the Creative Commons Attribution 4.0 License.
the Creative Commons Attribution 4.0 License.
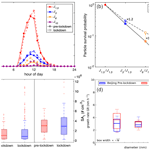
The effect of COVID-19 restrictions on atmospheric new particle formation in Beijing
Yicheng Shen
Dominik Stolzenburg
Lubna Dada
Ximeng Qi
Simo Hakala
Anu-Maija Sundström
Yishuo Guo
Antti Lipponen
Tom V. Kokkonen
Jenni Kontkanen
Runlong Cai
Jing Cai
Tommy Chan
Liangduo Chen
Chenjuan Deng
Xiaolong Fan
Xu-Cheng He
Juha Kangasluoma
Joni Kujansuu
Mona Kurppa
Chang Li
Yiran Li
Zhuohui Lin
Yiliang Liu
Yuliang Liu
Jouni Pulliainen
Xiaohui Qiao
Yonghong Wang
Yifan Wen
Gan Yang
Rujing Yin
Gen Zhang
Shaojun Zhang
Feixue Zheng
Ying Zhou
Antti Arola
Johanna Tamminen
Pauli Paasonen
Neil M. Donahue
Yongchun Liu
Federico Bianchi
Kaspar R. Daellenbach
Douglas R. Worsnop
Veli-Matti Kerminen
Tuukka Petäjä
Jingkun Jiang
Markku Kulmala
During the COVID-19 lockdown, the dramatic reduction of anthropogenic emissions provided a unique opportunity to investigate the effects of reduced anthropogenic activity and primary emissions on atmospheric chemical processes and the consequent formation of secondary pollutants. Here, we utilize comprehensive observations to examine the response of atmospheric new particle formation (NPF) to the changes in the atmospheric chemical cocktail. We find that the main clustering process was unaffected by the drastically reduced traffic emissions, and the formation rate of 1.5 nm particles remained unaltered. However, particle survival probability was enhanced due to an increased particle growth rate (GR) during the lockdown period, explaining the enhanced NPF activity in earlier studies. For GR at 1.5–3 nm, sulfuric acid (SA) was the main contributor at high temperatures, whilst there were unaccounted contributing vapors at low temperatures. For GR at 3–7 and 7–15 nm, oxygenated organic molecules (OOMs) played a major role. Surprisingly, OOM composition and volatility were insensitive to the large change of atmospheric NOx concentration; instead the associated high particle growth rates and high OOM concentration during the lockdown period were mostly caused by the enhanced atmospheric oxidative capacity. Overall, our findings suggest a limited role of traffic emissions in NPF.
- Article
(2704 KB) - Full-text XML
-
Supplement
(5844 KB) - BibTeX
- EndNote
The COVID-19 pandemic has led to the death of more than 6.4 million individuals globally (WHO 2020, https://covid19.who.int/, last access: 14 August 2022). Restrictions on population movement (lockdowns) worldwide led to arguably the most significant reduction of primary anthropogenic emissions in recent history. NOx concentrations declined on average by about 50 %–60 % in several European, South American, Indian, and Chinese cities (Sicard et al., 2020; Krecl et al., 2020; Shi and Brasseur, 2020; Agarwal et al., 2020), and mixing ratios of other primary pollutants, such as black carbon (BC), carbon monoxide (CO), sulfur dioxide (SO2), and volatile organic compounds (VOCs), were also reduced to varying degrees (Bao and Zhang, 2020; Chu et al., 2021; X. Shen et al., 2021; Xing et al., 2020; Pei et al., 2020).
The reductions of primary emissions mitigated particulate pollution and improved air quality in many countries around the globe (Sicard et al., 2020; Krecl et al., 2020; Agarwal et al., 2020; Ciarelli et al., 2021), including many Chinese cities (P. Wang et al., 2020; Huang et al., 2020; Le et al., 2020). However, the reduction of PM2.5 was considerably weaker than those of the primary pollutants, and in some cities such as Beijing, the PM2.5 concentrations even increased after the lockdown policy was imposed (Huang et al., 2020). This persistent particulate pollution has been attributed to unfavorable meteorology, such as stagnant meteorological conditions and high relative humidity (RH) (Le et al., 2020; P. Wang et al., 2020), and to enhanced atmospheric oxidative capacity caused by increased O3 and NO3 radical formation (Huang et al., 2020; Le et al., 2020). To date, few studies have focused on either atmospheric new particle formation or the overall particle number size distribution (J. Shen et al., 2021; X. Shen et al., 2021) during the lockdown period, although NPF has been shown to enhance haze formation (Guo et al., 2014; Kulmala et al., 2021), and the particle number size distribution is known to influence the health effect of particles (Harrison et al., 2010).
NPF contains two consecutive stages: formation of particles via molecular clustering followed by particle growth (Kulmala et al., 2014). A complete understanding of both stages remains elusive in polluted urban environments. In the first stage, a key concern is the identity of the clustering molecules. Several laboratory studies (Almeida et al., 2013; Xiao et al., 2021) and ambient measurements (Yao et al., 2018; Yin et al., 2021; Yan et al., 2021; Cai et al., 2021b; Deng et al., 2020) indicate that clustering between sulfuric acid (SA) and amines drives the initial NPF in polluted environments. There are also studies suggesting that organic acids formed from oxidation of traffic emissions are key clustering species (Guo et al., 2020). The contrast between the enhanced NPF and reduced traffic load during the lockdown period seems to support the former mechanism, but a detailed investigation of how molecular clustering responded to those emission reductions remains lacking. For the growth phase, oxygenated organic molecules (OOMs) have been shown to dominate in some cases (Yan et al., 2021; Qiao et al., 2021). Further, a high fraction of nitrogen-containing OOMs suggests that RO2 + NOx reactions prevail in OOM formation (Qiao et al., 2021). For monoterpene-derived OOMs, which is characteristic of a remote atmosphere, high NOx levels can suppress particle growth by altering a fraction of products to organic nitrates with higher volatilities (Yan et al., 2020). However, the effect of NOx in OOM formation and particle growth needs to be examined in urban settings, where the VOC precursors are largely different.
Enhanced NPF during the lockdown period has been reported (X. Shen et al., 2021), but without a detailed explanation due to the lack of simultaneous measurements of both particles at the size where NPF starts (e.g., 1.5 nm) and key vapors for NPF, such as SA and OOMs. We fill that gap with comprehensive measurements from urban Beijing covering the lockdown period, enabling the investigation on how NPF responded to the emission reductions during lockdown on molecular and process levels.
2.1 Measurement location and period
The measurement campaign was conducted at the Aerosol and Haze Laboratory located at the west campus of Beijing University of Chemical Technology (BUCT station, latitude 39∘56′31′′ and longitude 116∘17′52′′). It is a representative urban station surrounded by residential and commercial areas and three main roads with heavy traffic loads. Measurements of atmospheric variables and pollutants have been conducted continuously in this station since early 2018. More details about the station and measurements can be found elsewhere (Liu et al., 2020).
The main data sets analyzed in this study were collected during 2019/12/15–2020/3/15, divided into pre-lockdown (2019/12/15–2020/01/22) and lockdown (2020/01/23–2020/03/15) periods. The Chinese Spring Festival (CSF) overlapped the lockdown period, but since they have a similar effect on population movement, the CSF and COVID-19 periods were not further separated in this study. As shown in Figs. S1–S2 in the Supplement, the traffic congestion index, as well as the NO2 concentration measured by 11 national monitoring stations in Beijing and by satellite, showed an apparent reduction and a slow rebound after the lockdown was imposed. In contrast, traffic and the NO2 concentration quickly rebounded after the CSF in 2019.
2.2 Instrumentation
The particle number size distribution over in the diameter range of 1 nm–10 µm was measured by the combination of a diethylene glycol scanning mobility particle spectrometer (DEG-SMPS, 1–7.5 nm) and a particle size distribution system (PSD, 3 nm–10 µm). Particle formation rates (, and J10) were calculated for all NPF cases using a new balance formula that is optimized for polluted environments (Cai and Jiang, 2017). NPF is classified according to the commonly used criteria originally described by Dal Maso and co-workers (Dal Maso et al., 2005), i.e., (1) a burst of sub-3 nm particles and (2) continuous particle growth in size. In some cases when only criterion 1 is satisfied, referred to as clustering events hereafter, particle formation rates can still be calculated because growth is negligible compared to coagulation and the term for such small particles is in the formula. Hence, we included both NPF events and clustering events when we investigated J1.5, J3, and their response to other relevant parameters. In addition, the condensation sink (CS) was calculated based on the measurement of particle number size distribution (Kulmala et al., 2012). Detailed calculations of particle formation rate and growth rate are provided in the Supplement.
SA and OOM concentrations were measured with a nitrate-ion-based chemical-ionization atmospheric-pressure-interface long-time-of-flight mass spectrometer (CI-APi-LTOF, Aerodyne Research, Inc.). The configuration of this instrument has been described previously (Yan et al., 2021). Two levels of calibrations were performed. First, the SA concentration was calibrated following the same procedure suggested by Kürten et al. (2012); second, the mass-dependent transmission efficiency of the instrument was obtained with the method developed by Heintrizi et al. (2016). After these calibrations, the concentration of SA and OOMs can be calculated using the equations below:
In the righthand side of Eqs. (1) and (2), the numerator and denominator are the signals of analytes and reagent ions, respectively, and C denotes the calibration coefficient obtained for SA, which was determined as 7.0×109 (molec. cm−3). TOOM in Eq. (2) is the mass-dependent transmission efficiency relative to the reagent ions.
In addition, we measured the concentrations of CO, SO2, NOx, and O3 using four Thermo Environmental Instruments (models 48i, 43i-TLE, 42i, 49i, respectively). These trace-gas pollutants were sampled through a 3 m tube from the building roof, which was heated to 313 K to reduce sampling losses. Calibrations of these instruments were performed once every 2 weeks using standard gases of known concentrations. In addition, several meteorological variables, including the ambient temperature, relative humidity, pressure, visibility, UVB radiation, and horizontal wind speed and direction, were measured with a weather station (AWS310, Vaisala Inc.) located on the rooftop of the building. More details of these instruments are provided in the Supplement.
3.1 Changes of atmospheric pollutants during the pre-lockdown and the lockdown periods
We first investigated the extent to which lockdown restrictions modified pollution concentrations. Figure 1 is an overview of the particle number size distributions and some other relevant pollutants. As shown in Fig. 1a and b, NPF occurred more frequently in the lockdown period (30.8 %, 16 out of 52 d) than in the pre-lockdown period (18.0 %, 7 out of 39 d). This difference is reduced if we include clustering events – to 34.6 % and 28.2 % for the lockdown and pre-lockdown periods, respectively. Consistent with a few recent studies (Cai et al., 2017; Yan et al., 2021; Deng et al., 2021), the burst in the concentration of sub-3 nm particles (N1−3 in Fig. 1b) corresponded to a low CS during both periods, suggesting that CS was the governing parameter for NPF.
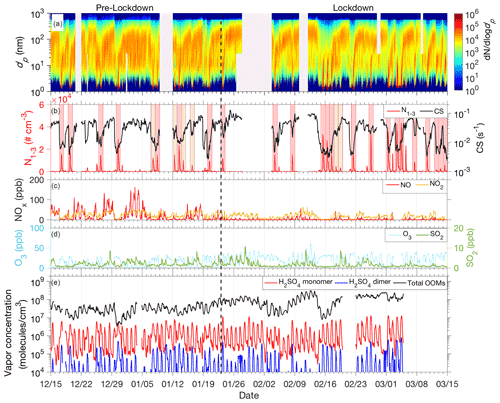
Figure 1Concentrations of atmospheric pollutants during the pre-lockdown and lockdown periods, including the particle number size distribution (a); number concentration of 1.5–3 nm particles (N1−3) and NPF classification (b); NO and NO2 (c); SO2 and O3 (d); and H2SO4 monomer, dimer, and total oxygenated organic molecules (OOMs) (e). The vertical dashed line denotes the separation of the pre-lockdown and the lockdown periods. (b) Days with NPF events and clustering events are shaded in red and orange, respectively.
One prominent change of the particle number size distribution during the lockdown was that particles in the size range of 10–30 nm were significantly reduced during the traffic rush hours (Fig. S3), indicating that vehicle emissions contributed substantially to particles of this size range during this time window. However, particles below this size range were not substantially depleted, indicating a limited contribution of traffic emissions to the sub-10 nm particle concentration in Beijing.
Significant changes in the concentration of trace-gas pollutants coincided with the lockdown. As shown in Fig. 2a–c, NO, NO2, and SO2 concentrations during NPF periods (07:00–18:00) decreased by 3.2-, 2.0-, and 3.0-fold (median values), respectively. As mentioned above, the reduction of NOx (NOx = NO + NO2) was directly related to the restriction of traffic. However, the reduced SO2 concentration was likely unrelated to the traffic restriction, because the SO2 concentrations did not exhibit a typical traffic pattern in either the pre-lockdown or the lockdown period. Unlike the primary pollutants, O3 concentrations increased by 25 % (Fig. 2d), consistent with previous studies (Huang et al., 2020). Moreover, in comparison to the pre-lockdown period, temperature and UVB radiation were higher during the lockdown period (Fig. S4), suggesting stronger atmospheric photochemistry.
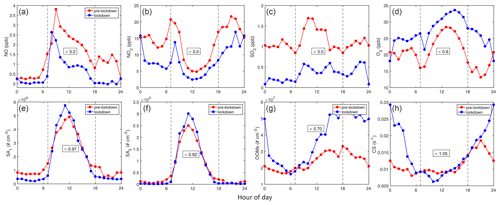
Figure 2Median diurnal cycles of atmospheric variables during the pre-lockdown and lockdown periods, including NO, NO2, SO2, O3, SA1, SA2, OOMs, and CS. The ratio of is given in the framed text. Here, [X] denotes the average value of a specific atmospheric variable during the NPF time window, i.e., 07:00–18:00, as marked by the two dashed lines.
The corresponding changes in the most NPF-relevant parameters, including sulfuric acid monomers (SA1), dimers (SA2), oxygenated organic molecules (OOMs), and CS, are shown in Fig. 2e–h. The CS was almost identical between the pre-lockdown and lockdown periods (Fig. 2h). The median SA1 and SA2 concentrations were also stable between the two periods. This is because the decline of the sulfuric acid precursor (i.e., SO2, Fig. 2c) was completely compensated for by the enhanced photochemistry, as indicated by the variation of UVB (Fig. S4b). In addition, the concentration of OOMs increased by about 50 % during the lockdown. This is because the concentration of volatile organic compounds (VOCs) only declined slightly in the lockdown period (X. Shen et al., 2021), but the photochemistry was much more enhanced.
3.2 Changes in initial particle formation rate and size-segregated growth rates
Based on our previous studies of the governing factors and mechanism of NPF in Beijing (Cai et al., 2017; Yan et al., 2021; Deng et al., 2021), we would expect the formation rates of 1.5 nm particles (J1.5) during the two periods to be very similar because SA1, SA2, and CS were nearly identical. However, this was not the case; a previous study in Beijing showed that NPF was more intense during the lockdown period than in the pre-lockdown period (X. Shen et al., 2021). In order to resolve this puzzle, we examined the detailed formation rates calculated for particles of different sizes, i.e., J1.5, J3, J6, and J10. We compare these formation rates in Fig. 3a. Consistent with our initial expectation, J1.5 was very similar in these two periods; however, at progressively larger particle sizes the difference of particle formation rates during the two periods becomes progressively more pronounced. This means that, while the nucleation rates remained constant, more of the newly formed particles survived during the lockdown period. As shown in Fig. 3b, the particle survival probabilities, calculated as from 1.5 to 3 nm, 6, and 10 nm during the lockdown period, were enhanced by factors of 1.2, 1.9, and 4.4, respectively, compared to pre-lockdown conditions. This provides one explanation for the enhanced particle formation rates reported previously – if the particles were only measured at a size larger than 1.5 nm, the calculated formation rate would be larger in the lockdown period due to the enhanced particle survival probability. In addition, despite the similar median values of J1.5, a few intense NPF cases occurred during the lockdown period, in contrast to the pre-lockdown period (Fig. 3c). In such cases, the classification of NPF events, which is to some extent subjective, could also affect the comparison (a classification bias). For instance, if weak NPF events were not detected or counted, the average J1.5 during the lockdown period would be higher. This could be another reason for the reported stronger NPF in the lockdown period (X. Shen et al., 2021).
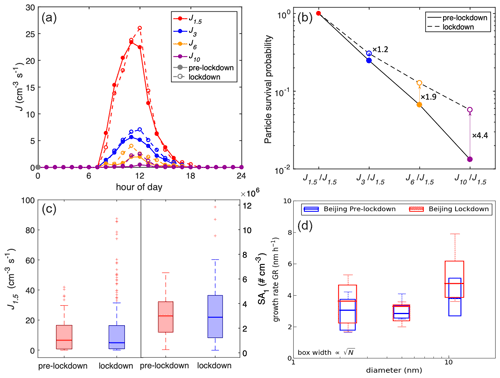
Figure 3The diurnal cycles of particle formation rates, growth rates, and survival probability (median values) at different sizes during the pre-lockdown and lockdown periods. (a) Diurnal variations of particle formation rates at different sizes, i.e., J1, J3, J6, and J10. (b) Particle survival probability as a function of size. (c) Box plots showing the distribution of J1.5 and SA1. (d) Size-segregated particle growth rates.
The particle survival probability is mostly determined by the competition between particle growth and scavenging by pre-existing large particles (Kerminen and Kulmala, 2002; Lehtinen et al., 2007). As the scavenging rate of nanoparticles is approximately proportional to CS, the particle survival probability is proportional to the ratio of particle growth rate (GR) to CS (GR/CS) (Kulmala et al., 2017). In our observations, CS values during the time windows of NPF events were similar in these two periods (Fig. 2h), so a change in GR must be the key to the different particle survival probability. To explore this, we calculated size-dependent growth rates of sub-10 nm particles in the pre-lockdown and lockdown periods with the appearance-time method. This method gives a higher GR than the mode-fitting method (Deng et al., 2020; Qiao et al., 2021). Consistent with previous studies (Deng et al., 2020; Qiao et al., 2021), larger particles had higher growth rates (Fig. 3d). The reason for the enhanced particle growth will be discussed in detail in Sect. 3.4.
3.3 Insights into the clustering mechanism and its response to the lockdown conditions
An important conclusion from our observations is that the clustering efficiency was not significantly affected by the lockdown restrictions, as otherwise J1.5 would have most likely changed drastically even though the SA concentration and CS were identical. For example, it has been shown that particle formation rates differ by up to a factor of 1000 when SA clusters with dimethylamine (DMA) instead of ammonia (Almeida et al., 2013), for constant SA and CS. Hence, we further investigated the clustering efficiency of SA and the relationship between SA2 and J1.5, focusing on comparisons between the pre-lockdown and lockdown periods.
An important diagnostic of SA clustering is the efficiency of SA2 formation via the collision of two SA1. Here, SA1 and SA2 denote monomers and dimers of SA, which may also contain base molecules acting as the stabilizer. Those base molecules cannot be seen by the nitrate CI-APi-LTOF because of their evaporation during charging processes or inside the instrument (Kurten et al., 2014). As the stabilizing effect of amines is much stronger than that of ammonia, SA2 formation efficiency is notably higher in the SA–amine system than in the SA–ammonia system (Almeida et al., 2013; Kurten et al., 2014). In addition, the SA2 formation efficiency also depends on the concentration of base molecules, CS, and on the temperature (Cai et al., 2021a). As shown in Fig. 4, the most prominent feature of the SA2 formation efficiency in our observations is a clear dependence on temperature; SA2 concentrations were consistently lower at higher temperatures. This dependence was identical for both the pre-lockdown and lockdown periods. On the other hand, the clustering efficiency appears to be independent of CS, because the loss of SA1-DMA1 clusters was dominated by evaporation over the temperature range of our observations (Fig. S5).
With a simplified SA–DMA clustering approach (Cai et al., 2021a), we were able to reproduce the SA2 formation, including its temperature dependence. Since the clustering efficiency was not affected by CS, we set CS = 0.01 s−1 for the simulations. For that CS, the best simulation result was obtained when the DMA concentration was constant at a volume mixing ratio of 1.3 ppt with a 50 % uncertainty, showing no systematic difference between the pre-lockdown and lockdown periods (Fig. S6). This is less than the measured DMA concentration in 2018 in Beijing (Deng et al., 2020). It should be noted that this effective DMA concentration (i.e., 1 ppt) is not the real concentration of DMA, but rather it means that the stabilizing effect of all base molecules is equivalent to that of 1 ppt DMA.
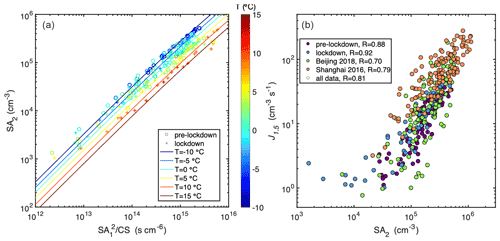
Figure 4Clustering of SA and formation of new particles during the pre-lockdown and lockdown periods. (a) Measured daytime (07:00–18:00) SA2 (dimer) concentration versus squared SA1 concentration divided by CS, color-coded by temperature. This represents the dimer production efficiency. Lines denote clustering model simulations (Cai et al., 2021a). The simulations deployed a constant dimethylamine concentration (1 ppt) and CS (0.01 s−1), which provided the best agreement with the ambient measurements. (b) Measured particle formation rate J1.5 versus SA2 concentration color-coded by different data sets. Measurements in 2018 wintertime Beijing (Yan et al., 2021) and in Shanghai (Yao et al., 2018) are also included. It should be noted that J1.7 was used in the study in Shanghai.
As shown in Fig. 4b, J1.5 correlates well with the SA2 concentration, indicating that particle formation is driven by SA clustering processes. The relationship between J1.5 and the SA2 concentration agrees well with earlier observations in Beijing (Yan et al., 2021) and Shanghai (Yao et al., 2018), with a correlation coefficient of 0.81 for all data. However, in comparison to those earlier studies, J1.5 in our observations is slightly lower, which could be attributed to the lower DMA concentration as discussed above. Most importantly, Fig. 4a and b clearly indicate that the mechanisms of both SA clustering and initial particle formation remained the same in the pre-lockdown and lockdown periods, although a clear temperature effect can be seen.
Ronkko et al. (2017) and Okuljar et al. (2021) both showed that in traffic-dense areas, the concentration of sub-3 nm particles is obviously higher than in background areas. Kanawade et al. (2022) conducted measurement of sub-3 nm particles at a site that is ∼1 km away from traffic emission and found an insignificant influence of traffic emission on the particle concentration. These studies suggest that the distance between the measurement site and the traffic emission source is crucial for the observation of the emitted sub-3 nm particles, likely due to the dilution and coagulation loss of these nano-particles. However, it is probably not the same reason for our study, because the measurement site of this study is very close to an arterial road with heavy traffic. One possibility of the discrepancy is that the emission factor of sub-3 nm particles is significantly lower for vehicles in Beijing. As shown in the laboratory study by Ronkko et al. (2017), the emission factor can vary by up to 3 orders of magnitude, being the highest for heavy-duty vehicles (e.g., diesel vehicles) and the lowest for light-duty cars. In Beijing, diesel vehicles are forbidden in downtown areas during traffic rush hours, so it is likely that the emission of sub-3 nm particles is weak. Also, the high coagulation sink in Beijing and India might be another reason for the small contribution of traffic emissions. Another possibility that cannot be fully ruled out is the potential biases due to different detection methods of sub-3 nm particles. The aforementioned studies utilized the particle size magnifier (PSM) to detect sub-3 nm particles, for which the size classification of particles is based on the saturation ratio of diethylene glycol (DEG), while we use the soft X-ray neutralizer and a DMA to classify particle size. The intrinsic difference between these two methods is not well quantified. It is also possible that the sub-3 nm particles by vehicles are not efficiently charged by the soft X-ray neutralizer and/or can be more efficiently activated by highly saturated DEG. Future research on the comparison between the PSM and SMPS is highly desired.
3.4 Characteristics of oxygenated organic molecules and the contribution to particle growth
Particle growth is key to particle survival and subsequently to the climate and health effects. Therefore, it is essential to understand the vapors responsible for particle growth, as well as the reason why particle growth was enhanced during the lockdown period, in spite of reduced primary emissions. As the sulfuric acid concentration remained stable (Fig. 2e) and it had a minor contribution to the growth of particles larger than 3 nm (Deng et al., 2020; Qiao et al., 2021), the enhanced particle growth rates were more likely associated with corresponding changes of OOMs than sulfuric acid.
Recent studies have suggested that elevated NOx can suppress the formation of low-volatility vapors by inhibiting the autoxidation of RO2 radicals (Yan et al., 2020). Due to the significant NOx reductions during the lockdown period, pronounced changes in OOM composition were expected. Such changes were indeed observed for some OOMs. For instance, as shown in Fig. 5a, the ratio between two indicative compound categories varied significantly as a function of NO. Here, the categories C6−9HO6N and C6−9HO5 are the termination products of bicyclic peroxy radicals originating from aromatics (C6−9HO5) (Wang et al., 2017) formed through reactions with NO and HO2, respectively. When the NO concentration declined from the pre-lockdown period to the lockdown period, the ratio of C6−9HO6N concentration to C6−9HO5 concentration decreased as well.
However, the majority of OOMs were insensitive to the declining NO. For example, the ratio of categories C6−9HO6N and C6−9HO5 did not depend on the NO concentration (Fig. 5b). These compounds are presumably termination products of C6−9HO4 radicals through reactions with NO and HO2, respectively. They have a double-bond equivalent (DBE) of 1, suggesting that they originate from aliphatic rather than aromatic precursors. Their NOx sensitivity differs from the OOMs derived from aromatics. It could be that even at low NOx concentrations, the reaction with NO is necessary to form OOMs from these peroxy radicals, as nitrogen-containing OOMs were consistently far more abundant than nitrogen-free OOMs. Figure 5c and d also show that the overall nitrogen number of OOMs did not depend on NO.
The overall OOM composition was surprisingly insensitive to changes in NOx concentrations. OOM chemical characteristics, i.e., the distributions of carbon number, oxygen number, nitrogen number, hydrogen number, hydrogen-to-carbon ratio, and oxygen-to-carbon ratio, remained almost identical during the two periods (Fig. S7). The stable OOM composition indicates similar intrinsic (300 K) volatility distributions in the pre-lockdown and lockdown periods, as shown in Fig. S8. The mean temperatures were about 274 and 280 K in these periods, respectively (Fig. S4a), and as a result, the ambient-temperature OOM volatilities were both lower than the intrinsic values but similar to each other due to the small temperature difference (Fig. S8). Therefore, we conclude that the influences of both temperature and RO2 + NOx chemistry on OOM vapor condensation and the resulting particle growth rates were very small. Though the OOM volatility distribution was stable between the pre-lockdown and lockdown periods, the OOM concentrations increased during the lockdown period, likely due to enhanced photochemistry.
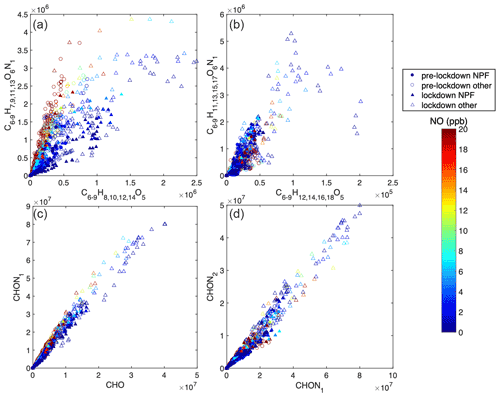
Figure 5The influence of NO (given by symbol color) on the composition of OOMs, indicated by the ratio between nitrogen-containing and nitrogen-free OOMs. (a) Selected OOMs with a double-bond equivalent (DBE) of 3, which are usually products from the oxidation of aromatic compounds (Molteni et al., 2018; Wang et al., 2017; Garmash et al., 2020). (b) Selected OOMs with a DBE of 1, which are more likely formed from the oxidation of aliphatic compounds, such as alkenes and alkanes. (c) OOMs containing 0 and 1 nitrogen atom. (d) OOMs containing 1 and 2 nitrogen atoms. In all panels, only daytime data (07:00–18:00) were included as they are directly relevant to NPF. Circles and triangles represent data in pre-lockdown and lockdown periods, respectively; filled and empty markers denote data during NPF days and other days, respectively.
Next, we examine contributions of SA and OOM to observed GRs in different size ranges, i.e., 1.5–3 nm (GR1.5−3), 3–7 nm (GR3−7), and 7–15 nm (GR7−15). Overall, this shows that different processes govern growth at different sizes and temperatures.
Sulfuric acid contributed a relatively constant 1–1.5 nm h−1 to GR1.5–3 as shown in Fig. 6a. At high temperatures (T>0 ∘C) this explains most of the growth. However, at low temperatures (T<0 ∘C), SA condensation alone does not explain the observed GR1−3, suggesting an important contribution of other vapors favored by low temperatures. The vapors and processes responsible for the residual GR1.5−3 remain unclear, but they do not appear to be OOMs, since the residual GR1.5−3 (GRmeasured–GRSA) after subtracting SA contribution does not show a positive correlation with condensable OOM concentration (Fig. S9). In fact, the residual GR1−3 shows a negative correlation with OOM concentration, mainly because of the coincidence of high OOM concentration and high temperature. One possibility could be the co-condensation of nitric acid and ammonia at low temperatures, as recently reported in controlled chamber experiments (M. Wang et al., 2020a). However, observational evidence is required to verify this hypothesis.
Above 3 nm, the growth rate from sulfuric acid condensation drops well below 1 nm h−1, and condensation from observed OOMs explains 1–4 nm h−1 of additional growth. For GR3−7 the OOM condensation correlates well with the observed GR (R=0.87), but the calculated GR was lower than the observed value by roughly a factor of 2. The largest observed and calculated growth was at the highest temperature during the lockdown, suggesting more efficient photochemical production, though a residual excess at lower temperature may be related to nitric acid and ammonia condensation. The correlation between calculated and observed growth degrades for GR7−15, though the highest observed and calculated values continue to be at higher temperature. Given the growth rates, these particles are several hours old, and so urban inhomogeneity may degrade this local analysis.
In laboratory experiments for growth by condensation of terpene oxidation products it has been shown that the nitrate cluster ionization can miss up to half of the condensable organic vapors (Trostl et al., 2016; Stolzenburg et al., 2018), and this could be true as well for these urban conditions. If we scale the measured OOM concentrations with the same factors used by Tröstl et al. (2016) (see Supplement), the measured and calculated GR fall close to the one-to-one line (Fig. S10). Hence, underestimated OOM concentrations may well explain the underpredictions above 3 nm, although other possible reasons cannot be fully excluded, for instance, the contribution of multiphase chemistry.
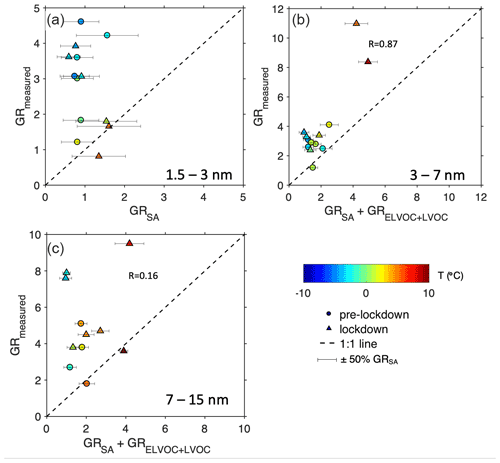
Figure 6Connections of size-segregated particle growth rates to the plausible vapor concentrations (a). The observed GR1.5−3 versus the GR1.5−3 predicted with SA (b). GR3−7 versus the predicted GR considering SA and condensable OOMs (the sum of ultra-low-volatility organic compounds, ULVOCs; extremely low-volatility organic compounds, ELVOCs; and low-volatility organic compounds, LVOCs; see Supplement) (c). GR7−15 versus the predicted GR considering SA and condensable OOMs. The contribution of SA to particle growth is estimated using the equation by Stolzenburg et al. (2020), and the contribution of condensable OOM was calculated assuming ELVOC and LVOC were effectively non-volatile (Nieminen et al., 2010; Ehn et al., 2014). The measurement uncertainty (±50 %) of SA (Kurten et al., 2012) is shown as the horizontal error bars. All plots are color-coded with the mean temperature at the corresponding time window. The linear correlation coefficients between the measured and calculated GRs in panel (b) and (c) are also given.
We examined the response of NPF to emission reductions in Beijing during the COVID-19 lockdown in both the molecular and the process levels. Clustering between SA and other base molecules drove the initial NPF in both pre-lockdown and lockdown periods. Our results show that this clustering was insensitive to the emission reductions. However, it is evident that the clustering efficiency of SA declined at high temperatures. This provides direct observational evidence that traffic emissions alone cannot be a major source of NPF in Beijing, in contrast to a few recent studies in urban areas (Ronkko et al., 2017; Guo et al., 2020).
The lockdown period showed an enhanced atmospheric oxidative capacity and reduced SO2 concentrations; these balanced, so that both the SA concentration and particle formation rates at 1.5 nm (J1.5) were similar during the pre-lockdown and lockdown periods. This appears to contradict a prior study reporting that NPF became stronger during the lockdown period based on a measurement of particles down to 2 nm (X. Shen et al., 2021). However, this apparent discrepancy is mainly due to an increased particle survival probability caused by enhanced particle growth during the lockdown period. To disentangle particle formation and growth, measurement of particles at or below 1.5 nm is crucial to understanding the formation mechanism of new particles.
The most obvious reason for the greater particle growth during the lockdown period was elevated OOM concentrations due to enhanced photochemistry. We also expected that lower NOx would favor particle growth, as NO can suppress particle growth by altering the OOM composition and increasing the overall OOM volatility (Yan et al., 2020). This turned out not to be the case in our study. We observed some changes in OOM composition in molecules derived from oxidation of aromatic VOCs, but for the most part changes in OOM composition and volatility were negligible. This suggests that the RO2 + NO reaction remains important to OOM formation even after such a dramatic NOx reduction. It has been proposed that atmospheric RO2 autoxidation will be increasingly more important if NOx keeps declining in North America (Praske et al., 2018), which might potentially enhance peroxide-driven particle toxicity and the yield of secondary organic aerosol (Zhao et al., 2017). However, our results suggest that these adverse effects on human health and air quality are less likely to occur in Beijing, at least in the near future.
A crucial challenge is to understand the key vapors and processes determining particle growth rates. We investigated particle growth over three consecutive size ranges: 1.5–3, 3–7, and 7–15 nm. Particle growth in each range shows distinct features, and its relationship with condensable vapors is the same in both periods. SA condensation almost completely explains GR1.5−3 at high temperatures. The co-condensation of nitric acid and ammonia might be an important contributor at low temperatures, but this needs further verification by observations. Condensation of OOMs plays a dominant role above 3 nm. Measured GR3−7 and OOMs are highly correlated. After scaling measured OOMs (by approximately a factor of 2) to account for compounds that escape detection by NO chemical ionization, the calculated GR3−7 and GR7−15 match the observed growth rates. The correlation with observations degrades at the larger size range, where particles are several hours old; there may be complex influences by other processes, such as the urban micro-meteorology and air mass inhomogeneity, which warrant future investigation.
Data and materials are available upon request to the corresponding authors.
The supplement related to this article is available online at: https://doi.org/10.5194/acp-22-12207-2022-supplement.
CY, YS, AD, JJ, and MK designed the study. CY, YS, XQ, AMS, YG, LC, CD, ZL, and FZ conducted the measurement or collected key materials. CY, YS, XQ, LD, DS, SH, AMS, YG, TK, and JK analyzed the data. CY wrote the manuscript. All co-authors have read and commented on the manuscript.
The contact author has declared that none of the authors has any competing interests.
Publisher’s note: Copernicus Publications remains neutral with regard to jurisdictional claims in published maps and institutional affiliations.
We thank the Beijing University of Chemical Technology for providing the observation platform.
This research has been supported by the National Key R&D Program of China (2019YFC0214701, 2017YFC0209503, and 2016YFC0200500), the National Natural Science Foundation of China (41877306 and 21876094), and Samsung PM2.5 SRP, as well as the Beijing University of Chemical Technology. This research has also been supported by the Academy of Finland (1251427, 1139656, 296628, 306853, 316114, and 311932) and Finnish Centre of Excellence (1141135 and 307331), the EC Seventh Framework Programme and European Union's Horizon 2020 program (ERC; project ATM-GTP, no. 742206, and project CHAPAs, no. 850614), the European Union's Horizon 2020 research and innovation program under Marie Skłodowska–Curie grant agreement no. 895875 (NPF-PANDA), the European Regional Development Fund, the Urban Innovative Actions initiative (HOPE: Healthy Outdoor Premises for Everyone; project no. UIA03-240), MegaSense by Business Finland (grant 7517/31/2018), the trans-national ERA-PLANET project SMURBS (grant agreement 689443) under the EU Horizon 2020 framework program, and Academy of Finland Flagship funding (grant no. 337549). The Centre of Excellence of Inverse Modeling and Imaging, Academy of Finland provided funding via project 312125. Kaspar R. Daellenbach received support from the Swiss National Science postdoc mobility grant (P2EZP2_18159). Juha Kangasluoma received funding from the UHEL 3-year grant (75284132) and a Finnish Academy of Science project (1325656). Simo Hakala and Mona Kurppa were supported by the doctoral program in atmospheric sciences (ATM-DP, University of Helsinki). Neil Donahue was supported by the US NSF (grant AGS1801897). Aijun Ding was supported by the National Natural Science Foundation of China (41725020). Lin Wang was supported by the National Natural Science Foundation of China (91644213 and 21925601).Open-access funding was provided by the Helsinki University Library.
This paper was edited by Kelley Barsanti and reviewed by two anonymous referees.
Agarwal, A., Kaushik, A., Kumar, S., and Mishra, R. K.: Comparative study on air quality status in Indian and Chinese cities before and during the COVID-19 lockdown period, Air Qual. Atmos. Health, 1–12, https://doi.org/10.1007/s11869-020-00881-z, 2020.
Almeida, J., Schobesberger, S., Kurten, A., Ortega, I. K., Kupiainen-Maatta, O., Praplan, A. P., Adamov, A., Amorim, A., Bianchi, F., Breitenlechner, M., David, A., Dommen, J., Donahue, N. M., Downard, A., Dunne, E., Duplissy, J., Ehrhart, S., Flagan, R. C., Franchin, A., Guida, R., Hakala, J., Hansel, A., Heinritzi, M., Henschel, H., Jokinen, T., Junninen, H., Kajos, M., Kangasluoma, J., Keskinen, H., Kupc, A., Kurten, T., Kvashin, A. N., Laaksonen, A., Lehtipalo, K., Leiminger, M., Leppa, J., Loukonen, V., Makhmutov, V., Mathot, S., McGrath, M. J., Nieminen, T., Olenius, T., Onnela, A., Petaja, T., Riccobono, F., Riipinen, I., Rissanen, M., Rondo, L., Ruuskanen, T., Santos, F. D., Sarnela, N., Schallhart, S., Schnitzhofer, R., Seinfeld, J. H., Simon, M., Sipila, M., Stozhkov, Y., Stratmann, F., Tome, A., Trostl, J., Tsagkogeorgas, G., Vaattovaara, P., Viisanen, Y., Virtanen, A., Vrtala, A., Wagner, P. E., Weingartner, E., Wex, H., Williamson, C., Wimmer, D., Ye, P., Yli-Juuti, T., Carslaw, K. S., Kulmala, M., Curtius, J., Baltensperger, U., Worsnop, D. R., Vehkamaki, H., and Kirkby, J.: Molecular understanding of sulphuric acid-amine particle nucleation in the atmosphere, Nature, 502, 359–363, 2013.
Bao, R., and Zhang, A.: Does lockdown reduce air pollution?, Evidence from 44 cities in northern China, Sci. Total Environ., 731, 139052, https://doi.org/10.1016/j.scitotenv.2020.139052, 2020.
Cai, R. and Jiang, J.: A new balance formula to estimate new particle formation rate: reevaluating the effect of coagulation scavenging, Atmos. Chem. Phys., 17, 12659–12675, https://doi.org/10.5194/acp-17-12659-2017, 2017.
Cai, R., Yang, D., Fu, Y., Wang, X., Li, X., Ma, Y., Hao, J., Zheng, J., and Jiang, J.: Aerosol surface area concentration: a governing factor in new particle formation in Beijing, Atmos. Chem. Phys., 17, 12327–12340, https://doi.org/10.5194/acp-17-12327-2017, 2017.
Cai, R., Yan, C., Worsnop, D. R., Bianchi, F., Kerminen, V.-M., Liu, Y., Wang, L., Zheng, J., Kulmala, M., and Jiang, J.: An indicator for sulfuric acid–amine nucleation in atmospheric environments, Aerosol Sci. Technol., 55, 1059–1069, 2021a.
Cai, R., Yan, C., Yang, D., Yin, R., Lu, Y., Deng, C., Fu, Y., Ruan, J., Li, X., Kontkanen, J., Zhang, Q., Kangasluoma, J., Ma, Y., Hao, J., Worsnop, D. R., Bianchi, F., Paasonen, P., Kerminen, V.-M., Liu, Y., Wang, L., Zheng, J., Kulmala, M., and Jiang, J.: Sulfuric acid–amine nucleation in urban Beijing, Atmos. Chem. Phys., 21, 2457–2468, https://doi.org/10.5194/acp-21-2457-2021, 2021b.
Chu, B., Zhang, S., Liu, J., Ma, Q., and He, H.: Significant concurrent decrease in PM2.5 and NO2 concentrations in China during COVID-19 epidemic, JJ. Environ. Sci., 99, 346–353, 2021.
Ciarelli, G., Jiang, J., El Haddad, I., Bigi, A., Aksoyoglu, S., Prévôt, A. S. H., Marinoni, A., Shen, J., Yan, C., and Bianchi, F.: Modeling the effect of reduced traffic due to COVID-19 measures on air quality using a chemical transport model: impacts on the Po Valley and the Swiss Plateau regions, Environ. Sci.-Atmos., 1, 228–240, 2021.
Dal Maso, M., Kulmala, M., Riipinen, I., Wagner, R., Hussein, T., Aalto, P. P., and Lehtinen, K. E.: Formation and growth of fresh atmospheric aerosols: eight years of aerosol size distribution data from SMEAR II, Hyytiala, Finland, Boreal Environ. Res., 10, 323–336, 2005.
Deng, C., Fu, Y., Dada, L., Yan, C., Cai, R., Yang, D., Zhou, Y., Yin, R., Lu, Y., Li, X., Qiao, X., Fan, X., Nie, W., Kontkanen, J., Kangasluoma, J., Chu, B., Ding, A., Kerminen, V. M., Paasonen, P., Worsnop, D. R., Bianchi, F., Liu, Y., Zheng, J., Wang, L., Kulmala, M., and Jiang, J.: Seasonal Characteristics of New Particle Formation and Growth in Urban Beijing, Environ. Sci. Technol., 54, 8547–8557, 2020.
Deng, C., Cai, R., Yan, C., Zheng, J., and Jiang, J.: Formation and growth of sub-3 nm particles in megacities: impact of background aerosols, Faraday Discuss., 226, 348–363, 2021.
Ehn, M., Thornton, J. A., Kleist, E., Sipila, M., Junninen, H., Pullinen, I., Springer, M., Rubach, F., Tillmann, R., Lee, B., Lopez-Hilfiker, F., Andres, S., Acir, I. H., Rissanen, M., Jokinen, T., Schobesberger, S., Kangasluoma, J., Kontkanen, J., Nieminen, T., Kurten, T., Nielsen, L. B., Jorgensen, S., Kjaergaard, H. G., Canagaratna, M., Maso, M. D., Berndt, T., Petaja, T., Wahner, A., Kerminen, V. M., Kulmala, M., Worsnop, D. R., Wildt, J., and Mentel, T. F.: A large source of low-volatility secondary organic aerosol, Nature, 506, 476–479, 2014.
Garmash, O., Rissanen, M. P., Pullinen, I., Schmitt, S., Kausiala, O., Tillmann, R., Zhao, D., Percival, C., Bannan, T. J., Priestley, M., Hallquist, Å. M., Kleist, E., Kiendler-Scharr, A., Hallquist, M., Berndt, T., McFiggans, G., Wildt, J., Mentel, T. F., and Ehn, M.: Multi-generation OH oxidation as a source for highly oxygenated organic molecules from aromatics, Atmos. Chem. Phys., 20, 515–537, https://doi.org/10.5194/acp-20-515-2020, 2020.
Guo, S., Hu, M., Zamora, M. L., Peng, J., Shang, D., Zheng, J., Du, Z., Wu, Z., Shao, M., Zeng, L., Molina, M. J., and Zhang, R.: Elucidating severe urban haze formation in China, P. Natl. Acad. Sci. USA, 111, 17373–17378, 2014.
Guo, S., Hu, M., Peng, J., Wu, Z., Zamora, M. L., Shang, D., Du, Z., Zheng, J., Fang, X., Tang, R., Wu, Y., Zeng, L., Shuai, S., Zhang, W., Wang, Y., Ji, Y., Li, Y., Zhang, A. L., Wang, W., Zhang, F., Zhao, J., Gong, X., Wang, C., Molina, M. J., and Zhang, R.: Remarkable nucleation and growth of ultrafine particles from vehicular exhaust, P. Natl. Acad. Sci. USA, 117, 3427–3432, 2020.
Harrison, R. M., Giorio, C., Beddows, D. C., and Dall'Osto, M.: Size distribution of airborne particles controls outcome of epidemiological studies, Sci. Total Environ. , 409, 289–293, 2010.
Heinritzi, M., Simon, M., Steiner, G., Wagner, A. C., Kürten, A., Hansel, A., and Curtius, J.: Characterization of the mass-dependent transmission efficiency of a CIMS, Atmos. Meas. Tech., 9, 1449–1460, https://doi.org/10.5194/amt-9-1449-2016, 2016.
Huang, X., Ding, A. J., Gao, J., Zheng, B., Zhou, D. R., Qi, X. M., Tang, R., Wang, J. P., Ren, C. H., Nie, W., Chi, X. G., Xu, Z., Chen, L. D., Li, Y. Y., Che, F., Pang, N. N., Wang, H. K., Tong, D., Qin, W., Cheng, W., Liu, W. J., Fu, Q. Y., Liu, B. X., Chai, F., Davis, J. S., Zhang, Q., and He, K. B.: Enhanced secondary pollution offset reduction of primary emissions during COVID-19 lockdown in China, Natl. Sci. Rev., 8, nwaa137, https://doi.org/10.1093/nsr/nwaa137, 2020.
Kanawade, V. P., Sebastian, M., and Dasari, P.: Reduction in Anthropogenic Emissions Suppressed New Particle Formation and Growth: Insights From the COVID-19 Lockdown, J. Geophys. Res.-Atmos., 127, e2021JD035392, https://doi.org/10.1029/2021JD035392, 2022.
Kerminen, V.-M. and Kulmala, M.: Analytical formulae connecting the “real” and the “apparent” nucleation rate and the nuclei number concentration for atmospheric nucleation events, J. Aerosol Sci., 33, 609–622, 2002.
Krecl, P., Targino, A. C., Oukawa, G. Y., and Cassino Junior, R. P.: Drop in urban air pollution from COVID-19 pandemic: Policy implications for the megacity of Sao Paulo, Environ. Pollut., 265, 114883, https://doi.org/10.1016/j.envpol.2020.114883, 2020.
Kulmala, M., Petaja, T., Nieminen, T., Sipila, M., Manninen, H. E., Lehtipalo, K., Dal Maso, M., Aalto, P. P., Junninen, H., Paasonen, P., Riipinen, I., Lehtinen, K. E., Laaksonen, A., and Kerminen, V. M.: Measurement of the nucleation of atmospheric aerosol particles, Nat. Protocol., 7, 1651–1667, 2012.
Kulmala, M., Petaja, T., Ehn, M., Thornton, J., Sipila, M., Worsnop, D. R., and Kerminen, V. M.: Chemistry of atmospheric nucleation: on the recent advances on precursor characterization and atmospheric cluster composition in connection with atmospheric new particle formation, Annu. Rev. Phys. Chem., 65, 21–37, 2014.
Kulmala, M., Kerminen, V. M., Petaja, T., Ding, A. J., and Wang, L.: Atmospheric gas-to-particle conversion: why NPF events are observed in megacities?, Faraday Discuss., 200, 271–288, 2017.
Kulmala, M., Dada, L., Daellenbach, K. R., Yan, C., Stolzenburg, D., Kontkanen, J., Ezhova, E., Hakala, S., Tuovinen, S., Kokkonen, T. V., Kurppa, M., Cai, R., Zhou, Y., Yin, R., Baalbaki, R., Chan, T., Chu, B., Deng, C., Fu, Y., Ge, M., He, H., Heikkinen, L., Junninen, H., Liu, Y., Lu, Y., Nie, W., Rusanen, A., Vakkari, V., Wang, Y., Yang, G., Yao, L., Zheng, J., Kujansuu, J., Kangasluoma, J., Petaja, T., Paasonen, P., Jarvi, L., Worsnop, D., Ding, A., Liu, Y., Wang, L., Jiang, J., Bianchi, F., and Kerminen, V. M.: Is reducing new particle formation a plausible solution to mitigate particulate air pollution in Beijing and other Chinese megacities?, Faraday Discuss., 226, 334–347, 2021.
Kurten, A., Rondo, L., Ehrhart, S., and Curtius, J.: Calibration of a chemical ionization mass spectrometer for the measurement of gaseous sulfuric acid, J. Phys. Chem. A., 116, 6375–6386, 2012.
Kurten, A., Jokinen, T., Simon, M., Sipila, M., Sarnela, N., Junninen, H., Adamov, A., Almeida, J., Amorim, A., Bianchi, F., Breitenlechner, M., Dommen, J., Donahue, N. M., Duplissy, J., Ehrhart, S., Flagan, R. C., Franchin, A., Hakala, J., Hansel, A., Heinritzi, M., Hutterli, M., Kangasluoma, J., Kirkby, J., Laaksonen, A., Lehtipalo, K., Leiminger, M., Makhmutov, V., Mathot, S., Onnela, A., Petaja, T., Praplan, A. P., Riccobono, F., Rissanen, M. P., Rondo, L., Schobesberger, S., Seinfeld, J. H., Steiner, G., Tome, A., Trostl, J., Winkler, P. M., Williamson, C., Wimmer, D., Ye, P., Baltensperger, U., Carslaw, K. S., Kulmala, M., Worsnop, D. R., and Curtius, J.: Neutral molecular cluster formation of sulfuric acid-dimethylamine observed in real time under atmospheric conditions, P. Natl. Acad. Sci. USA, 111, 15019–15024, 2014.
Le, T., Wang, Y., Liu, L., Yang, J., Yung, Y. L., Li, G., and Seinfeld, J. H.: Unexpected air pollution with marked emission reductions during the COVID-19 outbreak in China, Science, 369, 702–706, 2020.
Lehtinen, K. E. J., Dal Maso, M., Kulmala, M., and Kerminen, V.-M.: Estimating nucleation rates from apparent particle formation rates and vice versa: Revised formulation of the Kerminen–Kulmala equation, J. Aerosol Sci., 38, 988–994, 2007.
Liu, Y., Yan, C., Feng, Z., Zheng, F., Fan, X., Zhang, Y., Li, C., Zhou, Y., Lin, Z., Guo, Y., Zhang, Y., Ma, L., Zhou, W., Liu, Z., Dada, L., Dällenbach, K., Kontkanen, J., Cai, R., Chan, T., Chu, B., Du, W., Yao, L., Wang, Y., Cai, J., Kangasluoma, J., Kokkonen, T., Kujansuu, J., Rusanen, A., Deng, C., Fu, Y., Yin, R., Li, X., Lu, Y., Liu, Y., Lian, C., Yang, D., Wang, W., Ge, M., Wang, Y., Worsnop, D. R., Junninen, H., He, H., Kerminen, V.-M., Zheng, J., Wang, L., Jiang, J., Petäjä, T., Bianchi, F., and Kulmala, M.: Continuous and comprehensive atmospheric observations in Beijing: a station to understand the complex urban atmospheric environment, Big Earth Data, 4, 295–321, 2020.
Molteni, U., Bianchi, F., Klein, F., El Haddad, I., Frege, C., Rossi, M. J., Dommen, J., and Baltensperger, U.: Formation of highly oxygenated organic molecules from aromatic compounds, Atmos. Chem. Phys., 18, 1909–1921, https://doi.org/10.5194/acp-18-1909-2018, 2018.
Nieminen, T., Lehtinen, K. E. J., and Kulmala, M.: Sub-10 nm particle growth by vapor condensation – effects of vapor molecule size and particle thermal speed, Atmos. Chem. Phys., 10, 9773–9779, https://doi.org/10.5194/acp-10-9773-2010, 2010.
Okuljar, M., Kuuluvainen, H., Kontkanen, J., Garmash, O., Olin, M., Niemi, J. V., Timonen, H., Kangasluoma, J., Tham, Y. J., Baalbaki, R., Sipilä, M., Salo, L., Lintusaari, H., Portin, H., Teinilä, K., Aurela, M., Dal Maso, M., Rönkkö, T., Petäjä, T., and Paasonen, P.: Measurement report: The influence of traffic and new particle formation on the size distribution of 1–800 nm particles in Helsinki – a street canyon and an urban background station comparison, Atmos. Chem. Phys., 21, 9931–9953, https://doi.org/10.5194/acp-21-9931-2021, 2021.
Pei, Z., Han, G., Ma, X., Su, H., and Gong, W.: Response of major air pollutants to COVID-19 lockdowns in China, Sci. Total Environ., 743, 140879, https://doi.org/10.1016/j.scitotenv.2020.140879, 2020.
Praske, E., Otkjaer, R. V., Crounse, J. D., Hethcox, J. C., Stoltz, B. M., Kjaergaard, H. G., and Wennberg, P. O.: Atmospheric autoxidation is increasingly important in urban and suburban North America, P. Natl. Acad. Sci. USA, 115, 64–69, 2018.
Qiao, X., Yan, C., Li, X., Guo, Y., Yin, R., Deng, C., Li, C., Nie, W., Wang, M., Cai, R., Huang, D., Wang, Z., Yao, L., Worsnop, D. R., Bianchi, F., Liu, Y., Donahue, N. M., Kulmala, M., and Jiang, J.: Contribution of Atmospheric Oxygenated Organic Compounds to Particle Growth in an Urban Environment, Environ. Sci. Technol., 55, 13646–13656, 2021.
Ronkko, T., Kuuluvainen, H., Karjalainen, P., Keskinen, J., Hillamo, R., Niemi, J. V., Pirjola, L., Timonen, H. J., Saarikoski, S., Saukko, E., Jarvinen, A., Silvennoinen, H., Rostedt, A., Olin, M., Yli-Ojanpera, J., Nousiainene, P., Kousa, A., and Dal Maso, M.: Traffic is a major source of atmospheric nanocluster aerosol, P. Natl. Acad. Sci. USA, 114, 7549–7554, 2017.
Shen, J., Bigi, A., Marinoni, A., Lampilahti, J., Kontkanen, J., Ciarelli, G., Putaud, J. P., Nieminen, T., Kulmala, M., Lehtipalo, K., and Bianchi, F.: Emerging Investigator Series: COVID-19 lockdown effects on aerosol particle size distributions in northern Italy, Environ. Sci.-Atmos., 1, 214–227, 2021.
Shen, X., Sun, J., Yu, F., Wang, Y., Zhong, J., Zhang, Y., Hu, X., Xia, C., Zhang, S., and Zhang, X.: Enhancement of nanoparticle formation and growth during the COVID-19 lockdown period in urban Beijing, Atmos. Chem. Phys., 21, 7039–7052, https://doi.org/10.5194/acp-21-7039-2021, 2021.
Shi, X. and Brasseur, G. P.: The Response in Air Quality to the Reduction of Chinese Economic Activities During the COVID-19 Outbreak, Geophys. Res. Lett., 47, e2020GL088070, https://doi.org/10.1029/2020GL088070, 2020.
Sicard, P., De Marco, A., Agathokleous, E., Feng, Z., Xu, X., Paoletti, E., Rodriguez, J. J. D., and Calatayud, V.: Amplified ozone pollution in cities during the COVID-19 lockdown, Sci. Total Environ., 735, 139542, https://doi.org/10.1016/j.scitotenv.2020.139542, 2020.
Stolzenburg, D., Fischer, L., Vogel, A. L., Heinritzi, M., Schervish, M., Simon, M., Wagner, A. C., Dada, L., Ahonen, L. R., Amorim, A., Baccarini, A., Bauer, P. S., Baumgartner, B., Bergen, A., Bianchi, F., Breitenlechner, M., Brilke, S., Buenrostro Mazon, S., Chen, D., Dias, A., Draper, D. C., Duplissy, J., El Haddad, I., Finkenzeller, H., Frege, C., Fuchs, C., Garmash, O., Gordon, H., He, X., Helm, J., Hofbauer, V., Hoyle, C. R., Kim, C., Kirkby, J., Kontkanen, J., Kurten, A., Lampilahti, J., Lawler, M., Lehtipalo, K., Leiminger, M., Mai, H., Mathot, S., Mentler, B., Molteni, U., Nie, W., Nieminen, T., Nowak, J. B., Ojdanic, A., Onnela, A., Passananti, M., Petaja, T., Quelever, L. L. J., Rissanen, M. P., Sarnela, N., Schallhart, S., Tauber, C., Tome, A., Wagner, R., Wang, M., Weitz, L., Wimmer, D., Xiao, M., Yan, C., Ye, P., Zha, Q., Baltensperger, U., Curtius, J., Dommen, J., Flagan, R. C., Kulmala, M., Smith, J. N., Worsnop, D. R., Hansel, A., Donahue, N. M., and Winkler, P. M.: Rapid growth of organic aerosol nanoparticles over a wide tropospheric temperature range, P. Natl. Acad. Sci. USAA, 115, 9122–9127, 2018.
Stolzenburg, D., Simon, M., Ranjithkumar, A., Kürten, A., Lehtipalo, K., Gordon, H., Ehrhart, S., Finkenzeller, H., Pichelstorfer, L., Nieminen, T., He, X.-C., Brilke, S., Xiao, M., Amorim, A., Baalbaki, R., Baccarini, A., Beck, L., Bräkling, S., Caudillo Murillo, L., Chen, D., Chu, B., Dada, L., Dias, A., Dommen, J., Duplissy, J., El Haddad, I., Fischer, L., Gonzalez Carracedo, L., Heinritzi, M., Kim, C., Koenig, T. K., Kong, W., Lamkaddam, H., Lee, C. P., Leiminger, M., Li, Z., Makhmutov, V., Manninen, H. E., Marie, G., Marten, R., Müller, T., Nie, W., Partoll, E., Petäjä, T., Pfeifer, J., Philippov, M., Rissanen, M. P., Rörup, B., Schobesberger, S., Schuchmann, S., Shen, J., Sipilä, M., Steiner, G., Stozhkov, Y., Tauber, C., Tham, Y. J., Tomé, A., Vazquez-Pufleau, M., Wagner, A. C., Wang, M., Wang, Y., Weber, S. K., Wimmer, D., Wlasits, P. J., Wu, Y., Ye, Q., Zauner-Wieczorek, M., Baltensperger, U., Carslaw, K. S., Curtius, J., Donahue, N. M., Flagan, R. C., Hansel, A., Kulmala, M., Lelieveld, J., Volkamer, R., Kirkby, J., and Winkler, P. M.: Enhanced growth rate of atmospheric particles from sulfuric acid, Atmos. Chem. Phys., 20, 7359–7372, https://doi.org/10.5194/acp-20-7359-2020, 2020.
Trostl, J., Chuang, W. K., Gordon, H., Heinritzi, M., Yan, C., Molteni, U., Ahlm, L., Frege, C., Bianchi, F., Wagner, R., Simon, M., Lehtipalo, K., Williamson, C., Craven, J. S., Duplissy, J., Adamov, A., Almeida, J., Bernhammer, A. K., Breitenlechner, M., Brilke, S., Dias, A., Ehrhart, S., Flagan, R. C., Franchin, A., Fuchs, C., Guida, R., Gysel, M., Hansel, A., Hoyle, C. R., Jokinen, T., Junninen, H., Kangasluoma, J., Keskinen, H., Kim, J., Krapf, M., Kurten, A., Laaksonen, A., Lawler, M., Leiminger, M., Mathot, S., Mohler, O., Nieminen, T., Onnela, A., Petaja, T., Piel, F. M., Miettinen, P., Rissanen, M. P., Rondo, L., Sarnela, N., Schobesberger, S., Sengupta, K., Sipila, M., Smith, J. N., Steiner, G., Tome, A., Virtanen, A., Wagner, A. C., Weingartner, E., Wimmer, D., Winkler, P. M., Ye, P., Carslaw, K. S., Curtius, J., Dommen, J., Kirkby, J., Kulmala, M., Riipinen, I., Worsnop, D. R., Donahue, N. M., and Baltensperger, U.: The role of low-volatility organic compounds in initial particle growth in the atmosphere, Nature, 533, 527–531, 2016.
Wang, M., Kong, W., Marten, R., He, X.-C., Chen, D., Pfeifer, J., Heitto, A., Kontkanen, J., Dada, L., Kürten, A., Yli-Juuti, T., Manninen, H. E., Amanatidis, S., Amorim, A., Baalbaki, R., Baccarini, A., Bell, D. M., Bertozzi, B., Bräkling, S., Brilke, S., Murillo, L. C., Chiu, R., Chu, B., De Menezes, L.-P., Duplissy, J., Finkenzeller, H., Carracedo, L. G., Granzin, M., Guida, R., Hansel, A., Hofbauer, V., Krechmer, J., Lehtipalo, K., Lamkaddam, H., Lampimäki, M., Lee, C. P., Makhmutov, V., Marie, G., Mathot, S., Mauldin, R. L., Mentler, B., Müller, T., Onnela, A., Partoll, E., Petäjä, T., Philippov, M., Pospisilova, V., Ranjithkumar, A., Rissanen, M., Rörup, B., Scholz, W., Shen, J., Simon, M., Sipilä, M., Steiner, G., Stolzenburg, D., Tham, Y. J., Tomé, A., Wagner, A. C., Wang, D. S., Wang, Y., Weber, S. K., Winkler, P. M., Wlasits, P. J., Wu, Y., Xiao, M., Ye, Q., Zauner-Wieczorek, M., Zhou, X., Volkamer, R., Riipinen, I., Dommen, J., Curtius, J., Baltensperger, U., Kulmala, M., Worsnop, D. R., Kirkby, J., Seinfeld, J. H., El-Haddad, I., Flagan, R. C., and Donahue, N. M.: Rapid growth of new atmospheric particles by nitric acid and ammonia condensation, Nature, 581, 184–189, 2020.
Wang, P., Chen, K., Zhu, S., Wang, P., and Zhang, H.: Severe air pollution events not avoided by reduced anthropogenic activities during COVID-19 outbreak, Resour Conserv Recycl, 158, 104814, https://doi.org/10.1016/j.resconrec.2020.104814, 2020.
Wang, S., Wu, R., Berndt, T., Ehn, M., and Wang, L.: Formation of Highly Oxidized Radicals and Multifunctional Products from the Atmospheric Oxidation of Alkylbenzenes, Environ. Sci. Technol., 51, 8442–8449, 2017.
Xiao, M., Hoyle, C. R., Dada, L., Stolzenburg, D., Kürten, A., Wang, M., Lamkaddam, H., Garmash, O., Mentler, B., Molteni, U., Baccarini, A., Simon, M., He, X.-C., Lehtipalo, K., Ahonen, L. R., Baalbaki, R., Bauer, P. S., Beck, L., Bell, D., Bianchi, F., Brilke, S., Chen, D., Chiu, R., Dias, A., Duplissy, J., Finkenzeller, H., Gordon, H., Hofbauer, V., Kim, C., Koenig, T. K., Lampilahti, J., Lee, C. P., Li, Z., Mai, H., Makhmutov, V., Manninen, H. E., Marten, R., Mathot, S., Mauldin, R. L., Nie, W., Onnela, A., Partoll, E., Petäjä, T., Pfeifer, J., Pospisilova, V., Quéléver, L. L. J., Rissanen, M., Schobesberger, S., Schuchmann, S., Stozhkov, Y., Tauber, C., Tham, Y. J., Tomé, A., Vazquez-Pufleau, M., Wagner, A. C., Wagner, R., Wang, Y., Weitz, L., Wimmer, D., Wu, Y., Yan, C., Ye, P., Ye, Q., Zha, Q., Zhou, X., Amorim, A., Carslaw, K., Curtius, J., Hansel, A., Volkamer, R., Winkler, P. M., Flagan, R. C., Kulmala, M., Worsnop, D. R., Kirkby, J., Donahue, N. M., Baltensperger, U., El Haddad, I., and Dommen, J.: The driving factors of new particle formation and growth in the polluted boundary layer, Atmos. Chem. Phys., 21, 14275–14291, https://doi.org/10.5194/acp-21-14275-2021, 2021.
Xing, J., Li, S., Jiang, Y., Wang, S., Ding, D., Dong, Z., Zhu, Y., and Hao, J.: Quantifying the emission changes and associated air quality impacts during the COVID-19 pandemic on the North China Plain: a response modeling study, Atmos. Chem. Phys., 20, 14347–14359, https://doi.org/10.5194/acp-20-14347-2020, 2020.
Yan, C., Nie, W., Vogel, A. L., Dada, L., Lehtipalo, K., Stolzenburg, D., Wagner, R., Rissanen, M. P., Xiao, M., Ahonen, L., Fischer, L., Rose, C., Bianchi, F., Gordon, H., Simon, M., Heinritzi, M., Garmash, O., Roldin, P., Dias, A., Ye, P., Hofbauer, V., Amorim, A., Bauer, P. S., Bergen, A., Bernhammer, A. K., Breitenlechner, M., Brilke, S., Buchholz, A., Mazon, S. B., Canagaratna, M. R., Chen, X., Ding, A., Dommen, J., Draper, D. C., Duplissy, J., Frege, C., Heyn, C., Guida, R., Hakala, J., Heikkinen, L., Hoyle, C. R., Jokinen, T., Kangasluoma, J., Kirkby, J., Kontkanen, J., Kurten, A., Lawler, M. J., Mai, H., Mathot, S., Mauldin, R. L., 3rd, Molteni, U., Nichman, L., Nieminen, T., Nowak, J., Ojdanic, A., Onnela, A., Pajunoja, A., Petaja, T., Piel, F., Quelever, L. L. J., Sarnela, N., Schallhart, S., Sengupta, K., Sipila, M., Tome, A., Trostl, J., Vaisanen, O., Wagner, A. C., Ylisirnio, A., Zha, Q., Baltensperger, U., Carslaw, K. S., Curtius, J., Flagan, R. C., Hansel, A., Riipinen, I., Smith, J. N., Virtanen, A., Winkler, P. M., Donahue, N. M., Kerminen, V. M., Kulmala, M., Ehn, M., and Worsnop, D. R.: Size-dependent influence of NOx on the growth rates of organic aerosol particles, Sci. Adv., 6, eaay4945, https://doi.org/10.1126/sciadv.aay4945, 2020.
Yan, C., Yin, R., Lu, Y., Dada, L., Yang, D., Fu, Y., Kontkanen, J., Deng, C., Garmash, O., Ruan, J., Baalbaki, R., Schervish, M., Cai, R., Bloss, M., Chan, T., Chen, T., Chen, Q., Chen, X., Chen, Y., Chu, B., Dällenbach, K., Foreback, B., He, X., Heikkinen, L., Jokinen, T., Junninen, H., Kangasluoma, J., Kokkonen, T., Kurppa, M., Lehtipalo, K., Li, H., Li, H., Li, X., Liu, Y., Ma, Q., Paasonen, P., Rantala, P., Pileci, R. E., Rusanen, A., Sarnela, N., Simonen, P., Wang, S., Wang, W., Wang, Y., Xue, M., Yang, G., Yao, L., Zhou, Y., Kujansuu, J., Petäjä, T., Nie, W., Ma, Y., Ge, M., He, H., Donahue, N. M., Worsnop, D. R., Kerminen, V.-M., Wang, L., Liu, Y., Zheng, J., Kulmala, M., Jiang, J., and Bianchi, F.: The Synergistic Role of Sulfuric Acid, Bases, and Oxidized Organics Governing New-Particle Formation in Beijing, Geophys. Res. Lett., 48, e2020GL091944, https://doi.org/10.1029/2020GL091944, 2021.
Yao, L., Garmash, O., Bianchi, F., Zheng, J., Yan, C., Kontkanen, J., Junninen, H., Mazon, S. B., Ehn, M., Paasonen, P., Sipilä, M., Wang, M., Wang, X., Xiao, S., Chen, H., Lu, Y., Zhang, B., Wang, D., Fu, Q., Geng, F., Li, L., Wang, H., Qiao, L., Yang, X., Chen, J., Kerminen, V.-M., Petäjä, T., Worsnop, D. R., Kulmala, M., and Wang, L.: Atmospheric new particle formation from sulfuric acid and amines in a Chinese megacity, Science, 361, 278–281, 2018.
Yin, R., Yan, C., Cai, R., Li, X., Shen, J., Lu, Y., Schobesberger, S., Fu, Y., Deng, C., Wang, L., Liu, Y., Zheng, J., Xie, H., Bianchi, F., Worsnop, D. R., Kulmala, M., and Jiang, J.: Acid–Base Clusters during Atmospheric New Particle Formation in Urban Beijing, Environ. Sci. Technol., 55, 10994–11005, 2021.
Zhao, Y., Saleh, R., Saliba, G., Presto, A. A., Gordon, T. D., Drozd, G. T., Goldstein, A. H., Donahue, N. M., and Robinson, A. L.: Reducing secondary organic aerosol formation from gasoline vehicle exhaust, P. Natl. Acad. Sci. USA, 114, 6984, https://doi.org/10.1073/pnas.1620911114, 2017.