the Creative Commons Attribution 4.0 License.
the Creative Commons Attribution 4.0 License.
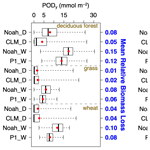
Satellite soil moisture data assimilation impacts on modeling weather variables and ozone in the southeastern US – Part 2: Sensitivity to dry-deposition parameterizations
James H. Crawford
Gregory R. Carmichael
Kevin W. Bowman
Sujay V. Kumar
Colm Sweeney
Ozone (O3) dry deposition is a major O3 sink. As a follow-up study of Huang et al. (2021), we quantify the impact of satellite soil moisture (SM) on model representations of this process when different dry-deposition parameterizations are implemented, based on which the implications for interpreting O3 air pollution levels and assessing the O3 impacts on human and ecosystem health are provided. The SM data from NASA's Soil Moisture Active Passive mission are assimilated into the Noah-Multiparameterization (Noah-MP) land surface model within the NASA Land Information System framework, semicoupled with Weather Research and Forecasting model with online Chemistry (WRF-Chem) regional-scale simulations covering the southeastern US. Major changes in the modeling system used include enabling the dynamic vegetation option, adding the irrigation process, and updating the scheme for the surface exchange coefficient. Two dry-deposition schemes are implemented, i.e., the Wesely scheme and a “dynamic” scheme, in the latter of which dry-deposition parameterization is coupled with photosynthesis and vegetation dynamics. It is demonstrated that, when the dynamic scheme is applied, the simulated O3 dry-deposition velocities vd and their stomatal and cuticular portions, as well as the total O3 fluxes Ft, are larger overall; vd and Ft are 2–3 times more sensitive to the SM changes due to the data assimilation (DA). Further, through case studies at two forested sites with different soil types and hydrological regimes, we highlight that, applying the Community Land Model type of SM factor controlling stomatal resistance (i.e., β factor) scheme in replacement of the Noah-type β factor scheme reduced the vd sensitivity to SM changes by ∼75 % at one site, while it doubled this sensitivity at the other site. Referring to multiple evaluation datasets, which may be associated with variable extents of uncertainty, the model performance of vegetation, surface fluxes, weather, and surface O3 concentrations shows mixed responses to the DA, some of which display land cover dependency. Finally, using model-derived concentration- and flux-based policy-relevant O3 metrics as well as their matching exposure–response functions, the relative biomass/crop yield losses for several types of vegetation/crops are estimated to be within a wide range of 1 %–17 %. Their sensitivities to the model's dry-deposition scheme and the implementation of SM DA are discussed.
- Article
(23655 KB) - Companion paper
-
Supplement
(6458 KB) - BibTeX
- EndNote
Ground-level ozone (O3) is a regulated secondary air pollutant harmful to human and ecosystem health (Fleming et al., 2018; Mills et al., 2018a, b). It is closely connected with O3 at higher altitudes where O3 plays a more important role in the Earth's climate system by trapping infrared radiation and absorbing ultraviolet radiation (e.g., Lacis et al., 1990). To better protect human health and public welfare, in 2015, the US primary and secondary National Ambient Air Quality Standards were lowered from 75 to 70 ppbv, in the format of daily maximum 8 h average (MDA8). Several other O3-exposure-based metrics have also been applied and/or proposed to assess O3 impacts on vegetation, such as the accumulated O3 exposure over given thresholds (e.g., SUM40, SUM60, and AOT40), the averaged O3 exposure during daylight hours (e.g., M7 and M12), and the sigmoidal-weighted W126 cumulative exposure (e.g., Fredericksen et al., 1996; van Dingenen et al., 2009; Hemispheric Transport of Air Pollution, 2010, and references therein; Avnery et al., 2011; Hollaway et al., 2012; Huang et al., 2013; Lapina et al., 2014; Mills et al., 2007, 2018a, b). To help comply with the tighter air quality standards, an improved understanding of the individual processes affecting the (near-)surface O3 concentrations and exceedances is demanded. Many O3-related processes are highly sensitive to environmental and/or biophysical conditions (e.g., Steinkamp and Lawrence, 2011; Strode et al., 2015; Jiang et al., 2018; Huang et al., 2021, and references therein). These O3-related processes include dry deposition of O3 and its precursors, which is a major sink for near-surface O3 and depends on dry-deposition velocities (vd) and the deposited chemicals' concentrations (Baublitz et al., 2020; Huang et al., 2021). As recognized in numerous studies, accurately estimating dry-deposition fluxes is critical to understanding O3 budgets and exceedances in the past, present, and future (e.g., Stevenson et al., 2006; Griffiths et al., 2021); moreover, it could contribute to a more reasonable assessment of the O3 impacts on vegetation (e.g., Mills et al., 2011; Lombardozzi et al., 2015; Mills et al., 2018a; Ducker et al., 2018; Ronan et al., 2020; Fu et al., 2022), which is also relevant to the budgets of other greenhouse gases, weather, and climate.
Ozone uptake by plants is generally higher in warm/growing seasons and during the daytime when O3 concentrations and vd values peak. As introduced in Huang et al. (2021) and references therein, over the land, surface resistance rc, which is composed of stomatal–mesophyll (rs–rm), cuticular (rlu), in-canopy, and ground resistance terms, often exerts the strongest effects on the magnitude and variability of vd. vd also includes the aerodynamic resistance (ra) and quasi-laminar sublayer resistance (rb) terms.
Soil moisture (SM) and its variability impact vd in the following ways: (1) SM can play a key role in controlling the opening and closing of plants' stomata as well as the mesophyll functioning (Egea et al., 2011; Baillie and Fleming, 2019), and thus it can directly affect the rs and rm terms of vd. (2) SM is closely linked with vegetation attributes, such as the growing-season above-ground biomass, which is often expressed as leaf area index (LAI) or vegetation optical depth (VOD) and controls the stomatal and cuticular uptake of O3-related species. (3) SM as well as vegetation conditions can affect multiple vd terms through its interactions with other environmental conditions (e.g., temperatures, radiation, precipitation, and humidity fields) that modulate these vd terms, and such effects are generally stronger over transitional climate zones located between dry and wet climates. The SM impacts on vd and atmospheric states through the above-mentioned pathways are likely to continue to grow in future. This is because, according to Intergovernmental Panel on Climate Change (2021), the occurrence and severity of droughts, some of which are characterized by surface and/or column-averaged SM deficits, are projected to increase over many US regions under warmer future environments. Better understanding the potentially enhanced SM dependency of dry deposition and weather conditions under the changing climate is important because O3 stress, together with heat, water, and other stresses, can pose more complex threats to plant health than single stress alone (Otu-Larbi et al., 2020).
Single-point models and three-dimensional chemical transport models have long been used to estimate vd values and their responses to climate change. In the widely used, empirical Wesely scheme (Wesely, 1989), vd is sensitive to only a few meteorological variables, with SM and plants' physiological effects ignored. In previous studies, Wesely-scheme-based vd fluxes as well as their various terms from different global, regional, and point-scale modeling systems were intercompared and/or evaluated with vd and rs observations from sparsely distributed sites (e.g., Val Martin et al., 2014; Hardacre et al., 2015; Clifton et al., 2017; Silva and Heald, 2018; Wu et al., 2018; Lin et al., 2019) in terms of their magnitude and variability. Studies such as Hardacre et al. (2015) show that, even when similar (Wesely and Wesely-like) vd schemes were applied, various models behaved differently in calculating vd, reflecting the impacts of land use and land cover (LULC) and meteorological fields which depend on the individual models' configurations (e.g., scales, inputs). In almost all above-cited studies, large model–model and model–observation discrepancies (i.e., by a factor of 2 or more) have been found in places, suggesting the strong need of diagnosing and addressing issues in the models' configurations and dry-deposition parameterizations.
Revised or alternative dry-deposition schemes have been applied in an increasing number of global- and regional-scale modeling studies. In some of these works, stomatal conductance is calculated based on one-big-leaf multiplicative algorithms that are more complicated than the Wesely (1989) approach, in the way that the empirical maximum stomatal conductance is adjusted by more factors, including water availability and vegetation attributes (e.g., Anav et al., 2018; Falk and Søvde Haslerud, 2019; Emmerichs et al., 2021). In others, vd calculations are coupled with photosynthesis and vegetation phenology (e.g., Val Martin et al., 2014; Wu et al., 2018; Lin et al., 2019; Wong et al., 2019; Clifton et al., 2020), which in this paper are frequently referred to as “dynamic” schemes. Such types of modifications have been the recommended directions for improving the estimates of vd as well as the vd and O3 responses to climate change, in that they have been demonstrated to be capable of enhancing the dynamics and reducing the systematic biases of the modeled vd. However, results based on such updated vd schemes are still associated with variable extents of uncertainty due to limitations in model parameterizations (related to structures, empirical parameters, and stress functions) and/or configurations. In some existing works that applied the dynamic schemes, such uncertainty was quantified and addressed by simply scaling the fluxes resulting from the dynamic schemes towards flux measurements available at very limited locations during non-recent time periods (e.g., Val Martin et al., 2014). These types of modified dry-deposition schemes still require further investigations and optimizations, which can be approached by (1) quantifying the sensitivities of process-based model variables to SM and other environmental and/or biophysical variables for various LULC and soil types; (2) improving model representations of processes central to SM states and land–atmosphere interactions, such as including irrigation and other human activities, tuning physics schemes (e.g., those related to the surface exchange coefficient, CH) in land surface models (LSMs), and using available observations to constrain (some of) the key land variables in models; and (3) including a wide range of observations and/or observation-derived carbon, water, and energy fluxes as well as vegetation states in model evaluation for broad geographical regions. Furthermore, it is important to explicitly connect the progress in dry-deposition modeling with the impact assessments of O3 and other air pollutants on ecosystem health, productivity, and diversity.
A regional-scale land modeling and SM data assimilation (DA) framework coupled with weather and atmospheric chemistry modeling by the Weather Research and Forecasting model with online Chemistry (WRF-Chem) is implemented in this work. Using this tool, we quantify and discuss the responses of vd and its key components as well as O3 concentrations and plant uptake to SM changes due to the DA, for different soil texture, LULC, and crop types. The central parts of this work rely on the Noah-Multiparameterization (Noah-MP; Niu et al., 2011) LSM with dynamic vegetation that enables the implementation of a modified dynamic dry-deposition scheme. This implemented dynamic scheme couples the rs calculation with photosynthesis for sunlit and shaded leaves and the rlu calculation with vegetation phenology. With this modified scheme, both the indirect (i.e., via changing weather and vegetation fields) and direct effects of SM on dry deposition are considered in this modeling system. Results based on this modified scheme and the WRF-Chem default Wesely scheme are compared and evaluated with independent datasets. As an extended work of Huang et al. (2021), here we continue to focus on the southeastern US during summer 2016 for which period prior Noah- and Wesely-based model calculations were conducted and aircraft observations are available. This paper introduces the applied two dry-deposition schemes in Sect. 2. It then presents SM and vegetation states (Sect. 3.1), surface fluxes, and weather fields (Sect. 3.2) from this Noah-MP-based modeling system, in comparison with those from Huang et al. (2021). Discussions on O3 concentrations and fluxes based on all related WRF-Chem simulations are also connected with the assessment of O3 impacts on societies, ecosystem health, and crop yield (Sect. 3.3). Summary and suggestions on future directions are provided in Sect. 4.
2.1 Modeling and DA experiment design
The modeling tools and DA experiment design of this study were largely consistent with the Huang et al. (2021) study: we conducted model simulations over the southeastern US in a semicoupled Land Information System (LIS)–WRF-Chem system without and with the assimilation of the enhanced SM retrievals from NASA's Soil Moisture Active Passive (SMAP; Entekhabi et al., 2010) mission. Two dry-deposition schemes (details in Sect. 2.3) were applied in cases without and with the SM DA. The 12 km/63 vertical layer Lambert conformal grid, atmospheric/land initialization, and SM DA methods were adapted from our previous study based on the Noah LSM. Major model input datasets and physics and chemistry schemes were kept similar to before except a few aspects relevant to the upgrade of LSM from Noah to Noah-MP (version 3.6) and the implementation of an irrigation scheme to be introduced in Sect. 2.2.
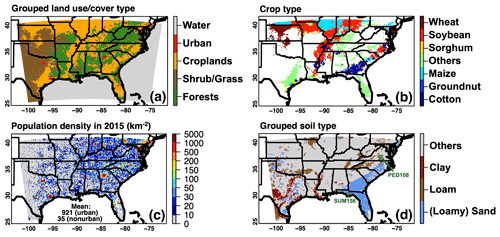
Figure 1(a) Grid-dominant land use/land cover types grouped from the original 20-category model input (Fig. 1c in Huang et al., 2021) based on the method in Table S1, (b) grid-dominant crop type over cropland-dominant regions, (c) gridded population density in 2015, and (d) highlighted grid-dominant soil types of sand/loamy sand, loam, and clay which are most relevant to discussions in this paper. The original soil type input from the State Soil Geographic database is shown in Fig. S1 in Huang et al. (2021). Locations of the two CASTNET sites for the case studies are denoted in green.
As in Huang et al. (2021), the LULC and soil texture type inputs of our coupled modeling system were based on the International Geosphere-Biosphere Programme-modified Moderate Resolution Imaging Spectroradiometer dataset (Table S1) and the State Soil Geographic dataset, respectively. Crop type data from Monfreda et al. (2008) were used in the irrigation scheme and the assessment of the O3 impacts on vegetation (Fig. 1b), which are roughly consistent with the 2016 records from the US Department of Agriculture National Agricultural Statistics Service for several major crops such as maize, soybean, and wheat (https://nassgeodata.gmu.edu/CropScape, last access: 8 November 2021). In Sect. 3 of this paper, model results are summarized and/or discussed by groups of grid-dominant LULC and soil type that are shown in Fig. 1a and d. The original 20 LULC types were grouped into urban and non-urban areas and for vegetation-dominant areas, into forests, croplands, and shrub/grasslands, following the criteria introduced in Table S1. The grid-dominant LULC groups for vegetated regions used in our analysis are vastly similar to independently developed data products, e.g., a dataset derived from the European Space Agency–Climate Change Initiative Land Cover project (https://gwis.jrc.ec.europa.eu/apps/country.profile/overview/USA, last access: 8 November 2021) and the 2016 National Land Cover Database (Wickham et al., 2021). Urban-dominant grid cells are well aligned with dense population areas (Fig. 1c) based on the Gridded Population of the World version 4.11 (NASA Socioeconomic Data and Applications Center, 2018). Grid-scale discrepancies exist between the LULC input used and independent LULC products, which, however, are not anticipated to considerably impact the results averaged by LULC groups. Three groups of soil are highlighted, namely sand/loamy sand, loam, and clay. The original sand and loamy sand categories are combined because of their high sand fractions (http://www.soilinfo.psu.edu/index.cgi?soil_data&conus&data_cov&fract&methods, last access: 10 December 2021).
2.2 Physics and configurations of the Noah-MP LSM
The Noah-MP LSM includes a number of improvements from Noah, and one of the enhanced features in Noah-MP is that it contains a separate canopy layer that explicitly computes photosynthetically active radiation, canopy temperature, and related energy, water, and carbon fluxes so that it facilities a dynamic vegetation model. A modified two-stream radiation transfer scheme was used to compute fractions of sunlit and shaded leaves and their absorbed solar radiation. The Ball–Berry type of rs scheme (e.g., Ball et al., 1987) was applied as required by the dynamic vegetation option. When this option is used, the green vegetation fraction (GVF) does not come from an input dataset as in Huang et al. (2021) but is related to the LAI based on Eq. (1):
Niyogi and Raman (1997) concluded that Ball–Berry, along with two other physiological schemes, performed better on rs than the multiplicative Jarvis type, which has been frequently used with the prescribed vegetation option. Specifically, it helps better capture the variance in rs and is more responsive to environmental changes. As described in Appendix B of Niu et al. (2011), this scheme relates stomatal resistance rs,i of sunlit and shaded leaves i to the photosynthesis rates (Ai) per unit LAI of sunlit and shaded leaves i separately:
where Cair is CO2 concentration at the leaf surface. For our study period, this was set at 400 ppmv according to the median value of Atmospheric Carbon and Transport (ACT)-America B-200 aircraft near-surface (i.e., >900 hPa) CO2 observations, which is close to the global monthly-mean CO2 concentrations in August 2016 (https://gml.noaa.gov/webdata/ccgg/trends/co2/co2_mm_gl.txt, last access: 8 November 2021); TV, Pair, eair, and esat(TV) are canopy temperature, surface air pressure, vapor pressure at the leaf surface, and saturation vapor pressure inside leaf, respectively; gmin and m are land-cover-dependent empirical parameters. Ai is determined by Eqs. (3)–(6):
where Igs is a TV-dependent growing season index, and AC, AL,i, and AS are carboxylase-limited, light-limited, and export-limited photosynthesis rates per unit LAI, respectively; ci and oi are CO2 concentrations inside leaf cavity, which is about 0.7 times of the atmospheric CO2 concentration and atmospheric O2 concentration, respectively. PAR represents the photosynthetically active radiation per unit LAI. ccp is the CO2 compensation point, and it is equal to , where Kc and Ko are the Michaelis–Menten constants for CO2 and O2, respectively, varying with TV; and α is the quantum efficiency.
Vmax represents the maximum rate of carboxylation, expressed as
where Vmax25 is the maximum carboxylation rate at 25 ∘C; f(TV) is a function that mimics thermal breakdown of metabolic processes; f(N) is a foliage nitrogen factor; and β is the SM factor controlling rs, which presents strong dependencies on soil type and hydrological regime. In this study model results based on the Noah and the Community Land Model (CLM; versions 4.5 and earlier) types of β schemes are compared (Table 1), the latter of which is known to often result in sharper and narrower ranges of variation with SM than the former does. The Noah and CLM types of β parameterizations are based on Eqs. (8) and (9), respectively:
where
θliq,i, θwilt, θref, and θsat are SM at soil layer i, wilting point, and reference and saturated SM, respectively. Nroot and zroot are the numbers of soil layers containing roots and total depth of the root zone, respectively. ψi, ψwilt, and ψsat are matric potential at soil layer i, and wilting and saturated matric potential, respectively, and b is the Clapp–Hornberger parameter. Major parameters for the calculations of β in both schemes are soil-type-dependent.
Other Noah-MP configurations which can affect the modeled land state and flux variables include the three-layer snowpack physics and the CLASS snow surface albedo; the Jordan scheme for partitioning precipitation into rainfall and snowfall; the Niu-Yang-2006 frozen soil permeability and supercooled liquid water option; the SIMple Groundwater Model runoff scheme; and the Monin–Obukhov CH scheme, which is based on more general Monin–Obukhov similarity theory and, unlike Noah's default Chen97 (Chen et al., 1997) scheme (Niu et al., 2011; and Sect. S1 of Huang et al., 2021), accounts for the zero-displacement height. Being affected by stability correction and additional effects of planetary boundary layer height on friction velocity, it is likely that the Monin–Obukhov scheme can result in either weaker or greater CH (i.e., less or more efficient ventilation of the land surface) than the Chen97 scheme during the daytime in summer (Niu et al., 2011; Yang et al., 2011).
The irrigation process was included in all Noah-MP-based simulations in this study. The benefit of including irrigation relies on the choice and parameterization of the irrigation scheme, as well as the LSM's inputs (Lawston et al., 2015). The sprinkler scheme was chosen as it was reported as the prevalent irrigation method in 2015 across the US and many of the states within our model domain (Dieter et al., 2018). Irrigation was triggered over irrigated land in growing season within local morning times (06:00–10:00) when root zone SM drops below 50 % of the soil field capacity. The irrigated land was determined by the model's LULC input and irrigation intensity information in Salmon et al. (2015), and the root zone area was derived from the maximum root depth, which varies by crop type and GVF.
2.3 Wesely and dynamic O3 dry-deposition schemes
Dry-deposition velocity vd is estimated based on the resistance analogy approach:
ra and rb are aerodynamic resistance and quasi-laminar sublayer resistance, respectively, sensitive to surface properties such as surface roughness, and follow the Monin–Obukhov similarity theory. Over the land, surface resistance rc, the major component of vd, is classified into stomatal–mesophyll resistance (rs–rm), cuticular resistance (rlu), in-canopy resistance (rdc and rcl), and ground resistance (rac and rgs):
where rdc is resistance for gas-phase transfer affected by buoyant convection in the canopy when sunlight heats the (near-)surface, rcl is resistance for leaves, twigs, bark, and others in the lower canopy, rac is resistance for transfer that depends mostly on canopy structure, and rgs is resistance for soil, leaf litter, snow, and others at the ground surface.
Two deposition schemes, namely the Wesely and a dynamic scheme, were applied in this study, in which rs and rlu are treated differently. In the Wesely scheme, rs and rlu are calculated based on Eqs. (12) and (13):
where the LULC- and season-dependent constants ri and rlu,min represent the minimum stomatal and cuticular resistances, respectively, which are subject to uncertainty; G and Ts are radiation and surface temperature, respectively, whose definitions are different than those of PAR and TV in Eqs. (2)–(7); and Dx are molecular diffusivities for water vapor and trace gas x (e.g., O3), respectively; H, which is sensitive to surface temperature, represents the Henry's law constant for the focused trace gas; and f0 is a reactivity factor for oxidation. The Wesely-scheme-related results that are new from this study and those from Huang et al. (2021) are compared (Table 1).
As expressed in Eq. (14), in the dynamic scheme, rs used in dry-deposition modeling was taken from what's calculated from Noah-MP's dynamic vegetation model and thus considers the physiological process of leaf responses to photosynthesis rate, humidity, and CO2 concentrations. The direct effects of SM, as reflected in the β formula, as well as other environmental variables, are included in this method, and this work quantifies the impact of the β factor configurations in Noah-MP (Table 1) on the dynamic-scheme-related results.
where rs, sunlit and rs, shaded are computed based on Eqs. (2)–(7), and Lsunlit and Lshaded are proportional to the sunlit and shaded fractions of canopy, respectively, calculated based on the modified two-stream radiation transfer scheme.
In the dynamic scheme, rlu for dry surfaces is modified from the Wesely formula by considering its LAI dependency:
In both the Wesely and the dynamic schemes, rdc is sensitive to surface radiation, and rm is expressed as
Similar to the rlu calculations in Eqs. (13) and (15), to approximate an effect that coldness sometimes reduces the uptake, is added to LULC- and season-dependent constants to derive rgs and rcl. It is worth mentioning that the direct effects of water stress on mesophyll resistance have been recognized (e.g., Egea et al., 2011). Yet, in neither scheme we applied have such effects been incorporated into the rm formula as part of the vd calculation.
2.4 Model evaluation, analysis, and O3 impact assessments
For the cases listed in Table 1, we quantify the impacts of SM DA on the modeled SM, vegetation dynamics, surface fluxes, and meteorological and surface O3 fields during the 16–28 August 2016 period. The focused surface fluxes are gross primary productivity (GPP), which is integrated by LAI from A in Eqs. (2)–(3), energy fluxes and their partitioning in the format of evaporative fraction (EF = daily latent heat (daily latent heat + daily sensible heat)), dry-deposition flux and individual vd terms for O3, particularly the rs- and rlu-related terms. The SM DA impacts on most of these model fields are expressed as daily and/or daytime (around 13:00–24:00 UTC) averaged absolute or relative changes referring to the results from the no-DA cases. For O3 dry-deposition fluxes, we also conducted linear regression analyses to determine the relationships between the relative flux changes and the relative changes in column-averaged initial SM due to the DA. Results of O3 dry-deposition fluxes and the regression analyses (i.e., slopes and their standard errors, correlation coefficient r values, and p values) are summarized by grouped LULC types defined in Fig. 1a. Case studies were also conducted at two low-elevation forested sites where we investigated in detail the diurnal and daily variability of O3 dry-deposition fluxes from various model cases and an independent dataset.
Table 2Evaluation datasets relevant to this study, along with their key attributes. References of these products can be found in the “Data availability” section of this work and Huang et al. (2021).
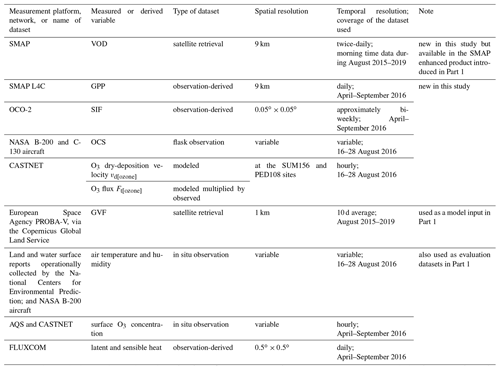
Acronyms: AQS – Air Quality System, CASTNET – Clean Air Status and Trends Network, GPP – gross primary productivity, GVF – green vegetation fraction, L4C – level 4 carbon, OCO-2 – Orbiting Carbon Observatory-2, OCS – carbonyl sulfide, PROBA-V – Project for On-Board Autonomy – Vegetation, SIF – solar-induced chlorophyll fluorescence, SMAP – Soil Moisture Active Passive, and VOD – vegetation optical depth.
A variety of data products were utilized in this study to assess the model performance in no-DA and DA cases (Table 2). Many of these evaluation datasets have been applied and introduced in detail in Huang et al. (2021), which are (1) National Centers for Environmental Prediction Global Surface Observational Weather Data as well as weather data collected on board the NASA B-200 aircraft during the ACT-America campaign; (2) hourly surface O3 measurements at the US Environmental Protection Agency Clean Air Status and Trends Network (CASTNET) and Air Quality System (AQS) sites; and (3) daily, 0.5∘ × 0.5∘ FLUXCOM latent and sensible heat fluxes. New evaluation datasets used in this work include (1) VOD retrievals from the 9 km enhanced SMAP product, which indicates the attenuation of microwave signals by vegetation, proportional to above-ground canopy biomass, and was used together with a 10 d average Copernicus Global Land Service GVF product to derive GVF for the focused 13 d period; (2) daily GPP estimates from the 9 km SMAP level 4 carbon (L4C) product version 6, developed based on the SMAP L4 surface (0–5 cm) and root zone (0–100 cm) SM together with satellite LULC and vegetation datasets; (3) two independent GPP proxies (Whelan et al., 2020) of satellite-derived solar-induced chlorophyll fluorescence (SIF) data (Yu et al., 2019) and the Portable Flask Package (Sweeney et al., 2015) carbonyl sulfide (OCS) measurements collected on board the B-200 and C-130 aircraft during the ACT-America campaign, with the OCS data being analyzed together with other airborne trace gas (e.g., benzene) measurements during this campaign to help distinguish the influences of combustion sources from plant CO2 uptake on the observed OCS distributions; and (4) vd data from two selected CASTNET sites, estimated using a multilayer model (MLM; not supported by CASTNET as of 2017) version 3.0, which has known limitations and biases against eddy covariance flux measurements as well as vd estimated using other methods (e.g., Finkelstein et al., 2000; Saylor et al., 2014; Wu et al., 2018). The known limitations of MLM and how they may affect our model comparisons with the CASTNET vd data are discussed. Our O3 dry-deposition results are also compared with eddy covariance measurements reported in independent works for similar climate and/or LULC types during other time periods.
This study also evaluates how the SM DA affected the assessments of surface O3 impacts on human and ecosystem health. Specifically, (1) MDA8 O3 fields over urban and nonurban terrestrial regions were investigated linked to their respective population ranges, and (2) the LULC-specific phytotoxic ozone dose above the critical level of y nmol m−2 s−1 (PODy) and the crop-specific AOT40, which are defined in Eqs. (17) and (18), were evaluated.
for hourly daytime stomatal uptake Fs>y nmol m−2 s−1, and
for hourly daytime O3 concentration C>0.04 ppmv.
According to Convention on Long-Range Transboundary Air Pollution (CLRTAP, 2017), the stomatal O3 uptake Fs needed in PODy calculations was derived based on Eq. (19):
where gs, L, and u are stomatal conductance, leaf width (0.04 m in this work), and surface wind speed, respectively.
The calculated PODy and AOT40 were used to estimate the relative biomass loss (RBL) or relative yield loss (RYL) for several types of vegetation or crops based on dose–response functions reported in literature (Table 3, CLRTAP, 2017; Mills et al., 2007, 2018b). Our 13 d WRF-Chem model results were linearly extrapolated to approximately 3 months to derive the PODy and AOT40 fields. While we assess the uncertainty due to such linear extrapolations by relating our 13 d/extrapolated surface O3 and flux results to seasonal (e.g., averaged for 3 consecutive months) conditions in 2016, we focus on qualitatively interpreting the results and discussing their implications. The outcome from this analysis is also compared with the findings from several independent O3 impact assessment studies for different time periods.
Table 3Dose–response functions used to estimate the LULC- and crop-specific relative yield losses (i.e., 1 – relative yield, RY) due to O3 exposure and uptake, along with their references.
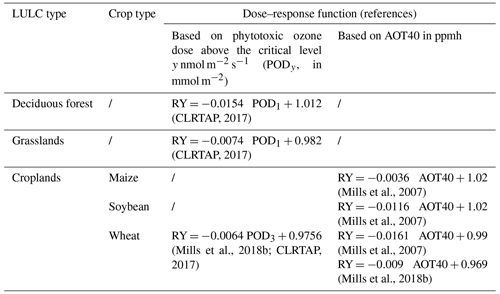
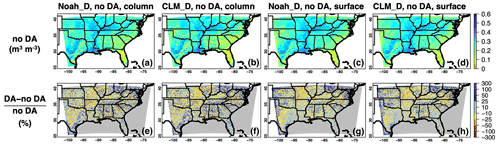
Figure 2Period-mean (16–28 August 2016) WRF-Chem (a, b) column-averaged and (c, d) surface-layer soil moisture fields at initial times and (e–h) their relative changes in percent due to the SMAP DA. Results based on the Noah_D and CLM_D cases are shown in (a), (c), (e), and (g) and (b), (d), (f), and (h), respectively.
3.1 Modeled SM and vegetation fields
Figure 2 compares the horizontal and vertical gradients of the model's initial SM conditions from the Noah_D and CLM_D cases defined in Table 1, in which the Noah and CLM types of β factor schemes were applied. At the surface layer (0–10 cm belowground), both cases produced SM horizontal gradients that resemble the Noah-based results presented in Huang et al. (2021). They are moderately correlated with the column-averaged SM fields (r=0.875 and 0.871, respectively), and the mean differences in column-averaged and surface SM from the Noah_D and CLM_D cases are 0.003 and −0.006 m3 m−3, respectively. Kumar et al. (2009) have found that, compared to other LSMs such as the Catchment model (based on which the SMAP L4 datasets are produced), the 4-soil-layer Noah and 10-soil-layer CLM LSMs display successively weaker surface–subsurface coupling strengths, and the weakest coupling strength of CLM was primarily attributed to its significantly larger number of soil layers. The slightly weaker surface–subsurface correlations in the CLM_D case than in the Noah_D from this work, both based on a 4-soil-layer Noah-MP modeling system, indicate the minor role of the LSM physics, in particular the β factor scheme, in controlling the vertical coupling strength of SM conditions.
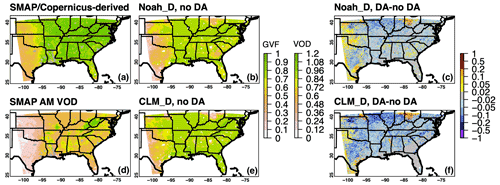
Figure 3Period-mean (16–28 August 2016) green vegetation fraction (GVF) (a) derived from the Copernicus Global Land Service product and the SMAP morning-time (AM) vegetation optical depth (VOD) using the method described in Fig. S2 and (b, c, e, f) based on WRF-Chem calculations as well as their responses to the SMAP DA. The GVF results from the Noah_D and CLM_D cases are shown in (b) and (c) and (e) and (f), respectively. Period-mean SMAP AM VOD is shown in (d). In (a) and (d), grey indicates missing data over terrestrial regions.
The modeled SM fields from the Noah_D and CLM_D differ on grid scale, particularly in the subsurface zones (Fig. 2a and b). For example, in sand-dominant regions that were experiencing drought conditions during this period (e.g., Florida and the Texas–Oklahoma border regions, where simulated SM is mostly under 0.2 m3 m−3), column-averaged SM values from the CLM_D case are notably smaller than those from the Noah_D case. These results contrast with those reported by Niu et al. (2011), in which cases using Noah-MP with the CLM-type β factor led to less soil water consumption and thus smaller SM variability during drought periods than using it with the Noah-type β factor. In their cases focusing on loam and clay soil that have higher wilting points when the CLM-type β factor scheme was applied, plant transpiration ceased to save soil water under drought conditions. Our results can be explained by the steeper CLM-type β–SM curve than the Noah-type β–SM curve for low SM, sand-dominant areas, as illustrated in Fig. 3a of Niu et al. (2011). For such conditions, Noah-MP with the CLM-type β factor produces stronger evapotranspiration (ET) and consumes more soil water, resulting in drier soil. For wet regions where SM values exceed 0.4 m3 m−3, such as Louisiana and Arkansas, the CLM- and Noah-type β values are close to 1.0 and insensitive to soil type and SM variations; therefore, SM and ET produced from the Noah_D and CLM_D cases do not diverge. These findings corroborate the conclusions by Yang et al. (2011) that the degree of the β impacts on the SM–ET relationship should depend on the soil type and hydrological regime, and they are important for understanding the vegetation and surface flux results to be presented in the later parts of this paper.
Referring to the SMAP SM data, in general, surface SM produced by the no-DA modeling systems shows wet biases in non-forested regions and dry biases over the forests for the study period. These SMAP–model discrepancies were successfully reduced by the DA for all vegetated LULC groups (Fig. S1, left), leading to overall slightly drier soil in DA-enabled simulations. For both the Noah_D and CLM_D cases, the DA adjusted the modeled SM fields across the entire soil columns, demonstrating that observational information at the surface was propagated into deep soil layers. The SM responses to the DA as a function of soil layer from the Noah_D and CLM_D cases are roughly similar but different at small spatial scales, which reflect the controls of the β factor scheme on the surface–subsurface coupling strengths of the modeling/DA system used. With the SMAP DA enabled, the r values between column-averaged and surface SM from the Noah_D and CLM_D cases increased to 0.902 and 0.897, respectively.
The satellite-derived GVF fields (methods introduced in Fig. S2 caption) transition from low to moderate (<0.6) to high (>0.8) values from the western (mostly shrub/grasslands) to the central and eastern parts (forests- and croplands-dominant) of the study region, and such spatial gradients are highly correlated with the SMAP VOD retrievals (Fig. 3a and d). The Noah_D and CLM_D cases both reproduced these spatial patterns moderately well. Major differences between these cases are found in dry sandy regions, where, as discussed in previous paragraphs, more soil water was consumed for ET and plant growth in the CLM_D case and therefore higher GVF values are given. Overall, the DA adjustments to the modeled GVF and SM fields are positively correlated (Fig. S1, right), and the relative changes in GVF are smaller. While the SM changes in the Noah_D and CLM_D cases are of close magnitude, GVF responded more strongly in the CLM_D case except for sandy regions. Referring to the satellite-derived GVF fields which are also subject to large uncertainty (as discussed in Fig. S2 caption), the modeled vegetation fields are more effectively improved by the DA over sparsely vegetated regions such as the South Central Plains. The DA also remarkably reduced the model–satellite mismatches over some of the dense vegetation regions such as southwestern Ohio. The likely degraded model performance over certain dense vegetation areas can be partially explained by weaknesses related to the SM–vegetation growth feedbacks (more details in Fig. S1 caption) in the dynamic vegetation model parameterizations which need to be identified and addressed in future work. It is also suggested that joint assimilation of satellite SM and vegetation phenology products such as the VOD retrievals needs to be attempted, which may maximize the positive DA impacts on multiple land variables and their atmospheric feedbacks.
Table 4Evaluation of daily-averaged WRF-Chem gross primary productivity and evaporative fraction, referring to the SMAP L4C and FLUXCOM datasets.
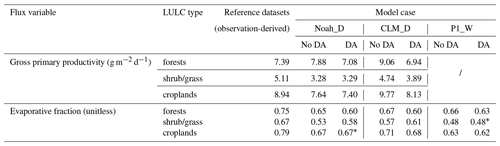
∗ The increases from no-DA cases, which led to improved model performance, are <0.005.
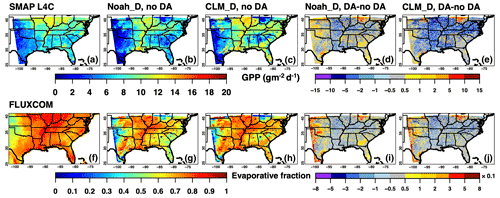
Figure 4Period-mean (16–28 August 2016) WRF-Chem calculated (b–e) gross primary productivity (GPP) and (g–j) evaporative fraction as well as their responses to the SMAP DA. Results based on the Noah_D and CLM_D cases are shown in (b), (d), (g), and (i) and (c), (e), (h), and (j), respectively. Period-mean SMAP L4C GPP and FLUXCOM evaporative fractions are shown in (a) and (f), respectively, which are also used to evaluate the model results (Table 4).
3.2 Modeled fluxes and weather conditions
3.2.1 Carbon/energy fluxes and weather conditions
Figure 4 compares the spatial distributions of the period-mean WRF-Chem carbon and energy fluxes with SMAP L4C and FLUXCOM products which contain observation information, and Table 4 summarizes WRF-Chem and observation-derived flux results by three LULC groups. The observation-derived products indicate the highest GPP and EF over croplands. Without the DA, the Noah-MP-related cases outperformed the Noah-related P1_W case on simulating EF, especially over shrub/grassland and cropland regions. This indicates that, from Noah to Noah-MP, the multiple updates in LSM physics related to rs, irrigation, and CH are beneficial. Larger GPP and EF values are found in CLM_D than in Noah_D; most of these larger values match better with the SMAP L4C and FLUXCOM data. The DA led to increased EF over shrub/grasslands in all model cases as well as over croplands in the Noah_D case, bringing the model results closer to the FLUXCOM data. The EF values were unfavorably reduced by the DA in the CLM_D and P1_W cases over croplands and in all model cases over forests, reflecting the challenges of satellite SM DA over regions with dense vegetation and/or affected by human activities, which have also been reported and discussed in previous studies (e.g., Huang et al., 2021). For the Noah_D and CLM_D cases, this may also be due to the possibly degraded vegetation performance discussed in Sect. 3.1. The modeled GPP in the CLM_D cases was lowered by the DA overall, which helped reduce the model–SMAP L4C discrepancies over forests and croplands. In the Noah_D case, GPP was improved by the DA over forests and (slightly) over shrub/grasslands. Based on the evaluation statistics, for this case, the CLM-type β factor scheme is shown to be slightly superior to the Noah type. Note that the quality of the SMAP L4C and FLUXCOM products may also be strongly LULC-dependent; e.g., it has been known that the uncertainty of SMAP L4C data is generally larger for highly productive plant functional types (Kimball et al., 2021). Such evaluation, therefore, has demonstrated the critical role of LULC type in understanding the model performance of carbon and energy fluxes and its responses to satellite SM DA.
Additional datasets were also utilized to help understand terrestrial carbon uptake, including satellite SIF and ACT-America aircraft OCS, as well as its vertical gradients (Fig. S3). Consistent with the SMAP L4C- and WRF-Chem-based results, the largest SIF values are shown over croplands, especially maize and soybean fields in Illinois and Indiana, 2–3 times as high as those over shrub/grasslands in the South Central Plains. All these datasets suggest moderate to high terrestrial carbon uptake around the Lower Mississippi croplands and the forests and croplands near the Texas–Oklahoma border, which is supported by the large OCS drawdowns (i.e., the free tropospheric-near surface gradients far exceeded 60 pptv) along with other trace gas measurements taken on board the B-200 and C-130 aircraft.
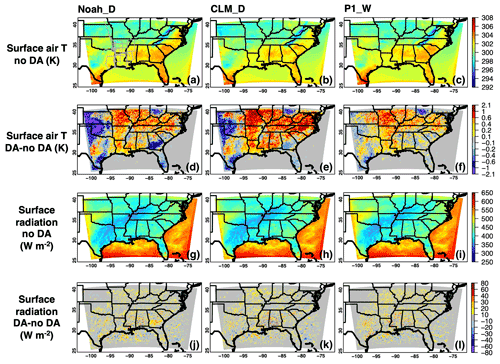
Figure 5Period-mean (16–28 August 2016) WRF-Chem calculated daytime (a–c) surface air temperature and (g–i) surface radiation as well as (d–f, j–l) their responses to the SMAP DA. Results based on the Noah_D, CLM_D, and P1_W cases are shown in (a), (d), (g), and (j), (b), (e), (h), and (k), and (c), (f), (i), and (l), respectively. Overall, the weather fields from Noah_D and Noah_W (not shown in figures) cases are nearly the same. Grey lines in (a) indicate the B-200 flight paths over the southeastern US during the 2016 ACT-America campaign.
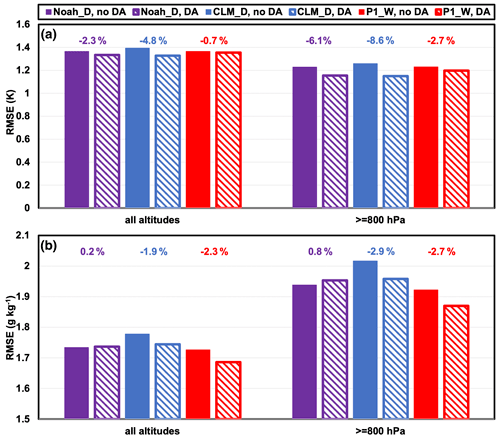
Figure 6Evaluation of (a) air temperature and (b) water vapor mixing ratios from several WRF-Chem simulations with the B-200 aircraft observations during the 2016 ACT-America campaign. The RMSEs are summarized in barplots based on model comparisons against observations at all altitudes and near the surface (i.e., ≥800 hPa). Colored texts above the barplots indicate the SMAP DA impacts on RMSEs. The B-200 flight paths are indicated in Fig. 5a.
In general, the modeled EF fields as well as their directions of changes due to the DA resemble those of latent heat flux and relative humidity (RH), which are opposite to those of sensible heat and surface temperatures (Figs. 5 and S4). The model reproduced the observed spatiotemporal variability of 2 m air temperature (T2) and RH, as well as FLUXCOM latent and sensible heat fluxes, well overall. The diagnostic 2 m weather fields and their responses to the DA strongly correlate with the model's surface-level results. The Noah-MP-related cases reacted more strongly to the DA than the Noah-related cases, with the responses in the CLM_D case larger than in the Noah_D case except for dry, sandy regions, which can be attributed to combined effects of the CH and stomatal resistance schemes used. It is important to note that diagnostic temperature and humidity variables are represented differently in Noah and Noah-MP and thus are not directly comparable. Specifically, in Noah, T2 is an explicit function of surface temperature, air density, specific heat of dry air at constant pressure, and 2 m surface exchange coefficient for heat, and 2 m specific humidity is a function of surface specific humidity, upward moisture flux at the surface, air density, and 2 m surface exchange coefficient for moisture, whereas in Noah-MP, they are expressed as functions of temperatures and water vapor for vegetated land and bare soil being weighted by their respective fractions. We therefore focus on quantitatively evaluating and intercomparing prognostic model weather variables (i.e., the model-level air temperature and humidity) against ACT-America aircraft observations (Fig. 6). For air temperature, at all altitudes and near the surface (i.e., ≥800 hPa), the CLM_D case responded most strongly to the DA, and the DA-enabled CLM_D case outperformed the Noah_D and P1_W cases. This performance is qualitatively consistent with the model's sensible heat performance referring to the FLUXCOM data. As for humidity, despite the most significant DA improvements in CLM_D, the Noah-MP-related cases did not perform as well as the Noah-related cases, which is also found in the model's latent heat performance in comparison with the FLUXCOM data. However, note that the model's humidity performance is more strongly related to that of rs and vd in the Noah-MP-based cases via the direct impacts of humidity on rs calculations (Eq. 2). The solar radiation fields from all model cases, which play vital roles in controlling the land–atmosphere exchanges of water and trace gases, do not differ remarkably, and their responses to the DA are negligible (e.g., Fig. 5g–l). This indicates that the DA impacts on the modeled surface fluxes resulted primarily from the changes in the modeled SM, humidity, and canopy and/or surface temperatures, as well as vegetation fields. In many cases, these primary contributing factors to the DA impacts are interdependent, and their relative contributions vary by location and time.
Table 5The 24 h and daytime mean O3 deposition velocity (vd[ozone]) and flux (Ft[ozone]) for three LULC groups, from various model cases.
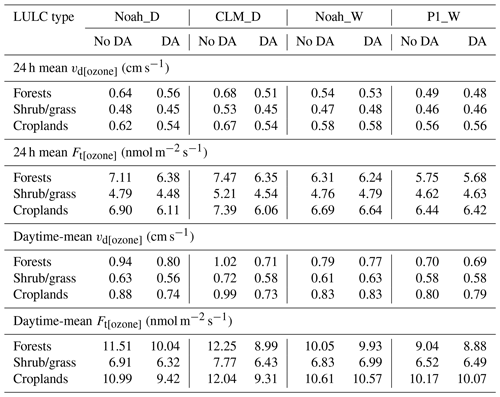
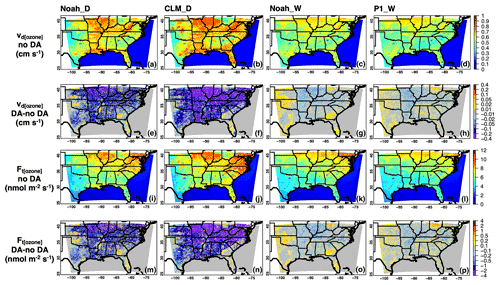
Figure 7Period-mean (16–28 August 2016) WRF-Chem (a–d) O3 dry-deposition velocity and (i–l) O3 dry-deposition flux, as well as (e–h, m–p) the impacts of SMAP DA on these model fields. Results are shown for (a, e, i, m) Noah_D, (b, f, j, n) CLM_D, (c, g, k, o) Noah_W, and (d, h, l, p) P1_W cases, averaged throughout the day.
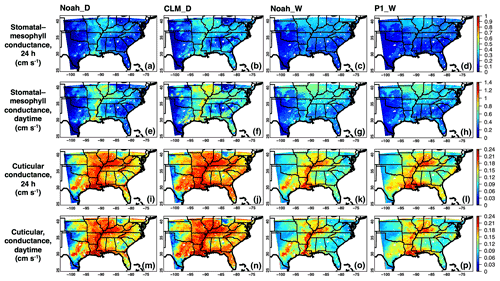
Figure 8Period-mean (16–28 August 2016) WRF-Chem (a–h) stomatal–mesophyll and (i–p) cuticular conductances over terrestrial regions that do not belong to the urban category in Fig. 1a. Results are shown for (a, e, i, m) Noah_D, (b, f, j, n) CLM_D, (c, g, k, o) Noah_W, and (d, h, l, p) P1_W no-DA cases, averaged (a–d, i–l) throughout the day and (e–h, m–p) during the daytime.
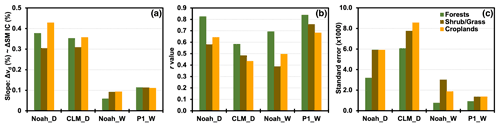
Figure 9(a) Regression slopes of the relative changes of O3 dry-deposition velocity vd versus the relative changes of column-averaged soil moisture initial conditions (SM ICs) due to the SMAP DA, summarized by three LULC groups for all model cases listed in Table 1. The r values of these regression analyses and the standard errors of slopes (%, scaled by 1000) are indicated in (b) and (c), respectively. The p values for all regression analyses are ≪0.01. Regression results for the relative changes of O3 deposition flux versus the relative changes of SM ICs are similar (not shown in figures).
3.2.2 Ozone dry-deposition velocities and fluxes
Figure 7 presents the period-mean, daily-averaged vd and dry-deposition flux Ft (i.e., vd multiplied by concentration at the surface level, Wesely, 1989) for O3 from all model cases, along with their responses to the SMAP DA. The daytime averages of these fields have similar spatial gradients but of larger magnitudes (not shown in figures). Table 5 summarizes for three LULC groups the daily- and daytime-averaged results. The modeled stomatal–mesophyll and cuticular conductances, as well as their diurnal variability, are indicated in Fig. 8. All model cases produced lower vd and Ft values over shrub/grasslands than over forests and croplands, qualitatively consistent with results from many existing model- and measurement-based studies (e.g., Val Martin et al., 2014; Hardacre et al., 2015; Silva and Heald, 2018; Lin et al., 2019). The results from Noah_W and P1_W, both of which are based on the same scheme (Wesely), are generally similar, with minor differences largely attributed to different surface temperature fields (Figs. 5 and S4). The WRF-Chem-modeled vd and Ft fluxes were more strongly affected by the upgrade from the Wesely to the dynamic scheme; i.e., with the updated scheme, they show enhanced magnitudes, stronger spatial variability, and more intensive responses to the DA, especially over forests and croplands. These results can be mainly explained by the fact that the stomatal–mesophyll and cuticular resistances in the dynamic scheme are sensitive to more environmental and biophysical variables, accounting for both the direct and indirect (i.e., via influencing the weather fields and plants' physiology) effects of SM on vd. vd from the Noah_D and CLM_D cases, as well as its major term stomatal–mesophyll conductance, shows strong correlations with the modeled GPP, latent heat, and EF fields, which have been discussed in earlier sections. Comparing the cases that implemented the CLM- and Noah-type β schemes, O3-related fluxes resulting from the former configuration are of notably larger magnitude, spatial variability, and absolute changes due to the DA. The SM impacts on the modeled vd and Ft were further quantified using linear regression analyses between the relative changes in the modeled O3 fluxes due to the DA versus those in column-averaged SM initial conditions. All regression models yielded low p values (i.e., ≪0.01), suggesting good Δvd–ΔSM and ΔFt–ΔSM relationships. The regression slopes, all with standard errors of <0.01 %, are summarized in barplots (Fig. 9) by three LULC groups for all model cases in Table 1. For all LULC groups, the slopes based on the two cases that implemented the dynamic scheme are 2–3 times larger than those from the two cases using the Wesely scheme, and the slopes differ most strongly among the cases over forests and croplands. The low r values (<0.5) associated with several regression models reflect the stronger nonlinear relationships between the changes in the studied O3 fluxes and SM. These results emphasize the importance of better understanding and representing in models the SM control on plants' stomatal behaviors which regulate the land–atmosphere exchanges of water, energy, and trace gases. The earlier evaluation of the period-mean GPP and EF across the domain has demonstrated some advantages of using the CLM-type β scheme and that the DA more effectively improved the model performance in sparsely vegetated shrub/grassland regions. These conclusions are likely also applicable to the modeled O3 dry-deposition process, particularly its stomatal–mesophyll pathway.
In all no-DA and DA cases, the diurnal variability of O3-related surface fluxes shows clear LULC dependency. Over the shrub/grassland and forests/croplands regions, the daytime-averaged vd values are 24 %–31 % and 35 %–50 % higher than the 24 h mean, respectively, while the daytime-averaged Ft results are 40 %–50 % and 42 %–63 % higher than the 24 h mean, respectively (Table 5). Such vd diurnal cycles are a result of the strongest diurnal variability in stomatal–mesophyll conductance (i.e., its daytime mean values are approximately twice as high as the 24 h mean for all LULC types) being balanced out by weak diurnal variability associated with other vd terms. As the most diurnally variable vd component, stomatal–mesophyll conductance, on average, contributes less substantially to vd for shrub/grassland areas (24 h/daytime: up to ∼30 %/40 %) than for forests/croplands (24 h/daytime: up to ∼50 %/66 %), which helps explain the weaker diurnal variability in the modeled vd over shrub/grasslands. The stronger diurnal cycles in Ft than in vd reflect the impacts of higher daytime O3 surface concentrations used in the Ft calculations. The DA did not dominantly intensify or dampen the diurnal cycles of these fluxes for any given grouped LULC type. Whether the DA improved the estimated diurnal cycles of fluxes for various LULC types remains to be evaluated, which can benefit from independent observation-constrained flux products of broad spatial coverage and subdaily variability.
Table 6Period-mean (16–28 August 2016) soil moisture and surface fluxes at two CASTNET sites shown in Fig. 1d. Standard deviations calculated based on the hourly O3 dry-deposition velocity vd[ozone] and flux Ft[ozone] results are also included. Daytime is defined as approximately 08:00–19:00 local standard time.
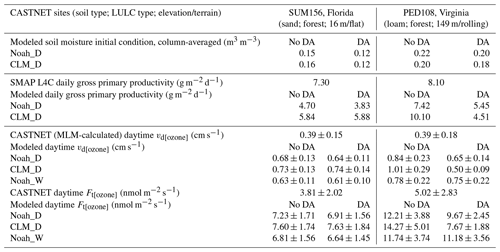
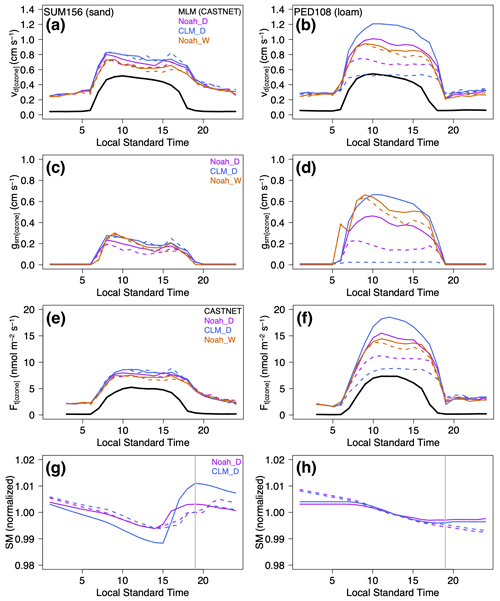
Figure 10Period-mean (16–28 August 2016) diurnal cycles of (a, b) O3 dry-deposition velocity vd and (e, f) O3 dry-deposition flux Ft based on the CASTNET dataset (shown by solid black lines) and their WRF-Chem counterparts (purple, blue, and brown lines) at the (a, c, e, g) SUM156 and (b, d, f, h) PED108 sites, whose locations are shown in Fig. 1d. Panels (c) and (d) and (g) and (h) indicate the diurnal variability of WRF-Chem stomatal–mesophyll conductance gsm and column-averaged soil moisture (normalized) at these two sites, respectively. The grey vertical lines in (g) and (h) denote the initial times of WRF-Chem. WRF-Chem results from the no-DA and DA cases are indicated by solid and dashed lines, respectively. Additional time series plots indicating the daily variability of these fluxes are shown in Fig. S5.
A detailed analysis was then conducted at two forest CASTNET sites with different soil types and hydrological regimes. The modeled vd and Ft from various cases are compared with the operational MLM-based calculations produced at a Florida site SUM156 and a Virginia site PED108 (Figs. 10a, b, e, f and S5; Table 6), where many, most, or all MLM assumptions apply. The dominant soil types at these sites are sand and loam, and the column-averaged SM values from various model cases are approximately 0.15 and 0.20 m3 m−3, respectively. These various datasets show that stomatal–mesophyll conductance, vd, and Ft sharply increase soon after sunrise, reaching their daily maxima in the late morning or early afternoon. The slight declines in fluxes around midday based on some simulations can result from the water and heat stresses which cause stomata closures (Fig. 10c and d). The water stress starts to get relieved from the mid-afternoon at the SUM156 site under the influences of convective precipitation, whereas it persists throughout the afternoon at the PED108 site (Fig. 10g and h). This helps shape the slightly different afternoon flux dynamics at these two locations. Without the DA, at both sites, the highest daytime fluxes were produced from the CLM_D case, followed by the Noah_D and Noah_W cases, which are 2–3 times as high as the MLM-estimated cases. The fluxes from all WRF-Chem cases during the nighttime are close, up to >80 % lower than their daytime maxima, contributed mostly by ra, rb, and non-stomatal rc pathways as stomatal–mesophyll conductance is shown to be negligible (Fig. 10c and d). Despite the uncertainty possibly introduced by the limitations of the Monin–Obukhov similarity theory, our nighttime vd results are close to flux observations at European forest sites during both dry and wet periods in the past decades (Lin et al., 2020). They are, however, dramatically higher than the MLM-based results that are nearly zero. Wu et al. (2018) compared vd observations with single-point model calculations based on the operational MLM, Wesely, and Noah-Gas Exchange Model photosynthesis-based scheme, at a Canadian mixed forest site dominated by sand-like soil. Their diverse model results are qualitatively consistent with our findings at the SUM156 and PED108 sites. The remarkably lower vd values from the operational MLM calculations can be partially attributed to the MLM's simplified approaches of calculating ra and rb using wind speed and direction, as well as the empirical approach of calculating rs which is subject to errors in the season- and LULC-dependent ri. The possible uncertainty in MLM vd can also be explained by the lack of continuous, accurate model input data. Specifically, the factual data such as plant and canopy attributes used in the MLM calculations are outdated, which, according to the CASTNET database, represent the conditions in the 2000s; and based on the little day-by-day variability found in the MLM vd data during the study period which contrasts with our WRF-Chem results (Fig. S5), it is likely that many but not all of these are filled historical average vd values due to the lack of meteorological measurements that are needed in the MLM calculation. Additionally, based on the surface heterogeneity within the WRF-Chem grids that these sites fall in, representation errors are estimated to be pronounced when comparing the point-scale MLM fluxes with our 12 km WRF-Chem results.
Within the respective ranges of the modeled SM at these two sites, β factors based on the CLM-type scheme are both larger than those based on the Noah-type β scheme (referring to Niu et al., 2011, Fig. 3), which helps explain the higher and more variable model fluxes from the CLM_D case than the Noah_D case without the DA. At SUM156, despite the strongest SM decrease (∼0.04 m3 m−3) by the DA in case CLM_D, the modeled fluxes responded least strongly to the DA, in part due to the flattened CLM-type SM–β curves in contrast to the linear Noah-type SM–β function for sand within the 0.12–0.16 m3 m−3 SM range. At PED108, the modeled SM values from all model cases were lowered by the DA by ∼0.02 m3 m−3. The stronger reactions of fluxes (i.e., vd, Ft, and their stomatal–mesophyll portions) to the DA from the CLM_D case than those from the Noah_D case can be partially explained by the steep CLM-type SM–β curve versus the linear Noah-type SM–β relationship for loam within the 0.18–0.22 m3 m−3 SM range. Our case studies at these two sites with the same type of LULC emphasize the importance of soil type and hydrological regimes for understanding SM controls on dry deposition, which was often omitted or discussed little in previous dry-deposition studies. It is noted that the effectiveness of SM DA in improving the accuracy of land surface states and fluxes at point scale is dependent on the representativeness of the assimilated satellite SM data for these sites, which is expected to increase with the resolutions of the model and the assimilated satellite land product.
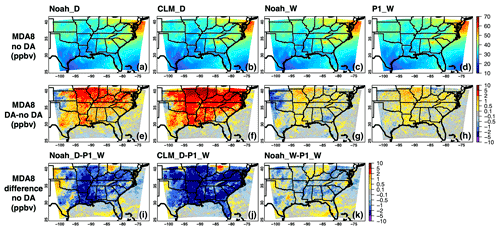
Figure 11Period-mean (16–28 August 2016) WRF-Chem (a–d) surface MDA8 O3 fields and (e–h) their responses to the SMAP DA. Results based on the Noah_D, CLM_D, Noah_W, and P1_W cases are shown in (a) and (e), (b) and (f), (c) and (g), and (d) and (h), respectively, and the differences between the Noah-MP-related cases and the P1_W case are shown in (i)–(k).
3.3 Policy-relevant O3 metrics and implications for O3 impact assessments
3.3.1 MDA8 and implications for O3 health impacts
Figure 11 illustrates the impacts of the choice of dry-deposition scheme and SM DA on WRF-Chem-modeled surface MDA8 O3. During the study period, several warmer- and drier-than-normal Atlantic states experienced high MDA8 at times (i.e., ≥60 ppbv, which can negatively affect lung function and, at ≥70 ppbv, cause respiratory symptoms and other adverse effects; Fleming et al., 2018, and references therein). Numerous populated urban centers reside in these areas. The levels of MDA8 are shown to be much lower (i.e., <40 ppbv) over the southern part of the domain, including several major urban/suburban regions such as the Texas Triangle, which was frequently influenced by passing cold fronts and tropical systems from the Gulf of Mexico.
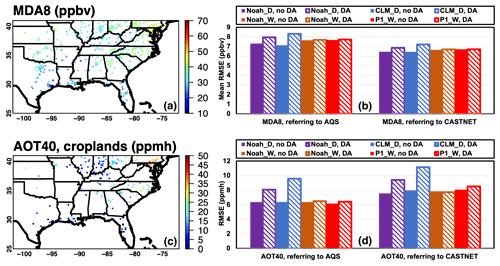
Figure 12(a) Period-mean (16–28 August 2016) observed surface MDA8 O3 and (c) AOT40 in cropland-dominant model grids derived from surface observations during 16–28 August 2016. The RMSEs of modeled MDA8 and model-derived AOT40 from various WRF-Chem cases referring to (a) and (c) are summarized in (b) and (d), respectively.
All model cases reproduced the observed MDA8 spatial patterns (Fig. 12a) moderately well. Referring to observations at AQS and CASTNET sites, their domain-wide mean root-mean-square errors (RMSEs) all fall within 6–8.5 ppbv (Fig. 12b). We first intercompare the MDA8 levels from all no-DA cases. Positive and negative differences between the results from Noah_W and P1_W, both of which implemented the Wesely scheme, are almost equally distributed across the domain, with the MDA8 from the former case associated with negligibly lower RMSEs (i.e., <0.02 ppbv on average) referring to AQS and CASTNET observations (Figs. 11k and 12b). The differences between these two cases are largely due to the impact of the chosen LSM on the model's meteorological fields, particularly temperatures, which affected the simulations of various O3-related processes including dry deposition. As Figs. 11i and j and 12b show, replacing Wesely with the dynamic dry-deposition scheme considerably lowered the calculated MDA8 levels in majority of the model grids, as well as their associated RMSEs (i.e., by >0.5 ppbv on average) relative to surface observations. These reductions in MDA8 are of comparable magnitude with those due to updating anthropogenic emissions from the National Emission Inventory 2014 to 2016 beta (Huang et al., 2021). Comparing the implementations of the CLM- and Noah-type β schemes, the former led to stronger reductions in the modeled MDA8 fields and their associated uncertainty. These results reflect the impacts of the faster O3 removal via dry deposition in the dynamic-scheme-related cases, as well as the different model meteorology. Our findings are qualitatively consistent with the conclusions from several global-scale modeling experiments that compared the Wesely and dynamic schemes (e.g., Val Martin et al., 2014; Lin et al., 2019).
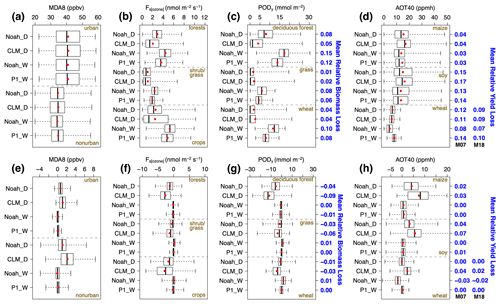
Figure 13Box-and-whisker plots of WRF-Chem (a) MDA8 O3, (b) daytime stomatal O3 uptake Fs[ozone], (c) derived PODy, and (d) derived AOT40, summarized by LULC and crop types from all DA-enabled cases. The impacts of the SMAP DA on these model fields are shown in (e)–(h). Red filled circles indicate the mean values. The mean relative biomass/crop yield losses estimated based on all DA-enabled cases, as well as the SMAP DA impacts on these values, are included in (c), (d), (g), and (h) in blue text. The crop yield losses for wheat, estimated based on the derived AOT40 and two dose–response functions (M07: Mills et al., 2007; M18: Mills et al., 2018b), are included in (d) and (h).
In all model cases, the DA reduced surface and subsurface SM in many of the grids, leading to enhanced MDA8 (Fig. 11e–h). The responses of the period-mean MDA8 to the DA from the Noah_W and P1_W cases are mostly within ±4 ppbv. When the dynamic dry-deposition scheme was applied, the modeled MDA8 responded several times more strongly to the DA (i.e., by up to 6 and 8 ppbv in the Noah_D and CLM_D cases, respectively), especially over nonurban regions, where surface MDA8 is on average several parts per billion by volume (ppbv) lower than in urban grids. In urban grids where population densities are ∼25 times higher than in nonurban grids (Fig. 1c), the DA impacts on MDA8 reach 3–4 ppbv in places, under the controls of the local-to-regional circulation patterns (Fig. 13a and e). As the no-DA cases are positively biased against surface observations in many places, corresponding to the DA-induced surface O3 changes, the overall model performance of MDA8 was not improved, or much degraded, by the DA. Over limited areas such as the South Central Plains, the modeled MDA8 decreased due to the DA by up to >2 ppbv, corresponding to improved performance. The no-DA and DA results based on different LSMs and dry-deposition schemes confirm that drier soil conditions exacerbate O3 air pollution, which, together with heat stress, threatens human health. Such O3–SM relationships have also been demonstrated by Falk and Søvde Haslerud (2019) and Anav et al. (2018) using other chemical transport models and multiplicative dry-deposition schemes. Our Noah_W- and P1_W-related results indicate the influences of SM on air quality via its feedbacks to weather; and results from the Noah_D and CLM_D cases provide valuable information regarding both the indirect (i.e., via adjusting vegetation phenology and weather conditions) and direct SM effects on O3. The complex SM impacts on O3 dry-deposition as well as surface O3 concentrations based on the coupled photosynthesis–rs calculations rely heavily on the application of water stress function (β scheme), soil properties, and hydrological regime. The WRF-Chem results from this case indicate that, to more accurately simulate MDA8, improving land DA must be combined with strong efforts to identify other sources of uncertainty in O3 modeling (e.g., emissions, chemistry, and extra-regional pollution contributions) and reduce their negative impacts on model performance.
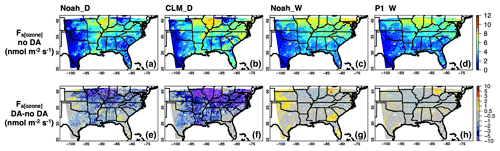
Figure 14Period-mean (16–28 August 2016) WRF-Chem (a–d) daytime stomatal O3 uptake Fs[ozone] fields over terrestrial regions that do not belong to the urban category in Fig. 1a and (e–h) their responses to the SMAP DA. Results based on the (a, e) Noah_D, (b, f) CLM_D, (c, g) Noah_W, and (d, h) P1_W cases are shown.
3.3.2 Implications for O3 vegetation impact assessments using concentration- and flux-based metrics
Both O3 flux- and concentration-based metrics have been applied to assess O3 impacts on vegetation as well as the associated economic loss. Estimating the plants' stomatal O3 uptake Fs is the basis for constructing flux-based O3 impact assessments. Figure 14 illustrates the period-mean daytime Fs fields based on all WRF-Chem no-DA cases as well as their responses to the SM DA. Box-and-whisker plots in Fig. 13b and f summarize these results by three LULC groups. The averaged Fs values for all three LULC groups exceed their respective critical levels (i.e., 1 nmol m−2 s−1 for forest and grasslands and 3 nmol m−2 s−1 for crops). As a major contributor to O3 dry-deposition flux during the daytime, Fs fields appear to be closely correlated in space and time with the surface humidity and flux fields (e.g., GPP, latent heat, and EF, as well as vd), which differ distinctly from the surface O3 concentration fields. For example, Fs hotspots are shown over some low O3 concentration areas including the humid, Lower Mississippi River regions, and the lowest Fs values occur in certain high O3 concentration regions strongly affected by urban pollution (e.g., Georgia) and pollution transport from upwind US states and/or the stratosphere (e.g., western Kansas and Oklahoma, as discussed in Huang et al., 2021). The changes in Fs and surface O3 concentrations due to the DA show opposite directions; i.e., drier soil enhances surface O3 concentrations, whereas it slows down the plants' stomatal O3 uptake (Figs. 11e–h and 14e–h). This comparison highlights how the choice of O3 metrics can affect the assessment of O3 vegetation impacts under the changing climate. As emphasized by Mills et al. (2018b) and Ronan et al. (2020), flux-based metrics have evident advantages over concentration-based metrics. To conduct reliable impact assessments using these flux-based metrics, accurate information on stomatal and non-stomatal fluxes as well as the various environmental and biophysical variables that they are sensitive to becomes increasingly important.
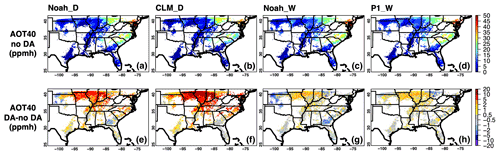
Figure 15WRF-Chem-based AOT40, derived from the modeled surface O3 fields during 16–28 August 2016, as well as their responses to the SMAP DA. Panels (a) and (e), (b) and (f), (c) and (g), and (d) and (h) show results derived from the Noah_D, CLM_D, Noah_W, and P1_W cases, respectively, in cropland-dominant model grids.
An assessment of O3 vegetation impacts was conducted based on the results from various model cases and different metrics, namely PODy (where y is the LULC-dependent critical level) and AOT40. For this demonstration, the 13 d model results were linearly extrapolated to approximately 3 months. This also assumed similar DA adjustments to SM dynamics (driven by factors such as clouds/radiation, rainfall, and irrigation for cropland-dominant regions) at the seasonal timescale. Based on the seasonal variability of surface O3 and surface fluxes in the study region in 2016 (Fig. S6), the linearly scaled PODy and AOT40 values are underestimated overall referring to the 2016 peak AOT40 and surface fluxes occurring during April–May–June and June–July–August, respectively. These overall underestimations may be invalid if the (sub)seasonal variability of surface O3 and surface fluxes of other years was referred to. We therefore focus on discussing the results qualitatively and highlighting their implications for O3 impact assessments using long-term records. Statistics of the derived PODy and AOT40 fields are summarized by O3-sensitive LULC and crop types in Fig. 13c, d, g, and h. Figures 15 and 12c and d present the estimated AOT40 fields and the evaluation of them, as well as their responses to the SM DA for cropland-dominant grids. The highs and lows in AOT40-related results are found over maize- and wheat-dominant fields, respectively. Among the three focused LULC types, the highest and lowest PODy values are estimated for forests and grasslands, respectively. Largely driven by daytime peak O3 concentrations, the spatial variability and biases (referring to AQS and CASTNET observations) of the model-derived AOT40 fields, as well as their responses to the DA, match those of the MDA8-based results (Fig. 11). In contrast, the spatial variability of PODy and Fs aligns well, and so do their responses to the DA. Both PODy and AOT40 reacted several times more intensively in the cases that implemented the dynamic dry-deposition scheme, especially the CLM_D case.
For selected LULC and crop types, the WRF-Chem-derived PODy and AOT40 fields were used together with dose–response functions in literature to evaluate the RBL/RYL due to O3 exposure and uptake. As reported in Fig. 13c and g, with the SM DA enabled, the mean RBLs based on Noah_D- and CLM_D-derived PODy are 0.05–0.08, 0.01–0.02, and 0.04 for deciduous forest, grasslands, and wheat, respectively, which are >33 % lower than the Noah_W- and P1_W-based RBL estimates. It is shown that, in response to the DA which lowered SM in many places, the Noah_W- and P1_W-based RBL estimates did not drop as strongly as the Noah_D- and CLM_D-based ones and even increased by 0.01 for grasslands and wheat. For wheat, one of the most O3-sensitive crops, the estimated RYL values based on the PODy and AOT40 approaches differ by up to a factor of 2–3, and the DA had contrasting effects on these estimates (Fig. 13c, d, g, h). The PODy- and AOT40-based RYL values differ more significantly when the model-derived PODy and AOT40 fields came from the Noah_D and CLM_D cases. Using the model-derived AOT40 and different AOT40 dose–response functions (Mills et al., 2007, 2018b, Table 3), the estimated RYLs and their changes due to the DA are nonnegligible (Fig. 13d and h). Our estimated RBL/RYL results for various LULC and crop types mostly fall within the ranges reported in previous studies which applied model-derived O3 metrics and dose–response functions (e.g., Avnery et al., 2011; Mills et al., 2007, 2018b). Our results emphasize that the selected O3 impact assessment metrics for various LULC/crop types and their matching dose–response functions, as well as the model results used to derive the chosen O3 metrics which are sensitive to dry-deposition schemes and SM, all introduce uncertainty to the estimated O3 impacts on vegetation. The widely used dose–response functions are considered appropriate for studying North America and Europe, but they may not be applicable to other regions (Emberson et al., 2009). Therefore, updating and developing dose–response relationships for a larger number of vegetation types in different regions of the world are needed, which may require new experiments to be conducted. Yue and Unger (2014) and Lombardozzi et al. (2015), as well as follow-on investigations, parameterized the O3 impacts on several types of vegetation using the relationships between cumulative O3 uptake and O3 damage factors for photosynthesis and conductance from empirical and experimental studies. Based on multidecadal model simulations, they reported <20 % changes of biomass, GPP, and energy fluxes due to O3, which are roughly consistent with our RBL/RYL results in Fig. 13. Such approaches that dynamically assess the impacts of O3 along with other factors (e.g., non-O3 pollutants and environmental stresses), as highlighted in Emberson et al. (2018), will be considered in future work.
We note that, revising the dry-deposition scheme and constraining the modeled SM fields with observations would not only better be combined with adding O3 impacts on vegetation but also multi-stress impacts on biogenic emissions. Considering O3-induced vegetation injuries would more evidently affect longer-term climate simulations via feedbacks to biomass, surface fluxes, weather, and weather-driven emissions. As for biogenic emissions, Fig. S7 shows SM anomalies during the study period determined by our Noah-MP modeling system as well as drought stress activity factor γd estimated from β of a multiyear, independent CLM (version 4.5) simulation by Jiang et al. (2018). Based on this analysis, we estimate that, depending on soil type, hydrological regime, and β configurations, omitting the direct impacts of water stress on biogenic emissions may have introduced larger uncertainty (i.e., >30 %) to biogenic emission and O3 modeling over several states experiencing drier-than-normal conditions, particularly South Carolina, Georgia, and Alabama. Quantitatively understanding the interplay between these processes and O3 pollution levels is recommended for more accurate air quality modeling and O3 impact assessments.
This paper described a follow-up study of Huang et al. (2021). It presented how the choice of O3 dry-deposition scheme affected our evaluation of SMAP SM DA impacts on coupled WRF-Chem modeling over the southeastern US in August 2016. In new Noah-MP LSM-related simulations, two dry-deposition schemes were implemented, namely the WRF-Chem default Wesely scheme and a dynamic scheme, in the latter of which the calculation of vd (particularly its stomatal and cuticular terms) was modified to be coupled with photosynthesis and vegetation phenology. We showed that dry-deposition parameterizations significantly affected the modeled O3 dry-deposition process, as well as its response to the DA. Comparing the no-DA cases, it was found that, when the dynamic scheme was applied, overall, the modeled O3 dry-deposition velocities and fluxes were larger and surface O3 concentrations were lower. The modeled O3 fluxes responded 2–3 times more strongly to the SM changes due to the DA, which can be mainly explained by the fact that both the direct and indirect (i.e., via influencing weather and vegetation fields) effects of SM on O3 dry-deposition modeling are considered in the dynamic scheme. Depending on soil type and hydrological regime, the selection of SM factor controlling rs (i.e., β factor, a key variable representing the direct effects of SM on the modeled surface fluxes) scheme can strongly affect the quantitative results. The Wesely-scheme-derived dry-deposition results driven by meteorological fields from Noah-MP-based and (from Huang et al., 2021) Noah-LSM-based WRF-Chem simulations displayed much smaller differences than those due to updating the dry-deposition parameterizations. While we note that accounting for physiological effects in dry-deposition modeling can be beneficial, the Ball–Berry rs scheme applied in land surface and dry-deposition modeling in this work needs to be compared with other semi-empirical rs schemes, for a better understanding of their respective strengths and weaknesses. Alternative schemes include the Medlyn scheme, which has been integrated into the CLM version 5. Model intercomparison efforts such as the ongoing Air Quality Model Evaluation International Initiative Phase 4 activity (Galmarini et al., 2021) can also help determine areas for improvement in commonly used dry-deposition modeling approaches for studying 2016 and other years, over North America and other regions of the world.
By analyzing the model responses to the SM DA from these various cases, we conclude that, in coupled modeling systems that consider the direct and indirect influences of SM on O3 dry deposition, the accuracy of SM is particularly critical to dry deposition and O3 modeling, as well as the scientific analyses and impact assessments based on model simulations. The usefulness of SM DA for improving the modeled state and flux variables was evaluated by multiple observation(-derived) data products. Referring to in situ measurements, key meteorological variables relevant to vd calculations such as surface temperature and humidity are shown to be improved by the DA by up to ∼9 %. Referring to satellite(-derived) datasets which may be associated with high uncertainty, the model performance of vegetation phenology, GPP, as well as energy fluxes and their partitioning, showed mixed, LULC-dependent reactions to the DA. According to the evaluation statistics, for this case, the CLM-type β factor scheme was slightly superior to the Noah-type one. The modeled carbon and energy fields, as well as their DA-related changes, correlated strongly with the modeled vd fields, implying that the DA impacts on the accuracy of vd were also possibly complicated, which is difficult to verify due to the lack of high-accuracy, independent vd evaluation datasets, a point that has also been brought up in previous dry-deposition modeling works (e.g., Baublitz et al., 2020; Clifton et al., 2020). Observation(-derived) vd datasets covering diverse LULC types nested in broad geographical regions and through more recent periods are in strong need. In places, the likely ineffectiveness of SM DA on vegetation and surface fluxes can not only be attributed to the quality of satellite SM retrievals and the DA approach used as discussed in previous Noah-LSM-based DA experiments, but also shortcomings in the Noah-MP LSM and its dynamic vegetation scheme regarding its surface–subsurface coupling and representation of SM-vegetation growth feedbacks. Continued efforts on advancing land measurement/retrieval skills and identifying and addressing deficits in LSMs, as well as practicing multivariate land DA, are recommended in future work.
This study also demonstrated that model-driven assessments of O3 impacts on human health and various types of vegetation can be significantly affected by the applied O3 dry-deposition scheme, the implementation of land DA, the chosen O3 metrics, and their matching exposure–response functions. Various model cases showed that the DA impacts on MDA8 were more evident in nonurban areas where the mean MDA8 was ∼5 ppbv lower and the average population density is of that in urban areas. Using concentration- and flux-based metrics AOT40 and PODy, the mean RYLs of maize, soybean, and wheat fell within ranges of 0.01–0.04, 0.10–0.17, and 0.04–0.14, respectively. The multiple no-DA and DA cases helped us better understand the indirect and/or direct effects of SM on O3 dry-deposition process, which have important implications for O3 impact assessments. It is also recognized that the DA often exacerbated the positive surface O3 biases in free-running systems, which has been a common issue shared by numerous regional and global models for this study region/season. It is necessary to combine land DA with efforts to identify, quantify, and reduce other sources of uncertainty in O3 modeling. These should include reasonably representing the impacts of O3 along with other factors on vegetation, the direct impacts of water stress on biogenic emissions of volatile organic compounds and nitrogen species, and the reduction of photolysis reaction rates and the modification of vertical transport due to the presence of foliage (Li et al., 2016; Jiang et al., 2018; Makar et al., 2017).
Dry-deposition-related updates to LIS/WRF-Chem since Huang et al. (2021) are undergoing reporting processes via NASA's New Technology Reporting System (https://invention.nasa.gov, last access: 4 June 2022). Model results as well as observations and observation-derived evaluation datasets emphasized in this work but not in Huang et al. (2021) can be found at the following locations: https://doi.org/10.5281/zenodo.6615022 (Huang, 2022), https://land.copernicus.eu/global/products/fcover (last access: 10 April 2022; Copernicus Global Land Service, 2020), https://doi.org/10.5067/L6C9EY1O8VIC (Kimball et al., 2021), https://doi.org/10.7927/H49C6VHW (NASA Socioeconomic Data and Applications Center, 2018), https://www-air.larc.nasa.gov/cgi-bin/ArcView/actamerica.2016 (last access: 8 November 2021; NASA, 2020), https://java.epa.gov/castnet/clearsession.do (last access: 8 November 2021; US Environmental Protection Agency, 2021), and https://doi.org/10.3334/ORNLDAAC/1696 (Yu et al., 2019).
The supplement related to this article is available online at: https://doi.org/10.5194/acp-22-7461-2022-supplement.
MH led the design and execution of the study as well as the paper writing, benefitting from discussions with JHC, GRC, KWB, and SVK, with the feedback from the Atmospheric Chemistry and Physics Editorial Board and reviewers for Huang et al. (2021) also accounted for. CS contributed to data collection during the ACT-America campaign. All authors helped finalize the paper.
The contact author has declared that neither they nor their co-authors have any competing interests.
Publisher's note: Copernicus Publications remains neutral with regard to jurisdictional claims in published maps and institutional affiliations.
We acknowledge NASA SMAP and ACT-America Science Teams and NASA's high-end computing systems and services at Ames and Goddard. We thank Jiang et al. (2018) for developing the γd dataset shown in Fig. S7. We also greatly appreciate active and relevant discussions with multiple colleagues from the Air Quality Model Evaluation International Initiative 4 and the Tropospheric Ozone Assessment Report II communities during and after recent conferences and workshops, particularly Christian Hogrefe, Jonathan Pleim, Paul Makar, Lisa Emberson, Bärbel Sinha, Danica Lombardozzi, Olivia Clifton, Louisa Emmons, Tamara Emmerichs, and Domenico Taraborrelli.
This research has been supported by NASA's Earth Science Division, through the Science Utilization of SMAP program (grant no. NNX16AN39G).
This paper was edited by Farahnaz Khosrawi and reviewed by two anonymous referees.
Anav, A., Proietti, C., Menut, L., Carnicelli, S., De Marco, A., and Paoletti, E.: Sensitivity of stomatal conductance to soil moisture: implications for tropospheric ozone, Atmos. Chem. Phys., 18, 5747–5763, https://doi.org/10.5194/acp-18-5747-2018, 2018.
Avnery, S., Mauzerall, D. L., Liu, J., and Horowitz, L. W.: Global Crop Yield Reductions due to Surface Ozone Exposure: 1. Year 2000 Crop Production Losses and Economic Damage, Atmos. Environ., 45, 2284–2296, https://doi.org/10.1016/j.atmosenv.2010.11.045, 2011.
Baillie, A. L. and Fleming, A. J.: The developmental relationship between stomata and mesophyll airspace, New Phytol., 225, 1120–1126, https://doi.org/10.1111/nph.16341, 2019.
Ball, J. T., Woodrow, I. E., and Berry, J. A.: A model predicting stomatal conductance and its contribution to the control of photosynthesis under different environmental conditions, Process in Photosynthesis Research, edited by: Biggins, J., 4, 221–224, Martinus Nijhoff, Dordrecht, the Netherlands, 1987.
Baublitz, C. B., Fiore, A. M., Clifton, O. E., Mao, J., Li, J., Correa, G., Westervelt, D. M., Horowitz, L. W., Paulot, F., and Williams, A. P.: Sensitivity of Tropospheric Ozone Over the Southeast USA to Dry Deposition, Geophys. Res. Lett., 47, e2020GL087158, https://doi.org/10.1029/2020GL087158, 2020.
Chen, F., Janjic, Z., and Mitchell, K. E.: Impact of atmospheric surface‐layer parameterizations in the new land‐surface scheme of the NCEP mesoscale Eta model, Bound.-Lay. Meteorol., 85, 391–421, https://doi.org/10.1023/A:1000531001463, 1997.
Clifton, O. E., Fiore, A. M., Munger, J. W., Malyshev, S., Horowitz, L. W., Shevliakova, E., Paulot, F., Murray, L. T., and Griffin, K. L.: Interannual variability in ozone removal by a temperate deciduous forest, Geophys. Res. Lett., 44, 542–552, https://doi.org/10.1002/2016GL070923, 2017.
Clifton, O. E., Paulot, F., Fiore, A. M., Horowitz, L. W., Correa, G., Baublitz, C. B., Fares, S., Goded, I., Goldstein, A. H., Gruening, C., Hogg, A. J., Loubet, B., Mammarella, I., Munger, J. W., Neil, L., Stella, P., Uddling, J., Vesala, T., and Weng, E.: Influence of dynamic ozone dry deposition on ozone pollution, J. Geophys. Res.-Atmos., 125, e2020JD032398, https://doi.org/10.1029/2020JD032398, 2020.
Convention on Long-Range Transboundary Air Pollution (CLRTAP): Mapping Critical Levels for Vegetation, Chapter 3 of Manual for modelling and mapping critical loads and levels, https://www.umweltbundesamt.de/en/manual-for-modelling-mapping-critical-loads-levels (last access: 10 December 2021), 2017.
Copernicus Global Land Service: Fraction of green vegetation cover 1 km version 2 data, Flemish Institute for Technological Research on behalf of the European Commission Joint Research Centre [data set], Boeretang, Belgium, https://land.copernicus.eu/global/products/fcover (last access: 10 April 2022), 2020.
Dieter, C. A., Maupin, M. A., Caldwell, R. R., Harris, M. A., Ivahnenko, T. I., Lovelace, J. K., Barber, N. L., and Linsey, K. S.: Estimated use of water in the United States in 2015, U. S. Geological Survey Circular 1441, 65, https://doi.org/10.3133/cir1441, 2018.
Ducker, J. A., Holmes, C. D., Keenan, T. F., Fares, S., Goldstein, A. H., Mammarella, I., Munger, J. W., and Schnell, J.: Synthetic ozone deposition and stomatal uptake at flux tower sites, Biogeosciences, 15, 5395–5413, https://doi.org/10.5194/bg-15-5395-2018, 2018.
Egea, G., Verhoef, A., and Vidale, P. L.: Towards an improved and more flexible representation of water stress in coupled photosynthesis–stomatal conductance models, Agric. For. Meteorol., 151, 1370–1384. https://doi.org/10.1016/j.agrformet.2011.05.019, 2011.
Emberson, L. D., Büker, P., Ashmore, M. R., Mills, G., Jackson, L., Agrawal, M., Atikuzzaman, M. D., Cinderby, S., Engardt, M., Jamir, C., Kobayashi, K., Oanh, N. T. K., Quadir, Q. F., and Wahid, A.: A comparison of North American and Asian exposure-response data for ozone effects on yield, Environ. Pollut., 43, 1945–1953, https://doi.org/10.1016/j.atmosenv.2009.01.005, 2009.
Emberson, L. D., Pleijel, H., Ainsworth, E. A., van den Berg, M., Ren, W., Osborne, S., Mills, G., Pandey, D., Dentener, F., Büker, P., Ewert, F., Koeble, R., and Van Dingenen, R.: Ozone effects on crops and consideration in crop models, Eur. J. Agron., 100, 19–34, https://doi.org/10.1016/j.eja.2018.06.002, 2018.
Emmerichs, T., Kerkweg, A., Ouwersloot, H., Fares, S., Mammarella, I., and Taraborrelli, D.: A revised dry deposition scheme for land–atmosphere exchange of trace gases in ECHAM/MESSy v2.54, Geosci. Model Dev., 14, 495–519, https://doi.org/10.5194/gmd-14-495-2021, 2021.
Entekhabi, D., Njoku, E. G., O'Neill, P. E., Kellogg, K. H., Crow, W. T., Edelstein, W. N., Entin, J. K., Goodman, S. D., Jackson, T. J., Johnson, J., Kimball, J., Piepmeier, J. R., Koster, R. D., Martin, N., McDonald, K. C., Moghaddam, M., Moran, S., Reichle, R., Shi, J. C., Spencer, M. W., Thurman, S. W., Tsang, L., and van Zyl, J.: The Soil Moisture Active Passive (SMAP) Mission, Proc. IEEE, 98, 704–716, https://doi.org/10.1109/JPROC.2010.2043918, 2010.
Falk, S. and Søvde Haslerud, A.: Update and evaluation of the ozone dry deposition in Oslo CTM3 v1.0, Geosci. Model Dev., 12, 4705–4728, https://doi.org/10.5194/gmd-12-4705-2019, 2019.
Finkelstein, P. L., Ellestad, T. G., Clarke, J. F., Meyers, T. P., Schwede, D. B., Hebert, E. O., and Neal, J. A.: Ozone and sulfur dioxide dry deposition to forests: Observations and model evaluation, J. Geophys. Res., 105, 15365–15377, https://doi.org/10.1029/2000JD900185, 2000.
Fleming, Z. L., Doherty, R. M., von Schneidemesser, E., Malley, C. S., Cooper, O. R., Pinto, J. P., Colette, A., Xu, X., Simpson, D., Schultz, M. G., Lefohn, A. S., Hamad, S., Moolla, R., Solberg, S., and Feng, Z.: Tropospheric Ozone Assessment Report: Present-day ozone distribution and trends relevant to human health, Elem. Sci. Anth., 6, 12, https://doi.org/10.1525/elementa.273, 2018.
Fredericksen, T. S., Skelly, J. M., Steiner, K. C., Kolb, T. E., and Kouterick, K. B.: Size-mediated foliar response to ozone in black cherry trees, Environ. Pollut., 91, 53–63, https://doi.org/10.1016/0269-7491(95)00032-m, 1996.
Fu, J. S., Carmichael, G. R., Dentener, F., Aas, W., Andersson, C., Barrie, L. A., Cole, A., Galy-Lacaux, C., Geddes, J., Itahashi, S., Kanakidou, M., Labrador, L., Paulot, F., Schwede, D., Tan, J., and Vet, R., Environ. Sci. Technol., 56, 2134–2142, https://doi.org/10.1021/acs.est.1c05929, 2022.
Galmarini, S., Makar, P., Clifton, O. E., Hogrefe, C., Bash, J. O., Bellasio, R., Bianconi, R., Bieser, J., Butler, T., Ducker, J., Flemming, J., Hodzic, A., Holmes, C. D., Kioutsioukis, I., Kranenburg, R., Lupascu, A., Perez-Camanyo, J. L., Pleim, J., Ryu, Y.-H., San Jose, R., Schwede, D., Silva, S., and Wolke, R.: Technical note: AQMEII4 Activity 1: evaluation of wet and dry deposition schemes as an integral part of regional-scale air quality models, Atmos. Chem. Phys., 21, 15663–15697, https://doi.org/10.5194/acp-21-15663-2021, 2021.
Griffiths, P. T., Murray, L. T., Zeng, G., Shin, Y. M., Abraham, N. L., Archibald, A. T., Deushi, M., Emmons, L. K., Galbally, I. E., Hassler, B., Horowitz, L. W., Keeble, J., Liu, J., Moeini, O., Naik, V., O'Connor, F. M., Oshima, N., Tarasick, D., Tilmes, S., Turnock, S. T., Wild, O., Young, P. J., and Zanis, P.: Tropospheric ozone in CMIP6 simulations, Atmos. Chem. Phys., 21, 4187–4218, https://doi.org/10.5194/acp-21-4187-2021, 2021.
Hardacre, C., Wild, O., and Emberson, L.: An evaluation of ozone dry deposition in global scale chemistry climate models, Atmos. Chem. Phys., 15, 6419–6436, https://doi.org/10.5194/acp-15-6419-2015, 2015.
Hemispheric Transport of Air Pollution: Impacts on Health, Ecosystems, and Climate, Chapter 5 of 2010 Final Assessment report, Part A: Ozone and particulate matter, https://doi.org/10.18356/2c908168-en, 2010.
Hollaway, M. J., Arnold, S. R., Challinor, A. J., and Emberson, L. D.: Intercontinental trans-boundary contributions to ozone-induced crop yield losses in the Northern Hemisphere, Biogeosciences, 9, 271–292, https://doi.org/10.5194/bg-9-271-2012, 2012.
Huang, M.: Ozone dry deposition and ozone fields modeled by WRF-Chem, Version 1, Zenodo [data set], https://doi.org/10.5281/zenodo.6615022, 2022.
Huang, M., Carmichael, G. R., Chai, T., Pierce, R. B., Oltmans, S. J., Jaffe, D. A., Bowman, K. W., Kaduwela, A., Cai, C., Spak, S. N., Weinheimer, A. J., Huey, L. G., and Diskin, G. S.: Impacts of transported background pollutants on summertime western US air quality: model evaluation, sensitivity analysis and data assimilation, Atmos. Chem. Phys., 13, 359–391, https://doi.org/10.5194/acp-13-359-2013, 2013.
Huang, M., Crawford, J. H., DiGangi, J. P., Carmichael, G. R., Bowman, K. W., Kumar, S. V., and Zhan, X.: Satellite soil moisture data assimilation impacts on modeling weather variables and ozone in the southeastern US – Part 1: An overview, Atmos. Chem. Phys., 21, 11013–11040, https://doi.org/10.5194/acp-21-11013-2021, 2021.
Intergovernmental Panel on Climate Change: the Sixth Assessment Report, Summary for Policymakers, https://www.ipcc.ch/report/ar6/wg1 (last access: 24 November 2021), 2021.
Jiang, X., Guenther, A., Potosnak, M., Geron, C., Seco, R., Karl, T., Kim, S., Gu, L., and Pallardy, S.: Isoprene emission response to drought and the impact on global atmospheric chemistry, Atmos. Environ., 183, 69–83, https://doi.org/10.1016/j.atmosenv.2018.01.026, 2018.
Kimball, J. S., Jones, L. A., Endsley, A., Kundig, T., and Reichle, R.: SMAP L4 Global Daily 9 km EASE-Grid Carbon Net Ecosystem Exchange, Version 6, NASA National Snow and Ice Data Center Distributed Active Archive Center [data set], Boulder, Colorado, USA, https://doi.org/10.5067/L6C9EY1O8VIC, 2021.
Kumar, S. V., Reichle, R. H., Koster, R. D., Crow, W. T., and Peters-Lidard, C. D.: Role of subsurface physics in the assimilation of surface soil moisture observations, J. Hydrometeorol., 10, 1534–1547, https://doi.org/10.1175/2009JHM1134.1, 2009.
Lacis, A. A., Wuebbles, D. J., and Logan, J. A.: Radiative forcing of climate by changes in the vertical distribution of ozone, J. Geophys. Res., 95, 9971–9981, https://doi.org/10.1029/JD095iD07p09971, 1990.
Lapina, K., Henze, D. K., Milford, J. B., Huang, M., Lin, M., Fiore, A. M., Carmichael, G., Pfister, G. G., and Bowman, K.: Assessment of source contributions to seasonal vegetative exposure to ozone in the U.S., J. Geophys. Res.-Atmos., 119, 324–340, https://doi.org/10.1002/2013JD020905, 2014.
Lawston, P. M., Santanello, J. A., Zaitchik, B. F., and Rodell, M.: Impact of irrigation methods on land surface model spinup and initialization of WRF forecasts, J. Hydrometeor., 16, 1135–1154, https://doi.org/10.1175/JHM-D-14-0203.1, 2015.
Li, J., Mahalov, A., and Hyde, P.: Simulating the impacts of chronic ozone exposure on plant conductance and photosynthesis, and on the regional hydroclimate using WRF/Chem, Environ. Res. Lett., 11, 114017, https://doi.org/10.1088/1748-9326/11/11/114017, 2016.
Lin, M., Malyshev, S., Shevliakova, E., Paulot, F., Horowitz, L. W., Fares, S., Mikkelsen, T. N., and Zhang, L.: Sensitivity of ozone dry deposition to ecosystem-atmosphere interactions: A critical appraisal of observations and simulations, Global Biogeochem. Cy., 33, 1264–1288, https://doi.org/10.1029/2018GB006157, 2019.
Lin, M., Horowitz, L.W., Xie, Y., Paulot, F., Malyshev, S., Shevlickova, E., Finco, A., Gerosa, G., Kubinstin, D., and Pilegaard, K.: Vegetation feedbacks during drought exacerbate ozone air pollution extremes in Europe, Nat. Clim. Chang., 10, 444–451, https://doi.org/10.1038/s41558-020-0743-y, 2020.
Lombardozzi, D., Levis, S., Bonan, G., Hess, P. G., and Sparks, J. P.: The Influence of Chronic Ozone Exposure on Global Carbon and Water Cycles, J. Climate, 28, 292–305, https://doi.org/10.1175/JCLI-D-14-00223.1, 2015.
Makar, P. A., Staebler, R. M., Akingunola, A., Zhang, J., McLinden, C., Kharol, S. K., Pabla, B., Cheung, P., and Zheng, Q.: The effects of forest canopy shading and turbulence on boundary layer ozone, Nat. Commun., 8, 15243, https://doi.org/10.1038/ncomms15243, 2017.
Mills, G., Buse, A., Gimeno, B., Bemejo, V., Holland, M., Emberson, L., and Pleijel, H.: A synthesis of AOT40-based response functions and critical levels of ozone for agricultural and horticultural crops, Atmos. Environ., 41, 2630–2643, https://doi.org/10.1016/j.atmosenv.2006.11.016, 2007.
Mills, G., Hayes, F., Simpson, D., Emberson, L., Norris, D., Harmens, H., and Büker, P.: Evidence of widespread effects of ozone on crops and (semi-) natural vegetation in Europe (1990–2006) in relation to AOT40-and flux-based risk maps, Global Change Biol., 17, 592–613, https://doi.org/10.1111/j.1365-2486.2010.02217.x, 2011.
Mills, G., Pleijel, H., Malley, C. S., Sinha, B., Cooper, O. R., Schultz, M. G., Neufeld, H. S., Simpson, D., Sharps, K., Feng, Z., Gerosa, G., Harmens, H., Kobayashi, K., Saxena, P., Paoletti, E., Sinha, V., and Xu, X.: Tropospheric Ozone Assessment Report: Present-day tropospheric ozone distribution and trends relevant to vegetation, Elem. Sci. Anth., 6, 47, https://doi.org/10.1525/elementa.302, 2018a.
Mills, G., Sharps, K., Simpson, D., Pleijel, H., Broberg, M., Uddling, J., Jaramillo, F., Davies, W. J., Dentener, F., van den Berg, M., Agrawal, M., Agrawal, S. B., Ainsworth, E. A., Buker, P., Emberson, L., Feng, Z., Harmens, H., Hayes, F., Kopbayashi, K., Paoletti, E., and Van Dingenen, R.: Ozone pollution will compromise efforts to increase global wheat production, Global Change Biol., 24, 3560–3574, https://doi.org/10.1111/gcb.14157, 2018b.
Monfreda, C., Ramankutty, N., and Foley, J. A.: Farming the planet: 2. Geographic distribution of crop areas, yields, physiological types, and net primary production in the year 2000, Global Biogeochem. Cycles, 22, GB1022, https://doi.org/10.1029/2007GB002947, 2008.
NASA: ACT-America 2016 1-minute Merged B-200/C-130 Data, Version R1/R4, NASA Langley Research Center Airborne Science Data for Atmospheric Composition [data set], Hampton, Virginia, USA, https://www-air.larc.nasa.gov/cgi-bin/ArcView/actamerica.2016 (last access: 8 November 2021), 2020.
NASA Socioeconomic Data and Applications Center: Gridded Population of the World, Version 4.11, Columbia University Center for International Earth Science Information Network [data set], Palisades, New York, USA, https://doi.org/10.7927/H49C6VHW, 2018.
Niu, G. Y., Yang, Z. L., Mitchell, K. E., Chen, F., Ek, M. B., Barlage, M., Kumar, A., Manning, K., Niyogi, D., Rosero, E., Tewari, M., and Xia, Y.: The community Noah land surface model with multiparameterization options (Noah-MP): 1. Model description and evaluation with local-scale measurements, J. Geophys. Res.-Atmos., 116, D12109, https://doi.org/10.1029/2010JD015139, 2011.
Niyogi, D. S. and Raman, S.: Comparison of Four Different Stomatal Resistance Schemes Using FIFE Observations, J. Appl. Meteorol. Climatol., 36, 903–917, https://doi.org/10.1175/1520-0450(1997)036<0903:COFDSR>2.0.CO;2, 1997.
Otu-Larbi, F., Conte, A., Fares, S., Wild, O., and Ashworth, K.: Current and future impacts of drought and ozone stress on Northern Hemisphere forests, Global Change Biol., 26, 6218–6234, https://doi.org/10.1111/gcb.15339, 2020.
Ronan, A. C., Ducker, J. A., Schnell, J. L., and Holmes, C. D.: Have improvements in ozone air quality reduced ozone uptake into plants?, Elem. Sci. Anth., 8, 2, https://doi.org/10.1525/elementa.399, 2020.
Salmon, J. M., Friedl, M. A., Frolking, S., Wisser, D., and Douglas, E. M.: Global rain-fed, irrigated, and paddy croplands: A new high resolution map derived from remote sensing, crop inventories and climate data, Int. J. Appl. Earth Obs., 38, 321–334, https://doi.org/10.1016/j.jag.2015.01.014, 2015.
Saylor, R. D., Wolfe, G. M., Meyers, T. P., and Hicks, B. B.: A corrected formulation of the Multilayer Model (MLM) for inferring gaseous dry deposition to vegetated surfaces, Atmos. Environ., 92, 141–145, https://doi.org/10.1016/j.atmosenv.2014.03.056, 2014.
Silva, S. J. and Heald, C. L.: Investigating dry deposition of ozone to vegetation, J. Geophys. Res.-Atmos., 123, 559–573, https://doi.org/10.1002/2017JD027278, 2018.
Steinkamp, J. and Lawrence, M. G.: Improvement and evaluation of simulated global biogenic soil NO emissions in an AC-GCM, Atmos. Chem. Phys., 11, 6063–6082, https://doi.org/10.5194/acp-11-6063-2011, 2011.
Stevenson, D. S., Dentener, F. J., Schultz, M. G., Ellingsen, K., van Noije, T. P. C., Wild, O., Zeng, G., Amann, M., Atherton, C. S., Bell, N., Bergmann, D. J., Bey, I., Butler, T., Cofala, J., Collins, W. J., Derwent, R. G., Doherty, R. M., Drevet, J., Eskes, H. J., Fiore, A. M., Gauss, M., Hauglustaine, D. A., Horowitz, L. W., Isaksen, I. S. A., Krol, M. C., Lamarque, J. F., Lawrence, M. G., Montanaro, V., Müller, J. F., Pitari, G., Prather, M. J., Pyle, J. A., Rast, S., Rodriquez, J. M., Sanderson, M. G., Savage, N. H., Shindell, D. T., Strahan, S. E., Sudo, K., and Szopa, S.: Multimodel ensemble simulations of present-day and near-future tropospheric ozone, J. Geophys. Res.-Atmos., 111, D08301, https://doi.org/10.1029/2005JD006338, 2006.
Strode, S. A., Rodriguez, J. M., Logan, J. A., Cooper, O. R., Witte, J. C., Lamsal, L. N., Damon, M., Van Aartsen, B., Steenrod, S. D., and Strahan, S. E.: Trends andvariability in surface ozone over the United States, J. Geophys. Res.-Atmos., 120, 9020–9042, https://doi.org/10.1002/2014JD022784, 2015.
Sweeney, C., Karion, A., Wolter, S., Newberger, T., Guenther, D., Higgs, J. A., Andrews, A. E., Lang, P. M., Neff, D., Dlugokencky, E., Miller, J. B., Montzka, S. A., Miller, B. R., Masarie, K. A., Biraud, S. C., Novelli, P. C., Crotwell, M., Crotwell, A. M., Thoning, K., and Tans, P. P.: Seasonal climatology of CO2 across North America from aircraft measurements in the NOAA/ESRL Global Greenhouse Gas Reference Network, J. Geophys. Res.-Atmos., 120, 5155–5190, https://doi.org/10.1002/2014jd022591, 2015.
US Environmental Protection Agency: CASTNET Historical Deposition Data, US EPA Office of Atmospheric Programs [data set], Research Triangle Park, North Carolina, USA, https://java.epa.gov/castnet/clearsession.do, last access: 8 November 2021.
Val Martin, M., Heald, C. L., and Arnold, S. R.: Coupling dry deposition to vegetation phenology in the Community Earth System Model: Implications for the simulation of surface O3, Geophys. Res. Lett., 41, 2988–2996, https://doi.org/10.1002/2014GL059651, 2014.
Van Dingenen, R., Dentener, F., Raes, F., Krol, M. C., Emberson, L., and Cofala, J.: The global impact of ozone on agricultural crop yields under current and future air quality legislation, Atmos. Environ., 43, 604–618, https://doi.org/10.1016/j.atmosenv.2008.10.033, 2009.
Wesely, M. L.: Parameterization of surface resistances to gaseous dry deposition in regional-scale numerical models, Atmos. Environ., 41, 52–63, https://doi.org/10.1016/j.atmosenv.2007.10.058, 1989.
Whelan, M. E., Anderegg, L. D., Badgley, G., Campbell, J. E., Commane, R., Frankenberg, C., Hilton, T. W., Kuai, L., Parazoo, N., Shiga, Y., Wang, Y., and Worden, J.: Scientific Communities Striving for a Common Cause: Innovations in Carbon Cycle Science, B. Am. Meteorol., 101, E1537–E1543, https://doi.org/10.1175/BAMS-D-19-0306.1, 2020.
Wickham, J., Stehman, S. V., Sorenson, D. G., Gass, L., and Dewitz, J. A.: Thematic accuracy assessment of the NLCD 2016 land cover for the conterminous United States, Remote Sens. Environ., 257, 2021, 112357, https://doi.org/10.1016/j.rse.2021.112357, 2021.
Wong, A. Y. H., Geddes, J. A., Tai, A. P. K., and Silva, S. J.: Importance of dry deposition parameterization choice in global simulations of surface ozone, Atmos. Chem. Phys., 19, 14365–14385, https://doi.org/10.5194/acp-19-14365-2019, 2019.
Wu, Z., Schwede, D. B., Vet, R., Walker, J. T., Shaw, M., Staebler, R., and Zhang, L.: Evaluation and Intercomparison of Five North American Dry Deposition Algorithms at a Mixed Forest Site, J. Adv. Model. Earth Sy., 10, 1571–1586, https://doi.org/10.1029/2017MS001231, 2018.
Yang, Z.-L., Niu, G.-Y., Mitchell, K. E., Chen, F., Ek, M. B., Barlage, M., Longuevergne, L., Manning, K., Niyogi, D., Tewari, M., and Xia, Y.: The community Noah land surface model with multiparameterization options (Noah-MP): 2. Evaluation over global river basins, J. Geophys. Res., 116, D12110, https://doi.org/10.1029/2010JD015140, 2011.
Yu, L., Wen, J., Chang, C. Y., Frankenberg, C., and Sun, Y.: High Resolution Global Contiguous SIF Estimates Derived from OCO-2 SIF and MODIS, Oak Ridge National Laboratory Distributed Active Archive Center [data set], Oak Ridge, Tennessee, USA, https://doi.org/10.3334/ORNLDAAC/1696, 2019.
Yue, X. and Unger, N.: Ozone vegetation damage effects on gross primary productivity in the United States, Atmos. Chem. Phys., 14, 9137–9153, https://doi.org/10.5194/acp-14-9137-2014, 2014.