the Creative Commons Attribution 4.0 License.
the Creative Commons Attribution 4.0 License.
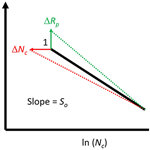
Factors affecting precipitation formation and precipitation susceptibility of marine stratocumulus with variable above- and below-cloud aerosol concentrations over the Southeast Atlantic
Greg M. McFarquhar
Joseph R. O'Brien
Michael R. Poellot
David J. Delene
Rose M. Miller
Jennifer D. Small Griswold
Aerosol–cloud–precipitation interactions (ACIs) provide the greatest source of uncertainties in predicting changes in Earth's energy budget due to poor representation of marine stratocumulus and the associated ACIs in climate models. Using in situ data from 329 cloud profiles across 24 research flights from the NASA ObseRvations of Aerosols above CLouds and their intEractionS (ORACLES) field campaign in September 2016, August 2017, and October 2018, it is shown that contact between above-cloud biomass burning aerosols and marine stratocumulus over the Southeast Atlantic Ocean was associated with precipitation suppression and a decrease in the precipitation susceptibility (So) to aerosols. The 173 “contact” profiles with aerosol concentration (Na) greater than 500 cm−3 within 100 m above cloud tops had a 50 % lower precipitation rate (Rp) and a 20 % lower So, on average, compared to 156 “separated” profiles with Na less than 500 cm−3 up to at least 100 m above cloud tops.
Contact and separated profiles had statistically significant differences in droplet concentration (Nc) and effective radius (Re) (95 % confidence intervals from a two-sample t test are reported). Contact profiles had 84 to 90 cm−3 higher Nc and 1.4 to 1.6 µm lower Re compared to separated profiles. In clean boundary layers (below-cloud Na less than 350 cm−3), contact profiles had 25 to 31 cm−3 higher Nc and 0.2 to 0.5 µm lower Re. In polluted boundary layers (below-cloud Na exceeding 350 cm−3), contact profiles had 98 to 108 cm−3 higher Nc and 1.6 to 1.8 µm lower Re. On the other hand, contact and separated profiles had statistically insignificant differences between the average liquid water path, cloud thickness, and meteorological parameters like surface temperature, lower tropospheric stability, and estimated inversion strength. These results suggest the changes in cloud microphysical properties were driven by ACIs rather than meteorological effects, and adjustments to existing relationships between Rp and Nc in model parameterizations should be considered to account for the role of ACIs.
- Article
(3852 KB) -
Supplement
(929 KB) - BibTeX
- EndNote
Clouds drive the global hydrological cycle with an annual average precipitation rate of 3 mm d−1 over the oceans (Behrangi et al., 2014). Marine stratocumulus cloud (MSC) is the most common cloud type with an annual coverage of 22 % over the ocean surface (Eastman et al., 2011). These low-level, boundary layer clouds typically exist over subtropical oceans in regions with large-scale subsidence such as the Southeast Atlantic Ocean (Klein and Hartmann, 1993). MSCs have higher reflectivity (albedo) than the ocean surface, which results in a strong, negative shortwave cloud radiative forcing (CRF) with a weak and positive longwave CRF (Oreopoulos and Rossow, 2011).
Low-cloud cover in the subsidence regions is negatively correlated with sea surface temperature (SST) (Eastman et al., 2011; Wood and Hartmann, 2006). CRF is thus sensitive to changes in SST, but there is a large spread in model estimates of CRF sensitivity (Bony and Dufresne, 2005). This provides uncertainty in the model estimates of Earth's energy budget in future climate scenarios (Trenberth and Fasullo, 2009). Uncertainty in the parameterization of boundary layer aerosol, cloud, and precipitation processes contributes to model uncertainties (Ahlgrimm and Forbes, 2014; Stephens et al., 2010).
MSC CRF is regulated by cloud processes that depend on cloud microphysical properties, like droplet concentration (Nc), effective radius (Re), and liquid water content (LWC), and macrophysical properties, like cloud thickness (H) and liquid water path (LWP). These cloud properties can depend on the concentration, composition, and size distributions of aerosols, which act as cloud condensation nuclei. Under conditions of constant LWC, increases in aerosol concentration (Na) can increase Nc and decrease Re, strengthening the shortwave CRF (Twomey, 1974, 1977). A decrease in droplet sizes in polluted clouds can inhibit droplet growth from collision–coalescence and suppress precipitation intensity, resulting in lower precipitation rate (Rp), higher LWP, and increased cloud lifetime (Albrecht, 1989). In combination, these aerosol–cloud–precipitation interactions (ACIs) and the resulting cloud adjustments lead to an effective radiative forcing, termed ERFaci (Boucher et al., 2013).
Satellite retrievals of Re and cloud optical thickness (τ) can be used to estimate Nc and LWP using the adiabatic assumption (Boers et al., 2006; Wood and Hartmann, 2006; Bennartz, 2007). LWC increases linearly with height in adiabatic clouds, and τ is parameterized as a function of Nc and LWP (LWP) (Brenguier et al., 2000). Since τ has greater sensitivity to LWP compared to Nc, assuming constant LWP under different aerosol conditions can lead to underestimation of the cloud albedo susceptibility to aerosol perturbations (Platnick and Twomey, 1994; McComiskey and Feingold, 2012).
LWP can have a positive or negative response to increasing Nc due to aerosols (Toll et al., 2019). The LWP response is regulated by environmental conditions (e.g., lower tropospheric stability (LTS), boundary layer depth (HBL), and relative humidity), cloud particle sizes (e.g., represented by Re), Rp, and by Nc and LWP themselves (Chen et al., 2014; Gryspeerdt et al., 2019; Toll et al., 2019; Possner et al., 2020). Accurate estimation of the LWP response to aerosol perturbations is important for regional and global estimates of ERFaci (Douglas and L'Ecuyer, 2019, 2020).
Droplet evaporation associated with cloud-top entrainment and precipitation constitutes the two major sinks of LWC in MSC. Smaller droplets associated with higher Nc or Na evaporate more readily, which leads to greater cloud-top evaporative cooling and a negative LWP response (Hill et al., 2008). The LWP response to the evaporation–entrainment feedback (Xue and Feingold, 2006; Small et al., 2009) also depends on above-cloud humidity (Ackerman et al., 2004). Precipitation susceptibility (So) to aerosol-induced changes in cloud properties is related to the change in Rp due to aerosol-induced changes in Nc and is a function of LWP or H (Feingold and Seibert, 2009).
The magnitude of So depends on precipitation formation processes like collision–coalescence which are parameterized in models using mass transfer rates, such as the autoconversion rate (SAUTO) and the accretion rate (SACC) (Morrison and Gettelman, 2008; Geoffroy et al., 2010). Autoconversion describes the process of collisions between cloud droplets that coalesce to form drizzle drops which initiate precipitation. Accretion refers to collisions between cloud droplets and drizzle drops which lead to larger drizzle drops and greater precipitation intensity. The variability in So as a function of LWP or H depends on the cloud type and the ratio of SACC versus SAUTO (Wood et al., 2009; Jiang et al., 2010; Sorooshian et al., 2010).
Recent field campaigns focused on studying ACIs over the Southeast Atlantic Ocean because unique meteorological conditions are present in the region (Zuidema et al., 2016; Redemann et al., 2021). Biomass burning aerosols from southern Africa are lofted into the free troposphere (Gui et al., 2021) and transported over the Southeast Atlantic by mid-tropospheric winds where the aerosols overlay an extensive MSC deck that exists off the coast of Namibia and Angola (Adebiyi and Zuidema, 2016; Devasthale and Thomas, 2011). The above-cloud aerosol plume is associated with elevated water vapor content (Pistone et al., 2021), which influences cloud-top humidity and dynamics following the mechanisms discussed by Ackerman et al. (2004). In situ observations of cloud and aerosol properties were collected over the Southeast Atlantic during the NASA ObseRvations of Aerosols above CLouds and their intEractionS (ORACLES) field campaign during three Intensive Observation Periods (IOPs) in September 2016, August 2017, and October 2018 (Redemann et al., 2021).
During ORACLES, the aerosol layer was comprised of shortwave-absorbing aerosols (500 nm single-scattering albedo of about 0.83), with above-cloud aerosol optical depth up to 0.42 (Pistone et al., 2019; LeBlanc et al., 2020). The sign of the forcing due to shortwave absorption by the aerosol layer depends on the location of aerosols in the vertical column and the albedo of the underlying clouds (Cochrane et al., 2019). Warming aloft due to aerosol absorption of solar radiation strengthens the temperature inversion, which decreases dry air entrainment into clouds, increases LWP and cloud albedo, and decreases the shortwave CRF (Wilcox, 2010). The net radiative forcing due to the aerosol and cloud layers thus depends on aerosol-induced changes in Nc, Re, and LWP and the resulting changes in τ. Sinks of Nc and LWP like precipitation and entrainment mixing lead to uncertainties in satellite retrievals of Nc, which pose the biggest challenge in the use of satellite retrievals to study the aerosol impact on Nc (Quaas et al., 2020). This motivates observational studies of ACIs that examine Nc and LWP under different aerosol and meteorological conditions.
During the 2016 IOP, variable vertical displacement (0 to 2000 m) was observed between above-cloud aerosols and the MSC (Gupta et al., 2021; hereafter G21). Instances of contact and separation between the aerosol and cloud layers were associated with differences in the above- and below-cloud Na, water vapor mixing ratio (wv), and cloud-top entrainment processes. These differences led to changes in Nc, Re, and LWC and their vertical profiles (G21). In this study, the response of the MSC to above- and below-cloud aerosols is further examined using data from all three ORACLES IOPs, and precipitation formation and So are evaluated as a function of H.
The paper is organized as follows. In Sect. 2, the ORACLES observations are discussed, along with the data quality assurance procedures (additional details are in the Supplement). In Sect. 3, the calculation of cloud properties is described. In Sect. 4, the influence of aerosols on Nc, Re, and LWC is examined by comparing the parameters for MSC in contact or separated from the above-cloud aerosol layer. In Sect. 5, the changes in precipitation formation due to aerosol-induced microphysical changes are examined. In Sect. 6, Nc, Rp, and So are examined as a function of H and the above- and below-cloud Na. In Sect. 7, the meteorological conditions are examined using reanalysis data. In Sect. 8, the conclusions are summarized with directions for future work.
The ORACLES IOPs were based at Walvis Bay, Namibia (23∘ S, 14.6∘ E), in September 2016, and at São Tomé and Príncipe (0.3∘ N, 6.7∘ E) in August 2017 and October 2018. The data analyzed in this study were collected during the three IOPs (Table 1 and Fig. 1): six P-3 research flights (PRFs) from 6 to 25 September 2016 with cloud sampling conducted between 1∘ W to 12∘ E and 9 to 20∘ S; seven PRFs from 12 to 28 August 2017 with cloud sampling conducted between 8∘ W to 6∘ E and 2 to 15∘ S; and 11 PRFs from 27 September to 23 October 2018 with cloud sampling conducted between 3∘ W to 9∘ E and 1∘ N to 15∘ S. These PRFs were selected because in situ cloud sampling was conducted during at least three vertical profiles through the cloud layer (Table 1).
Table 1The number of cloud profiles (n) for P-3 research flights (PRFs) analyzed in the study, number of contact and separated profiles with sampling time in parentheses, and instruments that provided valid samples of droplets with D<50 µm (instrument used for analysis is in bold).
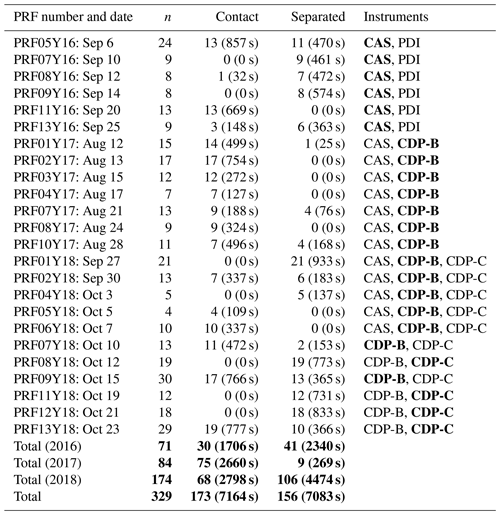
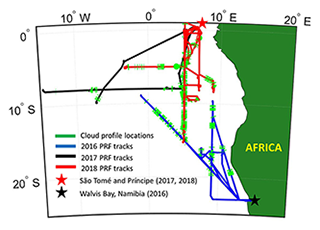
Figure 1PRF tracks from ORACLES IOPs with base of operations and cloud sampling locations (tracks for multiple 2017 and 2018 PRFs overlap along 5∘ E).
Three PRFs from the 2016 IOP had overlapping tracks when the P-3B aircraft flew northwest from 23∘ S, 13.5∘ E toward 10∘ S, 0∘ E and returned along the same track (Fig. 1). The 2017 and 2018 IOPs had 10 PRFs with overlapping flight tracks when the aircraft flew south from 0∘ N, 5∘ E toward 15∘ S, 5∘ E and returned along the same track. PRFs with overlapping tracks acquired statistics for model evaluation (Doherty et al., 2022), while the other PRFs targeted specific locations based on meteorological conditions (Redemann et al., 2021).
During ORACLES, the NASA P-3B aircraft was equipped with in situ probes. The data analyzed in this study were collected using Cloud Droplet Probes (CDPs) (Lance et al., 2010), a cloud and aerosol spectrometer (CAS) on the cloud, aerosol and precipitation spectrometer (Baumgardner et al., 2001), a phase Doppler interferometer (PDI) (Chuang et al., 2008), a two-dimensional stereo probe (2D-S) (Lawson et al., 2006), a high-volume precipitation sampler (HVPS-3) (Lawson et al., 1998), a King hot wire (King et al., 1978), and a Passive Cavity Aerosol Spectrometer Probe (PCASP) (Cai et al., 2013). A single CDP was used during the 2016 IOP (hereafter CDP-A), a second CDP (hereafter CDP-B) was added for the 2017 and 2018 IOPs, and CDP-A was replaced by a different CDP (hereafter CDP-C) for the 2018 IOP.
The CAS, CDP, King hot wire, and PCASP data were processed at the University of North Dakota using the Airborne Data Processing and Analysis processing package (Delene, 2011). The PDI data were processed at the University of Hawaii. The 2D-S and HVPS-3 data were processed using the University of Illinois/Oklahoma Optical Array Probe Processing Software (McFarquhar et al., 2018). The data processing procedures followed to reject artifacts were summarized by G21. Comparisons between the cloud probe datasets are described in the Supplement.
The King hot wire was used to sample LWC (hereafter King LWC). The PCASP was used to sample the accumulation-mode aerosols sized from 0.1 to 3.0 µm. The CAS, CDP, PDI, 2D-S, and HVPS-3 collectively sampled the number distribution function N(D) for particles with diameter D from 0.5 to 19200 µm. The size distribution covering the complete droplet size range was determined by merging the N(D) for 3<D<50 µm with the N(D) for 50<D<1050 µm from the 2D-S and the N(D) for 1050<D<19 200 µm from the HVPS-3. The HVPS-3 sampled droplets with D>1050 µm for a single 1 Hz data sample across the PRFs analyzed in this study. Measurement uncertainties in droplet sizes were expected to be within 20 % for droplets with D>5 µm from the CAS and the CDP, D>50 µm from the 2D-S, and D>750 µm from the HVPS-3 (Baumgardner et al., 2017).
During each PRF, at least two independent measurements of N(D) were made for 3<D<50 µm using the CAS, the PDI, or a CDP (Table 1). The differences between the Nc and LWC derived from the CAS, PDI, and CDP N(D) were quantified to determine if these differences were within measurement uncertainties. The LWC estimates from the CAS, PDI, and CDP were compared with the adiabatic LWC (LWCad), which represents the theoretical maximum for LWC (Brenguier et al., 2000). The N(D) for droplets with D<50 µm was determined using the probe which consistently had the LWC with better agreement with the LWCad during each IOP (see Supplement). LWCad can be used to compare LWC from different probes since it is derived using environmental conditions and does not depend on the cloud probe datasets. The relative differences between the LWCad and the LWC estimates from cloud probes provide a measure of the uncertainty associated with using one probe over the other for data analysis.
The differences between in-cloud datasets from different instruments were determined using a two-sample t test. The 95 % confidence intervals (CIs) between parameter means were reported if the differences were statistically significant. During the 2017 IOP, the CAS and the CDP-B sampled droplets with D<50 µm. The CDP-B LWC was higher than the CAS LWC (95 % CIs: 0.11 to 0.12 g m−3 higher), and the average CDP-B LWC (0.18 g m−3) had better agreement with the average LWCad (0.24 g m−3) compared to the average CAS LWC (0.08 g m−3). Thus, the CDP-B N(D) was used to represent the N(D) for droplets with D<50 µm for the 2017 IOP.
Similar results were obtained when the CAS LWC and the CDP-B LWC were compared with the LWCad for the 2018 IOP. During the 2018 IOP, the CDP-C was mounted at a different location relative to the aircraft wing compared to the CAS and CDP-B, and the positions of CDP-B and CDP-C were switched after 10 October 2018. O'Brien et al. (2022) found the CDP mounting positions had only a 6 % impact on the calculation of Nc, and the average CDP-B LWC and CDP-C LWC were within 0.02 g m−3. To maintain consistency with the 2017 IOP, data from the CDP mounted next to the CAS were used for droplets with D<50 µm for the 2018 IOP (except on 15 October 2018 when the CDP-C had a voltage issue).
During the 2016 IOP, measurements from the CDP-A were unusable for all PRFs due to an optical misalignment issue. Nevertheless, the CAS and the PDI sampled droplets with 3<D<50 µm. On average, the PDI LWC was higher than the CAS LWC (95 % CIs: 0.20 to 0.21 g m−3 higher). Since the PDI LWC was greater than the LWCad (95 % CIs: 0.04 to 0.06 g m−3 higher), it was hypothesized that the PDI LWC was an overestimate of the actual LWC. Thus, the CAS N(D) was used to represent the N(D) for droplets with D<50 µm for the 2016 IOP.
The 2D-S has two channels which concurrently sample the cloud volume. Nc and LWC were derived using data from the horizontal channel (NH and LWCH) and the vertical channel (NV and LWCV). NH and LWCH were used for the 2016 IOP because NV and LWCV were not available due to soot deposition on the inside of the receive-side mirror of the vertical channel. NH and NV as well as LWCH and LWCV were strongly correlated for the 2017 and 2018 IOPs, with Pearson's correlation coefficient R≥0.92 and the best-fit slope ≥0.90. The high correlation values suggest that little difference would have resulted from using the average of the two 2D-S channels. To maintain consistency with the 2016 IOP, NH and LWCH were used for all three IOPs.
The N(D) from the merged droplet size distribution was integrated to calculate Nc. The 1 Hz data samples with Nc>10 cm−3 and King LWC >0.05 g m−3 were defined as in-cloud measurements (G21). The PCASP N(D) was used to determine the out-of-cloud Na. In situ cloud sampling during ORACLES included flight legs when the P-3B aircraft ascended or descended through the cloud layer (hereafter cloud profiles). Data from 329 cloud profiles with just under 4 h of cloud sampling were examined (Table 1).
For every cloud profile, the cloud top height (ZT) was defined as the highest altitude with Nc>10 cm−3 and King LWC >0.05 g m−3 (Table 2). The average ZT during ORACLES was 1038±270 m, where the uncertainty estimate refers to the standard deviation. The cloud base height (ZB) was defined as the lowest altitude with Nc>10 cm−3 and King LWC >0.05 g m−3. In decoupled boundary layers, a layer of cumulus can be present below the stratocumulus layer with a gap between the cloud layers (Wood, 2012). Measurements from stratocumulus were used in this study, and ZB for the stratocumulus layer was identified as the altitude above which the King LWC increased without gaps greater than 25 m in the cloud sampling up to ZT.
The difference between ZT and ZB was defined as H. Due to aerosol-induced changes in entrainment and boundary layer stability, the aerosol impact on H and ZT can have the strongest influence on LWP adjustments associated with ACIs (Toll et al., 2019). Thus, the influence of ACIs on precipitation formation and So was examined as a function of H. Data collected during incomplete profiles of the stratocumulus or while sampling open-cell clouds (for example, on 2 October 2018) were excluded because of difficulties with estimating H for such profiles.
For each 1 Hz in-cloud data sample, the droplet size distribution was used to calculate Re following Hansen and Travis (1974), where
Based on the aircraft speed, 1 Hz data samples corresponded to roughly 5 m intervals in the vertical direction. LWC was calculated as
where ρw is the density of liquid water, and h is height in cloud above cloud base. LWC and King LWC were integrated over h from ZB to ZT to calculate LWP and King LWP, respectively. τ was calculated as
where βext is the cloud extinction, and Qext is the extinction coefficient (approximately 2 for cloud droplets, assuming geometric optics apply for visible wavelengths) (Hansen and Travis, 1974). The integrals in Eqs. (1) to (3) were converted to discrete sums for D>3 µm to consider the contributions of cloud drops and not aerosols.
According to the adiabatic model (Brenguier et al., 2000), LWCad and LWPad are functions of H (the subscript “ad” added to represent the adiabatic equivalents). These relationships help parameterize τad as
The MSCs over the Southeast Atlantic were overlaid by biomass burning aerosols from southern Africa (Adebiyi and Zuidema, 2016; Redemann et al., 2021), with instances of contact and separation between the MSC cloud tops and the base of the biomass burning aerosol layer (G21). Across the three IOPs, 173 profiles were conducted at locations where an extensive aerosol plume with Na>500 cm−3 was located within 100 m above ZT (hereafter contact profiles) (Table 1). 156 profiles were conducted at locations where the level of Na>500 cm−3 was located at least 100 m above ZT (hereafter separated profiles). About 50 % of the in situ cloud sampling across the three IOPs was conducted during contact profiles (Table 1). Due to inter-annual variability, contact profiles accounted for about 42 %, 91 %, and 39 % of the in situ cloud sampling during the 2016, 2017, and 2018 IOPs, respectively.
The average Nc and Re for all cloud profiles across the three IOPs were 157±96 cm−3 and 8.2±2.7 µm, respectively (Table 3). The high proportion of contact profiles during the 2017 IOP was associated with higher average Nc and lower average Re (229 cm−3 and 6.9 µm) compared to the 2016 IOP (150 cm−3 and 7.0 µm) and the 2018 IOP (132 cm−3 and 9.8 µm). It is possible that the use of CDP-B data for the 2017 IOP contributed to the increase in average Nc relative to the 2016 IOP. However, the difference between the average CAS Nc and the average CDP-B Nc for the 2017 IOP (12 cm−3) was lower than the difference between the average Nc for the 2016 and 2017 IOPs (79 cm−3). The difference between the Nc for these IOPs was thus primarily due to the conditions at the cloud sampling locations. The microphysical differences between the 2016 and 2017 IOPs were associated with differences in surface precipitation. Based on the W-band retrievals from the Jet Propulsion Laboratory Airborne Precipitation Radar Version 3 (APR-3), the 2017 IOP had fewer profiles with precipitation reaching the surface (13 %) compared to the 2016 IOP (34 %) (Dzambo et al., 2019).
Table 3Average values for cloud properties measured during cloud profiles from the PRFs listed in Table 1 for each IOP. Error estimates represent 1 standard deviation. R between LWP estimates and H in parentheses.
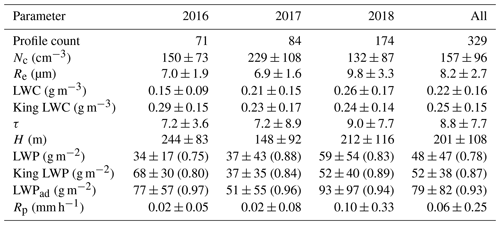
On average, contact profiles had significantly higher Nc (95 % CIs: 84 to 90 cm−3 higher) and lower Re (95 % CIs: 1.4 to 1.6 µm lower) compared to separated profiles (throughout the study, the term “significant” is exclusively used to represent statistical significance). The significant differences in Nc and Re were associated with significantly higher τ (95 % CIs: 0.04 to 3.06 higher) for contact profiles, in accordance with the Twomey effect (Twomey, 1974, 1977). These results were consistent with the 2016 IOP when the contact profiles had higher Nc (95 % CIs: 60 to 68 cm−3 higher), lower Re (95 % CIs: 1.1 to 1.3 µm lower), and higher τ (95 % CIs: 1.1 to 4.3 higher) (G21).
Figure 2 shows violin plots for cloud properties as a function of normalized height (ZN), defined as . The violin plots include box plots and illustrate the distribution of the data (Hintze and Nelson, 1998). The median Nc increased with ZN for ZN≤0.25, consistent with droplet nucleation (Fig. 2a). The median Nc decreased near cloud top for ZN≥0.75 from 204 to 154 cm−3 for contact and from 104 to 69 cm−3 for separated profiles. This is consistent with droplet evaporation associated with cloud-top entrainment (G21). The median Re increased with ZN consistent with condensational growth (Fig. 2b). There was a greater increase in the median Re from cloud base to cloud top for separated profiles (from 7.1 to 9.5 µm) compared to contact profiles (from 6.1 to 7.9 µm). This is consistent with previous observations of stronger droplet growth in cleaner conditions as a function of ZN (Braun et al., 2018; G21) and LWP (Rao et al., 2020). Statistically insignificant differences between the average H for contact and separated profiles suggest that the differential droplet growth was associated with differences in cloud processes like collision–coalescence (further discussed in Sect. 5).
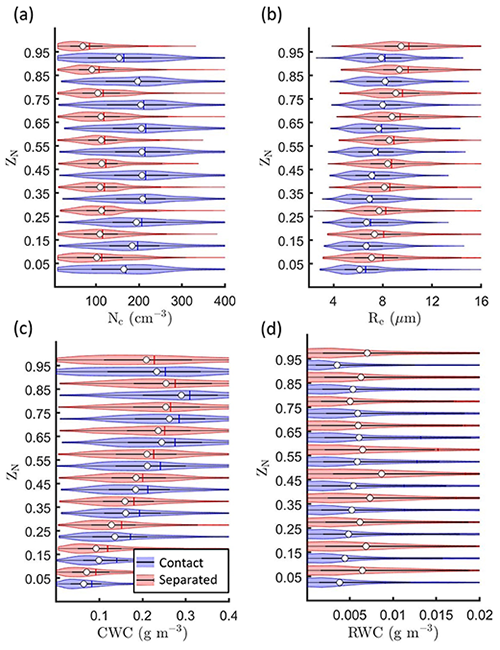
Figure 2Kernel density estimates (distribution of the data indicated by width of shaded area) and box plots showing the 25th, 50th (white circle), and 75th percentiles for (a) Nc, (b) Re, (c) CWC, and (d) RWC as a function of ZN for contact and separated profiles.
The LWC and LWP responses to changes in aerosol conditions were examined because the adiabatic model suggests (Eq. 4) (Brenguier et al., 2000). Contact profiles had significantly higher LWC, but the relative increase was less than 10 % (Table 4). LWC was divided into rainwater content (RWC) and cloud water content (CWC) based on droplet size. Droplets with D>50 µm were defined as drizzle (Abel and Boutle, 2012; Boutle et al., 2014), and the total drizzle mass was defined as RWC. The droplet mass for D<50 µm was defined as CWC. Rainwater path (RWP) and (cloud water path) CWP were defined as the vertical integrals of RWC and CWC, respectively. The median CWC increased with ZN but decreased over the top 10 % of the cloud layer for contact profiles and over the top 20 % of the cloud layer for separated profiles, consistent with cloud-top entrainment (Fig. 2c). For contact profiles, the median RWC increased with ZN before decreasing for ZN≥0.75. The median RWC for separated profiles varied with ZN. The bottom half of the cloud layer had higher median values (up to g m−3) compared to the top half (up to g m−3) (Fig. 2d).
Table 4Average and standard deviation for cloud properties measured during contact and separated profiles with 95 % confidence intervals (CIs) from a two-sample t test applied to contact and separated profile data. Positive CIs indicate higher average for contact profiles, and “insignificant” indicates statistically similar averages for contact and separated profiles.
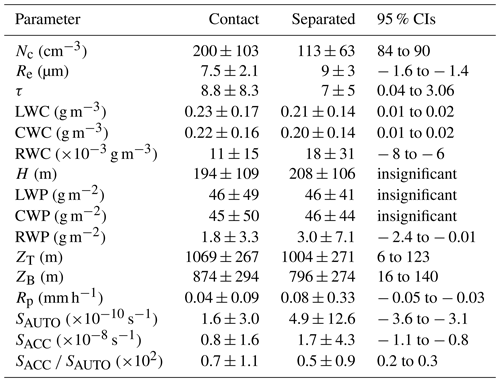
For contact profiles, there was a significant increase in the average CWC (10 %) and a significant decrease in the average RWC (60 %) compared to separated profiles (Table 4). Contact profiles also had significantly lower average RWP with insignificant differences for average CWP (Table 4). Contact profiles were located in deeper boundary layers with significantly higher ZB and ZT compared to separated profiles. However, the decrease in RWC cannot be attributed to differences in H or LWP (Kubar et al., 2009) because of statistically similar H and LWP for contact and separated profiles, on average (Table 4). These results show that instances of contact between above-cloud aerosols and the MSC were associated with more numerous and smaller cloud droplets and weaker droplet growth compared to instances of separation between the above-cloud aerosols and the MSC.
The precipitation rate Rp was calculated using the drizzle water content and fall velocity u(D) following Abel and Boutle (2012):
with fall velocity relationships from Rogers and Yau (1989) used in the computation.
Contact profiles had significantly lower Rp compared to separated profiles (95 % CIs: 0.03 to 0.05 mm h−1 lower). This suggests contact between the MSC and above-cloud biomass burning aerosols was associated with precipitation suppression. LWP and H impact the sign and magnitude of the precipitation changes in response to changes in aerosol conditions (Kubar et al., 2009; Christensen and Stephens, 2012). Thus, cloud and precipitation properties were evaluated as a function of H to examine the aerosol-induced changes in precipitation formation.
The 95th percentile was used to represent the maximum value of a variable. For example, the 95th percentile of Rp (denoted by Rp95) represents the maximum Rp during a cloud profile. Although more numerous contact profiles were drizzling compared to separated profiles, the latter had more numerous profiles with high precipitation intensity. For instance, 114 out of 173 contact and 95 out of 156 separated profiles were drizzling with Rp95>0.01 mm h−1, out of which 36 contact and 40 separated profiles had Rp95>0.1 mm h−1, and only 1 contact and 9 separated profiles had Rp95>1 mm h−1 (Fig. 3a). This is consistent with radar retrievals of surface Rp<1 mm h−1 for over 93 % of the radar profiles from 2016 and 2017 (Dzambo et al., 2019).
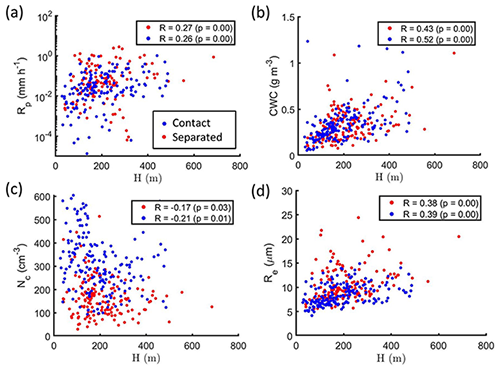
Figure 3The 95th percentile for (a) Rp, (b) CWC, (c) Nc, and (d) Re as a function of H. Each dot represents the 95th percentile from the 1 Hz measurements for a single cloud profile. Pearson's correlation coefficient (R) and p value for the correlation indicated in legend.
5.1 Microphysical properties
On average, separated profiles had greater Rp95 (0.22 mm h−1) compared to contact profiles (0.07 mm h−1). Rp95 was positively correlated with H as thicker profiles had higher precipitation intensity (Fig. 3a). The average Rp95 increased from thin (H<175 m) to thick clouds (H>175 m) from 0.04 to 0.10 mm h−1 for contact and 0.13 to 0.29 mm h−1 for separated profiles. Precipitation intensity thus decreased from separated to contact profiles for both thin and thick profiles. The average Rp95 for thin and thick contact profiles was 32 % and 37 % of the average Rp95 for thin and thick separated profiles, respectively.
CWC95 was positively correlated with H as thicker clouds had higher droplet mass (Fig. 3b). This was consistent with condensational and collision–coalescence growth continuing to occur with greater height above cloud base (Fig. 2b, c) and greater cloud depth allowing for greater droplet growth. Nc95 and Re95 were negatively and positively correlated with H, respectively (Fig. 3c, d). The trends in Nc and Re versus H were consistent with the process of collision–coalescence resulting in fewer and larger droplets.
On average, contact profiles had higher Nc95 and lower Re95 (311 cm−3 and 8.6 µm) compared to separated profiles (166 cm−3 and 10.8 µm). It can be inferred that the presence of more numerous and smaller droplets during contact profiles decreased the efficiency of collision–coalescence. Alternatively, there may not have been sufficient time for the updraft to produce the few large droplets needed to broaden the size distribution and initiate collision–coalescence. Since contact and separated profiles had statistically similar H (Table 4), the following discussion examines the link between precipitation suppression and the aerosol-induced changes in Nc, Re, and LWC and their impact on precipitation.
5.2 Precipitation properties
Precipitation formation process rates were estimated using equations used in numerical models to compare precipitation formation between contact and separated profiles. Precipitation development in models is parameterized using bulk microphysical schemes. General circulation models (GCMs) or large eddy simulation (LES) models parameterize precipitation formation using SAUTO and SACC (e.g., Penner et al., 2006; Morrison and Gettelman, 2008; Gordon et al., 2018). The most commonly used parameterizations were used to estimate equivalent rates of precipitation formation from models. SAUTO and SACC were calculated following Khairoutdinov and Kogan (2000):
and
where wc and wr are cloud water and rainwater mixing ratios, respectively, and are equal to the CWC and RWC divided by the density of air (ρa).
Contact profiles had significantly lower SAUTO and SACC compared to separated profiles (Table 4). This is consistent with significantly lower RWC and Rp for contact profiles and the association of SAUTO and SACC with precipitation onset and precipitation intensity, respectively. SAUTO95 and SACC95 were positively correlated with H (Fig. 4a, b). Separated profiles had higher SAUTO95 and SACC95 ( and s−1) compared to contact profiles ( and s−1), associated with the inverse relationship between SAUTO and Nc (Eq. 6). Faster autoconversion resulted in higher drizzle water content and greater accretion of droplets on drizzle drops.
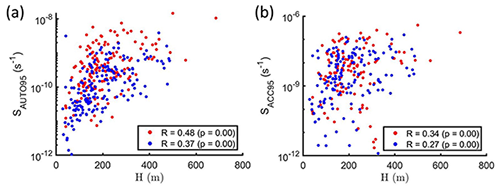
Figure 4The 95th percentile for (a) SAUTO and (b) SACC as a function of H. Each dot represents the 95th percentile from the 1 Hz measurements for a single cloud profile. R and p value for the correlation indicated in legend.
The sampling of lower Nc95 and higher Re95 compared to thinner profiles suggests that collision–coalescence was more effective in profiles with higher H (Fig. 3c, d). Thin contact profiles had the lowest SAUTO95 ( s−1), followed by thick contact ( s−1), thin separated ( s−1), and thick separated profiles ( s−1). High Nc and low CWC for thin contact profiles (Fig. 3b, c) are consistent with increased competition for cloud water leading to weaker autoconversion. It is hypothesized that these microphysical differences resulted in the lower SAUTO95 and Rp95 for thin contact profiles compared to other profiles. The differences between Rp for contact and separated profiles thus varied with H in addition to Nc, Re, and CWC. Nc, Re, and CWC varied with Na (Sect. 4), and ACIs were examined in Sects. 6 and 7.
6.1 Below-cloud Na
Polluted boundary layers in the Southeast Atlantic are associated with entrainment mixing between the free troposphere and the boundary layer (Diamond et al., 2018). Ground-based observations from Ascension Island have shown clean boundary layers can have elevated biomass burning trace gas concentrations during the burning season (Pennypacker et al., 2020). This suggests boundary layers could be clean in terms of Na despite the entrainment of biomass burning aerosols into the boundary layer due to precipitation scavenging of below-cloud aerosols. Carbon monoxide (CO) concentrations were examined since CO acts as a biomass burning tracer that is unaffected by precipitation scavenging (Pennypacker et al., 2020). For the 2016 IOP, contact profiles were located in boundary layers with significantly higher Na (95 % CIs: 93 to 115 cm−3 higher) and CO (95 % CIs: 13 to 16 ppb higher) compared to separated profiles (G21). This is consistent with data from all three IOPs when contact profiles were located in boundary layers with higher Na (95 % CIs: 231 to 249 cm−3 higher) and CO (95 % CIs: 27 to 29 ppb higher).
Following G21, 171 contact and 148 separated profiles from the IOPs were classified into four regimes: contact, high Na (C-H); contact, low Na (C-L); separated, high Na (S-H); and separated, low Na (S-L), where “low Na” meant the profile was in a boundary layer with Na<350 cm−3 up to 100 m below cloud base. Boundary layer CO concentration above 100 ppb was sampled during 107 contact and 31 separated profiles, respectively. Contact profiles were more often located in high Na boundary layers (131 out of 171 profiles classified as C-H), while separated profiles were more often located in low Na boundary layers (108 out of 148 profiles classified as S-L). This suggests contact between MSC cloud tops and above-cloud biomass burning aerosols was associated with the entrainment of biomass burning aerosols into the boundary layer.
Contact profiles had significantly higher Nc and significantly lower Re relative to separated profiles in both high Na (C-H relative to S-H) and low Na (C-L relative to S-L) boundary layers (Fig. 5, Table 5). This was associated with significantly higher above- and below-cloud Na for the contact profiles. The differences in Nc and Re were higher in high Na boundary layers, where the differences in above- and below-cloud Na were also higher compared to low Na boundary layers (Table 5). This is consistent with previous observations of MSC properties (Diamond et al., 2018; Mardi et al., 2019) and similar analysis for data from the 2016 IOP (G21).
Table 5Average values for aerosol and cloud properties from C-H, S-H, C-L, and S-L regimes (defined in text), along with differences reported as 95 % CIs.
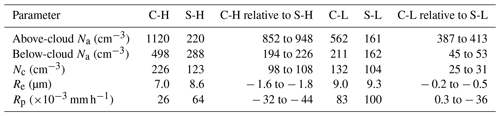
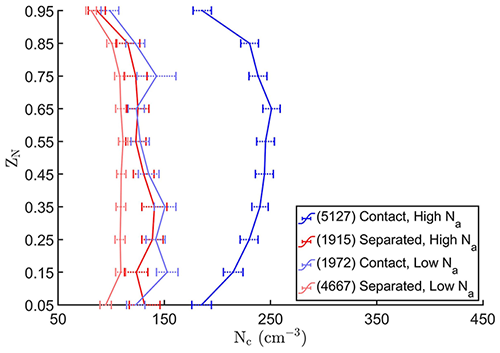
Figure 5Average Nc (error bars extend to 95 % CIs) as a function of ZN. Number of 1 Hz data points and corresponding regimes indicated in legend.
C-L profiles had significantly higher Nc (95 % CIs: 5 to 14 cm−3 higher) compared to S-H profiles, despite having significantly lower below-cloud Na (95 % CIs: 69 to 85 cm−3 lower). Significantly higher above-cloud Na for C-L profiles (95 % CIs: 321 to 361 cm−3 higher) suggests that this was associated with the influence of above-cloud Na on Nc. However, the smaller difference in Nc compared to the differences between C-H and S-H or C-L and S-L profiles suggests the combined impact of above- and below-cloud Na was stronger than the impact of above-cloud Na alone. These comparisons were qualitatively consistent when thresholds of 300 or 400 cm−3 were used to define a low Na boundary layer.
6.2 Nc and Rp versus H
The cloud profiles were divided into four populations based on H to compare Nc and Rp between different aerosols conditions, while H was constrained. The populations were divided at H=129, 175, and 256 m to ensure similar sample sizes (Table 6). For each population, contact profiles had higher Nc and lower Rp (Fig. 6a, b), consistent with comparisons averaged over all profiles (Table 4). Due to collision–coalescence, the average Nc decreased, and the average Rp increased with H (Fig. 6a, b). For contact profiles, the average Nc decreased with H from 221 to 191 cm−3, and the average Rp increased from 0.03 to 0.07 mm h−1. For separated profiles, the average Nc decreased from 149 to 92 cm−3, and the average Rp increased from 0.06 to 0.21 mm h−1 over the same range of H. C-H profiles had the highest average Nc and the lowest average Rp among the four regimes due to high above- and below-cloud Na (Fig. 6c, d). C-H profiles had the smallest increase in the average Rp with H (0.02 to 0.04 mm h−1). Conversely, low above- and below-cloud Na for S-L profiles was associated with the lowest average Nc, the highest average Rp, and the highest increase in the average Rp with H (0.12 to 0.29 mm h−1). For each regime, the average Nc decreased with H (except C-L), and the average Rp increased with H (Fig. 6c, d).
6.3 Precipitation susceptibility So
So was used to evaluate the dependence of Rp on Nc under the different aerosol conditions. So, defined as the negative slope between the natural logarithms of Rp and Nc (Feingold and Seibert, 2009), is given by
where a positive value indicates decreasing Rp with increasing Nc, in accordance with the “lifetime effect” (Albrecht, 1989). The average So across all profiles was 0.88±0.03, with lower So for contact profiles (0.87±0.04) compared to separated profiles (1.08±0.04) (Table 6). This is consistent with the hypothesis of lower values for So analogues (where Nc in Eq. (8) is replaced by Na) in the presence of above-cloud aerosols (Duong et al., 2011). So depends on the ratio of SACC to SAUTO because SACC is independent of Nc, and higher represents weaker dependence of Rp on Nc (Wood et al., 2009; Jiang et al., 2010). Lower So for contact profiles was associated with higher compared to separated profiles (Table 4).
So was calculated as a function of H using Nc and Rp for the four populations of cloud profiles (Fig. 7). The sensitivity of So to the number of populations is discussed in Appendix A. Averaged over all profiles, So had minor variations with H (e.g., 0.67, 0.68, and 0.54 as H increased) before increasing to 1.13 for H>256 m (Table 6). This trend in So versus H is consistent with previous analyses of So (Sorooshian et al., 2009; Jung et al., 2016). However, different trends emerged when So was calculated for contact and separated profiles.
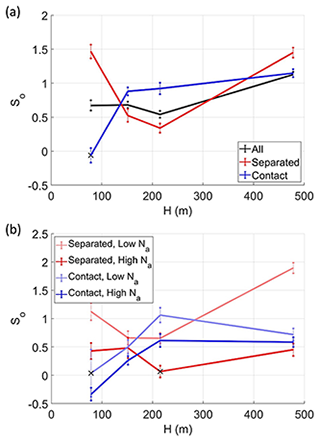
Figure 7So as a function of H (error bars extend to standard error from the regression model) for (a) contact, separated, and all profiles and (b) the regimes indicated in legend. So was statistically insignificant when marked with a cross.
The largest difference between So for contact and separated profiles was observed for thin clouds with H<129 m. The 30 separated profiles with H<129 m had the highest So (1.47±0.10) because of strong dependence of Rp on Nc. For these profiles, measurements with low Nc (<100 cm−3) had higher Rp (0.18 mm h−1) compared to measurements with higher Nc (0.01 mm h−1) (Fig. 8a). In contrast, the 52 contact profiles with H<129 m had a low and statistically insignificant value for So () due to poor (and statistically insignificant) correlation (). Poor correlation between Nc and Rp for contact profiles was associated with precipitation suppression and weaker droplet growth (Sect. 5). These factors resulted in Rp<0.03 mm h−1 independent of the Nc measurement (Fig. 8a).
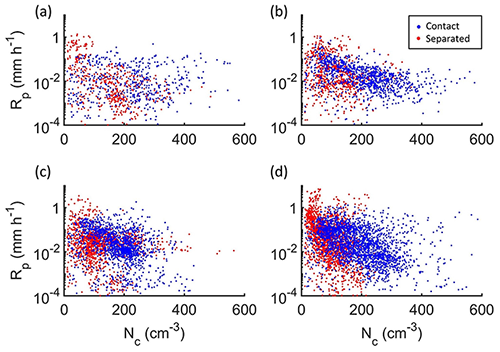
Figure 8Scatter plots of Rp and Nc for 1 Hz data points from contact and separated profiles with (a) 28<H<129 m, (b) 129<H<175 m, (c) 175<H<256 m, and (d) 256<H<700 m.
For separated profiles, So decreased with H from 1.47±0.10 for H<129 m to 0.53±0.09 for 129<H<175 m and to 0.34±0.07 for 175<H<256 m (Fig. 7a). This was due to the increase in average Rp for high Nc measurements as a function of H from 0.01 mm h−1 to 0.05 and 0.04 mm h−1, respectively. Rp increased with H due to stronger collision–coalescence as droplet mass increased with H. Separated profiles with H>256 m had lower Nc and higher Rp compared to the populations with lower H (Fig. 6a, b). For measurements with low Nc, collision–coalescence and stronger autoconversion (following Eq. 6) resulted in higher Rp (0.26 mm h−1) compared to measurements with higher Nc (0.13 mm h−1). This led to a strong gradient Rp as a function of Nc (Fig. 8d), and So increased to 1.45±0.07 for separated profiles with H>256 m.
For contact profiles with H>129 m, the average Rp increased with H, with a larger increase for measurements with low Nc (0.028 to 0.12 mm h−1) compared to measurements with high Nc (0.03 to 0.06 mm h−1). It is hypothesized that collision–coalescence was hindered by the presence of more numerous droplets for the latter. With droplet growth and collision–coalescence for higher H, the limiting factor for Rp changed from H to Nc. The dependence of Rp on Nc thus increased with H, and, as a result, So increased with H from 0.88±0.06 to 1.15±0.06 (Fig. 7a).
Among the four regimes defined based on the above- and below-cloud Na, S-L profiles had the highest So (1.12) (Table 7). This was associated with S-L profiles having the lowest Nc and the highest Rp among the regimes (Fig. 6c, d). In descending order of So, S-L profiles were followed by C-L (0.86), S-H (0.50), and C-H profiles (0.33). Profiles in low Na boundary layers (S-L and C-L) had higher So compared to profiles in high Na boundary layers (S-H and C-H), consistent with wet scavenging of below-cloud aerosols (Duong et al., 2011; Jung et al., 2016).
Table 7So ± standard error with sample size and R in parentheses for cloud regimes defined in text. The use of italics denotes the statistical insignificance of So.

C-L and C-H profiles had similar trends in So except for profiles with H<129 m (Fig. 7b). C-L profiles had an insignificant value for So due to low sample size (4), and C-H profiles had negative So. These were thin profiles with little cloud water (Fig. 4b), high Nc (Fig. 6c), and low Rp (Fig. 6d). It is hypothesized that increasing Nc would provide the cloud water required for precipitation initiation and aid collision–coalescence. A total of 107 out of 148 separated profiles were classified as S-L profiles. As a result, separated and S-L profiles had similar trends in So versus H (Fig. 7). On average, S-L profiles had higher So than S-H profiles, which could be associated with wet scavenging, resulting in the lower below-cloud Na for S-L profiles. For S-H profiles, So was constant with H at about 0.45 (except 175<H<256 m when the value for So was insignificant).
The sensitivity of So to removal of clouds based on Rp was examined in Appendix B. The removal of clouds with low Rp and high Nc or with high Rp and low Nc resulted in lower average So consistent with previous work (Duong et al., 2011). The So comparisons between profiles located in high Na or low Na boundary layers varied with the sample sizes of the populations. The sample sizes varied based on the threshold used to define a low Na boundary layer which is discussed in Appendix C. CAS data were used to represent measurements of droplets with D<50 µm collected during ORACLES 2016 in the absence of CDP data. The sensitivity of So to the use of CAS data was examined in Appendix D.
6.4 So discussion
Figure 9 shows how So varied with perturbations (Δ) in Nc or Rp. Previous studies hypothesized that increasing above-cloud Na or precipitation scavenging of below-cloud Na would lead to changes in So (Fig. 4, Duong et al., 2011; Fig. 11, Jung et al., 2016). Thus, ΔNc and ΔRp for clouds with variable above- and below-cloud Na were quantified in this study (Table 5). Higher Nc and lower Re for contact profiles led to precipitation suppression along with lower SAUTO, SACC, and Rp, which were associated with lower So compared to separated profiles. As a result, polluted clouds were 20 % less susceptible to precipitation suppression than cleaner clouds. Figure 9 shows that the impact of ΔNc or ΔRp on So depends on the original values for Nc and Rp as the same ΔNc and ΔRp can have an opposing effect on So. For example, a decrease in Nc at point 1 would decrease the slope and the So value, while the same decrease in Nc at point 2 would increase the slope and the So value.
Both average and maximum Nc and Rp varied with H due to increasing aerosols (Sect. 4) and droplet growth due to collision–coalescence, autoconversion, and accretion (Sect. 5). Further, co-variability between droplet growth processes and ACIs meant aerosol-induced ΔNc and ΔRp varied with H (Sect. 6.2). Consequently, the differences between So for clean and polluted clouds varied with H. The change in So was highest for thin polluted clouds due to poor correlation between Nc and Rp as limited droplet growth led to low Rp regardless of the Nc. Future work must examine the co-variability between ΔNc or ΔRp from cloud processes such as droplet growth, entrainment, invigoration, precipitation, and ΔNc or ΔRp due to ACIs. Model parameterizations with power-law relationships between Rp, Nc, and H (Geoffroy et al., 2008) must account for changes in the dependence of Rp on due to increasing aerosols or H.
The trends in So were only compared with studies analyzing airborne data due to the variability in So depending on whether aircraft, remote sensing, or modeling data were examined (Sorooshian et al., 2019). Consistent with Terai et al. (2012), So decreased with H for separated profiles with H<256 m. The results from Sect. 5 suggest droplet growth with H decreased the susceptibility to aerosols because Rp was limited by droplet growth instead of Na or Nc. In comparison, So increased with H for contact profiles, consistent with Jung et al. (2016). The low So for thin contact profiles was consistent with the low So (0.06) for thin MSC over the southeast Pacific (Jung et al., 2016). This was attributed to insufficient cloud water for precipitation initiation (as noted in Sect. 5). An airborne investigation of marine stratocumulus off the Californian coast attributed negative values of So to the influence of giant cloud condensation nuclei (Dadashazar et al., 2017). The authors hypothesized that the low statistical significance of the negative estimate of So could be associated with precipitation suppression by aerosol particles.
Jung et al. (2016) analyzed MSC sampled farther east and away from South America compared to Terai et al. (2012). They argued a westward increase in precipitation frequency and intensity, along with a decrease in aerosols and Nc, led to the differences between the two studies. This same attribution of the role of aerosols can be made for the ORACLES data as there were differences between contact and separated profiles because the MSCs sampled during these profiles were located in similar geographical locations with different aerosol conditions. Modeling studies (e.g., Wood et al., 2009; Gettelman et al., 2013) have shown that So increases with H when SAUTO dominates SACC (typically for Re<14 µm, the critical radius for precipitation initiation). Maximum Re<14 µm was sampled during all but 23 separated and 3 contact profiles (Fig. 4d). This would explain the increase in So with H for both contact (for H>129 m) and separated profiles (for H>256 m).
The relationships between LWP or H and Nc, Re, and LWC depend on meteorological conditions in addition to aerosol properties. The MSC LWP and cloud cover can vary with LTS (Klein and Hartmann, 1993; Mauger and Norris, 2007), estimated inversion strength (EIS) (Wood and Bretherton, 2006), and SST (Wilcox, 2010; Sakaeda et al., 2011). The correlations between LWP H and these parameters are examined using the European Centre for Medium-Range Weather Forecasts (ECMWF) atmospheric reanalysis (ERA5) (Hersbach et al., 2020) to define the meteorological conditions.
ERA5 provides hourly output with a horizontal resolution of for 37 pressure (p) levels (up to 1 hPa). The cloud sampling for most flights was conducted within 3 h of 12:00 UTC (Table 2). ERA5 data at 12:00 UTC were thus used for the grid box nearest to the profile (Dzambo et al., 2019). The low cloud cover (LCC), SST, HBL, total column liquid water (ERA5 LWP) and rainwater (ERA5 RWP), mean sea level pressure (po), 2 m temperature (To), and 2 m dew point temperature (Td) were examined (Table 8).
Table 8Meteorological and cloud properties from ERA5 reanalysis for contact, separated, and all profiles with LCC >0.95 (LCC is reported for all profiles), 95 % CIs from a two-sample t test applied to contact and separated profile data, and R between each parameter and LWP (RLWP) or H (RH) with statistically significant RH and RLWP in bold.
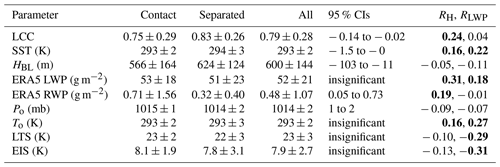
The difference between potential temperatures at 700 hPa and the surface was defined as LTS (Klein and Hartmann, 1993). EIS was calculated following Wood and Bretherton (2006):
where Γm is the moist adiabatic potential temperature gradient, z700 is the height at 700 mb, and LCL is the lifting condensation level (Lawrence, 2005). is Γm for 850 hPa and calculated following Wood and Bretherton (2006).
LCC refers to cloud fraction for p>0.8po, corresponding to p>810 hPa, where most profiles were sampled (Table 2). The ECMWF model used a threshold of EIS >7 K to distinguish between well-mixed boundary layers topped by stratocumulus and decoupled boundary layers with cumulus clouds (ECMWF, 2020). This distinction improved the agreement between the model LCC and LWP and observations (Köhler et al., 2011). LCC was proportional to EIS LTS, and LCC <0.8 was mostly observed for EIS <7 K (Fig. 10a). Decoupled boundary layers can be topped by MSC (G21; Wood, 2012). Profiles with EIS <7 K were included in the analysis if ERA5 had LCC >0.95. This included 64 contact and 88 separated profiles from the three IOPs. For the 2016, 2017, and 2018 IOPs, 50, 20, and 76 profiles, respectively, had LCC >0.95 out of which 0, 4, and 44 profiles, respectively, had EIS <7 K. The average ERA5 HBL (599±144 m) was lower than the average ZT (932±196 m). This underestimation of HBL by ERA5 has been observed for stratocumulus over the southeast and northeast Pacific (Ahlgrimm et al., 2009; Hannay et al., 2009).
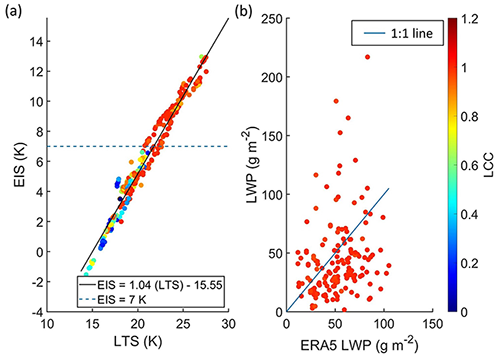
Figure 10(a) LTS versus EIS with regression coefficients in legend (R= 0.98) and (b) LWP from size-resolved probes versus LWP from the ERA5 reanalysis (R=0.18) where each dot represents a single cloud profile. LTS, EIS, ERA5 LWP, and LCC for each cloud profile taken from the nearest ERA5 grid box (within 0.25∘ of latitude and longitude) at 12:00 UTC. Panel (a) shows all cloud profiles and panel (b) shows cloud profiles with LCC >0.95.
On average, the ERA5 LWP (51±21 g m−2) was slightly greater than LWP (46±41 g m−2), but the differences were statistically insignificant. There was a significant but weak correlation between LWP and ERA5 LWP (R=0.18) (Fig. 10b). On average, the ERA5 RWP (0.48±1.07 g m−2) was lower than RWP (1.19±2.76 g m−2). There were insignificant differences between ERA5 LWP and LWP for contact and separated profiles with LCC >0.95 (Table 8). Contact profiles with LCC >0.95 had significantly higher ERA5 RWP (Table 8). While this is counterintuitive, given the precipitation suppression, it was due to the selection of profiles with LCC >0.95. Contact profiles with LCC >0.95 also had higher in situ RWP (95 % CIs: 0.32 to 2.08 g m−2 higher) compared to separated profiles with LCC >0.95.
LWP was positively correlated with SST and To and negatively correlated with LTS and EIS with weak but statistically significant correlations (Fig. 11). On average, separated profiles had significantly higher SST (95 % CIs: 0.01 to 1.48 K higher) compared to contact profiles with insignificant differences between the average To, EIS, and LTS. Since the correlation between LWP H and SST was weak, it is unlikely the differences between contact and separated profiles were driven by SST differences alone. When all profiles (irrespective of LCC) were considered, there were insignificant differences between the average ERA5 RWP, SST, To, EIS, and LTS for contact and separated profiles. This suggests the differences between contact and separated profiles found during the ORACLES IOPs were primarily associated with ACIs instead of meteorological effects.
In situ measurements of stratocumulus over the Southeast Atlantic Ocean were collected during the NASA ORACLES field campaign. The microphysical (Nc and Re), macrophysical (LWP and H), and precipitation properties (Rp and So) of the stratocumulus were analyzed. A total of 173 contact profiles with Na>500 cm−3 within 100 m above cloud tops were compared with 156 separated profiles with Na<500 cm−3 up to at least 100 m above cloud tops. Contact between above-cloud aerosols and the stratocumulus was associated with the following:
-
More numerous and smaller droplets with weaker droplet growth with height.
Contact profiles had significantly higher Nc (84 to 90 cm−3 higher) and lower Re (1.4 to 1.6 µm lower) compared to separated profiles. The median Re had a smaller increase from cloud base to cloud top for contact (6.1 to 7.9 µm) compared to separated profiles (7.1 to 9.5 µm). The profiles had similar LWP and H, and it is hypothesized the differences in droplet growth were associated with collision–coalescence.
-
Aerosol-induced cloud microphysical changes in both clean and polluted boundary layers.
Contact profiles had 25 to 31 cm−3 higher Nc and 0.2 to 0.5 µm lower Re in clean and 98 to 108 cm−3 higher Nc and 1.6 to 1.8 µm lower Re in polluted boundary layers compared to separated profiles. Contact profiles were more often located in polluted boundary layers and had higher below-cloud CO concentration (27 to 29 ppb higher), which suggests more frequent entrainment of biomass burning aerosols into the boundary layer compared to separated profiles.
-
Precipitation suppression with significantly lower precipitation intensity and precipitation formation process rates.
Separated profiles had Rp up to 0.22 mm h−1, while contact profiles had Rp up to 0.07 mm h−1. SAUTO and SACC had higher maxima for separated (up to and s−1) compared to contact profiles (up to and s−1).
-
Lower precipitation susceptibility, with the strongest impact in thin clouds (H<129 m).
Contact profiles had lower So (0.87±0.04) compared to separated profiles (1.08±0.04). Thin clouds had the highest difference in So ( for contact and 1.47±0.10 for separated). Lower So for thin contact profiles was associated with poor correlation between Nc and Rp (). For separated profiles, So decreased with H before increasing for H>256 m. In comparison, So increased with H for contact profiles for H>129 m.
-
Statistically insignificant differences in meteorological parameters that influence .
Based on ERA5 reanalysis data, LWP was correlated with SST (R=0.22), To (R=0.27), LTS (), and EIS (). Contact profiles with ERA5 LCC >0.95 had lower SST (0.01 to 1.48 K lower), with similar To, LTS, and EIS compared to separated profiles. The SST differences were insignificant when profiles with LCC <0.95 were included in the comparison.
Three important factors affecting So were discussed (Sorooshian et al., 2019): above-cloud Na, below-cloud Na, and meteorological conditions. This study analyzed ORACLES data from all three IOPs, and the first two conclusions were consistent with the analysis of ORACLES 2016 (Gupta et al., 2021). Future work will compare in situ data with Rp retrievals from APR-3 (Dzambo et al., 2021) to evaluate the sensitivity of So to the use of satellite retrievals of Rp (Bai et al., 2018). Vertical profiles of MSC properties will be used to evaluate satellite retrievals (Painemal and Zuidema, 2011; Zhang and Platnick, 2011) to address the uncertainties associated with satellite-based estimates of ACIs (Quaas et al., 2020). The ORACLES dataset can be combined with future investigations of marine stratocumulus to address the “lack of long-term datasets needed to provide statistical significance for a sufficiently large range of aerosol variability influencing specific cloud regimes over a range of macrophysical conditions” (Sorooshian et al., 2010).
The base analysis examined how cloud properties varied with H by separating cloud profiles into four populations of H using the following endpoints: 28, 129, 175, 256, and 700 m. Two sensitivity studies determine if trends describing the variation of Nc, Rp, and So with H were sensitive to the endpoints used to sort cloud profiles into different populations.
First, cloud profiles were classified into two populations using the median H (175 m) to divide the populations (Table A1). The average Nc decreased, and the average Rp increased with H for both contact (211 to 186 cm−3 and 0.03 to 0.07 mm h−1) and separated profiles (129 to 104 cm−3 and 0.07 to 0.15 mm h−1). So increased with H for contact profiles from 0.53 to 1.06 and slightly decreased with H for separated profiles from 1.05 to 1.02 (Table A1). The difference between So for contact and separated profiles was greater for thin profiles (H<175 m) compared to thick profiles (H>175 m). These results are consistent with trends using four populations but provide less detail about how So varies with H (Fig. A1).
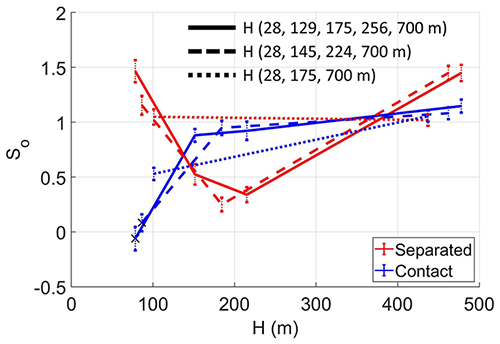
Figure A1So as a function of H for contact and separated profiles classified into different populations using the endpoints indicated in legend. So was statistically insignificant when marked with a cross.
Table A1So ± standard error with sample size and R in parentheses for contact, separated, and all profiles classified into a different number of populations.
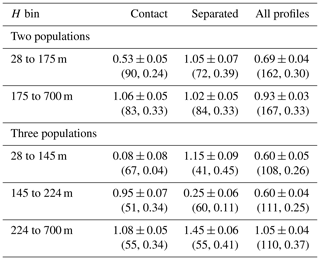
Second, cloud profiles were classified into three populations using the terciles of H (145 and 224 m) (Table A1). The average Nc decreased, and the average Rp increased from the lowest to the highest H for contact (231 to 187 cm−3 and 0.03 to 0.07 mm h−1) and separated profiles (138 to 95 cm−3 and 0.06 to 0.18 mm h−1). For separated profiles, So first decreased with H from 1.15 to 0.25 before increasing to 1.45 for the highest H (Fig. A1). Contact profiles had insignificant So for the lowest H, followed by So increasing from 0.95 to 1.08 with H. The results presented here are robust with regards to the number of populations used.
Another sensitivity study examined the Rp threshold used for cloud profiles included while calculating So. The average So decreased if weakly precipitating clouds with low Rp were excluded (Fig. B1, Table B1). It is possible that this was due to the higher Na and Nc associated with weakly precipitating clouds. The exclusion of weakly precipitating clouds provides biased trends in So since these clouds could have undergone precipitation suppression already. Conversely, strongly precipitating clouds were associated with cleaner conditions and lower Na and Nc. The exclusion of strongly precipitating clouds also leads to a decrease in the average So (Fig. B2, Table B1).
The occurrence of wet scavenging below strongly precipitating clouds (Duong et al., 2011) results in lower below-cloud Na (and subsequently Nc). Higher susceptibility to precipitation suppression for cleaner, strongly precipitating clouds would explain the increase in the average So. This is consistent with observations of So using different Rp thresholds (see Fig B1, Jung et al., 2016) and hypotheses regarding the impact of different Na on So (Duong et al., 2011; Fig. 11, Jung et al., 2016).
Table B1So ± standard error with sample size and R in parentheses for contact, separated, and all profiles with Rp above a certain threshold.
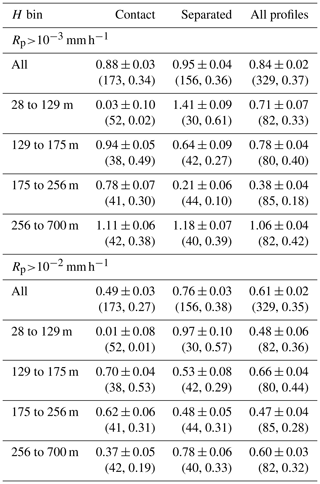
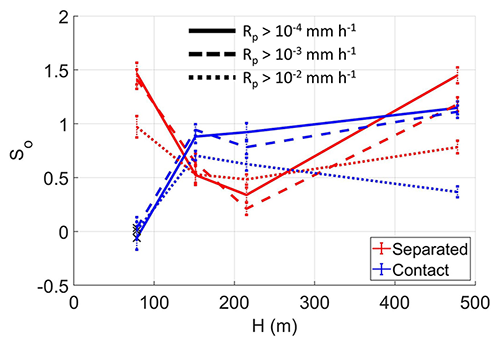
Figure B1So as a function of H for contact and separated profiles, with Rp greater than the thresholds indicated in legend. So was statistically insignificant when marked with a cross.
The number of cloud profiles classified into the S-L, C-L, S-H, and C-H regimes varied depending on the below-cloud Na threshold used to define a low Na or clean boundary layer. For the threshold used in the base analysis (350 cm−3), contact profiles were more often located in polluted boundary layers (131 out of 171 profiles classified as C-H) while separated profiles were more often located in clean boundary layers (108 out of 148 profiles classified as S-L). The comparisons between So in clean and polluted boundary layers varied with the threshold used.
As a sensitivity study, a lower threshold was used to define a clean boundary layer (300 cm−3). For this case, the C-L regime had no profiles in the population with the lowest H (H<129 m) when four populations of profiles were used to examine the dependence of So on H. Two out of the other three populations had an insignificant value for So due to poor and statistically insignificant correlations between Nc and Rp (Table C1). This was associated with a low sample size for the populations (six each). A second sensitivity study used a higher threshold to define a clean boundary layer (400 cm−3). For this case, the S-H regime has insignificant So for three out of the four populations of H and the remaining population had a small sample size (three profiles) (Table C1). The base analysis using a threshold of 350 cm−3 to define a clean boundary layer was used to compare So values that represent a larger number of cloud profiles.
Given the differences between the CAS and PDI Nc and LWC for droplets with D<50 µm during ORACLES 2016 (see Supplement), sensitivity tests were performed by first excluding ORACLES 2016 data and second by using PDI data to represent ORACLES 2016 size distributions for D<50 µm in the So calculations. These sensitivity tests resulted in minor changes in the trends of So versus H (Fig. D1), along with average changes in the magnitude of So up to 0.05 (Table D1). The noted changes suggest that the discussion of trends in So described in this study is robust as it relates to the inclusion of ORACLES 2016 data and the use of CAS data for the deployment. Since the 2016 deployment contributed about a third of the ORACLES measurements, data from the 2016 deployment were included in the study so as not to reduce the size of the dataset.
The slight decrease in So for thick clouds (H>256 m) upon removal of ORACLES 2016 data is associated with a decrease in the number of thick clouds (Table D1). The use of PDI data resulted in minor changes because So primarily depends on Nc and Rp. The CAS and PDI datasets had small differences in the average Nc (95 % confidence intervals of 9 to 12 cm−3), and Rp was calculated using droplets with D>50 µm, which do not include contributions from either the CAS or the PDI. The documentation of differences between the ORACLES cloud probes (see Supplement) highlights the measurement uncertainties associated with the cloud probe datasets.
University of Illinois/Oklahoma Optical Array Probe (OAP) Processing Software is available at https://doi.org/10.5281/zenodo.1285969 (McFarquhar et al., 2018). The Airborne Data Processing and Analysis software package is available at https://doi.org/10.5281/zenodo.3733448 (Delene et al., 2020).
All ORACLES data are accessible via the digital object identifiers provided under ORACLES Science Team references: https://doi.org/10.5067/Suborbital/ORACLES/P3/2018_V2 (ORACLES Science Team, 2020a), https://doi.org/10.5067/Suborbital/ORACLES/P3/2017_V2 (ORACLES Science Team, 2020b), and https://doi.org/10.5067/Suborbital/ORACLES/P3/2016_V2 (ORACLES Science Team, 2020c). ERA5 data were obtained from the Climate Data Store (last access: 18 May 2021): https://cds.climate.copernicus.eu/cdsapp#!/home (CDS, 2017; Hersbach et al., 2020).
The supplement related to this article is available online at: https://doi.org/10.5194/acp-22-2769-2022-supplement.
GMM and MRP worked with other investigators to design the ORACLES project and flight campaigns. SG designed the study with guidance from GMM. SG analyzed the data with inputs from GMM, JRO'B, and MRP. JRO'B and DJD processed PCASP data and cloud probe data, conducted data quality tests, and some of the data comparisons between cloud probes. SG processed 2D-S and HVPS-3 data and conducted some of the data comparisons between cloud probes. JDSG processed PDI data. GMM and MRP acquired funding. All authors were involved in data collection during ORACLES. SG wrote the manuscript with guidance from GMM and reviews from all authors.
The contact author has declared that neither they nor their co-authors have any competing interests.
Publisher’s note: Copernicus Publications remains neutral with regard to jurisdictional claims in published maps and institutional affiliations.
This article is part of the special issue “New observations and related modeling studies of the aerosol–cloud–climate system in the Southeast Atlantic and southern Africa regions (ACP/AMT inter-journal SI)”. This article is not associated with a conference.
The authors thank Yohei Shinozuka for providing merged instrument data files for the ORACLES field campaign. We acknowledge the entire ORACLES science team for their assistance with data acquisition, analysis, and interpretation. We thank the NASA Ames Earth Science Project Office and the NASA P-3B flight/maintenance crew for logistical and aircraft support. Some of the computing for this project was performed at the OU Supercomputing Center for Education & Research (OSCER) at the University of Oklahoma (OU).
ORACLES is funded by NASA Earth Venture Suborbital-2 (grant no. NNH13ZDA001N-EVS2). Siddhant Gupta was supported by NASA headquarters under the NASA Earth and Space Science Fellowship (grant nos. NNX15AF93G and NNX16A018H). Greg M. McFarquhar and Siddhant Gupta were supported by NASA (grant no. 80NSSC18K0222).
This paper was edited by J. M. Haywood and reviewed by three anonymous referees.
Abel, S. J. and Boutle, I. A.: An improved representation of the raindrop size distribution for single-moment microphysics schemes, Q. J. Roy. Meteor. Soc., 138, 2151–2162, https://doi.org/10.1002/qj.1949, 2012.
Ackerman, A. S., Kirkpatrick, M. P., Stevens, D. E., and Toon, O. B.: The impact of humidity above stratiform clouds on indirect aerosol climate forcing, Nature, 432, 1014–1017, 2004.
Adebiyi, A. A. and Zuidema, P.: The role of the southern African easterly jet in modifying the southeast Atlantic aerosol and cloud environments, Q. J. Roy. Meteor. Soc., 142, 1574–1589, https://doi.org/10.1002/qj.2765, 2016.
Ahlgrimm, M. and Forbes, R.: Improving the Representation of Low Clouds and Drizzle in the ECMWF Model Based on ARM Observations from the Azores, Mon. Weather Rev., 142, 668–685, https://doi.org/10.1175/mwr-d-13-00153.1, 2014.
Ahlgrimm, M., Randall, D. A., and Kohler, M.: Evaluating cloud frequency of occurrence and cloud-top height using spaceborne lidar observations, Mon. Weather Rev., 137, 4225–4237, 2009.
Albrecht, B.: Aerosols, Cloud Microphysics, and Fractional Cloudiness, Science, 245, 1227–1230, 1989.
Bai, H., Gong, C., Wang, M., Zhang, Z., and L'Ecuyer, T.: Estimating precipitation susceptibility in warm marine clouds using multi-sensor aerosol and cloud products from A-Train satellites, Atmos. Chem. Phys., 18, 1763–1783, https://doi.org/10.5194/acp-18-1763-2018, 2018.
Baumgardner, D., Jonsson, H., Dawson, W., Connor, D. O., and Newton, R.: The cloud, aerosol and precipitation spectrometer (CAPS): A new instrument for cloud investigations, Atmos. Res., 59, 59–60, 2001.
Baumgardner, D., Abel, S. J., Axisa, D., Cotton, R., Crosier, J., Field, P., Gurganus, C., Heymsfield, A., Korolev, A., Kraemer, M., Lawson, P., McFarquhar, G., Ulanowski, Z., and Um, J.: Cloud ice properties: in situ measurement challenges, Meteor. Mon., 58, 9.1–9.23, https://doi.org/10.1175/AMSMONOGRAPHS-D-16-0011.1, 2017.
Behrangi, A., Stephens, G., Adler, R. F., Huffman, G. J., Lambrigtsen, B., and Lebsock, M.: An update on the oceanic precipitation rate and its zonal distribution in light of advanced observations from space, J. Climate, 27, 3957–3965, https://doi.org/10.1175/JCLI-D-13-00679.1, 2014.
Bennartz, R.: Global assessment of marine boundary layer cloud droplet number concentration from satellite, J. Geophys. Res., 112, D02201, https://doi.org/10.1029/2006JD007547, 2007.
Boers, R., Acarreta, J. R., and Gras, J. L.: Satellite monitoring of the first indirect aerosol effect: Retrieval of the droplet concentration of water clouds, J. Geophys. Res.-Atmos., 111, D22208, https://doi.org/10.1029/2005JD006838, 2006.
Bony, S. and Dufrense, J.-L.: Marine boundary layer clouds at the heart of tropical feedback uncertainties in climate models, Geophys. Res. Lett., 32, L20806, https://doi.org/10.1029/2005GL023851, 2005.
Boucher, O., Randall, D., Artaxo, P., Bretherton, C., Feingold, G., Forster, P., Kerminen, V.-M., Kondo, Y., Liao, H., Lohmann, U., Rasch, P., Satheesh, S. K., Sherwood, S., Stevens, B., and Zhang, X. Y.: Clouds and Aerosols, in: Climate Change 2013: The Physical Science Basis, Contribution of Working Group I to the Fifth Assessment Report of the Intergovernmental Panel on Climate Change, edited by: Stocker, T. F., Qin, D., Plattner, G.-K., Tignor, M., Allen, S. K., Boschung, J., Nauels, A., Xia, Y., Bex, V., and Midgley, P. M., Cambridge University Press, Cambridge, United Kingdom and New York, NY, USA, 571–657, 2013.
Boutle, I. A., Abel, S. J., Hill, P. G., and Morcrette, C. J.: Spatial variability of liquid cloud and rain: observations and microphysical effects, Q. J. Roy. Meteor. Soc., 140, 583–594, https://doi.org/10.1002/qj.2140, 2014.
Braun, R. A., Dadashazar, H., MacDonald, A. B., Crosbie, E., Jonsson, H. H., Woods, R. K., Flagan, R. C., Seinfeld, J. H., and Sorooshian, A.: Cloud Adiabaticity and Its Relationship to Marine Stratocumulus Characteristics Over the Northeast Pacific Ocean, J. Geophys. Res.-Atmos., 123, 13790–13806, https://doi.org/10.1029/2018JD029287, 2018.
Brenguier, J.-L., Pawlowska, H., Schuller, L., Preusker, R., Fischer, J., and Fouquart, Y.: Radiative properties of boundary layer clouds: Droplet effective radius versus number concentration, J. Atmos. Sci., 57, 803–821, 2000.
Cai, Y., Snider, J. R., and Wechsler, P.: Calibration of the passive cavity aerosol spectrometer probe for airborne determination of the size distribution, Atmos. Meas. Tech., 6, 2349–2358, https://doi.org/10.5194/amt-6-2349-2013, 2013.
CDS: ERA5 Fifth generation of ECMWF atmospheric reanalyses of the global climate, CDS [data set], https://cds.climate.copernicus.eu/cdsapp#!/home (last access: 26 November 2019), 2017.
Chen, Y.-C., Christensen, M. W., Stephens, G. L., and Seinfeld, J. H.: Satellite-based estimate of global aerosol–cloud radiative forcing by marine warm clouds, Nat. Geosci., 7, 643–646, https://doi.org/10.1038/ngeo2214, 2014.
Christensen, M. W. and Stephens, G. L.: Microphysical and macrophysical responses of marine stratocumulus polluted by underlying ships. Part 2: Impacts of haze on precipitating clouds, J. Geophys. Res., 117, D11203, https://doi.org/10.1029/2011JD017125, 2012.
Chuang, P. Y., Saw, E. W., Small, J. D., Shaw, R. A., Sipperley, C. M., Payne, G. A., and Bachalo, W.: Airborne Phase 495 Doppler Interferometry for Cloud Microphysical Measurements, Aerosol Sci. Technol., 42, 685–703, 2008.
Cochrane, S. P., Schmidt, K. S., Chen, H., Pilewskie, P., Kittelman, S., Redemann, J., LeBlanc, S., Pistone, K., Kacenelenbogen, M., Segal Rozenhaimer, M., Shinozuka, Y., Flynn, C., Platnick, S., Meyer, K., Ferrare, R., Burton, S., Hostetler, C., Howell, S., Freitag, S., Dobracki, A., and Doherty, S.: Above-cloud aerosol radiative effects based on ORACLES 2016 and ORACLES 2017 aircraft experiments, Atmos. Meas. Tech., 12, 6505–6528, https://doi.org/10.5194/amt-12-6505-2019, 2019.
Dadashazar, H., Wang, Z., Crosbie, E., Brunke, M., Zeng, X., Jonsson, H., Woods, R. K., Flagan, R. C., Seinfeld, J. H., and Sorooshian, A.: Relationships between giant sea salt particles and clouds inferred from aircraft physicochemical data, J. Geophys. Res.-Atmos., 122, 3421–3434, https://doi.org/10.1002/2016JD026019, 2017.
Delene, D. J.: Airborne Data Processing and Analysis Software Package, Earth Sci. Inform., 4, 29–44, 2011.
Delene, D. J., Skow, A., O'Brien, J., Gapp, N., Wagner, S., Hibert, K., Sand, K., and Sova, G.: Airborne Data Processing and Analysis Software Package (Version 3981), Zenodo [code], https://doi.org/10.5281/zenodo.3733448, 2020.
Devasthale, A. and Thomas, M. A.: A global survey of aerosol-liquid water cloud overlap based on four years of CALIPSO-CALIOP data, Atmos. Chem. Phys., 11, 1143–1154, https://doi.org/10.5194/acp-11-1143-2011, 2011.
Diamond, M. S., Dobracki, A., Freitag, S., Small Griswold, J. D., Heikkila, A., Howell, S. G., Kacarab, M. E., Podolske, J. R., Saide, P. E., and Wood, R.: Time-dependent entrainment of smoke presents an observational challenge for assessing aerosol–cloud interactions over the southeast Atlantic Ocean, Atmos. Chem. Phys., 18, 14623–14636, https://doi.org/10.5194/acp-18-14623-2018, 2018.
Doherty, S. J., Saide, P. E., Zuidema, P., Shinozuka, Y., Ferrada, G. A., Gordon, H., Mallet, M., Meyer, K., Painemal, D., Howell, S. G., Freitag, S., Dobracki, A., Podolske, J. R., Burton, S. P., Ferrare, R. A., Howes, C., Nabat, P., Carmichael, G. R., da Silva, A., Pistone, K., Chang, I., Gao, L., Wood, R., and Redemann, J.: Modeled and observed properties related to the direct aerosol radiative effect of biomass burning aerosol over the southeastern Atlantic, Atmos. Chem. Phys., 22, 1–46, https://doi.org/10.5194/acp-22-1-2022, 2022.
Douglas, A. and L'Ecuyer, T.: Quantifying variations in shortwave aerosol–cloud–radiation interactions using local meteorology and cloud state constraints, Atmos. Chem. Phys., 19, 6251–6268, https://doi.org/10.5194/acp-19-6251-2019, 2019.
Douglas, A. and L'Ecuyer, T.: Quantifying cloud adjustments and the radiative forcing due to aerosol–cloud interactions in satellite observations of warm marine clouds, Atmos. Chem. Phys., 20, 6225–6241, https://doi.org/10.5194/acp-20-6225-2020, 2020.
Duong, H. T., Sorooshian, A., and Feingold, G.: Investigating potential biases in observed and modeled metrics of aerosol-cloud-precipitation interactions, Atmos. Chem. Phys., 11, 4027–4037, https://doi.org/10.5194/acp-11-4027-2011, 2011.
Dzambo, A. M., L'Ecuyer, T. S., Sy, O. O., and Tanelli, S.: The Observed Structure and Precipitation Characteristics of Southeast Atlantic Stratocumulus from Airborne Radar during ORACLES 2016–17, J. Appl. Meteor. Climatol., 58, 2197–2215, https://doi.org/10.1175/JAMC-D-19-0032.1, 2019.
Dzambo, A. M., L'Ecuyer, T., Sinclair, K., van Diedenhoven, B., Gupta, S., McFarquhar, G., O'Brien, J. R., Cairns, B., Wasilewski, A. P., and Alexandrov, M.: Joint cloud water path and rainwater path retrievals from airborne ORACLES observations, Atmos. Chem. Phys., 21, 5513–5532, https://doi.org/10.5194/acp-21-5513-2021, 2021.
Eastman, R., Warren, S. G., and Hahn, C. J.: Variations in Cloud Cover and Cloud Types over the Ocean from Surface Observations, 1954–2008, J. Climate, 24, 5914–5934, https://doi.org/10.1175/2011JCLI3972.1, 2011.
ECMWF: IFS Documentation, IFS Documentation CY47R1: IFS Documentation CY47R1 – Part IV: Physical Processes, https://doi.org/10.21957/cpmkqvhja, 2020.
Feingold, G. and Siebert, H.: Cloud – Aerosol Interactions from the Micro to the Cloud Scale, from the Strungmann Forum Report, Clouds in the Perturbed Climate System: Their Relationship to Energy Balance, Atmospheric Dynamics, and Precipitation, 2, edited by: Heintzenberg, J. and Charlson, R. J., MIT Press, ISBN 978-0-262-01287-4, 2009.
Gettelman, A., Morrison, H., Terai, C. R., and Wood, R.: Microphysical process rates and global aerosol–cloud interactions, Atmos. Chem. Phys., 13, 9855–9867, https://doi.org/10.5194/acp-13-9855-2013, 2013.
Geoffroy, O., Brenguier, J.-L., and Sandu, I.: Relationship between drizzle rate, liquid water path and droplet concentration at the scale of a stratocumulus cloud system, Atmos. Chem. Phys., 8, 4641–4654, https://doi.org/10.5194/acp-8-4641-2008, 2008.
Geoffroy, O., Brenguier, J.-L., and Burnet, F.: Parametric representation of the cloud droplet spectra for LES warm bulk microphysical schemes, Atmos. Chem. Phys., 10, 4835–4848, https://doi.org/10.5194/acp-10-4835-2010, 2010.
Gordon, H., Field, P. R., Abel, S. J., Dalvi, M., Grosvenor, D. P., Hill, A. A., Johnson, B. T., Miltenberger, A. K., Yoshioka, M., and Carslaw, K. S.: Large simulated radiative effects of smoke in the south-east Atlantic, Atmos. Chem. Phys., 18, 15261–15289, https://doi.org/10.5194/acp-18-15261-2018, 2018.
Gryspeerdt, E., Goren, T., Sourdeval, O., Quaas, J., Mülmenstädt, J., Dipu, S., Unglaub, C., Gettelman, A., and Christensen, M.: Constraining the aerosol influence on cloud liquid water path, Atmos. Chem. Phys., 19, 5331–5347, https://doi.org/10.5194/acp-19-5331-2019, 2019.
Gui, K., Che, H., Zheng, Y., Zhao, H., Yao, W., Li, L., Zhang, L., Wang, H., Wang, Y., and Zhang, X.: Three-dimensional climatology, trends, and meteorological drivers of global and regional tropospheric type-dependent aerosols: insights from 13 years (2007–2019) of CALIOP observations, Atmos. Chem. Phys., 21, 15309–15336, https://doi.org/10.5194/acp-21-15309-2021, 2021.
Gupta, S., McFarquhar, G. M., O'Brien, J. R., Delene, D. J., Poellot, M. R., Dobracki, A., Podolske, J. R., Redemann, J., LeBlanc, S. E., Segal-Rozenhaimer, M., and Pistone, K.: Impact of the variability in vertical separation between biomass burning aerosols and marine stratocumulus on cloud microphysical properties over the Southeast Atlantic, Atmos. Chem. Phys., 21, 4615–4635, https://doi.org/10.5194/acp-21-4615-2021, 2021.
Hannay, C., Williamson, D., Hack, J., Kiehl, J., Olson, J., Klein, S., Bretherton, C., and Kohler, M.: Evaluation of forecasted south-east pacific stratocumulus in the NCAR, GFDL, and ECMWF Models, J. Climate, 22, 2871–2889, 2009.
Hansen, J. and Travis, L. D.: Light scattering in planetary atmospheres, Space Sci. Rev., 16, 527–610, 1974
Hersbach, H., Bell, B., Berrisford, P., Hirahara, S., Horányi, A., Muñoz-Sabater, J., Nicolas, J., Peubey, C., Radu, R., Schepers, D., Simmons, A., Soci, C., Abdalla, S., Abellan, X., Balsamo, G., Bechtold, P., Biavati, G., Bidlot, J., Bonavita, M., De Chiara, G., Dahlgren, P., Dee, D., Diamantakis, M., Dragani, R., Flemming, J., Forbes, R., Fuentes, M., Geer, A., Haimberger, L., Healy, S., Hogan, R. J., Hólm, E., Janisková, M., Keeley, S., Laloyaux, P., Lopez, P., Lupu, C., Radnoti, G., de Rosnay, P., Rozum, I., Vamborg, F., Villaume, S., and Thépaut, J.-N.: The ERA5 Global Reanalysis, Q. J. Roy. Meteor. Soc., 146, 730, 1999–2049, https://doi.org/10.1002/qj.3803, 2020.
Hill, A. A., Dobbie, S., and Yin, Y.: The impact of aerosols on non-precipitating marine stratocumulus. Model description and prediction of the indirect effect, Q. J. Roy. Meteor. Soc., 134, 1143–1154, https://doi.org/10.1002/qj.278, 2008.
Hintze, J. L. and Nelson, R. D.: Violin Plots: A Box Plot-Density Trace Synergism, Am. Stat., 52, 181–184, 1998.
Jiang, H., Feingold, G., and Sorooshian, A.: Effect of aerosol on the susceptibility and efficiency of precipitation in trade cumulus clouds, J. Atmos. Sci., 67, 3525–3540, 2010.
Jung, E., Albrecht, B. A., Sorooshian, A., Zuidema, P., and Jonsson, H. H.: Precipitation susceptibility in marine stratocumulus and shallow cumulus from airborne measurements, Atmos. Chem. Phys., 16, 11395–11413, https://doi.org/10.5194/acp-16-11395-2016, 2016.
Khairoutdinov, M. and Kogan, Y.: A new cloud physics parameterization in a large-eddy simulation model of marine stratocumulus, Mon. Weather Rev., 128, 229–243, 2000.
King, W. D., Parkin, D. A., and Handsworth, R. J.: A hot-wire liquid water device having fully calculable response characteristics, J. Appl. Meteorol., 17, 1809–1813, https://doi.org/10.1175/1520-0450(1978)017<1809:AHWLWD>2.0.CO;2, 1978.
Klein, S. A. and Hartmann, D. L.: The seasonal cycle of low stratiform clouds, J. Climate, 6, 1587–1606, 1993.
Köhler, M., Ahlgrimm, M., and Beljaars, A.: Unified treatment of dry convective and stratocumulus-topped boundary layers in the ECMWF model, Q. J. Roy. Meteor. Soc., 137, 43–57, 2011.
Kubar, T. L., Hartmann, D. L., and Wood, R.: Understanding the importance of microphysics and macrophysics for warm rain in marine low clouds. Part I: Satellite observations, J. Atmos. Sci. 66, 2953–2972, 2009.
Lance, S., Brock, C. A., Rogers, D., and Gordon, J. A.: Water droplet calibration of the Cloud Droplet Probe (CDP) and in-flight performance in liquid, ice and mixed-phase clouds during ARCPAC, Atmos. Meas. Tech., 3, 1683–1706, https://doi.org/10.5194/amt-3-1683-2010, 2010.
Lawrence, M. G.: The relationship between relative humidity and the dewpoint temperature in moist air: A simple conversion and applications, B. Am. Meteorol. Soc., 86, 225–233, 2005.
Lawson, R. P., Stewart, R. E., and Angus, L. J.: Observations and numerical simulations of the origin and development of very large snowflakes, J. Atmos. Sci., 55, 3209–3229, 1998.
Lawson, R. P., O'Connor, D., Zmarzly, P., Weaver, K., Baker, B. A., Mo, Q., and Jonsson, H.: The 2D-S (Stereo) probe: Design and preliminary tests of a new airborne, high-speed, high-resolution imaging probe, J. Atmos. Ocean. Tech., 23, 1462–1477, 2006.
LeBlanc, S. E., Redemann, J., Flynn, C., Pistone, K., Kacenelenbogen, M., Segal-Rosenheimer, M., Shinozuka, Y., Dunagan, S., Dahlgren, R. P., Meyer, K., Podolske, J., Howell, S. G., Freitag, S., Small-Griswold, J., Holben, B., Diamond, M., Wood, R., Formenti, P., Piketh, S., Maggs-Kölling, G., Gerber, M., and Namwoonde, A.: Above-cloud aerosol optical depth from airborne observations in the southeast Atlantic, Atmos. Chem. Phys., 20, 1565–1590, https://doi.org/10.5194/acp-20-1565-2020, 2020.
Mardi, A. H., Dadashazar, H., MacDonald, A. B., Crosbie, E., Coggon, M. M., Aghdam, M. A., Woods, R. K., Jonsson, H. H., Flagan, R. C., Seinfeld, J. H., and Sorooshian, A.: Effects of Biomass Burning on Stratocumulus Droplet Characteristics, Drizzle Rate, and Composition, J. Geophys. Res.-Atmos., 124, 12301–12318, https://doi.org/10.1029/2019jd031159, 2019.
Mauger, G. S. and Norris, J. R.: Meteorological bias in satellite estimates of aerosol-cloud relationships, Geophys. Res. Lett., 34, L16824, https://doi.org/10.1029/2007GL029952, 2007.
McComiskey, A. and Feingold, G.: The scale problem in quantifying aerosol indirect effects, Atmos. Chem. Phys., 12, 1031–1049, https://doi.org/10.5194/acp-12-1031-2012, 2012.
McFarquhar, G. M., Finlon, J. A., Stechman, D. M., Wu, W., Jackson, R. C., and Freer, M.: University of Illinois/Oklahoma Optical Array Probe (OAP) Processing Software, Zenodo [code], https://doi.org/10.5281/zenodo.1285969, 2018.
Morrison, H. and Gettelman, A.: A new two-moment bulk stratiform cloud microphysics scheme in the community atmosphere model, version 3 (CAM3). Part I: Description and numerical tests, J. Climate, 21, 3642–3659, https://doi.org/10.1175/2008JCLI2105.1, 2008.
O’Brien, J. R., Poellot, M., Delene, D., McFarquhar, G., Spanu, A., Gupta, S., Detwiler, A., and Miller, R.: The Impacts of Airborne Cloud Microphysical Instrumentation Mounting Location on Measurements Made During the Observations of Aerosols and Clouds and Their Interactions (ORACLES) Project, in preparation, 2022.
ORACLES Science Team: Suite of Aerosol, Cloud, and Related Data Acquired Aboard P3 During ORACLES 2018, Version 2, NASA Ames Earth Science Project Office [data set], https://doi.org/10.5067/Suborbital/ORACLES/P3/2018_V2, 2020a.
ORACLES Science Team: Suite of Aerosol, Cloud, and Related Data Acquired Aboard P3 During ORACLES 2017, Version 2, NASA Ames Earth Science Project Office [data set], https://doi.org/10.5067/Suborbital/ORACLES/P3/2017_V2, 2020b.
ORACLES Science Team: Suite of Aerosol, Cloud, and Related Data Acquired Aboard P3 During ORACLES 2016, Version 2, NASA Ames Earth Science Project Office [data set], https://doi.org/10.5067/Suborbital/ORACLES/P3/2016_V2, 2020c.
Oreopoulos, L. and Rossow, W. B.: The cloud radiative effects of International Satellite Cloud Climatology Project weather states, J. Geophys. Res.-Atmos., 116, D12202, https://doi.org/10.1029/2010JD015472, 2011.
Painemal, D. and Zuidema, P.: Assessment of MODIS cloud effective radius and optical thickness retrievals over the Southeast Pacific with VOCALS-REx in situ measurements, J. Geophys. Res.-Atmos., 116, D24206, https://doi.org/10.1029/2011JD016155, 2011.
Penner, J. E., Quaas, J., Storelvmo, T., Takemura, T., Boucher, O., Guo, H., Kirkevåg, A., Kristjánsson, J. E., and Seland, Ø.: Model intercomparison of indirect aerosol effects, Atmos. Chem. Phys., 6, 3391–3405, https://doi.org/10.5194/acp-6-3391-2006, 2006.
Pennypacker, S., Diamond, M., and Wood, R.: Ultra-clean and smoky marine boundary layers frequently occur in the same season over the southeast Atlantic, Atmos. Chem. Phys., 20, 2341–2351, https://doi.org/10.5194/acp-20-2341-2020, 2020.
Pistone, K., Redemann, J., Doherty, S., Zuidema, P., Burton, S., Cairns, B., Cochrane, S., Ferrare, R., Flynn, C., Freitag, S., Howell, S. G., Kacenelenbogen, M., LeBlanc, S., Liu, X., Schmidt, K. S., Sedlacek III, A. J., Segal-Rozenhaimer, M., Shinozuka, Y., Stamnes, S., van Diedenhoven, B., Van Harten, G., and Xu, F.: Intercomparison of biomass burning aerosol optical properties from in situ and remote-sensing instruments in ORACLES-2016, Atmos. Chem. Phys., 19, 9181–9208, https://doi.org/10.5194/acp-19-9181-2019, 2019.
Pistone, K., Zuidema, P., Wood, R., Diamond, M., da Silva, A. M., Ferrada, G., Saide, P. E., Ueyama, R., Ryoo, J.-M., Pfister, L., Podolske, J., Noone, D., Bennett, R., Stith, E., Carmichael, G., Redemann, J., Flynn, C., LeBlanc, S., Segal-Rozenhaimer, M., and Shinozuka, Y.: Exploring the elevated water vapor signal associated with the free tropospheric biomass burning plume over the southeast Atlantic Ocean, Atmos. Chem. Phys., 21, 9643–9668, https://doi.org/10.5194/acp-21-9643-2021, 2021.
Platnick, S. and Twomey, S.: Determining the susceptibility of cloud albedo to changes in droplet concentration with the advanced very high resolution radiometer, J. Appl. Meteor., 33, 334–346, 1994.
Possner, A., Eastman, R., Bender, F., and Glassmeier, F.: Deconvolution of boundary layer depth and aerosol constraints on cloud water path in subtropical stratocumulus decks, Atmos. Chem. Phys., 20, 3609–3621, https://doi.org/10.5194/acp-20-3609-2020, 2020.
Quaas, J., Arola, A., Cairns, B., Christensen, M., Deneke, H., Ekman, A. M. L., Feingold, G., Fridlind, A., Gryspeerdt, E., Hasekamp, O., Li, Z., Lipponen, A., Ma, P.-L., Mülmenstädt, J., Nenes, A., Penner, J. E., Rosenfeld, D., Schrödner, R., Sinclair, K., Sourdeval, O., Stier, P., Tesche, M., van Diedenhoven, B., and Wendisch, M.: Constraining the Twomey effect from satellite observations: issues and perspectives, Atmos. Chem. Phys., 20, 15079–15099, https://doi.org/10.5194/acp-20-15079-2020, 2020.
Rao S., Dey S.: Consistent signal of aerosol indirect and semi-direct effect on water clouds in the oceanic regions adjacent to the Indian subcontinent, Atmos. Res., 232, 104677, https://doi.org/10.1016/j.atmosres.2019.104677, 2020.
Redemann, J., Wood, R., Zuidema, P., Doherty, S. J., Luna, B., LeBlanc, S. E., Diamond, M. S., Shinozuka, Y., Chang, I. Y., Ueyama, R., Pfister, L., Ryoo, J.-M., Dobracki, A. N., da Silva, A. M., Longo, K. M., Kacenelenbogen, M. S., Flynn, C. J., Pistone, K., Knox, N. M., Piketh, S. J., Haywood, J. M., Formenti, P., Mallet, M., Stier, P., Ackerman, A. S., Bauer, S. E., Fridlind, A. M., Carmichael, G. R., Saide, P. E., Ferrada, G. A., Howell, S. G., Freitag, S., Cairns, B., Holben, B. N., Knobelspiesse, K. D., Tanelli, S., L'Ecuyer, T. S., Dzambo, A. M., Sy, O. O., McFarquhar, G. M., Poellot, M. R., Gupta, S., O'Brien, J. R., Nenes, A., Kacarab, M., Wong, J. P. S., Small-Griswold, J. D., Thornhill, K. L., Noone, D., Podolske, J. R., Schmidt, K. S., Pilewskie, P., Chen, H., Cochrane, S. P., Sedlacek, A. J., Lang, T. J., Stith, E., Segal-Rozenhaimer, M., Ferrare, R. A., Burton, S. P., Hostetler, C. A., Diner, D. J., Seidel, F. C., Platnick, S. E., Myers, J. S., Meyer, K. G., Spangenberg, D. A., Maring, H., and Gao, L.: An overview of the ORACLES (ObseRvations of Aerosols above CLouds and their intEractionS) project: aerosol–cloud–radiation interactions in the southeast Atlantic basin, Atmos. Chem. Phys., 21, 1507–1563, https://doi.org/10.5194/acp-21-1507-2021, 2021.
Rogers, R. R. and Yau, M. K.: A Short Course in Cloud Physics, 3rd edn., International Series in Natural Philosophy, Butterworth-Heinemann, 290 pp., ISBN 9780750632157, 1989.
Sakaeda, N., Wood, R., and Rasch, P. J.: Direct and semidirect aerosol effects of southern African biomass burning aerosol, J. Geophys. Res., 116, D12205, https://doi.org/10.1029/2010JD015540, 2011.
Small, J. D., Chuang, P. Y., Feingold, G., and Jiang, H.: Can aerosol decrease cloud lifetime?, Geophys. Res. Lett., 36, 16806, https://doi.org/10.1029/2009GL038888, 2009.
Sorooshian, A., Feingold, G., Lebsock, M. D., Jiang, H., and Stephens, G. L.: On the precipitation susceptibility of clouds to aerosol perturbations, Geophys. Res. Lett., 36, L13803, https://doi.org/10.1029/2009GL038993, 2009.
Sorooshian, A., Feingold, G., Lebsock, M. D., Jiang, H., and Stephens, G.: Deconstructing the precipitation susceptibility construct: improving methodology for aerosol cloud precipitation studies, J. Geophys. Res., 115, D17201, https://doi.org/10.1029/2009JD013426, 2010.
Sorooshian, A., Anderson, B., Bauer, S. E., Braun, R. A., Cairns, B., Crosbie, E., Dadashazar, H., Diskin, G., Ferrare, R., Flagan, R. C., Hair, J., Hostetler, C., Jonsson, H. H., Kleb, M. M., Liu, H. Y., MacDonald, A. B., McComiskey, A., Moore, R., Painemal, D., Russell, L. M., Seinfeld, J. H., Shook, M., Smith, W. L., Thornhill, K., Tselioudis, G., Wang, H. L., Zeng, X. B., Zhang, B., Ziemba, L., and Zuidema, P.: Aerosol-Cloud-Meteorology Interaction Airborne Field Investigations: Using Lessons Learned from the US West Coast in the Design of ACTIVATE off the US East Coast, B. Am. Meteorol. Soc., 100, 1511–1528, https://doi.org/10.1175/Bams-D-18-0100.1, 2019.
Stephens, G. L., L'Ecuyer, T., Forbes, R., Gettlemen, A., Golaz, J. C., Bodas-Salcedo, A., Suzuki, K., Gabriel, P., and Haynes, J.: Dreary state of precipitation in global models, J. Geophys. Res.- Atmos., 115, D24211, https://doi.org/10.1029/2010jd014532, 2010.
Terai, C. R., Wood, R., Leon, D. C., and Zuidema, P.: Does precipitation susceptibility vary with increasing cloud thickness in marine stratocumulus?, Atmos. Chem. Phys., 12, 4567–4583, https://doi.org/10.5194/acp-12-4567-2012, 2012.
Toll, V., Christensen, M., Quaas, J., and Bellouin, N.: Weak average liquid-cloud-water response to anthropogenic aerosols, Nature, 572, 51–55, https://doi.org/10.1038/s41586-019-1423-9, 2019.
Trenberth, K. and Fasullo, J.: Global warming due to increasing absorbed solar radiation, Geophys. Res. Lett., 36, L07706, https://doi.org/10.1029/2009GL037527, 2009.
Twomey, S.: Pollution and the Planetary Albedo, Atmos. Environ., 8, 1251–1256, 1974.
Twomey, S.: The influence of pollution on the shortwave albedo of clouds, J. Atmos. Sci., 34, 1149–1152, 1977.
Wilcox, E. M.: Stratocumulus cloud thickening beneath layers of absorbing smoke aerosol, Atmos. Chem. Phys., 10, 11769–11777, https://doi.org/10.5194/acp-10-11769-2010, 2010.
Wood, R.: Stratocumulus Clouds, Mon. Weather Rev., 140, 2373–2423, https://doi.org/10.1175/MWR-D-11-00121.1, 2012.
Wood, R. and Bretherton, C. S.: On the relationship between stratiform low cloud cover and lower-tropospheric stability, J. Climate, 19, 6425–6432, 2006.
Wood, R. and Hartmann, D. L.: Spatial variability of liquid water path in marine low cloud: the importance of mesoscale cellular convection, J. Climate, 19, 1748–1764, 2006.
Wood, R., Kubar, T. L., and Hartmann, D. L.: Understanding the Importance of Microphysics and Macrophysics for Warm Rain in Marine Low Clouds. Part II: Heuristic Models of Rain Formation, J. Atmos. Sci., 66, 2973–2990, https://doi.org/10.1175/2009JAS3072.1, 2009.
Xue, H. and Feingold, G.: Large eddy simulations of trade wind cumuli: investigation of aerosol indirect effects, J. Atmos. Sci., 63, 1605–1622, 2006.
Zhang, Z. and Platnick, S.: An assessment of differences between cloud effective particle radius retrievals for marine water clouds from three MODIS spectral bands, J. Geophys. Res.-Atmos., 116, D20215, https://doi.org/10.1029/2011JD016216, 2011.
Zuidema, P., Redemann, J., Haywood, J., Wood, R., Piketh, S., Hipondoka, M. and Formenti, P.: Smoke and Clouds above the Southeast Atlantic: Upcoming Field Campaigns Probe Absorbing Aerosol's Impact on Climate, B. Am. Meteorol. Soc., 97, 1131–1135, https://doi.org/10.1175/BAMS-D-15-00082.1, 2016.
- Abstract
- Introduction
- Observations
- Cloud properties
- Aerosol influence on cloud microphysics
- Precipitation formation and H
- Aerosol influence on precipitation
- Meteorological influence on LWP
- Conclusions
- Appendix A: Sensitivity studies on dependence of So on H
- Appendix B: Sensitivity studies on dependence of So on Rp
- Appendix C: Dependence of So on the definition of clean and polluted boundary layers
- Appendix D: Sensitivity of So to the use of CAS data from ORACLES 2016
- Code availability
- Data availability
- Author contributions
- Competing interests
- Disclaimer
- Special issue statement
- Acknowledgements
- Financial support
- Review statement
- References
- Supplement
- Abstract
- Introduction
- Observations
- Cloud properties
- Aerosol influence on cloud microphysics
- Precipitation formation and H
- Aerosol influence on precipitation
- Meteorological influence on LWP
- Conclusions
- Appendix A: Sensitivity studies on dependence of So on H
- Appendix B: Sensitivity studies on dependence of So on Rp
- Appendix C: Dependence of So on the definition of clean and polluted boundary layers
- Appendix D: Sensitivity of So to the use of CAS data from ORACLES 2016
- Code availability
- Data availability
- Author contributions
- Competing interests
- Disclaimer
- Special issue statement
- Acknowledgements
- Financial support
- Review statement
- References
- Supplement