the Creative Commons Attribution 4.0 License.
the Creative Commons Attribution 4.0 License.
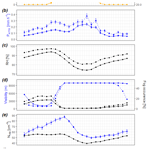
Occurrence and growth of sub-50 nm aerosol particles in the Amazonian boundary layer
Florian Ditas
Leslie A. Kremper
Luiz A. T. Machado
Meinrat O. Andreae
Alessandro Araújo
Henrique M. J. Barbosa
Joel F. de Brito
Samara Carbone
Bruna A. Holanda
Fernando G. Morais
Janaína P. Nascimento
Mira L. Pöhlker
Luciana V. Rizzo
Marta Sá
Jorge Saturno
David Walter
Stefan Wolff
Ulrich Pöschl
Paulo Artaxo
Christopher Pöhlker
New particle formation (NPF), referring to the nucleation of molecular clusters and their subsequent growth into the cloud condensation nuclei (CCN) size range, is a globally significant and climate-relevant source of atmospheric aerosols. Classical NPF exhibiting continuous growth from a few nanometers to the Aitken mode around 60–70 nm is widely observed in the planetary boundary layer (PBL) around the world but not in central Amazonia. Here, classical NPF events are rarely observed within the PBL, but instead, NPF begins in the upper troposphere (UT), followed by downdraft injection of sub-50 nm (CN<50) particles into the PBL and their subsequent growth. Central aspects of our understanding of these processes in the Amazon have remained enigmatic, however. Based on more than 6 years of aerosol and meteorological data from the Amazon Tall Tower Observatory (ATTO; February 2014 to September 2020), we analyzed the diurnal and seasonal patterns as well as meteorological conditions during 254 of such Amazonian growth events on 217 event days, which show a sudden occurrence of particles between 10 and 50 nm in the PBL, followed by their growth to CCN sizes. The occurrence of events was significantly higher during the wet season, with 88 % of all events from January to June, than during the dry season, with 12 % from July to December, probably due to differences in the condensation sink (CS), atmospheric aerosol load, and meteorological conditions. Across all events, a median growth rate (GR) of 5.2 nm h−1 and a median CS of 1.1 × 10−3 s−1 were observed. The growth events were more frequent during the daytime (74 %) and showed higher GR (5.9 nm h−1) compared to nighttime events (4.0 nm h−1), emphasizing the role of photochemistry and PBL evolution in particle growth. About 70 % of the events showed a negative anomaly of the equivalent potential temperature () – as a marker for downdrafts – and a low satellite brightness temperature (Tir) – as a marker for deep convective clouds – in good agreement with particle injection from the UT in the course of strong convective activity. About 30 % of the events, however, occurred in the absence of deep convection, partly under clear-sky conditions, and with a positive anomaly. Therefore, these events do not appear to be related to downdraft transport and suggest the existence of other currently unknown sources of sub-50 nm particles.
- Article
(2139 KB) - Full-text XML
-
Supplement
(576 KB) - BibTeX
- EndNote
New particle formation (NPF) refers to the nucleation of nanometer-sized molecular clusters from gaseous precursors and their subsequent condensational growth (e.g., Kulmala et al., 2004; Dal Maso, 2005; Kirkby et al., 2011; Kulmala et al., 2012; Kerminen et al., 2018). Under favorable atmospheric conditions, the newly formed particles grow through condensation of semi-volatile and low-volatility gases as well as coagulation into the cloud- and, thus, climate-relevant size range with diameters, D, larger than ∼ 80 nm (see definitions in Kulmala et al., 2012; Kerminen et al., 2018). NPF has been observed worldwide in the course of ground-based observations in different environments, such as rural and remote continental areas, urban environments, the Arctic and Antarctica, marine areas, and mountain sites (Kerminen et al., 2018, and references therein). A brief overview of the current knowledge on the occurrence of NPF worldwide and the chemical mechanisms involved can be found in Andreae et al. (2021). Its wide and frequent occurrence makes NPF a major and possible even dominant source of aerosol particle number concentrations and cloud condensation nuclei (CCN) on global scales (e.g., Merikanto et al., 2009; Spracklen et al., 2008; Nieminen et al., 2018; Yli-Juuti et al., 2020).
In the long list of locations where “classical NPF” has been detected in the planetary boundary layer (PBL) (Kerminen et al., 2018), the Amazon rain forest is a remarkable exception (e.g., Andreae, 2013; Varanda Rizzo et al., 2018; Wimmer et al., 2018). Here, events have been observed that indeed resemble the classical “banana plots” of NPF but differ clearly in the initial diameter of the growth curve. While the smallest diameters in, for instance, boreal forest are typically in the range of a few nanometers, the “Amazonian bananas” rather start between about 20 and 40 nm (Kulmala et al., 2012; Kerminen et al., 2018). Varanda Rizzo et al. (2018) discussed the occurrence of such sub-50 nm particle growth events in the Amazon and found them only in 3 % of the 749 d examined, associated mainly with convective downdrafts. Accordingly, the Amazonian bananas start at larger diameters and are comparatively rare relative to the classical events, e.g., in boreal forests (Nieminen et al., 2018; Dada et al., 2018).
This striking contrast to other environments has inspired researchers to investigate the underlying mechanisms that could explain the absence of NPF as well as alternative particle sources that sustain the Amazonian aerosol population. Reasons for the absence of NPF within the PBL could be the following:
-
suppression by isoprene (e.g., Kiendler-Scharr et al., 2009; Kanawade et al., 2011; McFiggans et al., 2019; Yli-Juuti et al., 2020), which is the most abundant volatile organic compound (VOC) in the Amazonian atmosphere (e.g., Andreae et al., 2018; Yáñez-Serrano et al., 2020);
-
the very low concentrations of inorganic precursor gases such as sulfur dioxide (SO2, being converted into sulfuric acid, H2SO4) as well as the bases ammonia (NH3) and amines (NR3) (Andreae et al., 1990; Trebs et al., 2004), which play key roles in the binary H2SO4–H2O and ternary NH3–H2SO4–H2O nucleation mechanisms (Kirkby et al., 2011; Andreae et al., 2021);
-
the high levels of relative humidity (RH), which have been associated with a low occurrence of NPF (e.g., Bonn and Moortgat, 2003; Hamed et al., 2011; Hyvönen et al., 2005).
The occurrence of NPF is dependent on the local conditions at individual sites, including meteorology, biogenic emissions, and air pollution levels, but regional and synoptic scales are also very important for this process. Particle growth events lasting on the order of hours are particularly influenced by larger geographic scales. Nieminen et al. (2018) emphasized that the NPF occurrence and growth rates (GRs) show a geographically inhomogeneous distribution, indicating that the underlying mechanisms are as manifold as complex. Typical atmospheric GR ranges from 1 to 12 nm h−1 (Yli-Juuti et al., 2020). Further, different meteorological conditions have been associated with the occurrence of NPF and particle growth. Specifically, photochemical reactions under daytime conditions have been regarded as a driving force for both nucleation and condensational growth (e.g., Nieminen et al., 2018; Kerminen et al., 2018; Hamed et al., 2011; Ma and Birmili, 2015). This is in line with a significantly higher occurrence of NPF under clear-sky conditions, as observed for instance in Hyytiälä, Finland, and British Columbia (Dada et al., 2017; Andreae et al., 2021). In addition, an association between the occurrence of NPF and convective clouds has been observed at different marine to continental sites (e.g., Perry and Hobbs, 1994; Clarke, 1992; Waddicor et al., 2012; De Reus et al., 2001; Wehner et al., 2015). Evidence of this phenomenon in the Amazon has also been reported by Andreae et al. (2018). While different potential explanations have emerged, the exact mechanisms, precursors, and spatial distribution in the context of clouds have remained unknown (Kerminen et al., 2018).
NPF has likely been altered as a result of industrialization, when anthropogenic emissions started to influence the atmospheric concentrations of trace species (Andreae et al., 2021). Relative to remote sites, rural and urban locations tend to show higher NPF frequencies (typically 10 %–30 % event days) and higher particle GR (4–12 nm h−1) (Kerminen et al., 2018; Nieminen et al., 2018). This relates to the fundamental question of whether atmospheric concentrations of certain, mainly anthropogenically derived, species such as H2SO4 have to exceed certain thresholds for NPF to occur. Recent evidence of pure biogenic ion-induced nucleation under controlled laboratory (Kirkby et al., 2016) and under real atmospheric conditions (Rose et al., 2018; Zhao et al., 2020) highlights possible mechanisms for NPF pathways in a clean atmosphere.
Accordingly, environments with low anthropogenic influence are of particular interest to investigate processes under conditions that approximate a preindustrial state of the atmosphere. Amazonia is an ideal outdoor laboratory for such investigations under pristine conditions (Hamilton et al., 2014; Pöhlker et al., 2018). Of particular relevance is the wet season with its episodic occurrence of pristine periods, which allows for the study of atmospheric processes – such as the occurrence of sub-50 nm particles (CN<50) – under conditions that approximate a preindustrial state of the rain forest atmosphere (Andreae et al., 2015). At the Amazon Tall Tower Observatory (ATTO) – which is located in a mostly untouched rain forest region and has become a landmark site for atmospheric research (Andreae et al., 2015; Pöhlker et al., 2019) – March to May represent the cleanest months of the year, with about 10 % of the time being considered pristine periods (Pöhlker et al., 2018).
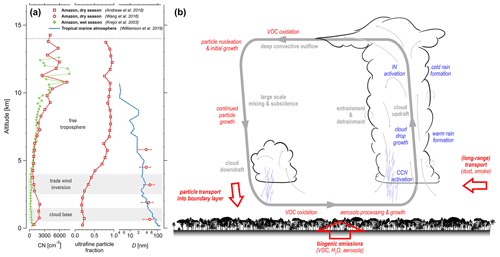
Figure 1Conceptual scheme of sources, redistribution, processing, and removal of volatile organic compounds (VOCs), aerosol particles and cloud condensation nuclei (CCN) over the Amazon (b) in combination with previously measured vertical profiles of particle concentrations and sizes (a). The scheme emphasizes the aerosol cycling in the course of deep convection, with an upward transport of VOCs and aerosol particles, new particle formation in the free troposphere with initial particle growth (Andreae et al., 2018), followed by the downward transport of sub-50 nm particles into the planetary boundary layer (Krejci et al., 2003; Wang et al., 2016). The three red arrows represent the main aerosol source categories in the Amazon, which are (i) primary and secondary particle formation from local and regional biogenic sources, (ii) long-range transport of dust, smoke, and other aerosols, and (iii) the vertical transport of sub-50 nm particles from aloft. The figure integrates concepts and data from various previous studies (i.e., Krejci et al., 2003; Baars et al., 2012; Wang et al., 2016; Andreae et al., 2018; Williamson et al., 2019).
Figure 1 illustrates the main sources of aerosol particles and CCN in Amazonia, which can be broadly grouped into the following three categories:
-
Biogenic particles are emitted by the rain forest ecosystem, which includes the release of primary biological aerosol particles (i.e., pollen, spores, bacteria, fragments). In addition, biogenic VOCs emitted by the ecosystem may undergo atmospheric oxidation, resulting in conversion into secondary organic aerosols (SOA). (e.g., Pöhlker et al., 2012; Huffman et al., 2012; Chen et al., 2015; Liu et al., 2016; Saturno et al., 2018a; Löbs et al., 2020; Prass et al., 2021).
-
Long-range transport of transatlantically advected African dust and pollution (e.g., Talbot et al., 1990; Pöhlker et al., 2018; Nascimento et al., 2021; Moran-Zuloaga et al., 2018; Holanda et al., 2020), as well as regional biomass burning smoke (Artaxo et al., 2013), which play an important role to increase the aerosol number concentration.
-
Driven by deep convective clouds, biogenic VOCs are transported into the upper troposphere (∼ 10 km), where VOC oxidation, nucleation of new aerosol particles, and initial particle growth occurs, fostered by low temperatures and a low preexisting aerosol surface area (Krejci et al., 2003; Andreae et al., 2018). Subsequently, the freshly formed particles are mixed downward into the PBL, where they continue to grow to CCN-relevant sizes (e.g., Krejci et al., 2003; Wang et al., 2016).
Several studies provide experimental and modeling support for the broad atmospheric relevance of UT particle production and the subsequent vertical mixing of the CN<50 (e.g., Krejci, 2003; Krejci et al., 2005; Wang et al., 2016; Andreae et al., 2018; Williamson et al., 2019; Leino et al., 2019; Zhao et al., 2020; Varanda Rizzo et al., 2018; Machado et al., 2021). Figure 1 shows the presence of a CN<50 pool in the Amazonian UT during the wet and dry seasons as well as an increase in D with decreasing altitude due to condensational particle growth. The downward motion of the CN<50 can be driven by strong convective downdrafts or weaker downward motions in stratiform cloud regions (Wang et al., 2016). Zhao et al. (2020) recently suggested that pure organic NPF based on biogenic VOCs dominates above 13 km, whereas ternary NPF involving organics and H2SO4 dominates between 8 and 13 km. In addition, an increase of sub-50 nm particles was observed, in particular in the early morning hours, suggesting a connection between these increased concentrations with vertical transport and deep convective clouds, as well as with lightning density. Major mechanistic questions regarding the vertical transport of the CN<50 remain open (e.g., Machado et al., 2021), however. This mechanism corroborates the fact that most of the observed sub-50 nm Aitken-mode particles in the PBL have relatively larger diameters (>20 nm) due to the aging process while transported from the free troposphere into the PBL. It means that the ion-induced biogenic nucleation in the uppermost troposphere potentially plays an important role and, therefore, substantially contributes to the particle population in the free troposphere and in the PBL (Wang et al., 2016; Andreae et al., 2018; Glicker et al., 2019).
This study aims to identify and characterize the occurrence of particle growth events in the size range from 10 to 50 nm within the PBL of central Amazonia. While previous studies have documented the occurrence and properties of freshly nucleated particles at high altitudes (Krejci et al., 2003; Andreae et al., 2018; Williamson et al., 2019), their growth in the course of downward transport (Wang et al., 2016), and the appearance of sub-50 nm particles in the PBL (Varanda Rizzo et al., 2018; Wimmer et al., 2018), major questions remain open. With this study, we take a step beyond the existing knowledge, based on more than 6 years of aerosol measurements and complementary meteorological and satellite observations. In particular, we focus on a statistically broad characterization of Amazonian particle growth events (Amazonian bananas) by means of GR, CS, seasonality, and diurnal cycle, as well as their relationship to meteorological variables and deep convection. We also document growth events under clear-sky conditions and thus in the absence of deep convective mixing. Therefore, the knowledge obtained here about the sub-50 nm particle growth events addresses an important gap in our understanding of the Amazonian aerosol life cycle and will help to constrain the CCN sources and properties in this globally important ecosystem.
2.1 The Amazon Tall Tower Observatory (ATTO) site
The Amazon Tall Tower Observatory (ATTO) is located 150 km northeast of Manaus, Brazil, in a forest reserve. Detailed descriptions of the site, its location, instrumentation, and scientific missions can be found elsewhere (Andreae et al., 2015; Pöhlker et al., 2019). At the ATTO site, the first aerosol measurements were initiated in 2011 (e.g., Pöhlker et al., 2012; Saturno et al., 2018b). Since 2014, multiple continuous measurements of physical and chemical particle properties have been established and gradually extended (e.g., Pöhlker et al., 2016, 2018; Holanda et al., 2020; Saturno et al., 2018a; Schrod et al., 2020).
2.2 Terminology
According to Pöhlker et al. (2016), we define the Amazonian seasons as follows: the wet season spans from February to May, followed by the wet to dry transition period (WtoD) including June and July. The dry season extends from August to November, followed by the dry to wet transition period (DtoW) including December and January. For the Amazonian submicron particle population, which is characterized by a multi-modal size distribution, we use the widely established terms Aitken mode (50–100 nm) and accumulation mode (100–1000 nm) (Pöhlker et al., 2016; Machado et al., 2021). In addition, we introduce the term sub-50 nm mode, defined as particles between 10 and 50 nm. We avoid using the term nucleation mode for this particle population as this term typically defines particles <25 nm and refers to an aerosol population relatively soon after nucleation (Kulmala et al., 2012; Nieminen et al., 2018). The sub-50 nm particles analyzed here, however, have experienced initial aging and growth to diameters between 10 and 50 nm already. As an abbreviation, we use CN<50 to refer to the particle fraction in the sub-50 nm mode. For the particle number concentrations in the individual modes, we use the symbols N<50, NAit, and Nacc. NCN is defined as the total particle number concentration.
2.3 Aerosol measurements
This study focuses on particle number size distributions (PNSDs) obtained from a scanning mobility particle sizer (SMPS) with an inlet located at 60 m above ground. The inlet used to sample the aerosols is installed on an 80 m high tower (02∘08.602′ S, 59∘00.033′ W; 130 m a.s.l.) at the ATTO site. The SMPS is manufactured by TSI Inc., and as classifiers we used model 3080 and, later, model 3082, coupled to a condensation particle counter (CPC) 3772. The inlet height was chosen to be approximately 30 m above the average canopy height, which enables measurements close to the canopy without direct contact with the largest trees. The SMPS is located in an air-conditioned laboratory container at the foot of the mast. Sample air is transported through a 25 mm diameter stainless steel tube (finetron tubes, Dockweiler AG, Neustadt-Glewe, Germany) and dried to a relative humidity (RH) below 40 %. An automatic regenerating silica gel adsorption aerosol dryer, as described in Tuch et al. (2009), was installed upstream of the instruments in 2014 and was replaced by a custom-built and automated condensation aerosol dryer in March 2020. For more detailed information on the aerosol measurements setup, see Andreae et al. (2015).
The SMPS measurements cover the particle size range from 10 to 400 nm and yield a temporal resolution of 5 min. The PNSD data cover more than 6 years, from February 2014 to September 2020, covering 1596 measurement days and comprising 426 272 sample runs in total. The data coverage of ∼ 67 % over the entire time frame (i.e., February 2014 to September 2020) can be considered a robust data foundation and statistical basis for the observations and conclusion presented here.
The sizing accuracy of the SMPS was frequently checked with monodisperse polystyrene latex particles. Additionally, the data quality was continuously verified by complementary measurements with a condensation particle counter (CPC, model 5412, Grimm Aerosol Technik, Ainring, Germany) measuring the total particle number concentration (NCN) >4 nm. All particle data were visually inspected for malfunction and contamination, further corrected for standard temperature and pressure (STP, 273.15 K, 1013.25 hPa) as well as inlet transmission efficiency according to Moran-Zuloaga et al. (2018). The PNSD data were used for this analysis if NCN from SMPS and CPC agreed within 15 %.
CN<50 are particularly prone to diffusion losses at surfaces (e.g., the tube surfaces of the inlet lines) (von der Weiden et al., 2009). Accordingly, the generally sparse occurrence of CN<50 in the Amazon frequently raises questions about whether these results are (systematically) biased by unaccounted diffusion losses (e.g., in the 60 m long inlets). The observations outlined below suggest the absence of large and unaccounted for particle losses in the size range that is particularly relevant here (i.e., 10 to 100 nm) and further indicate that the observed PNSDs correctly reflect the actual atmospheric aerosol distribution:
-
The inlet and particle transport is optimized for high particle transmission efficiency and short residence time of the sample air. According to the particle loss calculator provided by von der Weiden et al. (2009) and corresponding sensitivity tests, the 50 % transmission efficiency of the inlet at the lower end of the PNSD is reached at D50 %≈8 nm. All PNSDs in this study have been corrected for diffusional, sedimentation, and inertial losses according to von der Weiden et al. (2009).
-
Experiments with the SMPS running at the 60 m inlet line and a separate and mobile CPC running without inlet lines at the height of 60 m directly on the tower agreed well, which underlines that no significant fractions of CN<50 were lost in the inlet lines.
-
Finally, the PNSDs with the sparse particle occurrence <20 nm reported here agree well with results in previous studies (e.g., Gunthe et al., 2009; Varanda Rizzo et al., 2018).
2.4 Multi-modal log-normal fitting of PNSDs
Each measured PNSD was fitted by a multi-modal log-normal distribution function, according to Heintzenberg (1994):
where Dp is the particle diameter, and n is the number of aerosol size modes to be fitted (with n≤3; see Sect. 2.2). Each mode is characterized by three main parameters: the mode number concentration Ni, the mode geometric median diameter Di, and the mode geometric standard deviation σi. A script was developed – similarly to the procedure in Hussein et al. (2005) – to provide an automatic user-free decision algorithm to obtain the size modes according to the following steps:
-
In the first step, the maximum particle number concentration and the corresponding particle diameter, Ddom, are determined within the particle number size distribution. Within the size range of −30 % to +20 % of Ddom, a one-modal log-normal distribution is fitted.
-
The first one-modal fit is assigned as accumulation (), Aitken (), or sub-50 nm mode (), and two additional one-modal log-normal distributions are added for the remaining modes. The parameters of the three log-normal distributions are then varied within the mentioned diameter range and the standard deviation and for concentrations less than the maximum of the particle number size distribution.
-
The geometric standard (σi) deviation of all modes was constrained within the range of 1.1 to 1.55, which was optimized for the ATTO conditions.
-
Subsequently, a joint optimization of the previously obtained fit parameters (Di, σi, and Ni) for the modes was conducted. The procedure is developed by fixing two of the modes and leaving the third free so that its parameters are again optimized by minimizing the least-squares. The optimization order in this process was to optimize the sub-50 nm mode, then the accumulation mode, and, finally, the Aitken mode. In this case, all the free diameters of the modes could vary between 0.5Di and 1.5Di. As a measure of fitting quality, for each particle number size distribution, the algorithm compares the particle number concentrations of each bin of the measured and the fitted curve and obtains the R2 value. We considered only fits in which the agreement returned R2>0.8, which means that about 97 % of the data are covered by the developed mode fitting. Examples of fits can be seen in Fig. S1 in the Supplement.
-
Comparisons between the integrated particle number concentration from the SMPS measurements (Nconc, SMPS) and log-normal fitted size distributions () were made to further assure the quality of the fits. Within this data set, on average, fits with R2=0.97 were obtained, which yielded a linear fit of Nconc,SMPS and with R2=0.99 (Fig. S2).
2.5 Identification of particle growth events
We analyzed the occurrence and properties of particle growth events (Amazonian banana plots) in the sub-50 nm and Aitken-mode size range. Characteristic examples of such growth events are shown and discussed in Sect. 3. The growth event identification is based on the following main steps:
-
All data were smoothed to eliminate single exceptionally high or low values to avoid possible bias due to short intense particle peaks or dips. The moving window has two dimensions: one in time and the other in size. The SMPS measurements last 5 min to get a full-size distribution. In order to reduce noise, we average the time window at 25 min. The second parameter is the particle size window, which accounts for five SMPS bins. This is also made to reduce noise in terms of particle size. These two choices were shown as ideal to get reliable data, following suggestions by Kulmala et al. (2012). We also performed several tests to verify if sudden events were missing and found that, in general, the method could cover them without significant losses.
-
All PNSD data were divided into 24 h subsets.
-
Particle growth event days were then automatically flagged based on the guidelines in Kulmala et al. (2012). These guidelines were slightly modified by increasing the size threshold for the initial growth event identification from 20 to 40 nm to account for the characteristics of the Amazonian banana plots and PNSDs.
-
Further following Kulmala et al. (2012), the total particle number concentration of particle diameters >40 nm was then subtracted from the total particle number concentration of particle diameters . Positive values in the PNSDs are marked as regions of interest for the occurrence of CN<50 that could result in particle growth events. Days fulfilling these criteria are flagged as particle growth event days.
-
This method is sensitive to the integral particle number concentration in the Aitken and accumulation modes and their seasonal variation, which might result in false positive or false negative event flagging. To account for that, the results from the automated identification routine were visually inspected, and potentially misinterpreted events were excluded from the analysis. The inspection followed the procedure described in Dal Maso (2005), in which a particle growth event is characterized by (i) the appearance of a distinct new mode of particles in the PNSD, (ii) the particle size inside the sub-50 nm mode, (iii) the mode prevailing for more than 1 h, and (iv) it showing signs of growth in time.
2.6 Growth rate and condensation sink
The GR and the condensation sink (CS) – both important physical parameters in the characterization of growth events – were calculated following the procedures of Dal Maso (2005) and Kulmala et al. (2012). The GR is defined as the rate at which the mean geometric diameter Dp of the CN<50 population changes linearly with time:
where is the geometric diameter of the sub-50 nm mode obtained by the multi-modal fit at the beginning of the growth event at time t1, and is the geometric diameter at the end of the growth event at time t2. Thereby, the beginning of a growth event is defined as the moment at which Dp starts to increase. The end of a growth event is reached when either (i) Dp (10–50 nm) stops growing, (ii) the growth is interrupted due to sudden changes in air masses, or (iii) Dp reaches the Aitken mode – in this case, we selected D2 as the last observed growth Dp inside the sub-50 nm mode. There were a few events in which the growth stopped for a while and, afterward, restarted again. In these cases, we considered the second growth to be a new growth event. The growth events considered in this study have a duration of at least 1 h.
A moving average smoothing filter was applied at the mean geometric diameter interval , and the fit was obtained by applying a linear model fit at the referred diameter interval. The model returned the following parameters: R2, p value, and GR. To assure the data quality during the analyses, fits were statistically tested, and only fits with R2>0.6 and p value <0.05 were accepted. Additionally, we performed visual inspections of the quality of each of the fits. It is worth mentioning that fits statistically tested with R2>0.6 were able to represent the widest possible variability of growth events, without compromising the analyses.
The CS was calculated from the particle number concentration as (Dal Maso et al., 2002)
where Ni is the particle concentration at the diameter of the ith size bin, D is the diffusion coefficient of the precursor condensable vapor, and βm is the transition-regime correction (Fuchs and Sutugin, 1971), defined as
which depends on the dimensionless Knudsen number, . The Kn parameter represents the ratio of two length scales, where λ is the effective mean free path of the vapor molecules in the gas (Dal Maso et al., 2002).
Physically, CS is a parameter that quantifies the ability of particles to remove condensable vapors from the atmosphere, incorporating them into the particle population and directly influencing the particle growth process. In this study, CS was calculated assuming D=0.117 cm−2 s−1, i.e., the value for sulfuric acid (H2SO4) (Gong et al., 2008), which is commonly used in the literature, allowing comparisons to other studies. We used the term CSgrowth as the average CS during the particle growth event.
2.7 Meteorological parameter measurements
The meteorological parameters, air temperature (T), incoming shortwave radiation (SW), rainfall (PATTO), air pressure (p), and relative humidity (RH) were measured at an 80 m high tower (02∘08.647′ S, 59∘59.992′ W; 130 m a.s.l.) located approximately 100 m from the ATTO aerosol mast. The measurements performed at the 80 m tower ranged from 2013 to 2018. Specifically, SW and PATTO were measured at the top of the tower, whereas T, p, and RH were measured at 55, 55, and 81 m, respectively. From January 2019 to September 2020, the meteorological parameters air temperature (T), rainfall (PATTO), air pressure (p), and relative humidity (RH) were measured at the 325 m ATTO Tall Tower with a compact weather station (Lufft, WS600-LMB, G. Lufft Mess- und Regeltechnik GmbH, Fellbach, Germany). Overall, meteorological parameters span the time frame from May 2013 to September 2020. Furthermore, an optical fog sensor (OFS; Eigenbrodt GmbH, Königsmoor, Germany) measured the near-field visibility from September 2014 to December 2018 at the height of 50 m. Fog occurrence is defined as visibility below 5000 m, which represents a threshold for light fog. Detailed information on the meteorological instruments can be found in Andreae et al. (2015).
2.8 Equivalent potential temperature
Variations of the equivalent potential temperature, θe, have been used as a proxy to indicate downdraft occurrences (Machado et al., 2002; Betts et al., 2002; Varanda Rizzo et al., 2018; Wang et al., 2016; Gerken et al., 2016). θe quantifies the temperature of an air parcel, when lifted to a certain height where it condenses (characterized by its lift temperature, TL), releasing the latent heat, and lowered adiabatically to 1000 hPa. In this study, θe was calculated from meteorological parameters measured in situ and was analyzed similarly to Wang et al. (2016) and Varanda Rizzo et al. (2018), using the definition described in Bolton (1980) as
where Tk is the ambient temperature in Kelvin, p and r are the ambient pressure (hPa) and the water mixing ratio (g kg−1), respectively, and TL is the lifting condensation level temperature in Kelvin. To obtain the variations in θe, it was necessary to subtract seasonality and diurnal variations.
The steps of this process are illustrated in Fig. S3 and are described as follows: was obtained by subtracting the mean seasonal trend values, in which we considered both wet and dry seasons, for each year of the time series. Then, the calculated mean diurnal cycle of was subtracted from , at the same time of the day, resulting in a new time series: . The quantity is the anomaly in θe and represents the deviation of θe from its expected value for that time of the day and season. Values of are a proxy for the occurrence of downdrafts and indicate a decrease in θe due to air masses from the free troposphere that enter the PBL, typically related to the occurrence of rain (Wang et al., 2016; Varanda Rizzo et al., 2018). Other processes may also be related to a decrease in θe, such as evaporation of rainfall, river breeze, and advection mechanisms.
It should be mentioned that two time series of meteorological data were used to calculate : the first one, with measurements conducted close to the canopy (2013–2018), and the second one, with measurements conducted at 325 m elevation (2019–September 2020). This was necessary because meteorological data are not available at the 80 m tower for the final period of analysis. The consistency of the calculation was verified by comparing for a 2-month period with overlapping measurements at the two height levels (January and February 2019), as shown in Fig. S4. Figure S5 shows the correlation between obtained at the two levels, with the statistical results of the comparison. Although there are very small differences for single pairs of measurements, the overall agreement is reasonably good and, therefore, does not impact the conclusions of the analysis. These results encouraged us to use the meteorological data measured at 325 m height, which enabled us to extend the data analysis to the years 2019 and 2020. We can not rule out a very fine-scale stratification phenomenon close to the canopy, as observed by Zha et al. (2018), which could have some influence on but to a minor extent, as observed by the comparison analysis. Further studies are required to examine this aspect in detail for the ATTO site.
2.9 GOES-16 cloud brightness temperature
This study uses infrared brightness temperature (Tir) data obtained by the Geostationary Operational Environmental Satellite (GOES), GOES-16, from November 2017 to April 2020. This data set comprises a total of 914 d with measurements every 10 min, as an indication of the troposphere's meteorological conditions. The Advance Baseline Imager (ABI) – a state-of-the-art 16-band radiometer on board GOES-16 – was employed in this study, specifically, Band 13, the infrared window at 10.3 µm. These measurements are less sensitive than other infrared bands to gas absorption, which allows the cloud-top brightness temperature to be estimated. An area of 3 × 3 pixels centered at ATTO was selected for obtaining the time series of Tir, representing around 6.0 × 6.0 km2. Meteorological conditions representing shallow clouds/clear sky are described by a warm Tir and deep-convection conditions by a cold Tir. Here, we considered Tir >280 K to be a nearly clear-sky condition, 245 K to correspond to shallow clouds and cumuliform clouds, and Tir <245 K to correspond to all convective clouds associated with deep convection (Machado and Rossow, 1993; Machado et al., 2002). Tir under nearly clear-sky conditions corresponds roughly to the temperature in the PBL.
3.1 Particle number size distributions for wet and dry season
In agreement with previous studies, our long-term PNSD measurements showed the distinct characteristics of the Amazonian wet- and dry-season aerosol populations (e.g., Roberts et al., 2001; Gunthe et al., 2009; Artaxo et al., 2013; Pöhlker et al., 2016; Varanda Rizzo et al., 2018). We chose a different representation of the typical PNSD shapes in Fig. 2 by showing them as frequency distributions (FDs). The PNSDs differ significantly between both seasons: during the wet season, clear Aitken and accumulation modes stand out, separated by a distinct Hoppel minimum (Hoppel et al., 1986). On average, the Aitken mode is centered at 71 nm, the Hoppel minimum is centered at 102 nm, and the accumulation mode is centered at 153 nm. In contrast, Fig. 2b shows the typical dry-season PNSDs characterized by a strong mono-modal shape with a dominating accumulation mode, reflecting the prevalence of biomass burning pollution (e.g., Rissler et al., 2006; Brito et al., 2014). On average, the accumulation mode is centered at 146 nm. In addition, the distribution in Fig. 2b reveals a contribution of the Aitken mode, centered at 68 nm (mean) and visible as a small shoulder on the dominant accumulation mode.
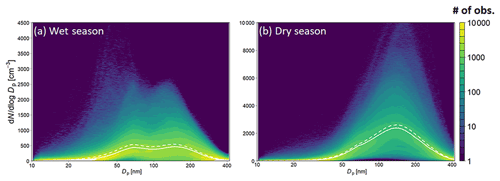
Figure 2Frequency distributions (FDs) of particle number size distributions (PNSDs) for the Amazonian wet (a) and dry season (b). The data basis comprises 6.5 years of SMPS data from February 2014 to September 2020. The color code denotes the number of observations. Solid lines represent the median and dashed lines the mean PNSDs. Please note the different y axes. The wet-season PNSD shows pronounced Aitken and accumulation modes. An overwhelming accumulation mode dominates the dry-season PNSD. The wet- and dry-season FDs of PNSDs emphasize a comparatively sparse occurrence of CN<50, which form a weak but distinct mode below 50 nm.
Regarding the abundance of CN<50, the FDs in Fig. 2 reveal that aerosols in this size range are rather sparse – though not absent – during both seasons. The occurrence of CN<50 in central Amazonia along with the absence of “classical” NPF, as is detected, e.g., in the Scandinavian boreal forests and shown in, e.g., Kulmala et al. (2004), Heintzenberg et al. (2017), Kerminen et al. (2018), and Dall'Osto et al. (2018), is well documented in the literature (e.g., Roberts et al., 2001; Pöhlker et al., 2016; Varanda Rizzo et al., 2018). Although occurring sparsely, the episodic presence of CN<50 causes a distinct mode below about 50 nm, noticeable in the wet-season FDs in Fig. 2a. However, the CN<50 do not show up clearly in the corresponding mean and median PNSDs. It is further worth noting that the PNSDs under the remote rain forest conditions at ATTO as shown here differ significantly from PNSDs that were obtained in the rain forest atmosphere with an influence of the urban emission plume from Manaus (e.g., Cirino et al., 2018; Fan et al., 2018; Wimmer et al., 2018; Glicker et al., 2019). These urban-influenced PNSDs are characterized by strongly enhanced particle concentrations below about 20 nm. As the mean and median PNSDs do not sufficiently reflect the abundance and properties of CN<50 in Fig. 2, the following paragraphs summarize the in-depth analysis that allowed us to extract their event characteristics, seasonal and diurnal variability, and estimated significance.
3.2 Particle growth event characterization
The abundance of CN<50, which show up as a weak, though noticeable, mode during the wet season (see the overall outline in Fig. 2a), results from the episodic occurrence of CN<50 events and their subsequent growth. Figure 3 shows a typical example of an Amazonian banana plot representing two subsequent growth events, as frequently observed at ATTO. The first example in Fig. 3 starts in the morning hours around 08:30 local time (LT), with an average initial diameter slightly larger than 30 nm. The particles grow for about 4 h, reaching the Aitken mode size range up to ∼ 60 nm. On the same day, a second growth event starts around noon, with an average initial growth diameter slightly larger than 20 nm, growing during the afternoon hours. The initial diameters at the onset of the growth events in Fig. 3 are well above the lower size limit of the SMPS (i.e., 10 nm), which implies that the event characterization is not distorted or limited by the effectively measured size range.
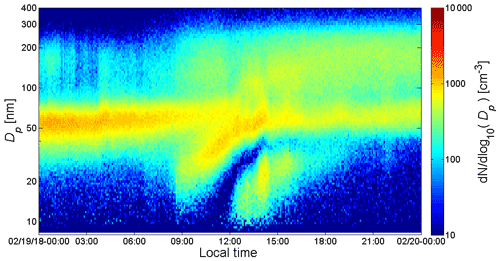
Figure 3Characteristic examples of two CN<50 growth events at ATTO on 19 and 20 February 2018. The temporal evolution of the particle number size distribution (PNSD) is shown as a heat map, emphasizing the pronounced Aitken mode as well as particle growth events from the sub-50 nm particle to the Aitken mode during daylight.
The growth events shown here resemble the events reported by Wang et al. (2016). Note that in all previous studies in Amazonia, the growth events were observed during the wet season, suggesting that this event type is a typical wet-season phenomenon associated with precipitation (Zhou, 2002; Wimmer et al., 2018; Varanda Rizzo et al., 2018). The procedure described in Sect. 2.5 returned 254 characteristic particle growth events on 217 of the 1596 measurement days, corresponding to a frequency of occurrence of ∼ 14 % for event days. For the entire measurement period (February 2014–September 2020) this corresponds to about 30 event days per year. The events have a clear seasonality, with more cases in the wet season, corresponding to ∼ 88 % of events from January to June, while in the dry season from July to December, only ∼ 12 % were observed. Additional aspects of seasonality are discussed in Sect. 3.3.
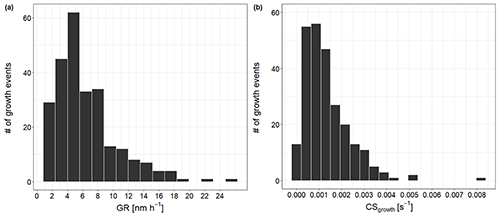
Figure 4Histograms showing the frequency distribution of the growth rate, GR (a), and condensation sink during the growth events, CSgrowth (b), for all observed 254 particle growth events.
The GR frequency distribution in Fig. 4a shows a clear peak centered around the median of 5.2 nm h−1. The median GR of this study agrees well with the median GR of 5.5 nm h−1 obtained by Varanda Rizzo et al. (2018). Figure S6a contrasts the median GR obtained at different sites in Amazonia and worldwide and shows that the median GR from this study is within the GR ranges obtained at remote boreal (0.5 to 5.3 nm h−1) or polar sites (0.2 to 5.5 nm h−1). The CSgrowth frequency distribution in Fig. 4b shows a clear peak centered around the median of 1.1 × 10−3 s−1. In contrast, the median CS calculated for all observation days is 3.2 × 10−3 s−1, which corroborates that particle growth events at remote sites are expected when CS values are low (Fig. 6d). The Amazonian CS also agrees with what is observed in other remote regions (Fig. S6b). For example, boreal sites have an average CS ranging from 9.8 × 10−4 to 3.9 × 10−3 s−1 (Kerminen et al., 2018).
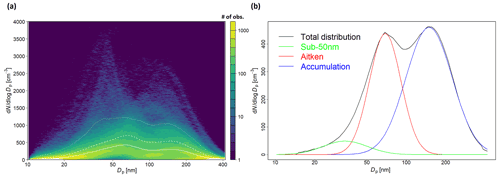
Figure 5(a) Frequency distribution of particle number size distributions exclusively for growth event periods. The color code denotes the number of observations, solid lines indicate the median, and dashed lines indicate the 75th and 90th percentiles. The PNSDs for the growth events show a high frequency of CN<50 and Aitken size modes. The FDs do not show any evidence of open PNSDs towards the lower detection limit. (b) Median wet-season PNSD (black line), showing its three log-normal modes: in green, the sub-50 nm size particle mode; in red, the Aitken mode; in blue, the accumulation mode; and in black, the total median distribution. The adjusted R2 obtained for the calculated multi-modal log-normal fit is 0.99.
Figure 5a shows the FD of PNSDs exclusively for the periods of growth events, starting 3 h before the event's onset and lasting until the time when growth stopped being observed (according to Sect. 2.6). Figure 5b shows the separated CN<50, Aitken, and accumulation modes for the median wet season. The characteristic multi-modal shape of the wet-season PNSDs stands out. The FD for the growth events further underlines the sparse particle abundance below 20 nm. Figure 5b shows that the Aitken and accumulation modes are the dominant modes of the median wet-season PNSD. The CN<50 mode, although small and not readily perceptible from the median PNSD, has a significant contribution, however, and is centered at nm, with . For comparison, the diameters selected as the initial values for the growth events, Dp,i, have a median value of 26.1 nm, with 25th and 75th percentiles of 19 and 33 nm, respectively. The Aitken mode of the median distribution is centered at DAIT=69 nm, with σAIT=1.3, and the accumulation mode is centered at DACC=149 nm, with σACC=1.5. This clearly shows that the initial diameter of growth events in the Amazon is typically larger than reported in other regions (Nieminen et al., 2018).
3.3 Seasonality
The pronounced atmospheric seasonality in central Amazonia has been characterized by means of meteorological, aerosol, and cloud microphysical data in previous studies (e.g., Pöhlker et al., 2018, 2019; Moran-Zuloaga et al., 2018; Saturno et al., 2018a). Figure 6a shows the typical seasonality of precipitation at ATTO, PATTO. The highest rain rate occurs during the wet season, with PATTO peaking in March and April, while the minimum in PATTO occurs between July to September. A similarly pronounced seasonality can be found in various aerosol properties. The NCN in the size range between 10 to 400 nm had its minimum in the wet-season months of March and April, with a median of ∼ 280 cm−3, and its maximum in the dry-season months of August to November, with a median of ∼ 1400 cm−3 (Fig. 6c).
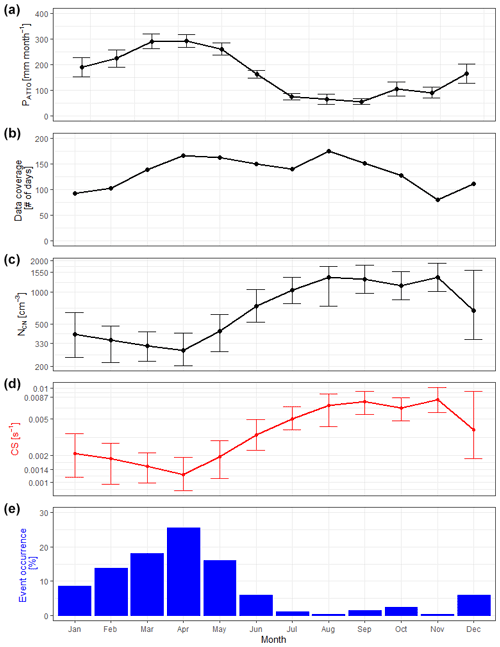
Figure 6The seasonal cycles of selected meteorological and aerosol parameters presented as monthly averages for the entire observation period from February 2014 to June 2020. From top to bottom: (a) PATTO monthly rain measured at ATTO, where error bars denote the standard deviation. (b) SMPS data coverage as the number of measurement days. (c) Median total particle number concentration in the size range of 10 nm < D < 400 nm, calculated from SMPS data, where error bars denote the interquartile range (please note the logarithmic scale). (d) Median condensation sink per month calculated from SMPS data, where error bars refer to the interquartile range (please note the logarithmic scale). (e) Annual cycle of the occurrence of CN<50 particle growth events.
The same pattern can be found in the monthly median CS in Fig. 6d. The strong seasonal differences in the physical aerosol properties – here manifested in a wide range of NCN and different PNSD shapes – have a substantial influence on the concentration of available CCN and, thus, cloud microphysical processes in the Amazon Basin (Pöhlker et al., 2016, 2018). These results agree well with long-term measurements at another central Amazonian site (i.e., the ZF2 site) presented by Varanda Rizzo et al. (2018) and allow to put earlier campaign-wise measurements into a broader context (e.g., Roberts et al., 2001; Roberts, 2003; Zhou, 2002; Rissler et al., 2004, 2006; Martin et al., 2010).
Figure 6e shows the seasonal pattern in the CN<50 growth event frequency. We found the highest frequencies during the wet season, peaking in April with about 26 %, and dropping down during the transition period (WtoD, June and July) to a minimum with almost zero events in August. The growth event occurrence stayed remarkably low during the dry-season months, with frequencies mostly below 3 % from July to November. Frequency levels increase again during the transition period (DtoW, December and January). The seasonality in growth event occurrence corresponds well with the seasonality in monthly rainfall and appears inversely related to the seasonality in NCN and CS. This agrees with previous studies in the Amazon, suggesting a close link between generally low particle concentrations and the appearance of CN<50- and Aitken-mode particles in the PBL (e.g., Krejci, 2003; Wang et al., 2016). The low CS might further favor the characteristic growth patterns of these events through the condensation of semi-volatile and low-volatility gaseous compounds on the CN<50 particle fraction as available surfaces (see example in Fig. 3a) (Dal Maso, 2005; Dal Maso et al., 2007; Dada et al., 2017; Kerminen et al., 2018; Nieminen et al., 2018; Wiedensohler et al., 2019).
The NCN, CS, and growth event occurrence in Fig. 6 are all based on the same multi-year SMPS data set. The underlying data availability is documented in Fig. 6b as the number of valid measurement days. To provide a seasonal overview, Table 1 shows statistical information on GR and CSgrowth during the observed growth events, separated by the month of the year. In the wet season, when ∼ 88 % of all growth events occurred, the GR values fluctuate around the median GR of 5.2 nm h−1. The CSgrowth shows a similar behavior, fluctuating around 1.1 × 10−3 s−1. The lowest median GR of the wet season is found in March, with 3.7 nm h−1, while the lowest CSgrowth of the wet season is found in April, with 8.0 × 10−4 s−1. The number of particle growth occurrences drops in the dry season, comprising only ∼ 12 % of the total growth events. During December, in the transition from the dry to wet season, the percentage of growth events starts to increase to ∼ 6 %. The average CSgrowth (during growth events, Table 1) in the dry season is lower compared to the average monthly CS (see Fig. 6), indicating that growth events occur on days with a cleaner atmosphere compared to average dry-season conditions. The only exceptions are the events from October, whose median CSgrowth was 4.4 × 10−3 s−1.
Table 1Monthly median with 25th and 75th percentiles (in parentheses) of GR and CSgrowth. The percentage of growth events in each month is also presented. Note that the statistics shown refer to the growth events only.
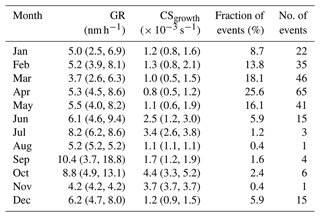
Table 2Median and 25th and 75th percentiles (in parenthesis) for Tir, , Dp,i, GR, and CSgrowth for each hourly group. Tir, , and Dp,i correspond to the onset of the particle growth event.

As outlined above, our results indicate that the occurrence of particle growth events is linked to the pronounced seasonality of the aerosol properties and the related underlying meteorological conditions and prevailing air mass history. In the dry season, regional and long-range-transported biomass burning aerosol from the Southern Hemisphere dominates the aerosol population in the lower troposphere (e.g., Moran-Zuloaga et al., 2018; Saturno et al., 2018b; Holanda et al., 2020). The resulting strong accumulation mode and, hence, the high CS likely suppress the growth of the smaller particles in the sub-50 nm mode. With the increase in precipitation (thus, in wet deposition) and the change in the average air mass history towards northern hemispheric origins, less polluted air masses arrive at the ATTO site (e.g., Moran-Zuloaga et al., 2018; Pöhlker et al., 2018). The number of particles of the accumulation mode and CS decreases significantly, coinciding with an increase in the occurrence of growth events.
3.4 Diurnal trends
The diurnal patterns of the growth event occurrence are shown in Fig. 7 in relation to meteorological parameters, such as air temperature (T), SW, PATTO, RH, and near-field visibility representing fog. Note that we contrasted the diurnal cycles for the entire observation period (i.e., February 2014 to September 2020, shown as solid lines) and the wet-season months (i.e., February to May, shown as dashed lines), since the majority of the growth events are observed during the wet season. The meteorological variables, T, SW, RH, and visibility were not discriminated with respect to days with and without events because no significant differences between event and non-event days were observed. Possible effects of deep convection, associated rainfall, and cloudiness are investigated in Sect. 3.5. In the diurnal cycles considering the whole period of observations, T and SW show the typical tropical rain forest conditions with about 12 h daylight and a remarkably low amplitude in T, spanning on average only 5 ∘C. Rainfall is most intense in the afternoon hours, with the highest precipitation intensity at 15:00 LT. The data also show a secondary maximum in the early morning, which has been associated with nocturnal long-lived mesoscale systems (Machado et al., 2021). The RH levels reach on average 100 % during the early morning and decrease during the day to around 75 %. Fog typically occurs in the second half of the night and often in the early morning before sunrise (i.e., between 03:00 and 07:00 LT), when T is lowest. Sporadically, fog also occurs shortly after rain showers, which is not reflected in the average conditions.
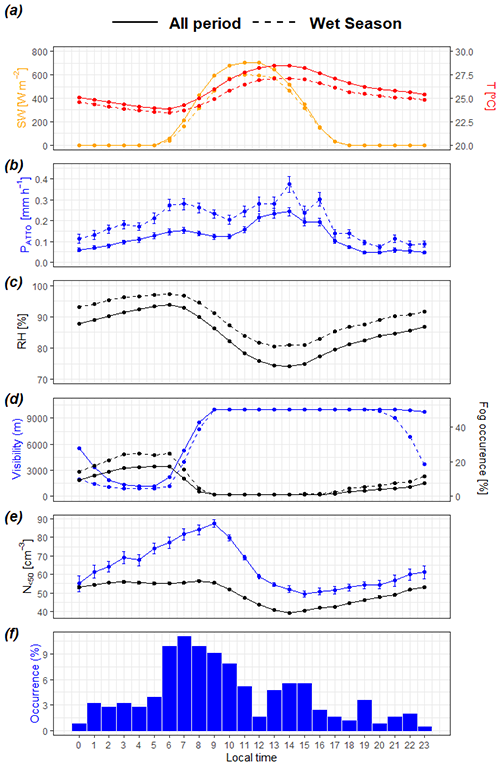
Figure 7Diurnal cycle of selected meteorological parameters and the diurnal occurrence of particle growth events. From top to bottom: (a) incoming shortwave radiation (SW, orange) and air temperature (T, red) at 26 m (canopy level) height, (b) average local rain rate (PATTO, blue), (c) relative humidity (RH) at 26 m, (d) visibility (blue) measured by a fog monitor and fog occurrence (black) with lines representing the first quartile, (e) median diurnal cycle of N<50 for all data (black) and only for the days on which particle growth events were observed (blue), and (f) the diurnal cycle of the particle growth event onsets. All error bars denote the standard error. The data shown represent all observations (full lines) and wet-season subsets (dashed lines).
Figure 7e shows the diurnal cycle of the median CN<50 number concentration, N<50, during the particle growth event days (blue line) and for comparison, the median diurnal cycle of N<50 comprising all measured PNSDs (black line). The particle concentration on growth event days is somewhat higher than that including all analyzed PNSDs, with median daily values and an interquartile range of 64 (38–108) cm−3, compared to 49 (29–81) cm−3 for all days. The diurnal cycles of both the particle concentration during growth events and for the entire measurement period also show some similarities. Around 09:00 LT in the morning, there is a remarkable decrease until noon, which is followed by an increase towards midnight. The decrease starting in the late morning is likely due to increased mixing after the breakup of the nocturnal boundary layer and the development of a well-mixed boundary layer. Towards the late afternoon, the well-mixed layer is then shrinking due to decreasing solar radiation. The N<50 diurnal cycle for growth event days shows further a strong increase from midnight to 09:00 LT, peaking at N<50=88 cm−3, compared to N<50=56 cm−3 at the same time for the total data. At this time, the difference between the growth event particle concentration and the average is largest and coincides with the highest occurrence of growth events.
The diurnal cycle of the growth event onsets has a rather broad maximum in the early morning hours from 06:00 to 10:00 LT. It peaks at about 07:00 LT and then gradually decreases towards noon (see Fig. 7f), which is in agreement with what is observed in Fig. 7e. In addition to the PBL development, particles are subject to atmospheric aging and likely condensation of semi-volatile and low-volatility compounds resulting in particle growth and a decrease in particle number concentration. It is interesting to note that the morning growth event maximum coincides with a maximum in RH and the occurrence of fog (see Fig. 7c, d). A second local and less pronounced maximum is visible from 13:00 to 15:00 LT. The growth events reported during daytime likely correspond to rainfall events, as reported by Machado et al. (2021) and probably the vertical transport of CN<50 and Aitken size particles due to strong downdrafts in the course of convective rainfall and the injection of these particle populations into the PBL, as reported in Wang et al. (2016) and Andreae et al. (2018).
PATTO shows two maxima: a pronounced and rather defined maximum in the early morning at around 07:00 LT, which follows a gradual increase in precipitation during the second half of the night, and a broader maximum during the afternoon hours between 13:00 and 17:00 LT. Although about 74 % of the particle growth events occur during the day, there are still ∼ 26 % that take place during night conditions, between 19:00–05:00 LT. In particular, the occurrence of growth events from 01:00–05:00, which represents about 16 % of the total observed events, is evidence for complexity in the causes and mechanisms of particle injection and growth.
The evolution of the PBL also has a strong influence on the diurnal pattern. At night, the nocturnal PBL close to the forest canopy is decoupled from the residual layer above (Fisch et al., 2004). In the morning hours – as soon as convection becomes effective – air masses transported into and within the residual layer are mixed into lower levels and measured at the canopy level. Consequently, CN<50 and Aitken-mode particles advected with the residual layer will be mixed downwards and appear at the 60 m inlet in the morning hours, typically around 08:00 LT. This behavior is in agreement with that observed in Fig. 7e, with the increase of CN<50 throughout the night and in the early morning. Machado et al. (2021) discuss this daily mechanism of particle growth in more detail. Section 3.5 further discusses the meteorological conditions regarding convective downdrafts and the atmospheric conditions under which the growth events are observed.
A contrast in GR and CS is observed when day and night events are compared, as shown in Fig. 8. Daytime events, which correspond to ∼ 74 % of the events, have significantly higher GR and CSgrowth, at 5.9 nm h−1 and 1.2 × 10−3 s−1, respectively. The nighttime events, which account for ∼ 26 %, have GR and CSgrowth of 4.0 nm h−1 and 9 × 10−4 s−1, respectively. To verify the statistical significance of the difference between day and nighttime values, the Wilcoxon rank-sum test was applied. The p value obtained for GR is 3.6, while the p value obtained for CSgrowth is 2 × 10−2, indicating that the data groups regarding day and night are statistically different considering a significance level of 5 × 10−2. The observed differences are likely due to the different atmospheric mechanisms during daytime and nighttime. Figure 7a and f clearly indicate an increase of growth events with the increase in the intensity of solar radiation after sunrise. With increasing solar radiation, atmospheric aging and oxidation of possible precursor intensify. Sunlight also drives the dynamics of the PBL. Other phenomena also play an important role in daytime events, such as the peaks of precipitation that coincide with the peaks of growth events, which is discussed in more detail in Sect. 3.5. In contrast, the occurrence of nocturnal events may be related to different mechanisms such as local meteorological phenomena, horizontal advection, entrainment of air and particles from the free atmosphere into the PBL, and perhaps the contribution of biogenic sources from the surface.
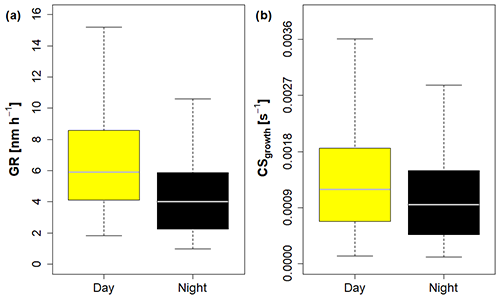
Figure 8Box plot of (a) GR and (b) CSgrowth related to growth events that occurred during the day or night. Nighttime events occurred between 19:00 and 05:00 LT, while daytime events occurred between 06:00 and 18:00 LT. The box represents the quartiles, whiskers represent 90th and 10th percentiles, and the horizontal lines represent the median.
3.5 Meteorological and cloud conditions during growth events
This section explores the weather and convective transport conditions linked to particle growth events. Figure 9a shows a histogram of the growth event frequency as a function of the associated anomaly of the equivalent potential temperature () at the onset of the growth events (see Sect. 2.8). A negative is an indicator for air mass downdrafts from higher altitudes, as has been shown for the events analyzed by Wang et al. (2016). We found that ∼ 63 % of events were likely associated with air mass downdrafts ( K), whereas ∼ 37 % were associated with K. Figure 9b shows a histogram of all growth events as a function of the associated cloud brightness temperature, which is an indicator for deep convective clouds (Tir<245 K) vs. clear-sky/shallow-cloud conditions (Tir>280 K). For all events after 2017 (when Tir data are available), we found that ∼ 36 % were likely associated with deep convective clouds (Tir<245 K, red), ∼ 26 % with clear sky/shallow clouds (Tir>280 K, blue), and ∼ 38 % with mixed-sky conditions (245 K < Tir < 280 K, gray).
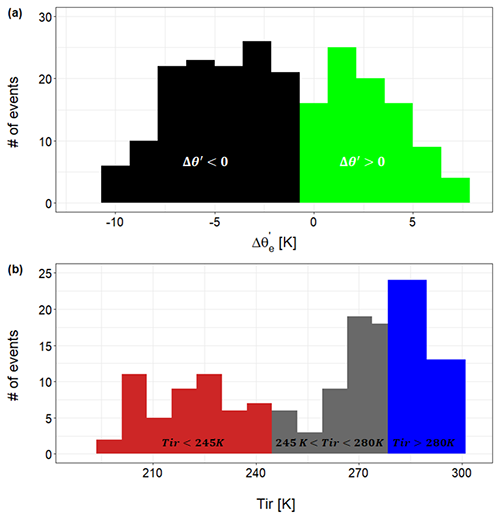
Figure 9Histograms of (a) the values of at the beginning of the particle growth events. Black bars represent events during downdraft conditions and the green bars during undefined conditions. (b) The values of Tir at the beginning of the particle growth events. Red bars represent events during deep-convection conditions, blue during clear-sky/shallow-cloud conditions, and gray during mixed-sky conditions.
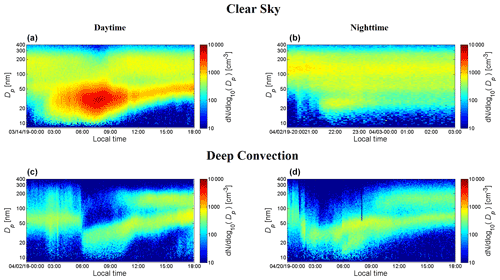
Figure 10Selected particle growth events according to the Tir value at the onset of the event. Events under clear-sky/shallow-cloud conditions during daytime (a, c) and nighttime (b, d) were selected based on Tir >281 K (third quartile), while deep-convection events were chosen based on Tir <228 K (first quartile).
The most contrasting Tir conditions were observed between the lower quartile (Tir<228 K), representing deep convective cloud conditions, and the upper quartile (Tir>281 K), representing clear-sky conditions, with 36 events contained in each group. As an example, four of these “extreme” events were selected (Fig. 10) for daytime and nighttime conditions. The growth events under clear-sky conditions are characterized by a trimodal aerosol population, with accumulation and Aitken modes as well as a third mode below 50 nm. As an example, the event on 14 March 2019 (daytime, clear sky) was characterized by the occurrence of a significant amount of CN<50. The particle concentration for particles smaller than 50 nm increased already during nighttime (starting after 02:00 LT) and resulted in a pronounced peak around 08:00 LT. While during the late night and early morning, the mean modal diameter for these small particles is rather constant, particle growth started around 08:00 LT and lasted for about 10 h. These events could be associated with advection processes, e.g., by a downdraft in the gust front (clear sky nighttime) or by nighttime rainfall. Afterwards, subsequent growth begins in the early morning coinciding with the evolution of the PBL.
The events under deep convective conditions – both during daytime and nighttime – resemble the events reported by Wang et al. (2016). Here, downdrafts transport air masses into the lower atmosphere and, hence, inject CN<50 upper tropospheric particles into the PBL, followed by particle growth into the Aitken mode. In both these cases, the atmosphere is very clean, with low concentrations in the accumulation mode. Before the growth event, most of the particle population is in the Aitken size mode, which is removed by the injection of upper or mid-tropospheric air during the downdraft event, so that only the CN<50 aerosol population remained. Note that about 4 h after the start of the growth event, the accumulation-mode particles (re)appeared, probably by mixing with surrounding air masses.
Figure 11 shows box plots for GR in panel (a) and CSgrowth in panel (b) for clear-sky and deep-convection conditions. The median GR for clear-sky conditions is 7.0 nm h−1, whereas the median GR for deep-convection conditions is 3.8 nm h−1. Regarding CS, under clear-sky conditions the median is 1.6 × 10−3 s−1, while under deep convection, the median CS is 5 × 10−4 s−1. The results show that different meteorological processes play an important role for the different particle growth events observed. The events that occurred under deep-convection conditions present much lower CSgrowth and considerable lower GR. Two main factors may influence this result: the precipitation during deep-convection conditions cleans the atmosphere by wet scavenging, resulting in lower CSgrowth values, and the presence of clouds reduces the availability of sunlight and thereby suppresses photochemical production of condensable species.
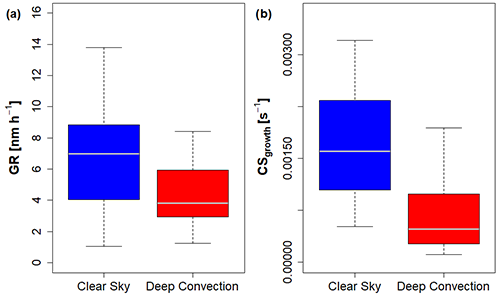
Figure 11Box plot of (a) GR and (b) CSgrowth for clear-sky conditions (Tir>281 K) vs. deep-convection conditions (Tir<228 K). Boxes represent the quartiles, whiskers represent 90th and 10th percentiles, and the horizontal line represents the median.
To further investigate typical conditions or processes related to the observed growth events, here we discriminate between different groups based on their daily frequency distribution. The growth events (see Fig. 7f) were divided into four groups (G1 to G4), where G1 and G4 represent nighttime, and G2 and G3 represent daytime events. The daytime events were divided considering the occurrence of two frequency peaks: the first peak, representing 53 % of the growth events, is included in G2, covering the time from 06:00 to 11:59 LT. The afternoon increase, representing 21 % of the growth events, is included in G3, covering the time from 12:00 to 17:59 LT. The nocturnal events were divided according to the evolution stage of the PBL. Events between 18:00 and 00:59 (G4), with 10 % of the events, are still influenced by some PBL turbulence but may also have had some influence from convective events in the late afternoon.
The growth events between 01:00 and 05:59 (G1), with 16 % of the total growth events, are the most enigmatic ones. They are not directly driven by photochemistry or variations in the PBL, since at this time the nocturnal PBL is already well established. Different mechanisms such as air mass entrainment into the PBL by, e.g., intermittent turbulence (Dias-Júnior et al., 2017), nighttime rainfall events, or even an unknown biogenic source could play a role in the aerosol particle dynamics of in this time period. Table 2 shows the median Tir, , the initial diameter at the onset of the growth event, Dp,i, and the GR and CSgrowth for the four hourly groups.
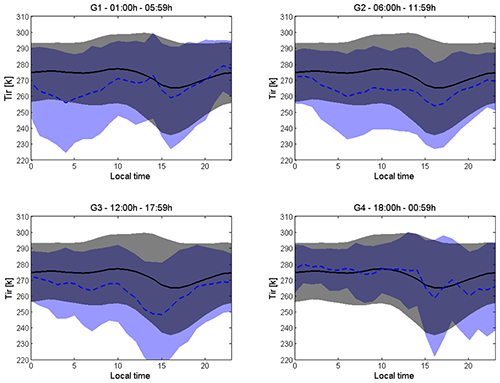
Figure 12Average diurnal cycle of Tir for the event days in the groups G1 to G4. The dashed blue line represents the average diurnal cycle of Tir relating to the days when the particle growth events for a given group were observed. The black line represents the average diurnal cycle for all days on which PNSD measurements were made. The colored shading represents the standard deviations.
The results indicate differences between the four groups (G1 to G4) regarding the Tir and conditions during the event onset. The nocturnal groups G1 and G4 have median of −0.8 and 0 K, respectively. Their median Tir indicates conditions closer to low clouds and clear skies, with median values equal to 269 and 274 K for G1 and G4, respectively. Figure 12 shows the average (mean and standard deviation) Tir for the entire event days separated for the different groups of events (blue). The Tir diurnal cycle shows clear differences between the groups. Within the G1 period, Tir shows a minimum at 04:00 LT, when the average brightness temperature reaches 256 K, indicating convective activity and early precipitation compared to all days.
The G4 group, with the smallest number of growth events, is not significantly different from the median diurnal cycle for all days, suggesting that this 10 % of growth cases appears not be related to specific meteorological events. There are signs of convection at 16:00 LT, which coincides with the precipitation peak in the afternoon. Afterward, Tir increases, going to clear-sky conditions during the night. The median GR for G1 and G4 varies from 3.8 to 4.2 nm h−1, respectively, and CSgrowth is approximately constant around 1.0 × 10−3 s−1, while the median Dp,i is similar for both nocturnal groups. The daytime groups G2 and G3 have the lowest median at the onset of the events, with −1.9 and −3.6 K, respectively, indicating that convective downdraft activity plays an essential role during these growth events. For these two groups, the diurnal cycle of Tir presents lower values during the whole day compared to the overall average, and the G2 group also shows minima in the early morning coinciding with the precipitation peaks and also in the afternoon (Fig. 7b).
The G3 group is the one with the most convective characteristics. The Tir values indicate an occurrence of strong convective systems throughout the day, mainly in the afternoon. In particular, the pronounced decrease at 15:00 LT reaches deep-convection conditions, with Tir = 249 K, approximately 1 h earlier than expected considering the entire observation days. The median GR ranges from 6.3 to 5.6 nm h−1 in G2 and G3, respectively, and the median CSgrowth remains similar for both groups, at 1.2 × 10−3 s−1. The smaller value of GR in G3 compared to G2 (diurnal events) could be associated with deep-convection systems, which reduce the solar irradiance and thereby influence the photochemical processes. The presence of clouds has been associated with lowering GR and even lower occurrences of NPF and particle growth events (Dada et al., 2017; Kerminen et al., 2018). The median Dp,i for G3 is the lowest of the four groups, at 24.6 nm, indicating that the strong convective downdrafts are more effective in transporting smaller particles from the free troposphere into the PBL, which agrees with what has been observed previously (Wang et al., 2016). Therefore, the growth events of groups G2 and G3 are probably influenced by the strong convective systems during daytime. In particular, the G3 group has the most significant characteristics of deep convection and intense occurrences of downdraft throughout the day.
When discriminating the growth events by positive or negative at the event's onset (here defined as Δt=0) and looking 10 h before and after this time, the different behavior of and Tir near the growth event is evident. Figure 13 shows the behavior of the mean ensembles of and Tir around Δt=0 for less than the 25th percentile (−5.3 K) and higher than the 75th percentile (+1.5 K) at the event's onset. Considering the case where K at the event's onset, both and Tir strongly decrease from 10 h before the event and reach a minimum at Δt=0, where K and Tir = 238 K, which represents deep-convection conditions with strong downdraft occurrence. Both parameters increase afterwards to cleaner sky conditions and out-of-downdraft conditions.
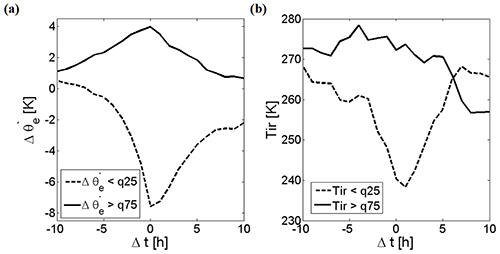
Figure 13Ensemble analysis for (a) and (b) Tir considering 10 h before and 10 h after the event's onset. The cases where at the event's onset is less than the 25th percentile (dashed lines) and more than the 75th percentile (full lines) are shown. In total, 72 cases were considered in the analysis (36 cases for each condition).
In contrast, for K at the event's onset, the ensembles show an opposite behavior of the parameters. The presents an increasing tendency from 10 h before the event until Δt=0, reaching a value of +4 K. Afterward, decreases but always retains positive values, indicating that there is a class of growth events that may not be driven by convective downdrafts. The Tir values from 10 h before the events up to Δt=0 are equivalent to conditions close to clear skies (Tir ∼ 280 K), which agrees with the results obtained for , as an indication of sky conditions not dominated by convection systems around the event's onset. After Δt=0, Tir values decrease but without presenting deep-convection conditions. This shows that clear-sky events can be associated with either advection or subsidence bringing particulates from another area (e.g., a nearby rain event) or from the upper troposphere.
This study reports the statistical characterization of aerosol particle growth events in the sub-50 nm size range (10–50 nm), based on continuous measurements (February 2014 to September 2020) of PNSDs at a remote site in central Amazonia. In total, 254 particle growth events were detected, comprising about 14 % of the analyzed days. Of all events, 88 % were found between January and June and 12 % between July and December. The diurnal cycle of the growth events shows that most of them occur during the daytime, accounting for 74 %, which still leaves a significant fraction of 26 % occurring during the night. During daytime, 53 % of the growth events start in the morning between 06:00 and 11:00 LT, with a pronounced peak at 07:00 LT, showing a relation to the photochemistry and links with the evolution of the PBL. These events also coincide with a precipitation peak in the morning. The nocturnal increase of N<50 is likely related to convective systems that result in the precipitation peak in the morning. The subsequent decrease of N<50 is likely due to condensation of semi- and less-volatile organic species on the sub-50 nm particles, resulting in growth. A second, less pronounced but significant peak occurs around 15:00 LT, coinciding with the strongest precipitation peak, also suggesting a relation to atmospheric convective systems.
The median GR, considering all the growth events, is 5.2 nm h−1, which agrees with what was reported by Varanda Rizzo et al. (2018). The median CSgrowth is 1.1 × 10−3 s−1. Monthly variations in GR and CS show that during the wet season the growth events occur under low CS values, although the average CSgrowth does not change much from month to month, oscillating around 1.1 × 10−3 s−1. A remarkable contrast is observed when comparing daytime (median GR of 5.9 nm h−1 and median CSgrowth of 1.2 × 10−3 s−1) and nighttime growth events (median GR of 4.0 nm h−1 and median CSgrowth of 9 × 10−4 s−1).
Daytime events are directly influenced by sunlight, which controls photochemistry and hence the oxidation of SOA precursors. In contrast, nocturnal events may have different causes and mechanisms. One particular mechanism for nighttime growth events could be supported both by the continued deposition of condensables formed during the day and by the production of condensables at night by ozonolysis reactions. However, the direct influence of meteorology, entrainment of air masses, and perhaps the contribution of biogenic sources can not be ruled out. Upcoming flight and in situ campaigns are expected to provide new important insights into the main drivers of the sources for the different particle growth events. It is worth noting that, for primary biogenic emissions, there is still the need to identify the particle sources, although earlier studies have shown that the growth of secondary aerosol particles can be initiated by biogenically emitted potassium-salt-rich particles (Pöhlker et al., 2012). Also, a primary source close in the canopy would be fundamental for the aerosol particle maintenance in the PBL (Varanda Rizzo et al., 2018).
An analysis performed using and Tir revealed that diverse atmospheric dynamics play different roles during particle growth event days. Many event onsets coincide with downdraft occurrences, when N<50 appear and grow afterward. We also observed that accumulation-mode particles processed in clouds appear sporadically, causing a prominent Hoppel minimum. The growth events occurring under clear skies present GR and CS higher than those related to deep convection: the median GR and CS for clear-sky conditions are 7.0 nm h−1 and 1.6 × 10−3 s−1, whereas under deep-convection conditions, the median GR and CS are 3.8 nm h−1 and 5 × 10−4 s−1.
The events were further classified according to their frequency of occurrence throughout the day, showing that they are mostly driven by local convective activities (73 %). However, when analyzing the growth events by at the event onset, the occurrence of downdrafts does not explain all the cases. The contrast is more evident in the ensembles of and Tir when growth events and their respective occurrence days are selected by th percentile ( K) at the event onset. For these events, representing about 27 % of the growth events, is maximum and positive at time Δt=0, and even 10 h before or after the growth event, it did not present negative values that could indicate the occurrence of convective downdrafts. Also, Tir fluctuates at around 270 K over the observed period, which represents shallow clouds, instead of convective systems conditions. In contrast, events with th percentile ( K) at the event onset are associated with downdraft occurrences: strongly decreases 10 h after the growth event up to Δt=0, which is also followed by a strong decrease in Tir.
Sources that could explain growth events in the absence of deep convection are perhaps related to primary biogenic aerosols emitted by the forest, smooth entrainment of air masses from the free troposphere into the PBL in the early morning or even different meteorological mechanisms such as gravity waves and particle production by lightning in the free troposphere, as reported by Machado et al. (2021). Another possible explanation is related to nighttime downdrafts far upwind, which get trapped above the nocturnal boundary layer and travel in the jet above the nocturnal inversion for potentially quite a large distance, being mixed down into the PBL after sunrise, as suggested by Krejci et al. (2005). Therefore future studies are required to unveil the aerosol sources that could explain the diversity of particle growth events observed in the lower troposphere over central Amazônia.
The data of the key results presented here have been deposited in associated data files for use in follow-up studies. The SMPS data used in this study are available under https://doi.org/10.17617/3.90 (Franco et al., 2022a). Additional fog data used in this study are available under https://doi.org/10.17617/3.8z (Franco et al., 2022b). Additional equivalent potential temperature data, the anomaly of the potential temperature, and the characteristics of the 254 particle growth events analyzed in this study are available under https://doi.org/10.17617/3.8y (Franco et al., 2022c). Additional Tir data can be found on the portal https://edmond.mpdl.mpg.de/imeji/ (last access: 16 February 2022) collection /Q9NOaAlgWZTq7f9s (Machado et al., 2021). Additional ATTO data can be found in the ATTO data portal under https://www.attodata.org/ (last access: 16 February 2022, ATTO, 2022). For data requests beyond the available data, please refer to the corresponding authors.
The supplement related to this article is available online at: https://doi.org/10.5194/acp-22-3469-2022-supplement.
MAF and FD contributed equally to this work. MAF, FD, and CP designed the study. MAF and FD analyzed the data. MAF, FD, LAK, BAH, FGM, JS, SC, JFdB, and SW collected and processed the ATTO aerosol data. LATM processed the satellite data. AA and MS collected the micrometeorological data at the INSTANT tower at ATTO. SW, FGM, PA, MOA, and UP provided essential scientific support for the ATTO measurements. DW supported the data management. MAF, CP, and FD wrote the paper. LATM, MOA, LVR, HMJB, JPN, FGM, MLP, SC, JFdB, and SC contributed with valuable ideas and comments to the analysis and the manuscript. All authors contributed to the discussion of the results as well as the finalization of the paper. PA and CP supervised the study.
At least one of the (co-)authors is a member of the editorial board of Atmospheric Chemistry and Physics. The peer-review process was guided by an independent editor, and the authors also have no other competing interests to declare.
This paper contains results of research conducted under the Technical/Scientific Cooperation Agreement between the National Institute for Amazonian Research, the State University of Amazonas, and the Max-Planck-Gesellschaft e.V.; the opinions expressed are the entire responsibility of the authors and not of the participating institutions.
Publisher's note: Copernicus Publications remains neutral with regard to jurisdictional claims in published maps and institutional affiliations.
This work has been funded by the Max Planck Society (MPG) and FAPESP – Fundação de Amparo à Pesquisa do Estado de São Paulo. Marco A. Franco acknowledges the financial support of CNPq for the PhD scholarship, and CAPES, for a sandwich doctorate at the Max Planck Institute for Chemistry. For the operation of the ATTO site, we acknowledge the support by the Max Planck Society (MPG), the German Federal Ministry of Education and Research, and the Brazilian Ministério da Ciência, Tecnologia e Inovação (MCTI/FINEP) as well as the Amazon State University (UEA), FAPEAM, LBA/INPA, and SDS/CEUC/RDS-Uatumã. We acknowledge the support by the Instituto Nacional de Pesquisas da Amazônia (INPA). We would like to thank Reiner Ditz, Jürgen Kesselmeier, Susan Trumbore, Alberto Quesada, Thomas Disper, Thomas Klimach, Andrew Crozier, Björn Nillius, Uwe Schulz, Steffen Schmidt, Delano Campos, Sam Jones, Fábio Jorge, Hermes Braga Xavier, Nagib Alberto de Castro Souza, Adir Vasconcelos Brandão, Amauri Rodriguês Perreira, Antonio Huxley Melo Nascimento, Roberta Pereira de Souza, Bruno Takeshi, and Wallace Rabelo Costa for technical, logistical, and scientific support within the ATTO project.
This research has been supported by the Fundação de Amparo à Pesquisa do Estado de São Paulo (FAPESP, grant no. 2017/17047-0), CNPq (grant no. 169842/2017-7), CAPES (grant no. 88887.368025/2019-00), the Max Planck Society (MPG), the German Federal Ministry of Education and Research (BMBF; contract nos. 01LB1001A, 01LK1602B, and 01LK2101B), and the Brazilian Ministério da Ciência, Tecnologia e Inovação (MCTI/FINEP; contract no. 01.11.01248.00).
The article processing charges for this open-access publication were covered by the Max Planck Society.
This paper was edited by Veli-Matti Kerminen and reviewed by two anonymous referees.
Andreae, M., Berresheim, H., Bingemer, H., Jacob, D. J., Lewis, B., Li, S.-M., and Talbot, R. W.: The atmospheric sulfur cycle over the Amazon Basin: 2. Wet season, J. Geophys. Res.-Atmos., 95, 16813–16824, 1990. a
Andreae, M. O.: The aerosol nucleation puzzle, Science, 339, 911–912, 2013. a
Andreae, M. O., Acevedo, O. C., Araùjo, A., Artaxo, P., Barbosa, C. G. G., Barbosa, H. M. J., Brito, J., Carbone, S., Chi, X., Cintra, B. B. L., da Silva, N. F., Dias, N. L., Dias-Júnior, C. Q., Ditas, F., Ditz, R., Godoi, A. F. L., Godoi, R. H. M., Heimann, M., Hoffmann, T., Kesselmeier, J., Könemann, T., Krüger, M. L., Lavric, J. V., Manzi, A. O., Lopes, A. P., Martins, D. L., Mikhailov, E. F., Moran-Zuloaga, D., Nelson, B. W., Nölscher, A. C., Santos Nogueira, D., Piedade, M. T. F., Pöhlker, C., Pöschl, U., Quesada, C. A., Rizzo, L. V., Ro, C.-U., Ruckteschler, N., Sá, L. D. A., de Oliveira Sá, M., Sales, C. B., dos Santos, R. M. N., Saturno, J., Schöngart, J., Sörgel, M., de Souza, C. M., de Souza, R. A. F., Su, H., Targhetta, N., Tóta, J., Trebs, I., Trumbore, S., van Eijck, A., Walter, D., Wang, Z., Weber, B., Williams, J., Winderlich, J., Wittmann, F., Wolff, S., and Yáñez-Serrano, A. M.: The Amazon Tall Tower Observatory (ATTO): overview of pilot measurements on ecosystem ecology, meteorology, trace gases, and aerosols, Atmos. Chem. Phys., 15, 10723–10776, https://doi.org/10.5194/acp-15-10723-2015, 2015. a, b, c, d, e
Andreae, M. O., Afchine, A., Albrecht, R., Holanda, B. A., Artaxo, P., Barbosa, H. M. J., Borrmann, S., Cecchini, M. A., Costa, A., Dollner, M., Fütterer, D., Järvinen, E., Jurkat, T., Klimach, T., Konemann, T., Knote, C., Krämer, M., Krisna, T., Machado, L. A. T., Mertes, S., Minikin, A., Pöhlker, C., Pöhlker, M. L., Pöschl, U., Rosenfeld, D., Sauer, D., Schlager, H., Schnaiter, M., Schneider, J., Schulz, C., Spanu, A., Sperling, V. B., Voigt, C., Walser, A., Wang, J., Weinzierl, B., Wendisch, M., and Ziereis, H.: Aerosol characteristics and particle production in the upper troposphere over the Amazon Basin, Atmos. Chem. Phys., 18, 921–961, https://doi.org/10.5194/acp-18-921-2018, 2018. a, b, c, d, e, f, g, h, i
Andreae, M. O., Andreae, T. W., Ditas, F., and Pöhlker, C.: Frequent new particle formation at remote sites in the subboreal forest of North America, Atmos. Chem. Phys., 22, 2487–2505, https://doi.org/10.5194/acp-22-2487-2022, 2022. a, b, c, d
Artaxo, P., Rizzo, L. V., Brito, J. F., Barbosa, H. M. J., Arana, A., Sena, E. T., Cirino, G. G., Bastos, W., Martin, S. T., and Andreae, M. O.: Atmospheric aerosols in Amazonia and land use change: from natural biogenic to biomass burning conditions, Faraday Discuss., 165, 203–235, https://doi.org/10.1039/c3fd00052d, 2013. a, b
ATTO: ATTO data portal, https://www.attodata.org/, last access: 16 February 2022. a
Baars, H., Ansmann, A., Althausen, D., Engelmann, R., Heese, B., Müller, D., Artaxo, P., Paixao, M., Pauliquevis, T., and Souza, R.: Aerosol profiling with lidar in the Amazon Basin during the wet and dry season, J. Geophys. Res.-Atmos., 117, D21, https://doi.org/10.1029/2012JD018338, 2012. a
Betts, A. K., Gatti, L. V., Cordova, A. M., Dias, M. A. S., and Fuentes, J. D.: Transport of ozone to the surface by convective downdrafts at night, J. Geophys. Res.-Atmos., 107, LBA 13-1–LBA 13-6, https://doi.org/10.1029/2000JD000158, 2002. a
Bolton, D.: The computation of equivalent potential temperature, Mon. Weather Rev., 108, 1046–1053, 1980. a
Bonn, B. and Moortgat, G. K.: Sesquiterpene ozonolysis: Origin of atmospheric new particle formation from biogenic hydrocarbons, Geophys. Res. Lett., 30, 11, https://doi.org/10.1029/2003GL017000, 2003. a
Brito, J., Rizzo, L. V., Morgan, W. T., Coe, H., Johnson, B., Haywood, J., Longo, K., Freitas, S., Andreae, M. O., and Artaxo, P.: Ground-based aerosol characterization during the South American Biomass Burning Analysis (SAMBBA) field experiment, Atmos. Chem. Phys., 14, 12069–12083, https://doi.org/10.5194/acp-14-12069-2014, 2014. a
Chen, Q., Farmer, D. K., Rizzo, L. V., Pauliquevis, T., Kuwata, M., Karl, T. G., Guenther, A., Allan, J. D., Coe, H., Andreae, M. O., Pöschl, U., Jimenez, J. L., Artaxo, P., and Martin, S. T.: Submicron particle mass concentrations and sources in the Amazonian wet season (AMAZE-08), Atmos. Chem. Phys., 15, 3687–3701, https://doi.org/10.5194/acp-15-3687-2015, 2015. a
Cirino, G., Brito, J., Barbosa, H. M., Rizzo, L. V., Tunved, P., de Sá, S. S., Jimenez, J. L., Palm, B. B., Carbone, S., Lavric, J. V., Souza, R. A., Wolff, S., Walter, D., Tota, J., Oliveira, M. B., Martin, S. T., and Artaxo, P.: Observations of Manaus urban plume evolution and interaction with biogenic emissions in GoAmazon 2014/5, Atmos. Environ., 191, 513–524, https://doi.org/10.1016/j.atmosenv.2018.08.031, 2018. a
Clarke, A. D.: Atmospheric nuclei in the remote free-troposphere, J. Atmos. Chem., 14, 479–488, 1992. a
Dada, L., Paasonen, P., Nieminen, T., Buenrostro Mazon, S., Kontkanen, J., Peräkylä, O., Lehtipalo, K., Hussein, T., Petäjä, T., Kerminen, V.-M., Bäck, J., and Kulmala, M.: Long-term analysis of clear-sky new particle formation events and nonevents in Hyytiälä, Atmos. Chem. Phys., 17, 6227–6241, https://doi.org/10.5194/acp-17-6227-2017, 2017. a, b, c
Dada, L., Chellapermal, R., Buenrostro Mazon, S., Paasonen, P., Lampilahti, J., Manninen, H. E., Junninen, H., Petäjä, T., Kerminen, V.-M., and Kulmala, M.: Refined classification and characterization of atmospheric new-particle formation events using air ions, Atmos. Chem. Phys., 18, 17883–17893, https://doi.org/10.5194/acp-18-17883-2018, 2018. a
Dall'Osto, M., Beddows, D. C. S., Asmi, A., Poulain, L., Hao, L., Freney, E., Allan, J. D., Canagaratna, M., Crippa, M., Bianchi, F., de Leeuw, G., Eriksson, A., Swietlicki, E., Hansson, H. C., Henzing, J. S., Granier, C., Zemankova, K., Laj, P., Onasch, T., Prevot, A., Putaud, J. P., Sellegri, K., Vidal, M., Virtanen, A., Simo, R., Worsnop, D., O'Dowd, C., Kulmala, M., and Harrison, R. M.: Novel insights on new particle formation derived from a pan-european observing system, Sci. Rep., 8, 1482, https://doi.org/10.1038/s41598-017-17343-9, 2018. a
Dal Maso, M.: Formation and Growth of Fresh Atmospheric Aerosols Eight Years of Aerosol Size Distribution Data From SMEAR, Boreal Environ. Res., 10, 323–336, 2005. a, b, c, d
Dal Maso, M., Kulmala, M., Lehtinen, K. E., Mäkelä, J., Aalto, P., and O'Dowd, C.: Condensation and coagulation sinks and formation of nucleation mode particles in coastal and boreal forest boundary layers, J. Geophys. Res.-Atmos., 107, PAR 2-1–PAR 2-10, https://doi.org/10.1029/2001JD001053, 2002. a, b
Dal Maso, M., Sogacheva, L., Aalto, P. P., Riipinen, I., Komppula, M., Tunved, P., Korhonen, L., Suur-Uski, V., Hirsikko, A., KurtéN, T., Kerminen, V.-M., Lihavainen, H., Viisanen, Y., Hansson, H.-C., and Kulmala, M.: Aerosol size distribution measurements at four Nordic field stations: identification, analysis and trajectory analysis of new particle formation bursts, Tellus B, 59, 350–361, https://doi.org/10.1111/j.1600-0889.2007.00267.x, 2007. a
De Reus, M., Krejci, R., Williams, J., Fischer, H., Scheele, R., and Ström, J.: Vertical and horizontal distributions of the aerosol number concentration and size distribution over the northern Indian Ocean, J. Geophys. Res.-Atmos., 106, 28629–28641, 2001. a
Dias-Júnior, C. Q., Sá, L. D., Marques Filho, E. P., Santana, R. A., Mauder, M., and Manzi, A. O.: Turbulence regimes in the stable boundary layer above and within the Amazon forest, Agr. Forest Meteorol., 233, 122–132, https://doi.org/10.1016/j.agrformet.2016.11.001, 2017. a
Fan, J., Rosenfeld, D., Zhang, Y., Giangrande, S. E., Li, Z., Machado, L. A. T., Martin, S. T., Yang, Y., Wang, J., Artaxo, P., Barbosa, H. M. J., Braga, R. C., Comstock, J. M., Feng, Z., Gao, W., Gomes, H. B., Mei, F., Pöhlker, C., Pöhlker, M. L., Pöschl, U., and de Souza, R. A. F.: Substantial convection and precipitation enhancements by ultrafine aerosol particles, Science, 359, 411–418, https://doi.org/10.1126/science.aan8461, 2018. a
Fisch, G., Tota, J., Machado, L. A. T., Silva Dias, M. A. F., da F. Lyra, R. F., Nobre, C. A., Dolman, A. J., and Gash, J. H. C.: The convective boundary layer over pasture and forest in Amazonia, Theor. Appl. Climatol., 78, 47–59, https://doi.org/10.1007/s00704-004-0043-x, 2004. a
Franco, M. A., Kremper, L. A., Ditas, F., Pöhlker, C., Artaxo, P., and Walter, D.: SMPS dataset at ATTO, Max Planck Society [data set], https://doi.org/10.17617/3.90, 2022a. a
Franco, M. A., Ditas, F., Pöhlker, C., Artaxo, P., Walter, D., and Kremper, L. A.: Fog dataset at ATTO, Max Planck Society [data set], https://doi.org/10.17617/3.8z, 2022b. a
Franco, M. A., Ditas, F., Pöhlker, C., Artaxo, P., and Walter, D.: Particle growth events and equivalent potential temperature at ATTO, Max Planck Society [data set], https://doi.org/10.17617/3.8y, 2022c. a
Fuchs, N. and Sutugin, A.: High-dispersed aerosols, in: Topics in current aerosol research, Elsevier, p. 1, https://doi.org/10.1016/B978-0-08-016674-2.50006-6, 1971. a
Gerken, T., Wei, D., Chase, R., Fuentes, J., Schumacher, C., Machado, L., Andreoli, R., Chamecki, M., Ferreira de Souza, R., Freire, L., Jardine, A., Manzi, A., Nascimento dos Santos, R., von Randow, C., dos Santos Costa, P., Stoy, P., Tóta, J., and Trowbridge, A.: Downward transport of ozone rich air and implications for atmospheric chemistry in the Amazon rainforest, Atmos. Environ., 124, 64–76, 2016. a
Glicker, H. S., Lawler, M. J., Ortega, J., de Sá, S. S., Martin, S. T., Artaxo, P., Vega Bustillos, O., de Souza, R., Tota, J., Carlton, A., and Smith, J. N.: Chemical composition of ultrafine aerosol particles in central Amazonia during the wet season, Atmos. Chem. Phys., 19, 13053–13066, https://doi.org/10.5194/acp-19-13053-2019, 2019. a, b
Gong, Y., Su, H., Cheng, Y., Liu, F., Wu, Z., Hu, M., Zeng, L., and Zhang, Y.: Analysis on concentration and source rate of precursor vapors participating in particle formation and growth at Xinken in the Pearl River Delta of China, Adv. Atmos. Sci., 25, 427–436, 2008. a
Gunthe, S. S., King, S. M., Rose, D., Chen, Q., Roldin, P., Farmer, D. K., Jimenez, J. L., Artaxo, P., Andreae, M. O., Martin, S. T., and Pöschl, U.: Cloud condensation nuclei in pristine tropical rainforest air of Amazonia: size-resolved measurements and modeling of atmospheric aerosol composition and CCN activity, Atmos. Chem. Phys., 9, 7551–7575, https://doi.org/10.5194/acp-9-7551-2009, 2009. a, b
Hamed, A., Korhonen, H., Sihto, S.-L., Joutsensaari, J., Järvinen, H., Petäjä, T., Arnold, F., Nieminen, T., Kulmala, M., Smith, J. N., Lehtinen, K. E. J., and Laaksonen, A.: The role of relative humidity in continental new particle formation, J. Geophys. Res.-Atmos., 116, D3, https://doi.org/10.1029/2010JD014186, 2011. a, b
Hamilton, D. S., Lee, L. A., Pringle, K. J., Reddington, C. L., Spracklen, D. V., and Carslaw, K. S.: Occurrence of pristine aerosol environments on a polluted planet, P. Natl. Acad. Sci. USA, 111, 18466–18471, https://doi.org/10.1073/pnas.1415440111, 2014. a
Heintzenberg, J.: Properties of the log-normal particle size distribution, Aerosol Sci. Technol., 21, 46–48, 1994. a
Heintzenberg, J., Tunved, P., Galí, M., and Leck, C.: New particle formation in the Svalbard region 2006–2015, Atmos. Chem. Phys., 17, 6153–6175, https://doi.org/10.5194/acp-17-6153-2017, 2017. a
Holanda, B. A., Pöhlker, M. L., Walter, D., Saturno, J., Sörgel, M., Ditas, J., Ditas, F., Schulz, C., Franco, M. A., Wang, Q., Donth, T., Artaxo, P., Barbosa, H. M. J., Borrmann, S., Braga, R., Brito, J., Cheng, Y., Dollner, M., Kaiser, J. W., Klimach, T., Knote, C., Krüger, O. O., Fütterer, D., Lavrič, J. V., Ma, N., Machado, L. A. T., Ming, J., Morais, F. G., Paulsen, H., Sauer, D., Schlager, H., Schneider, J., Su, H., Weinzierl, B., Walser, A., Wendisch, M., Ziereis, H., Zöger, M., Pöschl, U., Andreae, M. O., and Pöhlker, C.: Influx of African biomass burning aerosol during the Amazonian dry season through layered transatlantic transport of black carbon-rich smoke, Atmos. Chem. Phys., 20, 4757–4785, https://doi.org/10.5194/acp-20-4757-2020, 2020. a, b, c
Hoppel, W., Frick, G., and Larson, R.: Effect of nonprecipitating clouds on the aerosol size distribution in the marine boundary layer, Geophys. Res. Lett., 13, 125–128, 1986. a
Huffman, J. A., Sinha, B., Garland, R. M., Snee-Pollmann, A., Gunthe, S. S., Artaxo, P., Martin, S. T., Andreae, M. O., and Pöschl, U.: Size distributions and temporal variations of biological aerosol particles in the Amazon rainforest characterized by microscopy and real-time UV-APS fluorescence techniques during AMAZE-08, Atmos. Chem. Phys., 12, 11997–12019, https://doi.org/10.5194/acp-12-11997-2012, 2012. a
Hussein, T., Dal Maso, M., Petaja, T., Koponen, I. K., Paatero, P., Aalto, P. P., Hameri, K., and Kulmala, M.: Evaluation of an automatic algorithm for fitting the particle number size distributions, Boreal Environ. Res., 10, 337–355, 2005. a
Hyvönen, S., Junninen, H., Laakso, L., Dal Maso, M., Grönholm, T., Bonn, B., Keronen, P., Aalto, P., Hiltunen, V., Pohja, T., Launiainen, S., Hari, P., Mannila, H., and Kulmala, M.: A look at aerosol formation using data mining techniques, Atmos. Chem. Phys., 5, 3345–3356, https://doi.org/10.5194/acp-5-3345-2005, 2005. a
Kanawade, V. P., Jobson, B. T., Guenther, A. B., Erupe, M. E., Pressley, S. N., Tripathi, S. N., and Lee, S.-H.: Isoprene suppression of new particle formation in a mixed deciduous forest, Atmos. Chem. Phys., 11, 6013–6027, https://doi.org/10.5194/acp-11-6013-2011, 2011. a
Kerminen, V.-M., Chen, X., Vakkari, V., Petäjä, T., Kulmala, M., and Bianchi, F.: Atmospheric new particle formation and growth: review of field observations, Environ. Res. Lett., 13, 103003, https://doi.org/10.1088/1748-9326/aadf3c, 2018. a, b, c, d, e, f, g, h, i, j, k, l
Kiendler-Scharr, A., Wildt, J., Dal Maso, M., Hohaus, T., Kleist, E., Mentel, T. F., Tillmann, R., Uerlings, R., Schurr, U., and Wahner, A.: New particle formation in forests inhibited by isoprene emissions, Nature, 461, 381–384, 2009. a
Kirkby, J., Curtius, J., Almeida, J., Dunne, E., Duplissy, J., Ehrhart, S., Franchin, A., Gagne, S., Ickes, L., Kurten, A., Kupc, A., Metzger, A., Riccobono, F., Rondo, L., Schobesberger, S., Tsagkogeorgas, G., Wimmer, D., Amorim, A., Bianchi, F., Breitenlechner, M., David, A., Dommen, J., Downard, A., Ehn, M., Flagan, R., Haider, S., Hansel, A., Hauser, D., Jud,W., Junninen, H., Kreissl, F., Kvashin, A., Laaksonen, A., Lehtipalo, K., Lima, J., Lovejoy, E., Makhmutov, V., Mathot, S., Mikkila, J., Minginette, P., Mogo, S., Nieminen, T., Onnela, A., Pereira, P., Petaja, T., Schnitzhofer, R., Seinfeld, J., Sipila, M., Stozhkov, Y., Stratmann, F., Tome, A., Vanhanen, J., Viisanen, Y., Vrtala, A., Wagner, P., Walther, H., Weingartner, E., Wex, H., Winkler, P., Carslaw, K., Worsnop, D., Baltensperger, U., and Kulmala, M.: Role of sulphuric acid, ammonia and galactic cosmic rays in atmospheric aerosol nucleation, Nature, 476, 429–433, 2011. a, b
Kirkby, J., Duplissy, J., Sengupta, K., Frege, C., Gordon, H., Williamson, C., Heinritzi, M., Simon, M., Yan, C., Almeida, J., Trostl, J., Nieminen, T., Ortega, I. K., Wagner, R., Adamov, A., Amorim, A., Bernhammer, A. K., Bianchi, F., Breitenlechner, M., Brilke, S., Chen, X. M., Craven, J., Dias, A., Ehrhart, S., Flagan, R. C., Franchin, A., Fuchs, C., Guida, R., Hakala, J., Hoyle, C. R., Jokinen, T., Junninen, H., Kangasluoma, J., Kim, J., Krapf, M., Kurten, A., Laaksonen, A., Lehtipalo, K., Makhmutov, V., Mathot, S., Molteni, U., Onnela, A., Perakyla, O., Piel, F., Petaja, T., Praplan, A. P., Pringle, K., Rap, A., Richards, N. A. D., Riipinen, I., Rissanen, M. P., Rondo, L., Sarnela, N., Schobesberger, S., Scott, C. E., Seinfeld, J. H., Sipila, M., Steiner, G., Stozhkov, Y., Stratmann, F., Tome, A., Virtanen, A., Vogel, A. L., Wagner, A. C., Wagner, P. E., Weingartner, E., Wimmer, D., Winkler, P. M., Ye, P. L., Zhang, X., Hansel, A., Dommen, J., Donahue, N. M., Worsnop, D. R., Baltensperger, U., Kulmala, M., Carslaw, K. S., and Curtius, J.: Ion-induced nucleation of pure biogenic particles, Nature, 533, 521–526, https://doi.org/10.1038/nature17953, 2016. a
Krejci, R.: Evolution of aerosol properties over the rain forest in Surinam, South America, observed from aircraft during the LBA-CLAIRE 98 experiment, J. Geophys. Res., 108, 4561, https://doi.org/10.1029/2001JD001375, 2003. a, b
Krejci, R., Strom, J., de Reus, M., Hoor, P., Williams, J., Fischer, H., and Hansson, H. C.: Evolution of aerosol properties over the rain forest in Surinam, South America, observed from aircraft during the LBA-CLAIRE 98 experiment, J. Geophys. Res.-Atmos., 108, https://doi.org/10.1029/2001jd001375, 2003. a, b, c, d, e
Krejci, R., Ström, J., de Reus, M., Williams, J., Fischer, H., Andreae, M. O., and Hansson, H.-C.: Spatial and temporal distribution of atmospheric aerosols in the lowermost troposphere over the Amazonian tropical rainforest, Atmos. Chem. Phys., 5, 1527–1543, https://doi.org/10.5194/acp-5-1527-2005, 2005. a, b
Kulmala, M., Vehkamäki, H., Petäjä, T., Dal Maso, M., Lauri, A., Kerminen, V.-M., Birmili, W., and McMurry, P.: Formation and growth rates of ultrafine atmospheric particles: a review of observations, J. Aerosol Sci., 35, 143–176, https://doi.org/10.1016/j.jaerosci.2003.10.003, 2004. a, b
Kulmala, M., Petäjä, T., Nieminen, T., Sipilä, M., Manninen, H. E., Lehtipalo, K., Dal Maso, M., Aalto, P. P., Junninen, H., Paasonen, P., Riipinen, I., Lehtinen, K. E. J., Laaksonen, A., and Kerminen, V.-M.: Measurement of the nucleation of atmospheric aerosol particles, Nat. Protoc., 7, 1651–1667, https://doi.org/10.1038/nprot.2012.091, 2012. a, b, c, d, e, f, g, h
Leino, K., Lampilahti, J., Poutanen, P., Väänänen, R., Manninen, A., Buenrostro Mazon, S., Dada, L., Franck, A., Wimmer, D., Aalto, P. P., Ahonen, L. R., Enroth, J., Kangasluoma, J., Keronen, P., Korhonen, F., Laakso, H., Matilainen, T., Siivola, E., Manninen, H. E., Lehtipalo, K., Kerminen, V.-M., Petäjä, T., and Kulmala, M.: Vertical profiles of sub-3 nm particles over the boreal forest, Atmos. Chem. Phys., 19, 4127–4138, https://doi.org/10.5194/acp-19-4127-2019, 2019. a
Liu, Y., Brito, J., Dorris, M. R., Rivera-Rios, J. C., Seco, R., Bates, K. H., Artaxo, P., Duvoisin, S., Keutsch, F. N., Kim, S., Goldstein, A. H., Guenther, A. B., Manzi, A. O., Souza, R. A. F., Springston, S. R., Watson, T. B., McKinney, K. A., and Martin, S. T.: Isoprene photochemistry over the Amazon rainforest, P. Natl. Acad. Sci. USA, 113, 6125–6130, 2016. a
Löbs, N., Barbosa, C. G. G., Brill, S., Walter, D., Ditas, F., de Oliveira Sá, M., de Araújo, A. C., de Oliveira, L. R., Godoi, R. H. M., Wolff, S., Piepenbring, M., Kesselmeier, J., Artaxo, P., Andreae, M. O., Pöschl, U., Pöhlker, C., and Weber, B.: Aerosol measurement methods to quantify spore emissions from fungi and cryptogamic covers in the Amazon, Atmos. Meas. Tech., 13, 153–164, https://doi.org/10.5194/amt-13-153-2020, 2020. a
Ma, N. and Birmili, W.: Estimating the contribution of photochemical particle formation to ultrafine particle number averages in an urban atmosphere, Sci. Total Environ., 512, 154–166, 2015. a
Machado, L. A., Laurent, H., and Lima, A. A.: Diurnal march of the convection observed during TRMM-WETAMC/LBA, J. Geophys. Res.-Atmos., 107, 8064, https://doi.org/10.1029/2001JD000338, 2002. a, b
Machado, L. A. T. and Rossow, W. B.: Structural characteristics and radiative properties of tropical cloud clusters, Mon. Weather Rev., 121, 3234–3260, 1993. a
Machado, L. A. T., Franco, M. A., Kremper, L. A., Ditas, F., Andreae, M. O., Artaxo, P., Cecchini, M. A., Holanda, B. A., Pöhlker, M. L., Saraiva, I., Wolff, S., Pöschl, U., and Pöhlker, C.: How weather events modify aerosol particle size distributions in the Amazon boundary layer, Atmos. Chem. Phys., 21, 18065–18086, https://doi.org/10.5194/acp-21-18065-2021, 2021. a, b, c, d, e, f, g, h
Martin, S. T., Andreae, M. O., Althausen, D., Artaxo, P., Baars, H., Borrmann, S., Chen, Q., Farmer, D. K., Guenther, A., Gunthe, S. S., Jimenez, J. L., Karl, T., Longo, K., Manzi, A., Müller, T., Pauliquevis, T., Petters, M. D., Prenni, A. J., Pöschl, U., Rizzo, L. V., Schneider, J., Smith, J. N., Swietlicki, E., Tota, J., Wang, J., Wiedensohler, A., and Zorn, S. R.: An overview of the Amazonian Aerosol Characterization Experiment 2008 (AMAZE-08), Atmos. Chem. Phys., 10, 11415–11438, https://doi.org/10.5194/acp-10-11415-2010, 2010. a
McFiggans, G., Mentel, T. F.,Wildt, J., Pullinen, I., Kang, S., Kleist, E., Schmitt, S., Springer, M., Tillmann, R., Wu, C., Zhao, D., Hallquist, M., Faxon, C., Le Breton, M., Hallquist, Å. M., Simpson, D., Bergström, R., Jenkin, M. E., Ehn, M., Thornton, J. A., Alfarra, M. R., Bannan, T. J., Percival, C. J., Priestley, M., Topping, D., and Kiendler-Scharr, A.: Secondary organic aerosol reduced by mixture of atmospheric vapours, Nature, 565, 587–593, 2019. a
Merikanto, J., Spracklen, D. V., Mann, G. W., Pickering, S. J., and Carslaw, K. S.: Impact of nucleation on global CCN, Atmos. Chem. Phys., 9, 8601–8616, https://doi.org/10.5194/acp-9-8601-2009, 2009. a
Moran-Zuloaga, D., Ditas, F., Walter, D., Saturno, J., Brito, J., Carbone, S., Chi, X., Hrabě de Angelis, I., Baars, H., Godoi, R. H. M., Heese, B., Holanda, B. A., Lavrič, J. V., Martin, S. T., Ming, J., Pöhlker, M. L., Ruckteschler, N., Su, H., Wang, Y., Wang, Q., Wang, Z., Weber, B., Wolff, S., Artaxo, P., Pöschl, U., Andreae, M. O., and Pöhlker, C.: Long-term study on coarse mode aerosols in the Amazon rain forest with the frequent intrusion of Saharan dust plumes, Atmos. Chem. Phys., 18, 10055–10088, https://doi.org/10.5194/acp-18-10055-2018, 2018. a, b, c, d, e
Nascimento, J. P., Bela, M. M., Meller, B. B., Banducci, A. L., Rizzo, L. V., Vara-Vela, A. L., Barbosa, H. M. J., Gomes, H., Rafee, S. A. A., Franco, M. A., Carbone, S., Cirino, G. G., Souza, R. A. F., McKeen, S. A., and Artaxo, P.: Aerosols from anthropogenic and biogenic sources and their interactions – modeling aerosol formation, optical properties, and impacts over the central Amazon basin, Atmos. Chem. Phys., 21, 6755–6779, https://doi.org/10.5194/acp-21-6755-2021, 2021. a
Nieminen, T., Kerminen, V.-M., Petäjä, T., Aalto, P. P., Arshinov, M., Asmi, E., Baltensperger, U., Beddows, D. C. S., Beukes, J. P., Collins, D., Ding, A., Harrison, R. M., Henzing, B., Hooda, R., Hu, M., Hõrrak, U., Kivekäs, N., Komsaare, K., Krejci, R., Kristensson, A., Laakso, L., Laaksonen, A., Leaitch, W. R., Lihavainen, H., Mihalopoulos, N., Németh, Z., Nie, W., O'Dowd, C., Salma, I., Sellegri, K., Svenningsson, B., Swietlicki, E., Tunved, P., Ulevicius, V., Vakkari, V., Vana, M., Wiedensohler, A., Wu, Z., Virtanen, A., and Kulmala, M.: Global analysis of continental boundary layer new particle formation based on long-term measurements, Atmos. Chem. Phys., 18, 14737–14756, https://doi.org/10.5194/acp-18-14737-2018, 2018. a, b, c, d, e, f, g, h
Perry, K. D. and Hobbs, P. V.: Further evidence for particle nucleation in clear air adjacent to marine cumulus clouds, J. Geophys. Res.-Atmos., 99, 22803–22818, 1994. a
Pöhlker, C., Wiedemann, K. T., Sinha, B., Shiraiwa, M., Gunthe, S. S., Smith, M., Su, H., Artaxo, P., Chen, Q., Cheng, Y., Elbert, W., Gilles, M. K., Kilcoyne, A. L. D., Moffet, R. C., Weigand, M., Martin, S. T., Pöschl, U., and Andreae, M. O.: Biogenic potassium salt particles as seeds for secondary organic aerosol in the Amazon, Science, 337, 1075–1078, https://doi.org/10.1126/science.1223264, 2012. a, b, c
Pöhlker, C., Walter, D., Paulsen, H., Könemann, T., Rodríguez-Caballero, E., Moran-Zuloaga, D., Brito, J., Carbone, S., Degrendele, C., Després, V. R., Ditas, F., Holanda, B. A., Kaiser, J. W., Lammel, G., Lavrič, J. V., Ming, J., Pickersgill, D., Pöhlker, M. L., Praß, M., Löbs, N., Saturno, J., Sörgel, M., Wang, Q., Weber, B., Wolff, S., Artaxo, P., Pöschl, U., and Andreae, M. O.: Land cover and its transformation in the backward trajectory footprint region of the Amazon Tall Tower Observatory, Atmos. Chem. Phys., 19, 8425–8470, https://doi.org/10.5194/acp-19-8425-2019, 2019. a, b, c
Pöhlker, M. L., Pöhlker, C., Ditas, F., Klimach, T., Hrabe de Angelis, I., Araújo, A., Brito, J., Carbone, S., Cheng, Y., Chi, X., Ditz, R., Gunthe, S. S., Kesselmeier, J., Könemann, T., Lavrič, J. V., Martin, S. T., Mikhailov, E., Moran-Zuloaga, D., Rose, D., Saturno, J., Su, H., Thalman, R., Walter, D., Wang, J., Wolff, S., Barbosa, H. M. J., Artaxo, P., Andreae, M. O., and Pöschl, U.: Long-term observations of cloud condensation nuclei in the Amazon rain forest – Part 1: Aerosol size distribution, hygroscopicity, and new model parametrizations for CCN prediction, Atmos. Chem. Phys., 16, 15709–15740, https://doi.org/10.5194/acp-16-15709-2016, 2016. a, b, c, d, e, f
Pöhlker, M. L., Ditas, F., Saturno, J., Klimach, T., Hrabě de Angelis, I., Araùjo, A. C., Brito, J., Carbone, S., Cheng, Y., Chi, X., Ditz, R., Gunthe, S. S., Holanda, B. A., Kandler, K., Kesselmeier, J., Könemann, T., Krüger, O. O., Lavrič, J. V., Martin, S. T., Mikhailov, E., Moran-Zuloaga, D., Rizzo, L. V., Rose, D., Su, H., Thalman, R., Walter, D., Wang, J., Wolff, S., Barbosa, H. M. J., Artaxo, P., Andreae, M. O., Pöschl, U., and Pöhlker, C.: Long-term observations of cloud condensation nuclei over the Amazon rain forest – Part 2: Variability and characteristics of biomass burning, long-range transport, and pristine rain forest aerosols, Atmos. Chem. Phys., 18, 10289–10331, https://doi.org/10.5194/acp-18-10289-2018, 2018. a, b, c, d, e, f, g
Prass, M., Andreae, M. O., de Araùjo, A. C., Artaxo, P., Ditas, F., Elbert, W., Förster, J.-D., Franco, M. A., Hrabe de Angelis, I., Kesselmeier, J., Klimach, T., Kremper, L. A., Thines, E., Walter, D., Weber, J., Weber, B., Fuchs, B. M., Pöschl, U., and Pöhlker, C.: Bioaerosols in the Amazon rain forest: temporal variations and vertical profiles of Eukarya, Bacteria, and Archaea, Biogeosciences, 18, 4873–4887, https://doi.org/10.5194/bg-18-4873-2021, 2021. a
Rissler, J., Swietlicki, E., Zhou, J., Roberts, G., Andreae, M. O., Gatti, L. V., and Artaxo, P.: Physical properties of the sub-micrometer aerosol over the Amazon rain forest during the wet-to-dry season transition - comparison of modeled and measured CCN concentrations, Atmos. Chem. Phys., 4, 2119–2143, https://doi.org/10.5194/acp-4-2119-2004, 2004. a
Rissler, J., Vestin, A., Swietlicki, E., Fisch, G., Zhou, J., Artaxo, P., and Andreae, M. O.: Size distribution and hygroscopic properties of aerosol particles from dry-season biomass burning in Amazonia, Atmos. Chem. Phys., 6, 471–491, https://doi.org/10.5194/acp-6-471-2006, 2006. a, b
Roberts, G. C.: Impact of biomass burning on cloud properties in the Amazon Basin, J. Geophys. Res., 108, 4062, https://doi.org/10.1029/2001JD000985, 2003. a
Roberts, G. C., Andreae, M. O., Zhou, J., and Artaxo, P.: Cloud condensation nuclei in the Amazon Basin: “marine” conditions over a continent?, Geophys. Res. Lett., 28, 2807–2810, https://doi.org/10.1029/2000GL012585, 2001. a, b, c
Rose, C., Zha, Q., Dada, L., Yan, C., Lehtipalo, K., Junninen, H., Mazon, S. B., Jokinen, T., Sarnela, N., Sipilä, M., Petäjä, T., Kerminen, V.-M., Bianchi, F., and Kulmala, M.: Observations of biogenic ion-induced cluster formation in the atmosphere, Sci. Adv., 4, eaar5218, https://doi.org/10.1038/s41586-018-0871-y, 2018. a
Saturno, J., Ditas, F., Penning de Vries, M., Holanda, B. A., Pöhlker, M. L., Carbone, S., Walter, D., Bobrowski, N., Brito, J., Chi, X., Gutmann, A., Hrabe de Angelis, I., Machado, L. A. T., Moran-Zuloaga, D., Rüdiger, J., Schneider, J., Schulz, C., Wang, Q., Wendisch, M., Artaxo, P., Wagner, T., Pöschl, U., Andreae, M. O., and Pöhlker, C.: African volcanic emissions influencing atmospheric aerosols over the Amazon rain forest, Atmos. Chem. Phys., 18, 10391–10405, https://doi.org/10.5194/acp-18-10391-2018, 2018a. a, b, c
Saturno, J., Holanda, B. A., Pöhlker, C., Ditas, F., Wang, Q., Moran-Zuloaga, D., Brito, J., Carbone, S., Cheng, Y., Chi, X., Ditas, J., Hoffmann, T., Hrabe de Angelis, I., Könemann, T., Lavrič, J. V., Ma, N., Ming, J., Paulsen, H., Pöhlker, M. L., Rizzo, L. V., Schlag, P., Su, H., Walter, D., Wolff, S., Zhang, Y., Artaxo, P., Pöschl, U., and Andreae, M. O.: Black and brown carbon over central Amazonia: long-term aerosol measurements at the ATTO site, Atmos. Chem. Phys., 18, 12817–12843, https://doi.org/10.5194/acp-18-12817-2018, 2018b. a, b
Schrod, J., Thomson, E. S., Weber, D., Kossmann, J., Pöhlker, C., Saturno, J., Ditas, F., Artaxo, P., Clouard, V., Saurel, J.-M., Ebert, M., Curtius, J., and Bingemer, H. G.: Long-term deposition and condensation ice-nucleating particle measurements from four stations across the globe, Atmos. Chem. Phys., 20, 15983–16006, https://doi.org/10.5194/acp-20-15983-2020, 2020. a
Spracklen, D. V., Carslaw, K. S., Kulmala, M., Kerminen, V.-M., Sihto, S.-L., Riipinen, I., Merikanto, J., Mann, G. W., Chipperfield, M. P., Wiedensohler, A., Birmili, W., and Lihavainen, H.: Contribution of particle formation to global cloud condensation nuclei concentrations, Geophys. Res. Lett., 35, L06808, https://doi.org/10.1029/2007GL033038, 2008. a
Talbot, R., Andreae, M., Berresheim, H., Artaxo, P., Garstang, M., Harriss, R., Beecher, K., and Li, S.: Aerosol chemistry during the wet season in central Amazonia: The influence of long-range transport, J. Geophys. Res.-Atmos., 95, 16955–16969, 1990. a
Trebs, I., Meixner, F. X., Slanina, J., Otjes, R., Jongejan, P., and Andreae, M. O.: Real-time measurements of ammonia, acidic trace gases and water-soluble inorganic aerosol species at a rural site in the Amazon Basin, Atmos. Chem. Phys., 4, 967–987, https://doi.org/10.5194/acp-4-967-2004, 2004. a
Tuch, T. M., Haudek, A., Müller, T., Nowak, A., Wex, H., and Wiedensohler, A.: Design and performance of an automatic regenerating adsorption aerosol dryer for continuous operation at monitoring sites, Atmos. Meas. Tech., 2, 417–422, https://doi.org/10.5194/amt-2-417-2009, 2009. a
Varanda Rizzo, L., Roldin, P., Brito, J., Backman, J., Swietlicki, E., Krejci, R., Tunved, P., Petäjä, T., Kulmala, M., and Artaxo, P.: Multi-year statistical and modeling analysis of submicrometer aerosol number size distributions at a rain forest site in Amazonia, Atmos. Chem. Phys., 18, 10255–10274, https://doi.org/10.5194/acp-18-10255-2018, 2018. a, b, c, d, e, f, g, h, i, j, k, l, m, n, o
von der Weiden, S.-L., Drewnick, F., and Borrmann, S.: Particle Loss Calculator – a new software tool for the assessment of the performance of aerosol inlet systems, Atmos. Meas. Tech., 2, 479–494, https://doi.org/10.5194/amt-2-479-2009, 2009. a, b, c
Waddicor, D. A., Vaughan, G., Choularton, T. W., Bower, K. N., Coe, H., Gallagher, M., Williams, P. I., Flynn, M., Volz-Thomas, A., Pätz, H.-W., Isaac, P., Hacker, J., Arnold, F., Schlager, H., and Whiteway, J. A.: Aerosol observations and growth rates downwind of the anvil of a deep tropical thunderstorm, Atmos. Chem. Phys., 12, 6157–6172, https://doi.org/10.5194/acp-12-6157-2012, 2012. a
Wang, J., Krejci, R., Giangrande, S., Kuang, C., Barbosa, H. M. J., Brito, J., Carbone, S., Chi, X., Comstock, J., Ditas, F., Lavric, J., Manninen, H. E., Mei, F., Moran-Zuloaga, D., Pöhlker, C., Pöhlker, M. L., Saturno, J., Schmid, B., Souza, R. A. F., Springston, S. R., Tomlinson, J. M., Toto, T., Walter, D., Wimmer, D., Smith, J. N., Kulmala, M., Machado, L. A. T., Artaxo, P., Andreae, M. O., Petäjä, T., and Martin, S. T.: Amazon boundary layer aerosol concentration sustained by vertical transport during rainfall, Nature, 539, 416–419, https://doi.org/10.1038/nature19819, 2016. a, b, c, d, e, f, g, h, i, j, k, l, m, n, o, p
Wehner, B., Werner, F., Ditas, F., Shaw, R. A., Kulmala, M., and Siebert, H.: Observations of new particle formation in enhanced UV irradiance zones near cumulus clouds, Atmos. Chem. Phys., 15, 11701–11711, https://doi.org/10.5194/acp-15-11701-2015, 2015. a
Wiedensohler, A., Ma, N., Birmili, W., Heintzenberg, J., Ditas, F., Andreae, M., and Panov, A.: Infrequent new particle formation over the remote boreal forest of Siberia, Atmos. Environ., 200, 167–169, https://doi.org/10.1016/j.atmosenv.2018.12.013, 2019. a
Williamson, C. J., Kupc, A., Axisa, D., Bilsback, K. R., Bui, T., Campuzano-Jost, P., Dollner, M., Froyd, K., Hodshire, A. L., Jimenez, J. L., Kodros, J. K., Luo, G., Murphy, D. M., Nault, B. A., Ray, E. A., Weinzierl, B. B., Wilson, J. C., Yu, F., Yu, P., Pierce, J. F., and Brock C. A.: A large source of cloud condensation nuclei from new particle formation in the tropics, Nature, 574, 399–403, 2019. a, b, c
Wimmer, D., Buenrostro Mazon, S., Manninen, H. E., Kangasluoma, J., Franchin, A., Nieminen, T., Backman, J., Wang, J., Kuang, C., Krejci, R., Brito, J., Goncalves Morais, F., Martin, S. T., Artaxo, P., Kulmala, M., Kerminen, V.-M., and Petäjä, T.: Ground-based observation of clusters and nucleation-mode particles in the Amazon, Atmos. Chem. Phys., 18, 13245–13264, https://doi.org/10.5194/acp-18-13245-2018, 2018. a, b, c, d
Yáñez-Serrano, A. M., Bourtsoukidis, E., Alves, E. G., Bauwens, M., Stavrakou, T., Llusià, J., Filella, I., Guenther, A., Williams, J., Artaxo, P., Sindelarova, K., Doubalova, J., Kesselmeier, J., and Peñuelas, J.: Amazonian biogenic volatile organic compounds under global change, Glob. Change Biol., 26, 4722–4751, 2020. a
Yli-Juuti, T., Mohr, C., and Riipinen, I.: Open questions on atmospheric nanoparticle growth, Commun. Chem., 3, 1–4, 2020. a, b, c
Zha, Q., Yan, C., Junninen, H., Riva, M., Sarnela, N., Aalto, J., Quéléver, L., Schallhart, S., Dada, L., Heikkinen, L., Peräkylä, O., Zou, J., Rose, C., Wang, Y., Mammarella, I., Katul, G., Vesala, T., Worsnop, D. R., Kulmala, M., Petäjä, T., Bianchi, F., and Ehn, M.: Vertical characterization of highly oxygenated molecules (HOMs) below and above a boreal forest canopy, Atmos. Chem. Phys., 18, 17437–17450, https://doi.org/10.5194/acp-18-17437-2018, 2018. a
Zhao, B., Shrivastava, M., Donahue, N. M., Gordon, H., Schervish, M., Shilling, J. E., Zaveri, R. A., Wang, J., Andreae, M. O., Zhao, C., Gaudet, B., Liu, Y., Fan, J. W., and Fast, J. D.: High concentration of ultrafine particles in the Amazon free troposphere produced by organic new particle formation, P. Natl. Acad. Sci., 117, 25344–25351, 2020. a, b, c
Zhou, J.: Submicrometer aerosol particle size distribution and hygroscopic growth measured in the Amazon rain forest during the wet season, J. Geophys. Res., 107, 8055, https://doi.org/10.1029/2000JD000203, 2002. a, b