the Creative Commons Attribution 4.0 License.
the Creative Commons Attribution 4.0 License.
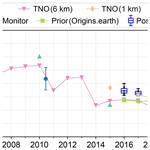
Can we use atmospheric CO2 measurements to verify emission trends reported by cities? Lessons from a 6-year atmospheric inversion over Paris
Thomas Lauvaux
Hervé Utard
François-Marie Bréon
Grégoire Broquet
Michel Ramonet
Olivier Laurent
Ivonne Albarus
Mali Chariot
Simone Kotthaus
Martial Haeffelin
Olivier Sanchez
Olivier Perrussel
Hugo Anne Denier van der Gon
Stijn Nicolaas Camiel Dellaert
Philippe Ciais
Existing CO2 emissions reported by city inventories usually lag in real-time by a year or more and are prone to large uncertainties. This study responds to the growing need for timely and precise estimation of urban CO2 emissions to support present and future mitigation measures and policies. We focus on the Paris metropolitan area, the largest urban region in the European Union and the city with the densest atmospheric CO2 observation network in Europe. We performed long-term atmospheric inversions to quantify the citywide CO2 emissions, i.e., fossil fuel as well as biogenic sources and sinks, over 6 years (2016–2021) using a Bayesian inverse modeling system. Our inversion framework benefits from a novel near-real-time hourly fossil fuel CO2 emission inventory (Origins.earth) at 1 km spatial resolution. In addition to the mid-afternoon observations, we attempt to assimilate morning CO2 concentrations based on the ability of the Weather Research and Forecasting model with Chemistry (WRF-Chem) transport model to simulate atmospheric boundary layer dynamics constrained by observed layer heights. Our results show a long-term decreasing trend of around 2 % ± 0.6 % per year in annual CO2 emissions over the Paris region. The impact of the COVID-19 pandemic led to a 13 % ± 1 % reduction in annual fossil fuel CO2 emissions in 2020 with respect to 2019. Subsequently, annual emissions increased by 5.2 % ± 14.2 % from 32.6 ± 2.2 Mt CO2 in 2020 to 34.3 ± 2.3 Mt CO2 in 2021. Based on a combination of up-to-date inventories, high-resolution atmospheric modeling and high-precision observations, our current capacity can deliver near-real-time CO2 emission estimates at the city scale in less than a month, and the results agree within 10 % with independent estimates from multiple city-scale inventories.
- Article
(4002 KB) - Full-text XML
-
Supplement
(2910 KB) - BibTeX
- EndNote
Most countries have actively committed to the 2015 Paris Agreement in order to limit global warming to well below 2 ∘C, preferably to 1.5 ∘C, compared with pre-industrial levels. To achieve this goal, governments have pledged to implement stringent climate actions to reduce their national emissions with the ultimate objective of reaching climate neutrality by 2050. Cities account for more than 70 % of annual global fossil fuel CO2 emissions and are thus key areas for mitigating CO2 emissions (Seto et al., 2014). To date, many metropolitan areas have pledged to reduce CO2 emissions and have begun to implement policies to achieve net-zero emissions (e.g., C40 Cities, Global Covenant of Mayors for Climate & Energy). The choice of mitigation actions, for cost-effectiveness and to maximize the emission reduction impact, mainly depends on the qualitative and quantitative knowledge of urban emission sources with temporal–spatial characteristics in order to understand evolving emission trends (Lauvaux et al., 2020; Mueller et al., 2021). However, the bottom-up carbon emissions based on public protocols for cities are prone to large uncertainties (Gurney et al., 2021). High-resolution gridded CO2 emission inventories, e.g., the Hestia dataset for some US cities (Gurney et al., 2019) or the London Atmospheric Emissions Inventory (LAEI) dataset for Greater London (Greater London Authority, 2021), provide detailed descriptions of emissions from urban domains. This approach relies on a collection of extensive activity data and emission factors and can thus be labor-intensive and time-consuming, especially when doing regular updates. Recently, Carbon Monitor Cities has been developed to provide near-real-time city-level CO2 emissions data for 1500 global cities from 2019 to 2021 (Huo et al., 2022).
The quantification of greenhouse gas (GHG) emissions from atmospheric measurements offers accounting complementary to the conventional bottom-up approach (Ciais et al., 2010). These methods combine atmospheric measurements with bottom-up inventories through inversion techniques (Tarantola, 2005). Scientific capabilities evolve rapidly with increasing model performances (Deng et al., 2017) and the deployment of dense networks in cities, e.g., the Washington DC–Baltimore metropolitan areas (Karion et al., 2020), the San Francisco Bay Area (Turner et al., 2020), Los Angeles (Yadav et al., 2021), Indianapolis (Davis et al., 2017), Paris, Munich and Zurich (https://www.icos-cp.eu/projects/icos-cities, last access: August 2023). The robustness of the inversion and the derived emission estimates need to be evaluated over periods of several months and years in order to check the stability and relevance of the seasonal cycle and the interannual variability. For example, McKain et al. (2012) indicated that their transport model showed a poor performance in modeling urban sites such that only relative changes in the emission estimates were considered relevant. To our knowledge, few estimates of city GHG emissions based on long-term tower-based measurements and atmospheric inversion systems have been published. These include studies covering a period over 1 to 5 years for the cities of Paris, Boston, Indianapolis and Los Angeles (Staufer et al., 2016; Sargent et al., 2018; Lauvaux et al., 2020; Yadav et al., 2023).
The Paris region, known as Île-de-France (IdF), is the most densely populated and most economically active region in France. Covering only 2 % of French territory, it is inhabited by around 18 % of the French population (12.2 out of 67.8 million inhabitants) and produces 31 % of the national gross domestic product (GDP) and 10 % of human-caused GHG emissions of France (source: AirParif, https://www.airparif.asso.fr/en/monitor-pollution/emissions, last access: August 2023; CITEPA, https://www.citepa.org/fr/2022-co2e/, last access: August 2023). Paris is one of the most active cities in tackling climate change and is part of the C40 Cities consortium. The first Paris Climate Plan was adopted in 2007 and targeted a 25 % reduction in GHG emissions by 2020 with respect to 2004 levels. Paris also has an ambitious 2020–2030 action plan which targets a 50 % decrease in local direct GHG emissions (Scope 1) compared with 2004 levels (Le Plan Climat de Paris, 2020). According to the AirParif (official air-quality agency of the Paris region; https://www.airparif.asso.fr/en/, last access: August 2023) inventory, the contribution of each of the main GHGs (in terms of CO2-equivalent emissions) was 94 % for CO2, 4 % for N2O and 2 % for CH4 in 2010 (AirParif, 2013). Regarding atmospheric CO2 monitoring capability, Paris is an important pilot city with the densest and most comprehensive atmospheric CO2 measurements in Europe (e.g., Lopez et al., 2013; Xueref-Remy et al., 2018; Vogel et al., 2019; Lian et al., 2019). The Parisian ground-based network, whose first measurements date back to 2010, has grown from an initial three to the current seven high-precision continuous in situ CO2 monitoring stations. Over the past few years, a series of studies have attempted to analyze the spatial–temporal variations in CO2 concentrations over the Paris region and have tried to monitor fossil fuel CO2 emissions through an atmospheric inversion technique (Bréon et al., 2015; Wu et al., 2016; Staufer et al., 2016). Lian et al. (2022) estimated CO2 emission reductions during COVID-19 confinements in Paris, which demonstrated the capability of the urban atmospheric monitoring system to identify significant emission changes (>20 %) at short-term monthly timescales.
This study performs the first long-term atmospheric CO2 inversions over the Paris metropolitan area and compares it with multiple city-scale inventories. It aims at assessing the robustness of the inversion and its ability to track absolute urban CO2 emission levels, as well as the relative changes in these emissions over multiple years. The 6-year (2016–2021) continuous CO2 measurements in Paris now provide sufficient information to investigate the variations in CO2 emissions at different timescales (daily, seasonal and interannual) across an urban area. In addition to the mid-afternoon CO2 concentration measurements that are commonly used for inversions, we also explore the potential of assimilating morning CO2 data, taking into account the performance of the Weather Research and Forecasting model with Chemistry (WRF-Chem; Grell et al., 2005) in capturing the evolution of the atmospheric boundary layer (ABL) dynamics. The height of the ABL is the main driver of uncertainties when assessing emissions from concentrations. This paper is organized as follows: Sect. 2 provides details of the city-scale Bayesian inversion methodology. Section 3 shows the variations in CO2 emissions at different timescales. Section 4 summarizes the main conclusions and perspectives for further research.
A city-scale Bayesian inversion was conducted to quantify CO2 emissions over a 6-year period spanning January 2016 to December 2021. The inversion system is based on atmospheric CO2 measurements at seven in situ stations combined with meteorological measurements, the WRF-Chem transport model run at 1 km×1 km horizontal resolution (Lian et al., 2021), a near-real-time fossil fuel CO2 inventory produced by Origins.earth, and the biogenic CO2 fluxes simulated by the Vegetation Photosynthesis and Respiration Model (VPRM) included in WRF-Chem (Mahadevan et al., 2008). Details regarding the inversion system setup are described in Lian et al. (2022) and are outlined briefly below.
2.1 CO2 measurement network
The seven stations are equipped with high-precision cavity ring-down spectroscopy (CRDS) CO2 analyzers, together with an automated data processing and quality-control system. CO2 observations are calibrated every 1 to 6 months with standards traceable to the World Meteorological Organization (WMO) CO2 X2019 calibration scales (Hall et al., 2021). The precision of the 1 h average CO2 concentration is better than 0.1 ppm (Xueref-Remy et al., 2018). These stations are roughly distributed along a northeast–southwest axis of the Paris urban area, which coincides with the predominant wind directions (Fig. 1).
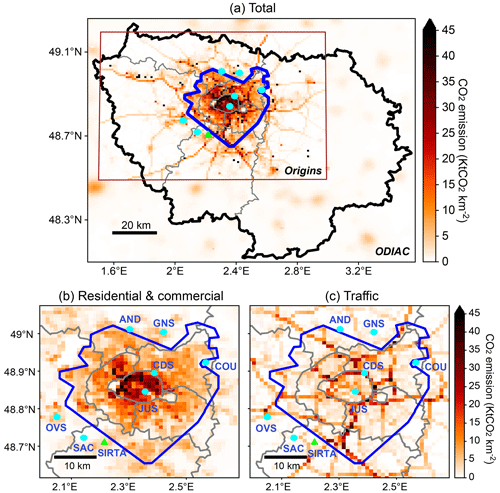
Figure 1Distributions of annual (a) total fossil fuel emissions, (b) residential and commercial emissions, and (c) traffic CO2 emissions for the year 2019 according to the Origins.earth inventory (brown rectangle) complemented by the ODIAC dataset. The seven in situ CO2 measurement stations are shown in cyan circles. The location of the SIRTA observatory with ABL measurements is marked by a green triangle. The bold black line shows the administrative limits of the Île-de-France (IdF) region. In the inversion system, emissions over two emitting regions are optimized: the greater Paris region (within the blue line) and the rest of the IdF region.
Figures 2 and S1 in the Supplement show the monthly and daily average daytime (08:00–17:00 UTC) CO2 concentrations at each in situ station from 2016 to 2021, respectively, and in addition Fig. 2 also shows the simulated background CO2 concentration at the SAC station using the Copernicus Atmosphere Monitoring Service (CAMS) CO2 dataset as the boundary and initial inputs for WRF-Chem. The atmospheric background CO2 concentrations have steadily been rising over the past 6 years, which is primarily attributed to global human activities. Generally, the average CO2 concentrations across the network vary seasonally between 390 and 450 ppm. They are mainly driven by the atmospheric transport, the CO2 biospheric cycle and their proximity to the urban anthropogenic CO2 emission sources. The interannual CO2 variations primarily depend on the year-to-year variations in synoptic weather conditions, air temperature and the associated emissions. For example, the notably high CO2 concentrations observed near the surface during the 2016/17 winter were caused by stagnant, often stable, atmospheric stratification associated with cold and dry air masses and low-ventilation weather conditions over the north of France (Bulletin Climatique Météo-France, 2016, 2017).
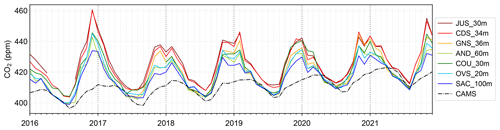
Figure 2Monthly average daytime-observed (08:00–17:00 UTC) CO2 concentrations at seven in situ stations. The dashed black line indicates the simulated background CO2 concentration over Paris by WRF-Chem driven by the CAMS global atmospheric CO2 dataset. The sampling heights above ground level are given in the legend.
The gradients of CO2 concentrations between the downwind and upwind stations are linked to the emissions within the Paris urban area. The CO2 concentrations are significantly higher at the two urban stations CDS and JUS than those at peri-urban sites across all seasons (Fig. 2). The magnitude in CO2 gradients between urban and suburban areas is around 5–10 ppm in summer, increasing to 20–30 ppm during the winter months as a result of more stable atmospheric conditions (lower vertical dispersion and shallow ABL height) combined with high emissions from residential heating. The citywide CO2 gradients across the Paris agglomerations have shown their potential in previous inversion studies to estimate the city-scale CO2 emissions (Bréon et al., 2015; Staufer et al., 2016).
2.2 Origins.earth and other CO2 inventories
Fossil fuel CO2 a priori fluxes used in this study are taken from a patent pending a high-resolution inventory produced by Origins.earth (https://www.origins.earth/, last access: August 2023) over the IdF region, in combination with the global Open-source Data Inventory for Anthropogenic CO2 (ODIAC) product (Oda et al., 2018) in surrounding areas. The Origins.earth bottom-up inventory is a gridded map of fossil fuel CO2 emissions within a rectangular (on a latitude and longitude grid) domain which encompasses most of the IdF region (Fig. 1) at a 1 km×1 km spatial and hourly temporal resolution. It has provided the Scope 1 CO2 emissions from the year 2018 until the present time. For the simulation period from 2016 to 2017, we used CO2 emissions from the Origins.earth inventory for the year 2018 as the WRF-Chem model inputs. The Origins.earth inventory includes more than 60 source types for carbon emission activities. These types of activities are grouped into six activity sectors (e.g., transportation, residential, tertiary, industrial (including cement), energy and waste sectors). The inventory compilation method is outlined in the Supplement (Text S1).
The IdF is a large urban region with estimated annual fossil fuel CO2 emissions exceeding 30 Mt CO2 yr−1, dominated by traffic and residential sectors. The spatial distributions of the emissions are shown in Fig. 1. According to the Origins.earth inventory, the annual emission budgets of the residential sector are 11.0, 10.9, 10.1 and 11.4 Mt CO2, representing 32 %, 34 %, 35 % and 38 % of total emissions for 2018, 2019, 2020 and 2021, respectively. For the traffic sector, averages are 12.3 Mt CO2 (36 %), 10.9 Mt CO2 (33 %), 8.7 Mt CO2 (30 %) and 8.7 Mt CO2 (29 %) from 2018 to 2021 (Fig. S2b). Figure S2a shows the daily fossil fuel CO2 emissions by sector and their respective proportions from 2018 to 2021. Generally, the temporal variations in CO2 emissions from the building sector show a large seasonal cycle, mainly related to the heating demand that is linearly driven by the variations in air temperature below a threshold of ≈19 ∘C. Emissions from the tertiary, industrial and energy sectors have a relatively flat seasonal variation.
In this study, the inverse emissions are compared with independent estimates from different inventories and a published study. These include (i) the TNO-GHGco inventory at a resolution of (long × lat) () for the years 2005–2020 (Denier van der Gon et al., 2021); (ii) the TNO-GHGco inventory at a resolution of (long × lat) () for the years 2015, 2017, and 2018 (Dellaert et al., 2019); (iii) the AirParif inventory developed by the local official air-quality agency at a 1 km×1 km resolution for the years 2005, 2010, 2015, and 2018, (iv) the city-level CO2 emissions from the Carbon Monitor Cities dataset (https://cities.carbonmonitor.org/, last access: August 2023, Huo et al., 2022) for the years 2019–2021; and (v) the study by Staufer et al. (2016), who reported a full year's (August 2010–July 2011) estimate of the IdF region fossil fuel CO2 emissions by assimilating citywide CO2 measurements from a sparse network of three stations with the inversion methodology. Here, we consider that these multiple emission estimates allow for a cross-validation as they were developed by several research groups using distinct data sources, methods and protocols.
2.3 Adding morning CO2 data and ABL height selection
The accuracy of atmospheric inversion results depends to a large extent on the quality of the atmospheric transport model. The major uncertainties in CO2 modeling are related to model errors in horizontal wind and vertical mixing within the atmospheric boundary layer (Kretschmer et al., 2012). A stringent data selection of CO2 concentrations to be assimilated for the inversion is generally applied (e.g., Staufer et al., 2016; Wu et al., 2016; Lauvaux et al., 2020). The aim of the data selection is to rule out observations that are difficult to simulate accurately with the transport model. Previous studies (Staufer et al., 2016; Lian et al., 2022) have assimilated only afternoon CO2 data from 12:00 to 17:00 UTC, during which the atmospheric boundary layer is expected to be well developed. Moreover, the wind speeds at downwind stations are required to be higher than 3 m s−1 to minimize possible contaminations from local sources of CO2 emissions near the measurement sites that cannot be reproduced by the model.
However, the selection of a limited number of suitable data produces more uncertain estimates over periods when no observations are selected. The high sensitivity of the inverse emissions to the diurnal variations in prior emissions also highlights the limitations induced by assimilating data only during the afternoon. Therefore, we attempt to include morning CO2 concentration data in this study. Given that atmospheric models have difficulties in correctly reproducing the mixing processes under stable conditions and when the boundary layer develops in the morning, caution is required when assimilating morning CO2 concentrations. We assumed that the ABL height could provide a good diagnostic to assess the vertical mixing and dilution associated with turbulence near the surface. We thus first evaluated the WRF-Chem-simulated ABL heights against observations at the SIRTA station (Haeffelin et al., 2005) located about 20 km southwest of the center of Paris (Fig. 1). The modeled ABL heights were diagnosed from the potential temperature using the 1.5-theta-increase method (Nielsen-Gammon et al., 2008), while the aerosol-based STRATfinder algorithm (Kotthaus et al., 2020) was applied to derive ABL heights from attenuated backscatter profiles measured with an automatic lidar ceilometer (Lufft CHM 15k).
Figure S3 shows hourly ABL heights derived from both measurements and simulations from 2016 to 2021 for morning (08:00–11:00 UTC) and afternoon (12:00–17:00 UTC) periods, respectively. In general, the model captures the ABL heights throughout the year with a fair correlation coefficient (>0.6) reasonably well. However, the simulated ABL heights are, on average, higher than the measurements with biases of 80–130 m. This could reflect an overestimation of the atmospheric instability in the lowest atmospheric layer by WRF or may in fact indicate that vertical dilution of atmospheric tracers (here observed aerosol profiles) may lag behind the thermodynamic evolution of the ABL morning development (Text S2). Particularly large relative discrepancies are detected during the winter and morning periods associated with stable atmospheric conditions. This model–observation comparison of ABL heights allows us to revise the recent selection of CO2 concentration measurements that can be assimilated into the inversion system as used in Lian et al. (2022) and to extend it into the morning period (Fig. S4). The revision consists primarily of adding two additional selection criteria concerning the morning data between 08:00–11:00 UTC. First, we discard the data when the relative error of the modeled ABL heights against observations is larger than 80 %. This threshold was set based on the distributions of the relative errors in ABL shown in Fig. S3. Second, we apply a tighter filtering for the morning data (08:00–11:00 UTC) by increasing the minimum wind speed threshold to 5 m s−1 compared with the 3 m s−1 used for the afternoon.
2.4 Inversion configuration
The main principle of the Bayesian atmospheric inversion presented in Lian et al. (2022) is to optimize the 6 h mean fossil fuel CO2 emission budgets of the greater Paris region (within the blue line in Fig. 1) and the rest of the IdF region over four time windows per day (00:00–05:00, 06:00–11:00, 12:00–17:00, 18:00–23:00 UTC). The approach assimilates atmospheric CO2 concentration gradients between pairs of stations located upwind and downwind of the city to decrease the uncertainties caused by the transport of remote and natural fluxes outside the urban area. The configurations of the atmospheric inversion system in this study are described in detail in Lian et al. (2022). The configurations comprise the setup of the control vectors, the selection of the assimilated downwind–upwind CO2 observation gradients (Fig. S4), the assignments of the observation error and the prior flux uncertainties. Compared with Lian et al. (2022), only two minor modifications are made in this study in order to assess the impacts of morning CO2 concentrations on the retrieved emissions. (1) Regarding the fossil fuel emissions, we assume a 60 % relative uncertainty in the prior estimates of individual 6 h emission budgets. (2) We assign no temporal correlation of prior uncertainties between the different 6 h time windows. The data selection criteria of the assimilated CO2 observations and the assignments of the prior flux uncertainties of the reference inversion are shown in Table S1 in the Supplement. In addition, we also conducted a series of sensitivity tests to investigate how the inverse estimates respond to changes in various setups of the inversion system (Table S1). This inversion ensemble was composed of five tests of the selection criteria of the assimilated CO2 observations as well as five tests of the uncertainties and the temporal correlations of the prior emissions.
3.1 Daily emission estimates
Statistical comparisons between the assimilated modeled and measured CO2 concentration gradients before and after flux optimizations are shown in Fig. S5. In general, the inversion leads to an overall improvement in the representation of observations, for both the morning period (08:00–11:00 UTC) (Fig. S5c) and the afternoon period (12:00–17:00 UTC) (Fig. S5d). The mean bias errors (MBEs) are reduced from −0.85 to −0.29 ppm in the morning and from −1.16 to −0.55 ppm in the afternoon after the inversion. The selected morning CO2 gradients correspond to ∼24.5 % (5168 of 21 091) of the total assimilated observation gradients.
Figure 3 compares the estimates from the reference inversion, which assimilates the daytime (08:00–17:00 UTC) CO2 concentration gradients, to the estimates from the two sensitivity tests that only use morning (08:00–11:00 UTC) or afternoon (12:00–17:00 UTC) CO2 data, respectively. Here, we mainly focus on the greater Paris region (Fig. 1) where the fossil fuel CO2 emissions can be well constrained from our observation network. Figure 3 shows the average prior and posterior fossil fuel CO2 emission estimates together with their associated uncertainties over the 6-year period spanning January 2016 to December 2022, for the four 6 h time windows and every day of the week. The time series of the daily prior and posterior fossil fuel CO2 emissions are shown in Fig. 4. The reference inversion (Fig. 3b) mainly imposes a direct constraint on the fossil fuel fluxes for the two 6 h windows of the day (06:00–11:00 and 12:00–17:00 UTC), while having little constraint on nighttime emissions (00:00–05:00 and 18:00–23:00 UTC). This is because the assimilated daytime CO2 concentrations are mainly sensitive to the emissions from the morning and afternoon periods. The inversion increases the average posterior emissions per day of the week by around 9 %–16 % and 13 %–23 % with uncertainty reductions of 14 %–21 % and 15 %–21 % for the 06:00–11:00 and 12:00–17:00 UTC time windows, respectively. The assimilation of only afternoon CO2 data (Fig. 3d) has similar retrieved emissions but with a smaller uncertainty reduction, especially for the 06:00–11:00 UTC period, compared with the reference one which assimilates morning data. Even though we assimilate a relatively small number of morning CO2 data (Fig. 3c), such a change still leads to a 11 %–16 % uncertainty reduction compared with a priori of the morning fossil fuel emissions (Fig. 3c).
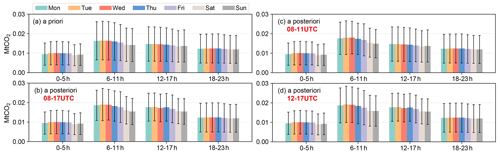
Figure 3Average (a) prior and (b–d) posterior fossil fuel CO2 emission estimates and their uncertainties over the greater Paris region over the years 2016–2021, for the four 6 h time windows and the days of the week. Panels (b)–(d) present the posterior CO2 estimates when assimilating (b) daytime (08:00–17:00 UTC), (c) morning (08:00–11:00 UTC) and (d) afternoon (12:00–17:00 UTC) selected CO2 concentration observations, respectively.
The inversion on average reduces the uncertainty in the daily fossil fuel CO2 emission estimates (Fig. 4a) from ∼31 % (prior) down to ∼24 % ± 4.5 % (posterior) (a range of 2.5 %–11.5 % uncertainty reduction) over the greater Paris region. On the contrary, CO2 emissions over the rest of the IdF region (Fig. S6) after the inversion remain close to their prior values due to insufficient observational constraints. The inverse CO2 emissions show a fairly good agreement with the prior estimate with a Pearson correlation coefficient (R) of 0.91, which indicates that the Origins.earth near-real-time inventory can already capture some timely emission variations that are closely related to meteorological effects and human activities relatively well. For instance, the lower emissions in February 2020 appear to be mainly due to weather conditions, with a mild winter leading to a lower demand for heating (Bulletin Climatique Météo-France, 2020). A decrease in the daily CO2 emissions is observed in July and August due to a reduction in traffic emissions during the summer holidays. It can also be seen that the daily fossil fuel CO2 emissions dropped by more than 30 % in the second half of March 2020, when strict COVID-19 lockdown measures were adopted. In general, the inverse CO2 emissions exhibit a larger daily variability compared with the prior. On the one hand, the temporal profiles used in the inventory rely on some degree of interpolation, proxy data and theoretical assumptions that can smooth out some temporal variability. On the other hand, the posterior daily variability represents both the actual variability in emissions caused by human activities and the sources of uncertainty in the inverse modeling system, especially the transport model errors.
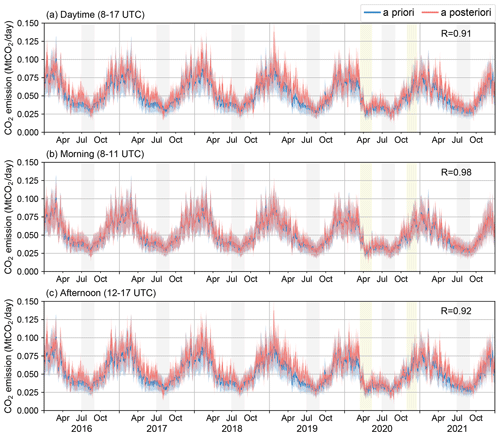
Figure 4Daily estimates of fossil fuel CO2 emissions over the greater Paris region when assimilating (a) daytime (08:00–17:00 UTC), (b) morning (08:00–11:00 UTC) and (c) afternoon (12:00–17:00 UTC) CO2 concentration observations. The blue line and shading show the prior flux according to the Origins.earth inventory together with its assumed uncertainty. The pink and shading show the posterior estimates with their uncertainty ranges. The yellow shaded areas are the two COVID-19 lockdown periods in France. The grey shaded areas are the summer holidays in July and August.
3.2 Monthly emission estimates
Figure 5a shows the posterior estimate of the monthly fossil fuel CO2 emissions over the greater Paris region derived through a temporal aggregation of the four 6 h period results. Results for the rest of the IdF region are given in Fig. S7. The inversion tends to increase the annual fossil fuel emissions by 2 %–6 % with respect to the prior estimates for every year from 2016 to 2021. It demonstrates the consistency of the measurement constraint on inverse fossil fuel CO2 emissions over time. It is important to note that the inversions for the years 2016 and 2017 were carried out with the prior emissions from the 2018 Origins.earth inventory, causing the inverse emission changes to mainly rely on the assimilated CO2 observations. With the same prior emissions for 2017 and 2018, the inversion leads to an emission reduction of 0.6 % over the greater Paris region from 2017 to 2018, which is consistent with the general trend towards a decrease in emission.
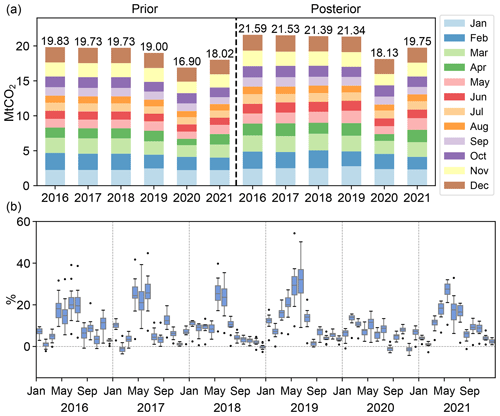
Figure 5(a) Prior and posterior estimates of the monthly total fossil fuel CO2 emission over the greater Paris region. (b) The change in CO2 emissions in percentage . The boxplots are the posterior emissions from an ensemble of sensitivity tests of the inversion configuration. Note that the prior emission for 2016 is slightly higher than for 2017 and 2018 since it is a leap year.
Figure 5b shows the relative difference between the posterior and prior fossil fuel CO2 emissions corresponding to the different inversion setups (e.g., prior errors and correlation length). It is worth noting that the optimized monthly emissions are very similar across the 6 years from 2016 to 2021. More precisely, the inversion consistently points to an average 10 % increase in fossil fuel emissions in winter months compared with the prior (January to March). Further, the inverse emissions show significantly higher values (>20 %) with respect to the prior during the spring period (especially in May and June). In contrast, minor differences between the prior and posterior estimates are observed for the rest of the year. The ensemble of posterior fluxes, as represented by the spread of the minimum and maximum values among multiple inversions, provides an estimate of the variability introduced by a wide range of inversion setups. Even though these sensitivity tests may not represent the full potential range of uncertainties in the posterior fluxes, they still give us a certain degree of confidence in the inversion results because these variabilities are mostly smaller than the posterior–prior emission differences and the emissions uncertainties.
We then conduct a series of analyses to investigate the potential explanations for the adjustments to the prior fluxes made by the inversion. First, we infer that the seasonal variability in flux adjustments is not caused by biases in the atmospheric transport model. This is because the model–observation misfits in wind speed exhibit a relatively constant pattern throughout a year without a pronounced seasonality (Fig. S8). Second, the 10 % increase in fossil fuel CO2 emissions during the winter months (January to March) could be due to an underestimation of the residential emissions in the Origins.earth inventory. The temporal profiles of the Origins.earth residential emissions are based on a proxy quantity derived from real-time domestic gas consumption data (Text S3), whereas in reality, this seasonal cycle in the prior emissions may underestimate wintertime energy demands from other fuel types, especially for petroleum and wood burning in the suburban residential areas (Fig. S9). Notably, the estimated monthly emissions are ∼20 % larger compared with the prior estimates from April to June. Part of the adjustments of emissions could be due to an underestimation of the biogenic fluxes from the VPRM model (Text S4). Figure S11 shows an obvious discrepancy between the VPRM-simulated hourly biogenic flux (net ecosystem exchange, NEE) and the eddy flux measurements, especially during the growing season, at the Grignon cropland station located about 40 km west of the center of Paris (Fig. S10). The model–observation comparison of the vertical differences in CO2 concentrations at the SAC station (15 and 100 m above ground level) shows that the contribution of the nighttime biogenic respiration to the CO2 concentration could also be a potential source of modeling errors during the growing season (Lian et al., 2021).
3.3 Annual emission estimates
Verifying annual emission totals and tracking emission trends over time using multiple methods constitute an indication of the reliability of inverse emissions estimates. Moreover, given the potential impact of atmospheric transport errors on the emission estimates, and assuming that the model errors do not change statistically over time, the emission trends are expected to be less sensitive to model biases than the emissions estimates. The interannual variations are a critical metric to assess the effectiveness of the CO2 mitigation efforts. Figure 6 shows the multiyear trend in the annual total fossil fuel CO2 emissions over the IdF region for the 2005–2021 period. The prior and inverse emissions are compared with multiple inventory datasets. The interannual variation in fossil fuel CO2 emissions indicates a decreasing trend over the IdF region during the 2005–2021 period using the Mann–Kendall (MK) trend test at a 5 % significance level (p values (0.0001)<0.05). According to the TNO 6 km inventory, this is mainly linked to the emission reductions in the residential and industrial sectors and is further a result of a decrease in coal use, an improvement in energy efficiency and building renovation. The inverse annual fossil fuel CO2 emissions have been declining at a rate of ∼2 % ± 0.6 % per year over the 6-year period from 2016 to 2021 (MK test p values (0.02)<0.05). In 2020, the COVID-19 pandemic and the continued levels of restrictions in place resulted in a dramatic decline in human activity. Our inversion results indicate a ∼13 % (12 %–14 %) reduction in the annual emissions in 2020 compared with 2019, which is 2 % (1 %–3 %) larger than the prior estimate based on the Origins.earth inventory (11 %). The inverse annual emissions in 2021 rose by about 5.2 % ± 14.2 % to 34.3 ± 2.3 Mt CO2 compared with 2020 (32.6 ± 2.2 Mt CO2) but still remained −8.0 % ± 12.6 % compared with the pre-COVID-19 level in 2019 (37.3 ± 2.6 Mt CO2).
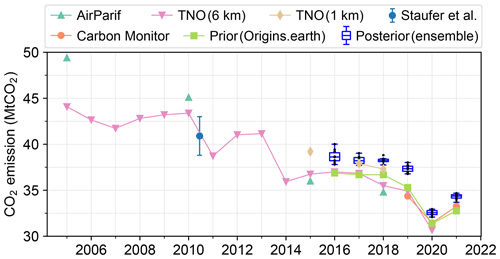
Figure 6Annual fossil fuel CO2 emissions over the IdF region from 2005 to 2021. The blue boxplots present the distribution of posterior CO2 emissions from an ensemble of sensitivity tests of the inversion configuration.
The aggregated annual fossil fuel CO2 emissions obtained with the inversion are on average higher by ∼5 % for each year compared with the prior estimates from the Origins.earth inventory. Our inversion results are in agreement with the TNO 1 km inventory for all city-scale fossil fuel CO2 estimates for the years 2017 and 2018, while they are approximately 8.6 %, 3.6 % and 3.1 % higher than those estimated by Carbon Monitor Cities for the years 2019, 2020 and 2021, respectively. In 2018, the optimized emission is 38.1 ± 2.6 Mt CO2. It is around 3.3, 2.7 and 0.8 Mt CO2 higher than that from the AirParif, TNO 6 km and TNO 1 km inventories, respectively. Generally, the agreement among the various estimates of the annual fossil fuel CO2 emissions over the IdF region is within 10 %, demonstrating robust emission estimates at the city scale through a combination of up-to-date inventories, atmospheric modeling and observations. It also provides evidence for a continuous and timely monitoring of urban fossil fuel CO2 emission trends toward the accomplishment of reduction targets in order to achieve carbon neutrality.
This study shows the capacity of our city-scale inversion system, with a state-of-the-art inventory and high-precision continuous CO2 measurements, for a timely and an effective monitoring of urban fossil fuel CO2 emissions over a long time period from 2016 to 2021. Our results indicate a decreasing trend in the annual CO2 emissions over the IdF region with an amplitude of ∼2 % ± 0.6 % per year at a 5 % significance level. The comparison of both prior and posterior annual emissions with independent estimates from other inventory datasets shows a less than 10 % difference, which is a satisfying target in terms of emission trend detection and verification for Paris in order to support its emissions-mitigation measures and related policy (Wu et al., 2016). In practice, few cities have such a high-resolution near-real-time local inventory like Paris. Through using the same annual total emission as a prior for the years 2016–2018, the posterior emissions exhibit an emission change by about −3 % ± 13.8 % over the IdF region when comparing the year 2016 with 2018. This demonstrates the ability of the inversion system to detect emission changes based on CO2 measurements, independently of the information provided by the prior inventory. In addition to the afternoon CO2 measurements that are commonly used in the CO2 inversion system, the assimilation of morning CO2 data when the ABL heights are well reproduced by the model could lead to an additional 11 %–16 % uncertainty reduction in the morning fossil fuel emissions. But it is worth pointing out that the selection of morning CO2 observations only for moderate-to-high wind speeds might reduce the observational constraint on the emissions in stable weather periods.
The uncertainties in the posterior estimates of CO2 emissions are caused to a certain extent by errors in the spatiotemporal distribution of emissions at scales finer than the targeted ones. The present inversion system mainly controls the city-scale fossil fuel CO2 emissions but not the finer resolution in space. We thus further conducted a sensitivity inversion test using the TNO 1 km inventory as a priori as an alternative to the Origins.earth dataset for 2018. The other parameters were kept identical to the reference inversion configuration. The TNO inventory indicates more fossil fuel CO2 emissions concentrated within inner Paris compared with the Origins.earth dataset (Fig. S12). The inversion was able to spatially differentiate between Paris' city center and the greater Paris region (excluding Paris), correcting the respective emissions differently (Fig. S13). The posterior fossil fuel CO2 emissions from Paris' city center were adjusted more upwardly with Origins.earth than with TNO, while those from the rest of the greater Paris region were corrected at a similar magnitude between Origins.earth and TNO. One can hope that a spatially explicit inversion system would allow us to solve the spatial distribution of urban emissions at the grid scale (Lauvaux et al., 2016). However, this will need additional information in order to determine the spatial correlation length of the inventory uncertainty or a high-density observation network in order to constrain the emissions from a large part of the city (Nalini et al., 2022).
Improvements in urban ecosystem modeling and monitoring for a precise accounting of urban biomass in the estimates of CO2 fluxes are a relatively recent endeavor. Focusing on the Paris urban area, two limitations of this study are acknowledged and are considered worthy of further investigation. Firstly, due to the coarse-resolution SYNMAP land use (1 km) data (Jung et al., 2006) and the MODIS satellite-derived vegetation indices (500 m) used for the VPRM model, the simulated biogenic fluxes in Paris in this study are almost zero, except for a few grid cells containing two big parks that are located in the eastern and western outskirts of Paris. In reality, there are still a number of green spaces and pervious landscaped areas unevenly distributed in Paris that need to be considered with a fine-scale (sub-kilometer) model. Secondly, there is a lack of detailed evaluation of the Paris VPRM model since no eddy covariance measurement is available within Paris and its surroundings yet. Our analyses indicate that the actual biogenic fluxes within the IdF region may have a recognizable influence on the measured CO2 concentration gradients, whereas these biosphere signals are not well reproduced by the VPRM model. These discrepancies question the validity of the assumption that the signature of the local biogenic fluxes is not significant compared with that of the fossil fuel emissions in the measured gradients. Even though we have scaled up the prescribed observation errors to account for this possible large model bias in the biogenic fluxes and thereby to reduce its interference in the inverse fossil fuel emissions, the results may inevitably still be hampered. This study highlights the need for an in-depth analysis of the seasonal and daily variations in the biogenic fluxes within the IdF region, especially during the cropland growing season. This could be achieved by improving the default VPRM model with a modified gross primary production and ecosystem respiration equations, domain-specific optimized parameters, high-resolution input datasets, and high-quality diagnostic phenology (Gourdji et al., 2022). In addition, measurements of carbon isotopes (14C, 13C) and tracers co-emitted with CO2 (e.g., CO, NOx, VOCs) could also be used to separate the contributions from fossil fuel and biogenic components to the total CO2 concentrations, which would be beneficial for the optimization of sectoral CO2 fluxes.
The hourly-averaged CO2 data measured at seven in situ stations are available on request from Michel Ramonet (michel.ramonet@lsce.ipsl.fr).
The observed ABL height data are available on request from Simone Kotthaus (simone.kotthaus@ipsl.polytechnique.fr).
The Origins.earth CO2 inventories are available on request from Hervé Utard (herve.utard@origins.earth).
The TNO CO2 inventories are available on request from Hugo Anne Denier van der Gon (hugo.deniervandergon@tno.nl).
The ODIAC fossil fuel emission dataset (version: ODIAC2020b) was downloaded from the Center for Global Environmental Research, National Institute for Environmental Studies, https://doi.org/10.17595/20170411.001 (Oda and Maksyutov, 2015).
The Carbon Monitor Cities dataset was downloaded from https://cities.carbonmonitor.org/ (Huo et al., 2022).
The eddy covariance measurements were downloaded from the ICOS (https://doi.org/10.18160/2G60-ZHAK, Warm Winter 2020 team and ICOS Ecosystem Thematic Centre, 2022).
The supplement related to this article is available online at: https://doi.org/10.5194/acp-23-8823-2023-supplement.
JL, TL and PC designed the study. JL did the coding and implementation of the research. JL, TL, PC, FMB, GB, HU, MR and IA contributed to the analysis and interpretation of the results. MR, OL and MC scientifically coordinated the development of the CO2 measurement stations and ensured the calibration of the dataset. SK and MH processed the observed ABL data and gave sound advice on the model–data evaluation. HU provided the Origins.earth inventory, OS and OP provided the AirParif emission estimates, and HADvdG and SNCD provided the TNO inventory; they all provided valuable feedback and opinions on the multiple dataset comparisons in Sect. 3.3. JL prepared the paper with contributions and suggestions from all the authors.
The contact author has declared that none of the authors has any competing interests.
Publisher's note: Copernicus Publications remains neutral with regard to jurisdictional claims in published maps and institutional affiliations.
The authors would like to thank the SUEZ Group, La Ville de Paris and ICOS Cities for the support of this study. We also thank Marc Jamous at CDS, the IPSL QUALAIR platform team, Cristelle Cailteau-Fischbach (LATMOS/IPSL) at JUS and LSCE/RAMCES technical staff for the maintenance of the CO2 monitoring network. We acknowledge Pauline Buysse, Jérémie Depuydt, Daniel Berveiller and Nicolas Delpierre for providing the eddy covariance flux measurements at the Grignon and Fontainebleau (Barbeau) ecosystem sites. We also acknowledge Xiaobo Yang and Anna Agusti-Panareda from ECMWF for providing the near-real-time CAMS CO2 dataset, Elise Potier for her help in processing the TNO inventory, and the technical and IT staff at the SIRTA observatory for operating the ALC. We thank Melania van Hove and the AERIS-ESPRI data center for supporting the MLH product retrieval.
The authors have received funding from ICOS Cities, a.k.a. the Pilot Applications in Urban Landscapes – Towards integrated city observatories for greenhouse gases (PAUL) project, from the European Union's Horizon 2020 research and innovation program under grant agreement no. 101037319. Thomas Lauvaux was supported by the project CIUDAD (Make Our Planet Great Again program) and the Chair Fellowship CASAL (French Ministry of Research – CNRS).
This paper was edited by Tim Butler and reviewed by two anonymous referees.
AirParif: Bilan des émissions de polluants atmosphériques et de gaz à effet de serre en Île-de-France pour l'année 2010 et historique 2000/2005, AIRPARIF association de surveillance de la qualité de l'air en Ile-de-France, https://docplayer.fr/10561897-Bilan-des-emissions-de-polluants-atmospheriques-et-de-gaz-a-effet-de-serre-en-ile-de-france-pour-l-annee-2010-et-historique-2000-2005.html (last access: August 2023), 2013.
Bréon, F. M., Broquet, G., Puygrenier, V., Chevallier, F., Xueref-Remy, I., Ramonet, M., Dieudonné, E., Lopez, M., Schmidt, M., Perrussel, O., and Ciais, P.: An attempt at estimating Paris area CO2 emissions from atmospheric concentration measurements, Atmos. Chem. Phys., 15, 1707–1724, https://doi.org/10.5194/acp-15-1707-2015, 2015.
Bulletin Climatique Météo-France: Mensuel sur la France, Décembre 2016, https://donneespubliques.meteofrance.fr/donnees_libres/bulletins/BCM/201612.pdf (last access: November 2022), 2016.
Bulletin Climatique Météo-France: Mensuel sur la France, Janvier 2017, https://donneespubliques.meteofrance.fr/donnees_libres/bulletins/BCM/201701.pdf (last access: November 2022), 2017.
Bulletin Climatique Météo-France: Mensuel régional Ile de France, Février 2020, https://donneespubliques.meteofrance.fr/donnees_libres/bulletins/BCMR/BCMR_08_202002.pdf (last access: November 2022), 2020.
Ciais, P., Rayner, P., Chevallier, F., Bousquet, P., Logan, M., Peylin, P., and Ramonet, M.: Atmospheric inversions for estimating CO2 fluxes: methods and perspectives, Climatic Change, 103, 69–92, https://doi.org/10.1007/s10584-010-9909-3, 2010.
Davis, K. J., Deng, A., Lauvaux, T., Miles, N. L., Richardson, S. J., Sarmiento, D. P., Gurney, K. R., Hardesty, R. M., Bonin, T. A., Brewer, W. A., Lamb, B. K., Shepson, P. B., Harvey, R. M., Cambaliza, M. O., Sweeney, C., Turnbull, J. C., Whetstone, J., and Karion, A.: The Indianapolis Flux Experiment (INFLUX): A test-bed for developing urban greenhouse gas emission measurements, Elem. Sci. Anth., 5, 21, https://doi.org/10.1525/elementa.188, 2017.
Dellaert, S., Super, I., Visschedijk, A., and Denier van der Gon, H. A. C.: High resolution scenarios of CO2 and CO emissions, https://www.che-project.eu/sites/default/files/2019-05/CHE-D4-2-V1-0.pdf (last access: August 2023), 2019.
Deng, A., Lauvaux, T., Davis, K. J., Gaudet, B. J., Miles, N., Richardson, S. J., Wu, K., Sarmiento, D. P., Hardesty, R. M., Bonin, T. A., Brewer, W. A., and Gurney, K. R.: Toward reduced transport errors in a high resolution urban CO2 inversion system, Elem. Sci. Anth., 5, 20, 2017.
Denier van der Gon, H. A. C., Dellaert, S., Super, I., Kuenen, J., and Visschedijk, A.: TNO GHGco emission inventory v3.0: final high resolution emission data 2005–2018, https://verify.lsce.ipsl.fr/images/PublicDeliverables/VERIFY_D2_3_TNO_v1.pdf (last access: August 2023), 2021.
Gourdji, S., Karion, A., Lopez-Coto, I., Ghosh, S., Mueller, K. L., Zhou, Y., Williams, C. A., Baker, I. T., Haynes, K., and Whetstone, J.: A modified Vegetation Photosynthesis and Respiration Model (VPRM) for the eastern USA and Canada, evaluated with comparison to atmospheric observations and other biospheric models, J. Geophys. Res.-Biogeo., 127, e2021JG006290, https://doi.org/10.1029/2021JG006290, 2022.
Greater London Authority (GLA): London Atmospheric Emissions Inventory (LAEI) 2019, https://data.london.gov.uk/dataset/london-atmospheric-emissions-inventory--laei--2019 (last access: August 2023), 2021.
Grell, G. A., Peckham, S. E., Schmitz, R., McKeen, S. A., Frost, G., Skamarock, W. C., and Eder, B.: Fully coupled “online” chemistry within the WRF model, Atmos. Environ., 39, 6957–6975, 2005.
Gurney, K. R., Patarasuk, R., Liang, J., Song, Y., O'Keeffe, D., Rao, P., Whetstone, J. R., Duren, R. M., Eldering, A., and Miller, C.: The Hestia fossil fuel CO2 emissions data product for the Los Angeles megacity (Hestia-LA), Earth Syst. Sci. Data, 11, 1309–1335, https://doi.org/10.5194/essd-11-1309-2019, 2019.
Gurney, K. R., Liang, J., Roest, G., Song, Y., Mueller, K., and Lauvaux, T.: Under-reporting of greenhouse gas emissions in US cities, Nat. Commun., 12, 1–7, https://doi.org/10.1038/s41467-020-20871-0, 2021.
Haeffelin, M., Barthès, L., Bock, O., Boitel, C., Bony, S., Bouniol, D., Chepfer, H., Chiriaco, M., Cuesta, J., Delanoë, J., Drobinski, P., Dufresne, J.-L., Flamant, C., Grall, M., Hodzic, A., Hourdin, F., Lapouge, F., Lemaître, Y., Mathieu, A., Morille, Y., Naud, C., Noël, V., O'Hirok, W., Pelon, J., Pietras, C., Protat, A., Romand, B., Scialom, G., and Vautard, R.: SIRTA, a ground-based atmospheric observatory for cloud and aerosol research, Ann. Geophys., 23, 253–275, https://doi.org/10.5194/angeo-23-253-2005, 2005.
Hall, B. D., Crotwell, A. M., Kitzis, D. R., Mefford, T., Miller, B. R., Schibig, M. F., and Tans, P. P.: Revision of the World Meteorological Organization Global Atmosphere Watch (WMO/GAW) CO2 calibration scale, Atmos. Meas. Tech., 14, 3015–3032, https://doi.org/10.5194/amt-14-3015-2021, 2021.
Huo, D., Huang, X., Dou, X., Ciais, P., Li, Y., Deng, Z., Wang, Y., Cui, D., Benkhelifa, F., and Sun, T.: Carbon Monitor Cities near-real-time daily estimates of CO2 emissions from 1500 cities worldwide, Sci. Data, 9, 533, https://doi.org/10.1038/s41597-022-01657-z, 2022 (data available at: https://cities.carbonmonitor.org/, last access: August 2023).
Jung, M., Henkel, K., Herold, M., and Churkina, G.: Exploiting synergies of global land cover products for carbon cycle modeling, Remote Sens. Environ., 101, 534–553, 2006.
Karion, A., Callahan, W., Stock, M., Prinzivalli, S., Verhulst, K. R., Kim, J., Salameh, P. K., Lopez-Coto, I., and Whetstone, J.: Greenhouse gas observations from the Northeast Corridor tower network, Earth Syst. Sci. Data, 12, 699–717, https://doi.org/10.5194/essd-12-699-2020, 2020.
Kotthaus, S., Haeffelin, M., Drouin, M.-A., Dupont, J.-C., Grimmond, S., Haefele, A., Hervo, M., Poltera, Y., and Wiegner, M.: Tailored Algorithms for the Detection of the Atmospheric Boundary Layer Height from Common Automatic Lidars and Ceilometers (ALC), Remote Sens.-Basel, 12, 3259, 2020.
Kretschmer, R., Gerbig, C., Karstens, U., and Koch, F.-T.: Error characterization of CO2 vertical mixing in the atmospheric transport model WRF-VPRM, Atmos. Chem. Phys., 12, 2441–2458, https://doi.org/10.5194/acp-12-2441-2012, 2012.
Lauvaux, T., Miles, N. L., Deng, A., Richardson, S. J., Cambaliza, M. O., Davis, K. J., Gaudet, B., Gurney, K. R., Huang, J., O'Keeffe, D., Song, Y., Karion, A., Oda, T., Patarasuk, R., Sarmiento, D., Shepson, P., Sweeney, C., Turnbull, J., and Wu, K.: High-resolution atmospheric inversion of urban CO2 emissions during the dormant season of the Indianapolis Flux Experiment (INFLUX), J. Geophys. Res.-Atmos., 121, 5213–5236, 2016.
Lauvaux, T., Gurney, K. R., Miles, N. L., Davis, K. J., Richardson, S. J., Deng, A., Nathan, B. J., Oda, T., Wang, J. A., Hutyra, L., and Turnbull, J.: Policy-Relevant Assessment of Urban CO2 Emissions, Environ. Sci. Technol., 54, 10237–10245, https://doi.org/10.1021/acs.est.0c00343, 2020.
Le Plan Climat de Paris: https://cdn.paris.fr/paris/2020/11/23/ 99f03e85e9f0d542fad72566520c578c.pdf (last access: September 2022), 2020.
Lian, J., Bréon, F.-M., Broquet, G., Zaccheo, T. S., Dobler, J., Ramonet, M., Staufer, J., Santaren, D., Xueref-Remy, I., and Ciais, P.: Analysis of temporal and spatial variability of atmospheric CO2 concentration within Paris from the GreenLITE™ laser imaging experiment, Atmos. Chem. Phys., 19, 13809–13825, https://doi.org/10.5194/acp-19-13809-2019, 2019.
Lian, J., Bréon, F.-M., Broquet, G., Lauvaux, T., Zheng, B., Ramonet, M., Xueref-Remy, I., Kotthaus, S., Haeffelin, M., and Ciais, P.: Sensitivity to the sources of uncertainties in the modeling of atmospheric CO2 concentration within and in the vicinity of Paris, Atmos. Chem. Phys., 21, 10707–10726, https://doi.org/10.5194/acp-21-10707-2021, 2021.
Lian, J., Lauvaux, T., Utard, H., Broquet, G., Bréon, F. M., Ramonet, M., Laurent, O., Albarus, I., Cucchi, K., and Ciais, P.: Assessing the Effectiveness of an Urban CO2 Monitoring Network over the Paris Region through the COVID-19 Lockdown Natural Experiment, Environ. Sci. Technol., 56, 2153–2162, https://doi.org/10.1021/acs.est.1c04973, 2022.
Lopez, M., Schmidt, M., Delmotte, M., Colomb, A., Gros, V., Janssen, C., Lehman, S. J., Mondelain, D., Perrussel, O., Ramonet, M., Xueref-Remy, I., and Bousquet, P.: CO, NOx and 13CO2 as tracers for fossil fuel CO2: results from a pilot study in Paris during winter 2010, Atmos. Chem. Phys., 13, 7343–7358, https://doi.org/10.5194/acp-13-7343-2013, 2013.
Mahadevan, P., Wofsy, S. C., Matross, D. M., Xiao, X., Dunn, A. L., Lin, J. C., Gerbig, C., Munger, J. W., Chow, V. Y., and Gottlieb, E. W.: A satellite-based biosphere parameterization for net ecosystem CO2 exchange: Vegetation Photosynthesis and Respiration Model (VPRM), Global Biogeochem. Cy., 22, GB2005, https://doi.org/10.1029/2006GB002735, 2008.
McKain, K., Wofsy, S. C., Nehrkorn, T., Eluszkiewicz, J., Ehleringer, J. R., and Stephens, B. B.: Assessment of ground-based atmospheric observations for verification of greenhouse gas emissions from an urban region, P. Natl. Acad. Sci. USA, 109, 8423–8428, 2012.
Mueller, K. L., Lauvaux, T., Gurney, K. R., Roest, G., Ghosh, S., Gourdji, S. M., Karion, A., DeCola, P., and Whetstone, J.: An emerging GHG estimation approach can help cities achieve their climate and sustainability goals, Environ. Res. Lett., 16, 084003, https://doi.org/10.1088/1748-9326/ac0f25, 2021.
Nalini, K., Lauvaux, T., Abdallah, C., Lian, J., Ciais, P., Utard, H., Laurent, O., and Ramonet, M.: High-resolution Lagrangian inverse modeling of CO2 emissions over the Paris region during the first 2020 lockdown period, J. Geophys. Res.-Atmos., 127, e2021JD036032, https://doi.org/10.1029/2021JD036032, 2022.
Nielsen-Gammon, J. W., Powell, C. L., Mahoney, M. J., Angevine, W. M., Senff, C. J., White, A., Berkowitz, C., Doran, C., and Knupp, K.: Multisensor estimation of mixing heights over a coastal city, J. Appl. Meteorol. Clim., 47, 27–43, 2008.
Oda, T. and Maksyutov, S.: ODIAC Fossil Fuel CO2 Emissions Dataset (version: ODIAC2020b), Center for Global Environmental Research, National Institute for Environmental Studies [data set], https://doi.org/10.17595/20170411.001, 2015.
Oda, T., Maksyutov, S., and Andres, R. J.: The Open-source Data Inventory for Anthropogenic CO2, version 2016 (ODIAC2016): a global monthly fossil fuel CO2 gridded emissions data product for tracer transport simulations and surface flux inversions, Earth Syst. Sci. Data, 10, 87–107, https://doi.org/10.5194/essd-10-87-2018, 2018.
Sargent, M., Barrera, Y., Nehrkorn, T., Hutyra, L. R., Gately, C. K., Jones, T., McKain, K., Sweeney, C., Hegarty, J., Hardiman, B., Wang, J. A., and Wofsy, S. C.: Anthropogenic and biogenic CO2 fluxes in the Boston urban region, P. Natl. Acad. Sci. USA, 115, 7491–7496, 2018.
Seto, K. C., Dhakal, S., Bigio, A., Blanco, H., Delgado, G. C., Dewar, D., Huang, L., Inaba, A., Kansal, A., Lwasa, S., McMahon, J., Müller, D. B., Murakami, J., Nagendra, H., and Ramaswami, A.: Human settlements, infrastructure and spatial planning, Chap. 12, in: Climate Change 2014: Mitigation of Climate Change. Contribution of Working Group III to the Fifth Assessment Report of the Intergovernmental Panel on Climate Change, edited by: Edenhofer, O., Pichs-Madruga, R., Sokona, Y., Farahani, E., Kadner, S., Seyboth, K., Adler, A., Baum, I., Brunner, S., Eickemeier, P., Kriemann, B., Savolainen, J., Schlömer, S., von Stechow, C., Zwickel, T., and Minx, J. C., Cambridge University Press, Cambridge, United Kingdom and New York, NY, USA, https://doi.org/10.1017/CBO9781107415416.018, 2014.
Staufer, J., Broquet, G., Bréon, F.-M., Puygrenier, V., Chevallier, F., Xueref-Rémy, I., Dieudonné, E., Lopez, M., Schmidt, M., Ramonet, M., Perrussel, O., Lac, C., Wu, L., and Ciais, P.: The first 1 year-long estimate of the Paris region fossil fuel CO2 emissions based on atmospheric inversion, Atmos. Chem. Phys., 16, 14703–14726, https://doi.org/10.5194/acp-16-14703-2016, 2016.
Tarantola, A.: Inverse problem theory and methods for model parameter estimation[M], Society for Industrial and Applied Mathematics, ISBN 978-0-89871-572-9, 2005.
Turner, A. J., Kim, J., Fitzmaurice, H., Newman, C., Worthington, K., Chan, K., Wooldridge, P. J., Köehler, P., Frankenberg, C., and Cohen, R. C.: Observed impacts of COVID-19 on urban CO2 emissions, Geophys. Res. Lett., 47, e2020GL090037, https://doi.org/10.1029/2020GL090037, 2020.
Vogel, F. R., Frey, M., Staufer, J., Hase, F., Broquet, G., Xueref-Remy, I., Chevallier, F., Ciais, P., Sha, M. K., Chelin, P., Jeseck, P., Janssen, C., Té, Y., Groß, J., Blumenstock, T., Tu, Q., and Orphal, J.: XCO2 in an emission hot-spot region: the COCCON Paris campaign 2015, Atmos. Chem. Phys., 19, 3271–3285, https://doi.org/10.5194/acp-19-3271-2019, 2019.
Warm Winter 2020 Team and ICOS Ecosystem Thematic Centre: Warm Winter 2020 ecosystem eddy covariance flux product for 73 stations in FLUXNET-Archive format—release 2022-1 (Version 1.0), ICOS Carbon Portal [data set], https://doi.org/10.18160/2G60-ZHAK, 2022.
Wu, L., Broquet, G., Ciais, P., Bellassen, V., Vogel, F., Chevallier, F., Xueref-Remy, I., and Wang, Y.: What would dense atmospheric observation networks bring to the quantification of city CO2 emissions?, Atmos. Chem. Phys., 16, 7743–7771, https://doi.org/10.5194/acp-16-7743-2016, 2016.
Xueref-Remy, I., Dieudonné, E., Vuillemin, C., Lopez, M., Lac, C., Schmidt, M., Delmotte, M., Chevallier, F., Ravetta, F., Perrussel, O., Ciais, P., Bréon, F.-M., Broquet, G., Ramonet, M., Spain, T. G., and Ampe, C.: Diurnal, synoptic and seasonal variability of atmospheric CO2 in the Paris megacity area, Atmos. Chem. Phys., 18, 3335–3362, https://doi.org/10.5194/acp-18-3335-2018, 2018.
Yadav, V., Ghosh, S., Mueller, K., Karion, A., Roest, G., Gourdji, S. M., Lopez-Coto, I., Gurney, K. R., Parazoo, N., Verhulst, K. R., Kim, J., Prinzivalli, S., Fain, C., Nehrkorn, T., Mountain, M., Keeling, R. F., Weiss, R. F., Duren, R., Miller, C. E., and Whetstone, J.: The impact of COVID-19 on CO2 emissions in the Los Angeles and Washington DC/Baltimore metropolitan areas, Geophys. Res. Lett., 48, e2021GL092744, https://doi.org/10.1029/2021GL092744, 2021.
Yadav, V., Verhulst, K., Duren, R., Thorpe, A., Kim, J., Keeling, R., Weiss, R., Cusworth, D., Mountain, M., Miller, C., and Whetstone, J.: A declining trend of methane emissions in the Los Angeles basin from 2015 to 2020, Environ. Res. Lett., 18, 034004, https://doi.org/10.1088/1748-9326/acb6a9, 2023.