the Creative Commons Attribution 4.0 License.
the Creative Commons Attribution 4.0 License.
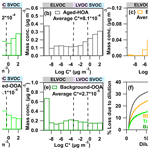
Impact of aging on the sources, volatility, and viscosity of organic aerosols in Chinese outflows
Tingting Feng
Yingkun Wang
Ming Zhu
Wei Song
Wei Chen
Yanyan Sang
Zheng Fang
Hua Fang
Shan Huang
Min Shao
Xiaofeng Huang
Lingyan He
Young Ro Lee
Lewis Gregory Huey
Francesco Canonaco
Andre S. H. Prevot
Xinming Wang
To investigate the impact of aging on the sources, volatility, and viscosity of organic aerosol (OA) in Chinese outflows, a high-resolution time-of-flight aerosol mass spectrometer (HR-AMS) coupled with a thermodenuder (TD) was deployed in the spring of 2018 in Dongying, which is a regional receptor site of metropolitan emissions in the North China Plain (NCP). The average mass concentration of PM1 is 31.5±22.7 µg m−3, which is mainly composed of nitrate (33 %) and OA (25 %). The source apportionment results show that the OA is mainly contributed by oxygenated OA (OOA) from secondary sources, including background-OOA (33 %) representing a background concentration of OA (2.6 µg m−3) in the NCP area, and transported-OOA (33 %) oxidized from urban emissions. The other two factors include aged hydrocarbon-liked OA (aged-HOA, 28 %) from transported vehicle emissions and biomass burning OA (BBOA, 5 %) from local open burning. The volatility of total OA (average µg m−3) in this study is generally lower than that reported in previous field studies, which is mainly due to the high OA oxidation level resulting from aging processes during transport. The volatilities of OA factors follow the order of background-OOA (average µg m−3) < transported-OOA aged-HOA BBOA (0.012 µg m−3). Extremely low volatilities in ambient air indicate that oligomers may exist in aged plumes. The viscosity estimation suggests that the majority of ambient OA in this study behaves as semisolid (60 %), liquifies at higher relative humidity (RH) (21 %), and solidifies (19 %) during noon when the RH is low and the oxidation level is high. Finally, the estimated mixing time of molecules in 200 nm OA varies dramatically from minutes at night to years in the afternoon, emphasizing the need to consider its dynamic kinetic limits when modeling OA. In general, the overall results of this study improve our understanding of the impact of aging on OA volatility and viscosity.
- Article
(8152 KB) - Full-text XML
-
Supplement
(2033 KB) - BibTeX
- EndNote
Atmospheric fine particles, which can substantially affect visibility, human health, and climate, have drawn great attention from the public (Song et al., 2018; Quan et al., 2011; Horowitz and Jacob, 1999; Huang et al., 2014; Y. Li et al., 2020; Huffman et al., 2009a). In the North China Plain (NCP) area, which is one of the most polluted areas in China, high concentrations of fine particles (21–178 µg m−3) in urban cities have been frequently observed (Zhou et al., 2020; Zhao et al., 2019; Duan et al., 2020; Xu et al., 2019). The city clusters in NCP areas act as urban emission sources and have a great impact on the atmospheric chemistry in downwind regions. During the transport processes, the pollutants undergo physical and chemical reactions continuously, which significantly change the properties of aerosols, especially of organic aerosol (OA), the dominating component of fine particles (Sun et al., 2017; Zhang et al., 2012; Jimenez et al., 2009; Zhang et al., 2007). In the past decade, numerous field measurements have been conducted to explore the concentration variations and sources of OA in urban and suburban areas in the NCP (Duan et al., 2020; Zhang et al., 2018; Sun et al., 2013; H. Li et al., 2017; Hu et al., 2016b; Elser et al., 2016; Hu et al., 2017a; Huang et al., 2010; Jiang et al., 2015; Wang et al., 2015; Xu et al., 2018, 2015; Zhang et al., 2015, 2016; Zhao et al., 2017; Zhou et al., 2018 and references therein); however, few studies were carried out in background areas (Zhou et al., 2020; Zhang et al., 2020; Zheng et al., 2015; Yan et al., 2021; Du et al., 2015). Studies which focus on the physicochemical properties of OA in background areas, i.e., volatility and viscosity, are even more scarce. The volatility and viscosity of OA can determine the gas–particle partitioning and particle phases, which represent crucial information for understanding aerosol growth and heterogeneous reactions in bulk OA (Y. Li et al., 2020; Reid et al., 2018; Shiraiwa et al., 2012). Moreover, quantifications of volatility and viscosity of OA in aged plumes are key for understanding the fate of aerosols in the atmosphere.
Multiple methods have been used to quantify the volatility of OA (Isaacman-Vanwertz and Aumont, 2021; Cappa and Jimenez, 2010; Saha et al., 2015; Y. Li et al., 2016; Yli-Juuti et al., 2017; Louvaris et al., 2017a). One of the most common methods is combining a heating module (e.g., thermodenuder, TD) with a detection instrument (e.g., aerosol mass spectrometer, AMS) to measure the mass fraction remaining (MFR) of different OA species at various temperatures. The volatility distribution of OA can be estimated based on either an empirical method (Faulhaber et al., 2009) or an evaporation kinetic model (Riipinen et al., 2010; Saha et al., 2015; Cappa, 2010a; Karnezi et al., 2014; Saha et al., 2017; Park et al., 2012; Epstein et al., 2009; Louvaris et al., 2017a). Based on the TD-AMS system, volatilities of ambient OA from urban/suburban (Xu et al., 2021; Louvaris et al., 2017b; Cao et al., 2018; Huffman et al., 2009a; Cappa and Jimenez, 2010; Saha et al., 2018; Paciga et al., 2016; Kang et al., 2022), rural (Zhu et al., 2021) and forest sites (Hu et al., 2016c; Saha et al., 2017; Kostenidou et al., 2018) have been explored. Huffman et al. (2009a) first directly characterized chemically resolved volatility of ambient aerosols in urban areas, supporting the notion that OA components are semivolatile and primary organic aerosol (POA) is more volatile than secondary organic aerosol (SOA). Using a similar approach, multiple studies (Huffman et al., 2009a; Louvaris et al., 2017b; Xu et al., 2016, 2021; Zhu et al., 2021) found that the OA volatility varies with different emission sources and oxidation levels, usually showing a trend of vehicle OA > biomass burning OA ≈ cooking OA > secondary OA. In addition, extremely low volatility of SOA due to the existence of oligomers after oxidation has also been reported (Lopez-Hilfiker et al., 2016; Hu et al., 2016c). The varied volatilities of POA and SOA emphasize the need to investigate OA volatility during aging processes.
Viscosity, which can be influenced by ambient relative humidity (RH), molecular weight, and oxidation level of OA, determines the mixing state and phase state of aerosols (Koop et al., 2011; Shiraiwa et al., 2017; DeRieux et al., 2018). Multiple studies in field campaigns and laboratory experiments suggest that, contrary to the traditional understanding, OA may occur as highly viscous semisolids or amorphous glassy solids under certain conditions (Shiraiwa et al., 2017; Virtanen et al., 2010; Reid et al., 2018; Koop et al., 2011; Renbaum-Wolff et al., 2013), which has important implications for atmospheric chemistry. Diffusion timescales within aerosols are affected by aerosol size, mass loading, viscosity, and volatility (Li and Shiraiwa, 2019). The diffusivity of species in aerosols is negatively correlated with viscosity. Thus it takes a longer time for highly viscous species to reach gas–particle equilibrium, which affects a series of atmospheric processes of aerosols (Shiraiwa and Seinfeld, 2012). A new parameterization proposed by Y. Li et al. (2020) has been used to estimate the diurnal variations of OA viscosity in ambient air based on the correlation between volatility and viscosity (Y. Li et al., 2020; Xu et al., 2021). The results show that OA viscosity can differ more than 4 orders of magnitude between day and night, as well as in the daytime among seasons. This great variation shows the sensitivity of OA viscosity to ambient conditions.
To investigate OA sources, volatility, and viscosity in the outflow of urban plumes, a TD-AMS system was applied in a ground-based campaign at a continental receptor site of downwind areas in the NCP. We systematically investigated the dynamic variations in chemical compositions of fine particles. OA sources were analyzed using positive matrix factorization (PMF) with multi-engine (ME-2) analysis. Coupled with the Hybrid Single-Particle Lagrangian Integrated Trajectory (HYSPLIT) model, the impact of regional transport on aerosols was further studied. Finally, a comprehensive explanation of volatilities and viscosities of OA and OA factors during the aging process, as well as their atmospheric implications, is presented and discussed.
2.1 Field campaign
The field study was conducted at the Yellow River Delta National Nature Reserve (YRDNNR) from 17 March to 12 April 2018. The YRDNNR site (37.75∘ N, 118.97∘ E, 1 m above sea level) is located in the northeast of Dongying City, Shandong Province, and the estuary of the Yellow River into the Bohai Sea (Yao et al., 2016; Zheng et al., 2019; Zhang et al., 2019), as shown in Fig. 1. Very few industrial or commercial areas are within 15 km of the sampling site, which is considered a regional background site of the NCP. The impacts of natural oil and gas (NO&G) (i.e., liquified petroleum gas, LPG), biomass burning on volatile organic compounds (VOCs), and O3 during the campaign were found at the site (Lee et al., 2021). The wind rose diagram combined with back trajectory clusters in Fig. 1a suggests that the sampling site during this campaign was affected by southern, southeast, and northwest winds, indicating the observed results were strongly influenced by the NCP urban outflows. The average wind speed was 3.0±1.8 m s−1 with a range of 0–9.1 m s−1. The average temperature during this study was 11.5±7.0 ∘C (from −2.8 to 29.2 ∘C) and RH was 59.1±19.0 % (from 14 % to 97 %).
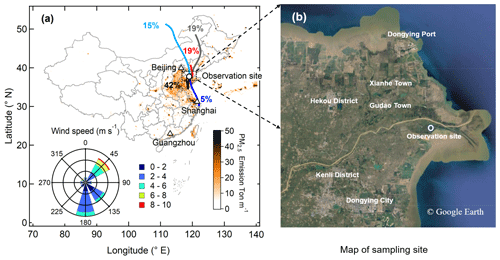
Figure 1(a) Location of observation site (circle) in China, along with wind speeds, wind directions, and back trajectories of air masses during this campaign. The map is color-coded according to PM2.5 emission inventory (M. Li et al., 2017). (b) Map of the Yellow River Delta National Nature Reserve (YRDNNR) region, labeled with the sampling site (circle) and adjacent cities and towns (source: Google Earth).
2.2 Operations and data processing of instruments
2.2.1 HR-ToF-AMS
The chemical composition of non-refractory PM1 was measured by a high-resolution time-of-flight aerosol mass spectrometer (HR-ToF-AMS; Aerodyne Research Inc. ARI, USA; “AMS” will be used hereinafter). A detailed introduction and operating principles of the AMS can be found in previous studies (Canagaratna et al., 2007; Drewnick et al., 2005; W. Chen et al., 2021). The setup and calibrations of AMS is briefly introduced in the following.
During this campaign, ambient air was sampled through a PM2.5 cyclone at a flow rate of 5 L min−1 and then introduced into the AMS through a -in. stainless steel tube. The sampling flow rate of the AMS was 0.1 L min−1. To avoid the effects of varied phase states on the collection efficiency (CE) of particles, a silicon dryer was set in front of the AMS to keep the RH of sampled air below 40 %. A schematic diagram is provided in Fig. S1 in the Supplement.
Calibrations including ionization efficiency (IE), inlet flow rate, and sizing distribution were conducted before and after the campaign (Jimenez et al., 2003; Jayne et al., 2000; Canagaratna et al., 2007). The IE calibration was performed based on a brute-force single particle (BFSP) mode (Decarlo et al., 2006), in which 400 nm monodisperse pure ammonium nitrate (NH4NO3) particles were introduced. For the calculation of mass concentration, the relative ionization efficiency (RIE) of ammonium was determined to be 3.7 by assuming a neutral balance of cation versus anion from standard NH4NO3 signals. The default RIE values of nitrate, sulfate, chloride, and OA were applied in this study (Huffman et al., 2009a). Taking into account particle loss in the AMS, the chemical composition-dependent CE (CDCE) followed by Middlebrook et al. (2012) was applied to correct mass concentration. The average value of CDCE is 0.52±0.04.
Pieber et al. (2016) found that the artificial CO ion can be caused by inorganic salts due to the interaction between thermal decomposition products of inorganic salts (i.e., NH4NO3) and OA deposits on the vaporizer surface. The ratio of to NO3 was estimated to be ∼2.3 % in this study by introducing the pure NH4NO3 particles into the AMS. Based on the calibration result, the interference of artificial CO was excluded in the series of subsequent analyses including OA quantification, elemental ratio calculation, and PMF analysis. Elemental ratios, including the oxygen-to-carbon ratio (O:C), hydrogen-to-carbon ratio (H:C), and total OA-to-organic carbon ratio (OA:OC), were determined with the Improved-Ambient (I-A) method in this study (Canagaratna et al., 2015). All the AMS quantification and elemental ratio data used here were based on the “V” mode at a time resolution of 1 min for the ambient dataset and 2.5 min for TD sampling (Fig. S1). The AMS data were analyzed with the software Squirrel (v1.62G) and PIKA (V. 1.22G) based on Igor pro 6.37.
2.2.2 Other instruments
The mass concentration of black carbon (BC) was measured by an AE-31 Aethalometer (Magee Scientific, USA) at a time resolution of 5 min (PM2.5 inlet). A scanning mobility particle sizer (SMPS, TSI, Inc.) was used to detect the particle number size distribution (mobility diameter: 15–600 nm) at a time resolution of 5 min. The total mass concentration measured by SMPS can be obtained by multiplying the integrated volume concentration (assuming the aerosol was spheric) by the corresponding density. The density of the total aerosol (1.63±0.04 g m−3 on average) was calculated based on the main chemical components of aerosols measured by the AMS and AE-31, as addressed in previous publications (Decarlo et al., 2008; Hu et al., 2017c; Kuwata et al., 2012). A good agreement in the time series between mass concentrations from the SMPS and the AMS + AE-31 (slope =0.97 and R=0.95, Fig. S2) was found, validating the robust measurement results shown here. VOCs were measured by online gas chromatography with a flame ionization detector (GC-FID) at a time resolution of 1 h (Lee et al., 2021). Regular gases of , SO2, CO, and O3 were measured with the NOx Analyzer (42i), SO2 Analyzer (43i), CO Analyzer (48i), and O3 monitor (49i) from Thermo Fisher Scientific at a time resolution of 1 min.
2.3 Source apportionments of PMF and ME-2
Sources of OA components were analyzed based on the conventional positive matrix factorization (PMF) method (Ulbrich et al., 2009) and the multi-linear engine (ME-2) approach (Canonaco et al., 2013). Unconstrained PMF analysis was conducted in the PMF Evaluation Tool (PET) v2.08 described by Ulbrich et al. (2009). Compared with conventional PMF, the ME-2 approach allows one to introduce a priori profiles of known OA factors, which can reduce the rotational ambiguity, and directly apportion toward environmentally meaningful solutions (Canonaco et al., 2013). In the ME-2 analysis of this study, we constrained the standard spectrum of biomass burning OA (BBOA) from the Changdao study (Hu et al., 2013) at varied α values using SoFi v6.81. By investigating the characteristics of mass spectra and time series, as well as their correlations with external tracers (Zhang et al., 2014; Ulbrich et al., 2009), four OA factors were finally chosen as the optimum solution for both ambient and TD dataset. The details of PMF and ME-2 analysis can be found in Sect. S1.1 in the Supplement.
2.4 Thermodenuder and volatility estimation
The TD used in this campaign is a commercial product from ARI and has been applied widely in laboratory experiments (Saha et al., 2015; Huffman et al., 2009b; Grieshop et al., 2009b; Lee et al., 2011; May et al., 2013c; X. Li et al., 2016; Saha and Grieshop, 2016; Sato et al., 2018; Champion et al., 2019 and reference therein) and field studies (Huffman et al., 2009a; Hu et al., 2016c; Paciga et al., 2016; Xu et al., 2016; Louvaris et al., 2017b; Cao et al., 2018; Kostenidou et al., 2018; Xu et al., 2021; Zhu et al., 2021 and reference therein). The TD consists of two parts: a heated section and a denuder section. The heated section can realize step-wise heating ranging from 54 to 230 ∘C to obtain concentrations at various temperatures in combination with AMS or SMPS. The denuder section is filled with activated charcoal to absorb the gas-phase species evaporated from particles within TD. The estimated residence time through the 55 cm heating section is 20.2 s, which is comparable to that (21.2 s) used by Huffman et al. (2008, 2009a, b). The setup of the TD-AMS system in this study is shown in Fig. S1, where sampling flow was switched to TD or bypass (ambient) paths at a time resolution of 5 min before being sampled by the AMS and SMPS. The switch of ambient and TD lines was controlled by the solenoid valve. When the instruments sample the ambient or TD lines, a make-up flow line with the same flow rate (0.62 L min−1) was set to refresh the air in the other sampling line by the air pump. The residence time after the solenoid valves is about 3–4 s. To avoid the mixing of air between the ambient and TD lines, a delay of 30s after each switch was set in the sampling strategy of the AMS and SMPS instruments. Bulk particle losses and temperatures in the TD were all corrected based on the calibration results from Huffman et al. (2008) since a similar TD and similar setups were applied in both studies. The corrected centerline temperature is 17 % higher than the measured temperature on average. More detailed information on TD calibrations can be found in Sect. S1.2.
We calculated the MFR of aerosols, which is the ratio of mass concentration through the TD path and bypass path (ambient line) at a set temperature. Then the thermogram, i.e., MFR as a function of corrected temperature, can be obtained. The thermograms of each species were depicted to semiquantitatively characterize aerosol volatility (Huffman et al., 2009b). Kinetic limitations of aerosol evaporation exist in the TD (Saha et al., 2015), which were mainly influenced by the residence time and OA concentration in the TD. Thus it is difficult to directly compare the thermograms in different studies with varying residence times and mass loadings (Cain and Pandis, 2017; Karnezi et al., 2014). To further describe the volatility of the complex OA matrix and make it comparable, volatility distribution, expressed as volatility bin set (VBS), was estimated based on the empirical relationship between LogP25 and the reciprocal of T50 developed by Faulhaber et al. (2009). T50 represents the temperature value where aerosol species evaporates 50 % compared to the input mass concentration under 25 ∘C (here referred to as “ambient concentration”) and P25 represents the vapor pressure of species at 298 K. A good agreement of OA volatility distributions between this empirical method and the kinetic model method was found previously (Cappa and Jimenez, 2010).
2.5 Predictions of glass transition temperature and viscosity of organic aerosols
Viscosity has been measured directly or indirectly by applying particle rebound, poke flow, and other offline measurements (Liu et al., 2017; Reid et al., 2018; Renbaum-Wolff et al., 2013). Viscosity is associated with the glass transition temperature (Tg) at which the phase transition occurs from semisolid to glassy states (Koop et al., 2011). Recently, more predictive models were developed to estimate the Tg of mixtures in field observations (Shiraiwa et al., 2017; Y. Li et al., 2020; Maclean et al., 2021). According to the new parameterization method introduced by Y. Li et al. (2020), Tg of OA (Tg,org) at dry conditions was estimated based on OA volatility distribution and the ratio of oxygen to carbon (O:C, “Aiken–Ambient” method). The Tg of OA–water mixtures (Tg,ωorg) at a given RH can be estimated using the Gordon–Taylor equation, in which the aerosol water content associated with OA can be calculated by the effective hygroscopicity parameter (κ) (Mei et al., 2013; Mikhailov et al., 2009). Finally, viscosity can then be calculated by applying the modified Vogel–Tammann–Fulcher (VTF) equation (Angell, 1991; DeRieux et al., 2018). The viscosity can affect aerosol equilibrium time (τmix), which can be estimated by the equation ) (Seinfeld and Pandis, 2016), where dp is the particle diameter (assuming 200 nm), and the bulk diffusion coefficient Db is calculated from the predicted viscosity through the fractional Stokes–Einstein relation (Xu et al., 2021; Evoy et al., 2019). The detailed computational processes can be found in Sect. S1.3.
2.6 Air mass trajectory analysis
Back trajectories with an altitude of 100 m were calculated every 6 h during this campaign using the HYSPLIT model (https://www.ready.noaa.gov/HYSPLIT.php, last access: 20 June 2022) developed by the Air Resources Laboratory (ARL) of the National Oceanic and Atmospheric Administration (NOAA) (Draxier and Hess, 1998; Cohen et al., 2015). The weather data were obtained from the Global Data Assimilation System (GDAS) archived by NOAA Air Resources Laboratory (ftp://arlftp.arlhq.noaa.gov/pub/archives/, last access: 30 March 2020). Then the back trajectories were divided into several groups based on their similarity in spatial distribution (cluster analysis) (Zheng et al., 2010).
In addition, the potential source contribution function (PSCF) (Polissar et al., 1999; Zhang et al., 2013) was also applied here to investigate the contribution of regional transport. This analysis was achieved through the Zefir software, which is an Igor-based package and specifically designed to achieve a comprehensive geographical origin analysis using a single statistical tool (Petit et al., 2017). Pollutant concentrations were entered into the Zefir software together with data obtained from HYSPLIT and NOAA. The geographic region was divided into grid cells (latitude i and longitude j). If the endpoint of the trajectory falls into a grid cell, the total number of trajectories is calculated as nij, and the number of these endpoints which are higher than a threshold (75th percentile used here) is counted as mij (Liu et al., 2021). To reduce the bias of PSCF for grid cells with low values of nij, the weight function (wij) recommended by Waked et al. (2014) was applied to the PSCF.
3.1 Concentrations of PM1 chemical compositions
The time series of mass concentration from the main components in PM1 are shown in Fig. 2. The overall PM1 concentration ranges dramatically from 2.5 to 152.8 µg m−3 with an average value of 31.5±22.7 µg m−3 (Fig. 2a, Table 1), which is generally lower than the average values in urban areas of the NCP, e.g., 45–53 µg m−3 in the spring of Beijing (Hu et al., 2017a; Sun et al., 2015). During this campaign, nitrate was the dominant species, accounting for 33 % of total PM1 mass concentration, followed by OA (25 %), sulfate (17 %), ammonium (17 %), BC (4 %), and chloride (3 %) (Fig. 2b).
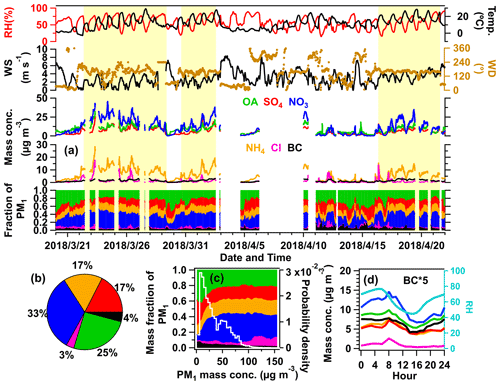
Figure 2(a) Time series of main species in PM1 and meteorological parameters (relative humidity, temperature, wind speed, and wind direction). The yellow background represents the polluted period in this campaign, which was selected based on the mass concentration of total PM1 above 20 µg m−3, and lasts for at least 2 d, accompanied by continuous south winds. (b) Average chemical compositions of PM1. (c) Variations of main compositions (left axis) and probability density as a function of PM1 mass concentration (right axis). (d) Average diurnal variations of PM1 species and PBL height during the entire campaign.
When the mass concentration of PM1 is below 20 µg m−3 (Fig. 2c), OA and sulfate account for the largest proportion, about 40 % and 22 % respectively. Nitrate increases dramatically and reaches 39 % of PM1 in the polluted periods (shaded in yellow in Fig. 2a). The ratio of ambient nitrate in the AMS (average value =0.27) is similar to the ratio from pure ammonium nitrate calibration (average value =0.28) (Fig. S16), suggesting that the observed nitrate in this study is nearly inorganic (>90 %). An increase in inorganic nitrate at a higher aerosol concentration has also been observed in other field studies including urban areas (C. Chen et al., 2021; W. Chen 2021; Griffith et al., 2015; Li et al., 2018), downwind sites (Qin et al., 2017; J. Li et al., 2020), and also other receptor sites (Hu et al., 2013; Li et al., 2014). As the air quality control policy was continuously applied in China, the emission of SO2 decreased substantially compared to that of NO2, and nitrate has become the dominant secondary component in fine particles (Xie et al., 2020; Geng et al., 2017a; Li et al., 2019).
The formation of nitrate has been identified as the following two main chemical pathways: (1) homogeneous reaction of gaseous nitric acid (HNO3) and ammonia (NH3), in which HNO3 is largely produced through photochemical oxidation of NO2 by OH radicals in the daytime (Yang et al., 2022); (2) heterogeneous hydrolysis of dinitrogen-pentoxide (N2O5), which is the major source of HNO3 at night (Griffith et al., 2015). In general, abundant precursors, high pH, and aerosol liquid water content (ALWC) during polluted periods might further promote these two processes (W. Chen et al., 2021; Wang et al., 2020). In the remote receptor site, in addition to the chemistry process, regional transport is another important source of high nitrate mass concentration (Squizzato et al., 2012). In this study, there is increased nitrate observed when high-speed winds from southeast to southwest dominate (above 8 km h−1) (Fig. 3a1), suggesting that regional transport in winter and spring in NCP areas plays an important role in the enhanced nitrate observed here (Huang et al., 2020; Hu et al., 2016b). The sulfate, ammonium, and BC show similar patterns to nitrate in diurnal variations (Fig. 2d) and bivariate polar plots (Fig. 3), suggesting the upwind anthropogenic emissions were transported to the regional receptor areas in the downwind direction. The PSCF results based on backward trajectory analysis were applied to further explore the potential transport from different regions to this receptor site. High concentrations of secondary inorganic aerosols are mainly contributed by the west and south regions of Shandong Province, and the northern part of Anhui and Jiangsu Province, where high anthropogenic PM2.5 emissions exist (Lei et al., 2011; Geng et al., 2017b). The results again verify the important influence of transport on aerosol chemistry from NCP urban outflows to receptor areas.
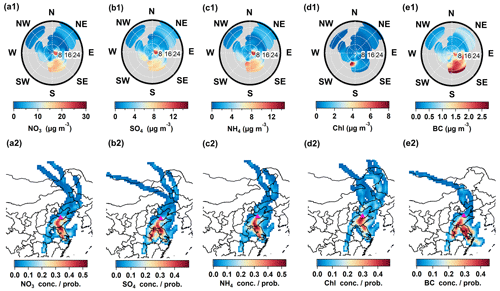
Figure 3Bivariate polar plots and the results of potential source contribution function (PSCF) from inorganic species, including (a1, a2) NO3; (b1, b2) SO4; (c1, c2) NH4; (d1, d2) Chl; (e1, e2) BC. The unit of wind speed is km h−1.
Table 1Summary of average mass concentrations of PM1 species and OA factors (µg m−3), average mixing ratios of gas-phase pollutants, and values of meteorological parameters during the entire campaign and polluted period. The definition of the polluted period is provided in the caption of Fig. 2.
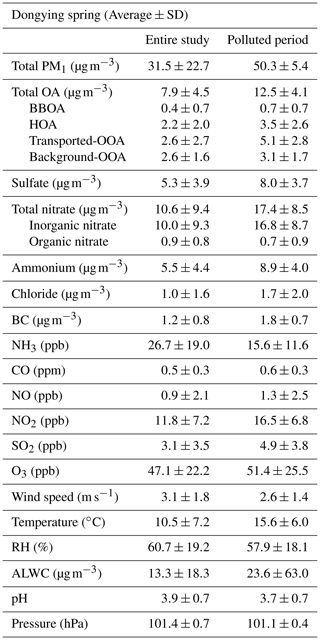
Total PM1= OA + sulfate + nitrate + ammonium + chloride + BC
3.2 Source apportionment of OA
Four OA factors were resolved in this study, including BBOA (5 %), aged-HOA (28 %), transported-OOA (33 %), and background-OOA (33 %), as shown in Fig. 4.
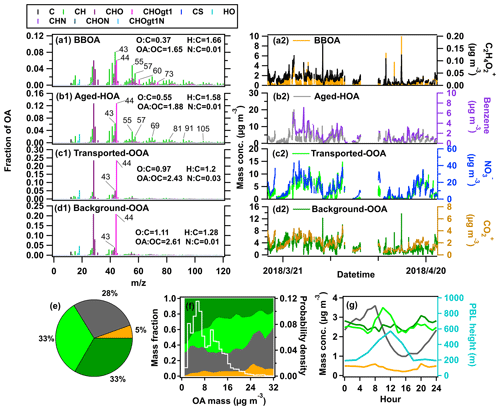
Figure 4Mass spectra (left) and time series (right) of four OA factors: (a1, a2) BBOA, (b1, b2) aged-HOA, (c1, c2) transported-OOA, (d1, d2) background-OOA. Time series of tracers associated with each factor are also displayed. (e) Average chemical compositions of OA. (f) Variations of OA compositions and probability density as a function of OA mass concentration. (g) Average diurnal variations of OA factors and planetary boundary layer (PBL) height during the whole campaign.
3.2.1 Biomass burning OA (BBOA)
BBOA is possibly from (1) biofuel/biomass burning from domestic heating in the NCP (Duan et al., 2020; Huang et al., 2020; Elser et al., 2016), and (2) open biomass burning around the observation site (H. Li et al., 2017; Liang et al., 2021). Indeed, biomass burning plumes from open fire burning were frequently seen during the campaign. As shown in Fig. S17, a positive correlation between BBOA with surrounding fire counts was found, suggesting that open biomass burning might play a more important role than domestic heating. Potassium (K+), regarded as a good indicator of biomass burning (Aiken et al., 2010), also shows a good correlation with BBOA (R=0.6), validating the BBOA resolved here.
C2H4O ion is mainly produced by the pyrolysis of levoglucosan and other anhydrous sugars from the thermal decomposition of cellulose and lignin (Bertrand et al., 2018), C2H4O ion ( 60) has been considered as a tracer for BBOA (Cubison et al., 2011). As depicted in Fig. S18a, the higher f60 value in OA above the background level (0.3 %) is concurrent with an increased BBOA fraction. The O:C value of BBOA is 0.37, which is in the range of reported values (0.2–0.65) from primary BBOA observed in other studies (Zhou et al., 2020; Hu et al., 2016b; Xu et al., 2017; Zhang et al., 2016). According to the bivariate polar plot and PSCF result of BBOA in Fig. 5b, the higher concentration of BBOA partially comes from local emissions of biomass burning southeast of the sampling site.
3.2.2 Aged hydrocarbon-like OA (aged-HOA)
HOA has been widely recognized and elaborated on in previous studies (Hao et al., 2014; Hu et al., 2016b; Aiken et al., 2009; Huang et al., 2020; Sun et al., 2014; Zhang et al., 2011; Zhou et al., 2020; Zhang et al., 2005a, 2007; Ng et al., 2011b). The HOA resolved in this study is not only contributed by fragments of CxHy (CnH2n+1 and CnH2n−1, 55, 57, 69, 81, 91, etc.) resembling the reported reference spectra of HOA (Hu et al., 2013; He et al., 2011; Ng et al., 2011a; Zhang et al., 2005b; Zhu et al., 2021; Jimenez et al., 2009) but also includes a high abundance of oxygenated ions (i.e., CO). This suggests that the HOA resolved here is partially aged, and thus is entitled “aged-HOA”. The diurnal variations of aged-HOA mass concentration and its fraction in total OA show a prominent peak during the traffic rush in the morning (08:00 UTC+8), then decrease sharply with the elevated boundary layer, suggesting this factor is influenced by primary vehicle emissions and meteorological conditions (Figs. 4, S19). The primary property of aged-HOA in this study was also identified by its good correlation with primary anthropogenic VOCs of benzene and ethyl toluene (with R=0.82 and 0.8, respectively) (Hu et al., 2016a; Lee et al., 2015; Mohr et al., 2012). In addition, aged-HOA has the characterization of secondary sources. The O:C value is 0.55, which is out of the range of O:C of HOA observed in China (0.05–0.44) (Hu et al., 2013; Zhou et al., 2020; Hu et al., 2016c). The high O:C value of aged-HOA in this study may be caused by long-distance transport from surrounding urban/town areas (Fig. 1a). Based on the bivariate polar plot and PCSF analysis in Fig. 5c, the higher aged-HOA concentration is in accord with urban plumes originating from the west to the south region, where Dongying city and northern Jiangsu are on this path. It generally will take 4–8 h for the vehicle emissions to be transported from Dongying and 31–70 h from northern Jiangsu to this site at a relatively high wind speed (7–16 km h−1).
3.2.3 Transported-OOA and background-OOA
Transported-OOA and background-OOA, representing two types of SOA with different origins, were resolved in this study. Both factors are fairly oxidized, which are characterized by the high mass abundance of oxidized 44 and 28 in total OA (0.23 for transported-OOA, 0.21 for background-OOA). Transported-OOA has an O:C ratio of 0.97, which is slightly lower than the 1.1 for background-OOA. Both OOA factors are in the high range compared with most OOA factors observed in urban and suburban areas (0.29–1.3) (Li et al., 2015; Xu et al., 2019) and in remote areas (0.47–1.45) (Zhou et al., 2020; Zheng et al., 2017; Gong et al., 2012).
The transported-OOA shows good correlations with secondary inorganic sulfate and nitrate (R=0.81 and 0.83 shown in Fig. S14, respectively), consistent with its secondary origins of OOA (He et al., 2011; Huang et al., 2020; Zhang et al., 2005a). The regression ratio between transported-OOA vs. sulfate + nitrate is around 0.84, within the range obtained in NCP areas (0.51–0.93) (Sun et al., 2016, 2012; Huang et al., 2010). Similar to secondary inorganic species, the higher mass concentration of transported-OOA is also driven by the south winds, thus named transported-OOA. The bivariate polar plots and PSCF results (Fig. 5d) show that the higher transported-OOA concentration might correspond to the transport processes from urban emissions located in the southern region of the sampling site. On the contrary, the background-OOA shows enhanced mass concentrations in all directions (Fig. 5e1) compared with transported-OOA, indicating its background origins, and representing the background concentration in NCP (2.6±1.6 µg m−3). In addition, in Sect. 3.4.2, we found very low volatility of background-OOA, confirming it is very aged in the ambient air.
On average, the sum of transported-OOA and background-OOA can account for 66 % of total OA. When the total OA concentration is below 3 µg m−3, background-OOA accounts for nearly 50 % of the OA mass concentration as shown in Fig. 4f. While the total OA increases from 4 to 20 µg m−3, the transported OOA from urban plumes starts to dominate (from 20 % to 44 %). The dominance of the OOA contribution to OA is consistent with findings in the urban outflow of Mexico City (Jimenez et al., 2009). As the OA concentration increases above 20 µg m−3, the aged-HOA and BBOA fractions increase from 29 % to 53 % and 6 % to 16 %, respectively, confirming that primary anthropogenic emissions are still an important contributor to the air pollution periods at a remote receptor site.
3.3 Volatility of PM1 species and OA factors
3.3.1 Volatility of inorganic species
Figure 6 shows the thermograms of non-refractory aerosols as well as their main chemical components. As the TD temperature increases, the MFR of sulfate remains constant until 115 ∘C, leading to a slight increase in its fraction in total PM1. The less steep thermogram of sulfate compared with those of other species is mainly due to the low volatility and its slight increase in collection efficiency caused by phase variation upon heating (Huffman et al., 2009a), which have been consistently observed in laboratory experiments (An et al., 2007; Huffman et al., 2009a) and field studies (Cao et al., 2018; Huffman et al., 2009a; Xu et al., 2016, 2021; Kang et al., 2022). When TD temperature is above 150 ∘C, the MFR curve of sulfate descends sharply to 6.5 %. However, a part of the sulfate remains at the highest temperature compared to that of pure (NH4)2SO4 from our laboratory experiment, indicating that the partial sulfate signals detected by the AMS might be organic sulfates or metallic sulfates (Fig. 6c). The decreased , , and ratios and the increased ratio as functions of TD temperature were indeed observed (Fig. S20), indicating the possible existence of organic sulfate (Chen et al., 2019). The CH3SO ion ( 78.99), which is considered to be a fragment ion from methanesulfonic acid (MSA) influenced by marine aerosols (Zhou et al., 2016; Huang et al., 2015; Zorn et al., 2008) and also possibly an indicator of sulfur-containing aerosols influenced by traffic and fossil carbon in urban areas (Daellenbach et al., 2017; Vlachou et al., 2019), shows higher volatility than sulfate in this study. The higher volatility of the CH3SO ion is probably influenced by its different bond types connected to the sulfate group than sulfate (Chen et al., 2019). Similarly, the volatility of laboratory generated-MSA particles is higher than that of ammonium sulfate (Fig. 6c), which is consistent with the ambient results.
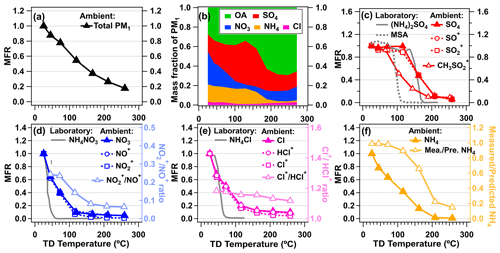
Figure 6(a) Mass fraction remaining (MFR) of total NR-PM1. (b) Fraction of main components in NR-PM1 as a function of TD temperature. (c) Variations in the average MFR of the inorganic species with their corresponding constituent ions as functions of TD temperature: (c) sulfate; (d) nitrate; (e) chloride; (f) ammonium. In addition, MFRs of pure ammonium salts of sulfate, nitrate, and chloride as well as methanesulfonic acid (MSA) measured in this study are shown for comparison. Particle acidity calculated as the measured NH4 vs. predicated NH4 assuming a neutral balance from the aforementioned cations as a function of TD temperature is also shown on the right axis of panel (f).
To quantitatively compare the thermograms of different species, T50, the temperature when MFR is equal to 0.5 is used here (Faulhaber et al., 2009; Cappa, 2010b). To obtain the T50 more accurately, the thermograms were fitted by Hill's equation (Sect. S1.2 in the Supplement) (Emanuelsson et al., 2013; Kolesar et al., 2015). Compared to sulfate (T50=159 ∘C), the T50 values of nitrate and chloride are 57 and 61 ∘C (Fig. 6d and e), respectively, supporting their semivolatile properties in ambient air (Cao et al., 2018; Xu et al., 2016, 2021). The evaporated loss of ambient nitrate has also been frequently observed in the offline membrane samples, in which 60 %–80 % of nitrate can evaporate in the summer (Chow et al., 2005; Hering and Cass, 1999). A similar phenomenon of NH4NO3 evaporation (30 %–40 %) has been found in a field-deployed oxidation flow tube, where the temperature inside the reactor is about 10 ∘C higher than that in the ambient air due to the heated cabin of the aircraft (Nault et al., 2018). Despite the quick evaporations of ambient nitrate and chloride, their T50 values are still higher than those of pure ammonium salts (i.e., NH4NO3 and NH4Cl) generated by an atomizer in the laboratory experiments (T50=37 and 54 ∘C, respectively). When TD temperature is above 150 ∘C, compared to the complete evaporations of pure NH4NO3 and NH4Cl (Fig. 6), ∼5 % of nitrate and ∼10 % of chloride in ambient air remained. The fractions of nitrate and chloride in total PM1 species also increase from 5 % and 1 % at middle temperatures to 14 % and 3 % at higher temperatures (>180 ∘C). These increases have also been observed in other field studies conducted with the TD-AMS system (Huffman et al., 2009a; Xu et al., 2019). For nitrate, the high remaining fraction is probably caused by the partial contribution from organic nitrate or non-refractory inorganic salts (e.g., NaNO3). This deduction is supported by the continuously decreased ratio (0.06 finally), consistent with similar low values in organic nitrate (≈0.1, a factor of 2.75 lower than the ratio of 0.28 in NH4NO3) (Day et al., 2022; Fry et al., 2013) and NaNO3 (≈0.006, a factor of 50 lower) (Hu et al., 2017c). The remaining chloride at the highest temperature might indicate the presence of metallic chlorides, such as ZnCl2, PbCl2, and KCl, which are usually emitted from combustion sources at high temperatures (Huffman et al., 2008; Xu et al., 2019; Aiken et al., 2009; Moffet et al., 2008). The positive correlation between Cl− and Pb2+ (R=0.51), as well as Cl− and K+ (R=0.54), supports this probability (Fig. S21).
Quick evaporation of ammonium species than cation species during heating was observed, leading to acidified aerosols (measured NH4 predicted NH4<1) (Fig. 6f). This is mainly due to the decomposition of ammonium salts and the yield of gas-phase NH3 upon heating (Hu et al., 2017b). Similar behavior of acidified aerosols in the TD line was exhibited in other field studies (Huffman et al., 2009a; Denkenberger et al., 2007).
In summary, the volatility of inorganic species in this study rank in the sequence of nitrate > chloride > ammonia > sulfate. Compared to their pure ammonium salts, all ambient inorganic aerosols have lower volatilities and part of them remain at high temperatures, indicating the existence of low volatile metallic or organic-related species in AMS-detected signals. These results support the complex property of ambient aerosols.
3.3.2 Volatility of OA and different OA factors
The thermograms of total OA and OA factors are shown in Fig. 7. The T50 of total OA is about 132 ∘C, which is higher than that of nitrate (T50=57 ∘C) but lower than that of sulfate (T50=159 ∘C). Total OA thermograms in Riverside, LA and Mexico City are also shown in Fig. 7a. Thermograms of these three studies should be comparable since they have a similar residence time of TD (about 21 s) and OA concentration (7.9–19.9 µg m−3 on average) (Huffman et al., 2009a; Docherty et al., 2011; Aiken et al., 2009), as well as a similar TD setup. During these two campaigns in Riverside, LA and Mexico City, the T50 of OA (104 and 106 ∘C, respectively) is lower than that obtained in Dongying (T50=132 ∘C). The slightly lower volatility of OA in Dongying is reasonable since the OA is more oxidized (O:C =0.85) compared with those from Riverside, LA and Mexico City (O:C =0.45 and 0.54, respectively) (Aiken et al., 2008; Docherty et al., 2011).
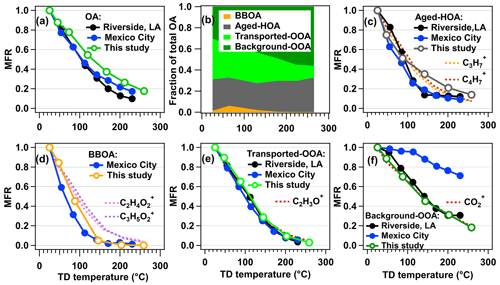
Figure 7(a) Thermogram of total OA; (b) fraction of four factors in total OA as functions of TD temperature; MFRs of (c) aged-HOA, (d) BBOA, (e) transported-OOA, and (f) background-OOA. For comparison, the MFR curves observed in other campaigns, such as Riverside, Los Angeles, USA, and Mexico City, Mexico (Huffman et al., 2009a), are also shown together due to their similar residence time in TD, which are marked by solid black and blue circles, respectively.
To further compare the volatility results among more studies, the volatility distribution of OA was estimated based on the empirical method of Faulhaber et al. (2009). As is shown in Fig. 8a, the volatility of OA is distributed in the range of –102 µg m−3 with two prominent peaks around and 10−4–10−3 µg m−3. If we categorize the entire distribution into different groups based on volatility introduced by Seinfeld and Pandis (2016), the OA in this study is composed of 52 % extremely low volatile organic compounds (ELVOCs, µg m−3), 26 % low volatile organic compounds (LVOCs, µg m−3), and 22 % semivolatile organic compounds (SVOCs, µg m−3). The SVOCs fraction in this study is lower than the values from urban areas (37 %–67 %); however, it is higher than that of OA strongly influenced by biogenic emissions (11 %–16 %), as shown in Fig. 9a. The former is mainly due to the OA in this study being more oxidized than those from urban areas where fresh primary emissions impact strongly. The lower SVOCs fraction of OA in forest areas is reasonable since the OA is mainly composed of the high fraction of biogenic SOA, which is strongly influenced by isoprene and monoterpene emissions. Autoxidation and oligomerization during biogenic SOA formation leading to lower volatility have also been reported (Ehn et al., 2014; Bianchi et al., 2017; Lopez-Hilfiker et al., 2019).
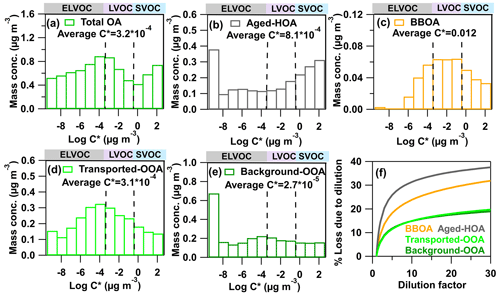
Figure 8Volatility distributions of (a) total OA, (b) aged-HOA, (c) BBOA, (d) transported-OOA, (e) background-OOA. The unit of average C* is µg m−3. (f) The additional mass losses (Eloss) of four OA factors as functions of the dilution factor.
To further investigate the OA volatilities from different sources, the fractions of OA factors as a function of TD temperature and their thermograms are shown in Fig. 7b–f. In general, aged-HOA, whose MFR is also characterized by its internal tracers (CxH), is the most volatile component before 120 ∘C among all OA factors. During this campaign, half of the aged-HOA evaporates at ∼92 ∘C (T50), which is similar to that in Riverside, LA (T50=92 ∘C) and slightly higher than that in Mexico City (T50=78 ∘C). While the temperature is above 100 ∘C, the MFR of aged-HOA in this study is much higher than those in the other two campaigns (Huffman et al., 2009a), consistent with the fact that HOA was oxidized during the transport process leading to a lower volatility. As shown in the plots of volatility distributions in Figs. 8b and 9b, the non-volatile ELVOCs and LVOCs fraction of aged-HOA are around 60 % in this study, which is higher than the HOA obtained in other areas (21 %–40 %) but similar to the HOA value obtained in Athens during wintertime (72 %, Fig. 9b), which was also conducted at an urban background site. This suggests the aging processes greatly decrease the POA volatility. For the laboratory-generated POA from diesel/gasoline emissions, their non-volatile fractions only account for 3 %–27 % of OA, indicating much higher volatility of POA than that of ambient HOA. The lower volatility supports that ambient HOA resolved in ambient air is a complex mixture and might be partially oxidized (Cappa and Jimenez, 2010). We examined whether low volatile PAH compounds might contribute to the low volatility of HOA, but a negligible contribution of PAH ions to OA was found. This suggests the PAH is not the main reason leading to the high non-volatile contribution of aged-HOA.
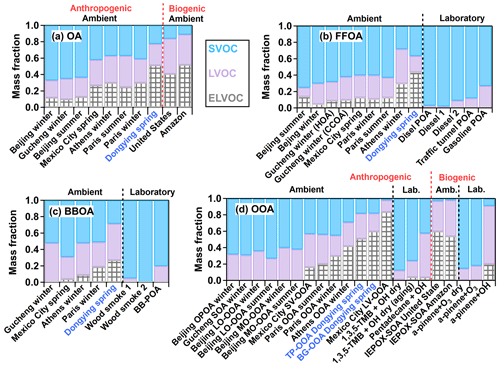
Figure 9Fractional contributions of different volatile species to (a) total OA, (b) fossil fuel-related OA (FFOA, which represents a sum of coal combustion OA (CCOA) and HOA), (c) BBOA, and (d) OOA in different studies. These studies includes Dongying (this study), Beijing and Gucheng (Xu et al., 2021), Paris (Paciga et al., 2016), Athens (Louvaris et al., 2017b), Mexico City (Cappa and Jimenez, 2010), southeastern United States and the Amazon (Hu et al., 2016c), and several laboratory experiments (Grieshop et al., 2009b, c; X. Li et al., 2016; May et al., 2013a, b, c; Sato et al., 2018, 2019; Ylisirniö et al., 2020). Detailed data can be found in Tables S2–S5. In panel (d) LO-OOA, MO-OOA, SV-OOA, and LV-OOA represent less oxidized OOA, more oxidized OOA, semivolatile OOA, and low volatile OOA, respectively. TP-OOA and BG-OOA represent transported- OOA and background-OOA resolved in this study. TMB and IEPOX-SOA represent trimethylbenzene and isoprene-epoxydiols-derived secondary OA, respectively. Lab. and Amb. represent studies from laboratories and field measurements of ambient air.
The thermogram of BBOA is shown in Fig. 7d. The T50 of BBOA in this study is 81 ∘C, which is slightly lower than that of HOA (92 ∘C). The BBOA shows a much lower residual (<5 %) than that of aged-HOA when TD temperature is above 150 ∘C, consistent with the fact that BBOA observed here is fresher and more local than aged-HOA. Although BBOA is the most volatile factor resolved in this study, it still shows the highest non-volatile fraction (72 %) compared with the results of BBOA in other studies (31 %–49 %) (Fig. 9c). The diversity of BBOA volatility distributions might be caused by the different burning materials and conditions, as well as the influence of aging processes (Huffman et al., 2009b; May et al., 2013c; Xu et al., 2021). It is also supported by the fact that ambient BBOA show lower volatility than that of laboratory-generated BBOA from different combustion conditions (Grieshop et al., 2009a, b; May et al., 2013c), emphasizing the aging impact on ambient BBOA volatility.
The thermograms of transported-OOA and background-OOA are shown in Fig. 7e and f. An obvious lower volatility of background-OOA than that of transported-OOA was found, which is consistent with the slightly higher O:C value of the latter (1.1 vs. 0.97). The thermogram of the CO ion, a reliable signature of SOA from thermal decarboxylation of organic acids in AMS (Aiken et al., 2009; Ng et al., 2010), resembled more the thermogram of background-OOA, which is consistent with the finding that highly oxidized OA is mainly composed of organic acids (Chen et al., 2020). We compared the volatilities of the two OOA factors resolved in this study with those reported in other ambient campaigns in Fig. 9d. It was found that the non-volatile fractions of transported-OOA and background-OOA (both are 82 %) are only lower than that of LV-OOA obtained in Mexico City (98 %) (Cappa and Jimenez, 2010); however, they are higher than those from the majority of other urban OOA factors (27 %–71 %). This is consistent with the fact that in addition to POA the aging process can substantially decrease SOA volatility in ambient air as well. Moreover, we found that the SOA volatility in this study is comparable to that of isoprene-epoxydiol-derived SOA (IEPOX-SOA, ELVOCs + LVOCs = 87 %–98 %), a large fraction of which is composed of oligomers (Hu et al., 2016c; Krechmer et al., 2015), suggesting the probable existence of oligomers in the two very aged SOA resolved here. In addition, we investigated the OA spectrum as a function of TD temperature, as shown in Fig. S22. When the TD temperature is above 200 ∘C, the fraction signals of some ions at were enhanced, suggesting the existence of oligomers.
By summarizing all the average volatilities of OA and OA factors in previous studies and this one (average log C* ranged from −5.34 to −0.015 µg m−3), the scatter plot of O:C vs. average log C* is shown in Fig. 10. In general, the average log C* exhibits a slight dependence on the ratio of O:C (slope ), which does not show a clear trend as was found in the laboratory SOA (Jimenez et al., 2009). The difference is possibly due to the fact that oligomerization and decomposition might result in different volatilities but with similar O:C values (Hildebrandt et al., 2010; Kroll and Seinfeld, 2008). For example, previous studies have shown that the OA, oligomers in particular, can decompose to more volatile components or form new/less non-volatile species upon heating (Cappa and Jimenez, 2010). Thus, the fraction of oligomers in OA did not show the same trend with O:C ratios during the heating process (Zhao et al., 2020). In addition, the ambient RH made the relationship between OA volatility and its O:C more complicated. On the one hand, the aqueous-phase reaction has been thought to be an important pathway for the formation of low volatility and higher molecular weight compounds (e.g., oligomer) (Zhou et al., 2016). While on the other hand, the volatility of OA increases after hydrolysis (Emanuelsson et al., 2013; Claflin and Ziemann, 2019). Therefore, the results here suggest that the ambient O:C ratio may not be a robust indicator of OA volatility among different studies based on heating detection techniques (Huffman et al., 2009a; Denkenberger et al., 2007; Cerully et al., 2015; Zhao et al., 2020).
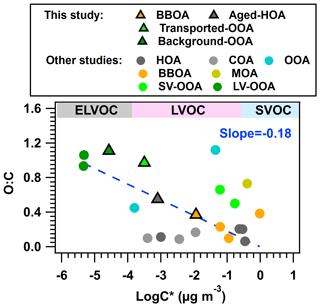
Figure 10Scatter plot of O:C and average log C* from this and previous studies. MOA represents marine OA. The detailed values in this plot are exhibited in Tables S2–S5.
Further, we investigated the evaporation loss of OA due to dilution according to the theoretical equation (Cappa and Jimenez, 2010), which is mainly dependent on its non-volatile/volatile fraction:
where DF is the dilution factor (here we assume a 30 times dilution), COA(DF) is the re-equilibrated COA after dilution, COA(0) is the OA mass before dilution, and Eloss is the relative mass loss due to evaporation of semivolatile components. The higher the amounts of non-volatile species that exist, the lower the evaporation losses to OA dilution. Finally, the evaporation loss of dilution follows reversely the order of the fraction of non-volatile species (fnv), aged-HOA transported-OOA background-OOA (fnv=0.82), as shown in Fig. 8f. After a factor of 10 dilution, aged-HOA would evaporate 30 % at ambient temperature, which suggests that even the HOA is quite aged in this study and it can still be oxidized continuously, and thus contributes to the SOA formation during long-distance transport. This suggests a high SOA formation potential in the outflow of NCP urban emissions.
3.4 Viscosity of OA
During this campaign, the predicted glass transmission temperature of OA (Tg,org) under dry conditions is 332.7 K, which is in the range of ambient Tg,org values estimated by Y. Li et al. (2020), and is much higher than the values (288.9–291.5 K) estimated in the urban area of Beijing and at the rural site of Gucheng (Xu et al., 2021). It is consistent with the lower volatility of OA in this study, where ∼78 % (shown in Fig. 9 above) of OA are non-volatile compounds compared with 30 %–40 % in Beijing and Gucheng (Xu et al., 2021).
The diurnal variations of OA viscosity as well as corresponding temperature and RH in previous studies and in this one are shown in Fig. 11a and b. According to the viscosity categories (Reid et al., 2018), the ambient OA can exist in three phase states: liquid (η<102 Pa s), semisolid ( Pa s), and amorphous solid (η>1012 Pa s). When Tg,org is higher than the ambient temperature, aerosol occurs as a solid phase, otherwise behaving as semisolid or liquid (Koop et al., 2011; DeRieux et al., 2018). The viscosity of ambient OA in this study generally ranges from 10−2 to more than 1012 Pa s, which shows that the OA phase exists mainly as semisolid (60 %), then followed by liquid (21 %, under high RH) and solid phases (19 %, low RH and high O:C). As shown in Fig. 11a and b, the average daily fluctuation of RH could inversely affect the viscosity of OA, which stayed low (5×102–106 Pa s) during the nighttime and then increased during daytime, thereby reaching a solid phase state (>1012 Pa s) between 14:00 and 16:00. The variation of OA viscosity is similar to the diurnal variation of O:C. This is expected since higher O:C due to photochemistry during the daytime leads to less volatile aerosol, which can result in a higher Tg,org, thus higher viscosity (Shiraiwa et al., 2011; Mikhailov et al., 2009).
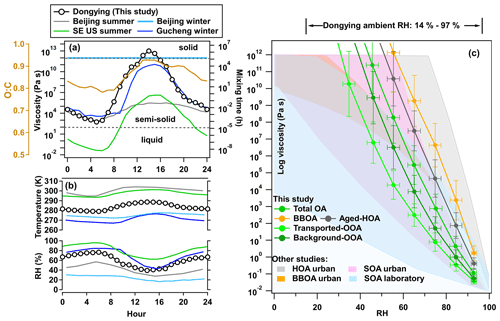
Figure 11Average diurnal variations of (a) estimated viscosity of total OA, (b) ambient RH, and temperature in different studies. In addition to this study, Beijing and Gucheng reported by Xu et al. (2021) and southeastern United States (SE US) reported by Y. Li et al. (2020) are also shown. The mixing time assuming the particle size is 200 nm is displayed on the right axis of panel (a). (c) Predicted viscosity of total OA, aged-HOA, BBOA, transported-OOA, and background-OOA as a function of RH. The ambient and laboratory viscosity results (Y. Li et al., 2020; DeRieux et al., 2018) are shaded in the background as a reference.
For fixed volatility and hygroscopicity (κ) of OA, ambient RH is the key parameter for determining the viscosity. We calculated the RH-dependent viscosity based on the volatilities of different OA factors in Fig. 11. Compared with the deployed field observations and laboratory experimental results, the predicted viscosities of two OOA factors are generally within the range of viscosity values from ambient OOA summarized in Y. Li et al. (2020) and in the higher ranges of values from laboratory-generated SOA summarized in DeRieux et al. (2018). This is reasonable since the laboratory-generated SOA usually has a lower viscosity due to a lower degree of oxidation (Aiken et al., 2008; Chen et al., 2015). Consistent with the inverse order of volatility, OOA factors in this study undergo liquifying transition when the RH is above 67 % for transported OOA and 73 % for background OOA. The viscosity curves of aged-HOA and BBOA as functions of RH are more viscous, which is consistent with what has been found in other urban HOA (the gray shade in Fig. 11c). Aged-HOA and BBOA in this study are more viscous on account of low f44 relative to the OOA factors, which is low hygroscopicity (DeRieux et al., 2018). It suggests the phase transition RH would be higher if higher fractions of anthropogenic HOA and BBOA were involved in the particle.
Furthermore, we estimated the mixing time τmix (right axis in Fig. 11a) to roughly clarify the time scale of organic molecule diffusion in 200 nm OA (Seinfeld and Pandis, 2016; Li and Shiraiwa, 2019), which can be compared with other studies conveniently. The results suggest that the mixing time of organic molecules in 200 nm OA varies dramatically from minutes at night to years in the afternoon. This large diurnal variation of OA mixing time might have significant impacts on aerosol evolution, such as gas–particle partitioning, multiphase chemical reactions, and nucleation, associated with the atmospheric fate of aerosols (Price et al., 2016; Shiraiwa et al., 2011; Gržinić et al., 2015). The higher mixing time during the day indicates that the kinetic limitation of diffusion after aging processes may impede gas–particle partitioning, and thus SOA formation and growth in ambient air (Xu et al., 2021; Galeazzo et al., 2021; Li and Shiraiwa, 2019). This result emphasizes the necessity of considering kinetic limit processes in the model, otherwise the LVOC in the particle phase might be overpredicted (Renbaum-Wolff et al., 2013).
To characterize the impact of aging on the sources, volatility, and viscosity of OA during the spring of 2018, a TD-AMS system was deployed in Dongying, a regional receptor site in the NCP. The average PM1 concentration is 31.5±22.7 µg m−3, of which secondary aerosols (inorganic + organic species) dominate in the entire campaign, accounting for nearly 84 % on average, which suggests the aging process plays an important role in the NCP urban outflows. Secondary inorganic aerosols occupy 67 % of PM1, which are mainly affected by south winds from urban areas in the NCP. Among secondary inorganic aerosols, nitrate accounts for the most (33 % of total PM1), suggesting that nitrate and its precursor (NO2) have become the primary pollutants in the NCP area, which should be considered in the regional pollution control in China.
Similar to previous studies (Xu et al., 2016; Cao et al., 2018; Huffman et al., 2009a; Xu et al., 2019), OA, as an integration of different types of organic species, is moderately volatile in this study, where nitrate is the most volatile species and sulfate is the least. Ambient inorganic aerosols all show higher residuals upon heating at higher TD temperatures than their laboratory-derived pure standards, indicating the universal existence of low volatile metallic or organic-related species in ambient aerosols. This supports the complexity of ambient aerosols. Source apportionment of OA resolved four OA factors: BBOA (5 %), aged-HOA (28 %), transported-OOA (33 %), and background-OOA (33 %). BBOA, which is considered to be emitted from open fire nearby, is the most volatile in this study (average µg m−3). Aged-HOA is characterized by high proportions of CxH ions and has good correlations with primary VOC species (benzene and ethyl toluene), as well as a pronounced peak of diurnal variations in the morning, suggesting that it is mainly transported from vehicle emissions from surrounding towns. The volatility of aged-HOA is proven to be impacted by the aging process, which has lower volatility (average µg m−3) than those of urban areas in the NCP (Beijing); however, it still shows high SOA formation potential. In this study, total OOA (transported-OOA + background-OOA) accounts for the largest fraction of OA (66 %) due to long-distance aging and fewer effects from local emissions than those of urban areas. Generally, transported-OOA (average µg m−3) shows a good agreement with secondary inorganic species, supporting their similar origins. Background-OOA shows the background concentration of OA in the outflow of the Chinese continent, which is around 2.6 µg m−3 on average. The background-OA is the least volatile factor in this study (average µg m−3), suggesting the existence of a large fraction of oligomers in this type of SOA and will be further investigated. The results suggest the aging processes during the transport of air plumes can significantly enhance the SOA formation and, thus, decrease the OA volatility from different sources.
The glass transition temperature (Tg,org) and RH-dependent viscosity (η) of OA were predicted based on the volatility distribution. The Tg,org of total OA under dry conditions is 332.7 K, which is in the range of values from other campaigns; however,´it is much higher than those in urban NCP due to the low volatility of OA in our study (72 % of OA are non-volatile species). The viscosity of OA varies from 10−2 to more than 1012 Pa, which mainly exist as semisolid particles. The solid phase usually occurs in the afternoon when the oxidation of OA is higher and RH is low. When the ambient RH is above 80 %, OA exists as liquid aerosols. Phase transition RH would be higher when higher fractions of anthropogenic HOA and BBOA are involved in the particle. The dynamic range of OA viscosity results in a wide variation in the mixing time. The mixing time of organic molecules in 200 nm OA ranges from 10−4 to 106 h, suggesting a large timescale to reach equilibrium. This emphasizes the need to consider dynamic kinetic limits of OA viscosity when modeling OA evolutions. Note that the OA viscosity estimated here is mainly derived based on a method from the volatility of pure organic compounds. There are also other factors that can influence OA viscosity in reality, e.g., OA functional group or molecular structure and organic/inorganic mixing state (Y. Li et al., 2020; Ditto et al., 2019), which will be further investigated in the future. Overall, our results reveal how the aging process in the continental outflow can significantly influence the source and physiochemical properties of OA, which should be considered in the modeling and policy-making work for evaluating its environmental impact in the future.
The data shown in the paper are available on request from the corresponding author (weiweihu@gig.ac.cn).
The supplement related to this article is available online at: https://doi.org/10.5194/acp-23-611-2023-supplement.
WH, WS, and YS, designed the research. MZ, ZF, WD, XY, BY, SH, and HF conducted the field measurements. TF, YW, WH, YL, LGH, CW, WC, MZ, ZF, and HF analyzed the data. TT and WC conducted the laboratory experiment on volatility. AP and FC supported the OA source analysis. WH and WC supported the viscosity analysis. TF and YW wrote the paper. WH, WC, CW, XH, LH, MS, XW, and AP reviewed and commented on the paper.
The contact author has declared that none of the authors has any competing interests.
Publisher's note: Copernicus Publications remains neutral with regard to jurisdictional claims in published maps and institutional affiliations.
This work was supported by the National Natural Science Foundation of China (grant no. 41875156), State Environmental Protection Key Laboratory of Formation and Prevention of Urban Air Pollution Complex (grant no. SEPAir-2021080540), Natural Science Foundation of Guangdong Province (grant no. 2019A1515011153), Guangdong Pearl River Talents Program (grant no. 2019QN01L948), Guangdong Foundation for Program of Science and Technology Research (grant no. 2020B1212060053), State Key Laboratory of Organic Geochemistry, GIGCAS (grant nos. SKLOG2020-5, SKLOG2020-6). Bin Yuan was supported by the National Key R&D Plan of China (grant no. 2019YFE0106300), the National Natural Science Foundation of China (grant no. 41877302), and Guangdong Innovative and Entrepreneurial Research Team Program (grant no. 2016ZT06N263). The authors also thank Ying Li for insightful suggestions and comments on this work.
This research has been supported by the National Natural Science Foundation of China (grant no. 41875156). State Environmental Protection Key Laboratory of Formation and Prevention of Urban Air Pollution Complex (grant no. SEPAir-2021080540), Natural Science Foundation of Guangdong Province (grant no.2019A1515011153), Guangdong Pearl River Talents Program (grant no. 2019QN01L948), Guangdong Foundation for Program of Science and Technology Research (grant no. 2020B1212060053), State Key Laboratory of Organic Geochemistry, GIGCAS (grant nos. SKLOG2020-5, SKLOG2020-6).
This paper was edited by Zhibin Wang and reviewed by two anonymous referees.
Aiken, A. C., DeCarlo, P. F., Kroll, J. H., Worsnop, D. R., Huffman, J. A., Docherty, K. S., Ulbrich, I. M., Mohr, C., Kimmel, J. R., Sueper, D., Sun, Y., Zhang, Q., Trimborn, A., Northway, M., Ziemann, P. J., Canagaratna, M. R., Onasch, T. B., Alfarra, M. R., Prevot, A. S. H., Dommen, J., Duplissy, J., Metzger, A., Baltensperger, U., and Jimenez, J. L.: and Ratios of Primary, Secondary, and Ambient Organic Aerosols with High-Resolution Time-of-Flight Aerosol Mass Spectrometry, Environ. Sci. Technol., 42, 4478–4485, https://doi.org/10.1021/es703009q, 2008.
Aiken, A. C., Salcedo, D., Cubison, M. J., Huffman, J. A., DeCarlo, P. F., Ulbrich, I. M., Docherty, K. S., Sueper, D., Kimmel, J. R., Worsnop, D. R., Trimborn, A., Northway, M., Stone, E. A., Schauer, J. J., Volkamer, R. M., Fortner, E., de Foy, B., Wang, J., Laskin, A., Shutthanandan, V., Zheng, J., Zhang, R., Gaffney, J., Marley, N. A., Paredes-Miranda, G., Arnott, W. P., Molina, L. T., Sosa, G., and Jimenez, J. L.: Mexico City aerosol analysis during MILAGRO using high resolution aerosol mass spectrometry at the urban supersite (T0) – Part 1: Fine particle composition and organic source apportionment, Atmos. Chem. Phys., 9, 6633–6653, https://doi.org/10.5194/acp-9-6633-2009, 2009.
Aiken, A. C., de Foy, B., Wiedinmyer, C., DeCarlo, P. F., Ulbrich, I. M., Wehrli, M. N., Szidat, S., Prevot, A. S. H., Noda, J., Wacker, L., Volkamer, R., Fortner, E., Wang, J., Laskin, A., Shutthanandan, V., Zheng, J., Zhang, R., Paredes-Miranda, G., Arnott, W. P., Molina, L. T., Sosa, G., Querol, X., and Jimenez, J. L.: Mexico city aerosol analysis during MILAGRO using high resolution aerosol mass spectrometry at the urban supersite (T0) – Part 2: Analysis of the biomass burning contribution and the non-fossil carbon fraction, Atmos. Chem. Phys., 10, 5315–5341, https://doi.org/10.5194/acp-10-5315-2010, 2010.
An, W. J., Pathak, R. K., Lee, B.-H., and Pandis, S. N.: Aerosol volatility measurement using an improved thermodenuder: Application to secondary organic aerosol, J. Aerosol Sci., 38, 305–314, https://doi.org/10.1016/j.jaerosci.2006.12.002, 2007.
Angell, C. A.: Relaxation in liquids, polymers and plastic crystals – strong/fragile patterns and problems, J. Non-Cryst. Solids, 131–133, 13–31, https://doi.org/10.1016/0022-3093(91)90266-9, 1991.
Bertrand, A., Stefenelli, G., Jen, C. N., Pieber, S. M., Bruns, E. A., Ni, H., Temime-Roussel, B., Slowik, J. G., Goldstein, A. H., El Haddad, I., Baltensperger, U., Prévôt, A. S. H., Wortham, H., and Marchand, N.: Evolution of the chemical fingerprint of biomass burning organic aerosol during aging, Atmos. Chem. Phys., 18, 7607–7624, https://doi.org/10.5194/acp-18-7607-2018, 2018.
Bianchi, F., Garmash, O., He, X., Yan, C., Iyer, S., Rosendahl, I., Xu, Z., Rissanen, M. P., Riva, M., Taipale, R., Sarnela, N., Petäjä, T., Worsnop, D. R., Kulmala, M., Ehn, M., and Junninen, H.: The role of highly oxygenated molecules (HOMs) in determining the composition of ambient ions in the boreal forest, Atmos. Chem. Phys., 17, 13819–13831, https://doi.org/10.5194/acp-17-13819-2017, 2017.
Cain, K. P. and Pandis, S. N.: A technique for the measurement of organic aerosol hygroscopicity, oxidation level, and volatility distributions, Atmos. Meas. Tech., 10, 4865–4876, https://doi.org/10.5194/amt-10-4865-2017, 2017.
Canagaratna, M. R., Jimenez, J. L., Kroll, J. H., Chen, Q., Kessler, S. H., Massoli, P., Hildebrandt Ruiz, L., Fortner, E., Williams, L. R., Wilson, K. R., Surratt, J. D., Donahue, N. M., Jayne, J. T., and Worsnop, D. R.: Elemental ratio measurements of organic compounds using aerosol mass spectrometry: characterization, improved calibration, and implications, Atmos. Chem. Phys., 15, 253–272, https://doi.org/10.5194/acp-15-253-2015, 2015.
Canagaratna, M. R., Jayne, J. T., Jimenez, J. L., Allan, J. D., Alfarra, M., Zhang, Q., Onasch, T. B., Drewnick, F., Coe, H., Middlebrook, A., Delia, A., Williams, L., Trimborn, A. M., Northway, M. J., DeCarlo, P., Kolb, C. E., Davidovcits, P., and Worsnop, D.: Chemical and microphysica characterization of ambient aerosols with the aerodyne aerosol mass spectrometer, Mass Spectrometry Reviews, 26, 185–222, https://doi.org/10.1002/mas.20115, 2007.
Canonaco, F., Crippa, M., Slowik, J. G., Baltensperger, U., and Prévôt, A. S. H.: SoFi, an IGOR-based interface for the efficient use of the generalized multilinear engine (ME-2) for the source apportionment: ME-2 application to aerosol mass spectrometer data, Atmos. Meas. Tech., 6, 3649–3661, https://doi.org/10.5194/amt-6-3649-2013, 2013.
Cao, L.-M., Huang, X.-F., Li, Y.-Y., Hu, M., and He, L.-Y.: Volatility measurement of atmospheric submicron aerosols in an urban atmosphere in southern China, Atmos. Chem. Phys., 18, 1729–1743, https://doi.org/10.5194/acp-18-1729-2018, 2018.
Cappa, C. D.: A model of aerosol evaporation kinetics in a thermodenuder, Atmos. Meas. Tech., 3, 579–592, https://doi.org/10.5194/amt-3-579-2010, 2010a.
Cappa, C. D.: A model of aerosol evaporation kinetics in a thermodenuder, Atmos. Meas. Tech., 3, 579–592, https://doi.org/10.5194/amt-3-579-2010, 2010b.
Cappa, C. D. and Jimenez, J. L.: Quantitative estimates of the volatility of ambient organic aerosol, Atmos. Chem. Phys., 10, 5409–5424, https://doi.org/10.5194/acp-10-5409-2010, 2010.
Cerully, K. M., Bougiatioti, A., Hite Jr., J. R., Guo, H., Xu, L., Ng, N. L., Weber, R., and Nenes, A.: On the link between hygroscopicity, volatility, and oxidation state of ambient and water-soluble aerosols in the southeastern United States, Atmos. Chem. Phys., 15, 8679–8694, https://doi.org/10.5194/acp-15-8679-2015, 2015.
Champion, W. M., Rothfuss, N. E., Petters, M. D., and Grieshop, A. P.: Volatility and Viscosity Are Correlated in Terpene Secondary Organic Aerosol Formed in a Flow Reactor, Environ. Sci. Tech. Let., 6, 513–519, https://doi.org/10.1021/acs.estlett.9b00412, 2019.
Chen, C., Zhang, H., Yan, W., Wu, N., Zhang, Q., and He, K.: Aerosol water content enhancement leads to changes in the major formation mechanisms of nitrate and secondary organic aerosols in winter over the North China Plain, Environ. Pollut., 287, 117625, https://doi.org/10.1016/j.envpol.2021.117625, 2021a.
Chen, Q., Heald, C. L., Jimenez, J. L., Canagaratna, M. R., Zhang, Q., He, L. Y., Huang, X. F., Campuzano-Jost, P., Palm, B. B., Poulain, L., Kuwata, M., Martin, S. T., Abbatt, J. P. D., Lee, A. K. Y., and Liggio, J.: Elemental composition of organic aerosol: The gap between ambient and laboratory measurements, Geophys. Res. Lett., 42, 4182–4189, https://doi.org/10.1002/2015gl063693, 2015.
Chen, W., Ye, Y., Hu, W., Zhou, H., Pan, T., Wang, Y., Song, W., Song, Q., Ye, C., Wang, C., Wang, B., Huang, S., Yuan, B., Zhu, M., Lian, X., Zhang, G., Bi, X., Jiang, F., Liu, J., Canonaco, F., Prevot, A. S. H., Shao, M., and Wang, X.: Real-time characterization of aerosol compositions, sources and aging processes in Guangzhou during PRIDE-GBA 2018 campaign, J. Geophys. Res.-Atmos., 126, e2021JD035114, https://doi.org/10.1029/2021jd035114, 2021b.
Chen, Y., Xu, L., Humphry, T., Hettiyadura, A. P. S., Ovadnevaite, J., Huang, S., Poulain, L., Schroder, J. C., Campuzano-Jost, P., Jimenez, J. L., Herrmann, H., O'Dowd, C., Stone, E. A., and Ng, N. L.: Response of the Aerodyne Aerosol Mass Spectrometer to Inorganic Sulfates and Organosulfur Compounds: Applications in Field and Laboratory Measurements, Environ. Sci. Technol., 53, 5176–5186, https://doi.org/10.1021/acs.est.9b00884, 2019.
Chen, Y., Takeuchi, M., Nah, T., Xu, L., Canagaratna, M. R., Stark, H., Baumann, K., Canonaco, F., Prévôt, A. S. H., Huey, L. G., Weber, R. J., and Ng, N. L.: Chemical characterization of secondary organic aerosol at a rural site in the southeastern US: insights from simultaneous high-resolution time-of-flight aerosol mass spectrometer (HR-ToF-AMS) and FIGAERO chemical ionization mass spectrometer (CIMS) measurements, Atmos. Chem. Phys., 20, 8421–8440, https://doi.org/10.5194/acp-20-8421-2020, 2020.
Chow, J. C., Watson, J. G., Lowenthal, D. H., and Magliano, K. L.: Loss of PM2.5 nitrate from filter samples in central California, J. Air Waste Manage., 55, 1158–1168, https://doi.org/10.1080/10473289.2005.10464704, 2005.
Claflin, M. S. and Ziemann, P. J.: Thermal desorption behavior of hemiacetal, acetal, ether, and ester oligomers, Aerosol Sci. Tech., 53, 473–484, https://doi.org/10.1080/02786826.2019.1576853, 2019.
Cohen, M. D., Stunder, B. J. B., Rolph, G. D., Draxler, R. R., Stein, A. F., and Ngan, F.: NOAA's HYSPLIT Atmospheric Transport and Dispersion Modeling System, B. Am. Meteorol. Soc., 96, 2059–2077, https://doi.org/10.1175/bams-d-14-00110.1, 2015.
Cubison, M. J., Ortega, A. M., Hayes, P. L., Farmer, D. K., Day, D., Lechner, M. J., Brune, W. H., Apel, E., Diskin, G. S., Fisher, J. A., Fuelberg, H. E., Hecobian, A., Knapp, D. J., Mikoviny, T., Riemer, D., Sachse, G. W., Sessions, W., Weber, R. J., Weinheimer, A. J., Wisthaler, A., and Jimenez, J. L.: Effects of aging on organic aerosol from open biomass burning smoke in aircraft and laboratory studies, Atmos. Chem. Phys., 11, 12049–12064, https://doi.org/10.5194/acp-11-12049-2011, 2011.
Daellenbach, K. R., Stefenelli, G., Bozzetti, C., Vlachou, A., Fermo, P., Gonzalez, R., Piazzalunga, A., Colombi, C., Canonaco, F., Hueglin, C., Kasper-Giebl, A., Jaffrezo, J.-L., Bianchi, F., Slowik, J. G., Baltensperger, U., El-Haddad, I., and Prévôt, A. S. H.: Long-term chemical analysis and organic aerosol source apportionment at nine sites in central Europe: source identification and uncertainty assessment, Atmos. Chem. Phys., 17, 13265–13282, https://doi.org/10.5194/acp-17-13265-2017, 2017.
Day, D. A., Campuzano-Jost, P., Nault, B. A., Palm, B. B., Hu, W., Guo, H., Wooldridge, P. J., Cohen, R. C., Docherty, K. S., Huffman, J. A., de Sá, S. S., Martin, S. T., and Jimenez, J. L.: A systematic re-evaluation of methods for quantification of bulk particle-phase organic nitrates using real-time aerosol mass spectrometry, Atmos. Meas. Tech., 15, 459–483, https://doi.org/10.5194/amt-15-459-2022, 2022.
DeCarlo, P. F., Kimmel, J. R., Trimborn, A., Northway, M. J., Jayne, J. T., Aiken, A. C., Gonin, M., Fuhrer, K., Horvath, T., Docherty, K. S., Worsnop, D. R., and Jimenez, J. L.: Field-deployable, high-resolution, time-of-flight aerosol mass spectrometer, Anal. Chem., 78, 8281–8289, https://doi.org/10.1021/ac061249n, 2006.
DeCarlo, P. F., Dunlea, E. J., Kimmel, J. R., Aiken, A. C., Sueper, D., Crounse, J., Wennberg, P. O., Emmons, L., Shinozuka, Y., Clarke, A., Zhou, J., Tomlinson, J., Collins, D. R., Knapp, D., Weinheimer, A. J., Montzka, D. D., Campos, T., and Jimenez, J. L.: Fast airborne aerosol size and chemistry measurements above Mexico City and Central Mexico during the MILAGRO campaign, Atmos. Chem. Phys., 8, 4027–4048, https://doi.org/10.5194/acp-8-4027-2008, 2008.
Denkenberger, K. A., Moffet, R. C., Holecek, J. C., Rebotier, T. P., and Prather, K. A.: Real-Time, Single-Particle Measurements of Oligomers in Aged Ambient Aerosol Particles, Environ. Sci. Technol., 41, 5439–5446, https://doi.org/10.1021/es070329l, 2007.
DeRieux, W.-S. W., Li, Y., Lin, P., Laskin, J., Laskin, A., Bertram, A. K., Nizkorodov, S. A., and Shiraiwa, M.: Predicting the glass transition temperature and viscosity of secondary organic material using molecular composition, Atmos. Chem. Phys., 18, 6331–6351, https://doi.org/10.5194/acp-18-6331-2018, 2018.
Ditto, J. C., Joo, T., Khare, P., Sheu, R., Takeuchi, M., Chen, Y., Xu, W., Bui, A. A. T., Sun, Y., Ng, N. L., and Gentner, D. R.: Effects of Molecular-Level Compositional Variability in Organic Aerosol on Phase State and Thermodynamic Mixing Behavior, Environ. Sci. Technol., 53, 13009–13018, https://doi.org/10.1021/acs.est.9b02664, 2019.
Docherty, K. S., Aiken, A. C., Huffman, J. A., Ulbrich, I. M., DeCarlo, P. F., Sueper, D., Worsnop, D. R., Snyder, D. C., Peltier, R. E., Weber, R. J., Grover, B. D., Eatough, D. J., Williams, B. J., Goldstein, A. H., Ziemann, P. J., and Jimenez, J. L.: The 2005 Study of Organic Aerosols at Riverside (SOAR-1): instrumental intercomparisons and fine particle composition, Atmos. Chem. Phys., 11, 12387–12420, https://doi.org/10.5194/acp-11-12387-2011, 2011.
Draxier, R. R. and Hess, G. D.: An overview of the HYSPLIT_4 modelling system for trajectories, dispersion and deposition, Aust. Meteorol. Mag., 47, 295–308, 1998.
Drewnick, F., Hings, S. S., DeCarlo, P., Jayne, J. T., Gonin, M., Fuhrer, K., Weimer, S., Jimenez, J. L., Demerjian, K. L., Borrmann, S., and Worsnop, D. R.: A New Time-of-Flight Aerosol Mass Spectrometer (TOF-AMS) – Instrument Description and First Field Deployment, Aerosol Sci. Tech., 39, 637–658, https://doi.org/10.1080/02786820500182040, 2005.
Du, W., Sun, Y. L., Xu, Y. S., Jiang, Q., Wang, Q. Q., Yang, W., Wang, F., Bai, Z. P., Zhao, X. D., and Yang, Y. C.: Chemical characterization of submicron aerosol and particle growth events at a national background site (3295 m a.s.l.) on the Tibetan Plateau, Atmos. Chem. Phys., 15, 10811–10824, https://doi.org/10.5194/acp-15-10811-2015, 2015.
Duan, J., Huang, R.-J., Li, Y., Chen, Q., Zheng, Y., Chen, Y., Lin, C., Ni, H., Wang, M., Ovadnevaite, J., Ceburnis, D., Chen, C., Worsnop, D. R., Hoffmann, T., O'Dowd, C., and Cao, J.: Summertime and wintertime atmospheric processes of secondary aerosol in Beijing, Atmos. Chem. Phys., 20, 3793–3807, https://doi.org/10.5194/acp-20-3793-2020, 2020.
Ehn, M., Thornton, J. A., Kleist, E., Sipila, M., Junninen, H., Pullinen, I., Springer, M., Rubach, F., Tillmann, R., Lee, B., Lopez-Hilfiker, F., Andres, S., Acir, I. H., Rissanen, M., Jokinen, T., Schobesberger, S., Kangasluoma, J., Kontkanen, J., Nieminen, T., Kurten, T., Nielsen, L. B., Jorgensen, S., Kjaergaard, H. G., Canagaratna, M., Maso, M. D., Berndt, T., Petaja, T., Wahner, A., Kerminen, V. M., Kulmala, M., Worsnop, D. R., Wildt, J., and Mentel, T. F.: A large source of low-volatility secondary organic aerosol, Nature, 506, 476–479, https://doi.org/10.1038/nature13032, 2014.
Elser, M., Huang, R.-J., Wolf, R., Slowik, J. G., Wang, Q., Canonaco, F., Li, G., Bozzetti, C., Daellenbach, K. R., Huang, Y., Zhang, R., Li, Z., Cao, J., Baltensperger, U., El-Haddad, I., and Prévôt, A. S. H.: New insights into PM2.5 chemical composition and sources in two major cities in China during extreme haze events using aerosol mass spectrometry, Atmos. Chem. Phys., 16, 3207–3225, https://doi.org/10.5194/acp-16-3207-2016, 2016.
Emanuelsson, E. U., Watne, A. K., Lutz, A., Ljungstrom, E., and Hallquist, M.: Influence of humidity, temperature, and radicals on the formation and thermal properties of secondary organic aerosol (SOA) from ozonolysis of β-pinene, J. Phys. Chem. A, 117, 10346–10358, https://doi.org/10.1021/jp4010218, 2013.
Epstein, S., Riipinen, I., and Donahue, N.: A Semiempirical Correlation between Enthalpy of Vaporization and Saturation Concentration for Organic Aerosol, Environ. Sci. Technol., 44, 743–748, https://doi.org/10.1021/es902497z, 2009.
Evoy, E., Maclean, A. M., Rovelli, G., Li, Y., Tsimpidi, A. P., Karydis, V. A., Kamal, S., Lelieveld, J., Shiraiwa, M., Reid, J. P., and Bertram, A. K.: Predictions of diffusion rates of large organic molecules in secondary organic aerosols using the Stokes–Einstein and fractional Stokes–Einstein relations, Atmos. Chem. Phys., 19, 10073–10085, https://doi.org/10.5194/acp-19-10073-2019, 2019.
Faulhaber, A. E., Thomas, B. M., Jimenez, J. L., Jayne, J. T., Worsnop, D. R., and Ziemann, P. J.: Characterization of a thermodenuder-particle beam mass spectrometer system for the study of organic aerosol volatility and composition, Atmos. Meas. Tech., 2, 15–31, https://doi.org/10.5194/amt-2-15-2009, 2009.
Fry, J. L., Draper, D. C., Zarzana, K. J., Campuzano-Jost, P., Day, D. A., Jimenez, J. L., Brown, S. S., Cohen, R. C., Kaser, L., Hansel, A., Cappellin, L., Karl, T., Hodzic Roux, A., Turnipseed, A., Cantrell, C., Lefer, B. L., and Grossberg, N.: Observations of gas- and aerosol-phase organic nitrates at BEACHON-RoMBAS 2011, Atmos. Chem. Phys., 13, 8585–8605, https://doi.org/10.5194/acp-13-8585-2013, 2013.
Galeazzo, T., Valorso, R., Li, Y., Camredon, M., Aumont, B., and Shiraiwa, M.: Estimation of secondary organic aerosol viscosity from explicit modeling of gas-phase oxidation of isoprene and α-pinene, Atmos. Chem. Phys., 21, 10199–10213, https://doi.org/10.5194/acp-21-10199-2021, 2021.
Geng, G., Zhang, Q., Tong, D., Li, M., Zheng, Y., Wang, S., and He, K.: Chemical composition of ambient PM2.5 over China and relationship to precursor emissions during 2005–2012, Atmos. Chem. Phys., 17, 9187–9203, https://doi.org/10.5194/acp-17-9187-2017, 2017a.
Geng, G., Zhang, Q., Martin, R. V., Lin, J., Huo, H., Zheng, B., Wang, S., and He, K.: Impact of spatial proxies on the representation of bottom-up emission inventories: A satellite-based analysis, Atmos. Chem. Phys., 17, 4131–4145, https://doi.org/10.5194/acp-17-4131-2017, 2017b.
Gong, Z., Lan, Z., Xue, L., Zeng, L., He, L., and Huang, X.: Characterization of submicron aerosols in the urban outflow of the central Pearl River Delta region of China, Front. Env. Sci. Eng., 6, 725–733, https://doi.org/10.1007/s11783-012-0441-8, 2012.
Grieshop, A. P., Donahue, N. M., and Robinson, A. L.: Laboratory investigation of photochemical oxidation of organic aerosol from wood fires 2: analysis of aerosol mass spectrometer data, Atmos. Chem. Phys., 9, 2227–2240, https://doi.org/10.5194/acp-9-2227-2009, 2009a.
Grieshop, A. P., Logue, J. M., Donahue, N. M., and Robinson, A. L.: Laboratory investigation of photochemical oxidation of organic aerosol from wood fires 1: measurement and simulation of organic aerosol evolution, Atmos. Chem. Phys., 9, 1263–1277, https://doi.org/10.5194/acp-9-1263-2009, 2009b.
Grieshop, A. P., Miracolo, M. A., Donahue, N. M., and Robinson, A. L.: Constraining the Volatility Distribution and Gas-Particle Partitioning of Combustion Aerosols Using Isothermal Dilution and Thermodenuder Measurements, Environ. Sci. Technol., 43, 4750–4756, https://doi.org/10.1021/es8032378, 2009c.
Griffith, S. M., Huang, X. H. H., Louie, P. K. K., and Yu, J. Z.: Characterizing the thermodynamic and chemical composition factors controlling PM2.5 nitrate: Insights gained from two years of online measurements in Hong Kong, Atmos. Environ., 122, 864–875, https://doi.org/10.1016/j.atmosenv.2015.02.009, 2015.
Gržinić, G., Bartels-Rausch, T., Berkemeier, T., Türler, A., and Ammann, M.: Viscosity controls humidity dependence of N2O5 uptake to citric acid aerosol, Atmos. Chem. Phys., 15, 13615–13625, https://doi.org/10.5194/acp-15-13615-2015, 2015.
Hao, L. Q., Kortelainen, A., Romakkaniemi, S., Portin, H., Jaatinen, A., Leskinen, A., Komppula, M., Miettinen, P., Sueper, D., Pajunoja, A., Smith, J. N., Lehtinen, K. E. J., Worsnop, D. R., Laaksonen, A., and Virtanen, A.: Atmospheric submicron aerosol composition and particulate organic nitrate formation in a boreal forestland–urban mixed region, Atmos. Chem. Phys., 14, 13483–13495, https://doi.org/10.5194/acp-14-13483-2014, 2014.
He, L.-Y., Huang, X.-F., Xue, L., Hu, M., Lin, Y., Zheng, J., Zhang, R., and Zhang, Y.-H.: Submicron aerosol analysis and organic source apportionment in an urban atmosphere in Pearl River Delta of China using high-resolution aerosol mass spectrometry, J. Geophys. Res., 116, D12304, https://doi.org/10.1029/2010jd014566, 2011.
Hering, S. and Cass, G.: The Magnitude of Bias in the Measurement of PM25 Arising from Volatilization of Particulate Nitrate from Teflon Filters, J. Air Waste Manage., 49, 725–733, https://doi.org/10.1080/10473289.1999.10463843, 1999.
Hildebrandt, L., Engelhart, G. J., Mohr, C., Kostenidou, E., Lanz, V. A., Bougiatioti, A., DeCarlo, P. F., Prevot, A. S. H., Baltensperger, U., Mihalopoulos, N., Donahue, N. M., and Pandis, S. N.: Aged organic aerosol in the Eastern Mediterranean: the Finokalia Aerosol Measurement Experiment – 2008, Atmos. Chem. Phys., 10, 4167–4186, https://doi.org/10.5194/acp-10-4167-2010, 2010.
Horowitz, L. W. and Jacob, D. J.: Global impact of fossil fuel combustion on atmospheric NOx, J. Geophys. Res.-Atmos., 104, 23823–23840, https://doi.org/10.1029/1999jd900205, 1999.
Hu, W., Hu, M., Hu, W.-W., Niu, H., Zheng, J., Wu, Y., Chen, W., Chen, C., Li, L., Shao, M., Xie, S., and Zhang, Y.: Characterization of submicron aerosols influenced by biomass burning at a site in the Sichuan Basin, southwestern China, Atmos. Chem. Phys., 16, 13213–13230, https://doi.org/10.5194/acp-16-13213-2016, 2016a.
Hu, W., Hu, M., Hu, W., Jimenez, J. L., Yuan, B., Chen, W., Wang, M., Wu, Y., Chen, C., Wang, Z., Peng, J., Zeng, L., and Shao, M.: Chemical composition, sources, and aging process of submicron aerosols in Beijing: Contrast between summer and winter, J. Geophys. Res.-Atmos., 121, 1955–1977, https://doi.org/10.1002/2015jd024020, 2016b.
Hu, W., Palm, B. B., Day, D. A., Campuzano-Jost, P., Krechmer, J. E., Peng, Z., de Sá, S. S., Martin, S. T., Alexander, M. L., Baumann, K., Hacker, L., Kiendler-Scharr, A., Koss, A. R., de Gouw, J. A., Goldstein, A. H., Seco, R., Sjostedt, S. J., Park, J.-H., Guenther, A. B., Kim, S., Canonaco, F., Prévôt, A. S. H., Brune, W. H., and Jimenez, J. L.: Volatility and lifetime against OH heterogeneous reaction of ambient isoprene-epoxydiols-derived secondary organic aerosol (IEPOX-SOA), Atmos. Chem. Phys., 16, 11563–11580, https://doi.org/10.5194/acp-16-11563-2016, 2016c.
Hu, W., Hu, M., Hu, W.-W., Zheng, J., Chen, C., Wu, Y., and Guo, S.: Seasonal variations in high time-resolved chemical compositions, sources, and evolution of atmospheric submicron aerosols in the megacity Beijing, Atmos. Chem. Phys., 17, 9979–10000, https://doi.org/10.5194/acp-17-9979-2017, 2017a.
Hu, W., Campuzano-Jost, P., Day, D. A., Croteau, P., Canagaratna, M. R., Jayne, J. T., Worsnop, D. R., and Jimenez, J. L.: Evaluation of the new capture vapourizer for aerosol mass spectrometers (AMS) through laboratory studies of inorganic species, Atmos. Meas. Tech., 10, 2897–2921, https://doi.org/10.5194/amt-10-2897-2017, 2017b.
Hu, W., Campuzano-Jost, P., Day, D. A., Croteau, P., Canagaratna, M. R., Jayne, J. T., Worsnop, D. R., and Jimenez, J. L.: Evaluation of the new capture vaporizer for aerosol mass spectrometers (AMS) through field studies of inorganic species, Aerosol Sci. Tech., 51, 735–754, https://doi.org/10.1080/02786826.2017.1296104, 2017c.
Hu, W. W., Hu, M., Yuan, B., Jimenez, J. L., Tang, Q., Peng, J. F., Hu, W., Shao, M., Wang, M., Zeng, L. M., Wu, Y. S., Gong, Z. H., Huang, X. F., and He, L. Y.: Insights on organic aerosol aging and the influence of coal combustion at a regional receptor site of central eastern China, Atmos. Chem. Phys., 13, 10095–10112, https://doi.org/10.5194/acp-13-10095-2013, 2013.
Huang, D. D., Li, Y. J., Lee, B. P., and Chan, C. K.: Analysis of organic sulfur compounds in atmospheric aerosols at the HKUST supersite in Hong Kong using HR-ToF-AMS, Environ. Sci. Technol., 49, 3672–3679, https://doi.org/10.1021/es5056269, 2015.
Huang, R.-J., He, Y., Duan, J., Li, Y., Chen, Q., Zheng, Y., Chen, Y., Hu, W., Lin, C., Ni, H., Dai, W., Cao, J., Wu, Y., Zhang, R., Xu, W., Ovadnevaite, J., Ceburnis, D., Hoffmann, T., and O'Dowd, C. D.: Contrasting sources and processes of particulate species in haze days with low and high relative humidity in wintertime Beijing, Atmos. Chem. Phys., 20, 9101–9114, https://doi.org/10.5194/acp-20-9101-2020, 2020.
Huang, X.-F., He, L.-Y., Hu, M., Canagaratna, M. R., Sun, Y., Zhang, Q., Zhu, T., Xue, L., Zeng, L.-W., Liu, X.-G., Zhang, Y.-H., Jayne, J. T., Ng, N. L., and Worsnop, D. R.: Highly time-resolved chemical characterization of atmospheric submicron particles during 2008 Beijing Olympic Games using an Aerodyne High-Resolution Aerosol Mass Spectrometer, Atmos. Chem. Phys., 10, 8933–8945, https://doi.org/10.5194/acp-10-8933-2010, 2010.
Huang, Y., Shen, H., Chen, H., Wang, R., Zhang, Y., Su, S., Chen, Y., Lin, N., Zhuo, S., Zhong, Q., Wang, X., Liu, J., Li, B., Liu, W., and Tao, S.: Quantification of global primary emissions of PM2.5, PM10, and TSP from combustion and industrial process sources, Environ. Sci. Technol., 48, 13834–13843, https://doi.org/10.1021/es503696k, 2014.
Huffman, J. A., Ziemann, P. J., Jayne, J. T., Worsnop, D. R., and Jimenez, J. L.: Development and Characterization of a Fast-Stepping/Scanning Thermodenuder for Chemically-Resolved Aerosol Volatility Measurements, Aerosol Sci. Tech., 42, 395–407, https://doi.org/10.1080/02786820802104981, 2008.
Huffman, J. A., Docherty, K. S., Aiken, A. C., Cubison, M. J., Ulbrich, I. M., DeCarlo, P. F., Sueper, D., Jayne, J. T., Worsnop, D. R., Ziemann, P. J., and Jimenez, J. L.: Chemically-resolved aerosol volatility measurements from two megacity field studies, Atmos. Chem. Phys., 9, 7161–7182, https://doi.org/10.5194/acp-9-7161-2009, 2009a.
Huffman, J. A., Docherty, K. S., Mohr, C., Cubison, M. J., Ulbrich, I. M., Ziemann, P. J., Onasch, T. B., and Jimenez, J. L.: Chemically-Resolved Volatility Measurements of Organic Aerosol from Different Sources, Environ. Sci. Technol., 43, 5351–5357, https://doi.org/10.1021/es803539d, 2009b.
Isaacman-VanWertz, G. and Aumont, B.: Impact of organic molecular structure on the estimation of atmospherically relevant physicochemical parameters, Atmos. Chem. Phys., 21, 6541–6563, https://doi.org/10.5194/acp-21-6541-2021, 2021.
Jayne, J. T., Leard, D. C., Zhang, X. F., Davidovits, P., Smith, K. A., Kolb, C. E., and Worsnop, D. R.: Development of an aerosol mass spectrometer for size and composition analysis of submicron particles, Aerosol Sci. Tech., 33, 49–70, 2000.
Jiang, Q., Sun, Y. L., Wang, Z., and Yin, Y.: Aerosol composition and sources during the Chinese Spring Festival: fireworks, secondary aerosol, and holiday effects, Atmos. Chem. Phys., 15, 6023–6034, https://doi.org/10.5194/acp-15-6023-2015, 2015.
Jimenez, J. L., Jayne, J. T., Shi, Q., Kolb, C. E., Worsnop, D. R., Yourshaw, I., Seinfeld, J. H., Flagan, R. C., Zhang, X., Smith, K. A., Morris, J. W., and Davidovits, P.: Ambient aerosol sampling using the Aerodyne Aerosol Mass Spectrometer, Journal of Geophysical Research: Atmospheres, 108, https://doi.org/10.1029/2001JD001213, 2003.
Jimenez, J. L., Canagaratna, M. R., Donahue, N. M., Prevot, A. S. H., Zhang, Q., Kroll, J. H., DeCarlo, P. F., Allan, J. D., Coe, H., Ng, N. L., Aiken, A. C., Docherty, K. S., Ulbrich, I. M., Grieshop, A. P., Robinson, A. L., Duplissy, J., Smith, J. D., Wilson, K. R., Lanz, V. A., Hueglin, C., Sun, Y. L., Tian, J., Laaksonen, A., Raatikainen, T., Rautiainen, J., Vaattovaara, P., Ehn, M., Kulmala, M., Tomlinson, J. M., Collins, D. R., Cubison, M. J., Dunlea, E. J., Huffman, J. A., Onasch, T. B., Alfarra, M. R., Williams, P. I., Bower, K., Kondo, Y., Schneider, J., Drewnick, F., Borrmann, S., Weimer, S., Demerjian, K., Salcedo, D., Cottrell, L., Griffin, R., Takami, A., Miyoshi, T., Hatakeyama, S., Shimono, A., Sun, J. Y., Zhang, Y. M., Dzepina, K., Kimmel, J. R., Sueper, D., Jayne, J. T., Herndon, S. C., Trimborn, A. M., Williams, L. R., Wood, E. C., Middlebrook, A. M., Kolb, C. E., Baltensperger, U., and Worsnop, D. R.: Evolution of Organic Aerosols in the Atmosphere, Science, 326, 1525–1529, https://doi.org/10.1126/science.1180353, 2009.
Kang, H. G., Kim, Y., Collier, S., Zhang, Q., and Kim, H.: Volatility of Springtime ambient organic aerosol derived with thermodenuder aerosol mass spectrometry in Seoul, Korea, Environ. Pollut., 304, 119203, https://doi.org/10.1016/j.envpol.2022.119203, 2022.
Karnezi, E., Riipinen, I., and Pandis, S. N.: Measuring the atmospheric organic aerosol volatility distribution: a theoretical analysis, Atmos. Meas. Tech., 7, 2953–2965, https://doi.org/10.5194/amt-7-2953-2014, 2014.
Kolesar, K. R., Chen, C., Johnson, D., and Cappa, C. D.: The influences of mass loading and rapid dilution of secondary organic aerosol on particle volatility, Atmos. Chem. Phys., 15, 9327–9343, https://doi.org/10.5194/acp-15-9327-2015, 2015.
Koop, T., Bookhold, J., Shiraiwa, M., and Pöschl, U.: Glass transition and phase state of organic compounds: dependency on molecular properties and implications for secondary organic aerosols in the atmosphere, Phys. Chem. Chem. Phys., 13, 19238–19255, https://doi.org/10.1039/C1CP22617G, 2011.
Kostenidou, E., Karnezi, E., Hite Jr., J. R., Bougiatioti, A., Cerully, K., Xu, L., Ng, N. L., Nenes, A., and Pandis, S. N.: Organic aerosol in the summertime southeastern United States: components and their link to volatility distribution, oxidation state and hygroscopicity, Atmos. Chem. Phys., 18, 5799–5819, https://doi.org/10.5194/acp-18-5799-2018, 2018.
Krechmer, J. E., Coggon, M. M., Massoli, P., Nguyen, T. B., Crounse, J. D., Hu, W., Day, D. A., Tyndall, G. S., Henze, D. K., Rivera-Rios, J. C., Nowak, J. B., Kimmel, J. R., Mauldin, R. L., 3rd, Stark, H., Jayne, J. T., Sipila, M., Junninen, H., Clair, J. M., Zhang, X., Feiner, P. A., Zhang, L., Miller, D. O., Brune, W. H., Keutsch, F. N., Wennberg, P. O., Seinfeld, J. H., Worsnop, D. R., Jimenez, J. L., and Canagaratna, M. R.: Formation of Low Volatility Organic Compounds and Secondary Organic Aerosol from Isoprene Hydroxyhydroperoxide Low-NO Oxidation, Environ. Sci. Technol., 49, 10330–10339, https://doi.org/10.1021/acs.est.5b02031, 2015.
Kroll, J. H. and Seinfeld, J. H.: Chemistry of secondary organic aerosol: Formation and evolution of low-volatility organics in the atmosphere, Atmos. Environ., 42, 3593–3624, https://doi.org/10.1016/j.atmosenv.2008.01.003, 2008.
Kuwata, M., Zorn, S. R., and Martin, S. T.: Using elemental ratios to predict the density of organic material composed of carbon, hydrogen, and oxygen, Environ. Sci. Technol., 46, 787–794, https://doi.org/10.1021/es202525q, 2012.
Lee, B.-H., Pierce, J. R., Engelhart, G. J., and Pandis, S. N.: Volatility of secondary organic aerosol from the ozonolysis of monoterpenes, Atmos. Environ., 45, 2443–2452, https://doi.org/10.1016/j.atmosenv.2011.02.004, 2011.
Lee, B. P., Li, Y. J., Yu, J. Z., Louie, P. K. K., and Chan, C. K.: Characteristics of submicron particulate matter at the urban roadside in downtown Hong Kong – Overview of 4 months of continuous high-resolution aerosol mass spectrometer measurements, J. Geophys. Res.-Atmos., 120, 7040–7058, https://doi.org/10.1002/2015jd023311, 2015.
Lee, Y., Huey, L. G., Wang, Y., Qu, H., Zhang, R., Ji, Y., Tanner, D. J., Wang, X., Tang, J., Song, W., Hu, W., and Zhang, Y.: Photochemistry of Volatile Organic Compounds in the Yellow River Delta, China: Formation of O3 and Peroxyacyl Nitrates, J. Geophys. Res.-Atmos., 126, e2021JD035296, https://doi.org/10.1029/2021jd035296, 2021.
Lei, Y., Zhang, Q., Nielsen, C., and He, K.: An inventory of primary air pollutants and CO2 emissions from cement production in China, 1990–2020, Atmos. Environ., 45, 147–154, https://doi.org/10.1016/j.atmosenv.2010.09.034, 2011.
Li, H., Zhang, Q., Zhang, Q., Chen, C., Wang, L., Wei, Z., Zhou, S., Parworth, C., Zheng, B., Canonaco, F., Prévôt, A. S. H., Chen, P., Zhang, H., Wallington, T. J., and He, K.: Wintertime aerosol chemistry and haze evolution in an extremely polluted city of the North China Plain: significant contribution from coal and biomass combustion, Atmos. Chem. Phys., 17, 4751–4768, https://doi.org/10.5194/acp-17-4751-2017, 2017.
Li, H., Zhang, Q., Zheng, B., Chen, C., Wu, N., Guo, H., Zhang, Y., Zheng, Y., Li, X., and He, K.: Nitrate-driven urban haze pollution during summertime over the North China Plain, Atmos. Chem. Phys., 18, 5293–5306, https://doi.org/10.5194/acp-18-5293-2018, 2018.
Li, H., Cheng, J., Zhang, Q., Zheng, B., Zhang, Y., Zheng, G., and He, K.: Rapid transition in winter aerosol composition in Beijing from 2014 to 2017: response to clean air actions, Atmos. Chem. Phys., 19, 11485–11499, https://doi.org/10.5194/acp-19-11485-2019, 2019.
Li, J., Liu, Z., Cao, L., Gao, W., Yan, Y., Mao, J., Zhang, X., He, L., Xin, J., Tang, G., Ji, D., Hu, B., Wang, L., Wang, Y., Dai, L., Zhao, D., Du, W., and Wang, Y.: Highly time-resolved chemical characterization and implications of regional transport for submicron aerosols in the North China Plain, Sci. Total Environ., 705, 135803, https://doi.org/10.1016/j.scitotenv.2019.135803, 2020.
Li, M., Zhang, Q., Kurokawa, J.-I., Woo, J.-H., He, K., Lu, Z., Ohara, T., Song, Y., Streets, D. G., Carmichael, G. R., Cheng, Y., Hong, C., Huo, H., Jiang, X., Kang, S., Liu, F., Su, H., and Zheng, B.: MIX: a mosaic Asian anthropogenic emission inventory under the international collaboration framework of the MICS-Asia and HTAP, Atmos. Chem. Phys., 17, 935–963, https://doi.org/10.5194/acp-17-935-2017, 2017.
Li, W., Shao, L., Shi, Z., Chen, J., Yang, L., Yuan, Q., Yan, C., Zhang, X., Wang, Y., Sun, J., Zhang, Y., Shen, X., Wang, Z., and Wang, W.: Mixing state and hygroscopicity of dust and haze particles before leaving Asian continent, J. Geophys. Res.-Atmos., 119, 1044–1059, https://doi.org/10.1002/2013jd021003, 2014.
Li, X., Dallmann, T. R., May, A. A., Tkacik, D. S., Lambe, A. T., Jayne, J. T., Croteau, P. L., and Presto, A. A.: Gas-Particle Partitioning of Vehicle Emitted Primary Organic Aerosol Measured in a Traffic Tunnel, Environ. Sci. Technol., 50, 12146–12155, https://doi.org/10.1021/acs.est.6b01666, 2016.
Li, Y. and Shiraiwa, M.: Timescales of secondary organic aerosols to reach equilibrium at various temperatures and relative humidities, Atmos. Chem. Phys., 19, 5959–5971, https://doi.org/10.5194/acp-19-5959-2019, 2019.
Li, Y., Pöschl, U., and Shiraiwa, M.: Molecular corridors and parameterizations of volatility in the chemical evolution of organic aerosols, Atmos. Chem. Phys., 16, 3327–3344, https://doi.org/10.5194/acp-16-3327-2016, 2016.
Li, Y., Day, D. A., Stark, H., Jimenez, J. L., and Shiraiwa, M.: Predictions of the glass transition temperature and viscosity of organic aerosols from volatility distributions, Atmos. Chem. Phys., 20, 8103–8122, https://doi.org/10.5194/acp-20-8103-2020, 2020.
Li, Y. J., Lee, B. P., Su, L., Fung, J. C. H., and Chan, C. K.: Seasonal characteristics of fine particulate matter (PM) based on high-resolution time-of-flight aerosol mass spectrometric (HR-ToF-AMS) measurements at the HKUST Supersite in Hong Kong, Atmos. Chem. Phys., 15, 37–53, https://doi.org/10.5194/acp-15-37-2015, 2015.
Liang, L., Engling, G., Liu, C., Xu, W., Liu, X., Cheng, Y., Du, Z., Zhang, G., Sun, J., and Zhang, X.: Measurement report: Chemical characteristics of PM2.5 during typical biomass burning season at an agricultural site of the North China Plain, Atmos. Chem. Phys., 21, 3181–3192, https://doi.org/10.5194/acp-21-3181-2021, 2021.
Liu, J., Li, J., and Yao, F.: Source-receptor relationship of transboundary particulate matter pollution between China, South Korea and Japan: Approaches, current understanding and limitations, Crit. Rev. Env. Sci. Tech., 52, 3896–3920, https://doi.org/10.1080/10643389.2021.1964308, 2021.
Liu, Y., Wu, Z., Wang, Y., Xiao, Y., Gu, F., Jing Zheng, Tianyi Tan, Shang, D., Wu, Y., Zeng, L., Min Hu, Adam Bateman, and Martin, S. T.: Submicrometer Particles are in the Liquid State during Heavy Haze Episodes in the Urban Atmosphere of Beijing, China, Environ. Sci. Tech. Let., 4, 427–432, https://doi.org/10.1021/acs.estlett.7b00352, 2017.
Lopez-Hilfiker, F. D., Mohr, C., D'Ambro, E. L., Lutz, A., Riedel, T. P., Gaston, C. J., Iyer, S., Zhang, Z., Gold, A., Surratt, J. D., Lee, B. H., Kurten, T., Hu, W. W., Jimenez, J., Hallquist, M., and Thornton, J. A.: Molecular Composition and Volatility of Organic Aerosol in the Southeastern U.S.: Implications for IEPOX Derived SOA, Environ. Sci. Technol., 50, 2200–2209, https://doi.org/10.1021/acs.est.5b04769, 2016.
Lopez-Hilfiker, F. D., Pospisilova, V., Huang, W., Kalberer, M., Mohr, C., Stefenelli, G., Thornton, J. A., Baltensperger, U., Prevot, A. S. H., and Slowik, J. G.: An extractive electrospray ionization time-of-flight mass spectrometer (EESI-TOF) for online measurement of atmospheric aerosol particles, Atmos. Meas. Tech., 12, 4867–4886, https://doi.org/10.5194/amt-12-4867-2019, 2019.
Louvaris, E. E., Karnezi, E., Kostenidou, E., Kaltsonoudis, C., and Pandis, S. N.: Estimation of the volatility distribution of organic aerosol combining thermodenuder and isothermal dilution measurements, Atmos. Meas. Tech., 10, 3909–3918, https://doi.org/10.5194/amt-10-3909-2017, 2017a.
Louvaris, E. E., Florou, K., Karnezi, E., Papanastasiou, D. K., Gkatzelis, G. I., and Pandis, S. N.: Volatility of source apportioned wintertime organic aerosol in the city of Athens, Atmos. Environ., 158, 138–147, https://doi.org/10.1016/j.atmosenv.2017.03.042, 2017b.
Maclean, A. M., Li, Y., Crescenzo, G. V., Smith, N. R., Karydis, V. A., Tsimpidi, A. P., Butenhoff, C. L., Faiola, C. L., Lelieveld, J., Nizkorodov, S. A., Shiraiwa, M., and Bertram, A. K.: Global Distribution of the Phase State and Mixing Times within Secondary Organic Aerosol Particles in the Troposphere Based on Room-Temperature Viscosity Measurements, ACS Earth Space Chem., 5, 3458–3473, https://doi.org/10.1021/acsearthspacechem.1c00296, 2021.
May, A. A., Presto, A. A., Hennigan, C. J., Nguyen, N. T., Gordon, T. D., and Robinson, A. L.: Gas-particle partitioning of primary organic aerosol emissions: (1) Gasoline vehicle exhaust, Atmos. Environ., 77, 128–139, https://doi.org/10.1016/j.atmosenv.2013.04.060, 2013a.
May, A. A., Presto, A. A., Hennigan, C. J., Nguyen, N. T., Gordon, T. D., and Robinson, A. L.: Gas-particle partitioning of primary organic aerosol emissions: (2) Diesel vehicles, Environ. Sci. Technol., 47, 8288–8296, https://doi.org/10.1021/es400782j, 2013b.
May, A. A., Levin, E. J. T., Hennigan, C. J., Riipinen, I., Lee, T., Collett, J. L., Jimenez, J. L., Kreidenweis, S. M., and Robinson, A. L.: Gas-particle partitioning of primary organic aerosol emissions: 3. Biomass burning, J. Geophys. Res.-Atmos., 118, 11327–311338, https://doi.org/10.1002/jgrd.50828, 2013c.
Mei, F., Setyan, A., Zhang, Q., and Wang, J.: CCN activity of organic aerosols observed downwind of urban emissions during CARES, Atmos. Chem. Phys., 13, 12155–12169, https://doi.org/10.5194/acp-13-12155-2013, 2013.
Middlebrook, A. M., Bahreini, R., Jimenez, J. L., and Canagaratna, M. R.: Evaluation of Composition-Dependent Collection Efficiencies for the Aerodyne Aerosol Mass Spectrometer using Field Data, Aerosol Sci. Tech., 46, 258–271, https://doi.org/10.1080/02786826.2011.620041, 2012.
Mikhailov, E., Vlasenko, S., Martin, S. T., Koop, T., and Pöschl, U.: Amorphous and crystalline aerosol particles interacting with water vapor: conceptual framework and experimental evidence for restructuring, phase transitions and kinetic limitations, Atmos. Chem. Phys., 9, 9491–9522, https://doi.org/10.5194/acp-9-9491-2009, 2009.
Moffet, R. C., de Foy, B., Molina, L. T., Molina, M. J., and Prather, K. A.: Measurement of ambient aerosols in northern Mexico City by single particle mass spectrometry, Atmos. Chem. Phys., 8, 4499–4516, https://doi.org/10.5194/acp-8-4499-2008, 2008.
Mohr, C., DeCarlo, P. F., Heringa, M. F., Chirico, R., Slowik, J. G., Richter, R., Reche, C., Alastuey, A., Querol, X., Seco, R., Peñuelas, J., Jiménez, J. L., Crippa, M., Zimmermann, R., Baltensperger, U., and Prévôt, A. S. H.: Identification and quantification of organic aerosol from cooking and other sources in Barcelona using aerosol mass spectrometer data, Atmos. Chem. Phys., 12, 1649–1665, https://doi.org/10.5194/acp-12-1649-2012, 2012.
Nault, B. A., Campuzano-Jost, P., Day, D. A., Schroder, J. C., Anderson, B., Beyersdorf, A. J., Blake, D. R., Brune, W. H., Choi, Y., Corr, C. A., de Gouw, J. A., Dibb, J., DiGangi, J. P., Diskin, G. S., Fried, A., Huey, L. G., Kim, M. J., Knote, C. J., Lamb, K. D., Lee, T., Park, T., Pusede, S. E., Scheuer, E., Thornhill, K. L., Woo, J.-H., and Jimenez, J. L.: Secondary organic aerosol production from local emissions dominates the organic aerosol budget over Seoul, South Korea, during KORUS-AQ, Atmos. Chem. Phys., 18, 17769–17800, https://doi.org/10.5194/acp-18-17769-2018, 2018.
Ng, N. L., Canagaratna, M. R., Zhang, Q., Jimenez, J. L., Tian, J., Ulbrich, I. M., Kroll, J. H., Docherty, K. S., Chhabra, P. S., Bahreini, R., Murphy, S. M., Seinfeld, J. H., Hildebrandt, L., Donahue, N. M., DeCarlo, P. F., Lanz, V. A., Prévôt, A. S. H., Dinar, E., Rudich, Y., and Worsnop, D. R.: Organic aerosol components observed in Northern Hemispheric datasets from Aerosol Mass Spectrometry, Atmos. Chem. Phys., 10, 4625–4641, https://doi.org/10.5194/acp-10-4625-2010, 2010.
Ng, N. L., Canagaratna, M. R., Jimenez, J. L., Chhabra, P. S., Seinfeld, J. H., and Worsnop, D. R.: Changes in organic aerosol composition with aging inferred from aerosol mass spectra, Atmos. Chem. Phys., 11, 6465–6474, https://doi.org/10.5194/acp-11-6465-2011, 2011a.
Ng, N. L., Canagaratna, M. R., Jimenez, J. L., Zhang, Q., Ulbrich, I. M., and Worsnop, D. R.: Real-Time Methods for Estimating Organic Component Mass Concentrations from Aerosol Mass Spectrometer Data, Environ. Sci. Technol., 45, 910–916, https://doi.org/10.1021/es102951k, 2011b.
Paciga, A., Karnezi, E., Kostenidou, E., Hildebrandt, L., Psichoudaki, M., Engelhart, G. J., Lee, B.-H., Crippa, M., Prévôt, A. S. H., Baltensperger, U., and Pandis, S. N.: Volatility of organic aerosol and its components in the megacity of Paris, Atmos. Chem. Phys., 16, 2013–2023, https://doi.org/10.5194/acp-16-2013-2016, 2016.
Park, S. H., Rogak, S. N., and Grieshop, A. P.: A Two-Dimensional Laminar Flow Model for Thermodenuders Applied to Vapor Pressure Measurements, Aerosol Sci. Technol., 47, 283–293, https://doi.org/10.1080/02786826.2012.750711, 2012.
Petit, J. E., Favez, O., Albinet, A., and Canonaco, F.: A user-friendly tool for comprehensive evaluation of the geographical origins of atmospheric pollution: Wind and trajectory analyses, Environ. Modell. Softw., 88, 183–187, https://doi.org/10.1016/j.envsoft.2016.11.022, 2017.
Pieber, S. M., El Haddad, I., Slowik, J. G., Canagaratna, M. R., Jayne, J. T., Platt, S. M., Bozzetti, C., Daellenbach, K. R., Fröhlich, R., Vlachou, A., Klein, F., Dommen, J., Miljevic, B., Jiménez, J. L., Worsnop, D. R., Baltensperger, U., and Prévôt, A. S. H.: Inorganic Salt Interference on CO in Aerodyne AMS and ACSM Organic Aerosol Composition Studies, Environ. Sci. Technol., 50, 10494–10503, https://doi.org/10.1021/acs.est.6b01035, 2016.
Polissar, A. V., Hopke, P. K., Paatero, P., Kaufmann, Y. J., Hall, D. K., Bodhaine, B. A., Dutton, E. G., and Harris, J. M.: The aerosol at Barrow, Alaska: long-term trends and source locations, Atmos. Environ., 33, 2441–2458, https://doi.org/10.1016/S1352-2310(98)00423-3, 1999.
Price, H. C., Mattsson, J., and Murray, B. J.: Sucrose diffusion in aqueous solution, Phys. Chem. Chem. Phys., 18, 19207–19216, https://doi.org/10.1039/c6cp03238a, 2016.
Qin, Y. M., Tan, H. B., Li, Y. J., Schurman, M. I., Li, F., Canonaco, F., Prévôt, A. S. H., and Chan, C. K.: Impacts of traffic emissions on atmospheric particulate nitrate and organics at a downwind site on the periphery of Guangzhou, China, Atmos. Chem. Phys., 17, 10245–10258, https://doi.org/10.5194/acp-17-10245-2017, 2017.
Quan, J., Zhang, Q., He, H., Liu, J., Huang, M., and Jin, H.: Analysis of the formation of fog and haze in North China Plain (NCP), Atmos. Chem. Phys., 11, 8205–8214, https://doi.org/10.5194/acp-11-8205-2011, 2011.
Reid, J. P., Bertram, A. K., Topping, D. O., Laskin, A., Martin, S. T., Petters, M. D., Pope, F. D., and Rovelli, G.: The viscosity of atmospherically relevant organic particles, Nat. Commun., 9, 956, https://doi.org/10.1038/s41467-018-03027-z, 2018.
Renbaum-Wolff, L., Grayson, J. W., Bateman, A. P., Kuwata, M., Sellier, M., Murray, B. J., Shilling, J. E., Martin, S. T., and Bertram, A. K.: Viscosity of α-pinene secondary organic material and implications for particle growth and reactivity, P. Natl. Acad. Sci. USA, 110, 8014–8019, https://doi.org/10.1073/pnas.1219548110, 2013.
Riipinen, I., Pierce, J. R., Donahue, N. M., and Pandis, S. N.: Equilibration time scales of organic aerosol inside thermodenuders: Evaporation kinetics versus thermodynamics, Atmos. Environ., 44, 597–607, https://doi.org/10.1016/j.atmosenv.2009.11.022, 2010.
Saha, P. K. and Grieshop, A. P.: Exploring Divergent Volatility Properties from Yield and Thermodenuder Measurements of Secondary Organic Aerosol from α-Pinene Ozonolysis, Environ. Sci. Technol., 50, 5740–5749, https://doi.org/10.1021/acs.est.6b00303, 2016.
Saha, P. K., Khlystov, A., and Grieshop, A. P.: Determining Aerosol Volatility Parameters Using a “Dual Thermodenuder” System: Application to Laboratory-Generated Organic Aerosols, Aerosol Sci. Tech., 49, 620–632, https://doi.org/10.1080/02786826.2015.1056769, 2015.
Saha, P. K., Khlystov, A., Yahya, K., Zhang, Y., Xu, L., Ng, N. L., and Grieshop, A. P.: Quantifying the volatility of organic aerosol in the southeastern US, Atmos. Chem. Phys., 17, 501–520, https://doi.org/10.5194/acp-17-501-2017, 2017.
Saha, P. K., Khlystov, A., and Grieshop, A. P.: Downwind evolution of the volatility and mixing state of near-road aerosols near a US interstate highway, Atmos. Chem. Phys., 18, 2139–2154, https://doi.org/10.5194/acp-18-2139-2018, 2018.
Sato, K., Fujitani, Y., Inomata, S., Morino, Y., Tanabe, K., Ramasamy, S., Hikida, T., Shimono, A., Takami, A., Fushimi, A., Kondo, Y., Imamura, T., Tanimoto, H., and Sugata, S.: Studying volatility from composition, dilution, and heating measurements of secondary organic aerosols formed during α-pinene ozonolysis, Atmos. Chem. Phys., 18, 5455–5466, https://doi.org/10.5194/acp-18-5455-2018, 2018.
Sato, K., Fujitani, Y., Inomata, S., Morino, Y., Tanabe, K., Hikida, T., Shimono, A., Takami, A., Fushimi, A., Kondo, Y., Imamura, T., Tanimoto, H., and Sugata, S.: A study of volatility by composition, heating, and dilution measurements of secondary organic aerosol from 1,3,5-trimethylbenzene, Atmos. Chem. Phys., 19, 14901–14915, https://doi.org/10.5194/acp-19-14901-2019, 2019.
Seinfeld, J. H. and Pandis, S. N.: Atmospheric chemistry and physics – from air pollution to climate change, 3rd Edn., ISBN 978-1-119-22117-3, Wiley, 2016.
Shiraiwa, M. and Seinfeld, J. H.: Equilibration timescale of atmospheric secondary organic aerosol partitioning, Geophys. Res. Lett., 39, L24801, https://doi.org/10.1029/2012gl054008, 2012.
Shiraiwa, M., Ammann, M., Koop, T., and Pöschl, U.: Gas uptake and chemical aging of semisolid organic aerosol particles, P. Natl. Acad. Sci. USA, 108, 11003–11008, https://doi.org/10.1073/pnas.1103045108, 2011.
Shiraiwa, M., Pfrang, C., Koop, T., and Pöschl, U.: Kinetic multi-layer model of gas-particle interactions in aerosols and clouds (KM-GAP): linking condensation, evaporation and chemical reactions of organics, oxidants and water, Atmos. Chem. Phys., 12, 2777–2794, https://doi.org/10.5194/acp-12-2777-2012, 2012.
Shiraiwa, M., Li, Y., Tsimpidi, A. P., Karydis, V. A., Berkemeier, T., Pandis, S. N., Lelieveld, J., Koop, T., and Pöschl, U.: Global distribution of particle phase state in atmospheric secondary organic aerosols, Nat. Commun., 8, 15002, https://doi.org/10.1038/ncomms15002, 2017.
Song, Z., Fu, D., Zhang, X., Wu, Y., Xia, X., He, J., Han, X., Zhang, R., and Che, H.: Diurnal and seasonal variability of PM2.5 and AOD in North China plain: Comparison of MERRA-2 products and ground measurements, Atmos. Environ., 191, 70–78, https://doi.org/10.1016/j.atmosenv.2018.08.012, 2018.
Squizzato, S., Masiol, M., Innocente, E., Pecorari, E., Rampazzo, G., and Pavoni, B.: A procedure to assess local and long-range transport contributions to PM2.5 and secondary inorganic aerosol, J. Aerosol Sci., 46, 64–76, https://doi.org/10.1016/j.jaerosci.2011.12.001, 2012.
Sun, J., Huang, L., Liao, H., Li, J., and Hu, J.: Impacts of Regional Transport on Particulate Matter Pollution in China: a Review of Methods and Results, Current Pollution Reports, 3, 182–191, https://doi.org/10.1007/s40726-017-0065-5, 2017.
Sun, Y., Wang, Z., Dong, H., Yang, T., Li, J., Pan, X., Chen, P., and Jayne, J. T.: Characterization of summer organic and inorganic aerosols in Beijing, China with an Aerosol Chemical Speciation Monitor, Atmos. Environ., 51, 250–259, https://doi.org/10.1016/j.atmosenv.2012.01.013, 2012.
Sun, Y., Jiang, Q., Wang, Z., Fu, P., Li, J., Yang, T., and Yin, Y.: Investigation of the sources and evolution processes of severe haze pollution in Beijing in January 2013, J. Geophys. Res.-Atmos., 119, 4380–4398, https://doi.org/10.1002/2014jd021641, 2014.
Sun, Y., Chen, C., Zhang, Y., Xu, W., Zhou, L., Cheng, X., Zheng, H., Ji, D., Li, J., Tang, X., Fu, P., and Wang, Z.: Rapid formation and evolution of an extreme haze episode in Northern China during winter 2015, Sci. Rep., 6, 27151, https://doi.org/10.1038/srep27151, 2016.
Sun, Y. L., Wang, Z. F., Fu, P. Q., Yang, T., Jiang, Q., Dong, H. B., Li, J., and Jia, J. J.: Aerosol composition, sources and processes during wintertime in Beijing, China, Atmos. Chem. Phys., 13, 4577–4592, https://doi.org/10.5194/acp-13-4577-2013, 2013.
Sun, Y. L., Wang, Z. F., Du, W., Zhang, Q., Wang, Q. Q., Fu, P. Q., Pan, X. L., Li, J., Jayne, J., and Worsnop, D. R.: Long-term real-time measurements of aerosol particle composition in Beijing, China: seasonal variations, meteorological effects, and source analysis, Atmos. Chem. Phys., 15, 10149–10165, https://doi.org/10.5194/acp-15-10149-2015, 2015.
Ulbrich, I. M., Canagaratna, M. R., Zhang, Q., Worsnop, D. R., and Jimenez, J. L.: Interpretation of organic components from Positive Matrix Factorization of aerosol mass spectrometric data, Atmos. Chem. Phys., 9, 2891–2918, https://doi.org/10.5194/acp-9-2891-2009, 2009.
Virtanen, A., Joutsensaari, J., Koop, T., Kannosto, J., Yli-Pirila, P., Leskinen, J., Makela, J. M., Holopainen, J. K., Pöschl, U., Kulmala, M., Worsnop, D. R., and Laaksonen, A.: An amorphous solid state of biogenic secondary organic aerosol particles, Nature, 467, 824–827, https://doi.org/10.1038/nature09455, 2010.
Vlachou, A., Tobler, A., Lamkaddam, H., Canonaco, F., Daellenbach, K. R., Jaffrezo, J.-L., Minguillón, M. C., Maasikmets, M., Teinemaa, E., Baltensperger, U., El Haddad, I., and Prévôt, A. S. H.: Development of a versatile source apportionment analysis based on positive matrix factorization: a case study of the seasonal variation of organic aerosol sources in Estonia, Atmos. Chem. Phys., 19, 7279–7295, https://doi.org/10.5194/acp-19-7279-2019, 2019.
Waked, A., Favez, O., Alleman, L. Y., Piot, C., Petit, J.-E., Delaunay, T., Verlinden, E., Golly, B., Besombes, J.-L., Jaffrezo, J.-L., and Leoz-Garziandia, E.: Source apportionment of PM10 in a north-western Europe regional urban background site (Lens, France) using positive matrix factorization and including primary biogenic emissions, Atmos. Chem. Phys., 14, 3325–3346, https://doi.org/10.5194/acp-14-3325-2014, 2014.
Wang, Q., Sun, Y., Jiang, Q., Du, W., Sun, C., Fu, P., and Wang, Z.: Chemical composition of aerosol particles and light extinction apportionment before and during the heating season in Beijing, China, J. Geophys. Res.-Atmos., 120, 12708–12722, https://doi.org/10.1002/2015jd023871, 2015.
Wang, Y., Chen, Y., Wu, Z., Shang, D., Bian, Y., Du, Z., Schmitt, S. H., Su, R., Gkatzelis, G. I., Schlag, P., Hohaus, T., Voliotis, A., Lu, K., Zeng, L., Zhao, C., Alfarra, M. R., McFiggans, G., Wiedensohler, A., Kiendler-Scharr, A., Zhang, Y., and Hu, M.: Mutual promotion between aerosol particle liquid water and particulate nitrate enhancement leads to severe nitrate-dominated particulate matter pollution and low visibility, Atmos. Chem. Phys., 20, 2161–2175, https://doi.org/10.5194/acp-20-2161-2020, 2020.
Xie, Y., Wang, G., Wang, X., Chen, J., Chen, Y., Tang, G., Wang, L., Ge, S., Xue, G., Wang, Y., and Gao, J.: Nitrate-dominated PM2.5 and elevation of particle pH observed in urban Beijing during the winter of 2017, Atmos. Chem. Phys., 20, 5019–5033, https://doi.org/10.5194/acp-20-5019-2020, 2020.
Xu, L., Williams, L. R., Young, D. E., Allan, J. D., Coe, H., Massoli, P., Fortner, E., Chhabra, P., Herndon, S., Brooks, W. A., Jayne, J. T., Worsnop, D. R., Aiken, A. C., Liu, S., Gorkowski, K., Dubey, M. K., Fleming, Z. L., Visser, S., Prévôt, A. S. H., and Ng, N. L.: Wintertime aerosol chemical composition, volatility, and spatial variability in the greater London area, Atmos. Chem. Phys., 16, 1139–1160, https://doi.org/10.5194/acp-16-1139-2016, 2016.
Xu, P., Zhang, J., Ji, D., Liu, Z., Tang, G., Jiang, C., and Wang, Y.: Characterization of submicron particles during autumn in Beijing, China, J. Environ. Sci., 63, 16–27, https://doi.org/10.1016/j.jes.2017.03.036, 2018.
Xu, W., Han, T., Du, W., Wang, Q., Chen, C., Zhao, J., Zhang, Y., Li, J., Fu, P., Wang, Z., Worsnop, D. R., and Sun, Y.: Effects of Aqueous-Phase and Photochemical Processing on Secondary Organic Aerosol Formation and Evolution in Beijing, China, Environ. Sci. Technol., 51, 762–770, https://doi.org/10.1021/acs.est.6b04498, 2017.
Xu, W., Xie, C., Karnezi, E., Zhang, Q., Wang, J., Pandis, S. N., Ge, X., Zhang, J., An, J., Wang, Q., Zhao, J., Du, W., Qiu, Y., Zhou, W., He, Y., Li, Y., Li, J., Fu, P., Wang, Z., Worsnop, D. R., and Sun, Y.: Summertime aerosol volatility measurements in Beijing, China, Atmos. Chem. Phys., 19, 10205–10216, https://doi.org/10.5194/acp-19-10205-2019, 2019.
Xu, W., Chen, C., Qiu, Y., Li, Y., Zhang, Z., Karnezi, E., Pandis, S. N., Xie, C., Li, Z., Sun, J., Ma, N., Xu, W., Fu, P., Wang, Z., Zhu, J., Worsnop, D. R., Ng, N. L., and Sun, Y.: Organic aerosol volatility and viscosity in the North China Plain: contrast between summer and winter, Atmos. Chem. Phys., 21, 5463–5476, https://doi.org/10.5194/acp-21-5463-2021, 2021.
Xu, W. Q., Sun, Y. L., Chen, C., Du, W., Han, T. T., Wang, Q. Q., Fu, P. Q., Wang, Z. F., Zhao, X. J., Zhou, L. B., Ji, D. S., Wang, P. C., and Worsnop, D. R.: Aerosol composition, oxidation properties, and sources in Beijing: results from the 2014 Asia-Pacific Economic Cooperation summit study, Atmos. Chem. Phys., 15, 13681–13698, https://doi.org/10.5194/acp-15-13681-2015, 2015.
Yan, Y., Liu, Z., Gao, W., Li, J., Zhang, X., Chai, W., Bai, J., Hu, B., and Wang, Y.: Physiochemistry characteristics and sources of submicron aerosols at the background area of North China Plain: Implication of air pollution control in heating season, Atmos. Res., 249, 105291, https://doi.org/10.1016/j.atmosres.2020.105291, 2021.
Yang, S., Yuan, B., Peng, Y., Huang, S., Chen, W., Hu, W., Pei, C., Zhou, J., Parrish, D. D., Wang, W., He, X., Cheng, C., Li, X.-B., Yang, X., Song, Y., Wang, H., Qi, J., Wang, B., Wang, C., Wang, C., Wang, Z., Li, T., Zheng, E., Wang, S., Wu, C., Cai, M., Ye, C., Song, W., Cheng, P., Chen, D., Wang, X., Zhang, Z., Wang, X., Zheng, J., and Shao, M.: The formation and mitigation of nitrate pollution: comparison between urban and suburban environments, Atmos. Chem. Phys., 22, 4539–4556, https://doi.org/10.5194/acp-22-4539-2022, 2022.
Yao, L., Yang, L., Yuan, Q., Yan, C., Dong, C., Meng, C., Sui, X., Yang, F., Lu, Y., and Wang, W.: Sources apportionment of PM2.5 in a background site in the North China Plain, Sci. Total Environ., 541, 590–598, https://doi.org/10.1016/j.scitotenv.2015.09.123, 2016.
Yli-Juuti, T., Pajunoja, A., Tikkanen, O. P., Buchholz, A., Faiola, C., Vaisanen, O., Hao, L., Kari, E., Perakyla, O., Garmash, O., Shiraiwa, M., Ehn, M., Lehtinen, K., and Virtanen, A.: Factors controlling the evaporation of secondary organic aerosol from alpha-pinene ozonolysis, Geophys. Res. Lett., 44, 2562–2570, https://doi.org/10.1002/2016GL072364, 2017.
Ylisirniö, A., Buchholz, A., Mohr, C., Li, Z., Barreira, L., Lambe, A., Faiola, C., Kari, E., Yli-Juuti, T., Nizkorodov, S. A., Worsnop, D. R., Virtanen, A., and Schobesberger, S.: Composition and volatility of secondary organic aerosol (SOA) formed from oxidation of real tree emissions compared to simplified volatile organic compound (VOC) systems, Atmos. Chem. Phys., 20, 5629–5644, https://doi.org/10.5194/acp-20-5629-2020, 2020.
Zhang, J., Wang, Y., Huang, X., Liu, Z., Ji, D., and Sun, Y.: Characterization of organic aerosols in Beijing using an aerodyne high-resolution aerosol mass spectrometer, Adv. Atmos. Sci., 32, 877–888, https://doi.org/10.1007/s00376-014-4153-9, 2015.
Zhang, J. K., Sun, Y., Liu, Z. R., Ji, D. S., Hu, B., Liu, Q., and Wang, Y. S.: Characterization of submicron aerosols during a month of serious pollution in Beijing, 2013, Atmos. Chem. Phys., 14, 2887–2903, https://doi.org/10.5194/acp-14-2887-2014, 2014.
Zhang, J. K., Cheng, M. T., Ji, D. S., Liu, Z. R., Hu, B., Sun, Y., and Wang, Y. S.: Characterization of submicron particles during biomass burning and coal combustion periods in Beijing, China, Sci. Total Environ., 562, 812–821, https://doi.org/10.1016/j.scitotenv.2016.04.015, 2016.
Zhang, J. P., Zhu, T., Zhang, Q. H., Li, C. C., Shu, H. L., Ying, Y., Dai, Z. P., Wang, X., Liu, X. Y., Liang, A. M., Shen, H. X., and Yi, B. Q.: The impact of circulation patterns on regional transport pathways and air quality over Beijing and its surroundings, Atmos. Chem. Phys., 12, 5031–5053, https://doi.org/10.5194/acp-12-5031-2012, 2012.
Zhang, Q., Worsnop, D. R., Canagaratna, M. R., and Jimenez, J. L.: Hydrocarbon-like and oxygenated organic aerosols in Pittsburgh: insights into sources and processes of organic aerosols, Atmos. Chem. Phys., 5, 3289–3311, https://doi.org/10.5194/acp-5-3289-2005, 2005a.
Zhang, Q., Alfarra, M. R., Worsnop, D. R., Allan, J. D., Coe, H., Canagaratna, M. R., and Jimenez, J. L.: Deconvolution and Quantification of Hydrocarbon-like and Oxygenated Organic Aerosols Based on Aerosol Mass Spectrometry, Environ. Sci. Technol., 39, 4938–4952, https://doi.org/10.1021/es048568l, 2005b.
Zhang, Q., Jimenez, J. L., Canagaratna, M. R., Allan, J. D., Coe, H., Ulbrich, I., Alfarra, M. R., Takami, A., Middlebrook, A. M., Sun, Y. L., Dzepina, K., Dunlea, E., Docherty, K., DeCarlo, P. F., Salcedo, D., Onasch, T., Jayne, J. T., Miyoshi, T., Shimono, A., Hatakeyama, S., Takegawa, N., Kondo, Y., Schneider, J., Drewnick, F., Borrmann, S., Weimer, S., Demerjian, K., Williams, P., Bower, K., Bahreini, R., Cottrell, L., Griffin, R. J., Rautiainen, J., Sun, J. Y., Zhang, Y. M., and Worsnop, D. R.: Ubiquity and dominance of oxygenated species in organic aerosols in anthropogenically-influenced Northern Hemisphere midlatitudes, Geophys. Res. Lett., 34, L13801, https://doi.org/10.1029/2007GL029979, 2007.
Zhang, Q., Jimenez, J. L., Canagaratna, M. R., Ulbrich, I. M., Ng, N. L., Worsnop, D. R., and Sun, Y.: Understanding atmospheric organic aerosols via factor analysis of aerosol mass spectrometry: a review, Anal. Bioanal. Chem., 401, 3045–3067, https://doi.org/10.1007/s00216-011-5355-y, 2011.
Zhang, R., Jing, J., Tao, J., Hsu, S.-C., Wang, G., Cao, J., Lee, C. S. L., Zhu, L., Chen, Z., Zhao, Y., and Shen, Z.: Chemical characterization and source apportionment of PM2.5 in Beijing: seasonal perspective, Atmos. Chem. Phys., 13, 7053–7074, https://doi.org/10.5194/acp-13-7053-2013, 2013.
Zhang, Y., Du, W., Wang, Y., Wang, Q., Wang, H., Zheng, H., Zhang, F., Shi, H., Bian, Y., Han, Y., Fu, P., Canonaco, F., Prévôt, A. S. H., Zhu, T., Wang, P., Li, Z., and Sun, Y.: Aerosol chemistry and particle growth events at an urban downwind site in North China Plain, Atmos. Chem. Phys., 18, 14637–14651, https://doi.org/10.5194/acp-18-14637-2018, 2018.
Zhang, Y., Sun, J., Zheng, P., Chen, T., Liu, Y., Han, G., Simpson, I. J., Wang, X., Blake, D. R., Li, Z., Yang, X., Qi, Y., Wang, Q., Wang, W., and Xue, L.: Observations of C1–C5 alkyl nitrates in the Yellow River Delta, northern China: Effects of biomass burning and oil field emissions, Sci. Total Environ., 656, 129–139, https://doi.org/10.1016/j.scitotenv.2018.11.208, 2019.
Zhang, Y., Liu, J., Tao, W., Xiang, S., Liu, H., Yi, K., Yang, H., Xu, J., Wang, Y., Ma, J., Wang, X., Hu, J., Wan, Y., Wang, X., and Tao, S.: Impacts of chlorine emissions on secondary pollutants in China, Atmos. Environ., 246, 118177, https://doi.org/10.1016/j.atmosenv.2020.118177, 2020.
Zhao, J., Du, W., Zhang, Y., Wang, Q., Chen, C., Xu, W., Han, T., Wang, Y., Fu, P., Wang, Z., Li, Z., and Sun, Y.: Insights into aerosol chemistry during the 2015 China Victory Day parade: results from simultaneous measurements at ground level and 260 m in Beijing, Atmos. Chem. Phys., 17, 3215–3232, https://doi.org/10.5194/acp-17-3215-2017, 2017.
Zhao, J., Qiu, Y., Zhou, W., Xu, W., Wang, J., Zhang, Y., Li, L., Xie, C., Wang, Q., Du, W., Worsnop, D. R., Canagaratna, M. R., Zhou, L., Ge, X., Fu, P., Li, J., Wang, Z., Donahue, N. M., and Sun, Y.: Organic Aerosol Processing During Winter Severe Haze Episodes in Beijing, J. Geophys. Res.-Atmos., 124, 10248–10263, https://doi.org/10.1029/2019jd030832, 2019.
Zhao, Z., Yang, X., Lee, J., Tolentino, R., Mayorga, R., Zhang, W., and Zhang, H.: Diverse Reactions in Highly Functionalized Organic Aerosols during Thermal Desorption, ACS Earth Space Chem., 4, 283–296, https://doi.org/10.1021/acsearthspacechem.9b00312, 2020.
Zheng, G. J., Duan, F. K., Su, H., Ma, Y. L., Cheng, Y., Zheng, B., Zhang, Q., Huang, T., Kimoto, T., Chang, D., Pöschl, U., Cheng, Y. F., and He, K. B.: Exploring the severe winter haze in Beijing: the impact of synoptic weather, regional transport and heterogeneous reactions, Atmos. Chem. Phys., 15, 2969–2983, https://doi.org/10.5194/acp-15-2969-2015, 2015.
Zheng, J., Hu, M., Du, Z., Shang, D., Gong, Z., Qin, Y., Fang, J., Gu, F., Li, M., Peng, J., Li, J., Zhang, Y., Huang, X., He, L., Wu, Y., and Guo, S.: Influence of biomass burning from South Asia at a high-altitude mountain receptor site in China, Atmos. Chem. Phys., 17, 6853–6864, https://doi.org/10.5194/acp-17-6853-2017, 2017.
Zheng, J., Zhong, L., Wang, T., Louie, P. K. K., and Li, Z.: Ground-level ozone in the Pearl River Delta region: Analysis of data from a recently established regional air quality monitoring network, Atmos. Environ., 44, 814–823, https://doi.org/10.1016/j.atmosenv.2009.11.032, 2010.
Zheng, P., Chen, T., Dong, C., Liu, Y., Li, H., Han, G., Sun, J., Wu, L., Gao, X., Wang, X., Qi, Y., Zhang, Q., Wang, W., and Xue, L.: Characteristics and sources of halogenated hydrocarbons in the Yellow River Delta region, northern China, Atmos. Res., 225, 70–80, https://doi.org/10.1016/j.atmosres.2019.03.039, 2019.
Zhou, S., Collier, S., Xu, J., Mei, F., Wang, J., Lee, Y.-N., Sedlacek, A. J., Springston, S. R., Sun, Y., and Zhang, Q.: Influences of upwind emission sources and atmospheric processing on aerosol chemistry and properties at a rural location in the Northeastern U.S, J. Geophys. Res.-Atmos., 121, 6049–6065, https://doi.org/10.1002/2015jd024568, 2016.
Zhou, W., Sun, Y., Xu, W., Zhao, X., Wang, Q., Tang, G., Zhou, L., Chen, C., Du, W., Zhao, J., Xie, C., Fu, P., and Wang, Z.: Vertical Characterization of Aerosol Particle Composition in Beijing, China: Insights From 3-Month Measurements With Two Aerosol Mass Spectrometers, J. Geophys. Res.-Atmos., 123, 13016–13029, https://doi.org/10.1029/2018jd029337, 2018.
Zhou, W., Xu, W., Kim, H., Zhang, Q., Fu, P., Worsnop, D. R., and Sun, Y.: A review of aerosol chemistry in Asia: insights from aerosol mass spectrometer measurements, Environ. Sci. Process. Impacts, 22, 1616–1653, https://doi.org/10.1039/d0em00212g, 2020.
Zhu, Q., Cao, L.-M., Tang, M.-X., Huang, X.-F., Saikawa, E., and He, L.-Y.: Characterization of Organic Aerosol at a Rural Site in the North China Plain Region: Sources, Volatility and Organonitrates, Adv. Atmos. Sci., 38, 1115–1127, https://doi.org/10.1007/s00376-020-0127-2, 2021.
Zorn, S. R., Drewnick, F., Schott, M., Hoffmann, T., and Borrmann, S.: Characterization of the South Atlantic marine boundary layer aerosol using an aerodyne aerosol mass spectrometer, Atmos. Chem. Phys., 8, 4711–4728, https://doi.org/10.5194/acp-8-4711-2008, 2008.