the Creative Commons Attribution 4.0 License.
the Creative Commons Attribution 4.0 License.
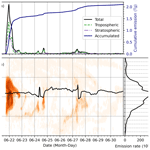
Improved estimation of volcanic SO2 injections from satellite retrievals and Lagrangian transport simulations: the 2019 Raikoke eruption
Sabine Griessbach
Lars Hoffmann
Monitoring and modeling of volcanic plumes are important for understanding the impact of volcanic activity on climate and for practical concerns, such as aviation safety or public health. Here, we apply the Lagrangian transport model Massive-Parallel Trajectory Calculations (MPTRAC) to estimate the SO2 injections into the upper troposphere and lower stratosphere by the eruption of the Raikoke volcano (48.29∘ N, 153.25∘ E) in June 2019 and its subsequent long-range transport and dispersion. First, we used SO2 retrievals from the AIRS (Atmospheric Infrared Sounder) and TROPOMI (TROPOspheric Monitoring Instrument) satellite instruments together with a backward trajectory approach to estimate the altitude-resolved SO2 injection time series. Second, we applied a scaling factor to the initial estimate of the SO2 mass and added an exponential decay to simulate the time evolution of the total SO2 mass. By comparing the estimated SO2 mass and the mass from TROPOMI retrievals, we show that the volcano injected 2.1 ± 0.2 Tg SO2, and the e-folding lifetime of the SO2 was about 13 to 17 d. The reconstructed SO2 injection time series are consistent between using the AIRS nighttime and the TROPOMI daytime products. Further, we compared forward transport simulations that were initialized by AIRS and TROPOMI SO2 products with a constant SO2 injection rate. The results show that the modeled SO2 change, driven by chemical reactions, captures the SO2 mass variations from TROPOMI retrievals. In addition, the forward simulations reproduce the SO2 distributions in the first ∼10 d after the eruption. However, diffusion in the forward simulations is too strong to capture the internal structure of the SO2 clouds, which is further quantified in the simulation of the compact SO2 cloud from late July to early August. Our study demonstrates the potential of using combined nadir satellite retrievals and Lagrangian transport simulations to further improve SO2 time- and height-resolved injection estimates of volcanic eruptions.
Injections of trace gases and ash by volcanic eruptions pose significant influences on the Earth's environment. Air pollutants such as sulfur dioxide (SO2), released by volcanic eruptions, can lead to a severe public health hazard and increase excess mortality (Schmidt et al., 2011). In addition, volcanic ash and gases can directly interrupt air flights passing through the volcanic plume and cause long-term damage to airplanes through physical and chemical corrosion, such as sulfidation due to SO2 (e.g., Grégoire et al., 2018; Prata, 2009). Furthermore, volcanic injections can influence the Earth's climate system through changes of radiative forcing (e.g., Robock, 2000; Kremser et al., 2016). Explosive volcanic eruptions can inject a significant amount of SO2 into the stratosphere, and oxidation of the SO2 forms stratospheric sulfate aerosol particles. Due to the limited potential of dry and wet deposition in the stratosphere, and due to the small sedimentation velocities, the sulfate aerosol particles have long lifetimes on timescales from months to years. In summary, as a precursor of stratospheric sulfate aerosol, and being a good proxy for other volcanic injections such as volcanic ash, monitoring and modeling of the injections and dispersion of volcanic SO2 can help to better understand the impacts of volcanic eruptions.
Although in situ observations are available for several well-studied volcanoes (e.g., Whitty et al., 2020), remote-sensing measurements from satellite instruments are more suited to provide long-term records and retrievals on a global scale. At present, there are several satellite instruments that can provide SO2 retrievals. Among them, the Atmospheric Infrared Sounder (AIRS) (Aumann et al., 2003; Chahine et al., 2006) aboard the National Aeronautics and Space Administration's (NASA) Aqua satellite provides retrievals of SO2 in the upper troposphere–lower stratosphere (UTLS) region (e.g., Carn et al., 2005; Prata and Bernardo, 2007; Hoffmann et al., 2014). AIRS observations have been available since 2002 and have near-global coverage during both day- and nighttime. The newly operational TROPOspheric Monitoring Instrument (TROPOMI) aboard the Sentinel 5 Precursor (S5P) (Veefkind et al., 2012), which has been a cooperative undertaking between the European Space Agency (ESA) and the Kingdom of the Netherlands since late 2017, provides daytime SO2 retrievals at an unprecedented spatial resolution (Theys et al., 2017, 2019), also covering the lower troposphere.
Beside the satellite retrievals, model simulations can help to characterize volcanic eruptions and provide forecasts of volcanic plume dispersion. In particular, Lagrangian particle dispersion models (LPDMs), which calculate air parcel trajectories following the fluid flow, are well suited for simulating complex transport processes (Lin et al., 2012). Widely used LPDMs include the Flexible Particle (FLEXPART) model (Stohl et al., 2005), the Hybrid Single-Particle Lagrangian Integrated Trajectory (HYSPLIT) model (Draxler and Hess, 1998), the Lagrangian Analysis Tool (LAGRANTO) (Wernli and Davies, 1997), the Numerical Atmospheric-dispersion Modeling Environment (NAME) (Jones et al., 2007), and the Stochastic Time-Inverted Lagrangian Transport model (Lin et al., 2003). A new LPDM, the Massive-Parallel Trajectory Calculations (MPTRAC) model, was recently developed at the Jülich Supercomputing Centre to take advantage of computing resources on state-of-the-art supercomputers (Hoffmann et al., 2016, 2022). The MPTRAC model has been successfully used to reconstruct volcanic SO2 injections (Heng et al., 2016; Hoffmann et al., 2016) and simulate the long-range transport of volcanic SO2 (Wu et al., 2017, 2018).
When simulating volcanic eruptions, suitable injection parameters, including the location, timing, and injection rate, are needed to initialize the LPDM simulations. Despite the importance of an accurate and reliable transport simulation, however, obtaining an accurate description of the injection parameters is challenging. Due to limited information regarding the injection parameters, the simplest assumption is a constant injection over the volcano (e.g., Muser et al., 2020; Kloss et al., 2021). However, uncertainties in the injection parameters can lead to errors in model simulations and consequently conflicting conclusions for a single volcanic eruption (Fromm et al., 2014). Complex modeling techniques using inversion algorithms and data assimilation have been developed to estimate volcanic injections (Eckhardt et al., 2008; Kristiansen et al., 2010; Flemming and Inness, 2013; Heng et al., 2016). In addition, the injection parameters can also be estimated based on backward trajectories (Hoffmann et al., 2016; Wu et al., 2017, 2018). The study of Heng et al. (2016) showed that forward transport simulation results using initialization strategies based on inverse modeling and the backward trajectory method may have comparable quality. Both the inverse modeling and backward trajectory methods considered here only give estimates of the altitude distribution and timing of volcanic injections. The SO2 mass of air parcels in the altitude- and time-resolved space is assigned using a prior assumption of the total mass of SO2 injections, which is usually estimated from satellite products. However, estimates of total SO2 mass can be very different from study to study. For instance, the estimation of total SO2 mass from the 2009 Sarychev eruption from different studies varies from 0.8 to 1.5 Tg (Fromm et al., 2014).
Several limitations may exist when using satellite products to estimate total SO2 mass from volcanic eruptions. Large uncertainties exist during the initial stage of volcanic eruptions. The high SO2 concentration in the early plume leads to saturation effects in satellite retrievals and, subsequently, an underestimation of the total mass. In addition, the co-presence of volcanic ash may also hamper the SO2 mass retrieval at the early stage of an eruption (Yang et al., 2010). Although there is higher confidence after the initial stage, the conversion process of SO2 to sulfate aerosol starts immediately after injection. Therefore, the SO2 total mass burden retrieved by the satellite at a later stage, when the plume is dispersed and the ash sedimented out, also tends to underestimate the total SO2 injection. In addition, the SO2 is often not injected by the volcano at a single time during the initial stage, which further complicates the estimation of the total injected SO2 mass.
The Raikoke volcano (48.29∘ N, 153.25∘ E) in the central Kuril Islands erupted during June 2019, sending a particularly large amount of ash and SO2 into the UTLS (Hedelt et al., 2019; Muser et al., 2020; de Leeuw et al., 2021; Horváth et al., 2021). It was estimated that the 2019 Raikoke eruption injected 1.5 ± 0.2 Tg SO2 into the atmosphere (Global Volcanism Program, 2019; Muser et al., 2020; de Leeuw et al., 2021), making it the largest SO2 injection into the UTLS since the 2011 Nabro eruption and the first large volcanic eruption since the beginning of operations of TROPOMI. Interestingly enough, the Raikoke eruption formed unique features of compact SO2 clouds with confined shapes and sizes (∼300 km in diameter) during the transport and dispersion of the SO2 injections (Chouza et al., 2020; Gorkavyi et al., 2021). Therefore, the 2019 Raikoke eruption provides an ideal test case to assess the ability to reconstruct the injection parameters using the state-of-the-art TROPOMI SO2 retrievals and to test how the reconstruction compares with retrievals using the older AIRS instrument. In addition, the compact SO2 cloud phenomenon related to the Raikoke eruption provides a unique opportunity to test the simulation of the transport and dispersion of the volcanic SO2. In this study, both questions are addressed.
The paper is structured as follows. In Sect. 2, we describe the satellite products of AIRS and TROPOMI and the MPTRAC model, as well as our method of reconstructing the injection parameters. The reconstructed injection parameters are presented in Sect. 3.1. In Sect. 3.2, we assess the performance of the MPTRAC model in simulating the transport and dispersion of the injected SO2 in terms of the total mass of the volcanic SO2, the spatial distribution of the SO2 cloud, and the degree of dispersion of the compact SO2 clouds. Finally, in Sect. 4 we discuss the results from our work through a comparison to previous studies, and main conclusions are drawn in Sect. 5.
2.1 AIRS SO2 retrievals
To estimate the injection parameters of volcanic SO2 and to initialize and validate the forward simulations with MPTRAC, we use SO2 retrievals from AIRS and TROPOMI. Since May 2002, AIRS/Aqua has operated on a polar sun-synchronous orbit with an equatorial crossing time of 01:30 local time (LT) for the descending orbit and 13:30 LT for the ascending orbit. The scan for each swath covers a width of 1780 km, consisting of 90 footprints, and the along-track distance of two adjacent swaths is 18 km. The sizes of the footprints are 13.5 km × 13.5 km at nadir and 41 km × 21.4 km at the scan extremes.
AIRS measures thermal infrared spectra in three bands between 3.74 and 15.4 µm. For the SO2 detection, we used the SO2 index (SI) defined by Hoffmann et al. (2014), which identifies the brightness temperature difference (BTD) between two different radiance channels (1407.2 and 1371.5 cm−1) from the AIRS spectral measurements in the 7.3 µm SO2 waveband. The SI provides SO2 information for the atmospheric column, but no vertical profile is directly available. The kernel function (Fig. 1) for the SI, based on radiative transfer calculations for a midlatitude atmosphere (Hoffmann et al., 2014), shows that the SI is most sensitive to SO2 layers at 8 to 13 km. The SI is measured in units of kelvin and increases with increasing SO2 column density. Here, we used a correlation function derived from the radiative transfer calculations of Hoffmann et al. (2014) for a midlatitude atmosphere to convert the SI to SO2 column density. Based on our inspection of the AIRS data, measurements beyond a threshold of 1.4 K or 5 Dobson units (DU) are clearly indicating the presence of volcanic SO2 from the 2019 Raikoke eruption. However, due to the conversion using an approximate correlation function, our estimates of total SO2 mass from AIRS are generally considered to be less reliable, and total SO2 mass will rather be obtained from the TROPOMI products in this study.
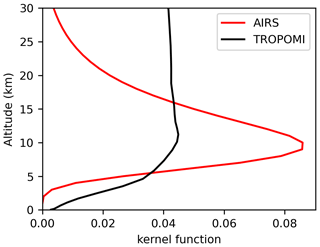
Figure 1Representative kernel functions for AIRS SO2 retrievals at midlatitudes and for TROPOMI SO2 retrievals over the Raikoke region.
As an example, Fig. 2 shows plots of the Raikoke SO2 clouds on 26 June 2019 as retrieved from AIRS (nighttime and daytime data, respectively) and TROPOMI (daytime data only) observations. Besides differences caused by the ∼12 h time shift, we found that the AIRS nighttime and daytime products were not always consistent with each other. They also showed some differences when reconstructing the Raikoke injection parameters. Therefore, the AIRS nighttime and daytime data are considered separately in this study.
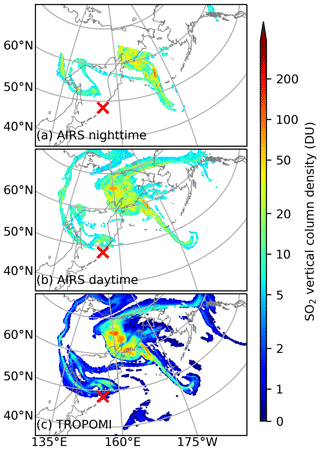
Figure 2Spatial distribution of SO2 vertical column density (DU) during the 24 h period between 26 June 2019, 12:00 UTC, and 27 June 2019, 12:00 UTC, from AIRS nighttime (a), AIRS daytime (b), and TROPOMI daytime (c) retrievals. Note that AIRS retrievals of SO2 vertical column density less than 5 DU are not shown here as those data are not actually used in the analysis because they are affected by background noise. The AIRS nighttime retrievals have a ∼12 h time shift compared with the AIRS daytime and TROPOMI retrievals.
2.2 TROPOMI SO2 retrievals
The TROPOspheric Monitoring Instrument (TROPOMI) is a single instrument on ESA's Copernicus Sentinel-5 Precursor satellite that was launched in October 2017. Sentinel-5P's mean local solar time of the ascending node is 13:30, and its orbit is aligned with NASA's Suomi-NPP mission (approximately 5 min behind) to allow for synergistic use with Suomi NPP's cloud products (Veefkind et al., 2012). TROPOMI consists of four passive grating imaging spectrometers measuring in the UV, Vis, NIR, and SWIR (Veefkind et al., 2012) and, hence, provides daytime measurements only. TROPOMI is a nadir instrument with a swath width of 2600 km and a very high spatial resolution of 7 × 3.5 km2 (until August 2019) (Veefkind et al., 2012; Romahn et al., 2021).
For the analysis of the Raikoke eruption we used the TROPOMI Level 2 offline (OFFL) V01.01.07 SO2 data product for the time period between 20 June 2019 and 16 August 2019. The TROPOMI SO2 data product provides four total vertical columns of SO2 in moles per square meter, one for the total atmospheric column between the surface and the top of the atmosphere and three columns assuming an SO2 layer at 1, 7, and 15 km altitude in the retrieval. The details of the retrieval are given in Theys et al. (2017, 2021). In studies investigating volcanic plumes, it is common to use the vertical column densities retrieved for distinct plume heights (e.g., Theys et al., 2019). In this study, we used the total vertical SO2 column of the 15 km retrieval, as we considered it to provide the best approximation for the Raikoke eruption as in other studies (e.g., Muser et al., 2020; de Leeuw et al., 2021). Compared with AIRS, the lower detection limit for TROPOMI is 0.3 DU (Theys et al., 2021), and data below this threshold are discarded in this study.
2.3 The MPTRAC model
Massive-Parallel Trajectory Calculations (MPTRAC) is a Lagrangian particle dispersion model for the analysis of atmospheric transport processes in the troposphere and stratosphere (Hoffmann et al., 2016). It calculates particle trajectories by solving the kinematic equation of motion using given wind fields from reanalysis or forecast meteorological data. The MPTRAC model currently uses the midpoint method to solve the equation of motion, which gives the optimized balance between accuracy and computational efficiency (Rößler et al., 2018). Besides vertical motion driven by the vertical velocity (i.e., kinematic trajectories), the MPTRAC model provides options to constrain the pressure of the air parcels to either constant pressure (isobaric surface), constant density (isopycnic surface), potential temperature (isentropic surface), or pressure time series from balloon measurements (Hoffmann et al., 2017). In addition, the model also includes turbulent diffusion and subgrid-scale wind fluctuations to simulate the diffusion. The turbulent diffusion is described by fixed diffusivity coefficients. Following the FLEXPART model (Stohl et al., 2005), the MPTRAC model uses a constant horizontal diffusivity of 50 m2 s−1 for the troposphere and a vertical diffusivity of 0.1 m2 s−1 for the stratosphere as default values. Subgrid-scale wind fluctuations are simulated using the Langevin equation to add time-correlated stochastic perturbations to the trajectories. The subgrid-scale wind standard deviations are downscaled from the grid scale standard deviations using a default scaling factor of 40 % (Stohl et al., 2005). To investigate the effect of parameterizations of turbulent diffusion and subgrid-scale wind fluctuations on the simulated SO2 dispersion for the Raikoke case, we varied the diffusivity and the scaling factor of the subgrid-scale variance separately. As the actual diffusivity can vary by several orders of magnitude (e.g., Ishikawa, 1995; Desiato et al., 1998; Legras et al., 2005; Pisso et al., 2009), we tested the turbulent diffusion by varying the diffusivities from 10−2 to 103 times the default values. For subgrid-scale wind fluctuations, we varied the scaling factor from 0 % to 100 %.
Additional modules are implemented to simulate convection, sedimentation, radioactive decay, hydroxyl chemistry, dry deposition, and wet deposition. In this study, we used the hydroxyl chemistry module to simulate the loss of SO2 by its reaction with the hydroxyl radical (OH). The MPTRAC model also provides variable output methods. In this study, we implemented a new module for “sample output”, which allows us to sample the model data at the exact time and location of the satellite overpasses/footprints. In addition to the trajectory and gridded outputs, the model also provides ways to directly evaluate the performance of the simulations, such as calculating the critical success index (CSI) (Wilks, 2011). Basically, the CSI is based on the counts of detection by the satellite retrieval and simulation on a regular grid basis. If the vertical column density in a grid cell passed a user-specified threshold, it will be counted as “yes”; otherwise it will be counted as “no”. The CSI is the ratio between the count of hits and the total number of hits, false alarms, and misses. Along with CSI, the probability of detection (POD) and the false alarm rate (FAR) are also calculated.
In this study, the main meteorological data used to drive the MPTRAC simulations are taken from the ERA5 reanalysis. The ERA5 is the ECMWF's (European Centre for Medium-Range Weather Forecasts) fifth-generation reanalysis (Hersbach et al., 2020), which is meant to replace its predecessor ERA-Interim (Dee et al., 2011). ERA5 provides hourly outputs of a comprehensive set of variables at a 31 km horizontal resolution and 137 vertical levels spanning from the surface up to 0.01 hPa. In this study, the ERA5 data are interpolated to a horizontal resolution. In comparison, the ERA-Interim data have a horizontal resolution of 80 km, 60 model levels, and output every 6 h, i.e., at 00:00, 06:00, 12:00, and 18:00 UTC. The differences between ERA5 and ERA-Interim in driving Lagrangian transport simulations have been assessed by Hoffmann et al. (2019), finding that the choice of data has a considerable impact on the simulations, in particular due to better spatial and temporal resolutions of the ERA5 data. We also considered both ERA5 and ERA-Interim data in this study, with a major focus on results derived from the ERA5 reanalysis.
2.4 Estimation of volcanic SO2 injections
To reconstruct the altitude- and time-resolved injection parameters of the Raikoke eruption, being represented by the altitude, time, and SO2 mass of each air parcel over the volcano, we used a method based on backward trajectories released from the columns of the AIRS and TROPOMI SO2 measurements (Hoffmann et al., 2016; Wu et al., 2017, 2018). The analysis was done separately for AIRS and TROPOMI data, covering time periods from a few days up to weeks after the eruption, and the results were compared against each other. As both AIRS and TROPOMI provide information on the horizontal location and time of SO2 retrievals but lack information on the vertical distribution of the SO2, we released multiple air parcels between 0 and 25 km altitude at each individual satellite footprint with volcanic SO2 detections. In contrast to our earlier work, the vertical profile of the number of air parcels has been made to follow the mean kernel function of the satellite measurements (Fig. 1) in order to take into account their different vertical sensitivity. The total number of air parcels at each location was linearly proportional to the total column density of the satellite retrievals. At the same time, a Gaussian scatter of the air parcels with 15 and 5 km full width at half maximum (FWHM) was introduced to represent the horizontal footprint size for AIRS and TROPOMI, respectively.
In total, 5 million air parcels were released to calculate backward trajectories. If a backward trajectory passed the Raikoke volcano within a search radius of 15 km, the location and time of the air parcel were saved to reconstruct the injection parameters. We note that, based on our sensitivity tests, the results are not very sensitive to FWHM (i.e., between 1 and 50 km) and the search radius around the volcano (i.e., between 1 and 100 km). However, it is possible that for a given TROPOMI pixel location, there may be multiple solutions in the backward trajectory method, especially if the wind does not change with height and over time. In the study, this uncertainty has been reduced through two ways. First, all TROPOMI overpasses over many days, in the final reconstruction 12 d, were used, and second, an increased number of particles, 5 million air parcels, were released in the backward run. All backward trajectories that met the selection condition were resampled to a total number of 5 million particles, and an initial total mass of 1.5 Tg was assigned to them. After the initial relative SO2 distribution has been estimated from the backward trajectories, we conducted forward simulations and applied a scaling factor to the SO2 total mass for further calibration. To calibrate the total mass, we assumed that the SO2 starts to decay exponentially with a fixed e-folding lifetime, representing the overall removal of the SO2, immediately after the injection and compared the change of the SO2 total mass from the simulations with the change of the SO2 total mass derived from TROPOMI retrievals.
3.1 Volcanic SO2 injections parameters
3.1.1 Final reconstruction of Raikoke SO2 injections
Figure 3 shows the final reconstruction of the Raikoke SO2 injections based on the TROPOMI SO2 product and Lagrangian transport simulations using ERA5 winds. The mass in the reconstruction has been tuned to achieve a total injection of 2.1 Tg (see Sect. 3.1.2 for details on how the total mass was derived). The altitude- and time-resolved injection and the integrated vertical profile are also shown in Fig. 3. A major SO2 injection was reconstructed during the first 2 d of the time series, i.e., between 21 and 22 June 2019. After this major eruption, significantly smaller amounts of SO2 were continuously injected by the volcano until the end of June, with a prominent second and third plume during 24–25 June and 27–28 June, respectively. The first plume crossed the temperature lapse-rate tropopause (WMO, 1957) and injected SO2 between 5 and 15 km of altitude, with ∼45 % of the SO2 mass reaching the stratosphere (Fig. 3a and b). The second and the third plumes mainly injected material into the troposphere. As the Raikoke eruption is dominated by the first plume, the overall injected SO2 (Fig. 3c) distributes around the tropopause, with peak injections at an altitude of 11 km.
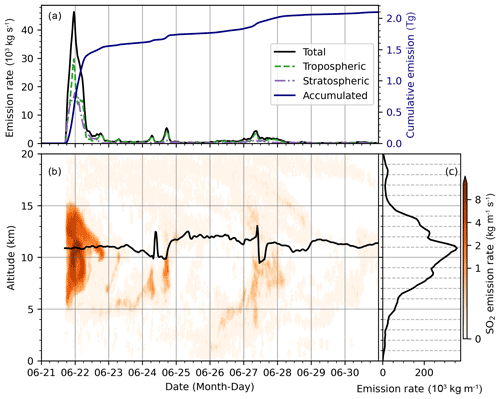
Figure 3Reconstructed SO2 injections of the 2019 Raikoke eruption based on the TROPOMI SO2 product. (a) Temporal evolution of the vertically integrated SO2 injection rates (kg s−1) for the whole atmosphere, the troposphere, and the stratosphere. The temporal change of accumulated SO2 injections (integrated for the whole atmospheric column and over time) is also plotted in (a). (b) Altitude-resolved SO2 injection rate time series (kg m−1 s−1). The black line represents the ERA5 temperature lapse-rate tropopause (WMO, 1957) over the Raikoke volcano. (c) Altitude profile of the SO2 injection rates (kg m−1).
As an intercomparison as well as a validation, the vertical profiles integrated over the entire eruption period (21–30 June 2019) of our different injection estimations and the profile derived by the VolRes team (de Leeuw et al., 2021) are shown in Fig. 4. The profile derived by the VolRes team is mainly based on IASI retrievals during the first 2 d of the Raikoke eruption (de Leeuw et al., 2021). Compared with the VolRes profile, the altitude of peak injections is about 1 km above the VolRes profile, no matter which satellite data, i.e., AIRS nighttime or TROPOMI daytime, and reanalysis data, i.e., ERA5 or ERA-Interim, have been used. Our reconstructions also show enhanced injections between 12 and 14 km, being consistent with de Leeuw et al. (2021) that the injections reached higher into the stratosphere than indicated by the VolRes estimation. When excluding the second and third plume, the vertical profile for the first major eruption (figure not shown) is similar to the overall injection profile (Fig. 4), with a slightly reduced injection rate in the stratosphere and a larger reduction in the tropospheric part. The VolRes profile also indicates a small peak at low altitudes around 2 km. However, this part is not found in our reconstruction. The most likely reason is that both AIRS and TROPOMI have a limited sensitivity in the lower troposphere, i.e., below 5 km (Fig. 1).
3.1.2 Calibration of the total mass of the SO2 injections
For the initial reconstruction, we estimated the SO2 injection rates under the assumption of a SO2 total mass of 1.5 Tg, as found by the VolRes team. Figure 5 shows the time series of the vertically integrated SO2 injections. The comparison of the reconstructions based on different satellite SO2 products (Fig. 5) shows that the results derived from AIRS nighttime and TROPOMI SO2 products agree well. The reconstruction derived from the AIRS daytime SO2 product shows weaker injections in the first plume, but the second and third plume are stronger compared to the reconstructions based on AIRS nighttime and TROPOMI SO2 products. As pointed out in Sect. 2.1, we will focus our analyses on the AIRS nighttime and TROPOMI results in the following parts.
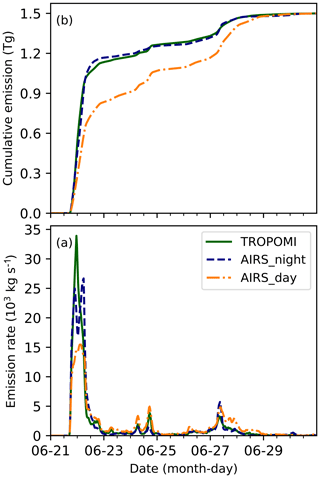
Figure 5Temporal change of the Raikoke SO2 injections reconstructed based on the TROPOMI SO2 product (green line) and AIRS SO2 product during nighttime (dashed blue line) and daytime (dashed–dotted orange line): (a) vertically integrated SO2 injection rate and (b) accumulated SO2 mass.
To estimate the final total injected SO2 mass, we derived the daily SO2 mass from the TROPOMI SO2 product (Fig. 6). The TROPOMI SO2 product shows that a total SO2 mass of ∼1.4 Tg peaked during 24–26 June, while the cumulative SO2 injection from the initial reconstruction at 26 June is only ∼1.2 Tg. When the cumulative SO2 injection in the initial reconstruction reached 1.5 Tg, the total SO2 mass from TROPOMI decreased to ∼1.2 Tg due to the removal of SO2. To better represent the evolution of the total SO2 mass in the simulations, we scaled our initial mass reconstruction to the TROPOMI data and applied an exponential decay to represent the overall removal of SO2 (Fig. 6; Sect. 3.2.1 gives more detailed information on the lifetime). In this experiment, we found that a total injection of 1.9 to 2.3 Tg SO2 and an e-folding lifetime of 13 to 17 d best represent the temporal evolution of total SO2 mass in the atmosphere. Therefore, we rescaled the initial reconstruction to a total mass of 2.1 Tg. Note that although the e-folding lifetime of 13–17 d represents the overall removal of SO2 injections for the Raikoke case well, SO2 removal rates in general are very sensitive to the altitude of the SO2 injections and the atmospheric background conditions.
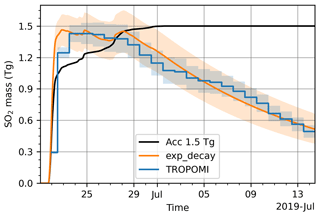
Figure 6Temporal change of total SO2 mass from TROPOMI measurements (blue) and calculated total mass with an e-folding lifetime of 15 d (orange) and a mass scaling factor of . Orange shadings show the combination of the scaling factor ranging between and and an e-folding lifetime ranging between 13 and 17 d. The black curve shows the accumulated SO2 injection with a total injection of 1.5 Tg (the initial reconstruction).
3.1.3 Sensitivities of estimated SO2 injections to data and model parameters
To investigate the sensitivity of the reconstructed injection time series to the underlying input data, we ran the reconstruction using the AIRS SO2 product together with ERA5 winds as well as the TROPOMI SO2 product together with ERA-Interim winds. In comparison to TROPOMI and ERA5, the overall patterns of injection estimations based on AIRS nighttime observations and ERA5 (Fig. 7a) or TROPOMI observations and ERA-Interim (Fig. 7b) are quite similar. For the first plume, i.e., between 21 and 22 June, the estimation based on AIRS nighttime observations and ERA5 shows stronger injections during the beginning and late stage of the plume, while the estimation based on TROPOMI observations and ERA-Interim shows weaker (stronger) injections at the beginning (late) stage of the first plume, respectively. Differences do exist during the second and the third plumes, but they are relatively small.
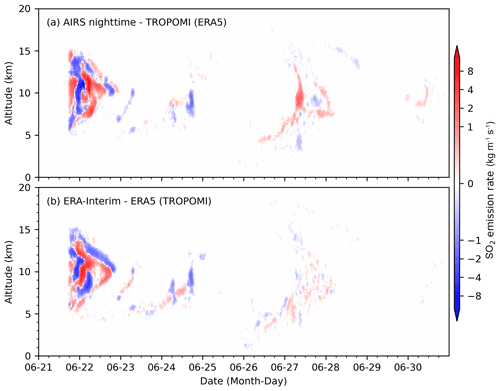
Figure 7Differences in reconstructed SO2 injections of the 2019 Raikoke eruption derived from different combinations of satellite products and reanalysis data compared with the TROPOMI-ERA5 combination: AIRS nighttime with ERA5 (a) and TROPOMI with ERA-Interim (b).
We conducted more than 200 simulations to test the sensitivity of the injection reconstructions. Among the tested parameters, we found that the coverage of the satellite observations has the largest impact on the injection reconstruction. More specifically, it matters how many days of satellite retrievals are used for the reconstruction and how close to the location of the volcano satellite retrievals are discarded. Here, we only describe the sensitivity tests on the temporal and spatial coverage of the satellite retrievals, whereas the sensitivity tests on other parameters are not shown.
Figure 8 shows the SO2 mass change in forward simulations initialized using TROPOMI observations covering different numbers of days since the beginning of the eruption. The total SO2 mass in all the simulations was assigned to 2.1 Tg. As shown in Fig. 8, when using just a few days of observations, the simulation produces too strong a peak at the beginning of the volcanic eruption. Increasing the time period of the satellite data, a gradual decrease of the first peak and redistribution of SO2 to a later stage of the eruption is observed in the different forward simulations. Therefore, using short-term observations will lead to a more pronounced first plume, and conversely, longer-term observations will increase the amplitude of the second and third plume. The sensitivity test shows that using 12 d of observations gives an optimal representation of the SO2 mass.
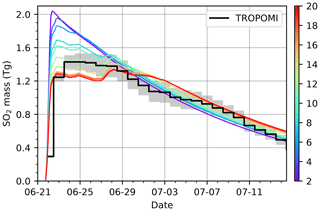
Figure 8Temporal change of SO2 total mass in MPTRAC forward simulations, which were initialized by considering different numbers of days of TROPOMI observations since the beginning of the Raikoke eruption (see color bar). Total mass of SO2 injection in all simulations is 2.1 Tg. In the forward simulations, the mass is derived from the chemistry module. The mass changes measured by TROPOMI are shown by the black line, and gray shading indicates the measurement errors.
As the backward trajectory method heavily relies on the quality of the trajectories, satellite observations too close to the volcano can not provide enough information to separate between different altitudes. Therefore, we defined a circle with a certain distance to the Raikoke volcano, where the satellite observations falling inside the circle are discarded. When using the TROPOMI SO2 product and the distance is set to a very small value, such as a few kilometers, most of the reconstructed SO2 is injected at the beginning of the eruption. When the distance is set to several hundred kilometers, similar to increasing the temporal duration of the trajectories, the injection of SO2 at the beginning of the eruption is weakened, and more SO2 is injected within a few to several days after the beginning of the eruption. When using the AIRS product, this effect becomes less pronounced. Overall, the AIRS and TROPOMI reconstructions agreed better with each other when setting a larger distance. In the final reconstruction, we used a distance of 750 km, which gave the most consistent results.
3.2 Forward simulations for the Raikoke eruption
3.2.1 Simulations of SO2 total mass
We performed forward simulations initialized by the different reconstructed SO2 injection parameters as well as a constant SO2 injection rate. For the forward simulation with a constant injection, we uniformly assigned 1.5 Tg SO2, which is the initial estimate of the total SO2 injection, from 5 to 15 km and from 21 June 2019, 18:00 UTC, to 22 June 2019, 06:00 UTC. Unless noted differently, all forward simulations that were initialized by a constant injection rate in the following sections have the same setup as described here. In addition, we also performed a forward simulation initialized by the VolRes profile with a total SO2 injection of 1.5 Tg assigned between 21 June 2019, 18:00 UTC, and 22 June 2019, 06:00 UTC. In the following subsections, we will present and compare the forward simulations of SO2 in terms of total mass burden and spatial distributions. In addition, we also performed different forward simulations driven by ERA5 and ERA-Interim data. However, the overall patterns of simulated SO2 were generally similar between ERA5 and ERA-Interim. Therefore, if not specified otherwise, the forward simulations driven by the ERA5 data are shown.
In the most recent version of the MPTRAC model, a hydroxyl (OH) chemistry module has been implemented to simulate the removal of SO2 due to the thermomolecular reaction with OH (Hoffmann et al., 2022). Monthly mean zonal mean OH concentrations are obtained from the study of Pommrich et al. (2014). This module enables the direct comparison of total SO2 mass change in model simulations with the simple exponential decay experiments and the satellite product by TROPOMI (Fig. 9). In the forward simulations, we have used different injection parameters with total injected SO2 mass ranging from 1.9 to 2.3 Tg. As shown in Fig. 9, the SO2 mass in the forward simulation initialized with a 2.1 Tg total injection, either using injection parameters derived from TROPOMI daytime (Fig. 9a) or AIRS nighttime (Fig. 9b) observations, agrees well with the exponential decay experiment of a 15 d e-folding lifetime. In addition, all the experiments are consistent with the total SO2 mass derived from TROPOMI SO2 retrievals. Figure 9 also shows the SO2 mass change in the forward simulation initialized by a constant injection time series. In contrast to the forward simulation initialized by our reconstructed injection time series, the simulation initialized with a constant injection rate or the VolRes profile produced an SO2 mass peak, which is comparable with the maximum SO2 mass in TROPOMI retrievals, at the beginning of the eruption. Then, the gradual removal of SO2 leads to lower mass in the model simulation than TROPOMI retrievals (Fig. 9). From these comparisons, we conclude that the June 2019 Raikoke eruption produced a total injection of 2.1 Tg SO2, which has an overall e-folding lifetime of 15 d in the UTLS region during the first 3 weeks after the eruption.
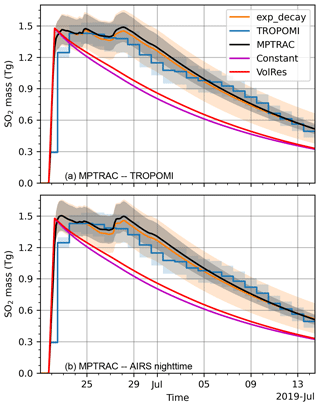
Figure 9Temporal change of total SO2 mass in the MPTRAC forward simulation (black lines) initialized by TROPOMI observations (a) and AIRS nighttime observations (b), respectively. Gray shadings show the range of total injection between 1.9 and 2.3 Tg. Temporal changes of total SO2 mass in the MPTRAC forward simulations initialized by the VolRes profile (red) and a constant injection rate (magenta) of 1.5 Tg total injection are also plotted. In the forward simulations, the mass is derived from the chemistry module. The mass changes measured by TROPOMI (blue) and modeled by an exponential decay (orange) from Fig. 6 are repeated here for comparison.
The comparison of the temporal changes of the SO2 total mass among the different forward simulation settings and TROPOMI retrievals (Fig. 9) suggests that our estimation of 2.1 Tg SO2 injection is reasonable. The initial estimation of 1.5 Tg mainly reflects SO2 injections of the major eruption during the first 2 d. Consistent with this estimate, the total mass in our estimation for the first plume is about 1.5 Tg (Fig. 3a). However, additional injections after the first plume are required to reproduce the retrieved SO2 mass in the model simulations (Fig. 9).
Although an e-folding lifetime of 15 d captures the overall mass reduction of injected SO2 in the atmosphere well, the real removal rates of SO2 at different altitudes are different. Figure 10 shows the remaining mass of SO2 injected to 1 km thick layers between 0–25 km during the first 12 h of the eruption (21 June 2019, 18:00 UTC to 22 June 2019, 06:00 UTC). In general, the removal rate decreases with altitude, mostly because of lower OH concentrations in the lower stratosphere compared to the troposphere. In the troposphere, the SO2 mass is reduced to less than 50 % within several days to a week, while the stratospheric injections still have ∼70 % at 10 d after the eruption. This means that the tropospheric injections are removed quickly during the early stage of the eruption, and the stratospheric injections gradually dominate. In the satellite SO2 retrievals, the vertical column density of the SO2 cloud associated with the tropospheric injection also decreases faster than the SO2 cloud associated with stratospheric injection (Fig. 11; see details below). This observation also confirms the faster removal of tropospheric parts of the SO2 injections.
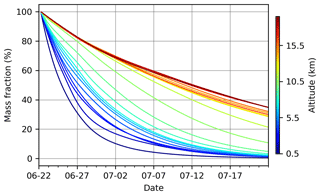
Figure 10Temporal change of the remaining fraction of SO2 mass for injections at different 1 km thick layers between 0 and 25 km and from 21 June 2019, 18:00 UTC, to 22 June 2019, 06:00 UTC. Colors indicate the altitude at the middle of each 1 km thick layer.
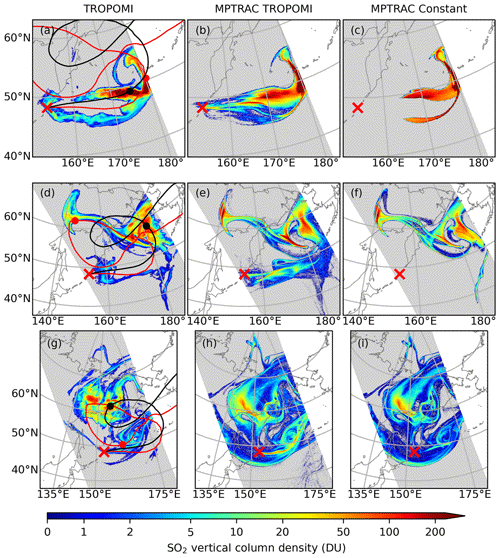
Figure 11TROPOMI retrievals and MPTRAC forward simulations of SO2 transport. Top row (a–c): spatial distribution of SO2 vertical column density (DU) from the TROPOMI orbit that starts at 23 June 2019, 01:05 UTC, and ends at 23 June 2019, 02:47 UTC (a), and the corresponding distribution in forward simulations, which were initialized by TROPOMI retrievals (b) and by a constant injection rate (c), respectively. Middle and bottom rows show the same as the top row but for the orbits of 25 June 2019, 00:27–02:09 UTC, and 28 June 2019, 01:12–02:53 UTC, respectively. The mean trajectories for injections between 7–8 km (red curve) and 11–15 km (black curves) are plotted on the maps on the left, and the mean locations at the corresponding time of each map are indicated by red and black dots.
3.2.2 Simulation of SO2 transport during the first ∼10 d
In general, the forward simulations initialized by both the TROPOMI daytime and AIRS nighttime SO2 products reproduce the spatial distribution of SO2 during the first ∼10 d of simulation time well, especially in terms of spatial location and extent. We note that the forward simulations initialized by the TROPOMI and AIRS nighttime products are highly consistent with each other, as the injection parameters estimated from these two datasets do not differ fundamentally (Fig. 7). Therefore, the results from the forward simulation initialized by the AIRS nighttime product are not shown here. As our forward simulations initialized by the VolRes profile are identical with the results presented by de Leeuw et al. (2021), SO2 distributions in these simulations are not shown here. To illustrate the performance after major SO2 injections of Raikoke, we selected three satellite overpasses on 23, 25, and 28 June to show the SO2 distribution in retrievals and model simulations (Fig. 11).
The TROPOMI retrievals and the MPTRAC simulations show that the SO2 injections are separated into two major clouds (Fig. 11). We added mean trajectories for injections between 7–8 and 11–15 km in Fig. 11 to indicate the major movements of the two SO2 clouds. Both of the SO2 clouds are moving cyclonically. A smaller SO2 cloud, which is represented by the mean trajectory for injections between 7 and 8 km, moves faster, and the SO2 column density also decreased very fast to less than 10 DU on 28 June. The SO2 column density in the other major cloud, which mainly reflects the stratospheric injections, decreased much slower compared with the SO2 cloud that reflects tropospheric injections. This observation by TROPOMI retrievals is consistent with the faster removal of SO2 in the troposphere (Fig. 10).
After the first injection, the TROPOMI SO2 product shows that the SO2 clouds are located to the east of the Raikoke volcano but split into two branches, with one branch being in the north and the other branch being in the south (Fig. 11a). The forward simulations initialized by the TROPOMI retrievals and a constant injection rate reproduce the main northern branch, which is located just to the east of the Raikoke volcano (Fig. 11b and c). However, both simulations only reproduce a part of the southern branch and the part reproduced in the simulation initialized by a constant injection rate is too strong. Compared with the major northern branch SO2 cloud, we find that the southern branch is very weak with SO2 column densities mostly less than 10 DU. The much lower column density reduces the chance of identifying a source associated with this part of the SO2 cloud. In addition, the TROPOMI averaging kernel significantly reduces the air parcels that started at altitudes below 5 km, which further reduces the chance of identifying a source at altitudes lower than 5 km. A sensitivity test suggested that the southern branch is mainly associated with transport of SO2 in the lower troposphere (between 0 and 5 km), which was not represented in both initializations.
After the second plume on 25 June (Fig. 11d–f), most of the SO2 injections were moved to the northwest direction over the Asian continent and to the northeast direction over the northwest Pacific Ocean (Fig. 11d). In addition to these two major SO2 clouds, there is a weaker SO2 cloud to the east of the Raikoke volcano, which is probably related to the injections between 23 and 25 June (Fig. 3). The forward simulation initialized by the TROPOMI SO2 product reproduced the general pattern of the three clusters. However, the forward simulation initialized by a constant injection rate only reproduces the two major SO2 clouds in the northwest and the northeast directions (Fig. 11f). Injections at lower altitudes from the VolRes profile could also partly explain the part not represented in Fig. 11f; however that part is also underestimated in the forward simulation initialized by the VolRes profile shown in de Leeuw et al. (2021).
After the third plume on 28 June (Fig. 11g), the SO2 cloud that was located over the Asian continent in the northwest direction now moved back to the east of Raikoke along a cyclonic circulation. In contrast, the SO2 cloud that was over the northwest Pacific Ocean showed a slower movement and is now located over the Asian continent (Fig. 11g). Both forward simulations reproduced the two major SO2 clouds. The forward simulation initialized by the TROPOMI SO2 product reproduced a stronger SO2 cloud to the east of the Raikoke volcano (Fig. 11h) due to injections during the third plume. Similarly, the simulated SO2 cloud over the volcano after the second plume is also stronger than in the satellite retrievals (Fig. 11e). This result indicates that our reconstructed injection parameters potentially overestimate the second and the third plume. However, totally removing the second and/or the third plume would severely reduce the ability to correctly simulate the total SO2 mass burden as shown in Fig. 9.
Although the forward simulations can reproduce the retrieved SO2 distributions with relatively high performance during the first week (Fig. 11), the simulation starts gradually losing the ability to capture the structures of the SO2 cloud thereafter. Figure 12 shows the retrieved and simulated spatial distribution of SO2 at the beginning of 1 July. Overall, the SO2 distributes like a strip pattern with major peaks over the Sea of Okhotsk and the west coast of the Bering Sea (Fig. 12a). The model simulation captured this overall strip-like pattern and even some fine details over northern high latitudes (Fig. 12b). However, the simulated SO2 distribution does not correctly reproduce the peaks over the Sea of Okhotsk and the west coast of the Bering Sea. Several days later, the retrieved SO2 over the west coast of the Bering Sea gradually spreads out, and its vertical column density gradually attenuates (not shown). In contrast, the two SO2 peaks over the Sea of Okhotsk retained their compact structures and relatively high vertical column density. From mid-July to late July, the two peaks over the Sea of Okhotsk eventually comprise the main parts of the remaining SO2 of the Raikoke eruption and developed into two compact SO2 clouds. As the simulated SO2 did not reproduce the two peaks over the Sea of Okhotsk, however, the forward simulation lost its ability to capture the retrieved SO2 distribution during the first week of July.
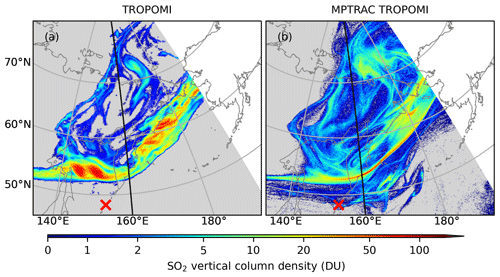
Figure 12Spatial distribution of SO2 vertical column density (DU) from the TROPOMI orbits that start at 1 July 2019, 00:15 UTC, and end at 1 July 2019, 03:38 UTC (a), and the corresponding distribution in the forward simulation, which was initialized by TROPOMI data (b). Note that data for the region in the east and west of 160∘ E are from two neighboring orbits.
3.2.3 Assessment of forward simulations by means of the Critical Success Index
We performed analyses of the Critical Success Index (CSI) to evaluate the forward simulations at five different detection thresholds ranging from 0.3 to 50.0 DU (Fig. 13). Figure 13a–c show the CSI, POD, and FAR time series for the forward simulation initialized with the TROPOMI SO2 product. The reference for calculating the CSI, POD, and FAR is also the TROPOMI retrievals to keep consistency of data between retrievals and simulations. The smallest threshold considered here represents the lower detection limit of the TROPOMI retrievals, which means that a threshold of 0.3 DU includes all available TROPOMI retrievals of the Raikoke event in the Northern Hemisphere. For the detection threshold of 0.3 DU, the forward simulation produced a very high POD, being around 80 % or larger. Such a high POD suggests that the overall spatial extent of the SO2 distribution is reproduced well by the forward simulation. However, the FAR shows a significantly increasing trend towards the end of the simulation, which suggests that the forward simulation transported some SO2 outside of the retrieved SO2 clouds. Due to the increasing trend of the FAR, the CSI values peak at the beginning of the simulation with a maximum value of 77 % and gradually decrease to ∼20 % after 10 d. Compared with CSI analyses in previous studies on other volcanic eruption events (Heng et al., 2016; Hoffmann et al., 2016), in which the CSI decreased to below 10 % after 10 d of forward simulations, our study for the Raikoke eruption shows improved performance of the model, meteorological input data, and satellite retrievals.
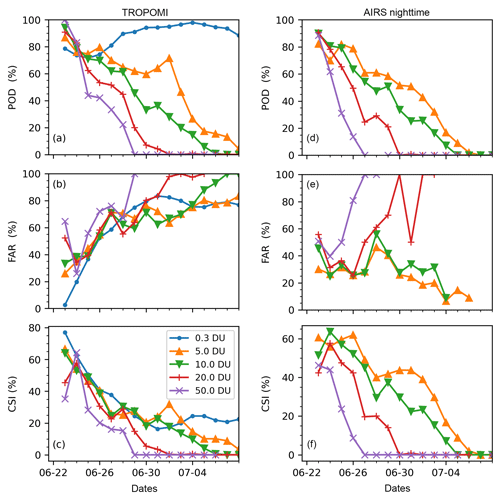
Figure 13Left panels (a–c): time series of the probability of detection (POD; a), false alarm rate (FAR; b), and critical success index (CSI; c) for a forward simulation initialized by the reconstructed injection parameter based on TROPOMI data. The TROPOMI retrievals are used as reference observations. Subplots in the right panels (d–f) are the same as the left panels but for AIRS nighttime data, and the reference observations are also from the AIRS nighttime data. Color coding indicates the column density threshold used to detect events (see plot key).
When increasing the detection threshold, however, the model performance decreases. For instance, the POD shows a clear decreasing trend when the detection threshold is increased from 0.3 to 50 DU (Fig. 13a). The differences of the FAR between the different detection thresholds are smaller (Fig. 13b). This result suggests that although the forward simulation reproduced the overall spatial extent of the SO2 clouds well, it has less ability to reproduce the internal structure and the location of the maxima of the SO2 clouds. For example, the POD at all SO2 thresholds except for the 0.3 DU level decreased notably during the first week of July (Fig. 13a), agreeing with our earlier findings that the forward simulation has lost the ability to capture structures of the SO2 clouds at this time.
For comparison, we also assessed the performance of the forward simulation initialized by the AIRS nighttime SO2 product with reference to the AIRS nighttime SO2 product (Fig. 13d–f). As the AIRS retrievals have a higher background level (about 5 DU), the lowest detection threshold of 0.3 DU to assess the CSI is not very meaningful in this case. The trend and magnitude of the POD from AIRS retrievals are very similar to the simulation with TROPOMI retrievals, but the FAR has lower values of 20 %–40 %. During the first 10 d of the simulation, the CSI values for a detection threshold of 5.0 DU are between 40–80 %, which is about 1.5 times higher than for the simulation with TROPOMI retrievals.
To make a comparison between forward simulations with different initializations, we used the TROPOMI retrievals as a common reference, and the detection threshold was set to 5.0 DU. Figure 14 shows the POD, FAR, and CSI time series of forward simulations initialized with the TROPOMI SO2 product, the AIRS nighttime SO2 product, a constant injection rate, and the VolRes profile. The POD, FAR, and CSI are very consistent between forward simulations initialized with TROPOMI and AIRS nighttime retrievals. Compared with the simulation with a constant injection rate, the POD is constantly higher for simulations initialized by satellite retrievals. However, the simulations initialized with the satellite retrievals suffer from higher FAR between 29 June and 4 July. The simulation using the VolRes profile yields a similar POD level and trend to the simulation initialized by a constant injection rate. In summary, the overall trends of POD, FAR, and CSI are generally similar between forward simulations with different initialization settings. This result indicates that the quality of the forward simulations is less affected by the injection parameters as estimated by the backward trajectory method, probably due to the fact that the major SO2 injection occurred during a small time window at the beginning of the eruption in this case.
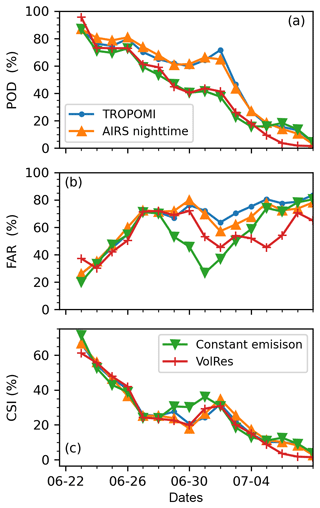
Figure 14Time series of POD (a), FAR (b), and CSI (c) for forward simulations initialized by a constant injection rate (green) and the VolRes profile (red), as well as injection parameters reconstructed using TROPOMI (blue) and AIRS nighttime data (orange), respectively. The reference observations are TROPOMI retrievals. The column density threshold used to detect events is 5.0 DU.
3.2.4 Simulation of compact SO2 clouds from late July to early August
From early July, the injected SO2 from the Raikoke eruption has gradually faded away, and two compact SO2 clouds became the major parts of the remaining SO2. Figure 15 shows the location and distribution of the SO2 clouds from 8 July to 9 August. During this period, the SO2 is mainly concentrated in two compact clouds with a size of the magnitude of several hundred kilometers. On 8 July, the two compact SO2 clouds are located close to each other, but eventually one of them moved toward the Asian continent (AC), and the other one moved toward North America (NA). In the following we refer to the SO2 cloud that moved toward the Asian continent as the AC SO2 cloud and the one toward North America as the NA SO2 cloud.
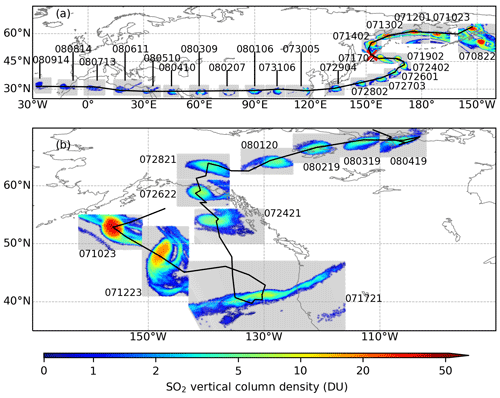
Figure 15Composite maps of the compact SO2 clouds moving toward the Asian continent (a) and North America (b), respectively. The time of observation for each patch is indicated as text in the form of MMDDHH (month–day–hour) of the year 2019.
The AC SO2 cloud first moved westward toward the Raikoke volcano and moved over the volcano on 15 July. After that, the AC SO2 cloud moved eastward, and from 24 July it moved southwestward. After it reached 30∘ N on 29 July, it stayed at this latitude and moved westward. During the whole period between late July and early August, the AC SO2 cloud remained confined in a compact structure. The AC SO2 cloud with a confined structure remains detectable in satellite retrievals until late August and September 2019 (Chouza et al., 2020; Gorkavyi et al., 2021).
The unique structure of the AC SO2 cloud motivated us to test the ability of the Lagrangian model in simulating the transport and dispersion of the SO2 cloud, especially the parameterization of the dispersion processes. In each orbit where TROPOMI detected the AC SO2 cloud, the SO2 detections were resampled to a number of 10 000 air parcels with equal mass to represent the SO2 cloud. The number of air parcels was scaled proportional to the SO2 vertical column density. The altitude for all the satellite retrievals is set to the altitude retrieved by the Cloud-Aerosol Lidar with Orthogonal Polarization (CALIOP) instrument (Gorkavyi et al., 2021). Note that CALIOP measures aerosol particles, which may introduce uncertainties regarding the altitude of gas phase SO2. To reduce uncertainties associated with the vertical spread of the SO2 in the simulations, the altitude of the air parcels corresponding to the same TROPOMI orbit was set to a constant value; i.e., the SO2 was restricted to occur at the same altitude, and no vertical spread was introduced during resampling. During 17 to 21 July 2019, the aerosol altitude is ∼18 km, and it rises to ∼20 km during 24–27 July, after which it gradually rises to 24 km around 14 August 2019 (Gorkavyi et al., 2021). We used the resampled air parcels for TROPOMI retrievals during 17 July 2019 (Fig. 15) to initialize the forward simulation. Medians of the locations (longitudes and latitudes) of the air parcels are used to represent the location of the SO2 cloud. The median absolute deviation (MAD) is used to measure the degree of dispersion of the SO2 cloud.
Qualitatively, we compared the simulated AC SO2 cloud with satellite retrievals at 1, 3, and 5 d after the initial release of the air parcels (Fig. 16). Dispersion in the simulations is in default settings, but different vertical motion schemes driven by vertical velocity (kinematic) and potential temperature (isentropic) are compared. As already shown in Fig. 15, the spatial extent of the AC SO2 cloud is restricted in a limited bubble-like area during these times. One day after the start of the forward simulations, the model still captures the spatial distribution of the AC SO2 cloud well (Fig. 16a and b). Despite the mean location being still captured by the model, the simulated SO2 cloud is already too dispersive after 3 d. Although dispersion in both simulations is too strong, the simulation with constant isentropic vertical motions shows relatively weaker dispersion. In addition to dispersion, the simulated SO2 cloud also shows some stretching effects along the west–southwest and east–northeast direction (Fig. 16g and h). Besides the horizontal location, however, both simulations driven by vertical velocity and constant potential temperature cannot correctly simulate the rising rate or the altitude of the SO2 cloud (not shown). Although manual corrections to the altitude position of the SO2 cloud have been performed in a previous study (Gorkavyi et al., 2021), in our study the vertical motion is freely driven by vertical velocity or remained confined to a constant potential temperature level.
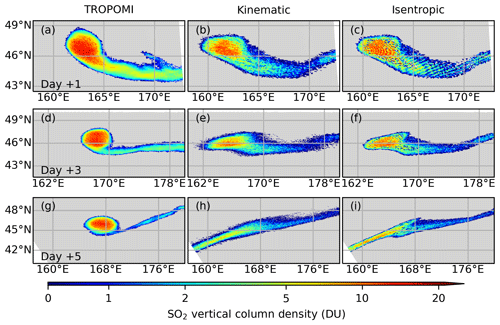
Figure 16Forward simulation of the dispersion of the AC SO2 cloud. Top row (a–c): spatial distribution of SO2 vertical column density from TROPOMI at 18 July 2019, 02:33 UTC (a), and the corresponding distribution in forward simulations, in which vertical motion was driven by vertical velocity (b) or by constant potential temperature (c), respectively. Middle and bottom rows are the same as the top row but for 20 July 2019, 01:55 UTC, and 22 July 2019, 01:18 UTC, respectively.
The simulation of the dispersion of the AC SO2 cloud was further evaluated by analyzing the MAD in longitude and latitude. Evaluation of the dispersion in the vertical direction is not available as the vertical spread of SO2 is not fully observed. We designed separate experiments to test the role of subgrid-scale variance and the role of diffusivity. When testing the subgrid-scale variance, the diffusivities were set to zero and vice versa when testing the subgrid-scale variance. Figure 17 shows the mean trajectories and the degree of dispersion of the AC SO2 cloud in satellite retrievals and forward simulations using different parameterizations of the subgrid-scale variance (the parameterizations on the horizontal and vertical directions are changed simultaneously). In general, all the forward simulations reproduce the trajectory of the retrieved AC SO2 cloud (Fig. 17a). The MAD of the AC SO2 in the TROPOMI SO2 product is ∼1∘ in the longitude dimension and ∼0.6∘ in the latitude dimension. A common problem in the simulations is that the simulated dispersion is much stronger than the dispersion in satellite observations, even when the dispersion parameters were set to zero, and just the initial horizontal spread of the cloud was taken into account. In the longitude dimension, the MAD in simulations is roughly 1 order of magnitude larger than the MAD in the TROPOMI data after 5 d of simulation time. In contrast, in the latitude dimension, the MAD in the simulation is similar to the MAD in the TROPOMI data during the first ∼10 d of the simulation. After 10 d the MAD increased substantially in the simulations but not in the TROPOMI data. Still, the differences of the MAD in latitude between satellite retrievals and simulations are much smaller than the MAD differences in longitude. This result again suggests a stretching effect due to horizontal wind shear along the longitude direction beside the stochastic effects of dispersion.
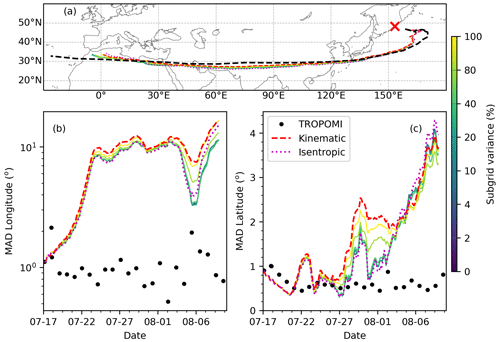
Figure 17Trajectories (a) and degree of dispersion (b) in the longitude dimension and (c) in the latitude dimension of the AC SO2 cloud in simulations driven by vertical velocity but with different subgrid-scale diffusion settings (see color bar). Results from TROPOMI data are shown in black. Results from the simulations (diffusion module is set to default in both simulations) with vertical motion driven by vertical velocity and potential temperature are shown in red and magenta, respectively.
We also tested the role of diffusivity ranging from 10−2 to 103 times of the default diffusivity values (see Sect. 2.3 for reference). Similar to the results in Fig. 17, the simulated dispersion is too strong, but the simulation is more sensitive to diffusivity than subgrid-scale variance (Fig. 18). When the diffusivity is on the order of ≥10 times of the default diffusivity values, the simulation also does not capture the trajectory of the AC SO2 cloud anymore.
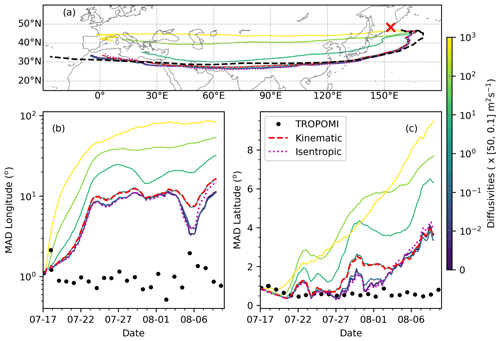
Figure 18Same as Fig. 17 but for simulations driven by different diffusivity settings (see color bar).
We performed forward trajectory simulations with vertical motion driven by constant potential temperature to avoid potential vertical velocity fluctuations due to data assimilation. The degree of dispersion shows less sensitivity to the dispersion parameters, and simulated MAD values for longitude and latitude are very close to the results from the default setting (Fig. 17). As the vertical position is adjusted at every time step to retain a constant potential temperature, the dispersion in the vertical direction is suppressed in the isentropic mode. Further, as the AC SO2 cloud is located in the stratosphere, the turbulent diffusion is driven by vertical diffusivity, which is also switched off in the isentropic mode. Therefore, dispersion in the isentropic trajectory simulation is less sensitive to the choice of dispersion parameters and is weaker than the dispersion in kinematic mode. Taken together, our tests on the dispersion parameters suggest that the reason why the simulated SO2 cloud generally is too dispersive is not only due to too strong diffusion in the model, but the stretching effect associated with horizontal wind shear also seems to play an important role.
The injection parameters of a volcanic eruption, i.e., the time, altitude, and rate of SO2 injections, have fundamental impacts on transport and dispersion simulations of volcanic ash and trace gases. Our study used two independent SO2 satellite data products (from AIRS and TROPOMI, respectively) and a backward trajectory method implemented with the MPTRAC Lagrangian transport model to estimate the injection parameters of the 2019 Raikoke eruption. The reconstructed injection parameters generally agree with each other when different satellite datasets and meteorological reanalyses were used, indicating the robustness of our approach.
Our reconstruction shows that the SO2 from the Raikoke eruption was mainly injected between 4 and 16 km of altitude, which falls within the range of previous studies (Hedelt et al., 2019; Muser et al., 2020; de Leeuw et al., 2021; Horváth et al., 2021). Similar to de Leeuw et al. (2021), our reconstruction also shows enhanced SO2 concentrations at 12–14 km of altitude. Besides the overall agreement with the injection parameters used in this previous study, our reconstruction differs in some aspects. First, we estimated a total SO2 injection of 2.1 ± 0.2 Tg, which is larger than earlier estimates of 1.5 ± 0.2 Tg (Global Volcanism Program, 2019; Muser et al., 2020; de Leeuw et al., 2021). Compared with the VolRes team estimation, de Leeuw et al. (2021) also argued that 1.5 Tg would underestimate the SO2 mass in their forward simulation with the NAME model, and they suggested that either more SO2 should be injected into the stratosphere (1.09 Tg), or a total of 2.0 Tg would be needed to match the TROPOMI retrievals on timescales >1 week. The stratospheric injection in our reconstruction is 0.85 ± 0.08 Tg, which is lower than the mass used by de Leeuw et al. (2021) but is significantly larger than the 0.64 Tg injection into the stratosphere in the VolRes profile.
Secondly, our reconstruction shows continuous but weak SO2 injections after the first major injection on 21–22 June 2019. The major eruption during 21–22 June injects 1.5–1.6 Tg of SO2, and a remaining fraction of 0.5–0.6 Tg of SO2 was injected during 23–30 June, mainly into the troposphere. Hedelt et al. (2019) also suggested minor injections on and after 23 June. A direct validation of injections after 22 June is difficult, as the injection rates are rather low. We inspected Vis and IR images from Himawari 8 (not shown) and found that some volcanic plumes are visible over the Raikoke during 24–25 June, corresponding to the second plume in our reconstruction. However, due to high-altitude clouds, it is hard to validate or rule out the possibility of a third plume. From our forward simulations, the second and third plume are potentially overestimated. In the current setting, the backward trajectory method would only pick the first hit to identify a new source, and the second overpass will be not counted anymore. In reality, however, the SO2 may have passed the volcano multiple times, which may lead to an overestimation for the second and the third plume. However, totally excluding either of these injections would cause other problems in the simulations, in particular in simulating the SO2 total mass. A future study, based on more sophisticated inverse modeling techniques (Heng et al., 2016), might yield an improved injection reconstruction.
Forward simulations using our reconstructed injection parameters compare well with the TROPOMI SO2 retrievals during the first 10 d after the eruption in terms of location and spatial extent. Similar to the simulation using NAME (de Leeuw et al., 2021), our simulation also shows limited skills in capturing the structures inside the SO2 clouds at a later stage. de Leeuw et al. (2021) argued that the limited ability to capture the internal structure of the SO2 clouds is because the diffusion in the model is too strong. Hence, we explored the influence of the diffusion parameterization on the simulation of the compact SO2 cloud from late July to early August, as in Gorkavyi et al. (2021). Although the simulation skill was improved when reducing the strength of the simulated diffusion, the simulation was still too diffusive. As the simulations were still too diffusive when diffusion was switched off or when isentropic vertical motion was enforced to avoid jumps in the vertical velocity due to data assimilation (Stohl et al., 2005), we conclude that the strong dispersion is due to the meteorological input data itself. In particular, the stretching of the simulated SO2 cloud (Fig. 16) and much stronger dispersion in the longitude direction (Figs. 17 and 18) suggest that the spread of the simulated SO2 cloud is likely caused by horizontal wind shear in the ERA5 data. We did a similar set of experiments using ERA-Interim data, leading to the same results and conclusions.
Besides the above limitations, the current reconstruction and in turn the forward simulations may also be influenced by the selection of the TROPOMI products, i.e. the altitude of assumed SO2 layer during retrieval, and by the lofting of the plume due to the co-existence of ash. TROPOMI SO2 products are available for different scenarios that assume the SO2 is at either 1, 7, or 15 km above sea level. The main difference between different products is the absolute value of the vertical column density, and it has a minor influence on the reconstruction of the relative injection rate. However, the different SO2 can result in a different mass estimate. Comparison of the total SO2 mass between the 7 and 15 km retrieval products for TROPOMI shows that the mass is identical during the first week of the eruption. After the first week, the mass derived from the 7 km product is consistently higher than the 15 km product by ∼10 %. Therefore, using the 7 km product would get an higher estimate of the total SO2 mass, which is at the upper limit of the estimate reported in this study. On the other hand, Muser et al. (2020) reported a lofting effect of ash for the Raikoke plume during the initial days after the eruption. The lofting effect may also exist during the period of the compact SO2 (Gorkavyi et al., 2021). Such a lofting effect would directly influence the forward simulation as it is not reflected in the meteorological data (Sect. 3.2.4) and may need manual tuning to correctly simulate the long-range transport of the SO2. As the vertical column density was used in the reconstruction, and it does not contain vertical information, the lofting effect may have less influence on the reconstruction. A quantitative assessment of the impact of the lofting effect is however unavailable from the current study, and it should be considered in a future study.
Our study estimated the overall SO2 e-folding lifetime during the first 3 weeks after the Raikoke eruption to be 13–17 d. This finding was consistent between using a simple exponential decay of the reconstructed SO2 injections and simulating chemical loss of SO2 due to reaction with hydroxyl. The SO2 mass burden, derived from both the exponential decay experiment and the hydroxyl module of MPTRAC, matches well with the TROPOMI retrievals. Our estimation also agrees with earlier studies. de Leeuw et al. (2021) estimated that the e-folding lifetime after 27 June is 14–15 d. Based on Ozone Mapping and Profiler Suite (OMPS) retrievals, Gorkavyi et al. (2021) estimated that the e-folding lifetime during the first 20 d after the eruption is 18.9 d, which is slightly larger than the e-folding lifetime in our study and in de Leeuw et al. (2021). We note that, however, the e-folding lifetime has a strong dependence on the altitude of the SO2 layer (Fig. 10), emphasizing that correctly determining the vertical profile of the injection rates is essential to reproduce the retrieved SO2 mass change.
Determining the injection parameters of volcanic eruptions, including the plume altitude, time, and injection rate, is essential for accurately simulating the dispersion of volcanic trace gases and aerosols. We used the MPTRAC model as well as AIRS and TROPOMI satellite retrievals to estimate the injection parameters of the 2019 Raikoke eruption. The altitude and time of the SO2 injection were estimated based on a backward trajectory method and the SO2 retrievals from the AIRS and TROPOMI satellites. Then, we used an exponential decay model to calibrate total injected SO2 mass with the SO2 mass from TROPOMI retrievals. The lifetime of SO2 was estimated to be 13–17 d. Our estimation of the SO2 mass change in the exponential experiments agrees well with the mass change in the forward simulation that is driven by chemical reactions. Both methods reproduced the mass change derived from TROPOMI retrievals. Therefore, our method is robust for estimating the whole set of injection parameters, i.e., the time, altitude, and injection rate, for SO2 injections.
Our estimated total SO2 mass for the 2019 Raikoke eruption is 2.1 ± 0.2 Tg, which is larger than the initial estimate of 1.5 ± 0.2 Tg from earlier studies; 40.5 % (0.85 Tg) of the total SO2 mass was injected into the lower stratosphere. We consider our new estimation of a larger amount of SO2 reasonable, as it better reproduces the satellite-retrieved mass change in the forward simulations than assuming an injection of 1.5 Tg SO2 either by a constant injection rate or following the VolRes profile (Fig. 9). The reconstructions of injection parameters are very consistent between using the TROPOMI daytime and AIRS nighttime products. Forward simulations driven by our reconstructed time- and height-resolved injection parameters compared with simulations driven by a simple constant injection rate, an approach that is common in global chemistry climate simulations, show better performance of reproducing the satellite retrievals, especially in terms of spatial extent and location. The findings from this study will help us to create a long-term volcanic SO2 injection inventory from AIRS, which we hope might be useful to improve chemistry climate simulations considering the effects of volcanic SO2 in future work.
The MPTRAC model (Hoffmann et al., 2016, https://doi.org/https://doi.org/10.1002/2015JD023749; Hoffmann et al., 2022, https://doi.org/10.5194/gmd-15-2731-2022) is available under the terms and conditions of the GNU General Public License, Version 3, via the repository at https://github.com/slcs-jsc/mptrac (last access: 10 January 2022) and has been archived on Zenodo (https://doi.org/10.5281/zenodo.5714528; Hoffmann et al., 2021). The TROPOMI SO2 product data were obtained from the Copernicus Open Data Hub at https://s5phub.copernicus.eu/ (last access: 10 January 2022; Copernicus, 2022). The AIRS SO2 data product (Hoffmann et al., 2014) (https://doi.org/10.1117/12.2066326) used in this study was derived from the AIRS Level-1B data obtained from NASA at https://doi.org/10.5067/YZEXEVN4JGGJ (AIRS project, 2007) and is publicly available at https://datapub.fz-juelich.de/slcs/airs/volcanoes/ (last access: 10 January 2022 or via https://doi.org/10.26165/JUELICH-DATA/VPHA3R; Hoffmann, 2021). The ERA5 and ERA-Interim reanalysis data were obtained from ECMWF's Meteorological Archival and Retrieval System (MARS). The ERA5 tropopause data (Hoffmann and Spang, 2022) (https://doi.org/10.5194/acp-22-4019-2022) are accessible at https://datapub.fz-juelich.de/slcs/tropopause/ (last access: 10 January 2022) or via https://doi.org/10.26165/JUELICH-DATA/UBNGI2 (Hoffmann and Spang, 2021).
ZC, SG, and LH jointly developed the concept of this study. ZC performed the simulations and analyzed the data and results. SG prepared the TROPOMI data. LH provided the AIRS SO2 data product and extended the MPTRAC Lagrangian transport model for application in this study. ZC wrote the manuscript with contributions from all co-authors.
The authors declare that no competing interests are present.
Publisher’s note: Copernicus Publications remains neutral with regard to jurisdictional claims in published maps and institutional affiliations.
This article is part of the special issue “Satellite observations, in situ measurements and model simulations of the 2019 Raikoke eruption (ACP/AMT/GMD inter-journal SI)”. It is not associated with a conference.
We acknowledge the Jülich Supercomputing Centre for providing computing time and storage resources on the supercomputer JUWELS.
This research has been supported by the Yunnan Provincial Science and Technology Department, Applied Basic Research Foundation of Yunnan Province (grant no. 202001BB050066), the International Postdoctoral Exchange Fellowship Program (grant no. 20191038), the China Postdoctoral Science Foundation (grant no. 2019M653505), and the Deutsche Forschungsgemeinschaft (DFG; grant no. HO5102/1-1).
The article processing charges for this open-access publication were covered by the Forschungszentrum Jülich.
This paper was edited by Farahnaz Khosrawi and reviewed by two anonymous referees.
AIRS project: AIRS/Aqua L1B Infrared (IR) geolocated and calibrated radiances V005, Goddard Earth Sciences Data and Information Services Center (GES DISC) [data set], https://doi.org/10.5067/YZEXEVN4JGGJ, 2007. a
Aumann, H., Chahine, M., Gautier, C., Goldberg, M., Kalnay, E., McMillin, L., Revercomb, H., Rosenkranz, P., Smith, W., Staelin, D., Strow, L., and Susskind, J.: AIRS/AMSU/HSB on the Aqua mission: design, science objectives, data products, and processing systems, IEEE Trans. Geosci. Remote, 41, 253–264, https://doi.org/10.1109/TGRS.2002.808356, 2003. a
Carn, S. A., Strow, L. L., de Souza-Machado, S., Edmonds, Y., and Hannon, S.: Quantifying tropospheric volcanic emissions with AIRS: The 2002 eruption of Mt. Etna (Italy), Geophys. Res. Lett., 32, L02301, https://doi.org/10.1029/2004GL021034, 2005. a
Chahine, M. T., Pagano, T. S., Aumann, H. H., Atlas, R., Barnet, C., Blaisdell, J., Chen, L., Divakarla, M., Fetzer, E. J., Goldberg, M., Gautier, C., Granger, S., Hannon, S., Irion, F. W., Kakar, R., Kalnay, E., Lambrigtsen, B. H., Lee, S.-Y., Marshall, J. L., Mcmillan, W. W., Mcmillin, L., Olsen, E. T., Revercomb, H., Rosenkranz, P., Smith, W. L., Staelin, D., Strow, L. L., Susskind, J., Tobin, D., Wolf, W., and Zhou, L.: AIRS: Improving Weather Forecasting and Providing New Data on Greenhouse Gases, B. Am. Meteorol. Soc., 87, 911–926, https://doi.org/10.1175/BAMS-87-7-911, 2006. a
Chouza, F., Leblanc, T., Barnes, J., Brewer, M., Wang, P., and Koon, D.: Long-term (1999–2019) variability of stratospheric aerosol over Mauna Loa, Hawaii, as seen by two co-located lidars and satellite measurements, Atmos. Chem. Phys., 20, 6821–6839, https://doi.org/10.5194/acp-20-6821-2020, 2020. a, b
Copernicus: Sentinel-5P Pro-Operations Data Hub, Copernicus, https://s5phub.copernicus.eu, last access: 10 January 2022. a
de Leeuw, J., Schmidt, A., Witham, C. S., Theys, N., Taylor, I. A., Grainger, R. G., Pope, R. J., Haywood, J., Osborne, M., and Kristiansen, N. I.: The 2019 Raikoke volcanic eruption – Part 1: Dispersion model simulations and satellite retrievals of volcanic sulfur dioxide, Atmos. Chem. Phys., 21, 10851–10879, https://doi.org/10.5194/acp-21-10851-2021, 2021. a, b, c, d, e, f, g, h, i, j, k, l, m, n, o, p, q
Dee, D. P., Uppala, S. M., Simmons, A. J., Berrisford, P., Poli, P., Kobayashi, S., Andrae, U., Balmaseda, M. A., Balsamo, G., Bauer, P., Bechtold, P., Beljaars, A. C. M., van de Berg, L., Bidlot, J., Bormann, N., Delsol, C., Dragani, R., Fuentes, M., Geer, A. J., Haimberger, L., Healy, S. B., Hersbach, H., Hólm, E. V., Isaksen, L., Kållberg, P., Köhler, M., Matricardi, M., McNally, A. P., Monge-Sanz, B. M., Morcrette, J.-J., Park, B.-K., Peubey, C., de Rosnay, P., Tavolato, C., Thépaut, J.-N., and Vitart, F.: The ERA-Interim reanalysis: configuration and performance of the data assimilation system, Q. J. Roy. Meteor. Soc., 137, 553–597, https://doi.org/10.1002/qj.828, 2011. a
Desiato, F., Anfossi, D., Castelli, S., Ferrero, E., and Tinarelli, G.: The role of wind field, mixing height and horizontal diffusivity investigated through two lagrangian particle models, Atmos. Environ., 32, 4157–4165, https://doi.org/10.1016/s1352-2310(98)00195-2, 1998. a
Draxler, R. R. and Hess, G.: An overview of the HYSPLIT_4 modelling system for trajectories, dispersion, and deposition, Aust. Meteorol. Mag., 47, 295–308, 1998. a
Eckhardt, S., Prata, A. J., Seibert, P., Stebel, K., and Stohl, A.: Estimation of the vertical profile of sulfur dioxide injection into the atmosphere by a volcanic eruption using satellite column measurements and inverse transport modeling, Atmos. Chem. Phys., 8, 3881–3897, https://doi.org/10.5194/acp-8-3881-2008, 2008. a
Flemming, J. and Inness, A.: Volcanic sulfur dioxide plume forecasts based on UV satellite retrievals for the 2011 Grímsvötn and the 2010 Eyjafjallajökull eruption, J. Geophys. Res.-Atmos., 118, 10172–10189, https://doi.org/10.1002/jgrd.50753, 2013. a
Fromm, M., Kablick III, G., Nedoluha, G., Carboni, E., Grainger, R., Campbell, J., and Lewis, J.: Correcting the record of volcanic stratospheric aerosol impact: Nabro and Sarychev Peak, J. Geophys. Res.-Atmos., 119, 10343–10364, https://doi.org/10.1002/2014jd021507, 2014. a, b
Global Volcanism Program: Report on Raikoke (Russia), in: Bulletin of the Global Volcanism Network, edited by Crafford, A. and Venzke, E., Smithsonian Institution, 44, https://doi.org/10.5479/si.gvp.bgvn201908-290250, 2019. a, b
Gorkavyi, N., Krotkov, N., Li, C., Lait, L., Colarco, P., Carn, S., DeLand, M., Newman, P., Schoeberl, M., Taha, G., Torres, O., Vasilkov, A., and Joiner, J.: Tracking aerosols and SO2 clouds from the Raikoke eruption: 3D view from satellite observations, Atmos. Meas. Tech., 14, 7545–7563, https://doi.org/10.5194/amt-14-7545-2021, 2021. a, b, c, d, e, f, g, h
Grégoire, B., Montero, X., Galetz, M. C., Bonnet, G., and Pedraza, F.: Mechanisms of hot corrosion of pure nickel at 700 ∘C: Influence of testing conditions, Corros. Sci., 141, 211–220, https://doi.org/10.1016/j.corsci.2018.06.009, 2018. a
Hedelt, P., Efremenko, D. S., Loyola, D. G., Spurr, R., and Clarisse, L.: Sulfur dioxide layer height retrieval from Sentinel-5 Precursor/TROPOMI using FP_ILM, Atmos. Meas. Tech., 12, 5503–5517, https://doi.org/10.5194/amt-12-5503-2019, 2019. a, b, c
Heng, Y., Hoffmann, L., Griessbach, S., Rößler, T., and Stein, O.: Inverse transport modeling of volcanic sulfur dioxide emissions using large-scale simulations, Geosci. Model Dev., 9, 1627–1645, https://doi.org/10.5194/gmd-9-1627-2016, 2016. a, b, c, d, e
Hersbach, H., Bell, B., Berrisford, P., Hirahara, S., Horányi, A., Muñoz-Sabater, J., Nicolas, J., Peubey, C., Radu, R., Schepers, D., Simmons, A., Soci, C., Abdalla, S., Abellan, X., Balsamo, G., Bechtold, P., Biavati, G., Bidlot, J., Bonavita, M., De Chiara, G., Dahlgren, P., Dee, D., Diamantakis, M., Dragani, R., Flemming, J., Forbes, R., Fuentes, M., Geer, A., Haimberger, L., Healy, S., Hogan, R. J., Hólm, E., Janisková, M., Keeley, S., Laloyaux, P., Lopez, P., Lupu, C., Radnoti, G., de Rosnay, P., Rozum, I., Vamborg, F., Villaume, S., and Thépaut, J.-N.: The ERA5 global reanalysis, Q. J. Roy. Meteor. Soc., 146, 1999–2049, https://doi.org/10.1002/qj.3803, 2020. a
Hoffmann, L.: AIRS/Aqua Observations of Volcanic Emissions, V1, Jülich DATA [data set], https://doi.org/10.26165/JUELICH-DATA/VPHA3R, 2021 (data available at: https://datapub.fz-juelich.de/slcs/airs/volcanoes/, last access: 10 January 2022). a
Hoffmann, L. and Spang, R.: Reanalysis Tropopause Data Repository, V1, Jülich DATA [data set], https://doi.org/10.26165/JUELICH-DATA/UBNGI2, 2021 (data available at: https://datapub.fz-juelich.de/slcs/tropopause/, last access: 10 January 2022). a
Hoffmann, L. and Spang, R.: An assessment of tropopause characteristics of the ERA5 and ERA-Interim meteorological reanalyses, Atmos. Chem. Phys., 22, 4019–4046, https://doi.org/10.5194/acp-22-4019-2022, 2022. a
Hoffmann, L., Griessbach, S., and Meyer, C. I.: Volcanic emissions from AIRS observations: detection methods, case study, and statistical analysis, in: Proc SPIE, edited by: Comerón, A., Kassianov, E. I., Schäfer, K., Picard, R. H., Stein, K., and Gonglewski, J. D., International Society for Optics and Photonics, SPIE, 9242, 305–312, https://doi.org/10.1117/12.2066326, 2014. a, b, c, d, e
Hoffmann, L., Clemens, J., Holke, J., Liu, M., and Mood, K. H.: slcs-jsc/mptrac: v2.2, Zenodo [code], https://doi.org/10.5281/zenodo.5714528, 2021 (data available at: https://github.com/slcs-jsc/mptrac, last access: 10 January 2022). a
Hoffmann, L., Rößler, T., Griessbach, S., Heng, Y., and Stein, O.: Lagrangian transport simulations of volcanic sulfur dioxide emissions: Impact of meteorological data products, J. Geophys. Res., 121, 4651–4673, https://doi.org/10.1002/2015JD023749, 2016. a, b, c, d, e, f, g
Hoffmann, L., Hertzog, A., Rößler, T., Stein, O., and Wu, X.: Intercomparison of meteorological analyses and trajectories in the Antarctic lower stratosphere with Concordiasi superpressure balloon observations, Atmos. Chem. Phys., 17, 8045–8061, https://doi.org/10.5194/acp-17-8045-2017, 2017. a
Hoffmann, L., Günther, G., Li, D., Stein, O., Wu, X., Griessbach, S., Heng, Y., Konopka, P., Müller, R., Vogel, B., and Wright, J. S.: From ERA-Interim to ERA5: the considerable impact of ECMWF's next-generation reanalysis on Lagrangian transport simulations, Atmos. Chem. Phys., 19, 3097–3124, https://doi.org/10.5194/acp-19-3097-2019, 2019. a
Hoffmann, L., Baumeister, P. F., Cai, Z., Clemens, J., Griessbach, S., Günther, G., Heng, Y., Liu, M., Haghighi Mood, K., Stein, O., Thomas, N., Vogel, B., Wu, X., and Zou, L.: Massive-Parallel Trajectory Calculations version 2.2 (MPTRAC-2.2): Lagrangian transport simulations on graphics processing units (GPUs), Geosci. Model Dev., 15, 2731–2762, https://doi.org/10.5194/gmd-15-2731-2022, 2022. a, b, c
Horváth, Á., Girina, O. A., Carr, J. L., Wu, D. L., Bril, A. A., Mazurov, A. A., Melnikov, D. V., Hoshyaripour, G. A., and Buehler, S. A.: Geometric estimation of volcanic eruption column height from GOES-R near-limb imagery – Part 2: Case studies, Atmos. Chem. Phys., 21, 12207–12226, https://doi.org/10.5194/acp-21-12207-2021, 2021. a, b
Ishikawa, H.: Evaluation of the Effect of Horizontal Diffusion on the Long-Range Atmospheric Transport Simulation with Chernobyl Data, J. Appl. Meteorol., 34, 1653–1665, https://doi.org/10.1175/1520-0450-34.7.1653, 1995. a
Jones, A., Thomson, D., Hort, M., and Devenish, B.: The U.K. Met Office's Next-Generation Atmospheric Dispersion Model, NAME III, in: Air Pollution Modeling and Its Application XVII, edited by: Borrego, C. and Norman, A.-L., Springer US, Boston, MA, 580–589, https://doi.org/10.1007/978-0-387-68854-1_62, 2007. a
Kloss, C., Berthet, G., Sellitto, P., Ploeger, F., Taha, G., Tidiga, M., Eremenko, M., Bossolasco, A., Jégou, F., Renard, J.-B., and Legras, B.: Stratospheric aerosol layer perturbation caused by the 2019 Raikoke and Ulawun eruptions and their radiative forcing, Atmos. Chem. Phys., 21, 535–560, https://doi.org/10.5194/acp-21-535-2021, 2021. a
Kremser, S., Thomason, L. W., von Hobe, M., Hermann, M., Deshler, T., Timmreck, C., Toohey, M., Stenke, A., Schwarz, J. P., Weigel, R., Fueglistaler, S., Prata, F. J., Vernier, J.-P., Schlager, H., Barnes, J. E., Antuña-Marrero, J.-C., Fairlie, D., Palm, M., Mahieu, E., Notholt, J., Rex, M., Bingen, C., Vanhellemont, F., Bourassa, A., Plane, J. M. C., Klocke, D., Carn, S. A., Clarisse, L., Trickl, T., Neely, R., James, A. D., Rieger, L., Wilson, J. C., and Meland, B.: Stratospheric aerosol—Observations, processes, and impact on climate, Rev. Geophys., 54, 278–335, https://doi.org/10.1002/2015RG000511, 2016. a
Kristiansen, N. I., Stohl, A., Prata, A. J., Richter, A., Eckhardt, S., Seibert, P., Hoffmann, A., Ritter, C., Bitar, L., Duck, T. J., and Stebel, K.: Remote sensing and inverse transport modeling of the Kasatochi eruption sulfur dioxide cloud, J. Geophys. Res., 115, D00L16, https://doi.org/10.1029/2009JD013286, 2010. a
Legras, B., Pisso, I., Berthet, G., and Lefèvre, F.: Variability of the Lagrangian turbulent diffusion in the lower stratosphere, Atmos. Chem. Phys., 5, 1605–1622, https://doi.org/10.5194/acp-5-1605-2005, 2005. a
Lin, J., Gerbig, C., Wofsy, S., Andrews, A., Daube, B., Davis, K., and Grainger, C.: A near-field tool for simulating the upstream influence of atmospheric observations: The Stochastic Time-Inverted Lagrangian Transport (STILT) model, J. Geophys. Res., 108, 4493, https://doi.org/10.1029/2002JD003161, 2003. a
Lin, J., Brunner, D., Gerbig, C., Stohl, A., Luhar, A., and Webley, P. (Eds.): Lagrangian modeling of the atmosphere, American Geophysical Union, Washington DC, Geoph. Monog. Ser., 200, https://doi.org/10.1029/GM200, 2012. a
Muser, L. O., Hoshyaripour, G. A., Bruckert, J., Horváth, Á., Malinina, E., Wallis, S., Prata, F. J., Rozanov, A., von Savigny, C., Vogel, H., and Vogel, B.: Particle aging and aerosol–radiation interaction affect volcanic plume dispersion: evidence from the Raikoke 2019 eruption, Atmos. Chem. Phys., 20, 15015–15036, https://doi.org/10.5194/acp-20-15015-2020, 2020. a, b, c, d, e, f, g
Pisso, I., Real, E., Law, K. S., Legras, B., Bousserez, N., Attié, J. L., and Schlager, H.: Estimation of mixing in the troposphere from Lagrangian trace gas reconstructions during long-range pollution plume transport, J. Geophys. Res., 114, D19301, https://doi.org/10.1029/2008JD011289, 2009. a
Pommrich, R., Müller, R., Grooß, J.-U., Konopka, P., Ploeger, F., Vogel, B., Tao, M., Hoppe, C. M., Günther, G., Spelten, N., Hoffmann, L., Pumphrey, H.-C., Viciani, S., D'Amato, F., Volk, C. M., Hoor, P., Schlager, H., and Riese, M.: Tropical troposphere to stratosphere transport of carbon monoxide and long-lived trace species in the Chemical Lagrangian Model of the Stratosphere (CLaMS), Geosci. Model Dev., 7, 2895–2916, https://doi.org/10.5194/gmd-7-2895-2014, 2014. a
Prata, A. J.: Satellite detection of hazardous volcanic clouds and the risk to global air traffic, Nat. Hazards, 51, 303–324, https://doi.org/10.1007/s11069-008-9273-z, 2009. a
Prata, A. J. and Bernardo, C.: Retrieval of volcanic SO2 column abundance from Atmospheric Infrared Sounder data, J. Geophys. Res., 112, D20204, https://doi.org/10.1029/2006JD007955, 2007. a
Robock, A.: Volcanic eruptions and climate, Rev. Geophys., 38, 191–219, https://doi.org/10.1029/1998RG000054, 2000. a
Romahn, F., Pedergnana, M., Loyola, D., Apituley, A., Sneep, M., and Veefkind, J. P.: Sentinel-5 precursor/TROPOMI Level 2 Product User Manual Sulphur Dioxide SO2, Sentinel-5 precursor/TROPOMI, Tech. rep. number: S5P-L2-DLR-PUM-400E, 1–157, http://www.tropomi.eu/data-products/sulphur-dioxide, last access: 4 February 2021. a
Rößler, T., Stein, O., Heng, Y., Baumeister, P., and Hoffmann, L.: Trajectory errors of different numerical integration schemes diagnosed with the MPTRAC advection module driven by ECMWF operational analyses, Geosci. Model Dev., 11, 575–592, https://doi.org/10.5194/gmd-11-575-2018, 2018. a
Schmidt, A., Ostro, B., Carslaw, K. S., Wilson, M., Thordarson, T., Mann, G. W., and Simmons, A. J.: Excess mortality in Europe following a future Laki-style Icelandic eruption, P. Natl. Acad. Sci. USA, 108, 15710–15715, https://doi.org/10.1073/pnas.1108569108, 2011. a
Stohl, A., Forster, C., Frank, A., Seibert, P., and Wotawa, G.: Technical note: The Lagrangian particle dispersion model FLEXPART version 6.2, Atmos. Chem. Phys., 5, 2461–2474, https://doi.org/10.5194/acp-5-2461-2005, 2005. a, b, c, d
Theys, N., De Smedt, I., Yu, H., Danckaert, T., van Gent, J., Hörmann, C., Wagner, T., Hedelt, P., Bauer, H., Romahn, F., Pedergnana, M., Loyola, D., and Van Roozendael, M.: Sulfur dioxide retrievals from TROPOMI onboard Sentinel-5 Precursor: algorithm theoretical basis, Atmos. Meas. Tech., 10, 119–153, https://doi.org/10.5194/amt-10-119-2017, 2017. a, b
Theys, N., Hedelt, P., De Smedt, I., Lerot, C., Yu, H., Vlietinck, J., Pedergnana, M., Arellano, S., Galle, B., Fernandez, D., Carlito, C. J. M., Barrington, C., Taisne, B., Delgado-Granados, H., Loyola, D., and Van Roozendael, M.: Global monitoring of volcanic SO2 degassing with unprecedented resolution from TROPOMI onboard Sentinel-5 Precursor, Sci. Rep., 9, 2643, https://doi.org/10.1038/s41598-019-39279-y, 2019. a, b
Theys, N., De Smedt, I., Lerot, C., Yu, H., and Van Roozendael, M.: S5P ATBD of the Sulfur dioxide product, Sentinel-5 precursor/TROPOMI, Tech. rep. S5P-BIRA-L2-400E-ATBD, 1–68, http://www.tropomi.eu/data-products/sulphur-dioxide/, last access: 4 February 2021. a, b
Veefkind, J. P., Aben, I., McMullan, K., Förster, H., de Vries, J., Otter, G., Claas, J., Eskes, H. J., de Haan, J. F., Kleipool, Q., van Weele, M., Hasekamp, O., Hoogeveen, R., Landgraf, J., Snel, R., Tol, P., Ingmann, P., Voors, R., Kruizinga, B., Vink, R., Visser, H., and Levelt, P. F.: TROPOMI on the ESA Sentinel-5 Precursor: A GMES mission for global observations of the atmospheric composition for climate, air quality and ozone layer applications, Remote Sens. Environ., 120, 70–83, https://doi.org/10.1016/j.rse.2011.09.027, 2012. a, b, c, d
Wernli, H. and Davies, H. C.: A Lagrangian-based analysis of extratropical cyclones I: The method and some applications, Q. J. Roy. Meteor. Soc., 123, 467–489, https://doi.org/10.1002/qj.49712353811, 1997. a
Whitty, R. C. W., Ilyinskaya, E., Mason, E., Wieser, P. E., Liu, E. J., Schmidt, A., Roberts, T., Pfeffer, M. A., Brooks, B., Mather, T. A., Edmonds, M., Elias, T., Schneider, D. J., Oppenheimer, C., Dybwad, A., Nadeau, P. A., and Kern, C.: Spatial and Temporal Variations in SO2 and PM2.5 Levels Around Kīlauea Volcano, Hawai'i During 2007–2018, Front. Earth Sci., 8, 36, https://doi.org/10.3389/feart.2020.00036, 2020. a
Wilks, D. S.: Statistical methods in the atmospheric sciences, Academic Press, vol. 100, ISBN: 9780123850225, 2011. a
WMO: Meteorology A Three-Dimensional Science: Second Session of the Commission for Aerology, WMO Bull., iv, 134–138, 1957. a, b
Wu, X., Griessbach, S., and Hoffmann, L.: Equatorward dispersion of a high-latitude volcanic plume and its relation to the Asian summer monsoon: a case study of the Sarychev eruption in 2009, Atmos. Chem. Phys., 17, 13439–13455, https://doi.org/10.5194/acp-17-13439-2017, 2017. a, b, c
Wu, X., Griessbach, S., and Hoffmann, L.: Long-range transport of volcanic aerosol from the 2010 Merapi tropical eruption to Antarctica, Atmos. Chem. Phys., 18, 15859–15877, https://doi.org/10.5194/acp-18-15859-2018, 2018. a, b, c
Yang, K., Liu, X., Bhartia, P. K., Krotkov, N. A., Carn, S. A., Hughes, E. J., Krueger, A. J., Spurr, R. J. D., and Trahan, S. G.: Direct retrieval of sulfur dioxide amount and altitude from spaceborne hyperspectral UV measurements: Theory and application, J. Geophys. Res., 115, D00L09, https://doi.org/10.1029/2010JD013982, 2010. a