the Creative Commons Attribution 4.0 License.
the Creative Commons Attribution 4.0 License.
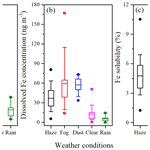
Sources and processes of iron aerosols in a megacity in Eastern China
Yanhong Zhu
Yue Wang
Jian Zhang
Liang Xu
Jingsha Xu
Jinhui Shi
Longyi Shao
Pingqing Fu
Daizhou Zhang
Iron (Fe) in aerosol particles is a major external source of micronutrients for marine ecosystems and poses a potential threat to human health. To understand the impacts of aerosol Fe, it is essential to quantify the sources of dissolved Fe and total Fe. In this study, we applied receptor modeling for the first time to apportion the sources of dissolved Fe and total Fe in fine particles collected under five different weather conditions in the Hangzhou megacity of Eastern China, which is upwind of the East Asian outflow. Results showed that Fe solubility (dissolved Fe to total Fe) was the largest on fog days (6.7 ± 3.0 %), followed by haze (4.8 ± 1.9 %), dust (2.1 ± 0.7 %), clear (1.9 ± 1.0 %), and rain (0.9 ± 0.5 %) days. Positive matrix factorization (PMF) analysis suggested that industrial emissions were the largest contributor to dissolved Fe (44.5 %–72.4 %) and total Fe (39.1 %–55.0 %, except for dust days) during haze, fog, dust, and clear days. Transmission electron microscopy analysis of individual particles showed that > 75 % of Fe-containing particles were internally mixed with acidic secondary aerosol species on haze, fog, dust, and clear days. Furthermore, Fe solubility showed significant positive correlations with aerosol acidity/total Fe and liquid water content. These results indicated that the wet surface of aerosol particles promotes heterogeneous reactions between acidic species and Fe aerosols, contributing to a high Fe solubility.
- Article
(1905 KB) - Full-text XML
-
Supplement
(675 KB) - BibTeX
- EndNote
The deposition of atmospheric aerosols is a major external source of iron (Fe) in the ocean (Li et al., 2017; Pinedo-González et al., 2020; Yang et al., 2020). Fe is an essential micronutrient that can impact phytoplankton primary productivity, thereby modulating marine ecosystems, global carbon cycling, and climate (Jickells et al., 2005; Tagliabue et al., 2017; Matsui et al., 2018; Lei et al., 2018). In addition, atmospheric Fe-containing particles have an adverse effect on human health, by generating reactive oxygen species (ROS; Abbaspour et al., 2014), and can convert S(IV) to S(VI) by catalytic oxidation for atmospheric sulfate (SO) production (Alexander et al., 2009). These roles of Fe largely depend on the atmospheric Fe solubility (Shi et al., 2012; Baker et al., 2021). Unfortunately, field observations on atmospheric Fe solubility are still limited, and the available data show a wide range of Fe solubility (0.02 % to 98 %) in different atmospheric environments (Schroth et al., 2009; Shi et al., 2012; Oakes et al., 2012; Myriokefalitakis et al., 2015).
There are two major processes that can significantly increase Fe solubility in atmospheric aerosols, including aerosol primary emissions and atmospheric acidification processes (Shi et al., 2012). Dissolved Fe can be derived from natural and anthropogenic sources, such as mineral dust, fossil fuel combustion, biomass burning, and traffic exhaust (Chen et al., 2012; Pant et al., 2015; Conway et al., 2019; Rathod et al., 2020; Ito et al., 2020). Although natural emissions have a high emission flux, their contribution to Fe solubility is less than 1 % (Schroth et al., 2009). Recent studies have highlighted anthropogenic sources due to their high contribution to Fe solubility. For example, Schroth et al. (2009) suggested that Fe solubility was less than 1 % of the iron in arid soils, while oil combustion emissions had a pronounced effect on Fe solubility (77 %–81 %); Oakes et al. (2012) studied Fe solubility in anthropogenic source emission samples and found that Fe solubility was 0.06 % in coal fly, 46 % in biomass burning, 51 % in diesel exhaust, and 75 % in gasoline exhaust. These results imply that an increase in relative amounts of aerosols from these mixed anthropogenic sources may be responsible for the increase in Fe solubility.
There are a number of atmospheric processes which can affect Fe solubility in atmospheric aerosol particles. One of the most important processes is the mobilization of Fe in an acidic solution on the surface of aerosol particles because acidic pH can trigger faster Fe dissolution and increase Fe solubility (Shi et al., 2011; Maters et al., 2017; Li et al., 2017; Zhou et al., 2020). When ambient relative humidity (RH) is above 60 %, aerosol particles can take up water and change the surface to a wet or liquid state (with liquid–liquid separation or a homogenous state, depending on the composition and RH; Sun et al., 2018; Liu et al., 2017). The wet or liquid surface can take up acid gases (such as SO2 and NO2) and form acidic salts to promote the conversion of Fe from an undissolved to a dissolved form, thereby increasing Fe solubility (Li et al., 2017; G. Zhang et al., 2019; Yang et al., 2020; Wong et al., 2020).
The two major contributors mentioned above (aerosol primary sources and atmospheric acidification processes) to Fe solubility are associated with weather conditions, which can change the dispersion efficiency (such as boundary layer height, wind, and convection), dry/wet deposition and the chemical conversion loss rate (Leibensperger et al., 2008; Zhang et al., 2018), temperature, relative humidity, and solar radiation (Camalier et al., 2007). Recently, Shi et al. (2020) found that different levels of Fe solubility are closely related with different weather conditions in one coastal city. However, to our knowledge, studies that have attempted to investigate Fe solubility under different weather conditions in the megacity are still sparse in the world, even though the sources of aerosol Fe (such as coal combustion, vehicle emissions, and industry emissions) are densely distributed in megacities (Q. Zhang et al., 2019). Therefore, to better understand how aerosol primary sources and atmospheric acidification processing influence Fe solubility in the megacity, the planned studies should be conducted under different weather conditions.
In this study, we collected atmospheric fine particles (PM2.5) and individual particle samples on haze, fog, dust, clear, and rain days at Hangzhou, a megacity of the Yangtze River Delta (YRD), which is one of the largest modern megacity clusters in China. This study characterized Fe content and solubility under haze, fog, dust, clear, and rain weather conditions and discussed the impacts of primary sources and atmospheric acidification processes on Fe solubility.
2.1 Sampling site
The sampling site was located on the Zijingang Campus of the Zhejiang University in Hangzhou (120∘12′ E, 30∘16′ N), a megacity in the YRD, China (Fig. S1 in the Supplement). Industrial emissions are relatively low in Hangzhou in comparison to other megacities in China, but traffic emissions are serious (Xu et al., 2020). In addition, pollutants emitted in surrounding regions and northern China can be transported to Hangzhou city (Liu et al., 2021b).
2.2 Sample collection
PM2.5 aerosol and individual particle samples were collected under haze, fog, dust, clear, and rain weather conditions between November 2018 and January 2020. Details on the sampling periods are shown in Table S1. The definitions of haze, fog, dust, clear, and rain weather conditions are shown in Table S2. When the duration of haze, fog, or dust exceeded 70 % of the collection time of a sample, the sample was classified as a haze, fog, or dust sample. In total, there were 34 haze samples, 17 fog samples, 12 dust samples, 37 clear samples, and 9 rain samples in this study.
A Th-16a intelligent sampler (Wuhan Tianhong Environmental Protection Industry Co., Ltd., China) with a flow rate of 100 L min−1 was used to collect PM2.5 samples on 90 mm diameter quartz filters for 11.5 h (daytime is 08:30–20:00 local time (LT); nighttime is 20:30–08:00 LT of the next day). The sampler was installed on the rooftop of a four-story teaching building (approximately 20 m above the ground) on the Zijingang campus of Zhejiang University. All quartz filters were firstly baked at 600 ∘C in a muffle furnace for 4 h to remove contaminants. Then, these filters were conditioned in a room with a temperature of 20 ± 1 ∘C and RH of 50 ± 2 %. After 24 h, these filters were weighed using a Sartorius analytical balance (detection limit 0.001 mg). After sample collection, the loaded filters were similarly conditioned and weighed in order to determine the PM2.5 mass concentrations. Daytime and nighttime blank samples were collected by the same method with real samples but without operating the sampler. The collected filters were preserved in a freezer at −4 ∘C until further analysis.
Individual particle samples were collected four times, at 08:00, 12:00, 18:00 and 00:00 LT, on sampling days, except for rain days. The sampler is a single-stage cascade impactor with a 0.5 mm diameter jet nozzle and a flow rate of 1.0 L min−1. The samples were collected on copper grids coated with carbon film. According to weather and visibility, the sampling duration spanned 30 s to 8 min. The collection efficiency is 50 % for particles with an aerodynamic diameter of 0.1 µm and a density of 2 g cm−3. After sampling, the grids were placed in a dry plastic tube and stored in a desiccator at 25 ∘C and 20 ± 3 % RH to minimize the exposure to ambient air.
2.3 Elemental analysis
Element concentrations were determined by an energy dispersive X-ray fluorescence (EDXRF) spectrometer (Epsilon 4; Malvern Panalytical Ltd). In this method, element concentrations on a given elemental map were measured. The measured values firstly divided by the elemental map area, then multiplied by the total sample area to obtain element concentrations of the sample. Because quartz filter contains a large amount of silicon (Si), the Si measured by EDXRF is not used in this study. Elements including Na, Mg, Al, P, S, Cl, K, Ca, Ti, V, Cr, Mn, Fe, Co, Ni, Cu, Zn, Ga, As, Se, Sr, Ba, and Pb were measured. The National Institute of Standards and Technology (NIST) standard was used as reference material for standardizing the instrument. The analysis values of NIST standard are given in Table S3, showing that the relative errors between the measured and standard value for the standard samples were less than 10 %. The average element concentrations of field blank samples (n = 4) were well below those of the samples (Table S3), indicating that there was no significant contribution of blank subtraction to the observed concentrations. The elemental concentrations used in this study were corrected by subtracting the filter blank values.
2.4 Sample preparation and analysis of dissolved Fe
Chemical analysis of the dissolved Fe was conducted using the ferrozine technique described by Viollier et al. (2000). Sample extraction and analysis were on the basis of Majestic et al. (2006) and Oakes et al. (2012). We conducted the analysis as follows: (1) half of the sample filters were placed in clean tubes with 20 mL ammonium acetate (0.5 mM; pH = 4.3). Then, the tubes were placed in an ultrasonic bath for 60 min. The extractions were filtered through a 0.22 µm PTFE syringe filter to remove undissolved particles. (2) The concentrated HCl was immediately added to the filtrate to adjust pH equal to about 1, and then the filtrate was stored in the refrigerator. (3) Before starting to analyze the stored solution, a solution of 0.01 M ascorbic acid was added to the filtrate to reduce Fe(III) to Fe(II) and held for 30 min to ensure complete Fe reduction. (4) 0.01 M ferrozine solution was added to the filtrate. (5) Ammonium acetate buffer (pH = 9.5) was added to the filtrate, making the pH between 4 and 9. Light absorption of the mixture was immediately measured by an ultraviolet–visible spectrophotometer at 562 nm (max light absorption of Fe(II)-Ferrozine complex) and 700 nm (background measurement) to yield dissolved Fe measurement. SigmaUltra-grade ammonium Fe(II) sulfate was used for Fe(II) standards. The concentration of Fe(II) obtained from the standard curve was the concentration of dissolved Fe. The detection limit of the method for Fe(II) was 0.11 ng m−3, calculated as 3 times the standard deviation of filter blank values (n = 9). The concentrations of Fe(II) in the field blanks were all below the detection limit, and the data reported in this study were corrected by subtracting the filter blank values.
2.5 Individual particle analysis
Individual particle samples were analyzed with a JEM-2100 (JEOL Ltd.) transmission electron microscope (TEM) operated at 200 kV. Elemental composition was semi-quantitatively determined by an energy-dispersive X-ray spectrometer (EDS) that can detect elements heavier than carbon (C). Copper (Cu) was excluded from the analyses because the TEM grids are made of Cu. The relative percentages of the elements were estimated based on the EDS spectra acquired through the INCA software (Oxford Instruments, Oxfordshire, UK). The distribution of aerosol particles on TEM grids was not homogeneous; coarser particles occur near the center, and finer particles are on the periphery. Therefore, to be more representative, four areas were chosen from the center to the periphery of the sampling spot on each grid. The projected areas of individual particles were determined using iTEM software (Olympus Soft Imaging Solutions GmbH, Germany), which is the standard image analysis platform for electron microscopy.
2.6 Water-soluble inorganic ions, organic carbon, and elemental carbon
The concentrations of water-soluble inorganic ions, including Na+, NH, K+, Mg2+, Ca2+, F−, Cl−, NO, and SO were obtained by an ion chromatograph (Dionex ICS-600; Thermo Fisher Scientific). Detailed descriptions about filter extraction and analysis were given in Zhu et al. (2015). Organic carbon (OC) and elemental carbon (EC) were analyzed by a carbon analyzer (Sunset Laboratory Inc.) with the thermal–optical transmittance method.
2.7 Aerosol acidity and liquid water content
A thermodynamic equilibrium model (E-AIM model II; Clegg et al., 1998) was used to calculate the aerosol acidity (in situ acidity) and liquid water content (available at http://www.aim.env.uea.ac.uk/aim/aim.php, last access: 5 December 2021). The input data include temperature, relative humidity, and the concentrations of NH, SO, NO, and H+. It was assumed that the concentration of H.
2.8 Positive matrix factorization (PMF)
The U.S. Environmental Protection Agency (U.S. EPA) PMF 5.0 model was used to identify sources of dissolved Fe and total Fe. A detailed description about PMF 5.0 is given in the user manual (Norris et al., 2014). There are two input files required to initiate the model, where one contains the concentration values and one contains the uncertainty values for each species. Uncertainty was determined as follows (Polissar et al., 1998):
where Ci is the concentration value, MDL is the method detection limit, and Unc is the uncertainty. The principals of PMF running and species choice have been described in the PMF 5.0 user manual and our previous study (Zhu et al., 2017). Since the number of samples should be 3 times higher than the number of species used in PMF, accurate PMF results could be obtained, so we used the sum of all samples under haze, fog, dust, and clear weather conditions to run PMF model. In this study, 100 samples were used to run the PMF model. PM2.5, OC, EC, SO, NO, NH, Mg, Al, K, Ca, Ti, Cr, Mn, Co, Ni, Cu, Zn, As, Se, Sr, Ba, Pb, dissolved Fe, and undissolved Fe (= total Fe − dissolved Fe) were used for the PMF analysis, and six factors were resolved as the optimal solution. Dissolved Fe was set as total variable, and PM2.5 was set as a weak variable. The changes in Q values can provide insight into the rotation of factors. The QRobust (2392.94) was close to QTrue (2474.51), suggesting that PMF results can reasonably explain potential sources of dissolved Fe.
3.1 Pollution levels
The average PM2.5 concentration was the highest at 98.5 ± 19.6 µg m−3 on haze days, followed by 59.3 ± 11.1 µg m−3 on dust days, 56.6 ± 21.4 µg m−3 on fog days, 33.7 ± 14.3 µg m−3 on clear days, and 24.9 ± 6.4 µg m−3 on rain days. About 100 %, 29 %, and 8 % of PM2.5 concentrations on haze, fog, and dust days were higher than the grade II national PM2.5 standard of 75 µg m−3 (24 h average standard; GuoBiao (GB) 3095-2012, China), respectively. However, all of PM2.5 concentrations on clear and rain days were lower than the PM2.5 grade II standard. PM2.5 concentrations differed significantly according to the weather conditions (p < 0.01; independent sample T test; Table S4).
3.2 Fe content and solubility
The average concentrations of total Fe and dissolved Fe were 777.6 ± 295.1 and 37.0 ± 18.4 ng m−3 on haze days, 929.7 ± 412.7 and 59.1 ± 38.2 ng m−3 on fog days, 2945.9 ± 735.1 and 57.4 ± 12.4 ng m−3 on dust days, 639.6 ± 195.7 and 12.8 ± 8.9 ng m−3 on clear days, and 652.5 ± 306.5 and 5.4 ± 4.3 ng m−3 on rain days (Fig. 1a and b). Total Fe concentrations differed significantly according to the weather conditions (p < 0.01 or 0.05; independent sample T test; Table S4), except between haze and clear days (p > 0.1) and between fog and clear days (p > 0.5). Dissolved Fe concentrations differed significantly according to weather conditions (p < 0.01 or 0.05). The contributions of total and dissolved Fe concentrations to PM2.5 concentration are shown in Table 1. The contribution of total Fe to PM2.5 was the largest on dust days (5.2 %), followed by rain (2.8 %), clear (2.2 %), fog (2.0 %), and haze (0.8 %) days. However, the contribution of dissolved Fe to PM2.5 was the highest on fog days (0.12 %), followed by dust (0.10 %), haze (0.04 %), clear (0.03 %), and rain (0.02 %) days.
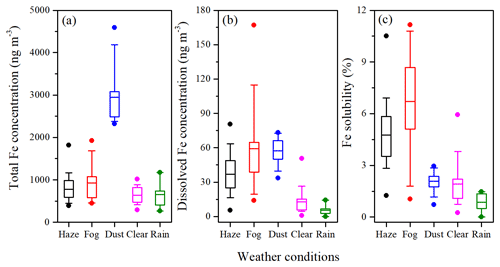
Figure 1The box-and-whisker plot of the concentrations of total Fe (a), dissolved Fe (b), and Fe solubility (c) under haze, fog, dust, clear, and rain conditions. The solid circles above and below the box show the maximum and minimum values, respectively.
Table 1Percentage contributions of total Fe and dissolved Fe concentrations to PM2.5 concentration under haze, fog, dust, clear, and rain conditions. The maximum and minimum values are in parentheses.
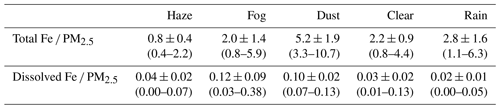
Fe solubility in aerosols was calculated as dissolved Fe total Fe concentration ×100 %. The average Fe solubility was the largest on fog days (6.7 ± 3.0 %), which was about 1.4, 3.2, 3.5, and 7.4 times higher than that on haze days (4.8 ± 1.9 %), dust days (2.1 ± 0.7 %), clear days (1.9 ± 1.0 %), and rain days (0.9 ± 0.5 %; Fig. 1c). Although the concentration of total Fe in dust days was the highest, Fe solubility was lower than that in fog and haze days. Fe solubility was extremely low in rain days, likely due to the removal of aged aerosols by wet deposition. Fe solubility differed significantly according to weather conditions (p < 0.01 or 0.05).
3.3 Factors influencing Fe solubility
3.3.1 Sources of dissolved Fe and total Fe
In order to identify the sources of dissolved Fe and total Fe, a PMF model was used to apportion their sources. PMF was run for 5 (Fig. S2), 6 (Fig. 2), and 7 (Fig. S3) factors for the evaluation of factor profiles. In Fig. S2, factor 1 of the five-factor solution is represented by high contributions of secondary inorganic ions (SO, NO, and NH) and other species from primary emissions, such as Cr, Mn, Co, Cu, Sr, and Ba, indicating an unresolved mixing factor. In Fig. S3, factor 4 of the seven-factor solution only contains a relatively high contribution of EC and As, and this factor contributes insignificantly to either PM2.5 or dissolved Fe, possibly suggesting a split of meaningful factors, such as coal combustion or industrial emissions. Hence, six factors were selected as the final solution. The selection of the optimal solution in PMF analysis was also based on the following evaluation criteria: a good correlation coefficient (r2) between the observed and predicted concentrations of fitting species, which were mostly in the range of 0.70–0.99 in this work; bootstrapping on the six-factor solution showed stable results, with more than 95 out of 100 bootstrap mapped factors; factor chemical profiles between the base and the constrained runs showed no significant difference (p > 0.05).
As shown in Fig. 2, factor 1 was identified as dust, with relatively high loads of undissolved Fe, K, Ca, and Ti (Marsden et al., 2019). Factor 2 was identified as a source of combustion, considering its high loading of EC (Hou et al., 2012). Since there was no contribution of SO and lower contributions of K and dust elements (such as Ca and Ti), factor 2 was not associated with coal and biomass burning but with traffic emissions (such as petroleum and diesel combustion; Du et al., 2018; Hao et al., 2019). Small contributions of traffic-related elements (such as Zn and Cu) suggested that factor 2 represented non-exhaust traffic emissions (Lin et al., 2015). Factor 3 was represented by high loads of SO, NO, and NH, suggesting secondary sources (Pakkanen et al., 2001; Yao et al., 2016). Factor 4 implied coal combustion because it had high loads of SO and As (Cui et al., 2019; Vedantham et al., 2014). Factor 5 was characterized by high loads of Cr, Co, Ni, Cu, Sr, Ba, and Pb, indicating industrial emissions (Cai et al., 2017; Chang et al., 2018; Liu et al., 2019; Rai et al., 2020). High loads of Co and Ni, a low load of EC, and no OC indicated heavy oil refinery processes (Zhang et al., 2007; Rao et al., 2012; Guo et al., 2016; Yeletsky et al., 2020). Similar to factor 5, factor 6 was also observed with high loads of Cr, Cu, and Pb, but it also had high contributions of Mn, Zn, and Se. Since factors 5 and 6 were not correlated in both time series and concentrations (Figs. S4 and S5), they represented two different industrial emissions. Mn, Zn, and Pb are representative elements for steel industry sources (Okuda et al., 2004; Chang et al., 2018); thus, factor 6 was associated with steel industry emissions.
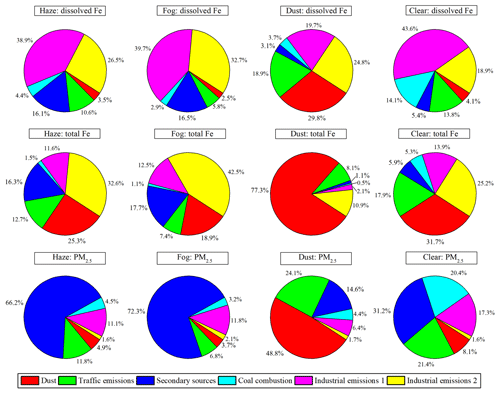
Figure 3Contributions of identified sources to dissolved Fe, total Fe, and PM2.5 on haze, fog, dust, and clear days by the PMF model.
As shown in Fig. 3, traffic emissions contributed 10.6 %, 5.8 %, 18.9 %, and 13.8 % to dissolved Fe and 12.7 %, 7.4 %, 8.1 %, and 17.9 % to total Fe on haze, fog, dust, and clear days, respectively. Although Fe solubility is as high as 51 % in diesel exhaust and 75 % in gasoline exhaust (Oakes et al., 2012), total Fe content from engine exhaust particles is extremely low. It is more than likely that traffic emissions associated with non-exhaust particles have relatively low Fe solubility. Since traffic emissions are urban sources, which are closer to the sampling site, there is less time for them to be chemically processed in the atmosphere. These results may explain why the contribution of traffic emissions to dissolved Fe is relatively low.
Figure 3 also shows that, although industrial emissions (factors 5 and 6 or industrial emissions 1 and industrial emissions 2) contributed less than 20 % to PM2.5 on haze, fog, dust, and clear days, they were the largest contributor to dissolved Fe on haze (65.4 %), fog (72.4 %), dust (44.5 %), and clear (62.5 %) days, and they were also the largest contributor to total Fe on haze (44.2 %), fog (55.0 %), and clear (39.1 %) days (with the exception of dust days). Industrial emissions 1 (factor 5) similarly contributed to dissolved Fe, regardless of the weather conditions (38.9 % to 43.6 %; with the exception of dust days), while it only contributed 11.6 % to 13.9 % to total Fe (with the exception of dust days). Heavy oil-combustion-related aerosols have the highest Fe solubility (up to 78 %) from all major Fe aerosol sources (Schroth et al., 2009; Ito et al., 2021). This may explain the much larger contribution of industrial emissions 1 to dissolved Fe than total Fe. Rathod et al. (2020) suggested that metal smelting is a dominant source of anthropogenic Fe emissions. Limited data are available on the Fe solubility in particles from metal smelting measured in high-purity water (as we did in this paper), but Mulholland et al. (2021) showed that the Fe solubility of industrial ash from an Fe–Mn alloy metallurgical plant is only about 2.8 % after 60 min at pH = 2 synthetic solutions, suggesting a very low Fe solubility in the particles. Thus, it is unlikely that primary emissions of dissolved Fe from industrial emissions 2 (factor 6) can explain its large contribution to dissolved Fe. Furthermore, PMF results indicated that secondary sources were the largest contributor to PM2.5 on haze (66.2 %), fog (72.3 %), and clear (31.2 %) days (with the exception of dust days). However, the contribution of secondary sources to dissolved Fe was relatively low, with 16.1 % on haze days, 16.5 % on fog days, 3.1 % on dust days, and 5.4 % on clear days.
The likely reason for the high contribution of industrial emissions 2 and the relatively low contribution of secondary sources to dissolved Fe is that PMF is unable to completely separate secondary sources of dissolved Fe (i.e., dissolved from insoluble Fe due to atmospheric processing) from primary sources. This means that some of the dissolved Fe due to atmospheric processing may still be assigned to its primary factors if there is a strong co-variation between the dissolved Fe and primary tracers. This suggests that the contribution of secondary sources to dissolved Fe is likely higher than that indicated by the PMF. It should also be noted that industrial emissions are outside the city, and thus, particles from these sources undergo long-range transport before reaching the sampling site. This provides more time for chemical processing in the atmosphere, leading to Fe solubilization. In the following, we further investigated the mixing of acidic species and Fe aerosols to provide further evidence for Fe solubilization from primary insoluble Fe aerosols.
3.3.2 Atmospheric acidification processing
A number of studies have considered atmospheric acidification processing as being a key factor influencing Fe solubility, in addition to direct emission of dissolved Fe from primary sources (Ito and Shi, 2016; Li et al., 2017; G. Zhang et al., 2019; Shi et al., 2020; Zhu et al., 2020; Liu et al., 2021a). As mentioned above, a proportion of dissolved Fe was associated with a PMF factor identified as secondary sources during haze, fog, dust, and clear days, thereby suggesting a contribution from atmospheric processing. To further support this result, a total of 688, 404, 580, and 311 individual particles on haze, fog, dust, and clear days were analyzed by TEM/EDS, respectively. On rain days, individual particle samples were not collected. TEM analysis showed two types of Fe-containing particles, i.e., Fe-rich and S-Fe particles. Figure 4 shows that Fe-rich particles usually contain aggregates of multiple spherical Fe particles. TEM/EDS also detected minor Fe, besides major elements (S, C, and O), in acidic secondary aerosols, and these were named S-Fe particles (Fig. 4). This is similar to that reported by Li et al. (2017), who confirmed that such Fe was presented as Fe sulfate from nanoscale secondary ion mass spectrometry (NanoSIMS) observations, indicative of acid dissolution. It should be noted that individual secondary sulfate particles in urban air normally contain nitrate, which has been confirmed in single particle mass spectrometry studies (Whiteaker et al., 2002; Li et al., 2016).
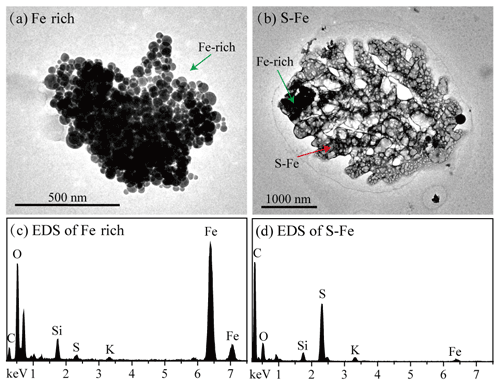
Figure 4Typical TEM images and corresponding EDS spectra of Fe-rich and S-Fe particles. (a) TEM image of Fe-rich particles. (b) TEM image of S-Fe particles. (c) EDS of Fe-rich particle. (d) EDS of S-Fe particle.
We further calculated the number contribution of S-Fe particles to Fe-containing particles, with 76.3 % on haze days, 87.1 % on fog days, 78.3 % on dust days, and 81.8 % on clear days. The result suggested that Fe particles were mostly internally mixed with acidic secondary aerosol species. To further investigate the impact of aerosol acidification on Fe solubility, the correlation of aerosol acidity/total Fe with Fe solubility was calculated. Aerosol acidity was estimated by the E-AIM model. As shown in Fig. 5, aerosol acidity/total Fe and Fe solubility show a good correlation on fog (r = 0.85, p < 0.01), haze (r = 0.56, p < 0.01), and clear (r = 0.53, p < 0.01) days (with the exception of dust days). These results further supported the above argument that the solubilization of Fe aerosols by acids. In addition, Fig. 6 shows that acidic secondary aerosol species (e.g., sulfate and nitrate) increase the size of Fe particles by about 3.6, 2.4, 4.7, and 1.9 times under haze, fog, dust, and clear conditions, respectively.
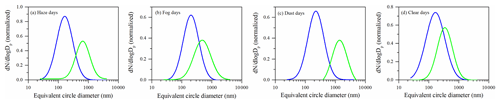
Figure 6Size distributions of Fe-rich (blue line) and S-Fe (green line) particles under haze (a), fog (b), dust (c), and clear (d) conditions. The size of the S-Fe particles represents the dry state of individual particles on the substrate. The distribution pattern is normalized.
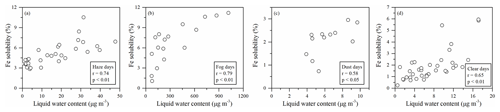
Figure 7Correlations between Fe solubility and liquid water content on haze (a), fog (b), dust (c), and clear (d) days.
On the other hand, particles with a wet surface can easily take up acidic gases (such as SO2 and NO2) to produce acidic salts (such as sulfate and nitrate), which can promote Fe dissolution (Wang et al., 2019; Li et al., 2017, 2021). Under fog conditions, the RH was higher than 90 %, which was much higher than the threshold (60 %) of the particle surface changed to a wet or liquid state (Sun et al., 2018; Liu et al., 2017). On haze and clear days, RH ranged from 35 %–79 % and 47 %–78 %, respectively. When RH > 60 %, average aerosol acidity/total Fe was 2.3 and 2.1 µmol µmol−1 on haze and clear days, respectively, and similar to that on fog days (2.4 µmol µmol−1). However, Fe solubility on haze and clear days at 5.7 % and 2.6 % were lower than 6.7 % on fog days. This could be due to the low RH on haze and clear days, which led to lower water content on the particles relative to fog days. The low water content in the aerosol particles may have limited the uptake and oxidation of acidic gases. When RH < 60 %, Fe solubility on haze and clear days was lower than 3.9 % and 2.3 %, respectively, even when aerosol acidity/total Fe was high. On dust days, RH only ranged from 22 % to 48 % and Fe solubility was less than 2.9 %. Furthermore, the E-AIM model was also employed to estimate liquid water content. Lower correlations between the Fe solubility and liquid water content on haze (r = 0.74; p < 0.01), clear (r = 0.65; p < 0.01), and dust (r = 0.58; p < 0.05) days than on fog days (r = 0.79; p < 0.01) further supported these results (Fig. 7).
The average Fe solubility was the largest on fog days (6.7 ± 3.0 %), which was about 1.4 times higher than on haze days (4.8 ± 1.9 %), 3.2 times higher than on dust days (2.1 ± 0.7 %), 3.5 times higher than on clear days (1.9 ± 1.0 %), and 7.4 times higher than on rain days (0.9 ± 0.5 %). Industrial emissions were the largest contributor to dissolved Fe (44.5 %–72.4 %) and total Fe (39.1 %–55.0 %; with the exception of dust days) during haze, fog, dust, and clear conditions. Although small on dust (3.1 %) and clear (5.4 %) days, secondary sources significantly contributed to dissolved Fe on haze (16.1 %) and fog (16.5 %) days. Individual particle analysis further showed that about 76.3 %, 87.1 %, 78.3 %, and 81.8 % of Fe-containing particles were internally mixed with acidic secondary aerosol particles under haze, fog, dust, and clear conditions, respectively. Our study indicated that the wet surface of aerosol particles (when RH > 60 %) may facilitate the update of acidic species and, thereby, promote Fe dissolution and increase Fe solubility. Higher RH on fog days (> 90 %) compared with haze (35 %–79 %), dust (22 %–48 %), and clear (47 %–78 %) days resulted in more effective aerosol acidification and higher Fe solubility.
Maher et al. (2016) and Lu et al. (2020) reported that, when the atmospheric Fe3O4 particle has a size of < 200 nm, it can access the brain directly via transport through the neuronal axons of the olfactory or trigeminal nerves. In this study, the peak size of Fe-rich particles was 175, 200, 225, and 175 nm on haze, fog, dust, and clear days, respectively. Therefore, Fe aerosols, regardless of the weather conditions, are a potential hazard to human health in densely populated megacities.
Fe-containing particles from the continent can be transported and further deposited to the ocean (Winton et al., 2015; Yoshida et al., 2018; Conway et al., 2019). Li et al. (2017) found large amounts of anthropogenic fine Fe-containing particles in the East China Sea. In this study, the prevailing winds during the sampling period were dominated by the westerly or northwesterly winds under haze, fog, and dust conditions, suggesting that Fe-containing particles were likely transported into the ocean. In the future, biogeochemical cycle model should consider Fe-containing particles from upwind continental areas of the ocean.
The data used in this study are available from the corresponding author upon request (liweijun@zju.edu.cn).
The supplement related to this article is available online at: https://doi.org/10.5194/acp-22-2191-2022-supplement.
YZ, WL, and ZS designed the study. JZ, YZ, LL, and LX collected aerosol and individual particle samples. YZ and YW contributed the laboratory experiments. YZ, WL, ZS, and JX performed the data analysis. YZ and WL wrote the paper and prepared the material, with contributions from all the co-authors. JS, LS, PF, DZ and ZS commented on the paper.
The contact author has declared that neither they nor their co-authors have any competing interests.
Publisher's note: Copernicus Publications remains neutral with regard to jurisdictional claims in published maps and institutional affiliations.
We thank the Atmospheric Science Practice Center of the School of Earth Sciences, Zhejiang University, for sharing the meteorological data during the sampling period.
This research has been supported by the National Natural Science Foundation of China (grant nos. 41907186 and 42075096), the China Postdoctoral Science Foundation (grant no. 2019M652059), the Natural Science Foundation of Zhejiang Province (grant no. LZ19D050001), and the UK Natural Environment Research Council (grant nos. NE/N007190/1 and NE/R005281/1).
This paper was edited by Timothy Bertram and reviewed by two anonymous referees.
Abbaspour, N., Hurrell, R., and Kelishadi, R.: Review on iron and its importance for human health, J. Res. Med. Sci., 19, 164–174, 2014.
Alexander, B., Park, R. J., Jacob, D. J., and Gong, S.: Transition metal-catalyzed oxidation of atmospheric sulfur: global implications for the sulfur budget, J. Geophys. Res.-Atmos., 114, D02309, https://doi.org/10.1029/2008JD010486, 2009.
Baker, A., Kanakidou, M., Nenes, A., Myriokefalitakis, S., Croot, P. L., Duce, R. A., Gao, Y., Guieu, C., Ito, A., Jickells, T., Mahowald, N. M., Middag, R., Perron, M. M. G., Sarin, M. M., Shelley, R., and Turner, D. R.: Changing atmospheric acidity as a modulator of nutrient deposition and ocean biogeochemistry, Sci. Adv., 7, eabd8800, https://doi.org/10.1126/sciadv.abd8800, 2021.
Cai, J., Wang, J., Zhang, Y., Tian, H., Zhu, C., Gross, D. S., Hu, M., Hao, J., He, K., Wang, S., and Zheng, M.: Source apportionment of Pb-containing particles in Beijing during January 2013, Environ. Pollut., 226, 30–40, https://doi.org/10.1016/j.envpol.2017.04.004, 2017.
Camalier, L., Cox, W., and Dolwick, P.: The effects of meteorology and their use in assessing ozone trends, Atmos. Environ., 41, 7127–7137, 2007.
Chang, Y., Huang, K., Xie, M., Deng, C., Zou, Z., Liu, S., and Zhang, Y.: First long-term and near real-time measurement of trace elements in China's urban atmosphere: temporal variability, source apportionment and precipitation effect, Atmos. Chem. Phys., 18, 11793–11812, https://doi.org/10.5194/acp-18-11793-2018, 2018.
Chen, H., Laskin, A., Baltrusaitis, J., Gorski, C. A., Scherer, M. M., and Grassian, V. H.: Coal Fly Ash as a Source of Iron in Atmospheric Dust, Environ. Sci. Technol., 46, 2112–2120, https://doi.org/10.1021/es204102f, 2012.
Clegg, S. L., Brimblecombe, P., and Wexler, A. S.: Thermodynamic model of the system H+-NH-SO-NO-H2O at tropospheric temperatures, J. Phys. Chem. A, 102, 2137–2154, https://doi.org/10.1021/jp973042r, 1998.
Conway, T. M., Hamilton, D. S., Shelley, R. U., Aguilar-Islas, A. M., Landing, W. M., Mahowald, N. M., and John, S. G.: Tracing and constraining anthropogenic aerosol iron fluxes to the North Atlantic Ocean using iron isotopes, Nat. Commun., 10, 1–10, https://doi.org/10.1038/s41467-019-10457-w, 2019.
Cui, Y., Ji, D., Chen, H., Gao, M., Maenhaut, W., He, J., and Wang, Y.: Characteristics and Sources of Hourly Trace Elements in Airborne Fine Particles in Urban Beijing, China, J. Geophys. Res.-Atmos., 124, 11595–11613, https://doi.org/10.1029/2019jd030881, 2019.
Du, Z., Hu, M., Peng, J., Zhang, W., Zheng, J., Gu, F., Qin, Y., Yang, Y., Li, M., Wu, Y., Shao, M., and Shuai, S.: Comparison of primary aerosol emission and secondary aerosol formation from gasoline direct injection and port fuel injection vehicles, Atmos. Chem. Phys., 18, 9011–9023, https://doi.org/10.5194/acp-18-9011-2018, 2018.
Guo, K., Li, H., and Yu, Z.: In-situ heavy and extra-heavy oil recovery: A review, Fuel, 185, 886–902, https://doi.org/10.1016/j.fuel.2016.08.047, 2016.
Hao, Y., Gao, C., Deng, S., Yuan, M., Song, W., Lu, Z., and Qiu, Z.: Chemical characterisation of PM2.5 emitted from motor vehicles powered by diesel, gasoline, natural gas and methanol fuel, Sci. Total Environ., 674, 128–139, https://doi.org/10.1016/j.scitotenv.2019.03.410, 2019.
Hou, L., Wang, S., Dou, C., Zhang, X., Yu, Y., Zheng, Y., Avula, U., Hoxha, M., Díaz, A., McCracken, J., Barretta, F., Marinelli, B., Bertazzi, P. A., Schwartz, J., and Baccarelli, A. A.: Air pollution exposure and telomere length in highly exposed subjects in Beijing, China: A repeated-measure study, Environ. Int., 48, 71–77, https://doi.org/10.1016/j.envint.2012.06.020, 2012.
Ito, A. and Shi, Z.: Delivery of anthropogenic bioavailable iron from mineral dust and combustion aerosols to the ocean, Atmos. Chem. Phys., 16, 85–99, https://doi.org/10.5194/acp-16-85-2016, 2016.
Ito, A., Perron, M. M., Proemse, B. C., Strzelec, M., Gault-Ringold, M., Boyd, P. W., and Bowie, A. R.: Evaluation of aerosol iron solubility over Australian coastal regions based on inverse modeling: implications of bushfires on bioaccessible iron concentrations in the Southern Hemisphere, Prog. Earth Planet. Sc., 7, 1–17, https://doi.org/10.1186/s40645-020-00357-9, 2020.
Ito, A., Ye, Y., Baldo, C., and Shi, Z. B.: Ocean fertilization by pyrogenic aerosol iron, npj Clim. Atmos. Sci., 4, 30, https://doi.org/10.1038/s41612-021-00185-8, 2021.
Jickells, T., An, Z., Andersen, K. K., Baker, A., Bergametti, G., Brooks, N., Cao, J., Boyd, P., Duce, R., and Hunter, K.: Global iron connections between desert dust, ocean biogeochemistry, and climate, Science, 308, 67–71, https://doi.org/10.1126/science.1105959, 2005.
Lei, C., Sun, Y., Tsang, D. C. W., and Lin, D.: Environmental transformations and ecological effects of iron-based nanoparticles, Environ. Pollut., 232, 10–30, https://doi.org/10.1016/j.envpol.2017.09.052, 2018.
Leibensperger, E. M., Mickley, L. J., and Jacob, D. J.: Sensitivity of US air quality to mid-latitude cyclone frequency and implications of 1980–2006 climate change, Atmos. Chem. Phys., 8, 7075–7086, https://doi.org/10.5194/acp-8-7075-2008, 2008.
Li, W., Sun, J., Xu, L., Shi, Z., Riemer, N., Sun, Y., Fu, P., Zhang, J., Lin, Y., and Wang, X.: A conceptual framework for mixing structures in individual aerosol particles, J. Geophys. Res.-Atmos., 121, 13784–13798, https://doi.org/10.1002/2016JD025252, 2016.
Li, W., Xu, L., Liu, X., Zhang, J., Lin, Y., Yao, X., Gao, H., Zhang, D., Chen, J., and Wang, W.: Air pollution–aerosol interactions produce more bioavailable iron for ocean ecosystems, Sci. Adv., 3, e1601749, https://doi.org/10.1126/sciadv.1601749, 2017.
Li, W., Teng, X., Chen, X., Liu, L., Xu, L., Zhang, J., Wang, Y., Zhang, Y., and Shi, Z.: Organic Coating Reduces Hygroscopic Growth of Phase-Separated Aerosol Particles, Environ. Sci. Technol., 55, 16339–16346, https://doi.org/10.1021/acs.est.1c05901, 2021.
Lin, Y.-C., Tsai, C.-J., Wu, Y.-C., Zhang, R., Chi, K.-H., Huang, Y.-T., Lin, S.-H., and Hsu, S.-C.: Characteristics of trace metals in traffic-derived particles in Hsuehshan Tunnel, Taiwan: size distribution, potential source, and fingerprinting metal ratio, Atmos. Chem. Phys., 15, 4117–4130, https://doi.org/10.5194/acp-15-4117-2015, 2015.
Liu, L., Lin, Q. H., Liang, Z., Du, R. G., Zhang, G. Z., Zhu, Y. H., Qi, B.; Zhou, S. Z., and Li, W. J.: Variations in concentration and solubility of iron in atmospheric fine particles during the COVID-19 pandemic: An example from China, Gondwana Res., 97, 138–144, https://doi.org/10.1016/j.gr.2021.05.022, 2021a.
Liu, L., Zhang, J., Du, R., Teng, X., Hu, R., Yuan, Q., Tang, S., Ren, C., Huang, X., Xu, L., Zhang, Y., Zhang, X., Song, C., Liu, B., Lu, G., Shi, Z., and Li, W.: Chemistry of Atmospheric Fine Particles During the COVID-19 Pandemic in a Megacity of Eastern China, Geophys. Res. Lett., 48, 2020GL091611, https://doi.org/10.1029/2020GL091611, 2021b.
Liu, S., Zhu, C., Tian, H., Wang, Y., Zhang, K., Wu, B., Liu, X., Hao, Y., Liu, W., Bai, X., Lin, S., Wu, Y., Shao, P., and Liu, H.: Spatiotemporal Variations of Ambient Concentrations of Trace Elements in a Highly Polluted Region of China, J. Geophys. Res.-Atmos., 124, 4186–4202, https://doi.org/10.1029/2018jd029562, 2019.
Liu, Y., Wu, Z., Wang, Y., Xiao, Y., Gu, F., Zheng, J., Tan, T., Shang, D., Wu, Y., Zeng, L., Hu, M., Bateman, A. P., and Martin, S. T.: Submicrometer particles are in the liquid state during heavy haze episodes in the urban atmosphere of Beijing, China, Environ. Sci. Technol. Lett., 4, 427–432, https://doi.org/10.1021/acs.estlett.7b00352, 2017.
Lu, D., Luo, Q., Chen, R., Zhuansun, Y., Jiang, J., Wang, W., Yang, X., Zhang, L., Liu, X., Li, F., Liu, Q., and Jiang, G.: Chemical multi-fingerprinting of exogenous ultrafine particles in human serum and pleural effusion, Nat. Commun., 11, 2567, https://doi.org/10.1038/s41467-020-16427-x, 2020.
Maher, B. A., Ahmed, I. A. M., Karloukovski, V., MacLaren, D. A., Foulds, P. G., Allsop, D., Mann, D. M. A., Torres-Jardón, R., and Calderon-Garciduenas, L.: Magnetite pollution nanoparticles in the human brain, P. Natl. Acad. Sci. USA, 113, 10797–10801, https://doi.org/10.1073/pnas.1605941113, 2016.
Majestic, B. J., Schauer, J. J., Shafer, M. M., Turner, J. R., Fine, P. M., Singh, M., and Sioutas, C.: Development of a wet-chemical method for the speciation of iron in atmospheric aerosols, Environ. Sci. Technol., 40, 2346–2351, https://doi.org/10.1021/es052023p, 2006.
Marsden, N. A., Ullrich, R., Möhler, O., Eriksen Hammer, S., Kandler, K., Cui, Z., Williams, P. I., Flynn, M. J., Liu, D., Allan, J. D., and Coe, H.: Mineralogy and mixing state of north African mineral dust by online single-particle mass spectrometry, Atmos. Chem. Phys., 19, 2259–2281, https://doi.org/10.5194/acp-19-2259-2019, 2019.
Maters, E. C., Delmelle, P., and Gunnlaugsson, H. P.: Controls on iron mobilisation from volcanic ash at low pH: Insights from dissolution experiments and Mössbauer spectroscopy, Chem. Geol., 449, 73–81, https://doi.org/10.1016/j.chemgeo.2016.11.036, 2017.
Matsui, H., Mahowald, N. M., Moteki, N., Hamilton, D. S., Ohata, S., Yoshida, A., Koike, M., Scanza, R. A., and Flanner, M. G.: Anthropogenic combustion iron as a complex climate forcer, Nat. Commun., 9, 1–10, https://doi.org/10.1038/s41467-018-03997-0, 2018.
Mulholland, D. S., Flament, P., de Jong, J., Mattielli, N., Deboudt, K., Dhont, G., and Bychkov, E.: In-cloud processing as a possible source of isotopically light iron from anthropogenic aerosols: New insights from a laboratory study, Atmos. Environ., 259, 118505, https://doi.org/10.1016/j.atmosenv.2021.118505, 2021.
Myriokefalitakis, S., Daskalakis, N., Mihalopoulos, N., Baker, A. R., Nenes, A., and Kanakidou, M.: Changes in dissolved iron deposition to the oceans driven by human activity: a 3-D global modelling study, Biogeosciences, 12, 3973–3992, https://doi.org/10.5194/bg-12-3973-2015, 2015.
Norris, G., Duvall, R., Brown, S., and Bai, S.: EPA positive matrix factorization (PMF) 5.0 fundamentals and user guide, US Environmental Protection Agency, 1-136, EPA/600/R-14/108, 2014.
Oakes, M., Ingall, E., Lai, B., Shafer, M., Hays, M., Liu, Z., Russell, A., and Weber, R.: Iron solubility related to particle sulfur content in source emission and ambient fine particles, Environ. Sci. Technol., 46, 6637–6644, https://doi.org/10.1021/es300701c, 2012.
Okuda, T., Kato, J., Mori, J., Tenmoku, M., Suda, Y., Tanaka, S., He, K., Ma, Y., Yang, F., Yu, X., Duan, F., and Lei, Y.: Daily concentrations of trace metals in aerosols in Beijing, China, determined by using inductively coupled plasma mass spectrometry equipped with laser ablation analysis, and source identification of aerosols, Sci. Total Environ., 330, 145–158, https://doi.org/10.1016/j.scitotenv.2004.04.010, 2004.
Pakkanen, T. A., Loukkola, K., Korhonen, C. H., Aurela, M., Makela, T., Hillamo, R. E., Aarnio, P., Koskentalo, T., Kousa, A., and Maenhaut, W.: Sources and chemical composition of atmospheric fine and coarse particles in the Helsinki area, Atmos. Environ., 35, 5381–5391, https://doi.org/10.1016/S1352-2310(01)00307-7, 2001.
Pant, P., Baker, S. J., Shukla, A., Maikawa, C., Pollitt, K. J. G., and Harrison, R. M.: The PM10 fraction of road dust in the UK and India: Characterization, source profiles and oxidative potential, Sci. Total Environ., 530-531, 445–452, https://doi.org/10.1016/j.scitotenv.2015.05.084, 2015.
Pinedo-González, P., Hawco, N. J., Bundy, R. M., Armbrust, E. V., Follows, M. J., Cael, B., White, A. E., Ferrón, S., Karl, D. M., and John, S. G.: Anthropogenic Asian aerosols provide Fe to the North Pacific Ocean, P. Natl. Acad. Sci. USA, 117, 27862–27868, https://doi.org/10.1073/pnas.2010315117, 2020.
Polissar, A. V., Hopke, P. K., Paatero, P., Malm, W. C., and Sisler, J. F.: Atmospheric aerosol over Alaska: 2. Elemental composition and sources, J. Geophys. Res.-Atmos., 103, 19045–19057, https://doi.org/10.1029/98JD01212, 1998.
Rai, P., Furger, M., Slowik, J. G., Canonaco, F., Fröhlich, R., Hüglin, C., Minguillón, M. C., Petterson, K., Baltensperger, U., and Prévôt, A. S. H.: Source apportionment of highly time-resolved elements during a firework episode from a rural freeway site in Switzerland, Atmos. Chem. Phys., 20, 1657–1674, https://doi.org/10.5194/acp-20-1657-2020, 2020.
Rao, B. P. S., Chauhan, C., Mhaisalkar, V. A., Kumar, A., Devotta, S., and Wate, S. R.: Factor Analysis for Estimating Source Contribution to Ambient Airborne Particles in and Around a Petroleum Refinery in India, Indian Chem. Eng., 54, 12–21, https://doi.org/10.1080/00194506.2012.714138, 2012.
Rathod, S. D., Hamilton, D., Mahowald, N., Klimont, Z., Corbett, J., and Bond, T.: A Mineralogy-Based Anthropogenic Combustion-Iron Emission Inventory, J. Geophys. Res.-Atmos., 125, e2019JD032114, https://doi.org/10.1029/2019JD032114, 2020.
Schroth, A. W., Crusius, J., Sholkovitz, E. R., and Bostick, B. C.: Iron solubility driven by speciation in dust sources to the ocean, Nat. Geosci., 2, 337–340, https://doi.org/10.1038/ngeo501, 2009.
Shi, J., Guan, Y., Ito, A., Gao, H., Yao, X., Baker, A. R., and Zhang, D.: High production of soluble iron promoted by aerosol acidification in fog, Geophys. Res. Lett., 47, e2019GL086124, https://doi.org/10.1029/2019GL086124, 2020.
Shi, Z., Krom, M. D., Bonneville, S., Baker, A. R., Bristow, C., Drake, N., Mann, G., Carslaw, K., McQuaid, J. B., Jickells, T., and Benning, L. G.: Influence of chemical weathering and aging of iron oxides on the potential iron solubility of Saharan dust during simulated atmospheric processing, Global Biogeochem. Cy., 25, GB2010, https://doi.org/10.1029/2010GB003837, 2011.
Shi, Z., Krom, M. D., Jickells, T. D., Bonneville, S., Carslaw, K. S., Mihalopoulos, N., Baker, A. R., and Benning, L. G.: Impacts on iron solubility in the mineral dust by processes in the source region and the atmosphere: A review, Aeolian Res., 5, 21–42, https://doi.org/10.1029/2010GB003837, 2012.
Sun, J., Liu, L., Xu, L., Wang, Y., Wu, Z., Hu, M., Shi, Z., Li, Y., Zhang, X., Chen, J., and Li, W.: Key role of nitrate in phase transitions of urban particles: implications of important reactive surfaces for secondary aerosol formation, J. Geophys. Res.-Atmos., 123, 1234–1243, https://doi.org/10.1002/2017JD027264, 2018.
Tagliabue, A., Bowie, A. R., Boyd, P. W., Buck, K. N., Johnson, K. S., and Saito, M. A.: The integral role of iron in ocean biogeochemistry, Nature, 543, 51–59, https://doi.org/10.1038/nature21058, 2017.
Vedantham, R., Landis, M. S., Olson, D., and Pancras, J. P.: Source Identification of PM2.5 in Steubenville, Ohio Using a Hybrid Method for Highly Time-Resolved Data, Environ. Sci. Technol., 48, 1718–1726, https://doi.org/10.1021/es402704n, 2014.
Viollier, E., Inglett, P., Hunter, K., Roychoudhury, A., and Van Cappellen, P.: The ferrozine method revisited: Fe (II)/Fe (III) determination in natural waters, Appl. Geochem., 15, 785–790, https://doi.org/10.1016/S0883-2927(99)00097-9, 2000.
Wang, Z., Wang, T., Fu, H., Zhang, L., Tang, M., George, C., Grassian, V. H., and Chen, J.: Enhanced heterogeneous uptake of sulfur dioxide on mineral particles through modification of iron speciation during simulated cloud processing, Atmos. Chem. Phys., 19, 12569–12585, https://doi.org/10.5194/acp-19-12569-2019, 2019.
Whiteaker, J. R., Suess, D. T., and Prather, K. A.: Effects of Meteorological Conditions on Aerosol Composition and Mixing State in Bakersfield, CA, Environ. Sci. Technol., 36, 2345–2353, https://doi.org/10.1021/es011381z, 2002.
Winton, V. H. L., Bowie, A. R., Edwards, R., Keywood, M., Townsend, A. T., van der Merwe, P., and Bollhöfer, A.: Fractional iron solubility of atmospheric iron inputs to the Southern Ocean, Mar. Chem., 177, 20—32, https://doi.org/10.1016/j.marchem.2015.06.006, 2015.
Wong, J. P. S., Yang, Y., Fang, T., Mulholland, J. A., Russell, A. G., Ebelt, S., Nenes, A., and Weber, R. J.: Fine Particle Iron in Soils and Road Dust Is Modulated by Coal-Fired Power Plant Sulfur, Environ. Sci. Technol., 54, 7088–7096, https://doi.org/10.1021/acs.est.0c00483, 2020.
Xu, L., Zhang, J., Sun, X., Xu, S., Shan, M., Yuan, Q., Liu, L., Du, Z., Liu, D., Xu, D., Song, C., Liu, B., Lu, G., Shi, Z., and Li, W.: Variation in Concentration and Sources of Black Carbon in a Megacity of China During the COVID-19 Pandemic, Geophys. Res. Lett., 47, e2020GL090444, https://doi.org/10.1029/2020GL090444, 2020.
Yang, T., Chen, Y., Zhou, S., Li, H., Wang, F., and Zhu, Y.: Solubilities and deposition fluxes of atmospheric Fe and Cu over the Northwest Pacific and its marginal seas, Atmos. Environ., 239, 117763, https://doi.org/10.1016/j.atmosenv.2020.117763, 2020.
Yao, L., Yang, L. X., Yuan, Q., Yan, C., Dong, C., Meng, C. P., Sui, X., Yang, F., Lu, Y. L., and Wang, W. X.: Sources apportionment of PM2.5 in a background site in the North China Plain, Sci. Total Environ., 541, 590–598, https://doi.org/10.1016/j.scitotenv.2015.09.123, 2016.
Yeletsky, P. M., Zaikina, O. O., Sosnin, G. A., and Kukushkin, R. G.: Heavy oil cracking in the presence of steam and nanodispersed catalysts based on different metals, Fuel Process. Technol., 199, 106239, https://doi.org/10.1016/j.fuproc.2019.106239, 2020.
Yoshida, A., Ohata, S., Moteki, N., Adachi, K., Mori, T., Koike, M., and Takami, A.: Abundance and Emission Flux of the Anthropogenic Iron Oxide Aerosols From the East Asian Continental Outflow, J. Geophys. Res.-Atmos., 123, 11194–11209, https://doi.org/10.1029/2018JD028665, 2018.
Zhang, G., Lin, Q., Peng, L., Yang, Y., Jiang, F., Liu, F., Song, W., Chen, D., Cai, Z., and Bi, X.: Oxalate formation enhanced by Fe-containing particles and environmental implications, Environ. Sci. Technol., 53, 1269–1277, https://doi.org/10.1021/acs.est.8b05280, 2019.
Zhang, Q., Zheng, Y., Tong, D., Shao, M., Wang, S., Zhang, Y., Xu. X., Wang. J., He, H., Liu, W., Ding, Y., Lei, Y., Li, J., Wang, Z., Zhang, X., Wang, Y., Cheng, J., Liu, Y., Shi, Q., Yan, L., Geng, G., Hong, C., Li, M., Liu, F., Zheng, B., Cao, J., Ding, A., Gao, J., Fu, Q., Huo, J., Liu, B., Liu, Z., Yang, F., He, K., and Hao, J.: Drivers of improved PM2.5 air quality in China from 2013 to 2017, P. Natl. Acad. Sci. USA, 116, 24463–24469, https://doi.org/10.1073/pnas.1907956116, 2019.
Zhang, S., Liu, D., Deng, W., and Que, G.: A Review of Slurry-Phase Hydrocracking Heavy Oil Technology, Energ. Fuel., 21, 6, 3057–3062, https://doi.org/10.1021/ef700253f, 2007.
Zhang, X., Zhong, J., Wang, J., Wang, Y., and Liu, Y.: The interdecadal worsening of weather conditions affecting aerosol pollution in the Beijing area in relation to climate warming, Atmos. Chem. Phys., 18, 5991–5999, https://doi.org/10.5194/acp-18-5991-2018, 2018.
Zhou, Y., Zhang, Y., Griffith, S. M., Wu, G., Li, L., Zhao, Y., Li, M., Zhou, Z., and Yu, J. Z.: Field Evidence of Fe-Mediated Photochemical Degradation of Oxalate and Subsequent Sulfate Formation Observed by Single Particle Mass Spectrometry, Environ. Sci. Technol., 54, 6562–6574, https://doi.org/10.1021/acs.est.0c00443, 2020.
Zhu, Y., Yang, L., Meng, C., Yuan, Q., Yan, C., Dong, C., Sui, X., Yao, L., Yang, F., and Lu, Y.: Indoor/outdoor relationships and diurnal/nocturnal variations in water-soluble ion and PAH concentrations in the atmospheric PM2.5 of a business office area in Jinan, a heavily polluted city in China, Atmos. Res., 153, 276–285, https://doi.org/10.1016/j.atmosres.2014.08.014, 2015.
Zhu, Y., Yang, L., Kawamura, K., Chen, J., Ono, K., Wang, X., Xue, L., and Wang, W.: Contributions and source identification of biogenic and anthropogenic hydrocarbons to secondary organic aerosols at Mt. Tai in 2014, Environ. Pollut., 220, 863–872, https://doi.org/10.1016/j.envpol.2016.10.070, 2017.
Zhu, Y., Li, W., Lin, Q., Yuan, Q., Liu, L., Zhang, J., Zhang, Y., Shao, L., Niu, H., and Yang, S.: Iron solubility in fine particles associated with secondary acidic aerosols in east China, Environ. Pollut., 264, 114769, https://doi.org/10.1016/j.envpol.2020.114769, 2020.