the Creative Commons Attribution 4.0 License.
the Creative Commons Attribution 4.0 License.
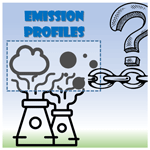
A new steady-state gas–particle partitioning model of polycyclic aromatic hydrocarbons: implication for the influence of the particulate proportion in emissions
Fu-Jie Zhu
Peng-Tuan Hu
Wan-Li Ma
Gas–particle (G–P) partitioning is a crucial atmospheric process for semi-volatile organic compounds (SVOCs), particularly polycyclic aromatic hydrocarbons (PAHs). However, accurately predicting the G–P partitioning of PAHs has remained a challenge. In this study, we established a new steady-state G–P partitioning model based on the level-III multimedia fugacity model, with a particular focus on the particulate proportion (ϕ0) of PAHs in emissions. Similar to previous steady-state models, our new model divided the G–P partitioning behavior into three domains based on the threshold values of log KOA (octanol–air partitioning coefficient), with slopes of 1, from 1 to 0, and 0 for the three domains. However, our model differed significantly from previous models in different domains. We found that deviations from the equilibrium-state G–P partitioning models were caused by both gaseous interference and particulate interference, with ϕ0 determining the influence of this interference. Different forms of the new steady-state model were observed under different values of ϕ0, highlighting its significant impact on the G–P partitioning of PAHs. Comparison of the G–P partitioning of PAHs between the prediction results of our new steady-state model and monitored results from 11 cities in China suggested varying prediction performances under different values of ϕ0, with the lowest root mean square error observed when ϕ0 was set to 0.9 or 0.99. The results indicated that the ϕ0 was a crucial factor for the G–P partitioning of PAHs. Furthermore, our new steady-state model also demonstrated excellent performance in predicting the G–P partitioning of PAHs with entirely gaseous emission and polybrominated diphenyl ethers with entirely particulate emission. Therefore, we concluded that the ϕ0 should be considered in the study of G–P partitioning of PAHs, which also provided a new insight into other SVOCs.
- Article
(1780 KB) -
Supplement
(4774 KB) - BibTeX
- EndNote
The phenomenon of long-range atmospheric transport is capable of transporting semi-volatile organic compounds (SVOCs) from their sources to remote regions, such as the Arctic and the Tibetan Plateau, where they are neither produced nor utilized (Hung et al., 2005, 2010; C. Wang et al., 2018). The gas–particle (G–P) partitioning of SVOCs is an important atmospheric process that governs their fate and long-range transport (Zhao et al., 2020; Li et al., 2015). Furthermore, the distribution between the gas and particle phases plays a pivotal role in controlling the wet and dry depositions of SVOCs, thereby impacting the efficiency and extent of their long-range transport from sources to remote regions (Bidleman, 1988). Furthermore, the G–P partitioning of SVOCs is a significant issue for human exposure assessment, as gaseous and particulate SVOCs enter the human body through different routes (Weschler et al., 2015; Hu et al., 2021).
The G–P partitioning of SVOCs has been the subject of extensive research for several decades. Various models have been developed to predict the G–P partitioning coefficient (KP) of SVOCs (Zhu et al., 2022; Qiao et al., 2020). Qiao et al. (2020) recently categorized eight G–P partitioning models into three groups: (1) models based on equilibrium-state theory (Pankow, 1987; Harner and Bidleman, 1998; Dachs and Eisenreich, 2000; Goss, 2005), (2) empirical models based on monitoring data (Li and Jia, 2014; Wei et al., 2017; Shahpoury et al., 2016), and (3) models based on steady-state theory (Li et al., 2015). Additionally, a new empirical model (equation) for polycyclic aromatic hydrocarbons (PAHs) (Zhu et al., 2022) and a new steady-state mass balance model for polybrominated diphenyl ethers (PBDEs) (Zhao et al., 2020) have recently been established. These models have been evaluated using field monitoring programs (Vuong et al., 2020; Qiao et al., 2019) and are frequently used to predict the G–P partitioning behavior of SVOCs (Qiao et al., 2020).
The G–P partitioning process of PAHs is more complex than that of other SVOCs due to concurrent particle formation (Dachs and Eisenreich, 2000; Shahpoury et al., 2016; Zhu et al., 2022). For instance, when the octanol–air partitioning coefficient (log KOA) exceeds 12, the monitored values of KP-M (monitoring data of G–P partitioning) of PAHs deviate from the predictions of both equilibrium-state and steady-state G–P partitioning models (Ma et al., 2020; Zhu et al., 2022). Recent studies have found that the particulate proportion (ϕ0) of SVOCs in the emissions could affect the G–P partitioning of SVOCs (Qin et al., 2021; Zhao et al., 2020). As ϕ0 increases, the predictions can diverge from the steady-state G–P partitioning model to the equilibrium-state G–P partitioning model (Qin et al., 2021; Zhao et al., 2020). Moreover, the emission sources of PAHs in the atmosphere are complex, including stationary sources (residential combustion, industrial production, and agricultural burning) and mobile sources (motor vehicles, railways, and shipping) (Zhang et al., 2020; Tang et al., 2020), in which both gaseous and particulate PAHs exist (Zimmerman et al., 2019; R. Wang et al., 2018; Shen et al., 2011; Cai et al., 2018b). Therefore, the detailed influence of ϕ0 on the G–P partitioning of PAHs could be considered to explain the deviation of the measured KP-M from both the equilibrium-state and the steady-state G–P partitioning model predictions.
In this study, we establish a new steady-state G–P partitioning model (hereafter referred to as the new steady-state model) based on the level-III multimedia fugacity model for PAHs and comprehensively discuss the influence of ϕ0. Specifically, we (1) establish and deeply study the new steady-state model under different threshold values of log KOA, (2) comprehensively discuss the influence of ϕ0 on the G–P partitioning of PAHs, and (3) study the performance of the new steady-state model for prediction of KP of PAHs.
2.1 Establishment method of the new steady-state model
In the current study, a steady-state six-compartment six-fugacity model was employed. The intricacies of this model can be found in Text S1 of the Supplement. The input and output fluxes of PAHs in both the gaseous and the particulate phases were graphically depicted in Fig. 1. The comprehensive computational techniques utilized to determine these fluxes are elaborated upon in Text S2.
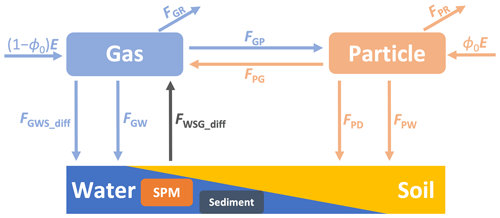
Figure 1The fluxes related to the gas and particle phase in the six-compartment model. FGR: degradation flux of gas-phase PAHs, FPR: degradation flux of particle-phase PAHs, FGP: migration flux from the gas phase to the particle phase, FPG: migration flux from the particle phase to the gas phase, FGWS_diff: diffusion fluxes from the gas phase to water and/or soil phases, FGW: wet deposition flux of gas-phase PAHs to water and/or soil phases, FWSG_diff: diffusion fluxes from soil and/or water phases to the gas phase, FPD: dry deposition flux of particle-phase PAHs to suspended particle matter (SPM) in water phase and/or soil phase, FPW: wet deposition flux of particle-phase PAHs to SPM and/or soil phases, (1−ϕ0)E: emission flux of gas-phase PAHs, and ϕ0E: emission flux of particle-phase PAHs.
Four groups were compared in terms of gas-phase and particle-phase input and output fluxes, namely input fluxes of the gas phase, output fluxes of the gas phase, input fluxes of the particle phase, and output fluxes of the particle phase. The results for PAHs were illustrated in Fig. S1. In order to establish a universal and concise model, the four fluxes (FGWS_diff, FWSG_diff, FPR, and FGW) were excluded from the system as their contributions were less than 10 % of the total fluxes. Furthermore, the special situation was not taken into account. For instance, even if the contribution of the flux of FGW for dibenzo[a,h]anthracene (DahA) exceeded 10 %, it was still removed. After simplifying the function in Text S1, the two linear equations describing the input and output fluxes of the gas phase and the particle phase were established as follows:
where fP is the fugacity for particle-phase PAHs; fG is the fugacity for gas-phase PAHs; DGP is the intermedia D value between the gas phase and the particle phase; DGR is the D value for the degradation of gas-phase PAHs; and DPD and DPW are the D values of the dry and wet depositions of particle-phase PAHs, respectively.
The fugacity ratio of the particle phase to the gas phase can be obtained by solving Eq. (1) as follows:
According to the fugacity method (Li et al., 2015) (see details in Text S3), the new steady-state model can be expressed as follows:
In Eq. (3), log KP-HB is the equilibrium-state G–P partitioning model (named the H–B model in this study, ; fOM is the fraction of organic matter in particles, and KOA is the octanol–air partitioning coefficient) (Harner and Bidleman, 1998). DGR, caused by the degradation of PAHs in the gas phase, is defined as the gaseous interference, and DPD+DPW, caused by the deposition of PAHs in the particle phase, is defined as the particulate interference. The magnitude of this interference is determined by the value of ϕ0.
By applying the calculation method of the D values in the multimedia fugacity model (Table S1) and the values of the related parameters in Tables S2, S3, S4, S5, and S6, Eq. (2) can be simplified as follows:
where kdeg is the degradation rate of PAHs in the gas phase (h−1).
Therefore, Eq. (3) can also be expressed as follows:
Thus, it can be found that the new steady-state model (log KP-NS) is a function of ϕ0, kdeg, fOM, and KOA.
2.2 Different domains of the new steady-state model
Three domains have been delineated based on the threshold values of log KOA. For example, if 10, the initial threshold of log KOA (log KOA1) can be derived. Subsequently, Eq. (5) can be expressed as follows:
In this domain, the value of log KOA was less than log KOA1, and log KP-NS was a function of KOA, fOM, ϕ0, and kdeg. As depicted in Fig. 2, the domain was illustrated with vertical lines serving as the backdrop. Notably, the prediction line of the new steady-state model was parallel to that of the H–B model within this domain.
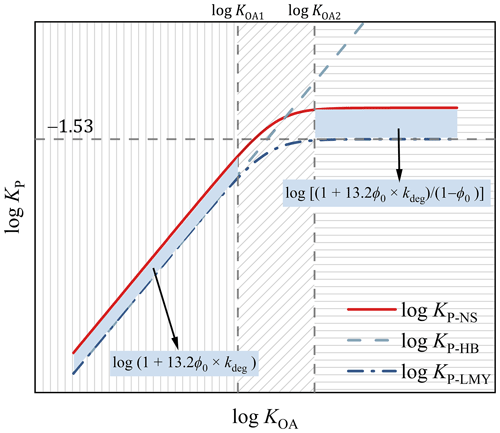
Figure 2The three domains of the new steady-state G–P partitioning model divided by the two threshold values of log KOA.
In addition, if 10, the secondary threshold of log KOA (log KOA2) can be determined. Eq. (5) can be expressed as follows:
Through substitution of log KP-HB using the equation 1 as proposed by Harner and Bidleman (1998), Eq. (7) can be simplified as follows:
Within this domain, the value of log KOA was higher than log KOA2; log KP-NS was solely dependent on ϕ0 and kdeg; and log KP-NS reached a maximum constant value (log KP-NSmax), as depicted by the section with horizontal lines in Fig. 2. Within this domain, the prediction line of the new steady-state model was parallel to that of the L–M–Y model.
Moreover, in the range where , log KP-NS exhibited a positive correlation with log KOA, with a decreasing slope from 1 to 0 (Eq. 5). Within this domain, log KP-NS was influenced by several factors, including KOA, fOM, ϕ0, and kdeg. This particular range is depicted in Fig. 2 with a background of diagonal lines. Notably, within this domain, the prediction line of the new steady-state model closely resembled that of the L–M–Y model.
2.3 Difference between the new steady-state model and previous models
The dissimilarity between the new steady-state model and the H–B (Text S4) and L–M–Y models (the steady-state model) (Li et al., 2015) (Text S4) can be computed using Eq. (5) in different domains. In essence, as shown in Fig. 2, when log KOA<log KOA1, the contrast between the new steady-state model and the H–B model or the L–M–Y model can be denoted as . The value of δ1 increased along with the increase in ϕ0 and reached the maximum value of log (1+13.2kdeg) when ϕ0=1 (Fig. S2a). When log KOA>log KOA2, the difference between the new steady-state model and the L–M–Y model can be expressed as . The value of δ2 also increased along with the increase in ϕ0 and approached infinity when ϕ0 is infinitely close to 1 (Fig. S2b). When , the difference between the new steady-state model and the L–M–Y model was the function of ϕ0 and KOA, which increased along with the increase in ϕ0 and KOA. Further information can be found in the subsequent section.
In general, varying values of ϕ0 correspond to distinct configurations of the new steady-state model (Eq. 3). Specifically, three different forms can be obtained depending on the values of ϕ0: , ϕ0=0, and ϕ0=1.
When , both the particulate and the gaseous PAHs are present in the emission, and the new steady-state model is expressed as Eq. (3). In this form, it is necessary to consider both gaseous interference and particulate interference for the G–P partitioning of PAHs in the atmosphere. The deviation of the new steady-state model from the H–B model depends on the ratio of ϕ0DGR to . When the ratio exceeds 1, log KP-NS deviates upwards from the prediction of the H–B model, whereas log KP-NS deviates downwards when the ratio is lower than 1.
When ϕ0=0, the PAHs in the emission are entirely in the form of gaseous PAHs, and Eq. (3) can be expressed as follows:
Indeed, this equation is identical to that of the L–M–Y model, wherein α is defined as (Li et al., 2015).
When ϕ0=1, the PAHs in the emission are entirely in the form of particulate PAHs, and Eq. (3) can be expressed as follows:
The disparity of the new steady-state model from the H–B model can primarily be attributed to the degradation of PAHs in the gas phase. In cases where kdeg is negligible, the new steady-state model is equivalent to the H–B model.
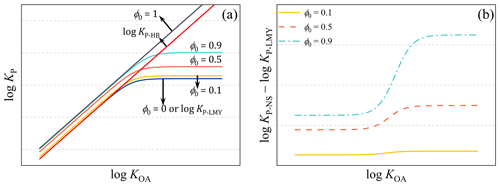
Figure 3The comparison between the new steady-state model and the H–B model and the L–M–Y model. (a) The prediction lines of the three models; (b) the difference between the new steady-state model and the L–M–Y model with different values of ϕ0.
The impact of ϕ0 on KP-NS of PAHs was investigated by analyzing different values of ϕ0, and the results are presented in Fig. 3. As depicted in Fig. 3a, the prediction line of the new steady-state model diverged from the L–M–Y model towards the H–B model as ϕ0 increased, which was consistent with previous studies (Zhao et al., 2020; Qin et al., 2021). In addition, obvious differences were observed between the prediction lines for the three models. Notably, when ϕ0=1, the line of log KP-NS was parallel to the line of log KP-HB. When ϕ0=0, the prediction line of log KP-NS was identical to that of log KP-LMY. When , the trend of the prediction lines of log KP-NS was similar to that of log KP-LMY. The deviation between the prediction lines of log KP-NS and log KP-LMY is illustrated in Fig. 3b. Generally, the deviations between the prediction lines varied with the values of ϕ0 and log KOA. Additionally, the deviation increased with the increase in ϕ0 and exhibited three distinct trends with the increase in log KOA, separated by the two threshold values of log KOA (log KOA1 and log KOA2).
4.1 Validation
As is widely acknowledged, the sources of atmospheric PAH emission are multifaceted, encompassing both stationary sources and mobile sources (Zhang et al., 2020). Moreover, varying proportions of particulate PAHs have been reported across different emission sources (Zimmerman et al., 2019; R. Wang et al., 2018; Shen et al., 2011; Cai et al., 2018b). As a result, determining precise values of ϕ0 is no easy feat. In this section, we consider different values of ϕ0 (0, 0.1, 0.5, 0.9, 0.99, and 1) in conjunction with the new steady-state model for predicting KP-M of PAHs, in order to obtain representative results.
To assess the performance of the new steady-state model, the monitored log KP-M values of PAHs from 11 cities across China were utilized (Ma et al., 2018, 2019, 2020). As depicted in Fig. 4, the prediction line of the new steady-state model exhibited a remarkable concurrence with the monitoring data of log KP-M. Notably, for the monitoring data with high log KOA, the data were predominantly distributed between the prediction lines of the steady-state model with the values of ϕ0 from 0 to 1. Furthermore, for different cities (Fig. S3), the values of ϕ0 for the best-matched prediction lines of the new steady-state model varied, which was anticipated, since the sources of PAHs also differed among the 11 cities. The degree of concurrence of the new steady-state model was also evaluated using the root mean square error (RMSE) method (Text S5). Generally, for PAHs with high values of log KOA (such as the high-molecular-weight PAHs), when ϕ0 was set to 0.9 or 0.99, the value of RMSE for each city was the lowest (Fig. S4), indicating the best degree of concurrence between the prediction results and the monitoring results. In fact, previous studies have shown that high-molecular-weight PAHs were dominant in the particle phase in emissions with higher ϕ0 (Shen et al., 2011; Mastral et al., 1996; Lu et al., 2009), which lends credence to our findings.
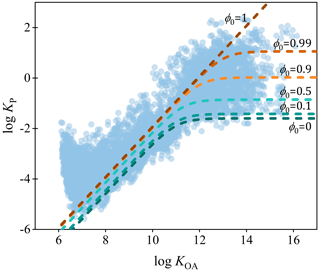
Figure 4The comparison between the monitored data of log KP-M of PAHs from 11 cities in China and the prediction lines of the new steady-state model with different values of ϕ0.
Moreover, the performance of the new steady-state model in predicting log KP-M of PAHs in a special scenario was also examined. Notably, in the prototype coking plant, the dust removal efficiency was an impressive 96 % (Liu et al., 2019). In this scenario, the gaseous PAHs were the primary source of emissions, and the values of ϕ0 were approximately 0. As illustrated in Fig. S5, the monitored data of log KP-M from the coking plant aligned most closely with the prediction line of the new steady-state model with ϕ0=0, exhibiting the lowest RMSE. Based on this comparison, the optimal ϕ0 in the steady-state model was consistent with that in the emission profile. This finding underscored the exceptional performance of the new steady-state model in this unique scenario.
It is possible to extend the steady-state model to other SVOCs by taking into account their comparable partitioning characteristics, while the model was originally developed based on the parameters of PAHs. To validate the performance of the new steady-state model for other SVOCs, a special scenario involving the recycling of electrical and electronic waste (e-waste) sites was considered. In this case, PBDEs were predominantly found in the particle phase of emissions, and the value of ϕ0 was estimated to be approximately 1 (Cai et al., 2018a). Fig. S6 depicts the comparison between the monitored data of log KP-M from several e-waste sites (Tian et al., 2011; Han et al., 2009; Chen et al., 2011) and the prediction lines of the new steady-state model with varying values of ϕ0 (0, 0.1, 0.5, 0.9, 0.99, and 1). The corresponding results for RMSE are presented in Fig. S7. Notably, the monitored data of log KP-M exhibited the best agreement with the prediction line of the new steady-state model with ϕ0=1, which also had the lowest values of RMSE. Thus, it can be inferred that the new steady-state model can be expanded to predict the KP-M of PBDEs in e-waste sites.
4.2 Implication
The present study has introduced a new steady-state G–P partitioning model, which incorporates the particulate proportion of SVOCs in emissions. In essence, the study has shed new light on the field of G–P partitioning and other related disciplines involving SVOCs. Firstly, in cases where SVOCs in the atmosphere originate from diverse emission sources with varying ϕ0, the new steady-state model is more appropriate for the G–P partitioning study and other related assessments, such as those pertaining to health risks. Secondly, when examining the pollution characteristics and regional transport of SVOCs from a single point source, such as the transport of PBDEs around an e-waste site or the transport of SVOCs around chemical factories, the G–P partitioning of SVOCs must account for the particulate fraction of SVOCs in emissions. Thirdly, for long-range atmospheric transport studies, if there are multiple sources of SVOCs along the transport way, the continuous impact of the particulate fraction of SVOCs in emissions on the transport and fate of SVOCs needs careful consideration, such as the development of an atmospheric transport model.
4.3 Limitation
In light of the foregoing discussion, it can be inferred that the new steady-state model exhibited commendable performance in predicting KP-M of PAHs in diverse real-world atmospheres, thereby providing a fresh avenue for investigating the G–P partitioning of PAHs and other SVOCs. Nonetheless, certain limitations of the new steady-state model persisted in the present study. Firstly, the values of ϕ0 varied across different compounds and different emission sources (Zimmerman et al., 2019; R. Wang et al., 2018; Shen et al., 2011; Cai et al., 2018b). In the present study, constant values of ϕ0 were employed for the new steady-state model, which were merely considered to be special examples. The precise values of ϕ0 should be utilized for the application of the new steady-state model in the future. Secondly, for kdeg and fOM, only one constant and common value was employed for the new steady-state model. Generally, these two parameters were also complex in the real atmosphere. For example, kdeg was related not only to the physicochemical properties of chemicals, but also to the environmental parameters, such as temperature and concentration (Wilson et al., 2021). Moreover, even though the fOM can be directly measured, the actual values of fOM also fluctuated with various factors, such as emission sources (Gaga and Ari, 2019; Lohmann and Lammel, 2004) and particle sizes (Hu et al., 2020). To evaluate the impact of the three parameters on KP-NS in the new steady-state model, the sensitivity analysis was conducted via a Monte Carlo analysis with 100 000 trials employing the commercial software package Oracle Crystal Ball. To obtain comprehensive results, the sensitivity analysis was conducted for different values of log KOA from 6 to 16. As presented in Fig. S8, it is noteworthy that three different ranges of log KOA were observed based on different characteristics. For the range of log KOA from 6 to 10, the influence of ϕ0 dominated followed by kdeg and fOM. Furthermore, for each parameter, the influence remained stable for different log KOA values in this range. For the range of log KOA from 10 to 12, the influence of ϕ0 dominated followed by kdeg and fOM. Additionally, the influence of ϕ0 increased, while for the other two parameters the influence decreased. In the third range of log KOA (12 to 16), the influences of the three parameters remained stable. Moreover, the influence of ϕ0 dominated, and the influence of fOM can be disregarded. In fact, the three ranges of log KOA were consistent with the three domains. It can be concluded that the different influences of the three parameters on KP-NS for different log KOA values should be considered for the new model. Therefore, the precise values of ϕ0, kdeg, and fOM for the real atmosphere should be employed for the application of the new steady-state model in the future.
Furthermore, the new steady-state model was established based on a single multimedia environment, in which the advections of air and water were not considered. Additionally, some fluxes were removed to simplify the parameters of the model. Therefore, the influence of all fluxes and parameters related to gas and particle compartments should comprehensively be evaluated in the future. Furthermore, the validation and implication of the new steady-state G–P partitioning model should also be conducted for other SVOCs in a real multimedia environment.
Code is available upon request to the corresponding author.
Data are available upon request to the corresponding author.
The supplement related to this article is available online at: https://doi.org/10.5194/acp-23-8583-2023-supplement.
FJZ: methodology, investigation, writing (original draft preparation). PTH: writing (review and editing). WLM: conceptualization, methodology, writing (review and editing).
The contact author has declared that none of the authors has any competing interests.
Publisher’s note: Copernicus Publications remains neutral with regard to jurisdictional claims in published maps and institutional affiliations.
This research has been supported by the Heilongjiang Touyan Innovation Team Program, China.
This research has been supported by the National Natural Science Foundation of China (grant nos. 41671470 and 42077341). This research has been partially supported by the State Key Laboratory of Urban Water Resource and Environment, Harbin Institute of Technology (grant no. 2023TS18), and the Heilongjiang Provincial Natural Science Foundation of China (grant no. YQ2020D004).
This paper was edited by Leiming Zhang and reviewed by two anonymous referees.
Bidleman, T. F.: Atmospheric processes wet and dry deposition of organic compounds are controlled by their vapor-particle partitioning, Environ. Sci. Technol., 22, 361–367, https://doi.org/10.1021/es00169a002, 1988.
Cai, C., Yu, S., Liu, Y., Tao, S., and Liu, W.: PBDE emission from E-wastes during the pyrolytic process: Emission factor, compositional profile, size distribution, and gas-particle partitioning, Environ. Pollut., 235, 419–428, https://doi.org/10.1016/j.envpol.2017.12.068, 2018a.
Cai, C., Yu, S., Li, X., Liu, Y., Tao, S., and Liu, W.: Emission characteristics of polycyclic aromatic hydrocarbons from pyrolytic processing during dismantling of electronic wastes, J. Hazard. Mater., 351, 270–276, https://doi.org/10.1016/j.jhazmat.2018.03.012, 2018b.
Chen, D., Bi, X., Liu, M., Huang, B., Sheng, G., and Fu, J.: Phase partitioning, concentration variation and risk assessment of polybrominated diphenyl ethers (PBDEs) in the atmosphere of an e-waste recycling site, Chemosphere, 82, 1246–1252, https://doi.org/10.1016/j.chemosphere.2010.12.035, 2011.
Dachs, J. and Eisenreich, S. J.: Adsorption onto aerosol soot carbon dominates gas-particle partitioning of polycyclic aromatic hydrocarbons, Environ. Sci. Technol., 34, 3690–3697, https://doi.org/10.1021/es991201+, 2000.
Gaga, E. O. and Ari, A.: Gas-particle partitioning and health risk estimation of polycyclic aromatic hydrocarbons (PAHs) at urban, suburban and tunnel atmospheres: Use of measured EC and OC in model calculations, Atmos. Pollut. Res., 10, 1–11, https://doi.org/10.1016/j.apr.2018.05.004, 2019.
Goss, K.-U.: Predicting the equilibrium partitioning of organic compounds using just one linear solvation energy relationship (LSER), Fluid Phase Equilibr., 233, 19–22, https://doi.org/10.1016/j.fluid.2005.04.006, 2005.
Han, W., Feng, J., Gu, Z., Chen, D., Wu, M., and Fu, J.: Polybrominated Diphenyl Ethers in the Atmosphere of Taizhou, a Major E-Waste Dismantling Area in China, Bull. Environ. Contam. Toxicol., 83, 783–788, https://doi.org/10.1007/s00128-009-9855-9, 2009.
Harner, T. and Bidleman, T. F.: Octanol-air partition coefficient for describing particle/gas partitioning of aromatic compounds in urban air, Environ. Sci. Technol., 32, 1494–1502, https://doi.org/10.1021/es970890r, 1998.
Hu, P.-T., Su, P.-H., Ma, W.-L., Zhang, Z.-F., Liu, L.-Y., Song, W.-W., Qiao, L.-N., Tian, C.-G., Macdonald, R. W., Nikolaev, A., Cao, Z.-G., and Li, Y.-F.: New equation to predict size-resolved gas-particle partitioning quotients for polybrominated diphenyl ethers, J. Hazard. Mater., 400, 123245, https://doi.org/10.1016/j.jhazmat.2020.123245, 2020.
Hu, P.-T., Ma, W.-L., Zhang, Z.-F., Liu, L.-Y., Song, W.-W., Cao, Z.-G., Macdonald, R. W., Nikolaev, A., Li, L., and Li, Y.-F.: Approach to Predicting the Size-Dependent Inhalation Intake of Particulate Novel Brominated Flame Retardants, Environ. Sci. Technol., 55, 15236–15245, https://doi.org/10.1021/acs.est.1c03749, 2021.
Hung, H., Blanchard, P., Halsall, C. J., Bidleman, T. F., Stern, G. A., Fellin, P., Muir, D. C. G., Barrie, L. A., Jantunen, L. M., Helm, P. A., Ma, J., and Konoplev, A.: Temporal and spatial variabilities of atmospheric polychlorinated biphenyls (PCBs), organochlorine (OC) pesticides and polycyclic aromatic hydrocarbons (PAHs) in the Canadian Arctic: Results from a decade of monitoring, Sci. Total Environ., 342, 119–144, https://doi.org/10.1016/j.scitotenv.2004.12.058, 2005.
Hung, H., Kallenborn, R., Breivik, K., Su, Y., Brorström-Lundén, E., Olafsdottir, K., Thorlacius, J. M., Leppänen, S., Bossi, R., Skov, H., Manø, S., Patton, G. W., Stern, G., Sverko, E., and Fellin, P.: Atmospheric monitoring of organic pollutants in the Arctic under the Arctic Monitoring and Assessment Programme (AMAP): 1993–2006, Sci. Total Environ., 408, 2854–2873, https://doi.org/10.1016/j.scitotenv.2009.10.044, 2010.
Li, Y. F. and Jia, H. L.: Prediction of gas/particle partition quotients of Polybrominated Diphenyl Ethers (PBDEs) in north temperate zone air: An empirical approach, Ecotox. Environ. Safe., 108, 65–71, https://doi.org/10.1016/j.ecoenv.2014.05.028, 2014.
Li, Y.-F., Ma, W.-L., and Yang, M.: Prediction of gas/particle partitioning of polybrominated diphenyl ethers (PBDEs) in global air: A theoretical study, Atmos. Chem. Phys., 15, 1669–1681, https://doi.org/10.5194/acp-15-1669-2015, 2015.
Liu, X., Zhao, D., Peng, L., Bai, H., Zhang, D., and Mu, L.: Gas–particle partition and spatial characteristics of polycyclic aromatic hydrocarbons in ambient air of a prototype coking plant, Atmos. Environ., 204, 32–42, https://doi.org/10.1016/j.atmosenv.2019.02.012, 2019.
Lohmann, R. and Lammel, G.: Adsorptive and Absorptive Contributions to the Gas-Particle Partitioning of Polycyclic Aromatic Hydrocarbons: State of Knowledge and Recommended Parametrization for Modeling, Environ. Sci. Technol., 38, 3793–3803, https://doi.org/10.1021/es035337q, 2004.
Lu, H., Zhu, L., and Zhu, N.: Polycyclic aromatic hydrocarbon emission from straw burning and the influence of combustion parameters, Atmos. Environ., 43, 978–983, https://doi.org/10.1016/j.atmosenv.2008.10.022, 2009.
Ma, W., Zhu, F., Hu, P., Qiao, L., and Li, Y.: Gas/particle partitioning of PAHs based on equilibrium-state model and steady-state model, Sci. Total Environ., 706, 136029, https://doi.org/10.1016/j.scitotenv.2019.136029, 2020.
Ma, W. L., Liu, L. Y., Jia, H. L., Yang, M., and Li, Y. F.: PAHs in Chinese atmosphere Part I: Concentration, source and temperature dependence, Atmos. Environ., 173, 330–337, https://doi.org/10.1016/j.atmosenv.2017.11.029, 2018.
Ma, W.-L., Zhu, F.-J., Liu, L.-Y., Jia, H.-L., Yang, M., and Li, Y.-F.: PAHs in Chinese atmosphere: Gas/particle partitioning, Sci. Total Environ., 693, 133623, https://doi.org/10.1016/j.scitotenv.2019.133623, 2019.
Mastral, A. M., Callén, M., and Murillo, R.: Assessment of PAH emissions as a function of coal combustion variables, Fuel, 75, 1533–1536, https://doi.org/10.1016/0016-2361(96)00120-2, 1996.
Pankow, J. F.: Review and comparative analysis of the theories on partitioning between the gas and aerosol particulate phases in the atmosphere, Atmos. Environ., 21, 2275–2283, https://doi.org/10.1016/0004-6981(87)90363-5, 1987.
Qiao, L., Zhang, Z., Liu, L., Song, W., Ma, W., Zhu, N., and Li, Y.: Measurement and modeling the gas/particle partitioning of organochlorine pesticides (OCPs) in atmosphere at low temperatures, Sci. Total Environ., 667, 318–324, https://doi.org/10.1016/j.scitotenv.2019.02.347, 2019.
Qiao, L., Hu, P., Macdonald, R., Kannan, K., Nikolaev, A., and Li, Y.-f.: Modeling gas/particle partitioning of polybrominated diphenyl ethers (PBDEs) in the atmosphere: A review, Sci. Total Environ., 729, 138962, https://doi.org/10.1016/j.scitotenv.2020.138962, 2020.
Qin, M., Yang, P., Hu, P., Hao, S., Macdonald, R. W., and Li, Y.: Particle/gas partitioning for semi-volatile organic compounds (SVOCs) in level III multimedia fugacity models: Both gaseous and particulate emissions, Sci. Total Environ., 790, 148012, https://doi.org/10.1016/j.scitotenv.2021.148012, 2021.
Shahpoury, P., Lammel, G., Albinet, A., Sofuoglu, A., Dumanoglu, Y., Sofuoglu, S. C., Wagner, Z., and Zdimal, V.: Evaluation of a conceptual model for gas-particle partitioning of polycyclic aromatic hydrocarbons using polyparameter linear free energy relationships, Environ. Sci. Technol., 50, 12312–12319, https://doi.org/10.1021/acs.est.6b02158, 2016.
Shen, G., Wang, W., Yang, Y., Ding, J., Xue, M., Min, Y., Zhu, C., Shen, H., Li, W., Wang, B., Wang, R., Wang, X., Tao, S., and Russell, A. G.: Emissions of PAHs from indoor crop residue burning in a typical rural stove: Emission factors, size distributions, and gas-particle partitioning, Environ. Sci. Technol., 45, 1206–1212, https://doi.org/10.1021/es102151w, 2011.
Tang, T., Cheng, Z., Xu, B., Zhang, B., Zhu, S., Cheng, H., Li, J., Chen, Y., and Zhang, G.: Triple Isotopes (delta C-13, delta H-2, and Delta C-4) Compositions and Source Apportionment of Atmospheric Naphthalene: A Key Surrogate of Intermediate-Volatility Organic Compounds (IVOCs), Environ. Sci. Technol., 54, 5409–5418, https://doi.org/10.1021/acs.est.0c00075, 2020.
Tian, M., Chen, S.-J., Wang, J., Zheng, X.-B., Luo, X.-J., and Mai, B.-X.: Brominated Flame Retardants in the Atmosphere of E-Waste and Rural Sites in Southern China: Seasonal Variation, Temperature Dependence, and Gas-Particle Partitioning, Environ. Sci. Technol., 45, 8819–8825, https://doi.org/10.1021/es202284p, 2011.
Vuong, Q. T., Thang, P. Q., Nguyen, T. N. T., Ohura, T., and Choi, S. D.: Seasonal variation and gas/particle partitioning of atmospheric halogenated polycyclic aromatic hydrocarbons and the effects of meteorological conditions in Ulsan, South Korea, Environ. Pollut., 263, 114529, https://doi.org/10.1016/j.envpol.2020.114592, 2020.
Wang, C., Wang, X., Gong, P., and Yao, T.: Long-term trends of atmospheric organochlorine pollutants and polycyclic aromatic hydrocarbons over the southeastern Tibetan Plateau, Sci. Total Environ., 624, 241–249, https://doi.org/10.1016/j.scitotenv.2017.12.140, 2018.
Wang, R., Liu, G., Sun, R., Yousaf, B., Wang, J., Liu, R., and Zhang, H.: Emission characteristics for gaseous- and size-segregated particulate PAHs in coal combustion flue gas from circulating fluidized bed (CFB) boiler, Environ. Pollut., 238, 581–589, https://doi.org/10.1016/j.envpol.2018.03.051, 2018.
Wei, X., Yuan, Q., Serge, B., Xu, T., Ma, G., and Yu, H.: In silico investigation of gas/particle partitioning equilibrium of polybrominated diphenyl ethers (PBDEs), Chemosphere, 188, 110–118, https://doi.org/10.1016/j.chemosphere.2017.08.146, 2017.
Weschler, C. J., Beko, G., Koch, H. M., Salthammer, T., Schripp, T., Toftum, J., and Clausen, G.: Transdermal Uptake of Diethyl Phthalate and Di(n-butyl) Phthalate Directly from Air: Experimental Verification, Environ. Health Perspect., 123, 928–934, https://doi.org/10.1289/ehp.1409151, 2015.
Wilson, J., Pöschl, U., Shiraiwa, M., and Berkemeier, T.: Non-equilibrium interplay between gas–particle partitioning and multiphase chemical reactions of semi-volatile compounds: mechanistic insights and practical implications for atmospheric modeling of polycyclic aromatic hydrocarbons, Atmos. Chem. Phys., 21, 6175–6198, https://doi.org/10.5194/acp-21-6175-2021, 2021.
Zhang, L., Yang, L., Zhou, Q., Zhang, X., Xing, W., Wei, Y., Hu, M., Zhao, L., Toriba, A., Hayakawa, K., and Tang, N.: Size distribution of particulate polycyclic aromatic hydrocarbons in fresh combustion smoke and ambient air: A review, J. Environ. Sci., 88, 370–384, https://doi.org/10.1016/j.jes.2019.09.007, 2020.
Zhao, F., Riipinen, I., and MacLeod, M.: Steady-state mass balance model for predicting particle–gas concentration ratios of PBDEs, Environ. Sci. Technol., 55, 9425–9433, https://doi.org/10.1021/acs.est.0c04368, 2020.
Zhu, F.-J., Ma, W.-L., Zhang, Z.-F., Yang, P.-F., Hu, P.-T., Liu, L.-Y., and Song, W.-W.: Prediction of the gas/particle partitioning quotient of PAHs based on ambient temperature, Sci. Total Environ., 811, 151411, https://doi.org/10.1016/j.scitotenv.2021.151411, 2022.
Zimmerman, N., Rais, K., Jeong, C.-H., Pant, P., Mari Delgado-Saborit, J., Wallace, J. S., Evans, G. J., Brook, J. R., and Pollitt, K. J. G.: Carbonaceous aerosol sampling of gasoline direct injection engine exhaust with an integrated organic gas and particle sampler, Sci. Total Environ., 652, 1261–1269, https://doi.org/10.1016/j.scitotenv.2018.10.332, 2019.
- Abstract
- Introduction
- Establishment of the new steady-state G–P partitioning model
- Influence of ϕ0 on KP of PAHs
- Validation of the new steady-state G–P partitioning model
- Code availability
- Data availability
- Author contributions
- Competing interests
- Disclaimer
- Acknowledgements
- Financial support
- Review statement
- References
- Supplement
- Abstract
- Introduction
- Establishment of the new steady-state G–P partitioning model
- Influence of ϕ0 on KP of PAHs
- Validation of the new steady-state G–P partitioning model
- Code availability
- Data availability
- Author contributions
- Competing interests
- Disclaimer
- Acknowledgements
- Financial support
- Review statement
- References
- Supplement