic emissions (Mao et al., 2018). In the last two decades, organic aerosol shows a decreasing trend, resulting from reductions in anthropogenic pollutants including SO2 and NOx (Marais et al., 2017; Blanchard et al., 2016; Ridley et al., 2018). The CMPX and CMPX_ag schemes successfully reproduce the summertime surface OA concentrations measured from the IMPROVE and SEARCH networks at 4 and 5.5 µg m−3, respectively (Fig. 1a). The Simple scheme has a significant overestimate (∼ 7 µg m−3).
We first examine the simulated decadal OA trend in the SEUS against filter-based measurements from the IMPROVE and SEARCH networks. From 2000 to 2016, the measured summer OA declines by −0.13 from SEARCH and by −0.09 from IMPROVE, both at a reduction rate of −2.3 % yr−1 (Fig. 1a). This decreasing trend is reproduced well by the CMPX_ag simulation with a decrease of −0.11 µg m−3 (−2.0 % yr−1) and a smaller decrease of −0.06 µg m−3 (−1.4 % yr−1) with the CMPX scheme. Considering the varying reduction trends among different sites (Fig. S4), both the CMPX and CMPX_ag schemes reproduce the SEUS OA trend well in general. In contrast, the Simple scheme shows a slight increase (+0.7 % yr−1) in surface OA due to the lack of AIBS and little change in POA in 2000–2016 in this region (Fig. 1c).
We further examine the summertime month-to-month variability in surface OA. We find that both CMPX_ag and CMPX schemes can reproduce the low summertime month-to-month variability in surface OA well (standard deviation smaller than 2 µg m−3) constrained by IMPROVE and SEARCH measurements (Fig. S2), using fixed uptake coefficients (γ=0.001) of IEPOX and glyoxal. This summertime month-to-month variability was found to be too high (standard deviation up to 5 µg m−3) in the early 2000s using an acidity-dependent IEPOX reactive uptake scheme (Marais et al., 2016, 2017), pointing to additional species besides SO2 driving the decreasing OA trend.
One unique feature of the CMPX_ag simulation is the dominance of TSOA (Fig. 1), mainly through enhanced sensitivity of TSOA production to NOx. Such dominance of TSOA in this region is also supported by recent field observations (Xu et al., 2018; H. Zhang et al., 2018). We find TSOA contributes 60 % of the surface OA trend in the CMPX_ag scheme, mainly through NOx reduction. The NO3-initiated pathway contributes to the majority of surface TSOA decrease (Fig. S5), resulting from the rapid decrease in NO3 (Fig. 1d) (Boyd et al., 2017; Rollins et al., 2012). Compared to the CMPX scheme, the dominant contribution of TSOA is largely due to the OH aging effect, which amplifies the SOA yield from all monoterpene oxidation channels. As a result, we find that NOx reduction accounts for 60 % of the OA decrease in SEUS. This enhanced sensitivity to NOx resonates with recent developments on monoterpene-derived highly oxygenated organic molecules or auto-oxidation (Pye et al., 2019), highlighting the importance of NOx in AIBS.
ISOA contributes 40 % of the surface OA trend in the CMPX_ag scheme, mainly through SO2 reduction. The decrease in surface ISOA, at −0.05 , is associated with the strong reduction in sulfate (−7 % yr−1). The rapidly decreasing sulfate, NOx, and O3 in the model are consistent with observations over the SEUS (Fig. S3) and previous studies (Zheng et al., 2020; Wells et al., 2021; Simon et al., 2015). In contrast to Marais et al. (2017), we find that this nondominant role of ISOA brings the model into much better agreement with observations, especially on the low summertime month-to-month variability in surface OA (standard deviation smaller than 2 µg m−3) constrained by IMPROVE and SEARCH measurements (Fig. S2) (Zheng et al., 2020). The observed summertime month-to-month variability also implies a weaker dependence of OA on sulfate aerosols in this region than that shown in Marais et al. (2017), highlighting the importance of TSOA.
We find a similar trend of summer OA column concentration to the surface OA trend in the model. The CMPX_ag simulation suggests a decreasing trend in summer OA column concentration, driven by both TSOA (−0.13 ) and ISOA (−0.12 ) (Fig. 1b). Similar to the surface, the aging effect increases the column production of TSOA in CMPX_ag and its sensitivity to changes in NOx compared with the CMPX scheme.
3.2 Present-day OA in vegetated regions and global budget
We further evaluate the modeled surface OA against measurements by the Aerosol Chemical Speciation Monitor (ACSM) in other vegetated regions in the Amazon, Europe, and US (Fig. 2). In the Amazon region, the CMPX_ag scheme successfully reproduces the high surface OA concentration from August to November and low OA in other months (Fig. 2c). The Simple scheme greatly overestimates surface OA in all seasons because of its high SOA yield (10 %) from isoprene emissions. The CMPX scheme reproduces the low OA concentrations from January to July well but only predicts half of the observed OA in months with high OA concentrations. In the three European sites from the ACTRIS network (Fig. 2d–f), all model simulations underestimate measured OA. One possible reason is uncertainties associated with BVOC emissions and biogenic SOA. Jiang et al. (2019) showed that MEGAN2.1 overestimates isoprene emissions but underestimates monoterpene emissions in Europe by a factor of 3. At the US Southern Great Plains site from the ARM network (Fig. 2b), the CMPX_ag and CMPX schemes successfully capture the measured OA seasonal variation but underestimate OA magnitude. In the SEUS compared to filter measurements (Fig. 2a), all simulations show lower OA in winter than observations, likely due to an underestimate of wintertime emissions of POA (Tsigaridis et al., 2014; Liu et al., 2021). In general, the updated CMPX_ag and CMPX schemes agree well with observations in the Amazon and US where biogenic emissions are high. The good performance of the CMPX_ag scheme in the Amazon, better than the CMPX scheme, gives us confidence that the traditional VBS in the CMPX scheme may underestimate the contribution of TSOA and its sensitivity to NOx.
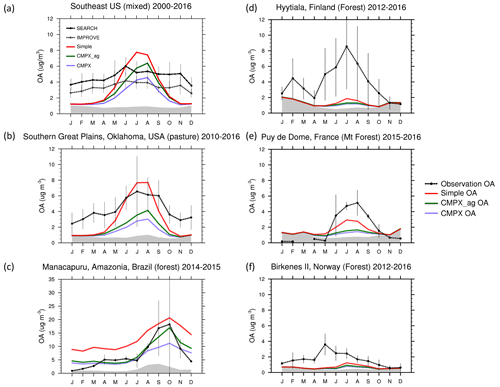
Figure 2Seasonal cycle of surface OA concentration. In panel (a), dashed black and grey lines represent filter measurements of OA from the SEARCH and IMPROVE networks. In other panels, dashed black lines represent ACSM measurements of OA from the ARM network in panels (b) and (c) and from the ACTRIS network in panels (d)–(f). Grey area represents POA.
Globally, the SOA burdens from the Simple, CMPX, and CMPX_ag schemes are 0.99, 0.50, and 1.05 Tg, respectively, and their SOA production rates are 82, 40, and 69 Tg yr−1 (Fig. 3), in agreement with other global modeling studies. The AeroCom phase II (AeroComII) model intercomparison summarizes a median SOA source of 51 Tg yr−1 with a range between 16 to 121 Tg yr−1 (Tsigaridis et al., 2014), although top-down methods indicate the SOA source could be up to 50–380 Tg yr−1 (Spracklen et al., 2011). Uncertainties associated with BVOC emissions contribute to the widespread SOA estimate by global models. In GFDL AM4.1, annual isoprene and monoterpene emissions are computed to be 505 ± 14 and 137 ± 5 Tg yr−1, respectively (Fig. 3), in line with previous estimates (Guenther et al., 2012).
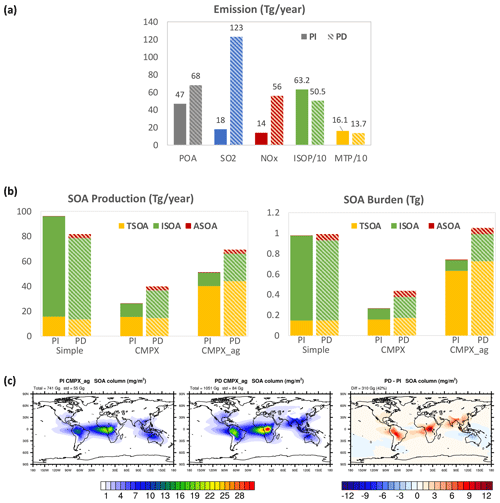
Figure 3(a) Emissions (Tg yr−1) of POA, SO2, NOx, isoprene (ISOP), and monoterpenes (MTP). ISOP and MTP emissions have been divided by 10. (b) Simulated SOA global production (Tg yr−1) and burden (Tg). (c) Simulated SOA column concentrations (mg m−2) at PI and PD and their difference in the CMPX_ag scheme.
Detailed SOA budgets for the three schemes are summarized in Table 2. The CMPX and CMPX_ag schemes have much less ISOA than the Simple scheme as the latter has high pseudo emissions of isoprene SOA, which is 10 % in GFDL AM4.1 as compared to 3 % used in other models like GEOS-Chem (Pai et al., 2020; Henze and Seinfeld, 2006). ISOA (22.2 Tg yr−1) and TSOA (14.4 Tg yr−1) in the CMPX scheme are consistent with previous estimate by GEOS-Chem (Pai et al., 2020; Zheng et al., 2020). The CMPX_ag scheme has higher TSOA (44 Tg yr−1) than CMPX and Simple due to the aging effect of semivolatile oxidation products from terpenes (Fig. 3) and is close to the high end of the estimate (12.7–40 Tg yr−1) by AeroComII (Tsigaridis et al., 2014). ASOA is often neglected by global models despite an estimate of 13.5 Tg yr−1 suggesting ASOA as a non-negligible source (Tsigaridis et al., 2014). In GFDL AM4.1, ASOA (3.3 Tg yr−1) only considers oxidation of C4H10, which does not represent all ASOAs well and warrants further research.
Table 2Annual mean budget of POA and SOA in all simulations. Results are averaged over 1872–1888 for preindustrial and 2000–2016 for present-day simulations. SOA includes ASOA (anthropogenic SOA), ISOA (isoprene SOA), and TSOA (monoterpene SOA).
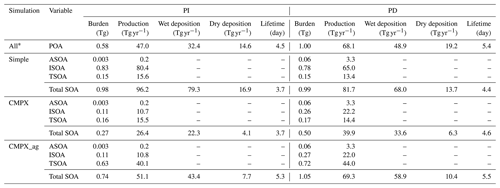
∗ For POA budget, the differences between different schemes are negligible.
3.3 Centennial change in biogenic SOA and direct radiative forcing
We now extend our analysis of AIBS from the decadal scale to the centennial scale. To represent the higher natural vegetation cover during PI, we scale up isoprene and monoterpene emission basal factors in the PI simulations by 35 %, equivalent to a 26 % reduction in natural vegetation cover from PI to PD (Unger, 2014). This simple scaling should be considered an idealized study to avoid uncertainties associated with historical vegetation reconstruction and the complex role of CO2 including both fertilization and inhibition effects. From the 1870s to 2000s, the simulated isoprene emissions decrease from 632 ± 15 to 505 ± 14 Tg yr−1 (−20 %) and monoterpene emissions decrease from 161 ± 5 to 137 ± 5 Tg yr−1 (−15 %) (Fig. 3a, maps in Fig. S6), consistent with previous studies (Heald and Spracklen, 2015).
Despite the reduction in BVOC emissions from PI to PD, we show a significant increase in biogenic SOA (Fig. 3b, maps in Figs. S7 and S8), resulting from an increase in anthropogenic emissions amplified by AIBS. With an increase of 1.4, 7, and 4 for emissions of POA, SO2, and NOx, total SOA production increases by 36 % and its burden increases by 42 % (in the CMPX_ag scheme). ASOA, ISOA, and TSOA contribute 17 %, 62 %, and 21 % to the changes in total SOA production, respectively. In contrast, the Simple scheme shows a decrease in SOA production following the reduction in BVOC emissions. The large increase in SOA from PI to PD differs from previous estimates (Spracklen et al., 2011; Heald and Spracklen, 2015; Zhu et al., 2019; Scott et al., 2017; Lin et al., 2014b; Heald and Geddes, 2016; Hoyle et al., 2009), largely due to AIBS constrained by observations.
The total PI-to-PD SOA rise is largely dominated by ISOA (62 %), resulting from the strong increase in anthropogenic SO2 emissions and uptake of IEPOX and glyoxal onto sulfate aerosols. The global burden of sulfate aerosol has doubled from 0.7 Tg at PI to 1.6 Tg at PD, with large increase over the tropics, SEUS, and Eurasia (Fig. S9). The increase in TSOA is due to both increased NOx and POA emissions. In contrast to the decadal trend where βNO barely changes, the PI-to-PD increase in TSOA due to the change in NOx is suppressed by the shift in βNO. The branching ratio βNO increases from a global average of 0.32 at PI to 0.61 at PD (Fig. S10), indicating a shift from low-NOx pathway (higher yields) to high-NOx pathway (lower yields) for the OH- and O3-initiated oxidation. These competing effects lead to a net +10 % change in TSOA production and a +14 % increase in burden from PI to PD. The PI-to-PD change in TSOA in the CMPX scheme is small (−7 % in production and +6 % in burden). Increased POA provides more organic mass for monoterpene oxidation products to condense on, especially in central Africa and central South America (Fig. S9).
The large increase in biogenic SOA leads to a cooling direct radiative forcing (DRF) from PI to PD, opposed to the warming suggested by the Simple scheme. DRF is usually defined as the difference between PI and PD direct radiative fluxes at top of atmosphere under all-sky conditions. We show in Fig. 4 the global instantaneous DRF at top of atmosphere of −(26–44) mW m−2, comparable to that of POA (−98 mW m−2). In contrast, the Simple scheme shows a warming DRF of +17 mW m−2, largely due to the lack of AIBS. The DRF of SOA in the updated schemes resides within reported AeroComII estimates, which range from −210 to −10 mW m−2, with a mean value of −60 mW m−2 and a median value of −20 mW m−2 (Myhre et al., 2013). Due to this increase in SOA burden, our results may also imply a large indirect radiative forcing from biogenic SOA that is missing from previous work (Carslaw et al., 2013).
Our work suggests a strong coupling between anthropogenic and biogenic emissions in biogenic SOA production. Constrained by observations in SEUS, we show that the summertime OA decreasing trend is likely driven by a reduction in both NOx and SO2 emissions through TSOA and ISOA. First, in a previous study (Zheng et al., 2020) we prove that the scheme of acidity-catalyzed aqueous ISOA formation (Marais et al., 2016) strongly overestimates summertime month-to-month variability in surface OA; therefore in this study we use fixed uptake coefficients for isoprene oxidation products to avoid uncertainties associated with acidity, relative humidity, and coating effect. Second, both the CMPX and CMPX_ag schemes reproduce the observed OA magnitude and the decadal trend in SEUS, in which SO2 alone cannot explain this trend. The CMPX_ag scheme shows a faster OA decrease and agrees better with long-term filter measurements, which is largely driven by NOx (60 %). Third, the CMPX_ag scheme successfully reproduces the observed OA magnitude and seasonal cycle in the Amazon, outperforming the CMPX and Simple schemes. Our results point to the important role of NOx in modulating biogenic SOA, in line with recent understanding of auto-oxidation (Crounse et al., 2013; Ehn et al., 2014; Pye et al., 2019), although further studies are warranted. For example, the CMPX_ag scheme with a simplified aging parameterization does not mechanistically represent the most up-to-date understanding of HOMs and organic nitrates (Takeuchi and Ng, 2019; Berkemeier et al., 2020; Pullinen et al., 2020; Yu et al., 2021). Long-term measurements of ISOA and TSOA tracers across different regions and seasons are needed to develop future mechanistic SOA schemes that are suited for global climate models with minimal computational cost. In this study, the success of the updated schemes in capturing the observed OA trend and month-to-month variability provides confidence in model simulations over longer timescales.
At centennial scales, atmospheric SOA mass increases significantly from PI to PD despite reductions in BVOC emissions, posing a top-of atmosphere instantaneous radiative forcing of −(26–44) mW m−2. ISOA dominates the total SOA change as a result of a significant rise in global sulfate aerosol from PI to PD, especially in the fast-developing regions like Africa, the Middle East, India, and China. POA increases greatly in central Africa and central South America, as well as India and eastern China, which enhances TSOA production. The significant increase in SOA due to AIBS in these regions poses new challenges to meet the World Health Organization's recommendation on annual fine particulate matter exposure (5 µg m−3) (Pai et al., 2022). Under future scenarios with reduced emissions of SO2, NOx, and POA, the AIBS may indicate larger reductions in SOA than current model predictions, but their relative importance cannot be linearly extrapolated based on PI and PD simulations. Model simulations with future emission scenarios are needed, which is beyond the scope of this study.
The updated SOA scheme in GFDL AM4.1 shows an advance in representing vegetation–chemistry–climate interactions over the default model which assumes fixed yields of SOA from biogenic hydrocarbons, although a variety of uncertainties still exist in the evaluation of SOA and its climate impact. First, the model likely underestimates wintertime POA in the US, total OA in Europe, and anthropogenic SOA globally. Second, the model does not consider absorbing SOA or brown carbon which could form from biomass burning and aging (Tsigaridis and Kanakidou, 2018). The model applies the same optical parameters for all SOAs as hydrophilic POAs. Third, other than the uncertainties discussed above about acidity-dependency, the formation of HOMs and organic nitrates, other properties that influence the multiphase growth of SOA, including coating and viscosity, is also not implemented in our model (Shrivastava et al., 2017). Finally, the model does not consider the nucleation of extremely low-volatility compounds from BVOC oxidation, which may increase SOA in pristine environments in the preindustrial period, thus reducing the PI-to-PD radiative forcing of SOA (Gordon et al., 2016; Zhu et al., 2019). These uncertainties warrant further research in studies on anthropogenic-influenced SOA in climate models.
Model outputs are available at https://doi.org/10.6084/m9.figshare.21493986.v1 (Zheng, 2022). Other datasets are available at https://doi.org/10.6084/m9.figshare.23894370.v1 (Zheng, 2023).
The supplement related to this article is available online at: https://doi.org/10.5194/acp-23-8993-2023-supplement.
Conceptualization: YZ, JM. Methodology: YZ, LWH, RM, DJP, VN, JM. Investigation: YZ, LWH, JM. Writing – original draft: YZ, JM. Writing – review and editing: YZ, LWH, RM, DJP, VN, JL, JM.
The contact author has declared that none of the authors has any competing interests.
Publisher's note: Copernicus Publications remains neutral with regard to jurisdictional claims in published maps and institutional affiliations.
We acknowledge Fabien Paulot and Songmiao Fan for internal GFDL review and support from GFDL's Model Development Team, Modeling Systems Division, Operations group, and the RDHPCS supercomputing resources. We also acknowledge the Electric Power Research Institute (EPRI) and Southern Company for their support with the SEARCH network and Atmospheric Research and Analysis, Inc; the US Environmental Protection Agency (EPA) for their support with the IMPROVE network and Air Quality System; and the Atmospheric Radiation Measurement (ARM) user facility, a U.S. Department of Energy (DOE) Office of Science user facility managed by the Biological and Environmental Research Program.
This research has been supported by NOAA (grant no. NA18OAR4310114) and NASA (grant no. 80NSSC21K0428). The ACTRIS network is supported by the European Union's Horizon 2020 research and innovation program (grant agreement no. 654109).
This paper was edited by Veli-Matti Kerminen and reviewed by three anonymous referees.
Attwood, A. R., Washenfelder, R. A., Brock, C. A., Hu, W., Baumann, K., Campuzano-Jost, P., Day, D. A., Edgerton, E. S., Murphy, D. M., Palm, B. B., McComiskey, A., Wagner, N. L., De Sá, S. S., Ortega, A., Martin, S. T., Jimenez, J. L., and Brown, S. S.: Trends in sulfate and organic aerosol mass in the Southeast U.S.: Impact on aerosol optical depth and radiative forcing, Geophys. Res. Lett., 41, 7701–7709, https://doi.org/10.1002/2014GL061669, 2014.
Bates, K. H. and Jacob, D. J.: A new model mechanism for atmospheric oxidation of isoprene: global effects on oxidants, nitrogen oxides, organic products, and secondary organic aerosol, Atmos. Chem. Phys., 19, 9613–9640, https://doi.org/10.5194/acp-19-9613-2019, 2019.
Berkemeier, T., Takeuchi, M., Eris, G., and Ng, N. L.: Kinetic modeling of formation and evaporation of secondary organic aerosol from NO3 oxidation of pure and mixed monoterpenes, Atmos. Chem. Phys., 20, 15513–15535, https://doi.org/10.5194/acp-20-15513-2020, 2020.
Blanchard, C. L., Hidy, G. M., Shaw, S., Baumann, K., and Edgerton, E. S.: Effects of emission reductions on organic aerosol in the southeastern United States, Atmos. Chem. Phys., 16, 215–238, https://doi.org/10.5194/acp-16-215-2016, 2016.
Boyd, C. M., Nah, T., Xu, L., Berkemeier, T., and Ng, N. L.: Secondary Organic Aerosol (SOA) from Nitrate Radical Oxidation of Monoterpenes: Effects of Temperature, Dilution, and Humidity on Aerosol Formation, Mixing, and Evaporation, Environ. Sci. Technol., 51, 7831–7841, https://doi.org/10.1021/acs.est.7b01460, 2017.
Carlton, A. G., Pinder, R. W., Bhave, P. V., and Pouliot, G. A.: To What Extent Can Biogenic SOA be Controlled?, Environ. Sci. Technol., 44, 3376–3380, https://doi.org/10.1021/es903506b, 2010.
Carslaw, K. S., Lee, L. A., Reddington, C. L., Pringle, K. J., Rap, A., Forster, P. M., Mann, G. W., Spracklen, D. V., Woodhouse, M. T., Regayre, L. A., and Pierce, J. R.: Large contribution of natural aerosols to uncertainty in indirect forcing, Nature, 503, 67–71, https://doi.org/10.1038/nature12674, 2013.
Christiansen, A. E., Carlton, A. G., and Porter, W. C.: Changing Nature of Organic Carbon over the United States, Environ. Sci. Technol., 54, 10524–10532, https://doi.org/10.1021/acs.est.0c02225, 2020.
Crenn, V., Sciare, J., Croteau, P. L., Verlhac, S., Fröhlich, R., Belis, C. A., Aas, W., Äijälä, M., Alastuey, A., Artiñano, B., Baisnée, D., Bonnaire, N., Bressi, M., Canagaratna, M., Canonaco, F., Carbone, C., Cavalli, F., Coz, E., Cubison, M. J., Esser-Gietl, J. K., Green, D. C., Gros, V., Heikkinen, L., Herrmann, H., Lunder, C., Minguillón, M. C., Močnik, G., O'Dowd, C. D., Ovadnevaite, J., Petit, J.-E., Petralia, E., Poulain, L., Priestman, M., Riffault, V., Ripoll, A., Sarda-Estève, R., Slowik, J. G., Setyan, A., Wiedensohler, A., Baltensperger, U., Prévôt, A. S. H., Jayne, J. T., and Favez, O.: ACTRIS ACSM intercomparison – Part 1: Reproducibility of concentration and fragment results from 13 individual Quadrupole Aerosol Chemical Speciation Monitors (Q-ACSM) and consistency with co-located instruments, Atmos. Meas. Tech., 8, 5063–5087, https://doi.org/10.5194/amt-8-5063-2015, 2015.
Crounse, J. D., Nielsen, L. B., Jørgensen, S., Kjaergaard, H. G., and Wennberg, P. O.: Autoxidation of Organic Compounds in the Atmosphere, J. Phys. Chem. Lett., 4, 3513–3520, 2013.
Donahue, N. M., Robinson, A. L., Stanier, C. O., and Pandis, S. N.: Coupled Partitioning, Dilution, and Chemical Aging of Semivolatile Organics, Environ. Sci. Technol., 40, 2635–2643, https://doi.org/10.1021/es052297c, 2006.
Donahue, N. M., Henry, K. M., Mentel, T. F., Kiendler-Scharr, A., Spindler, C., Bohn, B., Brauers, T., Dorn, H. P., Fuchs, H., Tillmann, R., Wahner, A., Saathoff, H., Naumann, K.-H., Möhler, O., Leisner, T., Müller, L., Reinnig, M.-C., Hoffmann, T., Salo, K., Hallquist, M., Frosch, M., Bilde, M., Tritscher, T., Barmet, P., Praplan, A. P., DeCarlo, P. F., Dommen, J., Prévôt, A. S. H., and Baltensperger, U.: Aging of biogenic secondary organic aerosol via gas-phase OH radical reactions, P. Natl. Acad. Sci. USA, 109, 13503–13508, https://doi.org/10.1073/pnas.1115186109, 2012.
Dunne, J. P., Horowitz, L. W., Adcroft, A. J., Ginoux, P., Held, I. M., John, J. G., Krasting, J. P., Malyshev, S., Naik, V., Paulot, F., Shevliakova, E., Stock, C. A., Zadeh, N., Balaji, V., Blanton, C., Dunne, K. A., Dupuis, C., Durachta, J., Dussin, R., Gauthier, P. P. G., Griffies, S. M., Guo, H., Hallberg, R. W., Harrison, M., He, J., Hurlin, W., McHugh, C., Menzel, R., Milly, P. C. D., Nikonov, S., Paynter, D. J., Ploshay, J., Radhakrishnan, A., Rand, K., Reichl, B. G., Robinson, T., Schwarzkopf, D. M., Sentman, L. T., Underwood, S., Vahlenkamp, H., Winton, M., Wittenberg, A. T., Wyman, B., Zeng, Y., and Zhao, M.: The GFDL Earth System Model Version 4.1 (GFDL-ESM 4.1): Overall coupled model description and simulation characteristics, J. Adv. Model. Earth Sy., 12, e2019MS002015, https://doi.org/10.1029/2019MS002015, 2020.
Edgerton, E. S., Hartsell, B. E., Saylor, R. D., Jansen, J. J., Hansen, D. A., and Hidy, G. M.: The Southeastern Aerosol Research and Characterization Study: Part II. Filter-Based Measurements of Fine and Coarse Particulate Matter Mass and Composition, J. Air Waste Manage., 55, 1527–1542, https://doi.org/10.1080/10473289.2005.10464744, 2005.
Ehn, M., Thornton, J. A., Kleist, E., Sipilä, M., Junninen, H., Pullinen, I., Springer, M., Rubach, F., Tillmann, R., Lee, B., Lopez- Hilfiker, F., Andres, S., Acir, I.-H., Rissanen, M., Jokinen, T., Schobesberger, S., Kangasluoma, J., Kontkanen, J., Nieminen, T., Kurtén, T., Nielsen, L. B., Jørgensen, S., Kjaergaard, H. G., Canagaratna, M., Maso, M. D., Berndt, T., Petäjä, T., Wahner, A., Kerminen, V.-M., Kulmala, M., Worsnop, D. R., Wildt, J., and Mentel, T. F.: A large source of low-volatility secondary organic aerosol, Nature, 506, 476–479, 2014.
Goldstein, A. H. and Galbally, I. E.: Known and unexplored organic constituents in the Earth's atmosphere, Environ. Sci. Technol., 41, 1514–1521, https://doi.org/10.1021/es072476p, 2007.
Gordon, H., Sengupta, K., Rap, A., et al.: Reduced anthropogenic aerosol radiative forcing caused by biogenic new particle formation, P. Natl. Acad. Sci. USA, 113, 12053–12058, https://doi.org/10.1073/pnas.1602360113, 2016.
Guenther, A. B., Jiang, X., Heald, C. L., Sakulyanontvittaya, T., Duhl, T., Emmons, L. K., and Wang, X.: The Model of Emissions of Gases and Aerosols from Nature version 2.1 (MEGAN2.1): an extended and updated framework for modeling biogenic emissions, Geosci. Model Dev., 5, 1471–1492, https://doi.org/10.5194/gmd-5-1471-2012, 2012.
Heald, C. L. and Geddes, J. A.: The impact of historical land use change from 1850 to 2000 on secondary particulate matter and ozone, Atmos. Chem. Phys., 16, 14997–15010, https://doi.org/10.5194/acp-16-14997-2016, 2016.
Heald, C. L. and Spracklen, D. V.: Land Use Change Impacts on Air Quality and Climate, Chem. Rev., 115, 4476–4496, https://doi.org/10.1021/cr500446g, 2015.
Henze, D. K. and Seinfeld, J. H.: Global secondary organic aerosol from isoprene oxidation, Geophys. Res. Lett., 33, L09812, https://doi.org/10.1029/2006gl025976, 2006.
Hoesly, R. M., Smith, S. J., Feng, L., Klimont, Z., Janssens-Maenhout, G., Pitkanen, T., Seibert, J. J., Vu, L., Andres, R. J., Bolt, R. M., Bond, T. C., Dawidowski, L., Kholod, N., Kurokawa, J.-I., Li, M., Liu, L., Lu, Z., Moura, M. C. P., O'Rourke, P. R., and Zhang, Q.: Historical (1750–2014) anthropogenic emissions of reactive gases and aerosols from the Community Emissions Data System (CEDS), Geosci. Model Dev., 11, 369–408, https://doi.org/10.5194/gmd-11-369-2018, 2018.
Horowitz, L. W., Naik, V., Paulot, F., Ginoux, P. A., Dunne, J. P., Mao, J., Schnell, J., Chen, X., He, J., John, J. G., Lin, M., Lin, P., Malyshev, S., Paynter, D., Shevliakova, E., and Zhao, M.: The GFDL Global Atmospheric Chemistry-Climate Model AM4.1: Model Description and Simulation Characteristics, J. Adv. Model. Earth Sy., 12, e2019MS002032, https://doi.org/10.1029/2019MS002032, 2020.
Hoyle, C. R., Myhre, G., Berntsen, T. K., and Isaksen, I. S. A.: Anthropogenic influence on SOA and the resulting radiative forcing, Atmos. Chem. Phys., 9, 2715–2728, https://doi.org/10.5194/acp-9-2715-2009, 2009.
Hoyle, C. R., Boy, M., Donahue, N. M., Fry, J. L., Glasius, M., Guenther, A., Hallar, A. G., Huff Hartz, K., Petters, M. D., Petäjä, T., Rosenoern, T., and Sullivan, A. P.: A review of the anthropogenic influence on biogenic secondary organic aerosol, Atmos. Chem. Phys., 11, 321–343, https://doi.org/10.5194/acp-11-321-2011, 2011.
Hu, W. W., Hu, M., Yuan, B., Jimenez, J. L., Tang, Q., Peng, J. F., Hu, W., Shao, M., Wang, M., Zeng, L. M., Wu, Y. S., Gong, Z. H., Huang, X. F., and He, L. Y.: Insights on organic aerosol aging and the influence of coal combustion at a regional receptor site of central eastern China, Atmos. Chem. Phys., 13, 10095–10112, https://doi.org/10.5194/acp-13-10095-2013, 2013.
Isaacman-VanWertz, G., Massoli, P., O'Brien, R., Lim, C., Franklin, J. P., Moss, J. A., Hunter, J. F., Nowak, J. B., Canagaratna, M. R., Misztal, P. K., Arata, C., Roscioli, J. R., Herndon, S. T., Onasch, T. B., Lambe, A. T., Jayne, J. T., Su, L., Knopf, D. A., Goldstein, A. H., Worsnop, D. R., and Kroll, J. H.: Chemical evolution of atmospheric organic carbon over multiple generations of oxidation, Nature Chem., 10, 462–468, https://doi.org/10.1038/s41557-018-0002-2, 2018.
Jiang, J., Aksoyoglu, S., Ciarelli, G., Oikonomakis, E., El-Haddad, I., Canonaco, F., O'Dowd, C., Ovadnevaite, J., Minguillón, M. C., Baltensperger, U., and Prévôt, A. S. H.: Effects of two different biogenic emission models on modelled ozone and aerosol concentrations in Europe, Atmos. Chem. Phys., 19, 3747–3768, https://doi.org/10.5194/acp-19-3747-2019, 2019.
Kim, P. S., Jacob, D. J., Fisher, J. A., Travis, K., Yu, K., Zhu, L., Yantosca, R. M., Sulprizio, M. P., Jimenez, J. L., Campuzano-Jost, P., Froyd, K. D., Liao, J., Hair, J. W., Fenn, M. A., Butler, C. F., Wagner, N. L., Gordon, T. D., Welti, A., Wennberg, P. O., Crounse, J. D., St. Clair, J. M., Teng, A. P., Millet, D. B., Schwarz, J. P., Markovic, M. Z., and Perring, A. E.: Sources, seasonality, and trends of southeast US aerosol: an integrated analysis of surface, aircraft, and satellite observations with the GEOS-Chem chemical transport model, Atmos. Chem. Phys., 15, 10411–10433, https://doi.org/10.5194/acp-15-10411-2015, 2015.
Koch, D., Bauer, S. E., Genio, A. D., Faluvegi, G., McConnell, J. R., Menon, S., Miller, R. L., Rind, D., Ruedy, R., Schmidt, G. A., and Shindell, D.: Coupled Aerosol-Chemistry–Climate Twentieth-Century Transient Model Investigation: Trends in Short-Lived Species and Climate Responses, J. Climate, 24, 2693–2714, https://doi.org/10.1175/2011JCLI3582.1, 2011.
Kroll, J. H. and Seinfeld, J. H.: Chemistry of secondary organic aerosol: Formation and evolution of low-volatility organics in the atmosphere, Atmos. Environ., 42, 3593–3624, https://doi.org/10.1016/j.atmosenv.2008.01.003, 2008.
Li, J., Mao, J., Min, K.-E., Washenfelder, R. A., Brown, S. S., Kaiser, J., Keutsch, F. N., Volkamer, R., Wolfe, G. M., Hanisco, T. F., Pollack, I. B., Ryerson, T. B., Graus, M., Gilman, J. B., Lerner, B. M., Warneke, C., de Gouw, J. A., Middlebrook, A. M., Liao, J., Welti, A., Henderson, B. H., McNeill, V. F., Hall, S. R., Ullmann, K., Donner, L. J., Paulot, F., and Horowitz, L. W.: Observational constraints on glyoxal production from isoprene oxidation and its contribution to organic aerosol over the Southeast United States, J. Geophys. Res.-Atmos., 121, 9849–9861, https://doi.org/10.1002/2016JD025331, 2016.
Li, J., Mao, J., Fiore, A. M., Cohen, R. C., Crounse, J. D., Teng, A. P., Wennberg, P. O., Lee, B. H., Lopez-Hilfiker, F. D., Thornton, J. A., Peischl, J., Pollack, I. B., Ryerson, T. B., Veres, P., Roberts, J. M., Neuman, J. A., Nowak, J. B., Wolfe, G. M., Hanisco, T. F., Fried, A., Singh, H. B., Dibb, J., Paulot, F., and Horowitz, L. W.: Decadal changes in summertime reactive oxidized nitrogen and surface ozone over the Southeast United States, Atmos. Chem. Phys., 18, 2341–2361, https://doi.org/10.5194/acp-18-2341-2018, 2018.
Liggio, J., Li, S.-M., and McLaren, R.: Reactive uptake of glyoxal by particulate matter, J. Geophys. Res., 110, D10304, https://doi.org/10.1029/2004JD005113, 2005.
Lin, G., Sillman, S., Penner, J. E., and Ito, A.: Global modeling of SOA: the use of different mechanisms for aqueous-phase formation, Atmos. Chem. Phys., 14, 5451–5475, https://doi.org/10.5194/acp-14-5451-2014, 2014a.
Lin, G., Penner, J. E., Flanner, M. G., Sillman, S., Xu, L., and Zhou, C.: Radiative forcing of organic aerosol in the atmosphere and on snow: Effects of SOA and brown carbon, J. Geophys. Res.-Atmos., 119, 7453–7476, https://doi.org/10.1002/2013JD021186, 2014b.
Liu, Y., Dong, X., Wang, M., Emmons, L. K., Liu, Y., Liang, Y., Li, X., and Shrivastava, M.: Analysis of secondary organic aerosol simulation bias in the Community Earth System Model (CESM2.1), Atmos. Chem. Phys., 21, 8003–8021, https://doi.org/10.5194/acp-21-8003-2021, 2021.
Liu, Y., Dong, X., Emmons, L. K., Jo, D. S., Liu, Y., Shrivastava, M., Yue, M., Liang, Y., Song, Z., He, X., and Wang, M.: Exploring the factors controlling the long-term trend (1988–2019) of surface organic aerosols in the continental United States by simulations, J. Geophys. Res.-Atmos., 128, e2022JD037935, https://doi.org/10.1029/2022JD037935, 2023.
Malm, W. C., Schichtel, B. A., Hand, J. L., and Collett, J. L.: Concurrent Temporal and Spatial Trends in Sulfate and Organic Mass Concentrations Measured in the IMPROVE Monitoring Program, J. Geophys. Res.-Atmos., 122, 10462–10476, https://doi.org/10.1002/2017JD026865, 2017.
Mao, J., Horowitz, L. W., Naik, V., Fan, S., Liu, J., and Fiore, A. M.: Sensitivity of tropospheric oxidants to biomass burning emissions: implications for radiative forcing, Geophys. Res. Lett., 40, 1241–1246, https://doi.org/10.1002/grl.50210, 2013.
Mao, J., Carlton, A., Cohen, R. C., Brune, W. H., Brown, S. S., Wolfe, G. M., Jimenez, J. L., Pye, H. O. T., Lee Ng, N., Xu, L., McNeill, V. F., Tsigaridis, K., McDonald, B. C., Warneke, C., Guenther, A., Alvarado, M. J., de Gouw, J., Mickley, L. J., Leibensperger, E. M., Mathur, R., Nolte, C. G., Portmann, R. W., Unger, N., Tosca, M., and Horowitz, L. W.: Southeast Atmosphere Studies: learning from model-observation syntheses, Atmos. Chem. Phys., 18, 2615–2651, https://doi.org/10.5194/acp-18-2615-2018, 2018.
Marais, E. A., Jacob, D. J., Jimenez, J. L., Campuzano-Jost, P., Day, D. A., Hu, W., Krechmer, J., Zhu, L., Kim, P. S., Miller, C. C., Fisher, J. A., Travis, K., Yu, K., Hanisco, T. F., Wolfe, G. M., Arkinson, H. L., Pye, H. O. T., Froyd, K. D., Liao, J., and McNeill, V. F.: Aqueous-phase mechanism for secondary organic aerosol formation from isoprene: application to the southeast United States and co-benefit of SO2 emission controls, Atmos. Chem. Phys., 16, 1603–1618, https://doi.org/10.5194/acp-16-1603-2016, 2016.
Marais, E. A., Jacob, D. J., Turner, J. R., and Mickley, L. J.: Evidence of 1991–2013 decrease of biogenic secondary organic aerosol in response to SO2 emission controls, Environ. Res. Lett., 12, 054018, https://doi.org/10.1088/1748-9326/aa69c8, 2017.
Myhre, G., Samset, B. H., Schulz, M., Balkanski, Y., Bauer, S., Berntsen, T. K., Bian, H., Bellouin, N., Chin, M., Diehl, T., Easter, R. C., Feichter, J., Ghan, S. J., Hauglustaine, D., Iversen, T., Kinne, S., Kirkevåg, A., Lamarque, J.-F., Lin, G., Liu, X., Lund, M. T., Luo, G., Ma, X., van Noije, T., Penner, J. E., Rasch, P. J., Ruiz, A., Seland, Ø., Skeie, R. B., Stier, P., Takemura, T., Tsigaridis, K., Wang, P., Wang, Z., Xu, L., Yu, H., Yu, F., Yoon, J.-H., Zhang, K., Zhang, H., and Zhou, C.: Radiative forcing of the direct aerosol effect from AeroCom Phase II simulations, Atmos. Chem. Phys., 13, 1853–1877, https://doi.org/10.5194/acp-13-1853-2013, 2013.
Ng, N. L., Brown, S. S., Archibald, A. T., Atlas, E., Cohen, R. C., Crowley, J. N., Day, D. A., Donahue, N. M., Fry, J. L., Fuchs, H., Griffin, R. J., Guzman, M. I., Herrmann, H., Hodzic, A., Iinuma, Y., Jimenez, J. L., Kiendler-Scharr, A., Lee, B. H., Luecken, D. J., Mao, J., McLaren, R., Mutzel, A., Osthoff, H. D., Ouyang, B., Picquet-Varrault, B., Platt, U., Pye, H. O. T., Rudich, Y., Schwantes, R. H., Shiraiwa, M., Stutz, J., Thornton, J. A., Tilgner, A., Williams, B. J., and Zaveri, R. A.: Nitrate radicals and biogenic volatile organic compounds: oxidation, mechanisms, and organic aerosol, Atmos. Chem. Phys., 17, 2103–2162, https://doi.org/10.5194/acp-17-2103-2017, 2017.
Oak, Y. J., Park, R. J., Jo, D. S., Hodzic, A., Jimenez, J. L., Campuzano-Jost, P., Nault, B. A., Kim, H., Kim, H., Ha, E. S., Song, C.-K., Yi, S.-M., Diskin, G. S., Weinheimer, A., J., Blake, D. R., Wisthaler, A., Shim, M., and Shin, Y.: Evaluation of secondary organic aerosol (SOA) simulations for Seoul, Korea, J. Adv. Model. Earth Sy., 14, e2021MS002760, https://doi.org/10.1029/2021MS002760, 2022.
Pai, S. J., Heald, C. L., Pierce, J. R., Farina, S. C., Marais, E. A., Jimenez, J. L., Campuzano-Jost, P., Nault, B. A., Middlebrook, A. M., Coe, H., Shilling, J. E., Bahreini, R., Dingle, J. H., and Vu, K.: An evaluation of global organic aerosol schemes using airborne observations, Atmos. Chem. Phys., 20, 2637–2665, https://doi.org/10.5194/acp-20-2637-2020, 2020.
Pai, S. J., Carter, T. S., Heald, C. L., and Kroll, J. H.: Updated World Health Organization Air Quality Guidelines Highlight the Importance of Non-anthropogenic PM2.5, Environ. Sci. Tech. Let., 9, 501–506, https://doi.org/10.1021/acs.estlett.2c00203, 2022.
Paulot, F., Crounse, J. D., Kjaergaard, H. G., Kurten, A., St Clair, J. M., Seinfeld, J. H., and Wennberg, P. O.: Unexpected Epoxide Formation in the Gas-Phase Photooxidation of Isoprene, Science, 325, 730–733, https://doi.org/10.1126/science.1172910, 2009.
Paulot, F., Ginoux, P., Cooke, W. F., Donner, L. J., Fan, S., Lin, M.-Y., Mao, J., Naik, V., and Horowitz, L. W.: Sensitivity of nitrate aerosols to ammonia emissions and to nitrate chemistry: implications for present and future nitrate optical depth, Atmos. Chem. Phys., 16, 1459–1477, https://doi.org/10.5194/acp-16-1459-2016, 2016.
Philip, S., Martin, R. V., Pierce, J. R., Jimenez, J. L., Zhang, Q., Canagaratna, M. R., Spracklen, D. V., Nowlan, C. R., Lamsal, L. N., Cooper, M. J., and Krotkov, N. A.: Spatially and seasonally resolved estimate of the ratio of organic mass to organic carbon, Atmos. Environ., 87, 34–40, https://doi.org/10.1016/j.atmosenv.2013.11.065, 2014.
Presto, A. A., Huff Hartz, K. E., and Donahue, N. M.: Secondary Organic Aerosol Production from Terpene Ozonolysis. 2. Effect of NOx Concentration, Environ. Sci. Technol., 39, 7046–7054, https://doi.org/10.1021/es050400s, 2005.
Pullinen, I., Schmitt, S., Kang, S., Sarrafzadeh, M., Schlag, P., Andres, S., Kleist, E., Mentel, T. F., Rohrer, F., Springer, M., Tillmann, R., Wildt, J., Wu, C., Zhao, D., Wahner, A., and Kiendler-Scharr, A.: Impact of NOx on secondary organic aerosol (SOA) formation from α-pinene and β-pinene photooxidation: the role of highly oxygenated organic nitrates, Atmos. Chem. Phys., 20, 10125–10147, https://doi.org/10.5194/acp-20-10125-2020, 2020.
Pye, H. O. T., Chan, A. W. H., Barkley, M. P., and Seinfeld, J. H.: Global modeling of organic aerosol: the importance of reactive nitrogen (NOx and NO3), Atmos. Chem. Phys., 10, 11261–11276, https://doi.org/10.5194/acp-10-11261-2010, 2010.
Pye, H. O. T., Pinder, R. W., Piletic, I. R., Xie, Y., Capps, S. L., Lin, Y.-H., Surratt, J. D., Zhang, Z., Gold, A., Luecken, D. J., Hutzell, W. T., Jaoui, M., Offenberg, J. H., Kleindienst, T. E., Lewandowski, M., and Edney, E. O.: Epoxide Pathways Improve Model Predictions of Isoprene Markers and Reveal Key Role of Acidity in Aerosol Formation, Environ. Sci. Technol., 47, 11056–11064, https://doi.org/10.1021/es402106h, 2013.
Pye, H. O. T., D'Ambro, E. L., Lee, B. H., Schobesberger, S., Takeuchi, M., Zhao, Y., Lopez-Hilfiker, F., Liu, J., Shilling, J. E., Xing, J., Mathur, R., Middlebrook, A. M., Liao, J., Welti, A., Graus, M., Warneke, C., de Gouw, J. A., Holloway, J. S., Ryerson, T. B., Pollack, I. B., and Thornton, J. A.: Anthropogenic enhancements to production of highly oxygenated molecules from autoxidation, P. Natl. Acad. Sci. USA, 116, 6641–6646, https://doi.org/10.1073/pnas.1810774116, 2019.
Pye, H. O. T., Ward-Caviness, C. K., Murphy, B. N., Appel, K. W., and Seltzer, K. M.: Secondary organic aerosol association with cardiorespiratory disease mortality in the United States, Nat. Commun., 12, 7215, https://doi.org/10.1038/s41467-021-27484-1, 2021.
Ridley, D. A., Heald, C. L., Ridley, K. J., and Kroll, J. H.: Causes and consequences of decreasing atmospheric organic aerosol in the United States, P. Natl. Acad. Sci. USA, 115, 290–295, https://doi.org/10.1073/pnas.1700387115, 2018.
Robinson, A. L., Donahue, N. M., Shrivastava, M. K., Weitkamp, E. A., Sage, A. M., Grieshop, A. P., Lane, T. E., Pierce, J. R., and Pandis, S. N.: Rethinking Organic Aerosols: Semivolatile Emissions and Photochemical Aging, Science, 315, 1259–1262, https://doi.org/10.1126/science.1133061, 2007.
Rollins, A. W., Browne, E. C., Min, K.-E., Pusede, S. E., Wooldridge, P. J., Gentner, D. R., Goldstein, A. H., Liu, S., Day, D. A., Russell, L. M., and Cohen, R. C.: Evidence for NOx Control over Nighttime SOA Formation, Science, 337, 1210–1212, https://doi.org/10.1126/science.1221520, 2012.
Scott, C. E., Monks, S. A., Spracklen, D. V., Arnold, S. R., Forster, P. M., Rap, A., Carslaw, K. S., Chipperfield, M. P., Reddington, C. L. S., and Wilson, C.: Impact on short-lived climate forcers (SLCFs) from a realistic land-use change scenario via changes in biogenic emissions, Faraday Discuss., 200, 101–120, https://doi.org/10.1039/C7FD00028F, 2017.
Shrivastava, M., Cappa, C. D., Fan, J., Goldstein, A. H., Guenther, A. B., Jimenez, J. L., Kuang, C., Laskin, A., Martin, S. T., Ng, N. L., Petaja, T., Pierce, J. R., Rasch, P. J., Roldin, P., Seinfeld, J. H., Shilling, J., Smith, J. N., Thornton, J. A., Volkamer, R., Wang, J., Worsnop, D. R., Zaveri, R. A., Zelenyuk, A., and Zhang, Q.: Recent advances in understanding secondary organic aerosol: Implications for global climate forcing, Rev. Geophys., 55, 509–559, https://doi.org/10.1002/2016RG000540, 2017.
Simon, H., Reff, A., Wells, B., Xing, J., and Frank, N.: Ozone Trends Across the United States over a Period of Decreasing NOx and VOC Emissions, Environ. Sci. Technol., 49, 186–195, https://doi.org/10.1021/es504514z, 2015.
Solomon, P. A., Crumpler, D., Flanagan, J. B., Jayanty, R. K. M., Rickman, E. E., and McDade, C. E.: U.S. National PM2.5 Chemical Speciation Monitoring Networks—CSN and IMPROVE: Description of networks, J. Air Waste Manage., 64, 1410–1438, https://doi.org/10.1080/10962247.2014.956904, 2014.
Spracklen, D. V., Jimenez, J. L., Carslaw, K. S., Worsnop, D. R., Evans, M. J., Mann, G. W., Zhang, Q., Canagaratna, M. R., Allan, J., Coe, H., McFiggans, G., Rap, A., and Forster, P.: Aerosol mass spectrometer constraint on the global secondary organic aerosol budget, Atmos. Chem. Phys., 11, 12109–12136, https://doi.org/10.5194/acp-11-12109-2011, 2011.
Takeuchi, M. and Ng, N. L.: Chemical composition and hydrolysis of organic nitrate aerosol formed from hydroxyl and nitrate radical oxidation of α-pinene and β-pinene, Atmos. Chem. Phys., 19, 12749–12766, https://doi.org/10.5194/acp-19-12749-2019, 2019.
Tilmes, S., Hodzic, A., Emmons, L. K., Mills, M. J., Gettelman, A., Kinnison, D. E., Park, M., Lamarque, J.-F., Vitt, F., Shrivastava, M., Campuzano-Jost, P., Jimenez, J. L., and Liu, X.: Climate forcing and trends of organic aerosols in the Community Earth System Model (CESM2). J. Adv. Model. Earth Sy., 11, 4323–4351, https://doi.org/10.1029/2019MS001827, 2019.
Tsigaridis, K. and Kanakidou, M.: The present and future of secondary organic aerosol direct forcing on climate, Curr. Clim. Change Rep., 4, 84–98, https://doi.org/10.1007/s40641-018-0092-3, 2018.
Tsigaridis, K., Daskalakis, N., Kanakidou, M., Adams, P. J., Artaxo, P., Bahadur, R., Balkanski, Y., Bauer, S. E., Bellouin, N., Benedetti, A., Bergman, T., Berntsen, T. K., Beukes, J. P., Bian, H., Carslaw, K. S., Chin, M., Curci, G., Diehl, T., Easter, R. C., Ghan, S. J., Gong, S. L., Hodzic, A., Hoyle, C. R., Iversen, T., Jathar, S., Jimenez, J. L., Kaiser, J. W., Kirkevåg, A., Koch, D., Kokkola, H., Lee, Y. H., Lin, G., Liu, X., Luo, G., Ma, X., Mann, G. W., Mihalopoulos, N., Morcrette, J.-J., Müller, J.-F., Myhre, G., Myriokefalitakis, S., Ng, N. L., O'Donnell, D., Penner, J. E., Pozzoli, L., Pringle, K. J., Russell, L. M., Schulz, M., Sciare, J., Seland, Ø., Shindell, D. T., Sillman, S., Skeie, R. B., Spracklen, D., Stavrakou, T., Steenrod, S. D., Takemura, T., Tiitta, P., Tilmes, S., Tost, H., van Noije, T., van Zyl, P. G., von Salzen, K., Yu, F., Wang, Z., Wang, Z., Zaveri, R. A., Zhang, H., Zhang, K., Zhang, Q., and Zhang, X.: The AeroCom evaluation and intercomparison of organic aerosol in global models, Atmos. Chem. Phys., 14, 10845–10895, https://doi.org/10.5194/acp-14-10845-2014, 2014.
Uin, J., Aiken, A. C., Dubey, M. K., Kuang, C., Pekour, M., Salwen, C., Sedlacek, A. J., Senum, G., Smith, S., Wang, J., Watson, T. B., and Springston, S. R.: Atmospheric Radiation Measurement (ARM) Aerosol Observing Systems (AOS) for Surface-Based In Situ Atmospheric Aerosol and Trace Gas Measurements, J. Atmos. Ocean. Tech., 36, 2429–2447, https://doi.org/10.1175/JTECH-D-19-0077.1, 2019.
Unger, N.: Human land-use-driven reduction of forest volatiles cools global climate, Nat. Clim. Change, 4, 907–910, https://doi.org/10.1038/nclimate2347, 2014.
van Marle, M. J. E., Kloster, S., Magi, B. I., Marlon, J. R., Daniau, A.-L., Field, R. D., Arneth, A., Forrest, M., Hantson, S., Kehrwald, N. M., Knorr, W., Lasslop, G., Li, F., Mangeon, S., Yue, C., Kaiser, J. W., and van der Werf, G. R.: Historic global biomass burning emissions for CMIP6 (BB4CMIP) based on merging satellite observations with proxies and fire models (1750–2015), Geosci. Model Dev., 10, 3329–3357, https://doi.org/10.5194/gmd-10-3329-2017, 2017.
Wells, B., Dolwick, P., Eder, B., Evangelista, M., Foley, K., Mannshardt, E., Misenis, C., and Weishampel, A.: Improved estimation of trends in U. S. ozone concentrations adjusted for interannual variability in meteorological conditions, Atmos. Environ., 248, 118234, https://doi.org/10.1016/j.atmosenv.2021.118234, 2021.
Xu, L., Guo, H., Boyd, C. M., Klein, M., Bougiatioti, A., Cerully, K. M., Hite, J. R., Isaacman-VanWertz, G., Kreisberg, N. M., Knote, C., Olson, K., Koss, A., Goldstein, A. H., Hering, S. V., de Gouw, J., Baumann, K., Lee, S.-H., Nenes, A., Weber, R. J., and Ng, N. L.: Effects of anthropogenic emissions on aerosol formation from isoprene and monoterpenes in the south- eastern United States, P. Natl. Acad. Sci. USA, 112, 37–42, https://doi.org/10.1073/pnas.1417609112, 2015.
Xu, L., Pye, H. O. T., He, J., Chen, Y., Murphy, B. N., and Ng, N. L.: Experimental and model estimates of the contributions from biogenic monoterpenes and sesquiterpenes to secondary organic aerosol in the southeastern United States, Atmos. Chem. Phys., 18, 12613–12637, https://doi.org/10.5194/acp-18-12613-2018, 2018.
Yu, Z., Jang, M., Zhang, T., Madhu A., and Han S.: Simulation of Monoterpene SOA Formation by Multiphase Reactions Using Explicit Mechanisms, ACS Earth Space Chem. 2021, 5, 1455−1467, 2021.
Zhang, H., Yee, L. D., Lee, B. H., Curtis, M. P., Worton, D. R., Isaacman-VanWertz, G., Offenberg, J. H., Lewandowski, M., Kleindienst, T. E., Beaver, M. R., Holder, A. L., Lonneman, W. A., Docherty, K. S., Jaoui, M., Pye, H. O. T., Hu, W., Day, D. A., Campuzano-Jost, P., Jimenez, J. L., Guo, H., Weber, R. J., de Gouw, J., Koss, A. R., Edgerton, E. S., Brune, W., Mohr, C., Lopez-Hilfiker, F. D., Lutz, A., Kreisberg, N. M., Spielman, S. R., Hering, S. V., Wilson, K. R., Thornton, J. A., and Goldstein, A. H.: Monoterpenes are the largest source of summertime organic aerosol in the southeastern United States, P. Natl. Acad. Sci. USA, 115, 2038–2043, https://doi.org/10.1073/pnas.1717513115, 2018.
Zhang, Y., Chen, Y., Lambe, A. T., Olson, N. E., Lei, Z., Craig, R. L., Zhang, Z., Gold, A., Onasch, T. B., Jayne, J. T., Worsnop, D. R., Gaston, C. J., Thornton, J. A., Vizuete, W., Ault, A. P., and Surratt, J. D.: Effect of the Aerosol-Phase State on Secondary Organic Aerosol Formation from the Reactive Uptake of Isoprene-Derived Epoxydiols (IEPOX), Environ. Sci. Tech. Let., 5, 167–174, https://doi.org/10.1021/acs.estlett.8b00044, 2018.
Zheng, Y.: Model_output_for_Anthropogenic amplification of biogenic secondary organic aerosol production, figshare [data set], https://doi.org/10.6084/m9.figshare.21493986.v1, 2022.
Zheng, Y.: Observational data set used in Anthropogenic amplification of biogenic secondary organic aerosol production, figshare [data set], https://doi.org/10.6084/m9.figshare.23894370.v1, 2023.
Zheng, Y., Unger, N., Hodzic, A., Emmons, L., Knote, C., Tilmes, S., Lamarque, J.-F., and Yu, P.: Limited effect of anthropogenic nitrogen oxides on secondary organic aerosol formation, Atmos. Chem. Phys., 15, 13487–13506, https://doi.org/10.5194/acp-15-13487-2015, 2015.
Zheng, Y., Thornton, J. A., Ng, N. L., Cao, H., Henze, D. K., McDuffie, E. E., Hu, W., Jimenez, J. L., Marais, E. A., Edgerton, E., and Mao, J.: Long-term observational constraints of organic aerosol dependence on inorganic species in the southeast US, Atmos. Chem. Phys., 20, 13091–13107, https://doi.org/10.5194/acp-20-13091-2020, 2020.
Zhu, J., Penner, J. E., Yu, F., Sillman, S., Andreae, M. O., and Coe, H.: Decrease in radiative forcing by organic aerosol nucleation, climate, and land use change, Nat. Commun., 10, 423, https://doi.org/10.1038/s41467-019-08407-7, 2019.