the Creative Commons Attribution 4.0 License.
the Creative Commons Attribution 4.0 License.
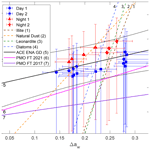
Micro-spectroscopic and freezing characterization of ice-nucleating particles collected in the marine boundary layer in the eastern North Atlantic
Daniel A. Knopf
Joseph C. Charnawskas
Peiwen Wang
Benny Wong
Jay M. Tomlin
Kevin A. Jankowski
Matthew Fraund
Daniel P. Veghte
Swarup China
Alexander Laskin
Ryan C. Moffet
Mary K. Gilles
Josephine Y. Aller
Matthew A. Marcus
Shira Raveh-Rubin
Jian Wang
Formation of atmospheric ice plays a crucial role in the microphysical evolution of mixed-phase and cirrus clouds and thus climate. How aerosol particles impact ice crystal formation by acting as ice-nucleating particles (INPs) is a subject of intense research activities. To improve understanding of atmospheric INPs, we examined daytime and nighttime particles collected during the Aerosol and Cloud Experiments in the Eastern North Atlantic (ACE-ENA) field campaign conducted in summer 2017. Collected particles, representative of a remote marine environment, were investigated for their propensity to serve as INPs in the immersion freezing (IMF) and deposition ice nucleation (DIN) modes. The particle population was characterized by chemical imaging techniques such as computer-controlled scanning electron microscopy with energy-dispersive X-ray analysis (CCSEM/EDX) and scanning transmission X-ray microscopy with near-edge X-ray absorption fine-structure spectroscopy (STXM/NEXAFS). Four major particle-type classes were identified where internally mixed inorganic–organic particles make up the majority of the analyzed particles. Following ice nucleation experiments, individual INPs were identified and characterized by SEM/EDX. The identified INP types belong to the major particle-type classes consisting of fresh sea salt with organics or processed sea salt containing dust and sulfur with organics. Ice nucleation experiments show IMF events at temperatures as low as 231 K, including the subsaturated regime. DIN events were observed at lower temperatures of 210 to 231 K. IMF and DIN observations were analyzed with regard to activated INP fraction, ice-nucleation active site (INAS) densities, and a water activity-based immersion freezing model (ABIFM) yielding heterogeneous ice nucleation rate coefficients. Observed IMF and DIN events of ice formation and corresponding derived freezing rates demonstrate that the marine boundary layer aerosol particles can serve as INPs under typical mixed-phase and cirrus cloud conditions. The derived IMF and DIN parameterizations allow for implementation in cloud and climate models to evaluate predictive effects of atmospheric ice crystal formation.
- Article
(9230 KB) - Full-text XML
-
Supplement
(1625 KB) - BibTeX
- EndNote
Understanding how atmospheric aerosol serves as ice-nucleating particles (INPs) is necessary to advance our understanding of cloud microphysical processes that impact precipitation and climate (Boucher et al., 2013; Storelvmo, 2017; Baker and Peter, 2008; Mülmenstädt et al., 2015; Lohmann and Feichter, 2005). To improve our predictive capabilities of atmospheric ice formation, laboratory and field-based research addressing the efficacy and physicochemical nature of INPs has intensified over recent years (Cantrell and Heymsfield, 2005; Murray et al., 2012; Kanji et al., 2017; Cziczo et al., 2017; DeMott et al., 2011; Knopf et al., 2018, 2021). The challenge lies in the fact that only a small fraction of total aerosol particles serves as INPs (DeMott et al., 2010). Furthermore, different ice formation pathways exist, which in turn depend on particle properties and ambient conditions such as temperature (T) and relative humidity (RH) (Pruppacher and Klett, 1997; Vali et al., 2015; Knopf et al., 2018; Marcolli, 2014). To contribute to our current understanding of atmospheric INPs, we performed aerosol particle collection, compositional analysis, and subsequent ice nucleation experiments as part of the Aerosol and Cloud Experiments in the Eastern North Atlantic (ACE-ENA) field campaign (Wang et al., 2021).
The motivation of the ACE-ENA campaign was to further our understanding of marine boundary layer clouds through field measurements of cloud condensation nuclei (CCN), drizzle, and cloud microphysics (Wang et al., 2021). This campaign involved ground site measurements at the ENA site and airborne instrument employment during two intensive operational periods in summer 2017 and winter 2018. The ground ENA site was established on the island of Graciosa in the Azores, Portugal (39∘5′30′′ N, 28∘1′32′′ W; 30.48 , Fig. S1 in the Supplement), by the U.S. Department of Energy (DOE) Atmospheric Radiation Measurement (ARM) Climate Research Facility (Wang et al., 2021; Mather and Voyles, 2013). This site experiences a wide range of meteorological and cloud conditions and can be prone to aerosol particles downwind of the North American continent (Wang et al., 2021). Furthermore, dry intrusion events from the free troposphere into the marine boundary layer can cause its deepening, drying, and cooling (Raveh-Rubin, 2017; Ilotoviz et al., 2021) and impact the make-up of the boundary layer aerosol population (Tomlin et al., 2021). In this study, we report on composition and ice nucleation analyses of particles collected at the ENA ground site during the first intensive operating period during the summer of 2017.
Laboratory and field studies have demonstrated large variety of particle types that can act as INPs. Inorganic particles such as mineral dust, metal oxides, and crystalline salts can serve as INPs (Murray et al., 2012; Kanji et al., 2017; Cziczo et al., 2017). Organic particles including primary organic aerosol (POA), e.g., from marine environments, biomass burning, and fossil fuel combustion, and secondary OA (SOA) from biogenic and anthropogenic precursor gases have been shown to initiate ice formation (Kanji et al., 2017; Knopf et al., 2018). Biological particles such as bacteria, fungi, spores, phytoplankton, and soil dust particles from agricultural lands can contribute to INPs (Murray et al., 2012; Kanji et al., 2017; Knopf et al., 2018; Despres et al., 2012). The majority of studies have focused on immersion freezing (IMF), where the immersed INPs initiate freezing of the supercooled droplets, compared to deposition ice nucleation (DIN), where ice forms from the supersaturated gas phase on the surface of the INPs (Knopf et al., 2018; Vali et al., 2015; Pruppacher and Klett, 1997). DIN may also be the consequence of homogeneous freezing of water in nanometer-sized pores due to a depression of the adjacent saturation vapor pressure, termed pore condensation freezing (PCF) (David et al., 2019; Marcolli, 2014, 2020). IMF is recognized as the dominant primary ice formation pathway in mixed-phase cloud regimes (Ansmann et al., 2009; de Boer et al., 2011; Westbrook and Illingworth, 2013), where supercooled droplets and ice crystals can coexist. DIN can contribute to ice crystal formation at lower temperatures and water-subsaturated conditions representing cirrus cloud regimes, typical of the upper troposphere (DeMott, 2002; Heymsfield et al., 2017; Cziczo et al., 2013).
The ENA ground site observatory is located in a remote marine region and close to the island shore (Fig. S1). For this reason, the boundary layer particle types likely constitute a large fraction of sea spray aerosol (SSA) particles. Aged and nascent SSA particles consist of inorganic and organic species, where smaller particle sizes often display greater enrichment of organic species (Cochran et al., 2017; Pham et al., 2017; Jayarathne et al., 2016; Aller et al., 2017; Facchini and O'Dowd, 2009; O'Dowd et al., 2004; Laskin et al., 2016; Ault et al., 2013). Field and laboratory experiments have demonstrated that SSA particles can act as INPs where aerosolized organic carbonaceous (OC) matter associated with exudates from phytoplankton serves as ice-nucleating agents (Creamean et al., 2019; McCluskey et al., 2018a, b, 2017; DeMott et al., 2016; Knopf et al., 2011, 2014; Alpert et al., 2011a, b; Ladino et al., 2016; Wilson et al., 2015; Prather et al., 2013; Schnell, 1975; Irish et al., 2019, 2017; Ickes et al., 2020; Wilbourn et al., 2020; Wolf et al., 2019; Schnell and Vali, 1975; Roy et al., 2021; Wagner et al., 2021).
Micro-spectroscopic single-particle analyses of the collected particle population and of INPs allow for a better understanding of how a particle population serves as a source of INPs and which particles preferentially act as INPs (China et al., 2017; Knopf et al., 2014, 2018; Wang et al., 2012a; Hiranuma et al., 2013; Cziczo et al., 2017). Nanoscale chemical imaging methods such as computer-controlled scanning electron microscopy with energy-dispersive X-ray analysis (CCSEM/EDX) and scanning transmission X-ray microscopy with near-edge X-ray absorption fine-structure spectroscopy (STXM/NEXAFS) are typically employed to examine the elemental composition of a large number of aerosol particles and to determine the organic speciation and mixing state of collected particles, respectively. Examination of large ensembles of individual particles by CCSEM/EDX allows for a statistically significant representation of the major particle-type classes present in a particle population (Knopf et al., 2014; Wang et al., 2012a; Thompson, 1987; Laskin and Cowin, 2001; Hopkins et al., 2007a, 2007b; Laskin et al., 2019, 2016, 2006). STXM/NEXAFS is applied to infer the particle population mixing state (MS) and the individual particle's composition and organic volume fraction (OVF) and to examine the nature of the organic carbon associated with the particle (Laskin et al., 2019, 2016; Moffet et al., 2016, 2010b; O'Brien et al., 2015; Tivanski et al., 2007; Bluhm et al., 2006; Knopf et al., 2021).
This study follows our previous analytical methods of ambient aerosol and INPs (Knopf et al., 2010, 2014, 2018, 2021; China et al., 2017; Wang et al., 2012a). Here, we collected particle samples over several days and nights at the ENA site during summer 2017 for analysis of ambient aerosol and INPs. We apply CCSEM/EDX and STXM/NEXAFS for identification and characterizations of the particle population present on the samples. We measure IMF and DIN for typical tropospheric RH and T as low as 210 K. We identify individual INPs using SEM/EDX and discuss those findings in the context of the particle population present on the samples. We derive IMF and DIN kinetics, report INP L−1 of air, ice nucleation active sites (INAS), and classical nucleation theory (CNT)-based parameters for each ice formation pathway, and provide corresponding IMF and DIN parameterizations for application in cloud and climate models.
2.1 Particle sampling
Particles were collected by impaction using a Multi Orifice Uniform Deposition Impactor (MOUDI, 110-R). Since ambient particle numbers were low (between 330 and 540 cm−3, Gallo et al., 2020) and sampling intervals for this ice nucleation study were limited, particle collection was conducted over several days intermittently. This ensured sufficient aerosol loading on substrates for single-particle chemical analyses and ice nucleation experiments based on our previous studies (China et al., 2017; Knopf et al., 2014; Wang et al., 2012a, b). Hence, particle analysis and INP characterization should not be interpreted as single defined events but as an average over 2–4 d. Table 1 lists the particle collection characteristics of the four samples (two daytime and two nighttime samples). The particle samples were collected on the sixth stage of the MOUDI with D50 % = 0.56 µm. The particle samples for a given MOUDI stage were chosen in such a way as to provide the optimal particle loading for ice nucleation experiments and application of single-particle analytical techniques across the different sampling periods to allow for more consistent analyses. Particle bounce, shattering, and non-sphericity of the ambient particles can lead to a much wider range in particle sizes collected on a stage with a specific cut-off diameter (Marple et al., 1991; Knopf et al., 2014; Pham et al., 2017; Mouri and Okada, 1993). Hence, single-particle micro-spectroscopic analyses are used to determine the geometric particle sizes on the sample substrate. Pre-arranged substrates were mounted on the MOUDI stage: Si3N4-coated silicon wafer chips for ice nucleation experiments and transmission electron microscopy (TEM) grids (copper 400 mesh grids, carbon type-B film, Ted Pella, Inc.) for CCSEM/EDX and STXM/NEXAFS analyses (Knopf et al., 2014; Charnawskas et al., 2017). The particle samples were stored in an airtight sealed container at room temperature under dry conditions (Knopf et al., 2014; Tomlin et al., 2021).
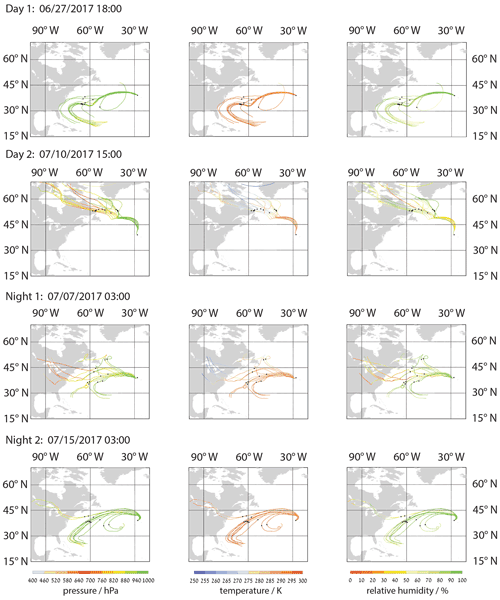
Figure 1Representative backward trajectories for examined samples and corresponding sampling periods. Color coding indicates pressure level (hPa), temperature (K), and relative humidity (%) from left to right. Black dots mark the sampling site and the trajectory locations 5 d prior to sampling. Local times are given and mark the time from which the 10 d backward trajectories are calculated.
Figure 1 depicts exemplary backward-trajectory calculations for the collection time periods given in Table 1. (Fig. S2 in the Supplement provides more backward-trajectory calculations for the 2–4 d sampling periods.) The trajectories were calculated using global wind data from the ECMWF reanalyses ERA5 (Hersbach et al., 2020). The data are horizontally interpolated to a 0.5∘ × 0.5∘ grid, with 3-hourly time intervals and 137 vertical hybrid levels. The Lagrangian analysis tool LAGRANTO version 2.0 (Sprenger and Wernli, 2015) was applied to calculate the trajectories from the measurement site, backwards for 10 d. Trajectories were calculated from the site at all available model levels from ground to a pressure level 50 hPa below ground pressure, resulting in 12 backward trajectories for each calculation (sampling time step). The black dots in Fig. 1 represent the locations of the air masses 5 d prior to their arrival at the site. In addition, T and RH were traced along the trajectory positions (Fig. 1). These additional parameters indicate that the air parcel, a few days before arrival at the measurement site, experienced RH > 50 %. It is worth noting that no dry intrusions (dry, deeply descending airstreams from the upper troposphere toward the marine boundary layer) according to the Lagrangian descent criterion in Raveh-Rubin (2017) were detected during the collection time periods (Tomlin et al., 2021; Ilotoviz et al., 2021).
2.2 Micro-spectroscopic single-particle analysis
We employed chemical imaging methods to analyze ensembles of particles collected on substrates and INPs following our previous work (China et al., 2017; Knopf et al., 2014; Wang et al., 2012a; Moffet et al., 2013, 2010b; Knopf et al., 2010; Hopkins et al., 2008, 2007a; Laskin et al., 2019, 2016, 2002; O'Brien et al., 2015; Tomlin et al., 2021), and thus our approach is only described briefly here. Characterization of the particle ensembles was performed by probing particle composition by CCSEM/EDX and STXM/NEXAFS. SEM/EDX was applied to infer the elemental composition of identified INPs. STXM/NEXAFS was used to give molecular information of particle carbonaceous content. For CCSEM/EDX and STXM/NEXAFS, we examined particles with an equivalent circle diameter larger than 200 nm.
Table 2Summary of particle sample information: sample name, number of particles examined by scanning electron microscopy (SEM), SEM sample surface area examined, SEM determined average circular equivalent diameter, number of particles examined by scanning transmission X-ray microscopy (STXM), number of particles examined for ice formation, particle surface area involved in ice formation experiments, and INP-activated fraction.
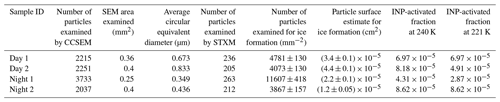
CCSEM/EDX was operated at 20 kV (CCSEM/EDX; FEI Quanta 3D, EDAX Genesis) to determine the size-resolved particle-type distribution in the ambient aerosol population using machine learning k-means cluster analysis (e.g., Knopf et al., 2014; Tomlin et al., 2021, 2020; Laskin et al., 2005; Moffet et al., 2012). Analysis of EDX particle spectra allowed us to quantify relative atomic fractions of the following elements: C, N, O, Na, Mg, Al, Si, P, S, Cl, K, Ca, Mn, and Fe. This type of analysis allows us to identify major particle-type classes that are significantly different in their elemental composition. Table 2 provides the number of particles and mean equivalent circle diameter for each particle sample. The equivalent circle diameter represents the diameter of a circle that would equal the same projected surface area as the imaged particle. More than 2000 particles were examined for each sample, though for identification of the major particle-type classes via k-means cluster analysis, the CCSEM/EDX data for all samples were taken together (Moffet et al., 2013; Seber, 1984; Spath, 1985; Tomlin et al., 2021, 2020). Such a large sample ensures a significant representation of the actual ambient particle population (Wang et al., 2012a; Thompson, 1987). For each particle sample, the distributions of the different particle types for examined equivalent circle diameters were derived. CCSEM/EDX allows for determination of the particle number density present on the substrates and the average particle diameter. Particle size information provides an estimate of the total particle number and surface area present during an ice nucleation experiment. Estimation of particle surface area applies an equivalent circle diameter and relies on the assumption that the particles are spherical. The collected particles are in most cases not spherical. Furthermore, nanoscale morphology, like nanopores, cavities, and cracks, is not considered in this analysis.
SEM/EDX was employed to characterize individual INPs spotted in optical microscopy (OM) ice nucleation experiments similar to our previous work (China et al., 2017; Knopf et al., 2014). Ice crystal formation by INPs was first recorded by OM at different magnifications as shown in Fig. S3 in the Supplement and as outlined below. Then, digital pattern recognition and triangulation allowed us to relocate the INP in the SEM for imaging and elemental composition analysis (Knopf et al., 2014; China et al., 2017).
We applied STXM/NEXAFS (Kilcoyne et al., 2003) to examine the particle carbon-specific MS, OC speciation, and OVF. STXM/NEXAFS was performed at the carbon K-edge (278–320 eV) to allow for chemical characterization of the particle composition and speciation of the OM fraction following our previous studies (Knopf et al., 2014; Moffet et al., 2010a, b, 2013, 2016; Hopkins et al., 2007b; Tomlin et al., 2020, 2021; Fraund et al., 2020; Laskin et al., 2019; O'Brien et al., 2014). In brief, two types of measurements were conducted. To examine a larger number of particles and their associated morphology and mixing state, STXM images at 35 nm pixel resolution and 1 ms dwell time were recorded at four selected energies: 278 eV (pre-edge, inorganic: IN), 285.4 eV (C=C, elemental carbon EC), 288.5 eV (COOH, OC), and 320 eV (post-edge). The recorded intensity across the particle segments for each energy was evaluated as optical density (OD). IN-rich regions were identified by having > 0.5. Total organic carbon was derived from the difference between OD320 eV and OD278 eV. Presence of carboxylic acid (COOH) was evaluated by the difference between OD288.5 eV and OD278 eV. Enrichment of elemental carbon (EC) was assessed following the method described in Hopkins et al. (2007b). These measurements allow for chemical composition mapping and derivation of the OVF of the individual particles (Fraund et al., 2019). For the OVF calculations, we used NaCl and adipic acid serving as representative mass absorption coefficients for the IN and OC particle fractions, respectively. These data were then applied to derive size-resolved mixing state and OVF distributions of the particle populations. The second type of STXM measurement involves the acquisition of high-resolution energy absorption (NEXAFS) spectra at 35 nm pixel resolution and 1 ms dwell time, where the particles were examined at 96 different energies between 278 and 320 eV. These NEXAFS spectra allow for a more detailed interpretation of the nature of the particulate OC.
2.3 Ice nucleation experiment
We employ a custom-built vapor-controlled cryo-cooling stage consisting of an ice nucleation cell and an OM to determine under which temperature and RH ice formation commences in the IMF and DIN modes as previously described (China et al., 2017; Charnawskas et al., 2017; Knopf et al., 2014, 2011; Wang et al., 2012b; Alpert et al., 2011a; Wang and Knopf, 2011). The particle samples were mounted into the ice nucleation cell that allows control of particle temperature from 200 K to room temperature and to exposure to humidity up to saturation. A humidified N2(g) ultra-high-purity (UHP) flow of about 1 slpm (standard liter per minute) is supplied into the ice nucleation cell. A chilled mirror hygrometer continuously measures the dew point temperature at the exit of the cell. RH in the ice nucleation cell is derived by the substrate temperature and the measured dew point (Wang and Knopf, 2011). Visual observation of the ice formation event allows us to distinguish between IMF and DIN (Wang and Knopf, 2011). Calibration of the humidity is performed by controlled ice crystal growth and sublimation experiments. The temperature accuracy of the cooling stage is independently verified by measuring the melting points of different organic compounds and ice, indicating less than 0.1 K difference between substrate and particle temperature (Knopf and Rigg, 2011). Typical uncertainty in RHice is about ± 4 % to ± 5.7 % and ± 0.15 K for temperature. For each sample and investigated temperature, at least three experiments were conducted. A typical experiment starts at a given particle temperature and for subsaturated conditions with respect to ice (i.e., RHice < 100 %) of about 30 %–60 %. Subsequently, RHice is continuously increased by about 1.5 % min−1–2.3 % min−1, reflecting actual vertical updraft velocities experienced in cirrus cloud formation, by decreasing the particle temperature by 0.1 K min−1 (Wang and Knopf, 2011; Knopf and Koop, 2006). Every 0.02 K or 12 s an image of the experiment and associated particle temperature and dew point is recorded. This allows for the analysis of ice nucleation mode, freezing temperature and humidity, INP-activated fraction, INAS density, and ice nucleation rate coefficient (China et al., 2017; Alpert et al., 2011a). It furthers enables detection of the ice nucleation mode (IMF and DIN) and identification of the individual INP (Fig. S3).
2.4 Ice nucleation analysis
The efficacy of INPs is typically expressed by different freezing parameterizations (Knopf et al., 2021, 2020, 2018; Vali, 1971; Connolly et al., 2009; DeMott et al., 2010; Knopf and Alpert, 2013). This includes the number concentration of INPs activated per liter of air for a given T and RH. Another commonly employed parameterization is the INAS density, ns, in unit cm−2 (Connolly et al., 2009). Both of these types of ice nucleation parameterizations are based on the deterministic or singular hypothesis approach (Vali, 1971; Connolly et al., 2009). This approach assumes that ns depends only on T, thereby neglecting time, and that each nucleation event is associated with a characteristic ice nucleation active site. Typically, ns(T) is reported for saturated conditions; i.e., an INP is engulfed in water (Murray et al., 2012). In contrast, CNT assumes that nucleation is time dependent and, thus, is stochastic in nature (Pruppacher and Klett, 1997; Knopf et al., 2020). Its time dependence yields an ice nucleation rate derived from the heterogeneous ice nucleation rate coefficient Jhet (). The water activity (aw)-based immersion freezing model (ABIFM) expresses Jhet as a function of the water activity criterion Δaw, thereby accounting for T and RH conditions including the subsaturated regime (Knopf et al., 2020; Alpert and Knopf, 2016; Knopf and Alpert, 2013). Δaw represents the difference between the water activity at the ice melting point, , for the observed freezing temperature and the water activity at the temperature for which ice formation was observed: Δaw(T) = (Koop et al., 2000; Knopf and Alpert, 2013). Assuming that the particle is in equilibrium with the gas phase, particle aw = RH. Hence, Δaw(T) depends on two variables, T and RH. Application of either INAS or ABIFM to experimental freezing data acquired at given conditions (i.e., T, RH, nucleation time) yields the same INP number concentrations. However, when applied to conditions different from experiments, e.g., when looking at different cloud activation timescales or particle size distributions, differences in predicted INP number concentrations by these parameterizations are expected, though the magnitude of difference depends on the activation rate and the efficacy of the INPs (Knopf et al., 2021).
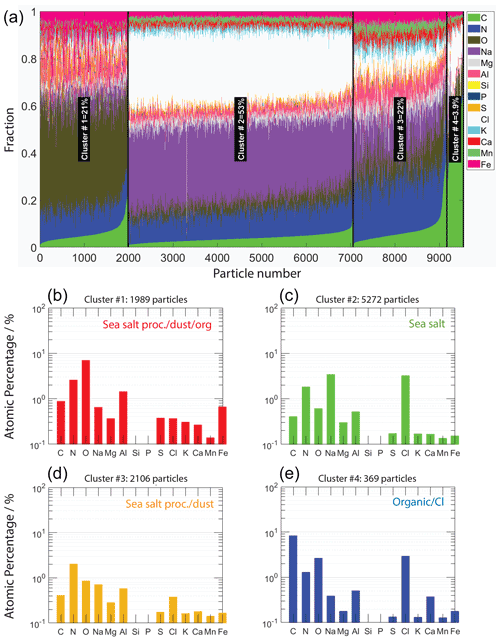
Figure 2CCSEM/EDX-derived cluster analysis for identification of particle-type classes present in ACE-ENA particle samples. Panel (a) represents the cumulative atomic fraction of all analyzed particles. Panels (b–e) give detailed views of the cluster-averaged elemental compositions of the four particle-type classes identified by k-means cluster analysis. The representative particle types include “processed sea salt with mineral dust, sulfur, and organic matter”, “sea salt particles”, “processed sea salt with mineral dust”, and “organic matter–chlorine”-containing particles.
3.1 Particle population characterization
Figure 2 shows the k-means cluster analysis results derived from CCSEM/EDX measurements. Over 9000 individual particles from two daytime and two nighttime samples were analyzed. This analysis yielded four significantly different particle types. According to the cluster-averaged elemental composition given by the atomic percentage, we have termed those particle types (i) processed sea salt with signatures of dust, sulfur, and OC (sea salt proc./dust/org), (ii) fresh sea salt (sea salt), (iii) processed sea salt with dust signatures (sea salt proc./dust), and (iv) organic with chlorine (organic/Cl). Processed sea salt indicates the loss of chlorine, likely due to chemical reactions with particulate nitric acid, sulfuric acid, and organic acids leading to gaseous HCl (Wang et al., 2015; Laskin et al., 2012; Angle et al., 2021). Particle type “sea salt proc./dust/org” contains the most sulfur of all particle types and shows a greater amount of carbon and oxygen compared to particle type “sea salt proc./dust”. Particle type “sea salt” indicates fresh sea salt particles with a ratio close to unity. Lastly, particle type “organic/Cl” represents particles with a greater atomic fraction of OC and chlorine. The naming of the different particle-type classes is arbitrary. It serves mostly the purpose of assessing whether identified INPs belong to those four major particle-type classes or whether they belong to completely different particle types, as will discussed below. Table 2 provides additional information on the number of particles per sample investigated and the average area-equivalent diameter (AED) for each sample. The mean AEDs of all examined particles were in the submicrometer range. Overall, the most abundant particle type was “fresh sea salt” particles, followed by “sea salt proc./dust”, “sea salt proc./dust/org”, and “organic/Cl”.
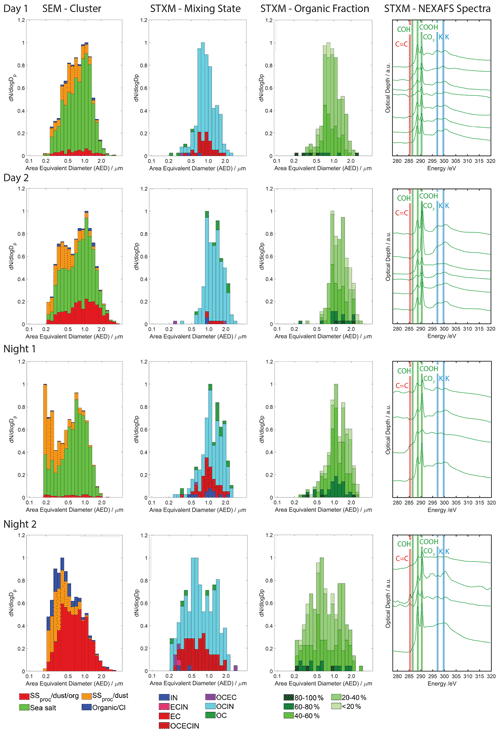
Figure 3Size-resolved particle composition for particle samples determined by micro-spectroscopic single-particle analysis as a function of area-equivalent diameter (AED). From left to right: first column: CCSEM/EDX particle-type classes. Second column: STXM/NEXAFS-derived groups of particles with different mixing states: IN – inorganic, EC – elemental carbon, OC – organic carbon. Third column: organic volume fraction (OVF). Fourth column: representative NEXAFS spectra at the carbon K-edge.
Figure 3 displays the CCSEM/EDX-derived particle-type size distribution derived from MOUDI stage 6 for the four particle samples. Day 1 and Day 2 and Night 1 samples show the dominance of fresh sea salt particles and differ only in the amounts of “sea salt proc./dust” and “sea salt proc./dust/org” particles. The Day 2 sample shows the greatest amount of “sea salt proc./dust/org” particles. For all samples, for particles larger than 1 µm, few “sea salt proc./dust” particles are present. The Night 2 sample displays a very different particle type distribution. Fresh “sea salt” is not present, and the examined particle size range is dominated by “sea salt proc./dust/org” particles. It is also the only sample that contains significant amounts of “organic/Cl” particles with diameters smaller than 1 µm.
We applied STXM/NEXAFS to derive the individual particle MS, OVF, and speciation of the organic matter. Particle numbers examined are significantly lower than for CCSEM/EDX analysis (Table 2). As such, the corresponding uncertainties in particle MS and OVF distributions are greater. For this analysis, with a 95 % confidence, the uncertainty in the representative distribution is between 20 % and 40 % (Thompson, 1987). For example, if the 30 % of particles in a size bin have been identified as OC, the uncertainty is about ± 4.5 %. The aim of the STXM/NEXAFS analysis is not to provide the most accurate physicochemical representation of the ambient particle population but to obtain additional information on the aerosol population serving as an INP source. Figure 3 displays the size-resolved particle MS and OVF and representative NEXAFS spectra. Figure S4 in the Supplement displays representative false-colored MS and OVF images of the particle samples for the purpose of visualization. Figure S5 in the Supplement presents the same data as Fig. 3, but the size-resolved particle MS and OVF are expressed as the fractional particle MS and OVF per size bin. The particle MS analysis shown in Fig. 3 indicates that all samples are dominated by particles made up by OCIN. Sample Day 1 contains the smallest number of pure OC particles. Overall, samples Day 1 and Day 2 have very similar characteristics in terms of particle MS and OVF. Day 1 sample shows a slightly higher fraction of OCIN particles that contain C=C bonds (EC). For both daytime samples, the OVF is dominated by particles that contain 20 %–40 % OC. The corresponding NEXAFS spectra point to sea salt coated with OC matter that consists of carboxylic acid components (R(C=O)OH at a 288.5 eV peak) and the presence of carbonate (CO3 at 290.4 eV). Figure S4 shows that all particles are associated with OC matter. For most NEXAFS spectra the presence of potassium is also evident. The NEXAFS spectra of samples Day 1 and Day 2 are similar to previous studies examining SSA with organic coatings or aged SSA (Laskin et al., 2012; Knopf et al., 2014; Pham et al., 2017; Tomlin et al., 2021; Ault et al., 2013).
Samples Night 1 and Night 2 possess some distinct features compared to the daytime samples. Night 1 samples are dominated by OCIN and OCECIN particles and contain very few IN particles and larger OC particles. The OVF analysis indicates that particles containing 40 %–60 % OC dominate the distribution. NEXAFS spectra include signatures of organic-coated SSA particles but also spectra that show the presence of C=C bonds. Night 2 samples are dominated by OCIN and OCECIN particles, where the latter is present in the largest fraction among all particle samples considered here and is limited to the sixth stage of the MOUDI. Purely OC particles are a very minor constituent of this particle population. The most prominent OVF values range between 20 % and 60 %. NEXAFS spectra mostly resemble OC associated with SSA, similarly to previous studies (Ault et al., 2013; Pham et al., 2017), though with lesser and more contribution of C=C bonds and hydroxyl groups. Overall, the STXM/NEXAFS results show that most of the particles are associated with OC matter at more than 20 % volume fraction and that this OC matter in most cases resembles organic components previously associated with SSA particles.
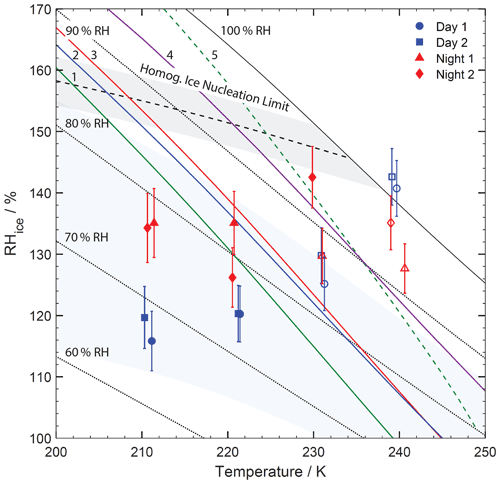
Figure 4IMF – immersion freezing (open symbols) – and DIN – deposition ice nucleation (solid symbols) – for examined ACE-ENA particle samples are given as a function of T and RHice. The data points and error bars reflect the mean of several measurements accounting for uncertainties in RH. Solid line represents conditions of water saturation (100 % RH). Dotted lines indicate constant relative humidity (RH). Dashed line and grey shading represent the homogeneous freezing limit for droplets of 10 µm in size and corresponding uncertainty (Koop, 2004; Koop et al., 2000). The glass transition temperature of laboratory-generated α-pinene SOA (green line or 1, Charnawskas et al., 2017), naphthalene SOA (blue line or 2, Charnawskas et al., 2017) field-derived SOA (red line or 3, Wang et al., 2012b), and Suwannee River Fulvic Acid (SRFA) particles (dark violet line or 4, Wang et al., 2012b) are plotted. The dashed green line (or 5) displays the FDRH for α-pinene SOA particles, 500 nm in diameter, under the humidification rate of this experiment (Charnawskas et al., 2017). The light bluish area indicates the conditions for pore condensation freezing for pore sizes of 7.5 to 20 nm (Marcolli, 2020, 2014).
3.2 Ice nucleation experiments
Figure 4 shows the results of the ice nucleation experiments. Plotted data points and uncertainties reflect the average and standard deviation of conducted measurements. Except for the warmest ice formation temperatures for Day 1 and Day 2 samples, all ice formation was detected under water-subsaturated conditions. Only for temperatures greater than 231 K was IMF observed, while sample Night 2 showed DIN for the highest temperature at 230 K. All observed ice formation events occurred under T and RH conditions that would not allow for homogeneous ice nucleation. In other words, ice formation was observed for temperatures greater than the maximum for homogeneous freezing and for humidity lower than the minimum humidity required for homogeneous ice nucleation (Koop et al., 2000).
Samples Day 1 and Day 2 display very similar conditions for DIN and IMF. In fact, they agree with each other within the measurements' uncertainties. Both samples show the greatest physicochemical similarity among the four particle samples as discussed above. Also, the particle loading is similar (Table 2). For temperatures below 240 K the Day 1 and Day 2 samples display IMF at below 130 % RHice, or about 85 % RH, and DIN for below 121 % RHice, or about 74 % RH, both reflecting subsaturated conditions. IMF at saturated conditions commenced at 240 K. Overall, these measurements indicate that the Day 1 and Day 2 samples could act as INPs at colder mixed-phase cloud temperatures and cirrus cloud temperatures.
Samples Night 1 and Night 2 show different IMF and DIN conditions. At 240 K, IMF occurs at subsaturated conditions. Sample Night 1 initiates IMF at ∼ 231 K similarly to Day 1 and Day 2 samples at ∼ 130 % RHice. For lower temperatures, DIN proceeds at higher RHice compared to Day 1 and Day 2 samples. The Night 2 sample induces DIN at ∼ 231 K, at ∼ 143 % RHice, and for all lower temperatures at greater RHice.
IMF and DIN proceed at similar conditions to those observed in our previous studies applying particle samples collected at the Pico Mountain Observatory (PMO) in the Azores (China et al., 2017; Lata et al., 2021). Since the particle samples are dominated by OCIN particles and display organic coatings (Figs. 3 and S4), we have plotted the glass transition point (Tg) of various organic aerosol (OA) surrogates (Charnawskas et al., 2017; Wang et al., 2012b) in Fig. 4. It is recognized that solid (glassy) particles can act as INPs (Murray et al., 2010; Wang et al., 2012b; Knopf et al., 2018). A measure of the viscosity of the OC particle fractions is given by the Tg and its full deliquescence RH (FDRH) (Knopf et al., 2018; Charnawskas et al., 2017). Lata et al. (2021) have shown the presence of highly viscous particles with Tg values greater than room temperature in samples collected at the PMO. The Tg of laboratory-generated α-pinene SOA displays the lowest Tg (green line, Charnawskas et al., 2017), followed by naphthalene SOA (blue line, Charnawskas et al., 2017), field-derived SOA Tg (red line, Wang et al., 2012b), and Tg of Suwannee River Fulvic Acid (SRFA) particles (dark violet line, Wang et al., 2012b). The dashed green line displays the FDRH for 500 nm α-pinene SOA particles under the humidification/cooling rate of this experiment (Charnawskas et al., 2017). This indicates that the particle system between the solid and dashed green line consists of a solid organic particle surrounded by an aqueous organic solution. At the FDRH point, an aqueous solution droplet exists which continues to take up more water if humidification or cooling commences (Berkemeier et al., 2014). However, OC matter present as a thin coating will reach its FDRH sooner, i.e., at lower temperatures and RH as discussed previously (Charnawskas et al., 2017). Since Tg of ambient SOA, naphthalene SOA, and SRFA are greater than those of α-pinene SOA, we would expect that corresponding FDRHs will also be greater. Considering these points, we cannot rule out that particulate OC matter served as IMF INPs, emphasizing the potential of OA to act as INPs (Knopf et al., 2021; Knopf et al., 2018). It is worthwhile noting that most DIN events occurred at conditions below the plotted Tg, implying that those likely solid organic or organic-coated particles acted as INPs.
Figure 4 includes the estimated range of PCF for pores in the size range of 7.5 to 20 nm. The majority of observed DIN falls in this area. Since we do not have detailed particle morphological data, the occurrence of PCF remains speculative. The particles are likely engulfed by a glassy organic coating at those lower temperatures. If the organic coating is secondary in nature, one would expect a smoother surface due to uniform condensation of low-volatile organic gases compared to when the organic is of primary origin. However, the underlying solid particle core may modulate surface structures, and it is known that OC matter experiencing T and RH cycles including freeze drying can change its morphology (Adler et al., 2013). More studies are needed to evaluate the possibility of the PCF mechanism being active on solid OA particles.
Table 3Information about identified ice-nucleating particles (INPs) including scanning electron microscopy (SEM)-derived averaged equivalent diameter and particle-type classification.
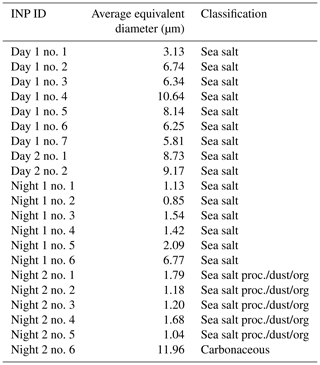
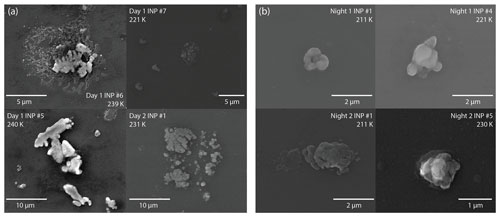
Figure 5Exemplary electron micrographs of identified ice-nucleating particles (INPs) for daytime particle samples (a) and nighttime particle samples (b). INP numbers refer to INPs shown in Fig. 6. The observed ice formation temperature is given for each INP.
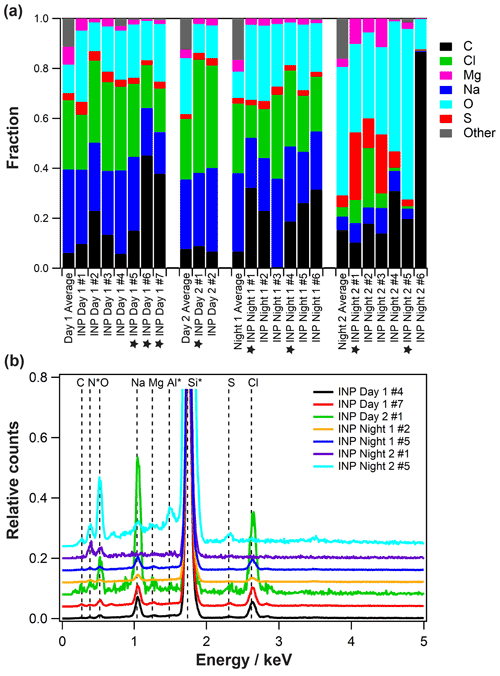
Figure 6Composition of experimentally identified INPs. (a) Cumulative atomic percent of elements for 21 identified individual INPs is shown as bars for examined ACE-ENA particle samples. The first column represents the average cumulative atomic percent of elements for the specific particle sample. Stars indicate INPs shown in Fig. 5. Panel (b) shows representative EDX spectra of identified INPs. ∗ corresponds to the signal from the substrate (Si3N4-coated silicon wafer chips) and the chamber/holder (Al).
3.3 INP identification
Twenty-one individual INPs were identified and analyzed by SEM/EDX, allowing for greater resolution compared to the OM. All identified INPs were in the supermicron size (Table 3). Figure 5 shows exemplary SEM-derived images of daytime and nighttime INPs and corresponding observed freezing temperatures. The elemental composition of the INPs is given in Fig. 6 (marked by a star). Daytime INPs show the dendritic morphology typical of processed sea salt particles, similarly to previous observations (Knopf et al., 2014; Wang et al., 2015; Laskin et al., 2012). This particle morphology is clearly different from the nighttime INPs. Figure 6 displays the average atomic percentages of each investigated sample (different from identified particle-type classes) and atomic percentages of identified INPs and exemplary EDX spectra. Additional EDX spectra of INPs are shown in Fig. S6 in the Supplement. These data demonstrate that the elemental composition of each INP (except INP Night 2 no. 6) reflects the typical particle composition on the sample. SEM/EDX analysis of the INPs allows for comparison with the particle types derived by the k-means cluster analysis. Table 3 provides the particle types that correspond to the identified INPs. Figure 6 indicates that INPs from samples Day 1, Day 2, and Night 1 possess very similar composition. Na and Cl dominate these particles in addition to OC, with minor contribution of S and Mg. All these identified INPs fall in the particle-type class “sea salt”. This corroborates previous studies indicating that SSA particles with OC matter act as INPs (McCluskey et al., 2018a; DeMott et al., 2016; Prather et al., 2013; Cornwell et al., 2021; Wilson et al., 2015; Knopf et al., 2011; Alpert et al., 2011a, b). This also indicates that with applied analytical methods we cannot distinguish the potential uniqueness of INPs from particles in the identified major particle-type classes. A similar conclusion was found in previous studies (China et al., 2017; Knopf et al., 2014; Lata et al., 2021). INPs of sample Night 2 show a different elemental composition (Fig. 6). The majority of those INPs are classified as “Sea salt proc./dust/org” (Table 3). This may be the reason for the different particle morphology seen in Fig. 5. One exception is INP Night 2 no. 6, which is purely carbonaceous. Carbonaceous particles were not identified as making up a significant particle-type class, though STXM/NEXAFS analysis clearly shows that those particles are present but in small numbers (Figs. 3, S4, and S5). In comparison to the identified particle-type classes, the identified carbonaceous INP represents a “rare” INP type. All INP types contain significant amounts of OC and as such support the above discussion about a potentially glassy organic coating acting as the INP. These findings further emphasize the need to better understand the role of OA particles and organic coatings acting as INPs (Knopf et al., 2021, 2018).
3.4 Immersion freezing kinetics
We analyzed observed IMF events for their freezing efficiency using commonly applied approaches. It should be emphasized that derived freezing efficiencies represent the behavior of a diverse particle population. For an IMF temperature of 240 K, we determined the activated INP fraction as given in Table 2. Applying mean total ambient particle concentrations for given sampling periods (Gallo et al., 2020) yields IMF INP number concentrations of about 15 to 40 INP L−1, in accordance with the range of previous measurements of INP number concentrations (Knopf et al., 2021; DeMott et al., 2016, 2010; Mason et al., 2015).
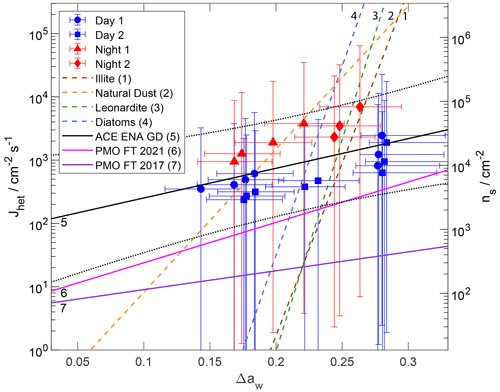
Figure 7Immersion freezing (IMF) data of examined ACE-ENA particle samples (solid symbols) and of previous studies (colored lines) as given in the legend. Heterogeneous ice nucleation rate coefficients (Jhet) and ice nucleation active site (INAS) density (ns) are presented as a function of the water activity criterion Δaw. Error bars include uncertainties in temperature, humidity, and surface area. Solid and dotted black lines represent a linear regression fit and associated fit uncertainties “ACE-ENA GD” to the data. Purple and magenta solid lines indicating “PMO FT 2017” and “PMO FT 2021”, respectively, represent IMF parameterizations of particles collected at the Pico Mountain Observatory (PMO) under free tropospheric (FT) conditions in the Azores on a neighboring island (China et al., 2017; Lata et al., 2021). Please note that only Jhet was reported for PMO FT 2021 (Lata et al., 2021).
We further analyzed the IMF freezing data using the surface-based deterministic approach INAS and the surface- and time-dependent approach of CNT expressed in our ABIFM following closely our previous methodologies (China et al., 2017; Wang et al., 2012a, b). As outlined in China et al. (2017), ) and , where Nice is the number of observed ice formation events usually equaling one; Atot is the total particle surface area accessible in the experiment (Table 2); t = 12 s is the time between two optical images during the experiment. Figure 7 shows the corresponding ns and Jhet values as a function of the Δaw for the four samples as solid symbols. Note that the greater the Δaw, the closer one approaches the homogeneous freezing limit (Koop et al., 2000; Knopf and Alpert, 2013). The IMF Jhet and ns values can be readily expressed as a function of temperature and droplet aw (e.g., aw = 1 for supercooled water) by application of the water-activity-based ice melting curve (Koop et al., 2000; Knopf and Alpert, 2013). The uncertainty in Δaw accounts for the uncertainty in temperature and RH. The uncertainty in Jhet accounts for the uncertainty in surface area (Table 2) and statistical uncertainty (Knopf et al., 2020), where the latter dominates the overall uncertainty. As outlined above, particle surface area is derived assuming that the particles are spherical and neglecting nanoscale morphological features. If the particle surface area is overestimated, derived Jhet values would represent lower limits. Taking into account nanoscale morphology, which was not achievable in this study, might yield greater particle surface area. This in turn would indicate the underestimation of the actual particle surface area. Hence, derived Jhet values would represent upper limits. However, the statistical uncertainty of nucleation spans several orders of magnitude and, thus, likely accounts for the uncertainty in particle surface area. In addition, the right y axis of Fig. 7 indicates the corresponding ns values. Since INAS is deterministic, it lacks the additional parameter of time, and the ns values are about a factor of 15 greater than the corresponding Jhet values. Some of the nighttime data show greater ns and Jhet values. This is, as shown in Fig. 5, because some of these samples induced IMF at subsaturated conditions at the highest temperatures.
Table 4Parameters for derivation of the immersion freezing (IMF) and deposition ice nucleation (DIN) heterogeneous ice nucleation rate coefficient (Jhet) and ice nucleation active site (INAS) density (ns) as a function of the water activity criterion, Δaw, according to and , are given. LCL and UCL represent lower and upper confidence levels at 95 %, respectively, for the fit parameters. RMSE indicates the root mean square error of the fit.
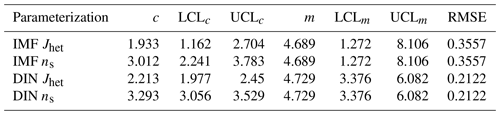
We derive an IMF parameterization for these remote marine boundary layer particle samples and for comparison with previous IMF parameterizations. Considering overall uncertainties, we merge all IMF data to derive a new Jhet and ns parameterization as a function of Δaw. The parameters for the ACE-ENA ground site (GD), Jhet and ns fit, shown as the black solid line in Fig. 7, are given in Table 4. The linear regression is performed without weighing of the data uncertainty due to the asymmetric error bars. Derived fit parameters (Table 4) reflect the approximate mean when performing the linear regression with maximum and minimum symmetric errors. The parameterization is valid for the Δaw range of measurements shown in Fig. 7. Our derived Jhet and ns values are larger than those derived from particles collected at the PMO during previous studies. However, it agrees well with very recently published IMF Jhet values for SSA derived from ambient measurements (Cornwell et al., 2021). That study derived median Jhet values of about 1000 at about 243 K (with 1–2 orders of magnitude uncertainty). This corresponds to Δaw ≈ 0.25 under saturated conditions (aw = 1). Our ABIFM model (Table 4) derives Jhet = 1270 , agreeing with their reported median Jhet values. Additionally, our IMF Jhet parameterization expands the temperature and humidity range of applicability since the Cornwell et al. (2021) parameterization is constrained to water saturation and temperatures from 240.15 to 244.65 K.
In general, IMF freezing rates from ambient particles of the Azores region display a shallower slope compared to measurements of INPs with laboratory surrogates. It can be expected that ambient aerosol contains a range of different INP types and sizes and, as such, freezing efficiencies may be broader and shallower (Knopf et al., 2020; Alpert and Knopf, 2016). Figure 7 displays natural desert dust freezing data by Niemand et al. (2012) that consist of different mineral dust types. Its slope is shallower than the single-component INP systems studied in the laboratory, as shown in Fig. 7 for illite dust particles, diatom cells, and leonardite particles. Ambient particles being more diverse than desert dust particles display the shallowest slopes. This is an important feature because, consequently, these ambient particles are more efficient INPs at warmer temperatures compared to efficient single-component INP types studied in the laboratory but are less efficient INPs at colder temperatures.
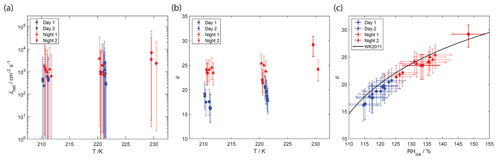
Figure 8Deposition ice nucleation (DIN) data of examined ACE-ENA particle samples (solid symbols). (a) Heterogeneous ice nucleation rate coefficients (Jhet) as a function of temperature. (b) Contact angles (θ) corresponding to Jhet values shown in (a). (c) θ values for relative humidity with respect to ice (RHice) under which DIN was observed. Solid line represents the DIN parameterization by Wang and Knopf (2011).
3.5 Deposition ice nucleation kinetics
For the analysis of DIN, we first employ CNT closely following the approach by Wang and Knopf (2011). Then we derive Jhet values as a function of Δaw, allowing for an alternative parametrization following the analysis in China et al. (2017). Experimentally derived Jhet values for DIN are shown in Fig. 8a. Jhet values range between 1000 and 10 000 . These Jhet values are about 1 order of magnitude greater than for IMF. Experimental Jhet values allow for the derivation of the contact angle, θ. Jhet is expressed as (Pruppacher and Klett, 1997)
where A is a pre-exponential factor, k is the Boltzmann constant, and the free energy of ice embryo formation is defined as (Pruppacher and Klett, 1997)
where R is the universal gas constant, Mw is the molecular weight of water, σi/v is the surface tension at the ice–vapor interface, ρ is the density of ice, Sice is the ice saturation ratio, and f(m,x) is the geometric factor. f depends on m and x. m is the compatibility factor and x represents the ratio of the radius of the substrate to the radius of the spherical ice germ. Since the INPs are > 0.1 µm, we can neglect x. Then, . The contact angle, θ, is defined as cos θ=m (Fletcher, 1958). The smaller the θ, the more efficient the INP.
θ is plotted in Fig. 8b. Daytime samples display lower θ values compared to nighttime samples, in line with Fig. 4 showing that daytime samples form ice at lower supersaturation than nighttime samples. Following Wang and Knopf (2011), we parameterize θ as a function of RHice, which allows us to describe several DIN data sets. Figure 8c shows the contact angles for the daytime and nighttime samples as a function of RHice. This analysis demonstrates that daytime samples display lower θ and as such are more efficient INPs. The derived θ values closely follow the parameterization (solid black line in Fig. 8c) given by Wang and Knopf (2011). This suggests that this parameterization can also be applied to estimate θ and thus Jhet for ACE-ENA ground site particle samples.
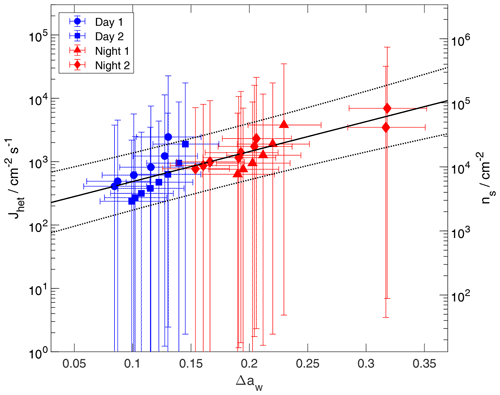
Figure 9Kinetic DIN data of examined ACE-ENA particle samples (solid symbols). Heterogeneous ice nucleation rate coefficients (Jhet) and INAS density (ns) are presented. Error bars included uncertainties in temperature, humidity, and surface area. Solid and dotted black lines represent a linear regression fit and associated fit uncertainties.
As an alternative parametrization, we apply the water activity criterion to describe Jhet for DIN. This allows for a computationally efficient way to describe DIN. This approach was introduced in China et al. (2017), showing agreement for measurements of kaolinite INPs with previous literature data. Figure 9 presents Jhet and ns values for DIN as a function of Δaw. As can be seen, Jhet and ns can be well represented by a linear fit given the experimental uncertainties. As in the case for IMF, the linear regression is performed without weighing of the data uncertainty due to the asymmetric error bars. The corresponding fit parameters are given in Table 4. As is evident from the CNT-based analysis shown in Fig. 8, daytime samples display the smallest contact angles indicating the greatest DIN efficiency. Here, daytime samples are associated with the lowest Δaw values. Jhet and ns values as a function of Δaw demonstrate an exponential behavior similar to the case of IMF.
This study shows that ambient sea salt and processed sea salt particles can affect mixed-phase and cirrus cloud formation by acting as IMF and DIN INPs. IMF and DIN conditions and corresponding nucleation rate coefficients and INAS densities are of a similar order of magnitude to other investigated INP types (Knopf et al., 2018; Murray et al., 2012; Kanji et al., 2017; Hoose and Möhler, 2012; Wang et al., 2012a; Wang and Knopf, 2011). For DIN, the contact angle for daytime samples is similarly low to some mineral dust and clay particles (Wang et al., 2012b; Wang and Knopf, 2011). Hence, these data suggest that ambient sea salt and processed sea salt particles could serve as competitive DIN INPs under cirrus cloud conditions. Note that only a very minor fraction of particles was identified as inorganic, and none of the identified INPs was purely inorganic in nature. This corroborates previous studies that indicated that the ocean can serve as a source of INPs (Creamean et al., 2019; McCluskey et al., 2018a, b, 2017; DeMott et al., 2016; Knopf et al., 2011, 2014; Alpert et al., 2011a, b; Ladino et al., 2016; Wilson et al., 2015; Prather et al., 2013; Schnell, 1975; Irish et al., 2019, 2017; Ickes et al., 2020; Wilbourn et al., 2020; Wolf et al., 2019; Schnell and Vali, 1975; Roy et al., 2021; Wagner et al., 2021; Cornwell et al., 2021). Furthermore, all identified INPs belong to the supermicrometer size regime. INP measurements at a coastal marine boundary layer site also indicated that the greatest INP number concentrations are associated with the largest particles (Mason et al., 2015). These results further emphasize the need to examine supermicrometer-sized particles for their ability to serve as INPs.
We derived IMF and DIN parameterizations based on Δaw. Δaw as a determinant of freezing combines temperature and RH parameters via the ice melting curve (Koop et al., 2000). As such, derived IMF and DIN parameterizations are applicable for subsaturated and saturated conditions. Homogeneous ice nucleation is also well described by Δaw (Koop et al., 2000). Hence, applying the same Δaw framework allows for a computationally efficient description of IMF, DIN, and homogeneous freezing. This could make it a method of choice for cloud-resolving model application (Knopf et al., 2021, 2018; Fan et al., 2017). The parameterizations presented here will allow us to estimate INP number concentrations for sea salt particle-dominated air masses in a remote marine region for mixed-phase and cirrus cloud conditions while accounting for the competing influence of homogeneous freezing.
Particle samples collected during daytime and nighttime in the remote marine boundary layer in the eastern North Atlantic were examined for the particle population composition, INPs, and IMF and DIN. Micro-spectroscopic single-particle analyses indicate that the sampled particle population is made up of four particle-type classes that all contain considerable amounts of organic matter. The particle types include “fresh sea salt particles” and “processed sea salt particles” with varying degrees of sulfur and dust inclusions. The dominance of sea salt in observed particle types is in order considering that particle collection was conducted in a remote marine region and close to the island's shoreline. All identified INPs belong to the supermicrometer size regime. All but 1 out of 21 identified INPs do not display different morphology or composition than the particle-type classes making up the sampled particle population. In other words, application of nanoscale analytical tools cannot resolve the difference between the major particle-type classes and the INPs, similar to findings of previous studies (Knopf et al., 2018, 2014; China et al., 2017; Lata et al., 2021). This is difficult to reconcile in a singular hypothesis/deterministic concept of ice nucleation but may be resolved assuming CNT, for example, assuming the major particle-type classes possess, on average, very similar surface features. Then Jhet acts on the total particle surface area until experimental conditions (supersaturation and time) allow for the detection of ice formation, without the need to specify which of the particles acted as INPs (Knopf et al., 2021, 2020, 2014). This hypothesis will need further exploration (Knopf et al., 2021, 2020).
IMF and DIN experiments point to the role of solid organic coatings as serving as INPs, though more compositional and kinetic details about the nature of the organic coating are needed to simulate observed ice formation (Knopf et al., 2018; Charnawskas et al., 2017; Berkemeier et al., 2014). Observed DIN proceeded in a range expected of PCF for pores in sizes of 7.5 to 20 nm (Marcolli, 2017, 2014). Since the nanoscale morphology of the organic coating is not well established in our experiments, it remains unclear whether PCF can act as the underlying freezing mechanism.
IMF and DIN experiments yielded INP-activated fraction and Jhet and ns values. We derived IMF and DIN parameterizations to express Jhet and ns for application in cloud and climate models. The derived freezing kinetics corroborate previous findings that marine-derived aerosol particles can serve as INPs under mixed-phase and cirrus cloud conditions. Parameterized IMF Jhet values agree with recently published IMF Jhet values derived from field campaigns (Cornwell et al., 2021). The similarity of IMF Jhet values from SSA acting as INPs stemming from different locations may point to the similar nature of the particulate organic matter impacting the particles' propensity to act as INPs. This study demonstrates, as have others, that the oceans can serve as a source of IMF and DIN INPs that should be considered when addressing cloud microphysical processes and climate.
All data needed to draw the conclusions in the present study are shown in the paper and/or the Supplement.
The supplement related to this article is available online at: https://doi.org/10.5194/acp-22-5377-2022-supplement.
DAK envisioned and supervised the project, performed STXM/NEXAFS experiments and analysis, conducted ice nucleation analyses, and wrote the first draft of the manuscript. JCC collected particle samples, conducted ice nucleation experiments, and assisted in STXM/NEXAFS experiments. PW, BW, AL, RCM, MKG, JYA, MF, and MAM assisted in STXM/NEXAFS experiments and analyses. DPV, SC, JMT, and KAJ conducted (CC)SEM/EDX experiments and analyses. SRR conducted backward-trajectory analyses. JW oversaw sampling site and particle collection. All the authors discussed interpretation of the data and contributed to the writing of the manuscript.
At least one of the authors is a member of the editorial board of AtmosphericChemistryandPhysics. The peer review process was guided by an independent editor, and the authors also have no other competing interests to declare.
This article is part of the special issue “Marine aerosols, trace gases, and clouds over the North Atlantic (ACP/AMT inter-journal SI)”. It is not associated with a conference.
Publisher's note: Copernicus Publications remains neutral with regard to jurisdictional claims in published maps and institutional affiliations.
The Stony Brook University, Purdue University, STI, and Washington University groups gratefully acknowledge support from the U.S. Department of Energy’s (DOE) Atmospheric System Research (ASR) program, Office of Biological and Environmental Research (OBER). A portion of this research was performed with a project award (https://doi.org/10.46936/lser.proj.2019.50738/60000088 and https://doi.org/10.46936/sthm.proj.2017.49857/60006200) from the Environmental Molecular Sciences Laboratory, a U.S. DOE Office of Science User Facility sponsored by the Biological and Environmental Research program. The STXM/NEXAFS particle analysis was performed at beamline 5.3.2.2 at the Advanced Light Source (ALS) at Lawrence Berkeley National Laboratory. The work at the ALS was supported by the Director, Office of Science, Office of Basic Energy Sciences, of the U.S. DOE.
This research has been supported by the U.S. Department of Energy (grant nos. DE-SC0016370 and DE-SC0021034 Stony Brook University; DE-SC0018948 Purdue University/STI; DE-SC0020259 Washington University, DE-AC05-76RL01830 EMSL, and DE-AC02-05CH11231 ALS).
This paper was edited by Hang Su and reviewed by two anonymous referees.
Adler, G., Koop, T., Haspel, C., Taraniuk, I., Moise, T., Koren, I., Heiblum, R. H., and Rudich, Y.: Formation of highly porous aerosol particles by atmospheric freeze-drying in ice clouds, P. Natl. Acad. Sci. USA, 110, 20414–20419, https://doi.org/10.1073/pnas.1317209110, 2013.
Aller, J. Y., Radway, J. C., Kilthau, W. P., Bothe, D. W., Wilson, T. W., Vaillancourt, R. D., Quinn, P. K., Coffman, D. J., Murray, B. J., and Knopf, D. A.: Size-resolved characterization of the polysaccharidic and proteinaceous components of sea spray aerosol, Atmos. Environ., 154, 331–347, https://doi.org/10.1016/j.atmosenv.2017.01.053, 2017.
Alpert, P. A., Aller, J. Y., and Knopf, D. A.: Ice nucleation from aqueous NaCl droplets with and without marine diatoms, Atmos. Chem. Phys., 11, 5539–5555, https://doi.org/10.5194/acp-11-5539-2011, 2011a.
Alpert, P. A., Aller, J. Y., and Knopf, D. A.: Initiation of the ice phase by marine biogenic surfaces in supersaturated gas and supercooled aqueous phases, Phys. Chem. Chem. Phys., 13, 19882–19894, 2011b.
Alpert, P. A. and Knopf, D. A.: Analysis of isothermal and cooling-rate-dependent immersion freezing by a unifying stochastic ice nucleation model, Atmos. Chem. Phys., 16, 2083–2107, https://doi.org/10.5194/acp-16-2083-2016, 2016.
Angle, K. J., Crocker, D. R., Simpson, R. M. C., Mayer, K. J., Garofalo, L. A., Moore, A. N., Garcia, S. L. M., Or, V. W., Srinivasan, S., Farhan, M., Sauer, J. S., Lee, C., Pothier, M. A., Farmer, D. K., Martz, T. R., Bertram, T. H., Cappa, C. D., Prather, K. A., and Grassian, V. H.: Acidity across the interface from the ocean surface to sea spray aerosol, P. Natl. Acad. Sci. USA, 118, 6, https://doi.org/10.1073/pnas.2018397118, 2021.
Ansmann, A., Baars, H., Tesche, M., Mueller, D., Althausen, D., Engelmann, R., Pauliquevis, T., and Artaxo, P.: Dust and smoke transport from Africa to South America: Lidar profiling over Cape Verde and the Amazon rainforest, Geophys. Res. Lett., 36, L11802, https://doi.org/10.1029/2009gl037923, 2009.
Ault, A. P., Moffet, R. C., Baltrusaitis, J., Collins, D. B., Ruppel, M. J., Cuadra-Rodriguez, L. A., Zhao, D., Guasco, T. L., Ebben, C. J., Geiger, F. M., Bertram, T. H., Prather, K. A., and Grassian, V. H.: Size-Dependent Changes in Sea Spray Aerosol Composition and Properties with Different Seawater Conditions, Environ. Sci. Technol., 47, 5603–5612, https://doi.org/10.1021/es400416g, 2013.
Baker, A. and Peter, T.: Small-scale cloud processes and climate, Nat. Geosci., 451, 299–300, 2008.
Berkemeier, T., Shiraiwa, M., Pöschl, U., and Koop, T.: Competition between water uptake and ice nucleation by glassy organic aerosol particles, Atmos. Chem. Phys., 14, 12513–12531, https://doi.org/10.5194/acp-14-12513-2014, 2014.
Bluhm, H., Andersson, K., Araki, T., Benzerara, K., Brown, G. E., Dynes, J. J., Ghosal, S., Gilles, M. K., Hansen, H. C., Hemminger, J. C., Hitchcock, A. P., Ketteler, G., Kilcoyne, A. L. D., Kneedler, E., Lawrence, J. R., Leppard, G. G., Majzlan, J., Mun, B. S., Myneni, S. C. B., Nilsson, A., Ogasawara, H., Ogletree, D. F., Pecher, K., Salmeron, M., Shuh, D. K., Tonner, B., Tyliszczak, T., Warwick, T., and Yoon, T. H.: Soft X-ray microscopy and spectroscopy at the molecular environmental science beamline at the Advanced Light Source, J. Electron Spectrosc., 150, 86–104, https://doi.org/10.1016/j.elspec.2005.07.005, 2006.
Boucher, O., Randall, D., Artaxo, P., Bretherton, C., Feingold, G., Forster, P., Kerminen, V.-M., Kondo, Y., Liao, H., Lohmann, U., Rasch, P., Satheesh, S. K., Sherwood, S., Stevens, B., and Zhang, X. Y.: Clouds and Aerosols, in: Climate Change 2013: The Physical Science Basis. Contribution of Working Group I to the Fifth Assessment Report of the Intergovernmental Panel on Climate Change, edited by: Stocker, T. F., Qin, D., Plattner, G.-K., Tignor, M., Allen, S. K., Boschung, J., Nauels, A., Xia, Y., Bex, V., and Midgley, P. M., Cambridge University Press, Cambridge, UK and New York, NY, USA, 2013.
Cantrell, W. and Heymsfield, A.: Production of ice in tropospheric clouds – A review, B. Am. Meteorol. Soc., 86, 795–807, https://doi.org/10.1175/bams-86-6-795, 2005.
Charnawskas, J. C., Alpert, P. A., Lambe, A. T., Berkemeier, T., O'Brien, R. E., Massoli, P., Onasch, T. B., Shiraiwa, M., Moffet, R. C., Gilles, M. K., Davidovits, P., Worsnop, D. R., and Knopf, D. A.: Condensed-phase biogenic-anthropogenic interactions with implications for cold cloud formation, Faraday Discuss., 200, 164–195, https://doi.org/10.1039/C7FD00010C, 2017.
China, S., Alpert, P. A., Zhang, B., Schum, S., Dzepina, K., Wright, K., Owen, R. C., Fialho, P., Mazzoleni, L. R., Mazzoleni, C., and Knopf, D. A.: Ice cloud formation potential by free tropospheric particles from long-range transport over the Northern Atlantic Ocean, J. Geophys. Res., 122, 3065–3079, https://doi.org/10.1002/2016jd025817, 2017.
Cochran, R. E., Ryder, O. S., Grassian, V. H., and Prather, K. A.: Sea Spray Aerosol: The Chemical Link between the Oceans, Atmosphere, and Climate, Accounts Chem. Res., 50, 599–604, https://doi.org/10.1021/acs.accounts.6b00603, 2017.
Connolly, P. J., Möhler, O., Field, P. R., Saathoff, H., Burgess, R., Choularton, T., and Gallagher, M.: Studies of heterogeneous freezing by three different desert dust samples, Atmos. Chem. Phys., 9, 2805–2824, https://doi.org/10.5194/acp-9-2805-2009, 2009.
Cornwell, G. C., McCluskey, C. S., DeMott, P. J., Prather, K. A., and Burrows, S. M.: Development of Heterogeneous Ice Nucleation Rate Coefficient Parameterizations From Ambient Measurements, Geophys. Res. Lett., 48, 10, https://doi.org/10.1029/2021gl095359, 2021.
Creamean, J. M., Cross, J. N., Pickart, R., McRaven, L., Lin, P., Pacini, A., Hanlon, R., Schmale, D. G., Ceniceros, J., Aydell, T., Colombi, N., Bolger, E., and DeMott, P. J.: Ice Nucleating Particles Carried From Below a Phytoplankton Bloom to the Arctic Atmosphere, Geophys. Res. Lett., 46, 8572–8581, https://doi.org/10.1029/2019gl083039, 2019.
Cziczo, D. J., Froyd, K. D., Hoose, C., Jensen, E. J., Diao, M. H., Zondlo, M. A., Smith, J. B., Twohy, C. H., and Murphy, D. M.: Clarifying the Dominant Sources and Mechanisms of Cirrus Cloud Formation, Science, 340, 1320–1324, https://doi.org/10.1126/science.1234145, 2013.
Cziczo, D. J., Ladino, L. A., Boose, Y., Kanji, Z. A., Kupiszewski, P., Lance, S., Mertes, S., and Wex, H.: Measurements of Ice Nucleating Particles and Ice Residuals, in: Ice Formation and Evolution in Clouds and Precipitation: Measurement and Modeling Challenges, edited by: Baumgardner, D., McFarquhar, G. M., and Heymsfield, A. J., American Meteorological Society, Chicago, 8.1–8.13, 2017.
David, R. O., Marcolli, C., Fahrni, J., Qiu, Y., Perez Sirkin, Y. A., Molinero, V., Mahrt, F., Brühwiler, D., Lohmann, U., and Kanji, Z. A.: Pore condensation and freezing is responsible for ice formation below water saturation for porous particles, P. Natl. Acad. Sci. USA, 116, 8184–8189, https://doi.org/10.1073/pnas.1813647116, 2019.
de Boer, G., Morrison, H., Shupe, M. D., and Hildner, R.: Evidence of liquid dependent ice nucleation in high-latitude stratiform clouds from surface remote sensors, Geophys. Res. Lett., 38, L01803, https://doi.org/10.1029/2010gl046016, 2011.
DeMott, P. J.: Laboratory Studies of Cirrus Cloud Processes, in: Cirrus, edited by: Lynch, D. K., Sassen, K., Starr, D. O., and Stephens, G., Oxford University Press, New York, 102–136, 2002.
DeMott, P. J., Prenni, A. J., Liu, X., Kreidenweis, S. M., Petters, M. D., Twohy, C. H., Richardson, M. S., Eidhammer, T., and Rogers, D. C.: Predicting global atmospheric ice nuclei distributions and their impacts on climate, P. Natl. Acad. Sci. USA, 107, 11217–11222, https://doi.org/10.1073/pnas.0910818107, 2010.
DeMott, P. J., Mohler, O., Stetzer, O., Vali, G., Levin, Z., Petters, M. D., Murakami, M., Leisner, T., Bundke, U., Klein, H., Kanji, Z. A., Cotton, R., Jones, H., Benz, S., Brinkmann, M., Rzesanke, D., Saathoff, H., Nicolet, M., Saito, A., Nillius, B., Bingemer, H., Abbatt, J., Ardon, K., Ganor, E., Georgakopoulos, D. G., and Saunders, C.: Resurgence in ice nuclei measurement research, B. Am. Meteorol. Soc., 92, 1623–1635, https://doi.org/10.1175/2011bams3119.1, 2011.
DeMott, P. J., Hill, T. C. J., McCluskey, C. S., Prather, K. A., Collins, D. B., Sullivan, R. C., Ruppel, M. J., Mason, R. H., Irish, V. E., Lee, T., Hwang, C. Y., Rhee, T. S., Snider, J. R., McMeeking, G. R., Dhaniyala, S., Lewis, E. R., Wentzell, J. J. B., Abbatt, J., Lee, C., Sultana, C. M., Ault, A. P., Axson, J. L., Martinez, M. D., Venero, I., Santos-Figueroa, G., Stokes, M. D., Deane, G. B., Mayol-Bracero, O. L., Grassian, V. H., Bertram, T. H., Bertram, A. K., Moffett, B. F., and Franc, G. D.: Sea spray aerosol as a unique source of ice nucleating particles, P. Natl. Acad. Sci. USA, 113, 5797–5803, https://doi.org/10.1073/pnas.1514034112, 2016.
Despres, V. R., Huffman, J. A., Burrows, S. M., Hoose, C., Safatov, A. S., Buryak, G., Fröhlich-Nowoisky, J., Elbert, W., Andreae, M. O., Pöschl, U., and Jaenicke, R.: Primary biological aerosol particles in the atmosphere: a review, Tellus B, 64, 1–58, https://doi.org/10.3402/tellusb.v64i0.15598, 2012.
Facchini, M. C. and O'Dowd, C. D.: Biogenic origin of primary and secondary organic components in marine aerosol, Geochim. Cosmochim. Ac., 73, A348–A348, 2009.
Fan, S. M., Knopf, D. A., Heymsfield, A. J., and Donner, L. J.: Modeling of Aircraft Measurements of Ice Crystal Concentration in the Arctic and a Parameterization for Mixed-Phase Cloud, J. Atmos. Sci., 74, 3799–3814, https://doi.org/10.1175/jas-d-17-0037.1, 2017.
Fletcher, N. H.: Size Effect in Heterogeneous Nucleation, J. Chem. Phys., 29, 572–576, 1958.
Fraund, M., Park, T., Yao, L., Bonanno, D., Pham, D. Q., and Moffet, R. C.: Quantitative capabilities of STXM to measure spatially resolved organic volume fractions of mixed organic/inorganic particles, Atmos. Meas. Tech., 12, 1619–1633, https://doi.org/10.5194/amt-12-1619-2019, 2019.
Fraund, M., Bonanno, D. J., China, S., Pham, D. Q., Veghte, D., Weis, J., Kulkarni, G., Teske, K., Gilles, M. K., Laskin, A., and Moffet, R. C.: Optical properties and composition of viscous organic particles found in the Southern Great Plains, Atmos. Chem. Phys., 20, 11593–11606, https://doi.org/10.5194/acp-20-11593-2020, 2020.
Gallo, F., Uin, J., Springston, S., Wang, J., Zheng, G., Kuang, C., Wood, R., Azevedo, E. B., McComiskey, A., Mei, F., Theisen, A., Kyrouac, J., and Aiken, A. C.: Identifying a regional aerosol baseline in the eastern North Atlantic using collocated measurements and a mathematical algorithm to mask high-submicron-number-concentration aerosol events, Atmos. Chem. Phys., 20, 7553–7573, https://doi.org/10.5194/acp-20-7553-2020, 2020.
Hersbach, H., Bell, B., Berrisford, P., Hirahara, S., Horanyi, A., Munoz-Sabater, J., Nicolas, J., Peubey, C., Radu, R., Schepers, D., Simmons, A., Soci, C., Abdalla, S., Abellan, X., Balsamo, G., Bechtold, P., Biavati, G., Bidlot, J., Bonavita, M., De Chiara, G., Dahlgren, P., Dee, D., Diamantakis, M., Dragani, R., Flemming, J., Forbes, R., Fuentes, M., Geer, A., Haimberger, L., Healy, S., Hogan, R. J., Holm, E., Janiskova, M., Keeley, S., Laloyaux, P., Lopez, P., Lupu, C., Radnoti, G., de Rosnay, P., Rozum, I., Vamborg, F., Villaume, S., and Thepaut, J. N.: The ERA5 global reanalysis, Q. J. Roy. Meteor. Soc., 146, 1999–2049, https://doi.org/10.1002/qj.3803, 2020.
Heymsfield, A. J., Krämer, M., Luebke, A., Brown, P., Cziczo, D. J., Franklin, C., Lawson, P., Lohmann, U., McFarquhar, G., Ulanowski, Z., and Van Tricht, K.: Cirrus Clouds, Meteor. Mon., 58, 2.1–2.26, https://doi.org/10.1175/amsmonographs-d-16-0010.1, 2017.
Hiranuma, N., Brooks, S. D., Moffet, R. C., Glen, A., Laskin, A., Gilles, M. K., Liu, P., Macdonald, A. M., Strapp, J. W., and McFarquhar, G. M.: Chemical characterization of individual particles and residuals of cloud droplets and ice crystals collected on board research aircraft in the ISDAC 2008 study, J. Geophys. Res., 118, 6564–6579, https://doi.org/10.1002/jgrd.50484, 2013.
Hoose, C. and Möhler, O.: Heterogeneous ice nucleation on atmospheric aerosols: a review of results from laboratory experiments, Atmos. Chem. Phys., 12, 9817–9854, https://doi.org/10.5194/acp-12-9817-2012, 2012.
Hopkins, R. J., Lewis, K., Desyaterik, Y., Wang, Z., Tivanski, A. V., Arnott, W. P., Laskin, A., and Gilles, M. K.: Correlations between optical, chemical and physical properties of biomass burn aerosols, Geophys. Res. Lett., 34, L18806, https://doi.org/10.1029/2007gl030502, 2007a.
Hopkins, R. J., Tivanski, A. V., Marten, B. D., and Gilles, M. K.: Chemical bonding and structure of black carbon reference materials and individual carbonaceous atmospheric aerosols, J. Aerosol Sci., 38, 573–591, https://doi.org/10.1016/j.jaerosci.2007.03.009, 2007b.
Hopkins, R. J., Desyaterik, Y., Tivanski, A. V., Zaveri, R. A., Berkowitz, C. M., Tyliszczak, T., Gilles, M. K., and Laskin, A.: Chemical Speciation of Sulfur in Marine Cloud Droplets and Particles: Analysis of Individual Particles from Marine Boundary Layer over the California Current, J. Geophys. Res., 113, D04209, https://doi.org/10.1029/2007JD008954, 2008.
Ickes, L., Porter, G. C. E., Wagner, R., Adams, M. P., Bierbauer, S., Bertram, A. K., Bilde, M., Christiansen, S., Ekman, A. M. L., Gorokhova, E., Höhler, K., Kiselev, A. A., Leck, C., Möhler, O., Murray, B. J., Schiebel, T., Ullrich, R., and Salter, M. E.: The ice-nucleating activity of Arctic sea surface microlayer samples and marine algal cultures, Atmos. Chem. Phys., 20, 11089–11117, https://doi.org/10.5194/acp-20-11089-2020, 2020.
Ilotoviz, E., Ghate, V. P., and Raveh-Rubin, S.: The Impact of Slantwise Descending Dry Intrusions on the Marine Boundary Layer and Air–Sea Interface Over the ARM Eastern North Atlantic Site, J. Geophys. Res.-Atmos., 126, e2020JD033879, https://doi.org/10.1029/2020jd033879, 2021.
Irish, V. E., Elizondo, P., Chen, J., Chou, C., Charette, J., Lizotte, M., Ladino, L. A., Wilson, T. W., Gosselin, M., Murray, B. J., Polishchuk, E., Abbatt, J. P. D., Miller, L. A., and Bertram, A. K.: Ice-nucleating particles in Canadian Arctic sea-surface microlayer and bulk seawater, Atmos. Chem. Phys., 17, 10583–10595, https://doi.org/10.5194/acp-17-10583-2017, 2017.
Irish, V. E., Hanna, S. J., Willis, M. D., China, S., Thomas, J. L., Wentzell, J. J. B., Cirisan, A., Si, M., Leaitch, W. R., Murphy, J. G., Abbatt, J. P. D., Laskin, A., Girard, E., and Bertram, A. K.: Ice nucleating particles in the marine boundary layer in the Canadian Arctic during summer 2014, Atmos. Chem. Phys., 19, 1027–1039, https://doi.org/10.5194/acp-19-1027-2019, 2019.
Jayarathne, T., Sultana, C. M., Lee, C., Malfatti, F., Cox, J. L., Pendergraft, M. A., Moore, K. A., Azam, F., Tivanski, A. V., Cappa, C. D., Bertram, T. H., Grassian, V. H., Prather, K. A., and Stone, E. A.: Enrichment of Saccharides and Divalent Cations in Sea Spray Aerosol During Two Phytoplankton Blooms, Environ. Sci. Technol., 50, 11511–11520, https://doi.org/10.1021/acs.est.6b02988, 2016.
Kanji, Z. A., Ladino, L. A., Wex, H., Boose, Y., Burkert-Kohn, M., Cziczo, D. J., and Krämer, M.: Overview of Ice Nucleating Particles, in: Ice Formation and Evolution in Clouds and Precipitation: Measurement and Modeling Challenges, Meteor. Mon., American Meteorological Society, 1.1–1.33, 2017.
Kilcoyne, A. L. D., Tyliszczak, T., Steele, W. F., Fakra, S., Hitchcock, P., Franck, K., Anderson, E., Harteneck, B., Rightor, E. G., Mitchell, G. E., Hitchcock, A. P., Yang, L., Warwick, T., and Ade, H.: Interferometer-controlled scanning transmission X-ray microscopes at the Advanced Light Source, J. Synchrotron Radiat., 10, 125–136, 2003.
Knopf, D. A. and Alpert, P. A.: A water activity based model of heterogeneous ice nucleation kinetics for freezing of water and aqueous solution droplets, Faraday Discuss., 165, 513–534, https://doi.org/10.1039/C3FD00035D, 2013.
Knopf, D. A. and Koop, T.: Heterogeneous nucleation of ice on surrogates of mineral dust, J. Geophys. Res., 111, D12201, https://doi.org/10.1029/2005jd006894, 2006.
Knopf, D. A. and Rigg, Y. J.: Homogeneous Ice Nucleation From Aqueous Inorganic/Organic Particles Representative of Biomass Burning: Water Activity, Freezing Temperatures, Nucleation Rates, J. Phys. Chem. A, 115, 762–773, https://doi.org/10.1021/jp109171g, 2011.
Knopf, D. A., Wang, B., Laskin, A., Moffet, R. C., and Gilles, M. K.: Heterogeneous nucleation of ice on anthropogenic organic particles collected in Mexico City, Geophys. Res. Lett., 37, L11803, https://doi.org/10.1029/2010GL043362, 2010.
Knopf, D. A., Alpert, P. A., Wang, B., and Aller, J. Y.: Stimulation of ice nucleation by marine diatoms, Nat. Geosci., 4, 88–90, https://doi.org/10.1038/ngeo1037, 2011.
Knopf, D. A., Alpert, P. A., Wang, B., O'Brien, R. E., Kelly, S. T., Laskin, A., Gilles, M. K., and Moffet, R. C.: Microspectroscopic imaging and characterization of individually identified ice nucleating particles from a case field study, J. Geophys. Res., 119, 10365–10381, https://doi.org/10.1002/2014JD021866, 2014.
Knopf, D. A., Alpert, P. A., and Wang, B.: The Role of Organic Aerosol in Atmospheric Ice Nucleation: A Review, ACS Earth Space Chem., 2, 168–202, https://doi.org/10.1021/acsearthspacechem.7b00120, 2018.
Knopf, D. A., Alpert, P. A., Zipori, A., Reicher, N., and Rudich, Y.: Stochastic nucleation processes and substrate abundance explain time-dependent freezing in supercooled droplets, npj Clim. Atmos. Sci., 3, 1–9, https://doi.org/10.1038/s41612-020-0106-4, 2020.
Knopf, D. A., Barry, K. R., Brubaker, T. A., Jahl, L. G., Jankowski, K. A., Li, J., Lu, Y., Monroe, L. W., Moore, K. A., Rivera-Adorno, F. A., Sauceda, K. A., Shi, Y., Tomlin, J. M., Vepuri, H. S. K., Wang, P., Lata, N. N., Levin, E. J. T., Creamean, J. M., Hill, T. C. J., China, S., Alpert, P. A., Moffet, R. C., Hiranuma, N., Sullivan, R. C., Fridlind, A. M., West, M., Laskin, A., DeMott, P. J., and Liu, X.: Aerosol–Ice Formation Closure: A Southern Great Plains Field Campaign, B. Am. Meteorol. Soc., 102, E1952–E1971 https://doi.org/10.1175/BAMS-D-20-0151.1, 2021.
Koop, T.: Homogeneous ice nucleation in water and aqueous solutions, Z. Phys. Chem., 218, 1231–1258, 2004.
Koop, T., Luo, B. P., Tsias, A., and Peter, T.: Water activity as the determinant for homogeneous ice nucleation in aqueous solutions, Nature, 406, 611–614, https://doi.org/10.1038/35020537, 2000.
Ladino, L. A., Yakobi-Hancock, J. D., Kilthau, W. P., Mason, R. H., Si, M., Li, J., Miller, L. A., Schiller, C. L., Huffman, J. A., Aller, J. Y., Knopf, D. A., Bertram, A. K., and Abbatt, J. P. D.: Addressing the ice nucleating abilities of marine aerosol: A combination of deposition mode laboratory and field measurements, Atmos. Environ., 132, 1–10, https://doi.org/10.1016/j.atmosenv.2016.02.028, 2016.
Laskin, A. and Cowin, J. P.: Automated single particle SEM/EDX analysis of submicrometer particles down to 0.1 µm, Anal. Chem., 73, 1023–1029, 2001.
Laskin, A., Iedema, M. J., and Cowin, J. P.: Quantitative time-resolved monitoring of nitrate formation in sea salt particles using a CCSEM/EDX single particle analysis, Environ. Sci. Techn., 36, 4948–4955, 2002.
Laskin, A., Wietsma, T. W., Krueger, B. J., and Grassian, V. H.: Heterogeneous chemistry of individual mineral dust particles with nitric acid: A combined CCSEM/EDX, ESEM, and ICP-MS study, J. Geophys. Res., 110, D10208, https://doi.org/10.1029/2004JD005206, 2005.
Laskin, A., Cowin, J. P., and Iedema, M. J.: Analysis of individual environmental particles using modern methods of electron microscopy and X-ray microanalysis, J. Electron Spectrosc., 150, 260–274, https://doi.org/10.1016/j.elspec.2005.06.008, 2006.
Laskin, A., Moffet, R. C., Gilles, M. K., Fast, J. D., Zaveri, R. A., Wang, B., Nigge, P., and Shutthanandan, J.: Tropospheric chemistry of internally mixed sea salt and organic particles: Surprising reactivity of NaCl with weak organic acids, J. Geophys. Res., 117, D15302, https://doi.org/10.1029/2012jd017743, 2012.
Laskin, A., Gilles, M. K., Knopf, D. A., Wang, B., and China, S.: Progress in the Analysis of Complex Atmospheric Particles, Annu. Rev. Anal. Chem., 9, 117–143, https://doi.org/10.1146/annurev-anchem-071015-041521, 2016.
Laskin, A., Moffet, R. C., and Gilles, M. K.: Chemical Imaging of Atmospheric Particles, Accounts Chem. Res., 52, 3419–3431, https://doi.org/10.1021/acs.accounts.9b00396, 2019.
Lata, N. N., Zhang, B., Schum, S., Mazzoleni, L., Brimberry, R., Marcus, M. A., Cantrell, W. H., Fialho, P., Mazzoleni, C., and China, S.: Aerosol Composition, Mixing State, and Phase State of Free Tropospheric Particles and Their Role in Ice Cloud Formation, ACS Earth Space Chem., 5, 3499–3510, https://doi.org/10.1021/acsearthspacechem.1c00315, 2021.
Lohmann, U. and Feichter, J.: Global indirect aerosol effects: a review, Atmos. Chem. Phys., 5, 715–737, 2005.
Marcolli, C.: Deposition nucleation viewed as homogeneous or immersion freezing in pores and cavities, Atmos. Chem. Phys., 14, 2071–2104, https://doi.org/10.5194/acp-14-2071-2014, 2014.
Marcolli, C.: Pre-activation of aerosol particles by ice preserved in pores, Atmos. Chem. Phys., 17, 1595–1622, https://doi.org/10.5194/acp-17-1595-2017, 2017.
Marcolli, C.: Technical note: Fundamental aspects of ice nucleation via pore condensation and freezing including Laplace pressure and growth into macroscopic ice, Atmos. Chem. Phys., 20, 3209–3230, https://doi.org/10.5194/acp-20-3209-2020, 2020.
Marple, V. A., Rubow, K. L., and Behm, S. M.: A microorifice unifrom deposit impactor (MOUDI) – description, calibration, and use, Aerosol Sci. Tech., 14, 434–446, https://doi.org/10.1080/02786829108959504, 1991.
Mason, R. H., Si, M., Li, J., Chou, C., Dickie, R., Toom-Sauntry, D., Pöhlker, C., Yakobi-Hancock, J. D., Ladino, L. A., Jones, K., Leaitch, W. R., Schiller, C. L., Abbatt, J. P. D., Huffman, J. A., and Bertram, A. K.: Ice nucleating particles at a coastal marine boundary layer site: correlations with aerosol type and meteorological conditions, Atmos. Chem. Phys., 15, 12547–12566, https://doi.org/10.5194/acp-15-12547-2015, 2015.
Mather, J. H. and Voyles, J. W.: The ARM climate research facility A Review of Structure and Capabilities, B. Am. Meteorol. Soc., 94, 377–392, https://doi.org/10.1175/bams-d-11-00218.1, 2013.
McCluskey, C. S., Hill, T. C. J., Malfatti, F., Sultana, C. M., Lee, C., Santander, M. V., Beall, C. M., Moore, K. A., Cornwell, G. C., Collins, D. B., Prather, K. A., Jayarathne, T., Stone, E. A., Azam, F., Kreidenweis, S. M., and DeMott, P. J.: A Dynamic Link between Ice Nucleating Particles Released in Nascent Sea Spray Aerosol and Oceanic Biological Activity during Two Mesocosm Experiments, J. Atmos. Sci., 74, 151–166, https://doi.org/10.1175/jas-d-16-0087.1, 2017.
McCluskey, C. S., Hill, T. C. J., Sultana, C. M., Laskina, O., Trueblood, J., Santander, M. V., Beall, C. M., Michaud, J. M., Kreidenweis, S. M., Prather, K. A., Grassian, V., and DeMott, P. J.: A Mesocosm Double Feature: Insights into the Chemical Makeup of Marine Ice Nucleating Particles, J. Atmos. Sci., 75, 2405–2423, https://doi.org/10.1175/jas-d-17-0155.1, 2018a.
McCluskey, C. S., Ovadnevaite, J., Rinaldi, M., Atkinson, J., Belosi, F., Ceburnis, D., Marullo, S., Hill, T. C. J., Lohmann, U., Kanji, Z. A., O'Dowd, C., Kreidenweis, S. M., and DeMott, P. J.: Marine and Terrestrial Organic Ice-Nucleating Particles in Pristine Marine to Continentally Influenced Northeast Atlantic Air Masses, J. Geophys. Res., 123, 6196–6212, https://doi.org/10.1029/2017jd028033, 2018b.
Moffet, R. C., Henn, T., Laskin, A., and Gilles, M. K.: Automated Chemical Analysis of Internally Mixed Aerosol Particles Using X-ray Spectromicroscopy at the Carbon K-Edge, Anal. Chem., 82, 7906–7914, 2010a.
Moffet, R. C., Tivanski, A. V., and Gilles, M. K.: Scanning X-ray Transmission Microscopy: Applications in Atmospheric Aerosol Research, in: Fundamentals and Applications in Aerosol Spectroscopy, edited by: Signorell, R. and Reid, J. P., Taylor and Francis Books, Inc., Boca Raton, 419–462, 2010b.
Moffet, R. C., Furutani, H., Rödel, T. C., Henn, T. R., Sprau, P. O., Prakash, S., Laskin, A., Uematsu, M., and Gilles, M. K.: Iron Speciation and Mixing in Single Aerosol Particles from the Asian Continental Outflow, J. Geophys. Res., 117, D07204, https://doi.org/10.1029/2011JD016746, 2012.
Moffet, R. C., Rödel, T. C., Kelly, S. T., Yu, X. Y., Carroll, G. T., Fast, J., Zaveri, R. A., Laskin, A., and Gilles, M. K.: Spectro-microscopic measurements of carbonaceous aerosol aging in Central California, Atmos. Chem. Phys., 13, 10445–10459, https://doi.org/10.5194/acp-13-10445-2013, 2013.
Moffet, R. C., O'Brien, R. E., Alpert, P. A., Kelly, S. T., Pham, D. Q., Gilles, M. K., Knopf, D. A., and Laskin, A.: Morphology and mixing of black carbon particles collected in central California during the CARES field study, Atmos. Chem. Phys., 16, 14515–14525, https://doi.org/10.5194/acp-16-14515-2016, 2016.
Mouri, H. and Okada, K.: Shattering and modification of sea-salt particles in the marine atmosphere, Geophys. Res. Lett., 20, 49–52, https://doi.org/10.1029/92gl03004, 1993.
Mülmenstädt, J., Sourdeval, O., Delanoe, J., and Quaas, J.: Frequency of occurrence of rain from liquid-, mixed-, and ice-phase clouds derived from A-Train satellite retrievals, Geophys. Res. Lett., 42, 6502–6509, https://doi.org/10.1002/2015gl064604, 2015.
Murray, B. J., Wilson, T. W., Dobbie, S., Cui, Z. Q., Al-Jumur, S. M. R. K., Möhler, O., Schnaiter, M., Wagner, R., Benz, S., Niemand, M., Saathoff, H., Ebert, V., Wagner, S., and Kärcher, B.: Heterogeneous nucleation of ice particles on glassy aerosols under cirrus conditions, Nat. Geosci., 3, 233–237, Doi https://doi.org/10.1038/Ngeo817, 2010.
Murray, B. J., O'Sullivan, D., Atkinson, J. D., and Webb, M. E.: Ice nucleation by particles immersed in supercooled cloud droplets, Chem. Soc. Rev., 41, 6519–6554, https://doi.org/10.1039/c2cs35200a, 2012.
Niemand, M., Möhler, O., Vogel, B., Vogel, H., Hoose, C., Connolly, P., Klein, H., Bingemer, H., DeMott, P., Skrotzki, J., and Leisner, T.: A Particle-Surface-Area-Based Parameterization of Immersion Freezing on Desert Dust Particles, J. Atmos. Sci., 69, 3077–3092, https://doi.org/10.1175/jas-d-11-0249.1, 2012.
O'Brien, R. E., Neu, A., Epstein, S. A., MacMillan, A. C., Wang, B., Kelly, S. T., Nizkorodov, S. A., Laskin, A., Moffet, R. C., and Gilles, M. K.: Physical properties of ambient and laboratory-generated secondary organic aerosol, Geophys. Res. Lett., 41, 4347–4353, https://doi.org/10.1002/2014gl060219, 2014.
O'Brien, R. E., Wang, B., Laskin, A., Riemer, N., West, M., Zhang, Q., Sun, Y. L., Yu, X. Y., Alpert, P., Knopf, D. A., Gilles, M. K., and Moffet, R. C.: Chemical imaging of ambient aerosol particles: Observational constraints on mixing state parameterization, J. Geophys. Res., 120, 9591–9605, https://doi.org/10.1002/2015jd023480, 2015.
O'Dowd, C. D., Facchini, M. C., Cavalli, F., Ceburnis, D., Mircea, M., Decesari, S., Fuzzi, S., Yoon, Y. J., and Putaud, J. P.: Biogenically driven organic contribution to marine aerosol, Nature, 431, 676–680, https://doi.org/10.1038/nature02959, 2004.
Pham, D. Q., O'Brien, R., Fraund, M., Bonanno, D., Laskina, O., Beall, C., Moore, K. A., Forestieri, S., Wang, X., Lee, C., Sultana, C., Grassian, V., Cappa, C. D., Prather, K. A., and Moffet, R. C.: Biological Impacts on Carbon Speciation and Morphology of Sea Spray Aerosol, ACS Earth Space Chem., 1, 551–561, https://doi.org/10.1021/acsearthspacechem.7b00069, 2017.
Prather, K. A., Bertram, T. H., Grassian, V. H., Deane, G. B., Stokes, M. D., DeMott, P. J., Aluwihare, L. I., Palenik, B. P., Azam, F., Seinfeld, J. H., Moffet, R. C., Molina, M. J., Cappa, C. D., Geiger, F. M., Roberts, G. C., Russell, L. M., Ault, A. P., Baltrusaitis, J., Collins, D. B., Corrigan, C. E., Cuadra-Rodriguez, L. A., Ebben, C. J., Forestieri, S. D., Guasco, T. L., Hersey, S. P., Kim, M. J., Lambert, W. F., Modini, R. L., Mui, W., Pedler, B. E., Ruppel, M. J., Ryder, O. S., Schoepp, N. G., Sullivan, R. C., and Zhao, D. F.: Bringing the ocean into the laboratory to probe the chemical complexity of sea spray aerosol, P. Natl. Acad. Sci. USA, 110, 7550–7555, https://doi.org/10.1073/pnas.1300262110, 2013.
Pruppacher, H. R. and Klett, J. D.: Microphysics of Clouds and Precipitation, Kluwer Academic Publishers, Dordrecht, 1997.
Raveh-Rubin, S.: Dry Intrusions: Lagrangian Climatology and Dynamical Impact on the Planetary Boundary Layer, J. Climate, 30, 6661–6682, https://doi.org/10.1175/jcli-d-16-0782.1, 2017.
Roy, P., Mael, L. E., Hill, T. C. J., Mehndiratta, L., Peiker, G., House, M. L., DeMott, P. J., Grassian, V. H., and Dutcher, C. S.: Ice Nucleating Activity and Residual Particle Morphology of Bulk Seawater and Sea Surface Microlayer, ACS Earth Space Chem., 5, 1916–1928, https://doi.org/10.1021/acsearthspacechem.1c00175, 2021.
Schnell, R. C.: Ice nuclei produced by laboratory cultured marine phytoplankton, Geophys. Res. Lett., 2, 500–502, 1975.
Schnell, R. C. and Vali, G.: Freezing nuclei in marine waters, Tellus, 27, 321–323, https://doi.org/10.1111/j.2153-3490.1975.tb01682.x, 1975.
Seber, G. A. F.: Multivariate Observations, John Wiley & Sons Inc., Hoboken, NJ, 1984.
Spath, H.: Cluster Dissection and Analysis: Theory, FORTRAN Programs, Examples, Halsted Press, New York, 1985.
Sprenger, M. and Wernli, H.: The LAGRANTO Lagrangian analysis tool – version 2.0, Geosci. Model Dev., 8, 2569–2586, https://doi.org/10.5194/gmd-8-2569-2015, 2015.
Storelvmo, T.: Aerosol Effects on Climate via Mixed-Phase and Ice Clouds, in: Annual Review of Earth and Planetary Sciences, Vol 45, edited by: Jeanloz, R. and Freeman, K. H., Ann. Rev. Earth Pl. Sc., 199–222, 2017.
Thompson, S. K.: Sample-size for estimating multinomial proportions, Am. Stat., 41, 42–46, https://doi.org/10.2307/2684318, 1987.
Tivanski, A. V., Hopkins, R. J., Tyliszczak, T., and Gilles, M. K.: Oxygenated interface on biomass burn tar balls determined by single particle scanning transmission X-ray microscopy, J. Phys. Chem. A, 111, 5448–5458, https://doi.org/10.1021/jp070155u, 2007.
Tomlin, J. M., Jankowski, K. A., Rivera-Adorno, F. A., Fraund, M., China, S., Stirm, B. H., Kaeser, R., Eakins, G. S., Moffet, R. C., Shepson, P. B., and Laskin, A.: Chemical Imaging of Fine Mode Atmospheric Particles Collected from a Research Aircraft over Agricultural Fields, ACS Earth Space Chem., 4, 2171–2184, https://doi.org/10.1021/acsearthspacechem.0c00172, 2020.
Tomlin, J. M., Jankowski, K. A., Veghte, D. P., China, S., Wang, P., Fraund, M., Weis, J., Zheng, G., Wang, Y., Rivera-Adorno, F., Raveh-Rubin, S., Knopf, D. A., Wang, J., Gilles, M. K., Moffet, R. C., and Laskin, A.: Impact of dry intrusion events on the composition and mixing state of particles during the winter Aerosol and Cloud Experiment in the Eastern North Atlantic (ACE-ENA), Atmos. Chem. Phys., 21, 18123–18146, https://doi.org/10.5194/acp-21-18123-2021, 2021.
Vali, G.: Quantitative evaluation of experimental results on heterogeneous freezing nucleation of supercooled liquids, J. Atmos. Sci., 28, 402–409, 1971.
Vali, G., DeMott, P. J., Möhler, O., and Whale, T. F.: Technical Note: A proposal for ice nucleation terminology, Atmos. Chem. Phys., 15, 10263–10270, https://doi.org/10.5194/acp-15-10263-2015, 2015.
Wagner, R., Ickes, L., Bertram, A. K., Els, N., Gorokhova, E., Möhler, O., Murray, B. J., Umo, N. S., and Salter, M. E.: Heterogeneous ice nucleation ability of aerosol particles generated from Arctic sea surface microlayer and surface seawater samples at cirrus temperatures, Atmos. Chem. Phys., 21, 13903–13930, https://doi.org/10.5194/acp-21-13903-2021, 2021.
Wang, B., Laskin, A., Roedel, T., Gilles, M. K., Moffet, R. C., Tivanski, A. V., and Knopf, D. A.: Heterogeneous ice nucleation and water uptake by field-collected atmospheric particles below 273 K, J. Geophys. Res., 117, D00V19, https://doi.org/10.1029/2012jd017446, 2012a.
Wang, B., Lambe, A. T., Massoli, P., Onasch, T. B., Davidovits, P., Worsnop, D. R., and Knopf, D. A.: The deposition ice nucleation and immersion freezing potential of amorphous secondary organic aerosol: Pathways for ice and mixed-phase cloud formation, J. Geophys. Res., 117, D16209, https://doi.org/10.1029/2012jd018063, 2012b.
Wang, B. and Knopf, D. A.: Heterogeneous ice nucleation on particles composed of humic-like substances impacted by O3, J. Geophys. Res., 116, D03205, https://doi.org/10.1029/2010jd014964, 2011.
Wang, B., O'Brien, R. E., Kelly, S. T., Shilling, J. E., Moffet, R. C., Gilles, M. K., and Laskin, A.: Reactivity of Liquid and Semisolid Secondary Organic Carbon with Chloride and Nitrate in Atmospheric Aerosols, J. Phys. Chem. A, 119, 4498–4508, https://doi.org/10.1021/jp510336q, 2015.
Wang, J., Wood, R., Jensen, M. P., Chiu, J. C., Liu, Y., Lamer, K., Desai, N., Giangrande, S. E., Knopf, D. A., Kollias, P., Laskin, A., Liu, X., Lu, C., Mechem, D., Mei, F., Starzec, M., Tomlinson, J., Wang, Y., Yum, S. S., Zheng, G., Aiken, A. C., Azevedo, E. B., Blanchard, Y., China, S., Dong, X., Gallo, F., Gao, S., Ghate, V. P., Glienke, S., Goldberger, L., Hardin, J. C., Kuang, C., Luke, E. P., Matthews, A. A., Miller, M. A., Moffet, R., Pekour, M., Schmid, B., Sedlacek, A. J., Shaw, R. A., Shilling, J. E., Sullivan, A., Suski, K., Veghte, D. P., Weber, R., Wyant, M., Yeom, J., Zawadowicz, M., and Zhang, Z.: Aerosol and Cloud Experiments in the Eastern North Atlantic (ACE-ENA), B. Am. Meteorol. Soc., 103, E619–E641, https://doi.org/10.1175/BAMS-D-19-0220.1, 2021.
Westbrook, C. D. and Illingworth, A. J.: The formation of ice in a long-lived supercooled layer cloud, Q. J. Roy. Meteor. Soc., 139, 2209–2221, https://doi.org/10.1002/qj.2096, 2013.
Wilbourn, E. K., Thornton, D. C. O., Ott, C., Graff, J., Quinn, P. K., Bates, T. S., Betha, R., Russell, L. M., Behrenfeld, M. J., and Brooks, S. D.: Ice Nucleation by Marine Aerosols Over the North Atlantic Ocean in Late Spring, J. Geophys. Res.-Atmos., 125, e2019JD03091, https://doi.org/10.1029/2019jd030913, 2020.
Wilson, T. W., Ladino, L. A., Alpert, P. A., Breckels, M. N., Brooks, I. M., Browse, J., Burrows, S. M., Carslaw, K. S., Huffman, J. A., Judd, C., Kilthau, W. P., Mason, R. H., McFiggans, G., Miller, L. A., Najera, J. J., Polishchuk, E., Rae, S., Schiller, C. L., Si, M., Temprado, J. V., Whale, T. F., Wong, J. P. S., Wurl, O., Yakobi-Hancock, J. D., Abbatt, J. P. D., Aller, J. Y., Bertram, A. K., Knopf, D. A., and Murray, B. J.: A marine biogenic source of atmospheric ice-nucleating particles, Nature, 525, 234–238, https://doi.org/10.1038/nature14986, 2015.
Wolf, M. J., Coe, A., Dove, L. A., Zawadowicz, M. A., Dooley, K., Biller, S. J., Zhang, Y., Chisholm, S. W., and Cziczo, D. J.: Investigating the Heterogeneous Ice Nucleation of Sea Spray Aerosols Using Prochlorococcus as a Model Source of Marine Organic Matter, Environ. Sci. Technol., 53, 1139–1149, https://doi.org/10.1021/acs.est.8b05150, 2019.