the Creative Commons Attribution 4.0 License.
the Creative Commons Attribution 4.0 License.
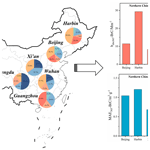
Winter brown carbon over six of China's megacities: light absorption, molecular characterization, and improved source apportionment revealed by multilayer perceptron neural network
Diwei Wang
Qian Zhang
Yali Lei
Tian Zhang
Shasha Huang
Jian Sun
Hongmei Xu
Junji Cao
Brown carbon (BrC) constitutes a large fraction of organic carbon and exhibits strong light absorption properties, thus affecting the global radiation budget. In this study, we investigated the light absorption properties, chemical functional bonds, and sources of BrC in six megacities in China, namely Beijing, Harbin, Xi'an, Chengdu, Guangzhou, and Wuhan. The average values of the BrC light absorption coefficient and the mass absorption efficiency at 365 nm in northern cities were higher than those in southern cities by 2.5 and 1.8 times, respectively, demonstrating the abundance of BrC present in northern China's megacities. Fourier transform infrared (FT-IR) spectra revealed sharp and intense peaks at 1640, 1458–1385, and 1090–1030 cm−1, which were ascribed to aromatic phenols, confirming the contribution of primary emission sources (e.g., biomass burning and coal combustion) to BrC. In addition, we noted peaks at 860, 1280–1260, and 1640 cm−1, which were attributed to organonitrate and oxygenated phenolic groups, indicating that secondary BrC also existed in the six megacities. Positive matrix factorization (PMF) coupled with multilayer perceptron (MLP) neural network analysis was used to apportion the sources of BrC light absorption. The results showed that primary emissions (e.g., biomass burning, tailpipe emissions, and coal combustion) made a major contribution to BrC in the six megacities. However, secondary formation processes made a greater contribution to light absorption in the southern cities (17.9 %–21.2 %) than in the northern cities (2.1 %–10.2 %). These results can provide a basis for the more effective control of BrC to reduce its impacts on regional climates and human health.
- Article
(3173 KB) - Full-text XML
-
Supplement
(1175 KB) - BibTeX
- EndNote
Brown carbon (BrC) constitutes a vital fraction of carbonaceous aerosols and exhibits strong light absorption properties in near-ultraviolet (UV) and visible wavelength regions (Laskin et al., 2015; Wu et al., 2021; Zhang et al., 2022). Therefore, it has received extensive attention in recent years (Laskin et al., 2015; Yan et al., 2018; Yuan et al., 2020). BrC has substantial effects on radiative forcing, cloud condensation, ice cores, and climate (Ma et al., 2020; Sreekanth et al., 2007). On the basis of remote sensing observations and chemical transport model results, studies have detected a BrC-induced non-negligible positive radiative forcing ranging from 0.1 to 0.6 W m−2 on a global scale (Jo et al., 2016; Wu et al., 2020).
BrC in urban atmospheres can originate from numerous sources, including incomplete combustion of fossil fuels (Soleimanian et al., 2020), biomass burning (Shen et al., 2017; Soleimanian et al., 2020), forest fires, and residential coal combustion (Kirchstetter et al., 2004; Soleimanian et al., 2020). In addition, both primary BrC and gaseous pollutants emitted from anthropogenic and biological activities can be converted into secondary BrC through a series of atmospheric chemical reactions (Kumar et al., 2018; Laskin et al., 2015). Studies have determined that the absorption properties of BrC exhibited distinct temporal and spatial variations in different regions and cities, and these properties were closely related to diverse emissions sources and complex atmospheric aging processes (Chung et al., 2012; Wu et al., 2021). For example, Devi et al. (2016) observed that BrC contributed differently to light absorption in the rural and urban southeast United States. Mo et al. (2021) studied the light absorption coefficient of BrC at 365 nm (BrC babs365) in 10 Chinese cities and found that the BrC babs365 value displayed obvious spatial (northern China > southern China) variations. Furthermore, a stronger light absorption ability in cold seasons (fall and winter) in Beijing (Cheng et al., 2016), Xi'an (Shen et al., 2017), Seoul (Kim et al., 2016), Taiyuan, and other cities (Mo et al., 2021) has been found to be strongly associated with increased biomass burning emissions for heating. The mass absorption efficiency at 365 nm (MAE365) of BrC has been widely used to evaluate the light-absorbing ability of BrC (Bao et al., 2022). Xie et al. (2017) found that the BrC MAE365 values from biomass burning (1.28±0.12 m2 g−1) were higher than those from vehicle emissions (0.62±0.76 m2 g−1). Ni et al. (2021) noted that BrC MAE365 values can be decreased from 1.43 to 0.11 m2 g−1 with the formation of secondary BrC aerosol from oxidation and aging processes. Another study noted that secondary organic aerosol (SOA) formation processes constituted a major source of BrC in Atlanta and Los Angeles; moreover, the optical properties of BrC differed considerably between the two cities due to differences in secondary BrC precursors (Zhang et al., 2011).
China has a high concentration of atmospheric water-soluble organic carbon, which has a major impact on regional air quality, visibility, and the climate (Mo et al., 2021). However, to our knowledge, limited study has been conducted on insight into the optical profiles, molecular composition, and sources apportionment of BrC on a large scale in China. Accurately understanding the spatial variations of the sources and light absorption properties of BrC in China is essential for reducing uncertainty about the effects of BrC on the climate. Many studies have used receptor modeling techniques such as positive matrix factorization (PMF) coupled with multiple linear regression analysis to assign the sources of BrC (Bao et al., 2022; Lei et al., 2019; Soleimanian et al., 2020). For example, Bao et al. (2022) obtained specific source contributions to BrC babs365 in Nanjing based on PMF and multiple linear regression (MLR) methods, confirming that the key contributors to BrC babs365 were mainly derived from biomass burning, primary industrial, and traffic emissions. Lei et al. (2018) investigated the source apportionment of BrC babs365 in Yulin and showed that the residential coal combustion was the highest contributor to BrC babs365 in winter. Soleimanian et al. (2020) used principal component analysis (PCA) coupled with an MLR source apportionment model, which identified fossil fuel combustion was the dominant source of BrC babs365 in central Los Angeles during summer (38 %), followed by SOA (30 %) and biomass burning (12 %). However, atmospheric processes are generally nonlinear in nature; thus traditional deterministic models could be limited. Artificial neural network (ANN)-based models, such as multilayer perceptron (MLP), have been shown to provide meaningful results closer to realistic estimates than most linear models (Borlaza et al., 2021a; Elangasinghe et al., 2014). Therefore, in this study a winter campaign for PM2.5 sampling was conducted over six of China's megacities. The purposes of this study were to (1) investigate the spatial variations of the carbonaceous matter concentrations and optical properties of BrC across six representative urban areas in China, (2) determine the molecular composition of BrC, and (3) gain insight into the relationship between light absorption and BrC sources by using PMF coupled with ANN MLP.
2.1 Sample collection
PM2.5 samples were collected in six cities in China (Fig. 1): three cities in northern China (Beijing (BJ), Harbin (HrB), and Xi'an (XA)) and three cities in southern China (Chengdu (CD), Guangzhou (GZ), and Wuhan (WH)). We classified the cities as being northern or southern according to their geographic location, such as “north or south of the Huai River”. Owing to geographical factors, these cities exhibit considerable differences in terms of energy structure and climate. The average annual temperature in northern cities is generally below 15 ∘C, while in southern cities it is usually above 15 ∘C (Mo et al., 2021). Information about the six cities and the sampling sites is summarized in Table S1 in the Supplement.
For sample collection, filter samplers were mounted on rooftops between 8 and 30 m above the ground, and samples were collected from 20 November to 22 December 2019. In BJ, HrB, and GZ, a mini-volume sampler operating at 5 L min−1 (AirMetrics, Springfield, OR, USA) was used to collect PM2.5 samples on 47 mm quartz-fiber filters (Whatman, Maidstone, UK) for 24 h. In CD, a medium-volume PM2.5 sampler operating at 100 L min−1 (HY-100SFB, Hengyuan, Qingdao, China) was used to collect PM2.5 samples on 90 mm quartz-fiber filters (Whatman). Moreover, in XA and WH, a high-volume sampler (HVS-PM2.5, Tisch Environmental Inc., Cleves, OH, USA) with a flow rate of 1.13 m3 min−1 was used to collect PM2.5 samples on quartz-fiber filters (203 mm × 254 mm, Whatman, QMA). Before sample collection, all quartz filters were prebaked at 780 ∘C for 7 h to eliminate any residual carbon. A detailed description of the quality control procedures for the filters before and after the sampling processes can be found in the article by Shen et al. (2017). After the sampling processes, the samples were sealed and stored below 0 ∘C to avoid evaporative losses before analysis.
2.2 Chemical analysis
The organic carbon (OC) and elemental carbon (EC) of the PM2.5 samples were analyzed using a thermal and optical carbon analyzer (DRI Model 2001A, Atmoslytic, Inc., USA) in accordance with the improved Interagency Monitoring of Protected Visual Environments (IMPROVE) thermal–optical reflectance protocol. Detailed descriptions of the OC and EC measurement methods can be found in the article by Cao et al. (2004). A portion of each filter (about 2.84 cm2) was extracted using 10 mL of ultrapure water to analyze water-soluble inorganic ions (Na+, NH, K+, Mg2+, Ca2+, Cl−, NO, and SO) through ion chromatography (Dionex 500, Dionex Corp, USA). A detailed description of the ion analysis method used in this study can be found in the article by Shen et al. (2008).
2.3 Optical properties of methanol extracts
A 0.526 cm2 punch was ultrasonically extracted from each filter sample by using 5 mL of methanol (HPLC grade, Fisher Scientific, NH, USA) for 30 min. Subsequently, all extracts were filtered through a microporous membrane with a diameter of 25 mm and pore size of 0.22 µm (Puradisc 25 TF, PTFE membrane) to remove insoluble components. The UV–visible absorption spectra of the BrC samples were determined using a liquid waveguide capillary cell–total OC spectrophotometer (LWCC-2100, World Precision, Sarasota, FL, USA) between the wavelengths of 200 and 700 nm. The BrC optical properties such as babs365, methanol (the absorption coefficient for methanol extracts at 365 nm) and MAE365, methanol (normalized by babs365, methanol to organic carbon, OC) were calculated as showed in a previous study (Lei et al., 2019), and details are listed in Sect. S1.
2.4 Fourier transform infrared spectroscopy spectra
Functional groups in the samples collected in six megacities were characterized using a Fourier transform infrared (FT-IR) spectrometer (Bruker Optics, Billerica, MA, USA). The method described in Sect. 2.3 was used to extract the BrC filtrates; then the BrC extracts were concentrated to 0.5 mL under a gentle nitrogen flow, after which they were mixed with 0.2 g of KBr (FT-IR grade, Sigma-Aldrich) and then blown with nitrogen to complete dryness. These dried mixtures were ground in an agate mortar and examined through FT-IR spectroscopy. The FT-IR spectrum of each sample was recorded in transmission mode by averaging 64 scans using a standard optical system with KBr windows. The spectra were recorded in the wavelength range of 4000–400 cm−1 at a resolution of 4 cm−1. Before analyzing the aerosol extract samples, we obtained the baseline spectrum by analyzing pure KBr.
2.5 Source apportionment of BrC light absorption coefficient at 365 nm
In this study, the source apportionment of BrC was conducted using the PMF coupled with ANN MLP methods by following the steps: (1) identification and quantification of the major sources of PM2.5 for the six cities using PMF (the United States Environmental Protection Agency, PMF 5.0) and (2) production of a predictive model by ANN MLP for one variable (BrC babs365) based on the values of the input variables (PM2.5 sources daily contributions). PMF is a bilinear factor model that has been widely used in source apportionment studies (Cao et al., 2012; Lei et al., 2018; Li et al., 2021; Shen et al., 2010; Tao et al., 2017). In the present study, water-soluble inorganic ions (Na+, NH, K+, Mg2+, Ca2+, NO, SO, and Cl−) and carbon fractions (OC1, OC2, OC3, OC4, EC1, and EC2) were used as data inputs for PMF. The PMF model was run multiple times, extracting four to six factors. A more detailed description of these items can be found in the article by Lei et al. (2019). Subsequently, an MLP model was constructed. The model was developed using IBM SPSS Statistics for Windows version 23 (IBM Corp., Armonk, NY, USA). The detail information of the ANN MLP model construction and training is described in Sect. S2. After ANN MLP model training, the obtained MLP model was applied to a set of virtual datasets. Each virtual dataset consists of each source with the same mass contribution (from PMF analysis) as the original dataset but with one source set to zero. The BrC babs365 contribution for a specific source was obtained by subtracting the BrC babs365 simulation value obtained using the virtual dataset from the BrC babs365 simulation value obtained using the original MLP model, which contains all the source contributions (Borlaza et al., 2021a).
3.1 General description of PM2.5 and its chemical species in six megacities
As presented in Table S2, the PM2.5 concentrations in the six cities ranged from 9.9 to 241.9 µg m−3 and exhibited a significant spatial variation (p<0.01), indicating the complexity of air pollution and spatial differences in air pollution levels in China. HrB had the highest average PM2.5 concentration (85.5±43.9 µg m−3), which exceeded National Air Quality Standard grade-II (24 h average: 75 µg m−3) and was 1.5, 1.1, 1.2, 2.0, and 1.3 times higher than those recorded in BJ, XA, CD, GZ, and WH, respectively. This phenomenon indicates that PM2.5 pollution is still a major challenge in China, particularly in northern China.
The average concentration of OC, a major chemical component of PM2.5, ranged from 5.6 to 19.4 µg m−3 in six megacities; these cities can be arranged (in descending order) as follows in terms of the average OC concentration: HrB > XA > BJ > WH > GZ > CD (Table S2). Similar to the PM2.5 trend, the average OC concentration in the northern cities (15.5±7.9 µg m−3) was higher than that in the southern cities (9.2±4.6 µg m−3), which can be attributed to substantial emissions from residential heating (i.e., coal and biomass combustion) in winter in northern China (Zhang et al., 2021). In addition, these residential fuels can emit an abundance of OC emissions (Lei et al., 2018; Sun et al., 2017). To assess the sources of atmospheric BrC, we estimated the concentrations of primary OC (POC) and secondary OC (SOC) by using the EC tracer method (Ram and Sarin, 2011). The detailed calculation method was described in Sect. S3. As presented in Table S2, the average SOC concentrations throughout the measurement period ranged from 1.0 (CD) to 9.2 µg m−3 (HrB), and the fractional contributions of SOC to OC varied from 22.6 % to 66.6 %. The average POC concentrations ranged from 4.0 (GZ) to 10.2 µg m−3 (HrB), and POC constituted 34.4 %–77.4 % of the total OC mass in the six cities. Accordingly, the SOC and POC concentrations exhibited typical spatial fluctuations, which were consistent with the fluctuations of the PM2.5 and total OC concentrations. These results reveal that primary emissions usually dominated secondary formation processes, especially in the northern cities.
3.2 Light absorption properties of BrC
As plotted in Fig. 2, the light absorption coefficient (babs, M m−1) values for BrC exhibited significant spatial variations across the six cities (1.7–64.1 M m−1; p<0.01). We executed Student t test at the 95 % confidence level and observed that HrB had the highest average BrC babs365 value (29.3±14.2 M m−1), followed by BJ (11.4±3.9 M m−1), WH (10.0±3.2 M m−1), XA (8.3±2.4 M m−1), CD (5.6±2.7 M m−1), and GZ (4.3±1.4 M m−1). The average BrC babs365 value in the northern cities was 15.7±12.3 M m−1, which was 2.5 times higher than that in the southern cities (p<0.01). The large variation in the measured BrC babs365 values in these megacities was observed, which reflected that the light absorption of BrC was heavily affected by chromophore sources (Huang et al., 2018; Soleimanian et al., 2020), aging during atmospheric transportation (Lambe et al., 2013), and meteorological conditions (Li et al., 2021). Light-absorbing carbonaceous aerosols were believed to be responsible for the considerable absorption of light in the atmosphere (Xie et al., 2020). As presented in Fig. S2, we observed positive correlations between BrC babs365 and POC in the six cities (r range: 0.61–0.92). Similar correlations were observed between BrC babs365 and SOC (r range: 0.51–0.80), indicating that the sources of atmospheric BrC in the six cities were quite complex. Apart from primary emissions, secondary formation processes also seemed to have a considerable contribution to BrC in these cities. Biomass burning was revealed to be the dominant source of BrC in these cities during winter (Cheng et al., 2016; Shen et al., 2017; Sun et al., 2017; Cheng et al., 2022). Furthermore, we observed high correlations (r range: 0.69–0.92) between BrC babs365 and K+, which is commonly regarded as a tracer of biomass burning (Shen et al., 2010), in HrB, BJ, XA, and WH (Fig. S3). This evidence supports the aforementioned findings that emissions from biomass burning might be the major BrC source in winter in these cities. For the southern cities CD and GZ, the low BrC babs365 values (1.7–11.5 M m−1) are of the same order of magnitude as those reported previously in Nanjing (3.3–13 M m−1; Chen et al., 2019, 2018), Seoul (0.9–7.3 M m−1; Kim et al., 2016), and Hong Kong (4.8–10.6 M m−1; Zhang et al., 2020). The aging or oxidation of aerosols was confirmed to be the major source of BrC in these regions, indicating that secondary aerosols are likely a major source of winter BrC in CD and GZ.
The mass absorption efficiency (MAE, m2 g−1) is a key parameter for describing the light absorption ability of atmospheric BrC (Li et al., 2021; Peng et al., 2020). Figure 2 illustrated the average MAE values measured at 365 nm (BrC MAE365) in the six cities; compared with the value measured in CD (0.37±0.18 m2 g−1), those measured in the other five cities were 1.1–3.3 times higher. These cities can be arranged as follows (in descending order) in terms of the measured BrC MAE365 values: HrB > BJ > WH > XA > GZ > CD. These differences in BrC MAE365 values can be attributed to the variance of the light absorption capacity of BrC in different megacities. The average BrC MAE365 values measured in BJ, HrB, XA, and WH (range: 0.68–1.21 m2 g−1) were within the MAE ranges of biomass burning, such as, the average MAE365 measured for BrC were 0.97±0.26 m2 g−1 for wood burning (Du et al., 2014), 1.05±0.08 m2 g−1 for corn stalk combustion (Du et al., 2014), and 1.28±0.12 m2 g−1 for wheat stubble burning (Xie et al., 2017; Lei et al., 2018), indicating that biomass burning may be a major source of winter BrC in these cities. Biomass burning is commonly regarded as the main emission source for BrC, which has a high absorption capacity, as indicated by field observations and model predictions (Desyaterik et al., 2013; Feng et al., 2013; Lei et al., 2018). Notably, the MAE365 values derived for BrC emitted from primary fossil fuel combustion are similar to those derived for biomass burning (Yan et al., 2017); for example, former studies have revealed that the BrC MAE365 values produced by primary emissions from residential coal combustion were in the range of 0.30–1.51 m2 g−1 (Ni et al., 2021; Yan et al., 2017). Therefore, coal combustion may also be a potential source of BrC in these cities. By contrast, we observed lower average BrC MAE365 values in GZ and CD (range: 0.37–0.39 m2 g−1). Previous studies have revealed relatively low BrC MAE values from motor vehicle emissions, including gasoline vehicle emissions (0.62±0.76 m2 g−1; Xie et al., 2017) and motorcycle emissions (0.20±0.08 m2 g−1; Du et al., 2014). These findings suggest that the BrC sampled in GZ and CD mainly originated from traffic emissions. In addition, laboratory experiments in a previous study revealed that MAE365 values decreased from 1.43 to 0.11 m2 g−1 with aerosol aging, which suggests the production of SOA (Ni et al., 2021). This finding demonstrates that secondary formation processes are among the main sources of BrC in CD and GZ.
The absorption Ångström exponent (AAE) measurements at 330–550 nm represent the wavelength dependence of light absorption by BrC (Cheng et al., 2017). We observed that the average AAE values for BrC varied from 5.4 to 6.8 in the six cities (Fig. 3). In general, the AAE values obtained in this study were higher than those obtained at the Nepal Climate Observatory-Pyramid (3.7–4.0; 330–500 nm) (Kirillova et al., 2016) and in the Los Angeles Basin (4.82±0.49; 300–600 nm) (Zhang et al., 2013) and lower than those obtained at the Tibetan Plateau (8.2±1.4; 365–550 nm) (Zhu et al., 2018). Nevertheless, the values obtained in this study were comparable to those obtained in Beijing (5.3–7.3; 310–450 nm) (Cheng et al., 2016; Wu et al., 2021), Nanjing (6.7; 300–600 nm) (Chen et al., 2018), the Indo-Gangetic Plain (5.3; 300–700 nm) (Srinivas et al., 2016), New Delhi (5.1; 330–400 nm) (Kirillova et al., 2014), Seoul (5.5–5.8; 300–700 nm) (Kim et al., 2016), and Xi'an (5.3–6.1; 330–550 nm) (Huang et al., 2018). These similarities can primarily be attributed to the consistent solubility of chromophores, which are sensitive to the type of fuel used, the combustion conditions, and the solvents used (Cao et al., 2021; Huo et al., 2018). Furthermore, the AAE values obtained in this study were within the range of those reported by previous studies for coal combustion (5.5–6.4; 300–500 nm) (Ni et al., 2021), biomass burning (4.4–8.7; 300–550 nm) (Xie et al., 2017), and gasoline vehicle emissions (6.2–6.9; 300–550 nm) (Xie et al., 2017). This suggested that BrC in our study may have multiple sources. Additionally, in contrast to the trends observed for the BrC babs365 and BrC MAE365 values in the various cities, the AAE values observed in CD and GZ were higher than those observed in the other cities. A previous study reported that the AAE values for SOA were higher than those for primary organic aerosols (Saleh et al., 2013), and previous laboratory combustion experiments revealed that the aging of biomass burning aerosols generally engenders an increase in AAE values (from 6.93 to 15.59; Sengupta et al., 2018). These findings suggested that BrC in the cities in this study was also affected by secondary formation processes.
3.3 Molecular structure of BrC
In order to further explore the reasons for the differences in the optical properties of BrC among these cities, the functional groups of BrC were measured using FT-IR spectroscopy. Figure 4 illustrates the FT-IR spectra of BrC fractions within the region of 4000–400 cm−1 in the six cities. The band in the region of 400–800 cm−1 resulted from the interference of water vapor inside the instrument and thus can be ignored (Zhang et al., 2020). The broad and strong peak at 3450 cm−1 contributed to the O–H stretch of H-bonded hydroxyl groups, phenols and carboxylic groups (Fan et al., 2016; Mukherjee et al., 2020). The sharp band near 1740 cm−1 was usually assigned to the C=O bonds of ketones, quinones, and amides (Duarte et al., 2005; Kristensen et al., 2015). We also attributed the sharp and intense absorption peaks at 2850–2990 cm−1 to aliphatic asymmetric and symmetric C–H stretching vibrations (Coury and Dillner, 2008). Some bands were also displayed near 1640, 1458, and 1030 cm−1, and previous studies confirmed that these bands were generally ascribed to the C=C and C–H stretching of aromatic rings (Fan et al., 2016; Zhao et al., 2022), indicating the presence of aromatic groups. These results demonstrate the complexity of the chemical composition of BrC in the six cities, mainly containing aliphatic chains, carboxylic groups, and aromatic groups.
In contrast to these similar functional groups, the apparent differences in typical functional bands were also found among these cities. The strong band near 3130 cm−1 denoting the O–H band (Fan et al., 2016; Mukherjee et al., 2020) was only detected in XA, CD, and WH, and the same peak was observed in the spectra from the corn straw burning (Fan et al., 2016) and coal combustion (Zhang et al., 2022), which stressed the emissions of biomass burning and coal combustion with a high abundance of oxygenated phenolic compounds in these cities. Moreover, the peak at 1385 cm−1 was generally considered to be derived from the O–H bond deformation and C–O stretching of phenolic groups (Fan et al., 2016; Mukherjee et al., 2020; Zhang et al., 2020), and the same peak was observed in the FT-IR spectra of BrC samples derived from the combustion of biomass materials (Fan et al., 2016). These observations indicated the contribution of biomass burning to BrC; this was because that biomass burning can release heat-modified lignin derivatives such as aromatic phenols (e.g., syringyl and guaiacyl) (Duarte et al., 2007; Fan et al., 2016; Zhao et al., 2022). It was noted that the abundance of this peak was different among the six cities and was significantly higher in HrB, XA, and WH, which indicated biomass burning contributed differently to BrC in the six cities, and higher a contribution was occurring in HrB, XA, and WH than in other cities. Previous studies have shown that BrC from biomass burning has a high light absorption capacity (Cao et al., 2021; Desyaterik et al., 2013; Kumar et al., 2018), which supported that these cities with a higher abundance of aromatic phenol functional groups were consisted of higher BrC babs365 (range: 8.3–29.3 M m−1) and BrC MAE365 (range: 0.68–1.21 m2 g−1) values in Sect. 3.2.
Furthermore, we observed three peaks at 860, 1280–1260, and 1640 cm−1, demonstrating the presence of organic-nitrate (C–ONO2) and oxygenated phenolic groups (Day et al., 2010; Zhang et al., 2020). Previous studies have shown that the anthropogenic volatile organic compounds, sulfates, nitrates, and other acidic particle components from coal and biomass combustion may enhance the contents of these functional groups through aqueous-phase formation under high-humidity conditions (Gilardoni et al., 2016; Wang et al., 2019; Zhang et al., 2020). Therefore, the FT-IR spectra indicated that all the BrC samples from the six cities have the contribution of a secondary generation. Besides, the abundance of functional groups at these wavenumbers, especially at 1640 cm−1, was higher in CD than that in the other cities. These results might indicate that the secondary source of BrC was relatively high in CD.
3.4 Source apportionment of BrC
Considering the complexity of atmospheric processes and the correlation or nonlinear interaction between independent variables (i.e., multicomponent or multi-source interactions), we attempted to apply ANN techniques of nonlinear functions, such as an MLP model, combined with PMF analysis to predict the source contribution of allocated BrC from PM2.5 sources in this study. The PMF-apportioned source contributions to PM2.5 in the six cities are presented in Figs. S4 and S5. A good correlation was observed between the measured and PMF-reconstructed PM2.5 mass concentrations in all sites (BJ: r=0.99; HrB: r=0.90; XA: r=0.97; CD: r=0.97; GZ: r=0.94; WH: r=0.95); theoretical Qtrue and Qrobust displayed a <5 % difference, and scaled residuals of >95 % data were in the range of −3 to 3. This evidence demonstrated the validity and robustness of our PMF solutions (Borlaza et al., 2021b; Tao et al., 2021). As illustrated in Fig. S4, the first source was dominated by sulfate, OC, and EC and was considered to represent coal combustion (Huang et al., 2014). The second source comprised high concentrations of NH, NO, and SO and was considered to represent secondary formation processes (Shen et al., 2010). Furthermore, the third source comprised high loadings of K+ and was considered to represent biomass burning (Shen et al., 2010). The fourth source primarily comprised Na+, Mg2+, and Ca2+ and was thus determined to represent fugitive dust (Shakeri et al., 2016; Shen et al., 2016; Sun et al., 2019). The fifth source contained high concentrations of Mg2+, Ca2+, NO, OC, and EC and was thus identified as representing traffic-related emissions (Shakeri et al., 2016). Finally, the sixth source comprised high concentrations of OC, EC, and NO and was considered to represent vehicle emissions (Shakeri et al., 2016).
The optimal neural network model for each site was explored by changing activation function types (tan H and sigmoid) optimizing algorithms (scaled conjugate and gradient descent) and was based on the lowest root mean square error (RMSE) and the highest correlation coefficient (r) between observed and MLP-modeled values (Borlaza et al., 2021a). Although there are other architectures that are more complex for MLP models, a basic MLP architecture was considered sufficient for the input and output datasets of this study.
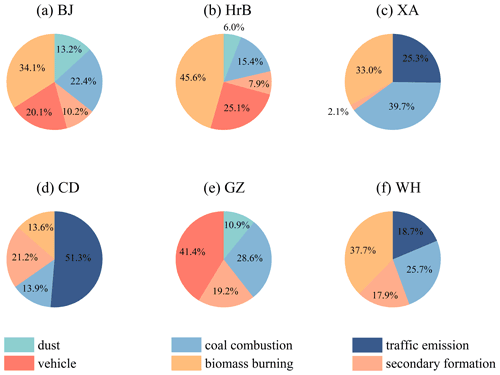
Figure 5The source contribution to BrC using multilayer perceptron neural network analysis in (a) BJ, (b) HrB, (c) XA, (d) CD, (e) GZ, and (f) WH.
Figure S6 shows the correlation between observed values and BrC babs365 predicted values from selected MLP models. The good correlation indicates the reliability of the model results. On the basis of the MLP results, we calculated the source-specific contributions to BrC in the six cities (Fig. 5). The primary sources include coal combustion, dust, vehicle, biomass burning, and traffic emissions, and their average contribution to BrC in the northern cities was 93.3 %, which was 1.2 times higher than that in the southern cities. Among these primary emissions, we noted a higher contribution of biomass burning to BrC in HrB, BJ, XA, and WH compared to other cities, which is consistent with the higher abundance of biomass burning products, such as the aromatic phenol functional groups that were found in these cities as discussed in Sect. 3.3. As supported, the BrC from biomass burning has high MAE365 values (Cao et al., 2021; Kumar et al., 2018), which can be also observed among these cities (range: 0.68–1.20 m2 g−1). In addition, we noted that the contribution of biomass burning to BrC in WH (37.7 %) was higher than that in CD (13.6 %) and GZ (0 %), which can explain the highest BrC MAE365 among the southern cities that was observed in WH as shown in Fig. 2. On average, the secondary formation source contribution to BrC in the southern cities was 19.4 %, which was 2.9 times higher than that in northern cities. Besides, the highest contribution was observed in CD with 21.2 %, followed by GZ > WH > BJ > HrB > XA. This result can be supported by the abundance of organic-nitrate functional groups, the relatively high AAE value, and the low BrC MAE365 value in CD, which were closely related to the contribution of secondary sources.
We investigated the sources and light absorption properties of BrC in wintertime in six megacities across China. Both the babs and the MAE365 of BrC at 365 nm in northern cities were approximately 2.5 and 1.8 times higher than those in the southern cities. The BrC MAE365 values measured in BJ, HrB, XA, and WH ranged from 0.68 to 1.21 m2 g−1, which were within the MAE ranges derived for biomass burning. Thus, these comparisons confirmed that emissions from biomass burning might be the major BrC source in winter in these cities. Previous studies have reported that MAE365 values decreased with aerosol aging while the AAE values of SOA were higher than those for primary organic aerosol (POA). Besides, we noticed that the average BrC MAE365 and AAE values showed different trends in the southern cities of CD and GZ; that is, the BrC MAE365 values of these two cities were lower than those of the other cities, while the AAE values were relatively high. This evidence supported that secondary formation processes were among the main sources of BrC in CD and GZ.
The chemical functional groups of BrC in the six cities mainly included aliphatic chains, carboxyl groups, and aromatic groups. However, the apparent difference in typical functional bands revealed the important contributions of primary biomass burning and coal combustion to BrC for the high abundance of oxygenated phenolic compounds in these cities, especially in HrB, XA, and WH. In contrast, the presence of organic-nitrate (C–ONO2) and oxygenated phenolic groups in the BrC molecule implied the contribution from secondary formation in the six megacities, especially in CD.
Due to the complexity of atmospheric processes, which are usually nonlinear in nature, the traditional linear-based source analytic models may be limited. Here, we used a multilayer perceptron (MLP) model based on an artificial neural network (ANN) to improve the source allocation of BrC in these cities. Source apportionment of BrC based on PMF and ANN MLP analysis revealed that primary emissions (e.g., biomass burning, coal combustion, and vehicle emissions) were key contributors to BrC and their average contribution in northern cities was about 93.3 %, which was 1.2 times higher than that in the southern cities. Secondary formation processes made a greater contribution to BrC in the southern cities (19.4 %) than the northern cities (6.7 %). The results of our work can provide a basis for the development of more effective practices to control BrC emissions at the regional level.
The key datasets are publicly available on the Zenodo data repository platform: https://doi.org/10.5281/zenodo.6790321 (Wang, 2022).
The supplement related to this article is available online at: https://doi.org/10.5194/acp-22-14893-2022-supplement.
ZS designed the study. Data analysis was done by DW, TZ and SH. DW and YL created the model. DW wrote the initial manuscript. ZS, QZ, HX, JS, JC and YL discussed results and commented on the manuscript. ZS and HX provided financial support for the project.
The contact author has declared that none of the authors has any competing interests.
Publisher's note: Copernicus Publications remains neutral with regard to jurisdictional claims in published maps and institutional affiliations.
The authors also thank Jun Tao, Renjian Zhang, Shaofei Kong, and Song Cui for their help in field sampling.
This research has been supported by the National Natural Science Foundation of China (grant no. 41877383) and the State Key Laboratory of Loess and Quaternary Geology (grant no. SKLLQG2103).
This paper was edited by Dantong Liu and reviewed by two anonymous referees.
Bao, M., Zhang, Y. L., Cao, F., Lin, Y. C., Hong, Y., Fan, M., Zhang, Y., Yang, X., and Xie, F.: Light absorption and source apportionment of water soluble humic-like substances (HULIS) in PM2.5 at Nanjing, China, Environ. Res., 206, 112554, https://doi.org/10.1016/j.envres.2021.112554, 2022.
Borlaza, L. J. S., Weber, S., Jaffrezo, J.-L., Houdier, S., Slama, R., Rieux, C., Albinet, A., Micallef, S., Trébluchon, C., and Uzu, G.: Disparities in particulate matter (PM10) origins and oxidative potential at a city scale (Grenoble, France) – Part 2: Sources of PM10 oxidative potential using multiple linear regression analysis and the predictive applicability of multilayer perceptron neural network analysis, Atmos. Chem. Phys., 21, 9719–9739, https://doi.org/10.5194/acp-21-9719-2021, 2021a.
Borlaza, L. J. S., Weber, S., Uzu, G., Jacob, V., Cañete, T., Micallef, S., Trébuchon, C., Slama, R., Favez, O., and Jaffrezo, J.-L.: Disparities in particulate matter (PM10) origins and oxidative potential at a city scale (Grenoble, France) – Part 1: Source apportionment at three neighbouring sites, Atmos. Chem. Phys., 21, 5415–5437, https://doi.org/10.5194/acp-21-5415-2021, 2021b.
Cao, J. J., Lee, S. C., Ho, K. F., Zou, S. C., Fung, K., Li, Y., Watson, J. G., and Chow, J. C.: Spatial and seasonal variations of atmospheric organic carbon and elemental carbon in Pearl River Delta Region, China, Atmos. Environ., 38, 4447–4456, https://doi.org/10.1016/j.atmosenv.2004.05.016, 2004.
Cao, J. J., Wang, Q. Y., Chow, J. C., Watson, J. G., Tie, X. X., Shen, Z. X., Wang, P., and An, Z. S.: Impacts of aerosol compositions on visibility impairment in Xi'an, China, Atmos. Environ., 59, 559–566, https://doi.org/10.1016/j.atmosenv.2012.05.036, 2012.
Cao, T., Li, M., Zou, C., Fan, X., Song, J., Jia, W., Yu, C., Yu, Z., and Peng, P.: Chemical composition, optical properties, and oxidative potential of water- and methanol-soluble organic compounds emitted from the combustion of biomass materials and coal, Atmos. Chem. Phys., 21, 13187–13205, https://doi.org/10.5194/acp-21-13187-2021, 2021.
Chen, D., Zhao, Y., Lyu, R., Wu, R., Dai, L., Zhao, Y., Chen, F., Zhang, J., Yu, H., and Guan, M.: Seasonal and spatial variations of optical properties of light absorbing carbon and its influencing factors in a typical polluted city in Yangtze River Delta, China, Atmos. Environ., 199, 45–54, https://doi.org/10.1016/j.atmosenv.2018.11.022, 2019.
Chen, Y., Ge, X., Chen, H., Xie, X., Chen, Y., Wang, J., Ye, Z., Bao, M., Zhang, Y., and Chen, M.: Seasonal light absorption properties of water-soluble brown carbon in atmospheric fine particles in Nanjing, China, Atmos. Environ., 187, 230–240, https://doi.org/10.1016/j.atmosenv.2018.06.002, 2018.
Cheng, Y., He, K. B., Du, Z. Y., Engling, G., Liu, J. M., Ma, Y. L., Zheng, M., and Weber, R. J.: The characteristics of brown carbon aerosol during winter in Beijing, Atmos. Environ., 127, 355–364, https://doi.org/10.1016/j.atmosenv.2015.12.035, 2016.
Cheng, Y., He, K. B., Engling, G., Weber, R., Liu, J. M., Du, Z. Y., and Dong, S. P.: Brown and black carbon in Beijing aerosol: Implications for the effects of brown coating on light absorption by black carbon, Sci. Total. Environ., 599–600, 1047–1055, https://doi.org/10.1016/j.scitotenv.2017.05.061, 2017.
Cheng, Y., Cao, X. B., Liu, J. M., Yu, Q. Q., Wang, P., Yan, C. Q., Du, Z. Y., Liang, L. L., Zhang, Q., and He, K. B.: Primary nature of brown carbon absorption in a frigid atmosphere with strong haze chemistry, Environ. Res., 204, 112324, https://doi.org/10.1016/j.envres.2021.112324, 2022.
Chung, C. E., Kim, S.-W., Lee, M., Yoon, S.-C., and Lee, S.: Carbonaceous aerosol AAE inferred from in-situ aerosol measurements at the Gosan ABC super site, and the implications for brown carbon aerosol, Atmos. Chem. Phys., 12, 6173–6184, https://doi.org/10.5194/acp-12-6173-2012, 2012.
Coury, C. and Dillner, A. M.: A method to quantify organic functional groups and inorganic compounds in ambient aerosols using attenuated total reflectance FTIR spectroscopy and multivariate chemometric techniques, Atmos. Environ., 42, 5923–5932, https://doi.org/10.1016/j.atmosenv.2008.03.026, 2008.
Day, D. A., Liu, S., Russell, L. M., and Ziemann, P. J.: Organonitrate group concentrations in submicron particles with high nitrate and organic fractions in coastal southern California, Atmos. Environ., 44, 1970–1979, https://doi.org/10.1016/j.atmosenv.2010.02.045, 2010.
Desyaterik, Y., Sun, Y., Shen, X., Lee, T., Wang, X., Wang, T., and Collett, J. L.: Speciation of “brown” carbon in cloud water impacted by agricultural biomass burning in eastern China, J. Geophys. Res.-Atmos., 118, 7389–7399, https://doi.org/10.1002/jgrd.50561, 2013.
Devi, J. J., Bergin, M. H., McKenzie, M., Schauer, J. J., and Weber, R. J.: Contribution of particulate brown carbon to light absorption in the rural and urban Southeast US, Atmos. Environ., 136, 95–104, https://doi.org/10.1016/j.atmosenv.2016.04.011, 2016.
Du, Z., He, K., Cheng, Y., Duan, F., Ma, Y., Liu, J., Zhang, X., Zheng, M., and Weber, R.: A yearlong study of water-soluble organic carbon in Beijing II: Light absorption properties, Atmos. Environ., 89, 235–241, https://doi.org/10.1016/j.atmosenv.2014.02.022, 2014.
Duarte, R. M. B. O., Pio, C. A., and Duarte, A. C.: Spectroscopic study of the water-soluble organic matter isolated from atmospheric aerosols collected under different atmospheric conditions, Anal. Chim. Acta, 530, 7–14, https://doi.org/10.1016/j.aca.2004.08.049, 2005.
Duarte, R. M. B. O., Santos, E. B. H., Pio, C. A., and Duarte, A. C.: Comparison of structural features of water-soluble organic matter from atmospheric aerosols with those of aquatic humic substances, Atmos. Environ., 41, 8100–8113, https://doi.org/10.1016/j.atmosenv.2007.06.034, 2007.
Elangasinghe, M. A., Singhal, N., Dirks, K. N., and Salmond, J. A.: Development of an ANN–based air pollution forecasting system with explicit knowledge through sensitivity analysis, Atmos. Pollut. Res., 5, 696–708, https://doi.org/10.5094/APR.2014.079, 2014.
Fan, X., Wei, S., Zhu, M., Song, J., and Peng, P.: Comprehensive characterization of humic-like substances in smoke PM2.5 emitted from the combustion of biomass materials and fossil fuels, Atmos. Chem. Phys., 16, 13321–13340, https://doi.org/10.5194/acp-16-13321-2016, 2016.
Feng, Y., Ramanathan, V., and Kotamarthi, V. R.: Brown carbon: a significant atmospheric absorber of solar radiation?, Atmos. Chem. Phys., 13, 8607–8621, https://doi.org/10.5194/acp-13-8607-2013, 2013.
Gilardoni, S., Massoli, P., Paglione, M., Giulianelli, L., Carbone, C., Rinaldi, M., Decesari, S., Sandrini, S., Costabile, F., Gobbi, G. P., Pietrogrande, M. C., Visentin, M., Scotto, F., Fuzzi, S., and Facchini, M. C.: Direct observation of aqueous secondary organic aerosol from biomass-burning emissions, P. Natl. Acad. Sci. USA., 113, 10013–10018, https://doi.org/10.1073/pnas.1602212113, 2016.
Huang, R. J., Yang, L., Cao, J., Chen, Y., Chen, Q., Li, Y., Duan, J., Zhu, C., Dai, W., Wang, K., Lin, C., Ni, H., Corbin, J. C., Wu, Y., Zhang, R., Tie, X., Hoffmann, T., O'Dowd, C., and Dusek, U.: Brown Carbon Aerosol in Urban Xi'an, Northwest China: The composition and light absorption properties, Environ. Sci. Technol., 52, 6825–6833, https://doi.org/10.1021/acs.est.8b02386, 2018.
Huang, X. H. H., Bian, Q. J., Louie, P. K. K., and Yu, J. Z.: Contributions of vehicular carbonaceous aerosols to PM2.5 in a roadside environment in Hong Kong, Atmos. Chem. Phys., 14, 9279–9293, https://doi.org/10.5194/acp-14-9279-2014, 2014.
Huo, Y., Li, M., Jiang, M., and Qi, W.: Light absorption properties of HULIS in primary particulate matter produced by crop straw combustion under different moisture contents and stacking modes, Atmos. Environ., 191, 490–499, https://doi.org/10.1016/j.atmosenv.2018.08.038, 2018.
Jo, D. S., Park, R. J., Lee, S., Kim, S.-W., and Zhang, X.: A global simulation of brown carbon: implications for photochemistry and direct radiative effect, Atmos. Chem. Phys., 16, 3413–3432, https://doi.org/10.5194/acp-16-3413-2016, 2016.
Kim, H., Kim, J. Y., Jin, H. C., Lee, J. Y., and Lee, S. P.: Seasonal variations in the light-absorbing properties of water-soluble and insoluble organic aerosols in Seoul, Korea, Atmos. Environ., 129, 234–242, https://doi.org/10.1016/j.atmosenv.2016.01.042, 2016.
Kirchstetter, T. W., Novakov, T., and Hobbs, P. V.: Evidence that the spectral dependence of light absorption by aerosols is affected by organic carbon, J. Geophys. Res.-Atmos., 109, D21208, https://doi.org/10.1029/2004JD004999, 2004.
Kirillova, E. N., Andersson, A., Tiwari, S., Srivastava, A. K., Bisht, D. S., and Gustafsson, Ö.: Water-soluble organic carbon aerosols during a full New Delhi winter: Isotope-based source apportionment and optical properties, J. Geophys. Res.-Atmos., 119, 3476–3485, https://doi.org/10.1002/2013JD020041, 2014.
Kirillova, E. N., Marinoni, A., Bonasoni, P., Vuillermoz, E., Facchini, M. C., Fuzzi, S., and Decesari, S.: Light absorption properties of brown carbon in the high Himalayas, J. Geophys. Res.-Atmos., 121, 9621–9639, https://doi.org/10.1002/2016JD025030, 2016.
Kristensen, T. B., Du, L., Nguyen, Q. T., Nøjgaard, J. K., Koch, C. B., Nielsen, O. F., Hallar, A. G., Lowenthal, D. H., Nekat, B., Pinxteren, D. V., Herrmann, H., Glasius, M., Kjaergaard, H. G., and Bilde, M.: Chemical properties of HULIS from three different environments, J. Atmos. Chem., 72, 65–80, https://doi.org/10.1007/s10874-015-9302-8, 2015.
Kumar, N. K., Corbin, J. C., Bruns, E. A., Massabó, D., Slowik, J. G., Drinovec, L., Močnik, G., Prati, P., Vlachou, A., Baltensperger, U., Gysel, M., El-Haddad, I., and Prévôt, A. S. H.: Production of particulate brown carbon during atmospheric aging of residential wood-burning emissions, Atmos. Chem. Phys., 18, 17843–17861, https://doi.org/10.5194/acp-18-17843-2018, 2018.
Lambe, A. T., Cappa, C. D., Massoli, P., Onasch, T. B., Forestieri, S. D., Martin, A. T., Cummings, M. J., Croasdale, D. R., Brune, W. H., Worsnop, D. R., and Davidovits, P.: Relationship between oxidation level and optical properties of secondary organic aerosol, Environ. Sci. Technol., 47, 6349–6357, https://doi.org/10.1021/es401043j, 2013.
Laskin, A., Laskin, J., and Nizkorodov, S. A.: Chemistry of atmospheric brown carbon, Chem. Rev., 115, 4335–4382, https://doi.org/10.1021/cr5006167, 2015.
Lei, Y., Shen, Z., Wang, Q., Zhang, T., Cao, J., Sun, J., Zhang, Q., Wang, L., Xu, H., Tian, J., and Wu, J.: Optical characteristics and source apportionment of brown carbon in winter PM2.5 over Yulin in Northern China, Atmos. Res., 213, 27–33, https://doi.org/10.1016/j.atmosres.2018.05.018, 2018.
Lei, Y., Shen, Z., Zhang, T., Lu, D., Zeng, Y., Zhang, Q., Xu, H., Bei, N., Wang, X., and Cao, J.: High time resolution observation of PM2.5 Brown carbon over Xi'an in northwestern China: Seasonal variation and source apportionment, Chemosphere, 237, 124530, https://doi.org/10.1016/j.chemosphere.2019.124530.124530, 2019.
Li, X., Zhao, Q., Yang, Y., Zhao, Z., Liu, Z., Wen, T., Hu, B., Wang, Y., Wang, L., and Wang, G.: Composition and sources of brown carbon aerosols in megacity Beijing during the winter of 2016, Atmos. Res., 262, https://doi.org/10.1016/j.atmosres.2021.105773, 2021.
Ma, Y., Ye, J., Xin, J., Zhang, W., Vilà-Guerau de Arellano, J., Wang, S., Zhao, D., Dai, L., Ma, Y., Wu, X., Xia, X., Tang, G., Wang, Y., Shen, P., Lei, Y., and Martin, S. T.: The stove, dome, and umbrella effects of atmospheric aerosol on the development of the planetary boundary layer in hazy regions, Geophys. Res. Lett., 47, e2020GL087373, https://doi.org/10.1029/2020GL087373, 2020.
Mo, Y., Li, J., Cheng, Z., Zhong, G., Zhu, S., Tian, C., Chen, Y., and Zhang, G.: Dual carbon isotope-based source apportionment and light absorption properties of water-soluble organic carbon in PM2.5 over China, J. Geophys. Res.-Atmos., 126, e2020JD033920, https://doi.org/10.1029/2020JD033920, 2021.
Mukherjee, A., Dey, S., Rana, A., Jia, S., Banerjee, S., and Sarkar, S.: Sources and atmospheric processing of brown carbon and HULIS in the Indo-Gangetic Plain: Insights from compositional analysis, Environ. Pollut., 267, 115440, https://doi.org/10.1016/j.envpol.2020.115440, 2020.
Ni, H., Huang, R. J., Pieber, S. M., Corbin, J. C., Stefenelli, G., Pospisilova, V., Klein, F., Gysel-Beer, M., Yang, L., Baltensperger, U., Haddad, I. E., Slowik, J. G., Cao, J., Prevot, A. S. H., and Dusek, U.: Brown carbon in primary and aged coal combustion emission, Environ. Sci. Technol., 55, 5701–5710, https://doi.org/10.1021/acs.est.0c08084, 2021.
Peng, C., Yang, F., Tian, M., Shi, G., Li, L., Huang, R. J., Yao, X., Luo, B., Zhai, C., and Chen, Y.: Brown carbon aerosol in two megacities in the Sichuan Basin of southwestern China: Light absorption properties and implications, Sci. Total. Environ., 719, 137483, https://doi.org/10.1016/j.scitotenv.2020.137483, 2020.
Ram, K. and Sarin, M. M.: Day–night variability of EC, OC, WSOC and inorganic ions in urban environment of Indo-Gangetic Plain: Implications to secondary aerosol formation, Atmos. Environ., 45, 460–468, https://doi.org/10.1016/j.atmosenv.2010.09.055, 2011.
Saleh, R., Hennigan, C. J., McMeeking, G. R., Chuang, W. K., Robinson, E. S., Coe, H., Donahue, N. M., and Robinson, A. L.: Absorptivity of brown carbon in fresh and photo-chemically aged biomass-burning emissions, Atmos. Chem. Phys., 13, 7683–7693, https://doi.org/10.5194/acp-13-7683-2013, 2013.
Sengupta, D., Samburova, V., Bhattarai, C., Kirillova, E., Mazzoleni, L., Iaukea-Lum, M., Watts, A., Moosmüller, H., and Khlystov, A.: Light absorption by polar and non-polar aerosol compounds from laboratory biomass combustion, Atmos. Chem. Phys., 18, 10849–10867, https://doi.org/10.5194/acp-18-10849-2018, 2018.
Shakeri, A., Madadi, M., and Mehrabi, B.: Health risk assessment and source apportionment of PAHs in industrial and bitumen contaminated soils of Kermanshah province; NW Iran, Toxicology and Environmental Health Sciences, 8, 201–212, https://doi.org/10.1007/s13530-016-0277-x, 2016.
Shen, Z., Arimoto, R., Cao, J., Zhang, R., Li, X., Du, N., Okuda, T., Nakao, S., and Tanaka, S.: Seasonal variations and evidence for the effectiveness of pollution controls on water-soluble inorganic species in total suspended particulates and fine particulate matter from Xi'an, China, J. Air. Waste. Manag. Assoc., 58, 1560–1570, https://doi.org/10.3155/1047-3289.58.12.1560, 2008.
Shen, Z., Cao, J., Arimoto, R., Han, Y., Zhu, C., Tian, J., and Liu, S.: Chemical Characteristics of Fine Particles (PM1) from Xi'an, China, Aerosol. Sci. Tech., 44, 461–472, https://doi.org/10.1080/02786821003738908, 2010.
Shen, Z., Sun, J., Cao, J., Zhang, L., Zhang, Q., Lei, Y., Gao, J., Huang, R. J., Liu, S., Huang, Y., Zhu, C., Xu, H., Zheng, C., Liu, P., and Xue, Z.: Chemical profiles of urban fugitive dust PM2.5 samples in Northern Chinese cities, Sci. Total. Environ., 569–570, 619–626, https://doi.org/10.1016/j.scitotenv.2016.06.156, 2016.
Shen, Z., Zhang, Q., Cao, J., Zhang, L., Lei, Y., Huang, Y., Huang, R. J., Gao, J., Zhao, Z., Zhu, C., Yin, X., Zheng, C., Xu, H., and Liu, S.: Optical properties and possible sources of brown carbon in PM2.5 over Xi'an, China, Atmos. Environ., 150, 322–330, https://doi.org/10.1016/j.atmosenv.2016.11.024, 2017.
Soleimanian, E., Mousavi, A., Taghvaee, S., Shafer, M. M., and Sioutas, C.: Impact of secondary and primary particulate matter (PM) sources on the enhanced light absorption by brown carbon (BrC) particles in central Los Angeles, Sci. Total. Environ., 705, 135902, https://doi.org/10.1016/j.scitotenv.2019.135902, 2020.
Sreekanth, V., Niranjan, K., and Madhavan, B. L.: Radiative forcing of black carbon over eastern India, Geophys. Res. Lett., 34, L17818, https://doi.org/10.1029/2007GL030377, 2007.
Srinivas, B., Rastogi, N., Sarin, M. M., Singh, A., and Singh, D.: Mass absorption efficiency of light absorbing organic aerosols from source region of paddy-residue burning emissions in the Indo-Gangetic Plain, Atmos. Environ., 125, 360–370, https://doi.org/10.1016/j.atmosenv.2015.07.017, 2016.
Sun, J., Shen, Z., Cao, J., Zhang, L., Wu, T., Zhang, Q., Yin, X., Lei, Y., Huang, Y., Huang, R. J., Liu, S., Han, Y., Xu, H., Zheng, C., and Liu, P.: Particulate matters emitted from maize straw burning for winter heating in rural areas in Guanzhong Plain, China: Current emission and future reduction, Atmos. Res., 184, 66–76, https://doi.org/10.1016/j.atmosres.2016.10.006, 2017.
Sun, J., Shen, Z., Zhang, L., Lei, Y., Gong, X., Zhang, Q., Zhang, T., Xu, H., Cui, S., Wang, Q., Cao, J., Tao, J., Zhang, N., and Zhang, R.: Chemical source profiles of urban fugitive dust PM2.5 samples from 21 cities across China, Sci. Total. Environ., 649, 1045–1053, https://doi.org/10.1016/j.scitotenv.2018.08.374, 2019.
Tao, J., Zhang, L., Cao, J., Zhong, L., Chen, D., Yang, Y., Chen, D., Chen, L., Zhang, Z., Wu, Y., Xia, Y., Ye, S., and Zhang, R.: Source apportionment of PM2.5 at urban and suburban areas of the Pearl River Delta region, south China - With emphasis on ship emissions, Sci. Total. Environ., 574, 1559–1570, https://doi.org/10.1016/j.scitotenv.2016.08.175, 2017.
Tao, Y., Sun, N., Li, X., Zhao, Z., Ma, S., Huang, H., Ye, Z., and Ge, X.: Chemical and Optical Characteristics and Sources of PM2.5 Humic-Like Substances at Industrial and Suburban Sites in Changzhou, China, Atmosphere, 12, 276, https://doi.org/10.3390/atmos12020276, 2021.
Wang, D.: Winter brown carbon over six China's megacities: Light absorption, molecular characterization, and improved source apportionment revealed by multilayer perceptron neural network, Zenodo [data set], https://doi.org/10.5281/zenodo.6790321, 2022.
Wang, Y., Hu, M., Wang, Y., Zheng, J., Shang, D., Yang, Y., Liu, Y., Li, X., Tang, R., Zhu, W., Du, Z., Wu, Y., Guo, S., Wu, Z., Lou, S., Hallquist, M., and Yu, J. Z.: The formation of nitro-aromatic compounds under high NOx and anthropogenic VOC conditions in urban Beijing, China, Atmos. Chem. Phys., 19, 7649–7665, https://doi.org/10.5194/acp-19-7649-2019, 2019.
Wu, C., Wang, G., Li, J., Li, J., Cao, C., Ge, S., Xie, Y., Chen, J., Li, X., Xue, G., Wang, X., Zhao, Z., and Cao, F.: The characteristics of atmospheric brown carbon in Xi'an, inland China: sources, size distributions and optical properties, Atmos. Chem. Phys., 20, 2017–2030, https://doi.org/10.5194/acp-20-2017-2020, 2020.
Wu, Y., Li, J., Jiang, C., Xia, Y., Tao, J., Tian, P., Zhou, C., Wang, C., Xia, X., Huang, R. J., and Zhang, R.: Spectral absorption properties of organic carbon aerosol during a polluted winter in Beijing, China, Sci. Total. Environ., 755, 142600, https://doi.org/10.1016/j.scitotenv.2020.142600, 2021.
Xie, M., Hays, M. D., and Holder, A. L.: Light-absorbing organic carbon from prescribed and laboratory biomass burning and gasoline vehicle emissions, Sci. Rep.-UK, 7, 7318, https://doi.org/10.1038/s41598-017-06981-8, 2017.
Xie, X., Chen, Y., Nie, D., Liu, Y., Liu, Y., Lei, R., Zhao, X., Li, H., and Ge, X.: Light-absorbing and fluorescent properties of atmospheric brown carbon: A case study in Nanjing, China, Chemosphere, 251, 126350, https://doi.org/10.1016/j.chemosphere.2020.126350, 2020.
Yan, C., Zheng, M., Bosch, C., Andersson, A., Desyaterik, Y., Sullivan, A. P., Collett, J. L., Zhao, B., Wang, S., He, K., and Gustafsson, O.: Important fossil source contribution to brown carbon in Beijing during winter, Sci. Rep.-UK, 7, 43182, https://doi.org/10.1038/srep43182, 2017.
Yan, J., Wang, X., Gong, P., Wang, C., and Cong, Z.: Review of brown carbon aerosols: Recent progress and perspectives, Sci. Total. Environ., 634, 1475–1485, https://doi.org/10.1016/j.scitotenv.2018.04.083, 2018.
Yuan, W., Huang, R.-J., Yang, L., Guo, J., Chen, Z., Duan, J., Wang, T., Ni, H., Han, Y., Li, Y., Chen, Q., Chen, Y., Hoffmann, T., and O'Dowd, C.: Characterization of the light-absorbing properties, chromophore composition and sources of brown carbon aerosol in Xi'an, northwestern China, Atmos. Chem. Phys., 20, 5129–5144, https://doi.org/10.5194/acp-20-5129-2020, 2020.
Zhang, Q., Shen, Z., Zhang, L., Zeng, Y., Ning, Z., Zhang, T., Lei, Y., Wang, Q., Li, G., Sun, J., Westerdahl, D., Xu, H., and Cao, J.: Investigation of primary and secondary particulate brown carbon in two Chinese cities of Xi'an and Hong Kong in wintertime, Environ. Sci. Technol., 54, 3803–3813, https://doi.org/10.1021/acs.est.9b05332, 2020.
Zhang, Q., Li, Z., Shen, Z., Zhang, T., Zhang, Y., Sun, J., Zeng, Y., Xu, H., Wang, Q., Hang Ho, S. S., and Cao, J.: Source profiles of molecular structure and light absorption of PM2.5 brown carbon from residential coal combustion emission in Northwestern China, Environ. Pollut., 299, 118866, https://doi.org/10.1016/j.envpol.2022.118866, 2022.
Zhang, T., Shen, Z., Zeng, Y., Cheng, C., Wang, D., Zhang, Q., Lei, Y., Zhang, Y., Sun, J., Xu, H., Ho, S. S. H., and Cao, J.: Light absorption properties and molecular profiles of HULIS in PM2.5 emitted from biomass burning in traditional “Heated Kang” in Northwest China, Sci. Total. Environ., 776, 146014, https://doi.org/10.1016/j.scitotenv.2021.146014, 2021.
Zhang, X., Lin, Y.-H., Surratt, J. D., Zotter, P., Prévôt, A. S. H., and Weber, R. J.: Light-absorbing soluble organic aerosol in Los Angeles and Atlanta: A contrast in secondary organic aerosol, Geophys. Res. Lett., 38, L21810, https://doi.org/10.1029/2011GL049385, 2011.
Zhang, X., Lin, Y. H., Surratt, J. D., and Weber, R. J.: Sources, composition and absorption Angstrom exponent of light-absorbing organic components in aerosol extracts from the Los Angeles Basin, Environ. Sci. Technol., 47, 3685–3693, https://doi.org/10.1021/es305047b, 2013.
Zhao, R., Zhang, Q., Xu, X., Wang, W., Zhao, W., Zhang, W., and Zhang, Y.: Light absorption properties and molecular compositions of water-soluble and methanol-soluble organic carbon emitted from wood pyrolysis and combustion, Sci. Total. Environ., 809, 151136, https://doi.org/10.1016/j.scitotenv.2021.151136, 2022.
Zhu, C. S., Cao, J. J., Huang, R. J., Shen, Z. X., Wang, Q. Y., and Zhang, N. N.: Light absorption properties of brown carbon over the southeastern Tibetan Plateau, Sci. Total. Environ., 625, 246–251, https://doi.org/10.1016/j.scitotenv.2017.12.183, 2018.