the Creative Commons Attribution 4.0 License.
the Creative Commons Attribution 4.0 License.
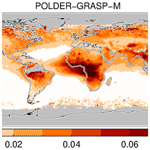
AEROCOM and AEROSAT AAOD and SSA study – Part 1: Evaluation and intercomparison of satellite measurements
Oleg Dubovik
Otto Hasekamp
Omar Torres
Hiren Jethva
Peter J. T. Leonard
Pavel Litvinov
Jens Redemann
Yohei Shinozuka
Gerrit de Leeuw
Stefan Kinne
Thomas Popp
Michael Schulz
Philip Stier
Global measurements of absorbing aerosol optical depth (AAOD) are scarce and mostly provided by the ground network AERONET (AErosol RObotic NETwork). In recent years, several satellite products of AAOD have been developed. This study's primary aim is to establish the usefulness of these datasets for AEROCOM (Aerosol Comparisons between Observations and Models) model evaluation with a focus on the years 2006, 2008 and 2010. The satellite products are super-observations consisting of min aggregated retrievals.
This study consists of two papers, the current one that deals with the assessment of satellite observations and a second paper (Schutgens et al., 2021) that deals with the evaluation of models using those satellite data. In particular, the current paper details an evaluation with AERONET observations from the sparse AERONET network as well as a global intercomparison of satellite datasets, with a focus on how minimum AOD (aerosol optical depth) thresholds and temporal averaging may improve agreement between satellite observations.
All satellite datasets are shown to have reasonable skill for AAOD (three out of four datasets show correlations with AERONET in excess of 0.6) but less skill for SSA (single-scattering albedo; only one out of four datasets shows correlations with AERONET in excess of 0.6). In comparison, satellite AOD shows correlations from 0.72 to 0.88 against the same AERONET dataset. However, we show that performance vs. AERONET and inter-satellite agreements for SSA improve significantly at higher AOD. Temporal averaging also improves agreements between satellite datasets. Nevertheless multi-annual averages still show systematic differences, even at high AOD. In particular, we show that two POLDER (Polarization and Directionality of the Earth's Reflectances) products appear to have a systematic SSA difference over land of ∼0.04, independent of AOD. Identifying the cause of this bias offers the possibility of substantially improving current datasets.
We also provide evidence that suggests that evaluation with AERONET observations leads to an underestimate of true biases in satellite SSA.
In the second part of this study we show that, notwithstanding these biases in satellite AAOD and SSA, the datasets allow meaningful evaluation of AEROCOM models.
- Article
(11819 KB) -
Supplement
(7331 KB) - BibTeX
- EndNote
Aerosol is an important component of the Earth's atmosphere that affects the planet's climate, the biosphere and human health. Aerosol particles scatter and absorb sunlight as well as modify clouds. Anthropogenic aerosol changes the radiative balance and influences global warming (Angstrom, 1962; Twomey, 1974; Albrecht, 1989; Hansen et al., 1997; Lohmann and Feichter, 1997, 2005). It may negatively affect solar power generation (Li et al., 2017; Labordena et al., 2018). Aerosol can transport soluble iron, phosphate and nitrate over long distances and provide nutrients for the biosphere (Swap et al., 1992; Vink and Measures, 2001; McTainsh and Strong, 2007; Maher et al., 2010; Lequy et al., 2012) . Aerosol can penetrate deep into lungs and may carry toxins or serve as disease vectors (Dockery et al., 1993; Brunekreef and Holgate, 2002; Ezzati et al., 2002; Smith et al., 2009; Beelen et al., 2013; Ballester et al., 2013).
Aerosol reflects visible radiation from the Sun, and some aerosol also absorbs it (Dubovik et al., 2002; Omar et al., 2005). The species that absorb the most visible sunlight are, in order of importance, black carbon, dust and brown carbon. Of these, black carbon is expected to exert a significant positive radiative forcing on the climate (Bond et al., 2013; Myhre et al., 2013). Absorbing aerosol's impact is mostly through heating of the atmospheric profile (direct effect) and subsequent stabilization or destabilization (Johnson et al., 2003) of the boundary layer (semi-direct effect). This affects cloud formation (Koren et al., 2008; Brioude et al., 2009) and precipitation (Hodnebrog et al., 2016; Samset et al., 2016; Hodzic and Duvel, 2018). In particular over bright surfaces (ice, deserts, clouds) the forcing due to absorbing aerosol can be significant (Haywood and Shine, 1995; Graaf et al., 2012; Tegen and Heinold, 2018).
On regional scales, biomass burning smoke has been implicated in increased tornado severity (Saide et al., 2015), while dust was observed to reduce cyclones (Chen et al., 2017); black carbon may affect the Hadley cell circulation (Allen et al., 2012; Tosca et al., 2013); and black carbon deposition can reduce glacier albedo (Thomas et al., 2017; Zhang et al., 2017; Dang et al., 2017), which may speed up glacier melt.
Currently, absorbing aerosol can be measured in a number of ways. AERONET (AErosol RObotic NETwork, Holben et al., 1998) is a global but spatially sparse network of Sun photometers that includes two scanning protocols (almucantar and hybrid) that allow the inversion of measured radiances into particle size distributions and refractive indices (Dubovik and King, 2000). From this inversion, columnar AAOD (absorbing aerosol optical depth) can be derived. There are also networks (Laj et al., 2020) of (filter-based) absorption photometers, as used in EMEP (European Monitoring and Evaluation Programme), ACTRIS (Aerosol, Clouds and Trace Gases Research Infrastructure) and IMPROVE (Interagency Monitoring of Protected Visual Environments). These networks are concentrated in Europe and North America, and there is no global coverage. Moreover, these are surface measurements that do not measure the full atmospheric column. Finally, absorption photometers like the SP2 were used on flight campaigns like HIPPO (Schwarz et al., 2010, 2013; Wang et al., 2014). Again, this yields spatially sparse in situ observations of absorbing aerosol. While these measurement networks have proven to be very important to our understanding of absorbing aerosol, a satellite-derived AAOD would contribute greatly by adding spatial context in regions with ground-based instruments and measurements in regions without such instruments. As it now stands, we have almost no observations of absorbing aerosol over the oceans, in particular in continental outflow regions.
However, in recent years a number of satellite AAOD products have been developed, often based on POLDER (Polarization and Directionality of the Earth's Reflectances) measurements. For example, Lacagnina et al. (2015) used POLDER data to evaluate SSA (single-scattering albedo) from AEROCOM (Aerosol Comparisons between Observations and Models) models over oceans, Peers et al. (2016) evaluated over ocean above-cloud SSA in AEROCOM models for the African fire season, Lacagnina et al. (2017) estimated the global direct radiative effect of aerosol, and Hasekamp et al. (2019b) estimated aerosol–cloud interactions. Chen et al. (2018, 2019) assimilated POLDER AOD and AAOD observations to estimate aerosol emissions, while Tsikerdekis et al. (2021) showed the benefit of jointly assimilating POLDER AOD (aerosol optical depth), AE (Ångström exponent) and SSA (single-scattering albedo) observations. Kacenelenbogen et al. (2019) used combinations of A-Train sensors to infer AAOD over clouds and estimate short-wave direct aerosol effects.
The challenge in retrieving AAOD from satellites is made clear by the challenge in retrieving AAOD from AERONET measurements. AERONET AAOD observations are known to be more uncertain than AOD observations. Dubovik et al. (2000) estimated that AERONET SSA uncertainties for AOD ≤0.2 at 440 nm would be at least 0.05, using numerical sensitivity tests. A recent in-depth estimate of the uncertainty in Inversion V3 data (Sinyuk et al., 2020) for four different sites suggested SSA uncertainties at AOD (at 440 nm) equal 0.2 from 0.037 to 0.048 at 440 nm and from 0.035 to 0.045 at 675 nm. It is not clear whether these uncertainties should be interpreted as site-specific biases or random errors. This distinction matters as random errors can be reduced through appropriate averaging of data. Large differences between AERONET SSA at low AOD and in situ measurements were indeed confirmed by Andrews et al. (2017). Even at higher AOD (≥0.5), Dubovik et al. (2000) suggested SSA errors of at least 0.03. Sinyuk et al. (2020) suggest smaller SSA uncertainties of 0.017 to 0.023 at 440 nm and 0.015 to 0.026 at 675 nm for AOD (at 440 nm) equal to 0.6. Given the challenges in satellite remote sensing compared to ground-based remote sensing, satellite AAOD and SSA products can be expected to have large errors as well.
Global Climate Observing System (GCOS) requirements (WMO, 2011) for SSA specify an accuracy within 0.03 and a stability per decade within 0.01, for a horizontal resolution of 5–10 km and a temporal resolution of 4 h. These requirements appear based on typical regional and yearly variations in SSA. However, SSA requirements are different for different applications (monitoring, trends, model evaluation, process studies), while the GCOS requirements are meant to provide a general broad estimate (Popp et al., 2016). In part 2 of our study we will show that current satellite AAOD and SSA capabilities allow for useful evaluation of models.
For measurements to be useful in model evaluation, their errors after averaging (spatially, temporally) need to be smaller than the model errors the observations should be able to identify. A traditional evaluation of satellite datasets with AERONET data is unlikely to establish this, partly because the model aspect is ignored and partly because AERONET covers some very interesting aerosol source regions (e.g. oceans, most deserts and boreal fire scapes) only sparsely. In the first part of this study (the current paper) we complement the traditional evaluation with a satellite intercomparison (in itself not unusual) to broaden our understanding of satellite performance over diverse regions. In the second part (a follow-up paper, Schutgens et al., 2021), we present a novel analysis that combines satellite evaluation and intercomparison with model evaluation and allows for the assessment of model biases in the context of satellite biases.
We will use satellite data aggregated over min as this allows spatio-temporal collocation amongst datasets (satellite, AERONET, AEROCOM) which should strongly reduce representation errors in our analyses (Schutgens et al., 2016a, b). All analyses, even of multi-year averages, will start from spatio-temporally collocated datasets.
This paper is the result of discussions in the AEROCOM (Aerosol Comparisons between Observations and Models, https://aerocom.met.no, last access: 4 May 2021) and AEROSAT (International Satellite Aerosol Science Network, https://aero-sat.org, last access: 4 May 2021) communities. Both are grassroots communities, the first organized around aerosol modellers and the second around retrieval groups. They meet every year to discuss common issues in the field of aerosol studies.
The observational datasets used in this study are described in Sect. 2. The collocation and analysis methodology are described in Sect. 3. A first look at the satellite datasets is presented in Sect. 4. Evaluation of satellite AOD, AAOD and SSA with AERONET is performed in Sect 5, and a more detailed intercomparison of satellite data is shown in Sect. 6. A summary and conclusions can be found in Sect. 7.
2.1 Remote sensing data
Original satellite L2 data (estimates of geophysical variables on the spatio-temporal sampling pattern of the radiances; see also Mittaz and Merchant, 2019) were aggregated unto a regular spatio-temporal grid with spatio-temporal grid boxes of min. The resulting super-observations ( min aggregates) are more representative of global model grid boxes (∼1–3∘ in size) while allowing accurate temporal collocation with other datasets. At the same time, the use of super-observations significantly reduces data amount without much loss of information (at the scale of global model grid boxes). A list of products used in this paper is given in Table 1. A colour legend to the different products can be found in Fig. 1. More explanation of the aggregation procedure can be found in Appendix A.
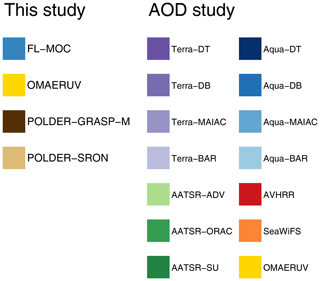
Figure 1Colour legend used throughout this paper to designate the different satellite products for both this study and the AOD study in Schutgens et al. (2020).
Table 1Remote sensing products used in this study.
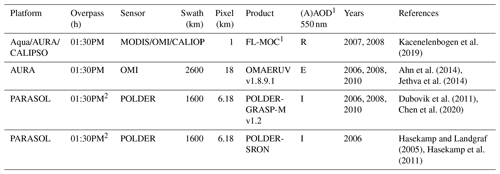
1 This product uses a combination of Aqua-MODIS, OMI and CALIOP observations. 2 PARASOL started drifting away from Aqua at the end of 2009. 3 Interpolated or extrapolated to 550 nm, depending on surface type; or retrieved at 550 nm.
Super-observations of AOD and AAOD at the same location and time were derived from the same set of L2 data and therefore measure the exact same scene (note an exception for the GRASP dataset described below).
The main data are AOD and AAOD at 550 nm, the wavelength at which models typically provide (A)AOD. If (A)AOD was not retrieved at this wavelength, it was logarithmically interpolated or extrapolated from surrounding wavelengths.
2.1.1 FL-MOC
FL-MOC (Fu–Liou – MODIS, OMI, CALIOP) is a technique for combining CALIOP (Cloud-Aerosol Lidar with Orthogonal Polarization) aerosol backscatter, MODIS (Moderate Resolution Imaging Spectroradiometer) spectral AOD and OMI (Ozone Monitoring Instrument) AAOD retrievals for estimating full spectral sets of aerosol radiative properties (SSA, asymmetry parameter and AOD). It is not a retrieval per se but a consistent reinterpretation of the combined data within their stated uncertainties. Details are given in Kacenelenbogen et al. (2019, Appendix A). In brief, FL-MOC uses the L2 retrieved aerosol properties as input to a simple lookup table retrieval of aerosol types and concentrations, under the assumption that aerosol properties are consistent with the L2 aerosol observations within the stated uncertainties of each sensor's retrieval. This technique also assumes that the surface reflectance and clouds are properly treated in the underlying retrievals.
Over land, FL-MOC uses OMAERUV (see Sect. 2.1.2) AAOD, and over ocean it uses OMAERO AAOD. OMAERO is an advanced multi-wavelength UV–VIS algorithm that uses 17 wavelengths in the 331–500 nm range in order to calculate the aerosol optical depth and to discriminate between various types of aerosols. It is an extension of the near-UV TOMS (Total Ozone Mapping Spectrometer) method (see the OMAERUV product) to a wider wavelength range. The OMAERO algorithm is applied over all surface types; however, its primary objective is to derive aerosol properties over the oceans due to the limited availability of spectral surface reflectivity databases over land.
2.1.2 OMAERUV
The Ozone Monitoring Instrument (OMI) on the EOS-Aura satellite was deployed in July 2004. It is a high-resolution spectrograph that measures the upwelling radiance at the top of the atmosphere in the ultraviolet and visible (270–500 nm) regions of the solar spectrum (Levelt et al., 2006). It had a 2600 km wide swath and provides daily global coverage at a spatial resolution varying from 13×24 km at nadir to 28×150 km at the extremes of the swath. OMI hyperspectral measurements are used as input to inversion algorithms to retrieve ozone vertical distribution and column amounts of O3, NO2, SO2, HCHO, BrO and OClO. OMI observations are also used to retrieve information on aerosols and clouds.
Aerosol properties in the near UV are derived from OMI observations at 354 and 388 nm (Torres et al., 2007). The OMI UV aerosol algorithm (OMAERUV) takes advantage of the large sensitivity to aerosol absorption in the near UV discovered in the mid-90s using heritage TOMS instruments (Herman et al., 1997) and the low reflectance of all ice/snow-free terrestrial surfaces, which facilitates the aerosol characterization over all arid and semi-arid regions of the world. The OMAERUV two-channel algorithm simultaneously retrieves AOD and SSA at 388 nm. The main sources of uncertainty are assumed aerosol layer height and cloud contamination, with the latter associated with the sensor's coarse spatial resolution. The OMAERUV 15-year record of AOD has been validated with AERONET observations (Torres et al., 2013; Ahn et al., 2014). The SSA record has also been evaluated by comparisons to AERONET and SKYNET (https://www.skynet-isdc.org/index.php, last access: 4 May 2021) ground-based retrievals (Jethva et al., 2014, 2019).
2.1.3 POLDER-SRON
The POLDER-3 instrument was a multi-angle, multi-wavelength polarimeter flying aboard the Polarization and Anisotropy of Reflectances for Atmospheric Sciences coupled with Observations from a Lidar (PARASOL) satellite. It was launched in 2004 and was a part of the satellite constellation A-Train until 2009. Initially designed to be operated for 2 years, POLDER-3 performed its measurements until late 2013, when it was decommissioned. PARASOL provides measurements of a ground scene under (up to) 16 different viewing geometries in 9 spectral bands (443, 490, 565, 670, 763, 765, 865, 910, 1020 nm). Linear polarization measurements (Stokes parameters Q and U) are performed in three spectral bands (490, 670, 865 nm). Its spatial resolution at the nadir was about 6 km, and its swath width was 2400 km.
An advanced retrieval algorithm making full use of the information content of the multi-angle photopolarimetric observations from POLDER-3/PARASOL has been developed at SRON (Netherlands Institute for Space Research). The algorithm has large flexibility in defining the aerosol properties included in the retrieval state vector (Fu and Hasekamp, 2018). The aerosol size distribution is described by the sum of an arbitrary number of log-normal functions, called modes, where for each mode the effective radius (reff), effective variance (veff), aerosol column number, real and imaginary parts of the refractive index (in the form of coefficients of spectrally dependent functions), fraction of spherical particles assuming the mixture of spheres and spheroids proposed by Dubovik et al. (2006), and the aerosol layer height can (in principle) be retrieved. In the setup used in the present study, the POLDER-SRON algorithm yields the different microphysical characteristics of a bimodal aerosol size distribution (fine and coarse mode), with the fraction of spheres only be retrieved for the coarse mode (fine mode assumed to consist only of spheres) and the aerosol layer height fixed to 1 km. For retrievals over ocean, the state vector also includes the wind speed, chlorophyll a concentration and whitecap fraction, while for retrievals over land, the state vector includes the parameters describing the surface BRDF (bidirectional reflectance distribution function) (Litvinov et al., 2011). The retrieval is based on an iterative fitting of a linearized radiative transfer model (Hasekamp and Landgraf, 2005) to the PARASOL data, using a cost function containing a misfit term between the forward model and measurement and a regularization term using a priori estimates of values of some of the retrieved parameters. The algorithm, including an application to PARASOL measurements over ocean, is described in Hasekamp et al. (2011). More recent refinements are described by Stap et al. (2015), Wu et al. (2015), Lacagnina et al. (2015), Fu and Hasekamp (2018), and Fu et al. (2020). Retrieval results from the SRON algorithm have been used for aerosol type determination by Russell et al. (2014), in studies related to aerosol absorption and direct radiative effect by Lacagnina et al. (2015) and Lacagnina et al. (2017), for aerosol–cloud interactions by Hasekamp et al. (2019b), and for data assimilation by Tsikerdekis et al. (2021). Currently, the algorithm has been applied to 1 year (2006) of global aerosol data.
2.1.4 POLDER-GRASP
For a description of the POLDER instrument, see the previous subsection.
GRASP (Generalized Retrieval of Aerosol and Surface Properties) is a unified retrieval algorithm for atmosphere properties from diverse remote sensing observations (Dubovik et al., 2011, 2014), based on earlier work by Dubovik and King (2000), and Dubovik et al. (2002) and Dubovik et al. (2006) for AERONET inversions.
In the current paper, retrievals from the so-called “models” dataset are used. Aerosol is assumed to be an external mixture of five different aerosol components which are retrieved together with spectral parameters of surface BRDF and BPDF (bidirectional polarization distribution function). The aerosol is assumed to be a mixture of spherical and non-spherical particles. Each fraction is characterized by particle size distributions similarly to AERONET retrievals. The non-spherical component is modelled as a mixture of randomly oriented spheroids with fixed shape distribution (Dubovik et al., 2006). The details of the “models” approach are discussed by Lopatin et al. (2021) and Chen et al. (2020).The actual inversion uses multi-pixel retrieval (Dubovik et al., 2011) where horizontal pixel-to-pixel variations of aerosol and day-to-day variations of surface reflectance are enforced to be smooth.
The full archive of POLDER/PARASOL observations was retrieved using GRASP and can be found at https://www.grasp-open.com (last access: 4 May 2021). In addition to the “models” dataset, two other datasets are available (“optimized” and “high-precision”) that use slightly different assumptions in the retrieval. The detailed discussion and validation of all three 0.1∘ PARASOL/GRASP retrievals are provided by Chen et al. (2020). The “models” dataset used in this paper is considered the most applicable for a wide range of circumstances.
The dataset used in the current paper is aggregated to 1∘ spatial resolution (details are listed at https://www.grasp-open.com). The “models” dataset provides AOD and AAOD aggregated from slightly different L2 samplings: an additional minimum AOD threshold is used when aggregating AAOD. To select data of higher quality, AAOD retrievals were used only for cases with sufficient aerosol loading. The same AOD threshold is used for SSA as well. Specifically, minimum AOD (at 440 nm) thresholds of 0.3 over land and 0.02 over ocean were applied (the threshold over ocean is probably too low to assure high-quality AAOD, but higher thresholds result in significant data loss).
In the current study we prefer to use aggregated AOD and AAOD data that describe the exact same scene, and this is the case for the FL-MOC, OMAERUV and POLDER-SRON datasets mentioned earlier. For the GRASP product, we decided to assume that the aggregated SSA represents the same scene as the AOD aggregate and recalculated an AAOD from that AOD and SSA. Consequently, the AAOD product (indicated as GRASP-M) presented in this paper is different from the AAOD found in the official L3 “models” product. In situ measurements (Delene and Ogren, 2002; Andrews et al., 2011, 2017; Schmeisser et al., 2018) have suggested a change in SSA at lower AOD, so our SSA assumption may introduce additional biases. However, GRASP-M AAOD evaluated better against AERONET than “models” AAOD which showed a high bias vs. AERONET due to the aforementioned minimum AOD threshold.
For this study the L3 GRASP data were additionally filtered based on the fitting residual field, which was required to be smaller than 0.05 (over land) or 0.1 (over ocean). This subset evaluates substantially better for AOD retrievals and somewhat better for AAOD retrievals than the full dataset.
2.1.5 AERONET
AERONET (Holben et al., 1998) DirectSun V3 L2.0 (Giles et al., 2019; Smirnov et al., 2000) and Inversion V3 L1.5 and 2.0 data were downloaded from https://aeronet.gsfc.nasa.gov (last access: 4 May 2021), logarithmically interpolated to values at 550 nm and aggregated by averaging over 30 min. The DirectSun dataset contains only AOD (at multiple wavelengths). These observations are based on direct transmission measurements of solar light and have a low uncertainty of ±0.01 (Eck et al., 1999; Schmid et al., 1999), at 400 nm and larger.
The Inversion dataset contains AAOD and SSA (at multiple wavelengths) based on measurements of scattered solar light from multiple directions. This inversion uses radiative transfer calculations (Dubovik and King, 2000) and yields larger errors than the DirectSun measurements. In particular, Dubovik et al. (2000) showed that SSA errors decrease with increasing AOD and estimated 440 nm SSA errors of ±0.03 for water-soluble aerosol at 440 nm AOD ≥0.2, although for dust and biomass burning aerosol higher AOD values ≥0.5 were needed. These error estimates were based on numerical calculations. A recent in-depth estimate of the uncertainty in Inversion V3 data (Sinyuk et al., 2020) suggested those thresholds to be 440 nm AOD >0.3 and ≥0.45, respectively. For an examination of the impact of geometrical configuration on SSA observations, see Torres et al. (2014). Schafer et al. (2014) showed that AERONET SSA retrievals were lower by 0.011 than flight campaign data (on average). Andrews et al. (2017) also compared flight campaign measurements to AERONET SSA and found that the data were usually within the expected errors, although at low AOD ≤0.2 significantly lower SSA values were observed by AERONET. A confounding issue for the evaluation of SSA (or, for that matter, AAOD) datasets is that there is no established gold standard.
The Inversion dataset also contains AOD (from DirectSun retrievals) which is actually used in the inversion. Here we only use those AOD values in the Inversion dataset that have corresponding AAOD and SSA values, so that aggregate values always describe the same scene.
Inversion L2.0 is a subset of L1.5 (which contains almost 30 times more observations), based on further cloud screening and the requirement that AOD at 440 nm ≥0.4. This last criterion results in a minimum AOD at 550 nm of 0.25 in the Inversion L2.0 product.
Since an individual AERONET site is not necessarily representative of a grid box, satellite evaluation may be negatively affected. To select only sites with high representativity, we use a list published in Kinne et al. (2013) as described in Schutgens et al. (2020), where we also tested this representativity (using 14 satellite AOD products). The Kinne list was developed with the AERONET DirectSun product (i.e. AOD) in mind, but a high-resolution modelling study by Schutgens (2020) suggests that spatial representativity for AOD and AAOD observations can differ substantially for individual sites. We chose to use the Kinne list because it also includes information on maintenance quality, likely more important for Inversion than DirectSun retrievals.
2.1.6 How independent are these satellite products?
An interesting question is how independent these satellite products are.
The GRASP and SRON algorithms are independent retrieval codes with many specific differences in the implementation. First, in the present study POLDER-SRON retrieves parameters of bimodal lognormal size distribution and complex refractive index for each size mode, while POLDER-GRASP-M retrieves the concentrations of five aerosol components with assumed properties of each component (Chen et al., 2020; Lopatin et al., 2021). Second, GRASP and SRON use the same mathematical function for the BRDF over land (Litvinov et al., 2011) but estimate the parameters to this function independently. In both algorithms, aerosol and surface properties are estimated simultaneously. Third, there are significant differences in the use of a priori constraints. POLDER-SRON follows Phillips–Tikhonov regularization (Phillips, 1962; Tikhonov, 1963) including a priori estimates for most of the retrieved state vector parameters (a globally constant value is used) and a flexible strength of the regularization term. The GRASP algorithm is based on the least squares multi-term approach (see Dubovik et al., 2011) and uses several a priori constraints simultaneously. Specifically, GRASP “models” uses smoothness constraints on the spectral dependence of surface BRDF parameters. Fourth, the SRON algorithm retrieves from measurements of individual pixels, while the GRASP algorithm retrieves from measurements of multiple pixels simultaneously, applying spatio-temporal constraints in the process. For example, over land constraints were used to limit the temporal variability of retrieved BRDF parameters as well as the spatial variability of aerosol retrieved parameters (see Dubovik et al., 2011, and Chen et al., 2020).
The FL-MOC product uses OMAERUV AAOD as input over land, but FL-MOC only uses OMAERUV AAOD as an a priori estimate and assigns this a sizeable uncertainty. CALIOP backscatter is expected to provide a constraint on SSA and consequently AAOD. As a matter of fact, our analysis shows that FL-MOC and OMAERUV exhibit rather low correlations for AAOD (and SSA). This suggests that the OMAERUV a priori estimate does not lead to a strong dependency of FL-MOC on OMAERUV. On the other hand, it also suggests that at least one of these products contains sizeable errors.
To evaluate and intercompare the remote sensing datasets, they will need to be collocated in time and space to reduce representation errors (Colarco et al., 2014; Schutgens et al., 2016a, 2017). In practice this collocation is another aggregation (performed for each dataset individually) to a spatio-temporal grid with slightly coarser temporal resolution (1 or 3 h; the spatial grid box size remains ). This is followed by a masking operation that retains only aggregated data if they exist in the same grid boxes for all involved datasets. More details can be found in Appendix A.
We need to allow some flexibility in the time separation between data (here 3 h) to ensure sufficient numbers of collocated data pairs for further analysis. Schutgens et al. (2020) showed that shorter time separations greatly limited the number of pairs but did not substantially alter the correlation of satellite AOD with AERONET. On the other hand, longer time separations appear to negatively affect the correlation of satellite AAOD with AERONET (see Fig. 2). The analysis shows that satellite AOD correlation with AERONET Inversion data slowly decreases as the collocation criterion is relaxed from 3 to 24 h. However, satellite AAOD shows a sharp drop in correlation with AERONET at 6 h (OMAERUV is the exception; the correlation is already low and barely changes). We surmise this is due to plumes of absorbing aerosol drifting over the sites, requiring tight temporal constraints on collocation. Consequences of this finding will be further discussed in Sect. 7.
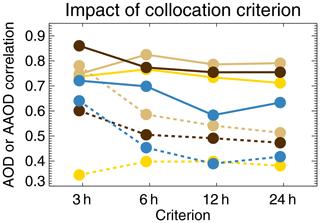
Figure 2Correlation of satellite AOD (solid) and AAOD (dashed) with AERONET Inversion L2.0 data, as a function of a temporal collocation criterion. Colours indicate the satellite product (see also Fig. 1). Satellite products were individually collocated with AERONET.
As the FL-MOC dataset, based on CALIOP measurements, is smaller than the other satellite datasets, we were compelled to collocate FL-MOC with AERONET within 2∘ instead of 1∘. Even so, the data count for the FL-MOC evaluation is low.
After spatio-temporally collocating two or more datasets, the data may be further averaged in space and/or time for analysis purposes. Spatio-temporally averaged SSA is always derived from averaged AOD and AAOD:
During the evaluation of products with AERONET, a distinction will be made between either land or ocean grid boxes in the common grid. A high-resolution land mask was used to determine which grid box contained at most 30 % land (designated an ocean box) or water (designated a land box). Most ocean boxes with AERONET observations will be in coastal regions, with some over isolated islands.
3.1 Taylor diagrams
A suitable graphic for displaying multiple datasets' correspondence with a reference dataset (truth) is provided by the Taylor diagram (Taylor, 2001). In this polar plot, each data point (r,ϕ) shows basic statistical metrics for an entire dataset. The distance from the origin (r) represents the internal variability (standard deviation) in the dataset. The angle ϕ through which the data point is rotated away from the horizontal axis represents the correlation with the reference dataset, which is conceptually located on the horizontal axis at radius 1 (i.e. every distance is normalized to the internal variability of the reference dataset). It can be shown (Taylor, 2001) that the distance between the point (r,ϕ) and this reference data point at (1,0) is a measure of the root mean square error (RMSE, unbiased). A line extending from the point (r,ϕ) is used to show the bias versus the reference dataset (positive for pointing clockwise). The distance from the end of this line to the reference data point is a measure of the root mean square difference (RMSD, no correction for bias).
3.2 Uncertainty analysis using bootstrapping
Our estimates of error metrics are inherently uncertain due to finite sampling. If the sampled error distribution is sufficiently similar to the underlying true error distribution, bootstrapping (Efron, 1979) can be used to assess uncertainties in for example biases or correlations due to finite sample size. Bootstrapping uses the sampled distribution to generate a large number of synthetic samples by random draws with replacement. For each of these synthetic samples, a bias can be calculated, and the distribution of these biases provides measures of the uncertainty, e.g. a standard deviation, in the bias due to statistical noise. Bootstrapping has been shown to be reliable even for relatively small sample sizes (that is, the size of the original sample and not the number of bootstraps; see Chernick, 2008). In this study, the uncertainty bars in some figures were generated by bootstrap analysis.
If the sampled error distribution is different from the true error distribution, bootstrapping will likely underestimate uncertainties. Sampled error distributions may be different from the true error distribution because the act of collocating satellite and AERONET data favours certain conditions. For example, the effective combination of two cloud screening algorithms (one for the satellite product and the other for AERONET) may favour clear-sky conditions and reduce our sampling of errors due to cloud contamination. This uncertainty due to sampling is unfortunately hard to assess (see e.g. Schutgens et al., 2020).
As an example of uncertainty due to sampling, we present Fig. 3, in which an evaluation of the current satellite AOD data with Inversion L2.0 data (only those AOD values that have corresponding AAOD inversions, which constrains AOD at 440 nm >0.4) shows substantial shifts compared to DirectSun L2.0. As the uncertainty ranges indicate, the changes in biases are not due to statistical noise. Neither is this due to differences in collocated DirectSun and Inversion L2.0 AOD values, which agree very well. Rather, the issue is that AERONET Inversion data are an unrepresentative subsample of the DirectSun data (Inversion data are skewed to high AOD). It is unclear what this means for the AAOD and SSA evaluation, but readers should be aware of this unaccounted-for sampling issue that may introduce biases.
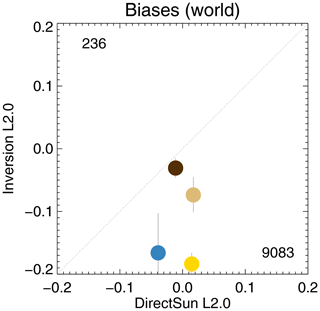
Figure 3Global biases in four satellite AOD datasets depending on the chosen reference dataset (DirectSun or Inversion). Colours indicate the satellite product (see also Fig. 1). Numbers in upper left and lower right corner indicate the amount of collocated data, averaged over all products. Error bars indicate a 5 %–95 % uncertainty range based on a bootstrap analysis (see Sect. 3.2). Satellite products were individually collocated with AERONET within 3 h.
3.3 Error metrics for evaluation
We will use the usual global error statistics (bias, standard deviation, Pearson correlation, regression slopes), treating all data as independent. Regression slopes were calculated with a robust ordinary least squares regressor (OLS bisector from the IDL sixlin
function, Isobe et al., 1990). This regressor is recommended when there is no proper understanding of the errors in the independent variable (see also Pitkänen et al., 2016).
Multi-year averages of satellite AAOD and their differences are shown in Fig. 4. The AAOD maps can only be compared with caution, as they are derived from products with different temporal sampling. The differences, on the other hand, are based on collocated data and confirm major features. The products all agree on a major AAOD hotspot (likely) from the African savannah biomass burning. Three products agree on AAOD hotspots in China and India, which are known polluted regions. (OMAERUV, which is relatively featureless, is the exception. We surmise this is due to the large pixel size of the OMI instrument (see Table 1), which will not resolve small-scale structure in AAOD. The existence of such small-scale structure was inferred from Fig. 2.) POLDER-GRASP-M and OMAERUV show a clear AAOD hotspot due to Amazonian biomass burning. POLDER-GRASP-M estimates relatively high values over land and the ocean at high northern latitudes. OMAERUV shows relatively low AAOD over land but high over the entire ocean. FL-MOC clearly estimates higher AAOD over the Sahara than either POLDER-GRASP-M or OMAERUV. POLDER-SRON estimates relatively high AAOD over the Rocky Mountains, the Andes and Australia. Unfortunately, even in multi-year averages significant differences in regional AAOD between the products are observed, in excess of 50 %. Figure S1 in the Supplement shows the corresponding SSA maps. As expected, POLDER-GRASP-M has relatively low SSA and OMAERUV relatively high SSA over land. FL-MOC has the highest SSA over ocean of all products. As the satellite AOD values are fairly similar, lower values of AAOD translate into higher values of SSA.
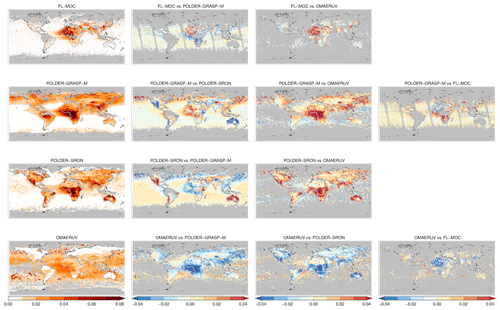
Figure 4Global maps of AAOD for four products and their differences. AAOD differences are based on collocated data (within 3 h). Note that the products are available for different years; e.g. POLDER-SRON and FL-MOC do not overlap. No minimum AOD was required.
One caveat is that AAOD and SSA retrievals are likely to be better (more accurate and precise) at high AOD. In the above analysis, no account was taken of AOD levels, and the products were discussed as they are. The impact of AOD will be discussed later, when discussing the evaluation with AERONET in Sect. 5.2 and the satellite intercomparison in Sect. 6.
Taylor plots of the performance of the satellite products are shown in Fig. 5. Satellite AOD is evaluated against AERONET DirectSun L2.0. Satellite AAOD and SSA are evaluated against AERONET Inversion L2.0 (which constrains AOD at 440 nm >0.4 and provides much less data than DirectSun). All products show high correlation with AERONET AOD (r≥0.76), although the correlations found are lower than those found in Schutgens et al. (2020) for several MODIS Aqua products (0.87–0.88). Correlations for AAOD and SSA are lower than for AOD, suggesting that it is more challenging to retrieve absorbing qualities.
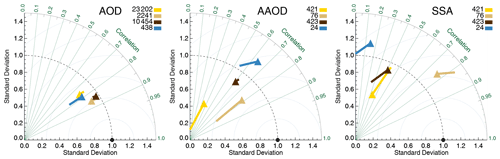
Figure 5Taylor diagrams (for an explanation, see Sect. 3.1) for the satellite products. AOD is evaluated against AERONET DirectSun L2.0, and AAOD and SSA are evaluated against AERONET Inversion L2.0. Colours indicate the satellite product (see also Fig. 1), and numbers next to coloured blocks indicate the amount of collocated data. The lines extending from the data points indicate the bias. Products were individually collocated with AERONET within 3 h.
Interestingly, POLDER-SRON's SSA correlates significantly better with AERONET than POLDER-GRASP-M's SSA, but this is a sampling effect: once both products are collocated together, POLDER-GRASP-M's SSA correlation with AERONET increases from 0.41 to 0.69. The explanation for this is not entirely clear, although it turns out that POLDER-GRASP-M evaluates more poorly with AERONET for 2010 than for 2006 and 2008 (POLDER-SRON is currently limited to 2006; see Table 2.1). Although the poorer evaluation for 2010 can be seen in AOD, AAOD and SSA, it is only statistically significant for SSA.
The impact of statistical noise on the AAOD evaluation is explored in Fig. 6. Using a bootstrapping technique, the spread in correlation and standard deviation were explored. For most datasets, the results seem fairly robust, except for FL-MOC, which yielded only 24 data points. A proper intercomparison of products requires collocation (of all the satellite data), which reduces available cases even further. Figure S2 shows that results are not very different from Fig. 5, but the statistical noise increases substantially. The sampling noise on such a small subset should be even larger (see also Fig. 3 and Schutgens et al., 2020). For a sense of perspective, 48 data points represents less than 0.0008 % of the total POLDER-GRASP-M data amount used in this paper.
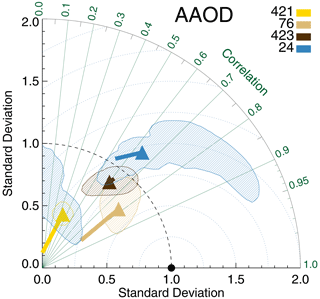
Figure 6Impact of statistical noise on the correlation and internal variability of satellite AAOD products, using bootstrapping. Shaded regions indicate a 5 %–95 % uncertainty range of correlation and standard deviation (uncertainty in bias is not shown). Colours indicate the satellite product (see also Fig. 1), and numbers next to coloured blocks indicate the amount of collocated data. Satellite products were individually collocated with AERONET Inversion L2.0 within 3 h.
5.1 Evaluation and intercomparison of AOD
In Fig. 7, we provide more detail on the satellite AOD products and their evaluation against AERONET DirectSun L2.0 AOD. In the central column, we show the products themselves, averaged over 1, 2 or 3 year(s), depending on availability (see Table 1). Note that the products exist for different years, and even for the same years products will have different temporal samplings, so comparisons should be made with caution (Colarco et al., 2014; Schutgens et al., 2016a). In the left and right column, we show satellite data collocated with AERONET. On the left-hand side is a scatter plot of the data (with associated statistics provided), and on the right-hand side is a map of multi-year difference with AERONET (provided at least 32 data points were available per site).
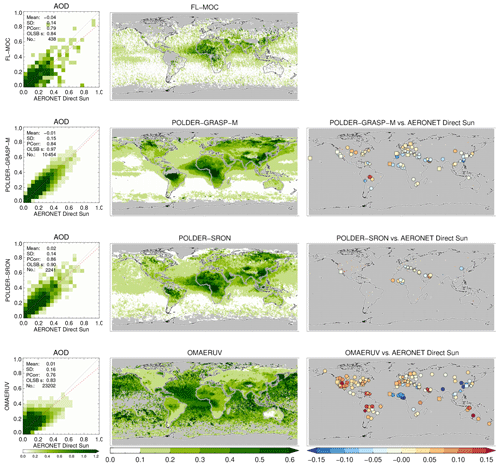
Figure 7For the four satellite products the following are shown: a scatter plot of individual super-observations versus AERONET (the colour indicates amount of data in percentages; see Sect. 3.3 for an explanation of the metrics), a global map of the 3-year AOD average and a global map of the 3-year AOD difference average with AERONET (if the site provided at least 32 observations; land sites are circles, ocean sites are squares, and diamonds are the remainder). For FL-MOC, insufficient data prevent the plotting of a difference map. Products were individually collocated with AERONET DirectSun L2.0 within 3 h.
The scatter plots show good correlation with AERONET. The POLDER products show higher correlations and slopes closer to one (1) than FL-MOC and OMAERUV. Nevertheless, differences in evaluation seem rather small, which unfortunately cannot be said for the global distributions of AOD. POLDER-GRASP-M has rather high AOD over land, and OMAERUV has rather high AOD over ocean (note that the satellite data themselves are not collocated). The multi-year differences with AERONET suggest that OMAERUV overestimates everywhere except in some regions with strongly absorbing aerosol. An intercomparison of satellite AOD with Aqua-DT (Dark Target) is presented in Fig. S3 and suggests typically higher estimates over (Southern Hemisphere) land for the POLDER products and over ocean for OMAERUV. Note that Aqua-DT is not without significant regional biases (see Schutgens et al., 2020).
Figure 8 shows results when bias (signless) and correlation per site (that yielded at least 32 collocations) are averaged over all sites for each satellite product. The same 52 sites are used for all datasets, although each product is individually collocated with AERONET. For FL-MOC, no site provided at least 32 observations, and this is not included in the analysis. For POLDER-SRON, only 18 sites provided at least 32 collocated observations, and this was similarly excluded. As was also shown in Schutgens et al. (2020), OMAERUV shows rather large biases compared to the other AOD products. POLDER-GRASP-M, on the other hand, shows the smallest bias. The filtering of GRASP retrievals described in Sect. 2.1 plays a significant role in this result (without filtering, POLDER-GRASP-M shows a bias twice as large).
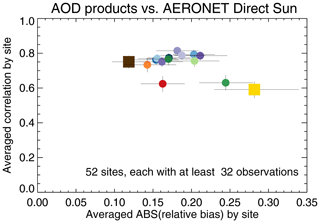
Figure 8Evaluation of satellite products with AERONET per site, averaged over all sites. Squares indicate products used in the present study, and circles indicate products used in Schutgens et al. (2020). Error bars indicate a 5 %–95 % uncertainty range based on a bootstrap analysis (see Sect. 3.2) of sample size 1000 (the bootstrap was performed on the contributing AERONET sites). Colours indicate the satellite product (see also Fig. 1). Products were individually collocated with AERONET DirectSun L2.0 within 3 h. All products use the same sites, each of which produced at least 32 collocations. POLDER-SRON and FL-MOC were excluded from this analysis due to lack of data.
5.2 Evaluation of AAOD and SSA
Figure 9 provides more detail on the evaluation of satellite (A)AOD and SSA products against AERONET Inversion L2.0 (which constrain AOD at 440 nm >0.4). In the first three columns, we show scatter plots for respectively AOD, AAOD and SSA. In the last column we show SSA differences with AERONET as a function of AERONET AOD (Inversion L1.5). All products underestimate AERONET AOD and AAOD, although only by a small amount in the case of POLDER-GRASP-M. More importantly, AAOD correlations can be as low as 0.34 (OMAERUV), and the regression slope can deviate substantially from 1 (0.6 for OMAERUV). In contrast, some products underestimate SSA, while others overestimate it. Due to data sparsity (e.g. for POLDER-GRASP-M, the count dropped from 10 454 to 423), it is not possible to do an analysis for each AERONET site (as was done for AOD) and see how the global bias relates to regional biases. The bootstrap analysis suggest that results are fairly robust against statistical noise (except FL-MOC; see also Fig. 6).
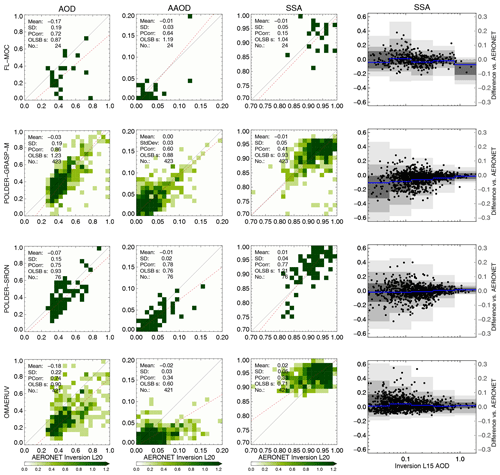
Figure 9Evaluation of super-observations of AOD, AAOD and SSA for the satellite products. SSA is also evaluated as a function of AOD (binned). In the three leftmost figures, the colour indicates amount of data in percentages; for an explanation of the metrics, see Sect. 3.3. The rightmost column uses two vertical axes: the left y axis is used for individual data points (subsampled), and the right y axis is used for the greyscale distribution (9 %, 25 %, 50 %, 75 % and 91 % quantiles of the differences) and the median difference (blue line). Products were individually collocated with AERONET Inversion L2.0 within 3 h, except for the rightmost column, which used Inversion L1.5.
The rightmost column in Fig. 9 shows the SSA difference as a function of (AERONET) AOD. To ensure the largest possible range in AOD values, Inversion L1.5 instead of L2.0 is used. Especially at lower AOD, this dataset will have larger errors in AAOD and SSA than L2.0. Interestingly, as AOD increases, all satellite products seem to agree better with AERONET (for FL-MOC, the bin with largest AOD values is affected by a very low data count). This is of course as one would expect. For smaller AOD, there is increasingly more spread, although the difference distribution remains fairly unbiased. The exception is POLDER-GRASP-M, which shows increasingly lower SSA than AERONET at low AOD. We suggest that it is rather unlikely that three different satellite products have an SSA bias at low AOD that is similar to AERONET (and hence show no bias in the difference with AERONET) and that this low bias in POLDER-GRASP-M analysis is real. However, a better understanding of the nature of errors (bias vs. random) in AERONET SSA at low AOD is desirable.
Summarizing, there is skill in satellite AAOD and SSA, but compared to AOD the correlations with AERONET are substantially lower. POLDER-SRON is the exception, with similar and fairly high correlations (∼0.75) for all three parameters. However, it seems to underestimate AAOD by ∼25 % at high AAOD (slope of 0.76 in the AAOD scatter plot). OMAERUV appears to show the largest deviations from AERONET (low correlations and slopes), but its overall error statistics (mean and standard deviation) is not too different from the other products. Results for FL-MOC may be a statistical fluke due to the low data count. POLDER-GRASP-M shows quite high correlations for AOD (0.86) and AAOD (0.6) with reasonable slopes but has a very low correlation with AERONET for SSA (0.41), but this seems to depend strongly on sampling as discussed at the start of this section. In addition, it appears to systematically underestimate SSA at low AOD. Yet another aspect to this dataset (not visible in any of the analysis shown) is that it appears to have a hard SSA cut-off as SSA values larger than 0.99 do not occur.
A profound problem is the paucity of data. Even for POLDER-GRASP-M, we can only evaluate its performance (against AERONET) for less than 0.006 % of the total number of available observations. Is this sufficient to make meaningful statements about the performance of a product at large? In Schutgens et al. (2020), we showed that the process of collocation can skew error statistics (by changing the sampling) to the point that it becomes hard to meaningfully distinguish the performance of several products. That study was done for AOD, which allows much higher numbers of collocated data with AERONET than AAOD.
To elucidate this, we compare the difference in SSA between the two POLDER products (collocated within 3 h, considering AOD ≥0.25 only) for three different samplings. First, we look at global POLDER SSA statistics. Secondly, we look at POLDER SSA statistics over AERONET sites only. Thirdly, we look at POLDER SSA statistics that are collocated with AERONET observations. Figure 10 shows the associated difference distributions. Using various non-parametric statistical tests (Mann–Whitney U, Student's t, Kolmogorov–Smirnov), we can show that the distribution means for the first and third sampling are significantly different. Not only that, but the mean difference in SSA for the first sampling is 2.6 times as large (−0.043 vs. −0.017) as for the third sampling. As POLDER-SRON is biased high and POLDER-GRASP-M is biased low vs. AERONET, the corollary to this is of course that at least one of the products has a larger bias vs. the truth globally than can be seen in the AERONET observations. Conversely this suggests that the AERONET Inversion dataset does not allow a truly global evaluation of satellite datasets: it provides a subsample with skewed statistics of SSA errors. Incidentally, it is the temporal subsampling enforced by collocation with AERONET observations that causes the largest shift in the difference distribution (POLDER measurements over AERONET sites show an SSA distribution similar to the global dataset). It is possible that the SSA difference is partly driven by cloud contamination, which we know is present in these satellite datasets (Schutgens et al., 2020), and may be ameliorated when a third cloud masking (from AERONET) is applied (through the collocation of data).
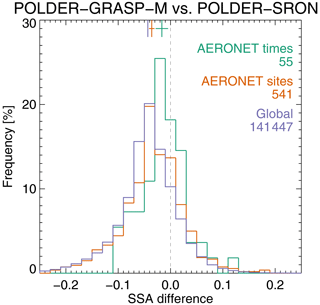
Figure 10SSA differences POLDER-GRASP-M vs. POLDER-SRON for three different samplings: all available data, data available over AERONET sites that provide Inversion L2.0 data, and data available at the times and locations of Inversion L2.0 data. The vertical coloured lines at the top show distribution means, and the short horizontal lines extending from the middle show 2σ ranges. The dashed vertical line shows zero difference. The number of collocated data is indicated in the figure as well. This analysis suggests that an evaluation with AERONET would underestimate the actual difference between the two products. In all cases, data were collocated within 3 h, and a minimum AOD >0.25 was required.
To get a better appreciation of the satellite products, we now present a global intercomparison. To start with, Fig. 11 shows SSA differences between two products as a function of their mean AOD. As in Fig. 9, these differences become smaller (i.e. show a smaller spread) at higher AOD, as expected (intercomparisons with FL-MOC are the exception). However, satellite SSA values still exhibit random differences of 0.03 or larger for AOD ⪆1, as also confirmed by the AERONET evaluation. In addition, substantial biases remain.
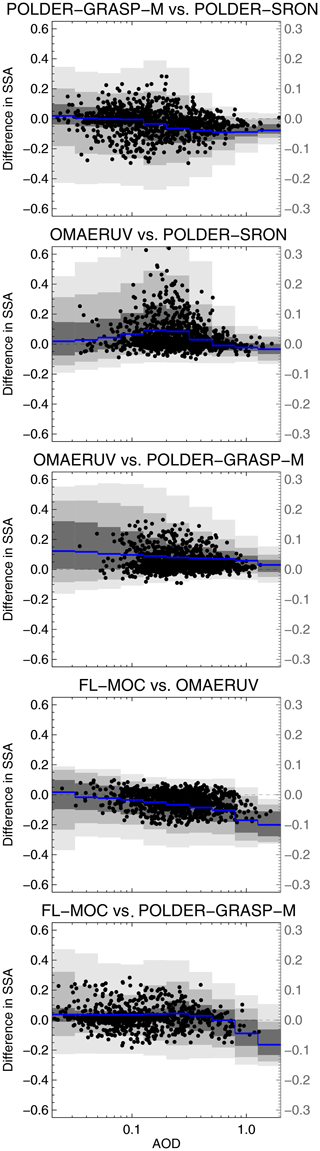
Figure 11Difference in satellite product SSA as a function of AOD (averaged over both products). Two vertical axes are used: the left y axis is used for individual data points (subsampled), and the right y axis is used for the greyscale distribution (9 %, 25 %, 50 %, 75 % and 91 % quantiles of the differences) and the median difference (blue line). Data were collocated within 3 h.
The previous analysis was global, but substantial differences can be seen between land and ocean scenes. For instance, the SSA bias between the POLDER products over land does not decrease at lower AOD but remains fairly constant. A more detailed analysis can be found in Fig. 12, which shows biases, correlations and regression slopes for different products. Unsurprisingly, correlations and slopes tend to improve with minimum AOD, while biases may remain fairly constant (POLDER products), decrease (OMAERUV vs. POLDER-GRASP-M) or even increase (FL-MOC). As a consequence it should be challenging to determine an AOD threshold above which products can be expected to perform within certain parameters. A similar analysis for AAOD can be found in Fig. S4.
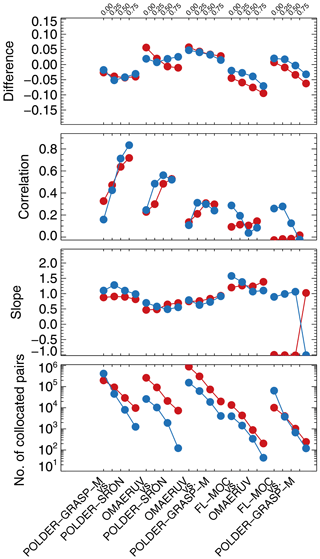
Figure 12Comparison of different pairs of satellite SSA, over land (red) and ocean (blue), for different thresholds of minimum AOD (0.0, 0.25, 0.5, and 0.75). The data were collocated within 3 h.
A final analysis concerns multi-year averages of these products. Model evaluation will be done on such averages, and it may be useful to better understand the agreement (or lack thereof) between products in that case, even though the aforementioned biases are unlikely to be much reduced. Figure 13 shows an intercomparison of three products (FL-MOC is excluded due to its low data count). The analysis shows statistics of the intercomparison of multi-year averages of SSA as a function of two thresholds: a minimum AOD and a minimum number of super-observations during 3 years (per grid box). The underlying super-observations were always collocated (to within 3 h) before temporal averaging took place. We see that, in general, correlations increase and the standard deviation in the difference decreases when either threshold increases. The improvement with increasing AOD has already been discussed and is due to better signal-to-noise conditions for the retrieval schemes. The improvement with increasing number of observations (used in the temporal averaging) can be interpreted as a significant random error in either product being lessened through averaging. In general, the AOD threshold has a more profound impact but the number of observations threshold allows more flexibility (by choosing a longer time series to work with, smaller SSA differences (up to a point) may be achieved).
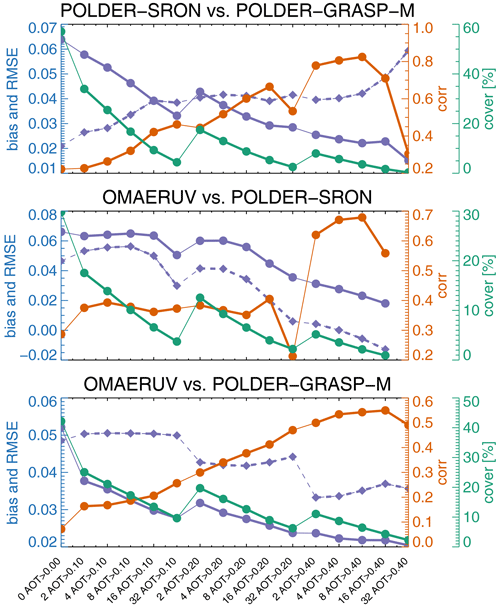
Figure 13Intercomparison of SSA satellite products after multi-year averaging, as a function of minimum AOD and number of collocated observations (thicker lines group cases with the same minimum AOD but increasing number of observations). The bias uses a dashed line and the RMSE a solid line. Cover is defined as fraction of surface area covered by data. FL-MOC is not present due to the scarcity of observations. The data were collocated within 3 h.
However, biases between products can be quite robust as is particularly clear for the POLDER products. The decreasing bias for OMAERUV vs. POLDER-SRON (and, incidentally, the sudden jump in correlation for AOD >0.4) is not really a sign of a better agreement between products at high AOD. Under these conditions, most observations come from the African dust and biomass burning regions. POLDER-SRON retrieves very reflective dust and very absorbing biomass burning aerosol, while OMAERUV retrieves fairly reflective dust and fairly absorbing biomass burning aerosol. Consequently, global SSA bias decreases due to a balancing of very different biases over these regions, while similar spatial patterns yield high correlations. Maps of the SSA difference between the POLDER products as a function of minimum AOD can be seen in Fig. S5. A higher minimum AOD mostly constrains data to a smaller portion of the globe but does not affect local biases greatly.
In this study, we evaluate several remote sensing datasets of AAOD and SSA from a variety of sensors (CALIOP on CALIPSO, OMI on Aura, POLDER on PARASOL), in preparation of an AEROCOM model evaluation. This is the first global study to intercompare satellite remotely sensed products of AAOD (and SSA).
The evaluation of the products (daily aggregates over ) is done through comparison with AERONET DirectSun (AOD) and Inversion (AAOD and SSA) observations. To minimize sampling issues, satellite products and AERONET data are collocated in time and space within 3 h and 1∘. One interesting finding is that AAOD evaluation requires a tighter temporal collocation criterion than AOD, with steep declines in correlation found for temporal collocation after 3 h or more. We interpret this to be due to absorbing aerosol primarily being found in plumes. While we do not explore this further, this high temporal variability in observed AAOD may affect model evaluation as well. It could suggest that models need emissions with diurnal profiles and output at higher frequencies than daily to obtain the best possible agreement with observations.
All satellite AOD products show significant correlation with AERONET (). Global biases are not very different from those found in an earlier study of traditional products (Schutgens et al., 2020). However, when considering typical multi-year biases per AERONET site, there is a suggestion that POLDER-GRASP-M has smaller biases than these traditional products (there is a hint this may also be true for POLDER-SRON, but the paucity of data makes this analysis less certain). In contrast, OMAERUV shows the largest (and mostly positive) biases in AOD. Compared to Aqua-DT, the four products studied in this paper tend to estimate higher AOD over most of the land.
Results for AAOD are more diverse, with generally lower correlations () than for AOD. For most products, SSA correlates significantly worse with AERONET than AAOD. All products show an improvement in SSA with regards to AERONET at higher AOD. POLDER-GRASP-M is noted for a low bias in SSA at low AOD.
The two POLDER products perform better against AERONET than the other two products, with typically (but not always) higher correlations, smaller biases and regression slopes closer to one (1) for all three parameters – AOD, AAOD and SSA. However, the dearth of measurements makes it very difficult to (1) meaningfully compare evaluation metrics amongst the products and (2) draw global conclusions. Theoretical evidence (Hasekamp and Landgraf, 2007; Hasekamp, 2010; Hasekamp et al., 2019a) suggests that retrieval schemes for absorptive properties will benefit from using polarization measurements at multiple view angles, which would support the idea that the POLDER products perform better. In addition, the OMAERUV product is based on measurements from a sensor with substantially larger pixels than POLDER and will struggle to resolve the fine-scale structure of aerosol plumes.
An intercomparison of multi-year satellite AAOD and SSA suggests significant biases across the globe. Differences of 50 % in multi-year averages of AAOD are not unusual. OMAERUV shows lower AAOD over land than the other products but slightly higher AAOD over ocean. FL-MOC shows significantly higher AAOD over the Sahara, and POLDER-GRASP-M is noted for a high AAOD at high northern latitudes, both over land and ocean. POLDER-SRON has much higher AAOD than the other products over high-altitude regions. Many of these regions are unfortunately poorly instrumented with AERONET sites. Satellite SSA does agree better at high AOD, as was also observed for AERONET, although the dearth of data means this can not be firmly concluded for FL-MOC. However, correlations for super-observations are often lower than 0.6, even at high AOD (0.75). Over ocean, SSA products tend to correlate better than over land. The two POLDER products correlate better than any other satellite pair ( over ocean for AOD >0.75). In addition to high AOD, we show that temporal averaging also improves agreement between satellite products, although it is not possible to give recommendations that work well with all products and for all regions. Even so, biases between products exist at high AOD after substantial temporal averaging.
Most surprisingly, POLDER-GRASP-M and POLDER-SRON show a fairly systematic difference in SSA (−0.04), independent of AOD (there are regional variations). For low AOD (<0.1) cases over ocean, this systematic difference becomes small in the global average because of two opposite biases organized roughly by hemisphere (see also Fig. S1). Identifying the cause of this bias may lead to substantial improvements of both products (or at least one of them). Based on a comparison with AERONET data, we suggest that cloud contamination is a possible candidate.
Throughout the paper, we have given examples of how limited sampling of observations (especially AERONET) constrains our ability to understand the true error statistics of satellite AAOD and SSA. The most prominent example is a much reduced systematic difference (−0.017) between POLDER-GRASP-M and POLDER-SRON SSA as seen in an evaluation with AERONET Inversion L2.0 observations, as compared to the global satellite dataset (−0.04). This suggest that biases inferred from an AERONET evaluation will be smaller than those actually present in the satellite products. To increase available SSA observations, one could use Inversion L1.5 data (which includes SSA at low AOD) and sample them to L2.0 AOD measurements (which, unlike SSA, exist at low AOD), thereby benefitting from the better L2.0 cloud screening. Especially if follow-up studies can show that inversion errors at individual sites behave as random errors (amenable to temporal averaging) and not systematic biases, such an intermediate product might be very useful.
This paper is one part of a two-part study into the use of satellite AAOD and SSA for aerosol model evaluation. In the companion paper, we use the datasets introduced in the current paper to evaluate AEROCOM (Aerosol Comparisons between Observations and Models) models. It turns out that robust and consistent evaluation of the models is possible, notwithstanding the biases in the satellite data we have detailed in the current paper. The main reason seems to be that model biases (and the diversity in those biases) are even larger than satellite biases. Hence these satellite AAOD and SSA products are very useful: in regions with AERONET sites, they provide spatial detail lacking in a surface network; in regions without AERONET sites, they are the only datasets of observed AAOD and SSA available.
The aggregation of satellite L2 products into super-observations in this paper and the subsequent collocation of different datasets for intercomparison and evaluation used the following scheme.
Assume a homogenous L2 dataset with times and geolocations and observations of AOD and AAOD. Homogenous means that AOD and AAOD are available for the same times, geolocations and wavelengths. Each observation has a known spatio-temporal footprint; e.g. in the case of satellite L2 retrievals that would be the L2 retrieved pixel size and the short amount of time (less than a second) needed for the original measurement.
Satellite L2 data are aggregated into super-observations as follows. A regular spatio-temporal grid is defined as in Fig. A1. The spatio-temporal size of the grid boxes (here min) exceeds that of the footprint of the L2 data that will be aggregated. All observations are assigned to a spatio-temporal grid box according to their times and geolocations. Once all observations have been assigned, observations are averaged by grid box. It is possible to require a minimum number of observations to calculate an average. Finally, all grid boxes that contain observations are used to construct a list of super-observations as in Fig. A2. Only times and geolocations with aggregated observations are retained. As the original L2 dataset was homogeneous, so is the resulting L3 dataset.
Station data are similarly aggregated over min. Point observations will suffer from spatial representativeness issues (Sayer et al., 2010; Virtanen et al., 2018; Schutgens et al., 2016b), but the representativity of AERONET sites for grid boxes is fairly well understood (Schutgens, 2020; see also Sect. 2.1.5). These aggregated L3 AERONET data will also be called super-observations.
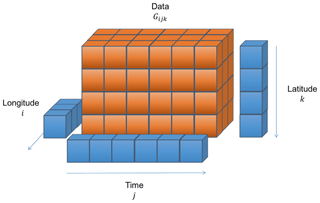
Figure A1A regular spatio-temporal grid in time, longitude and latitude. Such a grid is used for the aggregation operation that is at the heart of the collocation procedure used in this paper. Grid boxes may either contain data or be empty. Note that data may refer to any combination of observations, e.g. AOD at multiple wavelengths or AOD and AAOD at 550 nm. However, the dataset is homogenous. Reproduced from Watson-Parris et al. (2016).
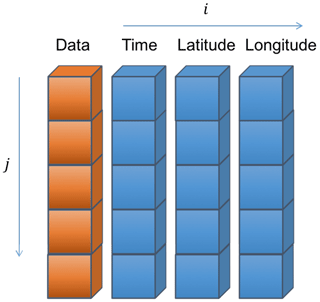
Figure A2A list of data. Such a list is the primary data format used for the observations in this paper. Note that data may refer to any combination of observations, e.g. AOD at multiple wavelengths or AOD and AAOD at 550 nm. However, the dataset is homogenous. Reproduced from Watson-Parris et al. (2016).
Different datasets of super-observations can be collocated in a very similar way. Again a regular spatio-temporal grid is defined as in Fig. A1 but now with grid boxes of larger temporal extent (typically h). Because this temporal extent is short compared to satellite revisit times, either a single satellite super-observation or none is assigned to each grid box. A single AERONET site however may contribute up to six super-observations per grid box (in which case they are averaged). After two or more datasets are aggregated individually, only grid boxes that contain data for both datasets will be used to construct two lists of aggregated data as in Fig. A2. Those two lists will have identical size and ordering of times and geolocations and are called collocated datasets. By choosing a larger temporal extent of the grid box, the collocation criterion can be relaxed.
As the super-observations are on a regular spatio-temporal grid and collocation requires further aggregation to another regular but coarser grid, the whole procedure is very fast. It is possible to collocate seven products from afternoon platforms over 3 years using an IDL (Interactive Data Language) code and a single processing core in just 30 min (Schutgens et al., 2020). This greatly facilitates sensitivity studies.
Starting from super-observations, a 3-year average can easily be constructed by once more performing an aggregation operation but now with a grid box of year. If two collocated datasets are aggregated in this fashion, their 3-year average can be compared with minimal representation errors. This allows us to construct global maps of for example a multi-year AOD difference between two sets of super-observations.
A software tool (the Community Intercomparison Suite) is available for these operations at http://www.cistools.net (last access: 20 December 2019) and is described in great detail in Watson-Parris et al. (2016).
All remote sensing data are freely available from the author upon request. The analysis code was written in IDL and is available from the author upon request.
The supplement related to this article is available online at: https://doi.org/10.5194/acp-21-6895-2021-supplement.
NS designed the study, with the help of GdL, TP, SK, MS and PS, and carried it out. OD, OH, OT, HJ, PJTL, PL, JR and YS provided the remote sensing data. NS prepared the manuscript, with OD (Sect. 2.1.4), OH (Sect. 2.1.3), OT (Sect. 2.1.2) and YS (Sect. 2.1.1) writing subsections. All authors provided feedback on earlier versions of the paper.
The authors declare that they have no conflict of interest.
We thank the principal investigator(s) and co-investigator(s) and their staff for establishing and maintaining the many AERONET sites used in this investigation. The figures in this paper were prepared using David W. Fanning's Coyote Library for IDL. Nick Schutgens thanks Tom Eck, Greg Schuster and Kostas Tsigaridis for insightful discussions on the use of AERONET observations. We would also like to thank four anonymous reviewers for attentive reading of our manuscript and many useful comments.
This research has been supported by the Nederlandse Organisatie voor Wetenschappelijk Onderzoek (grant no. VICI 016.160.324).
This paper was edited by Matthias Tesche and reviewed by four anonymous referees.
Ahn, C., Torres, O., and Jethva, H.: Assessment of OMI near-UV aerosol optical depth over land, J. Geophys. Res.-Atmos., 119, 2457–2473, https://doi.org/10.1002/2013JD020188, 2014. a, b
Albrecht, B. A.: Aerosols, cloud microphysics, and fractional cloudiness, Science, 245, 1227–1230, https://doi.org/10.1126/science.245.4923.1227, 1989. a
Allen, R. J., Sherwood, S. C., Norris, J. R., and Zender, C. S.: Recent Northern Hemisphere tropical expansion primarily driven by black carbon and tropospheric ozone, Nature, 485, 350–354, https://doi.org/10.1038/nature11097, 2012. a
Andrews, E., Ogren, J. A., Bonasoni, P., Marinoni, A., Cuevas, E., Rodríguez, S., Sun, J. Y., Jaffe, D. A., Fischer, E. V., Baltensperger, U., Weingartner, E., Coen, M. C., Sharma, S., Macdonald, A. M., Leaitch, W. R., Lin, N., Laj, P., Arsov, T., Kalapov, I., Jefferson, A., and Sheridan, P.: Climatology of aerosol radiative properties in the free troposphere, Atmos. Res., 102, 365–393, https://doi.org/10.1016/j.atmosres.2011.08.017, 2011. a
Andrews, E., Ogren, J. A., Kinne, S., and Samset, B.: Comparison of AOD, AAOD and column single scattering albedo from AERONET retrievals and in situ profiling measurements, Atmos. Chem. Phys., 17, 6041–6072, https://doi.org/10.5194/acp-17-6041-2017, 2017. a, b, c
Angstrom, B. A.: Atmospheric turbidity, global illumination and planetary albedo of the earth, Tellus, 14, 435–450, 1962. a
Ballester, J., Burns, J. C., Cayan, D., Nakamura, Y., Uehara, R., and Rodó, X.: Kawasaki disease and ENSO-driven wind circulation, Geophys. Res. Lett., 40, 2284–2289, https://doi.org/10.1002/grl.50388, 2013. a
Beelen, R., Raaschou-Nielsen, O., Stafoggia, M., Andersen, Z. J., Weinmayr, G., Hoffmann, B., Wolf, K., Samoli, E., Fischer, P., Nieuwenhuijsen, M., Vineis, P., Xun, W. W., Katsouyanni, K., Dimakopoulou, K., Oudin, A., Forsberg, B., Modig, L., Havulinna, A. S., Lanki, T., Turunen, A., Oftedal, B., Nystad, W., Nafstad, P., De Faire, U., Pedersen, N. L., Östenson, C.-G., Fratiglioni, L., Penell, J., Korek, M., Pershagen, G., Eriksen, K. T., Overvad, K., Ellermann, T., Eeftens, M., Peeters, P. H., Meliefste, K., Wang, M., Bueno-de Mesquita, B., Sugiri, D., Krämer, U., Heinrich, J., de Hoogh, K., Key, T., Peters, A., Hampel, R., Concin, H., Nagel, G., Ineichen, A., Schaffner, E., Probst-Hensch, N., Künzli, N., Schindler, C., Schikowski, T., Adam, M., Phuleria, H., Vilier, A., Clavel-Chapelon, F., Declercq, C., Grioni, S., Krogh, V., Tsai, M.-Y., Ricceri, F., Sacerdote, C., Galassi, C., Migliore, E., Ranzi, A., Cesaroni, G., Badaloni, C., Forastiere, F., Tamayo, I., Amiano, P., Dorronsoro, M., Katsoulis, M., Trichopoulou, A., Brunekreef, B., and Hoek, G.: Effects of long-term exposure to air pollution on natural-cause mortality: an analysis of 22 European cohorts within the multicentre ESCAPE project, Lancet, 383, 785–795, https://doi.org/10.1016/S0140-6736(13)62158-3, 2013. a
Bond, T. C., Doherty, S. J., Fahey, D. W., Forster, P. M., Berntsen, T., Deangelo, B. J., Flanner, M. G., Ghan, S., Kärcher, B., Koch, D., Kinne, S., Kondo, Y., Quinn, P. K., Sarofim, M. C., Schultz, M. G., Schulz, M., Venkataraman, C., Zhang, H., Zhang, S., Bellouin, N., Guttikunda, S. K., Hopke, P. K., Jacobson, M. Z., Kaiser, J. W., Klimont, Z., Lohmann, U., Schwarz, J. P., Shindell, D., Storelvmo, T., Warren, S. G., and Zender, C. S.: Bounding the role of black carbon in the climate system: A scientific assessment, J. Geophys. Res.-Atmos., 118, 5380–5552, https://doi.org/10.1002/jgrd.50171, 2013. a
Brioude, J., Cooper, O. R., Feingold, G., Trainer, M., Freitas, S. R., Kowal, D., Ayers, J. K., Prins, E., Minnis, P., McKeen, S. A., Frost, G. J., and Hsie, E.-Y.: Effect of biomass burning on marine stratocumulus clouds off the California coast, Atmos. Chem. Phys., 9, 8841–8856, https://doi.org/10.5194/acp-9-8841-2009, 2009. a
Brunekreef, B. and Holgate, S. T.: Air pollution and health, Lancet, 360, 1233–1242, https://doi.org/10.1016/S0140-6736(02)11274-8, 2002. a
Chen, C., Dubovik, O., Henze, D. K., Lapyonak, T., Chin, M., Ducos, F., Litvinov, P., Huang, X., and Li, L.: Retrieval of desert dust and carbonaceous aerosol emissions over Africa from POLDER/PARASOL products generated by the GRASP algorithm, Atmos. Chem. Phys., 18, 12551–12580, https://doi.org/10.5194/acp-18-12551-2018, 2018. a
Chen, C., Dubovik, O., Henze, D. K., Chin, M., Lapyonok, T., Schuster, G. L., Ducos, F., Fuertes, D., Litvinov, P., Li, L., Lopatin, A., Hu, Q., and Torres, B.: Constraining global aerosol emissions using POLDER/PARASOL satellite remote sensing observations, Atmos. Chem. Phys., 19, 14585–14606, https://doi.org/10.5194/acp-19-14585-2019, 2019. a
Chen, C., Dubovik, O., Fuertes, D., Litvinov, P., Lapyonok, T., Lopatin, A., Ducos, F., Derimian, Y., Herman, M., Tanré, D., Remer, L. A., Lyapustin, A., Sayer, A. M., Levy, R. C., Hsu, N. C., Descloitres, J., Li, L., Torres, B., Karol, Y., Herrera, M., Herreras, M., Aspetsberger, M., Wanzenboeck, M., Bindreiter, L., Marth, D., Hangler, A., and Federspiel, C.: Validation of GRASP algorithm product from POLDER/PARASOL data and assessment of multi-angular polarimetry potential for aerosol monitoring, Earth Syst. Sci. Data, 12, 3573–3620, https://doi.org/10.5194/essd-12-3573-2020, 2020. a, b, c, d, e
Chen, D., Liu, Z., Davis, C., and Gu, Y.: Dust radiative effects on atmospheric thermodynamics and tropical cyclogenesis over the Atlantic Ocean using WRF-Chem coupled with an AOD data assimilation system, Atmos. Chem. Phys., 17, 7917–7939, https://doi.org/10.5194/acp-17-7917-2017, 2017. a
Chernick, M.: Bootstrap Methods: A Guide for Practitioners and Researchers, 2nd edn., John Wiley & Sons, Inc., Hoboken, New Jersey, USA, 2008. a
Colarco, P. R., Kahn, R. A., Remer, L. A., and Levy, R. C.: Impact of satellite viewing-swath width on global and regional aerosol optical thickness statistics and trends, Atmos. Meas. Tech., 7, 2313–2335, https://doi.org/10.5194/amt-7-2313-2014, 2014. a, b
Dang, C., Warren, S. G., Fu, Q., Doherty, S. J., Sturm, M., and Su, J.: Measurements of light-absorbing particles in snow across the Arctic, North America, and China: Effects on surface albedo, J. Geophys. Res.-Atmos., 122, 149–168, https://doi.org/10.1002/2017JD027070, 2017. a
Delene, D. J. and Ogren, J. A.: Variability of Aerosol Optical Properties at Four North American Surface Monitoring Sites, J. Atmos. Sci., 59, 1135–1150, 2002. a
Dockery, D., Pope, A., Xu, X., Spengler, J., Ware, J., Fay, M., Ferris, B., and Speizer, F.: An association between air pollution and mortality in six US cities, New Engl. J. Med., 329, 1753–1759, 1993. a
Dubovik, O. and King, M. D.: A flexible inversion algorithm for retrieval of aerosol optical properties from Sun and sky radiance measurements, J. Geophys. Res.-Atmos., 105, 20673–20696, https://doi.org/10.1029/2000JD900282, 2000. a, b, c
Dubovik, O., Smirnov, A., Holben, B. N., King, M. D., Kaufman, Y. J., Eck, T. F., and Slutsker, I.: Accuracy assessments of aerosol optical properties retrieved from Aerosol Robotic Network (AERONET) Sun and sky radiance measurements, J. Geophys. Res.-Atmos., 105, 9791–9806, https://doi.org/10.1029/2000JD900040, 2000. a, b, c
Dubovik, O., Holben, B., Eck, T. F., Smirnov, A., Kaufman, Y. J., King, M. D., Tanre, D., and Slutsker, I.: Variability of Absorption and Optical Properties of Key Aerosol Types Observed in Worldwide Locations, J. Atmos. Sci., 59, 590–608, 2002. a, b
Dubovik, O., Sinyuk, A., Lapyonok, T., Holben, B. N., Mishchenko, M., Yang, P., Eck, T. F., Volten, H., Mun, O., Veihelmann, B., Zande, W. J. V. D., Leon, J.-F., Sorokin, M., and Slutsker, I.: Application of spheroid models to account for aerosol particle nonsphericity in remote sensing of desert dust, J. Geophys. Res.-Atmos., 111, D11208, https://doi.org/10.1029/2005JD006619, 2006. a, b, c
Dubovik, O., Herman, M., Holdak, A., Lapyonok, T., Tanré, D., Deuzé, J. L., Ducos, F., Sinyuk, A., and Lopatin, A.: Statistically optimized inversion algorithm for enhanced retrieval of aerosol properties from spectral multi-angle polarimetric satellite observations, Atmos. Meas. Tech., 4, 975–1018, https://doi.org/10.5194/amt-4-975-2011, 2011. a, b, c, d, e
Dubovik, O., Lapyonok, T., Litvinov, P., Herman, M., Fuertes, D., Ducos, F., Lopatin, A., Chaikovsky, A., Torres, B., Derimian, Y., Huang, X., Aspetsberger, M., and Federspiel, C.: GRASP: a versatile algorithm for characterizing the atmosphere, SPIE: Newsroom, https://doi.org/10.1117/2.1201408.005558, 2014. a
Eck, T. F., Holben, B. N., Reid, J. S., Smirnov, A., O'Neill, N. T., Slutsker, I., and Kinne, S.: Wavelength dependence of the optical depth of biomass burning, urban, and desert dust aerosols, J. Geophys. Res., 104, 31333–31349, 1999. a
Efron, B.: Bootstrap methods: another look at the jackknife, Ann. Stat., 7, 1–26, 1979. a
Ezzati, M., Lopez, A. D., Rodgers, A., Vander Hoorn, S., and Murray, C. J. L.: Selected major risk factors and global and regional burden of disease, Lancet, 360, 1347–1360, https://doi.org/10.1016/S0140-6736(02)11403-6, 2002. a
Fu, G. and Hasekamp, O.: Retrieval of aerosol microphysical and optical properties over land using a multimode approach, Atmos. Meas. Tech., 11, 6627–6650, https://doi.org/10.5194/amt-11-6627-2018, 2018. a, b
Fu, G., Hasekamp, O., Rietjens, J., Smit, M., Di Noia, A., Cairns, B., Wasilewski, A., Diner, D., Seidel, F., Xu, F., Knobelspiesse, K., Gao, M., da Silva, A., Burton, S., Hostetler, C., Hair, J., and Ferrare, R.: Aerosol retrievals from different polarimeters during the ACEPOL campaign using a common retrieval algorithm, Atmos. Meas. Tech., 13, 553–573, https://doi.org/10.5194/amt-13-553-2020, 2020. a
Giles, D. M., Sinyuk, A., Sorokin, M. G., Schafer, J. S., Smirnov, A., Slutsker, I., Eck, T. F., Holben, B. N., Lewis, J. R., Campbell, J. R., Welton, E. J., Korkin, S. V., and Lyapustin, A. I.: Advancements in the Aerosol Robotic Network (AERONET) Version 3 database – automated near-real-time quality control algorithm with improved cloud screening for Sun photometer aerosol optical depth (AOD) measurements, Atmos. Meas. Tech., 12, 169–209, https://doi.org/10.5194/amt-12-169-2019, 2019. a
Graaf, M. D., Tilstra, L. G., Wang, P., and Stammes, P.: Retrieval of the aerosol direct radiative effect over clouds from spaceborne spectrometry, J. Geophys. Res.-Atmos., 117, D07207, https://doi.org/10.1029/2011JD017160, 2012. a
Hansen, J., Sato, M., and Ruedy, R.: Radiative forcing and climate response, J. Geophys. Res., 102, 6831–6864, 1997. a
Hasekamp, O. P.: Capability of multi-viewing-angle photo-polarimetric measurements for the simultaneous retrieval of aerosol and cloud properties, Atmos. Meas. Tech., 3, 839–851, https://doi.org/10.5194/amt-3-839-2010, 2010. a
Hasekamp, O. P. and Landgraf, J.: Linearization of vector radiative transfer with respect to aerosol properties and its use in satellite remote sensing, J. Geophys. Res.-Atmos., 110, 4203, https://doi.org/10.1029/2004JD005260, 2005. a, b, c
Hasekamp, O. P. and Landgraf, J.: Retrieval of aerosol properties over land surfaces: capabilities of multiple-viewing-angle intensity and polarization measurements, Appl. Optics, 46, 3332–3344, 2007. a
Hasekamp, O. P., Litvinov, P., and Butz, A.: Aerosol properties over the ocean from PARASOL multiangle photopolarimetric measurements, J. Geophys. Res.-Atmos., 116, D14204, https://doi.org/10.1029/2010JD015469, 2011. a, b, c
Hasekamp, O. P., Fu, G., Rusli, S. P., Wu, L., Noia, A. D., Aan de Brugh, J., Landgraf, J., Smit, J. M., Rietjens, J., and Van Amerongen, A.: Aerosol measurements by SPEXone on the NASA PACE mission: expected retrieval capabilities, J. Quant. Spectrosc. Ra., 227, 170–184, https://doi.org/10.1016/j.jqsrt.2019.02.006, 2019a. a
Hasekamp, O. P., Gryspeerdt, E., and Quaas, J.: Analysis of polarimetric satellite measurements suggests stronger cooling due to aerosol-cloud interactions, Nat. Commun., 10, 5405, https://doi.org/10.1038/s41467-019-13372-2, 2019b. a, b
Haywood, J. M. and Shine, K. P.: The effect of anthropogenic sulfate and soot aerosol on the clear sky planetary radiation budget, Geophys. Res. Lett., 22, 603–606, 1995. a
Herman, J. R., Bhartia, P. K., Torres, O., Hsu, C., Seftor, C., and Celarier, E.: Global distribution of UV-absorbing aerosols from Nimbus 7/TOMS data, J. Geophys. Res.-Atmos., 102, 16911–16922, 1997. a
Hodnebrog, Ø., Myhre, G., Forster, P. M., Sillmann, J., and Samset, B. H.: Local biomass burning is a dominant cause of the observed precipitation reduction in southern Africa, Nat. Commun., 7, 11236, https://doi.org/10.1038/ncomms11236, 2016. a
Hodzic, A. and Duvel, J. P.: Impact of biomass-burning aerosols on the diurnal cycle of convective clouds and precipitation over a tropical island, J. Geophys. Res.-Atmos., 123, 1017–1036, https://doi.org/10.1002/2017JD027521, 2018. a
Holben, B., Eck, T., Slutsker, I., Tanré, D., Buis, J., Setzer, A., Vermote, E., Reagan, J., Kaufman, Y. J., Nakajima, T., Lavenu, F., Jankowiak, I., and Smirnov, A.: AERONET – A Federated Instrument Network and Data Archive for Aerosol Characterization, Remote Sens. Environ., 66, 1–16, https://doi.org/10.1016/S0034-4257(98)00031-5, 1998. a, b
Isobe, T., Feigelson, E. D., Akritas, M. G., and Babu, G. J.: Linear regression in Astronomy I, Astrophys. J., 364, 104–113, 1990. a
Jethva, H., Torres, O., and Ahn, C.: Global assessment of OMI aerosol single-scattering albedo using ground-based AERONET inversion, J. Geophys. Res.-Atmos., 119, 9020–9040, https://doi.org/10.1002/2014JD021672, 2014. a, b
Jethva, H., Torres, O., and Yoshida, Y.: Accuracy assessment of MODIS land aerosol optical thickness algorithms using AERONET measurements over North America, Atmos. Meas. Tech., 12, 4291–4307, https://doi.org/10.5194/amt-12-4291-2019, 2019. a
Johnson, B. B. T., Shine, K. P., and Forster, P. M.: The Semi-direct Aerosol Effect: Impact of Absorbing Aerosols on Marine Stratocumulus, Q. J. Roy. Meteor. Soc., 130, 1407–1422, https://doi.org/10.1256/qj.03.61, 2003. a
Kacenelenbogen, M. S., Vaughan, M. A., Redemann, J., Young, S. A., Liu, Z., Hu, Y., Omar, A. H., LeBlanc, S., Shinozuka, Y., Livingston, J., Zhang, Q., and Powell, K. A.: Estimations of global shortwave direct aerosol radiative effects above opaque water clouds using a combination of A-Train satellite sensors, Atmos. Chem. Phys., 19, 4933–4962, https://doi.org/10.5194/acp-19-4933-2019, 2019. a, b, c, d
Kinne, S., O'Donnel, D., Stier, P., Kloster, S., Zhang, K., Schmidt, H., Rast, S., Giorgetta, M., Eck, T. F., and Stevens, B.: MAC-v1: A new global aerosol climatology for climate studies, J. Adv. Model. Earth Sy., 5, 704–740, https://doi.org/10.1002/jame.20035, 2013. a
Koren, I., Martins, J. V., Remer, L. A., and Afargan, H.: Smoke invigoration versus inhibition of clouds over the Amazon, Science, 321, 946–949, https://doi.org/10.1126/science.1159185, 2008. a
Labordena, M., Neubauer, D., Folini, D., Patt, A., and Lilliestam, J.: Blue skies over China: The effect of pollution- control on solar power generation and revenues, PLoS ONE 13, e0207028, https://doi.org/10.1371/journal.pone.0207028, 2018. a
Lacagnina, C., Hasekamp, O. P., Bian, H., Curci, G., Myhre, G., Noije, T. V., Schulz, M., Skeie, R. B., Takemura, T., and Zhang, K.: Aerosol single-scattering albedo over the global oceans: Comparing PARASOL retrievals with AERONET, OMI, and AeroCom models estimates, J. Geophys. Res.-Atmos., 120, 9814–9836, https://doi.org/10.1002/2015JD023501, 2015. a, b, c
Lacagnina, C., Hasekamp, O. P., and Torres, O.: Direct radiative effect of aerosols based on PARASOL and OMI satellite observations, J. Geophys. Res.-Atmos., 122, 2366–2388, https://doi.org/10.1002/2016JD025706, 2017. a, b
Laj, P., Bigi, A., Rose, C., Andrews, E., Lund Myhre, C., Collaud Coen, M., Lin, Y., Wiedensohler, A., Schulz, M., Ogren, J. A., Fiebig, M., Gliß, J., Mortier, A., Pandolfi, M., Petäja, T., Kim, S.-W., Aas, W., Putaud, J.-P., Mayol-Bracero, O., Keywood, M., Labrador, L., Aalto, P., Ahlberg, E., Alados Arboledas, L., Alastuey, A., Andrade, M., Artíñano, B., Ausmeel, S., Arsov, T., Asmi, E., Backman, J., Baltensperger, U., Bastian, S., Bath, O., Beukes, J. P., Brem, B. T., Bukowiecki, N., Conil, S., Couret, C., Day, D., Dayantolis, W., Degorska, A., Eleftheriadis, K., Fetfatzis, P., Favez, O., Flentje, H., Gini, M. I., Gregorič, A., Gysel-Beer, M., Hallar, A. G., Hand, J., Hoffer, A., Hueglin, C., Hooda, R. K., Hyvärinen, A., Kalapov, I., Kalivitis, N., Kasper-Giebl, A., Kim, J. E., Kouvarakis, G., Kranjc, I., Krejci, R., Kulmala, M., Labuschagne, C., Lee, H.-J., Lihavainen, H., Lin, N.-H., Löschau, G., Luoma, K., Marinoni, A., Martins Dos Santos, S., Meinhardt, F., Merkel, M., Metzger, J.-M., Mihalopoulos, N., Nguyen, N. A., Ondracek, J., Pérez, N., Perrone, M. R., Petit, J.-E., Picard, D., Pichon, J.-M., Pont, V., Prats, N., Prenni, A., Reisen, F., Romano, S., Sellegri, K., Sharma, S., Schauer, G., Sheridan, P., Sherman, J. P., Schütze, M., Schwerin, A., Sohmer, R., Sorribas, M., Steinbacher, M., Sun, J., Titos, G., Toczko, B., Tuch, T., Tulet, P., Tunved, P., Vakkari, V., Velarde, F., Velasquez, P., Villani, P., Vratolis, S., Wang, S.-H., Weinhold, K., Weller, R., Yela, M., Yus-Diez, J., Zdimal, V., Zieger, P., and Zikova, N.: A global analysis of climate-relevant aerosol properties retrieved from the network of Global Atmosphere Watch (GAW) near-surface observatories, Atmos. Meas. Tech., 13, 4353–4392, https://doi.org/10.5194/amt-13-4353-2020, 2020. a
Lequy, É., Conil, S., and Turpault, M.-P.: Impacts of Aeolian dust deposition on European forest sustainability: A review, Forest Ecol. Manag., 267, 240–252, https://doi.org/10.1016/j.foreco.2011.12.005, 2012. a
Levelt, P. F., Hilsenrath, E., Leppelmeier, G. W., Oord, G. H. J. V. D., Bhartia, P. K., Tamminen, J., Haan, J. F. D., and Veefkind, J. P.: Science Objectives of the Ozone Monitoring Instrument, IEEE T. Geosci. Remote, 44, 1199–1208, 2006. a
Li, X., Wagner, F., Peng, W., Yang, J., and Mauzerall, D. L.: Reduction of solar photovoltaic resources due to air pollution in China, P. Natl. Acad. Sci. USA, 114, 11867–11872, https://doi.org/10.1073/pnas.1711462114, 2017. a
Litvinov, P., Hasekamp, O., and Cairns, B.: Remote Sensing of Environment Models for surface reflection of radiance and polarized radiance: Comparison with airborne multi-angle photopolarimetric measurements and implications for modeling top-of-atmosphere measurements, Remote Sens. Environ., 115, 781–792, https://doi.org/10.1016/j.rse.2010.11.005, 2011. a, b
Lohmann, U. and Feichter, J.: Impact of sulfate aerosols on albedo and lifetime of clouds: A sensitivity study with the ECHAM4 GCM, J. Geophys. Res., 102, 13685–13700, 1997. a
Lohmann, U. and Feichter, J.: Global indirect aerosol effects: a review, Atmos. Chem. Phys., 5, 715–737, https://doi.org/10.5194/acp-5-715-2005, 2005. a
Lopatin, A., Dubovik, O., Fuertes, D., Stenchikov, G., Lapyonok, T., Veselovskii, I., Wienhold, F. G., Shevchenko, I., Hu, Q., and Parajuli, S.: Synergy processing of diverse ground-based remote sensing and in situ data using the GRASP algorithm: applications to radiometer, lidar and radiosonde observations, Atmos. Meas. Tech., 14, 2575–2614, https://doi.org/10.5194/amt-14-2575-2021, 2021. a, b
Maher, B., Prospero, J., Mackie, D., Gaiero, D., Hesse, P., and Balkanski, Y.: Global connections between aeolian dust, climate and ocean biogeochemistry at the present day and at the last glacial maximum, Earth-Sci. Rev., 99, 61–97, https://doi.org/10.1016/j.earscirev.2009.12.001, 2010. a
McTainsh, G. and Strong, C.: The role of aeolian dust in ecosystems, Geomorphology, 89, 39–54, https://doi.org/10.1016/j.geomorph.2006.07.028, 2007. a
Mittaz, J. and Merchant, C. J.: Applying principles of metrology to historical Earth observations from satellites, Metrologia, 56, 032002, https://doi.org/10.1088/1681-7575/ab1705, 2019. a
Myhre, G., Samset, B. H., Schulz, M., Balkanski, Y., Bauer, S., Berntsen, T. K., Bian, H., Bellouin, N., Chin, M., Diehl, T., Easter, R. C., Feichter, J., Ghan, S. J., Hauglustaine, D., Iversen, T., Kinne, S., Kirkevåg, A., Lamarque, J.-F., Lin, G., Liu, X., Lund, M. T., Luo, G., Ma, X., van Noije, T., Penner, J. E., Rasch, P. J., Ruiz, A., Seland, Ø., Skeie, R. B., Stier, P., Takemura, T., Tsigaridis, K., Wang, P., Wang, Z., Xu, L., Yu, H., Yu, F., Yoon, J.-H., Zhang, K., Zhang, H., and Zhou, C.: Radiative forcing of the direct aerosol effect from AeroCom Phase II simulations, Atmos. Chem. Phys., 13, 1853–1877, https://doi.org/10.5194/acp-13-1853-2013, 2013. a
Omar, A. H., Won, J.-G., Winker, D. M., Yoon, S.-C., Dubovik, O., and Mccormick, M. P.: Development of global aerosol models using cluster analysis of Aerosol Robotic Network (AERONET) measurements, J. Geophys. Res.-Atmos., 110, D10S14, https://doi.org/10.1029/2004JD004874, 2005. a
Peers, F., Bellouin, N., Waquet, F., Ducos, F., Goloub, P., Mollard, J., Myhre, G., Skeie, R. B., Takemura, T., Tanré, D., Thieuleux, F., and Zhang, K.: Comparison of aerosol optical properties above clouds between POLDER and AeroCom models over the South East Atlantic Ocean during the fire season, Geophys. Res. Lett., 43, 3991–4000, https://doi.org/10.1002/2016GL068222, 2016. a
Phillips, P.: A technique for the numerical solution of certain integral equations of the first kind, J. Assoc. Comput. Mach., 9, 84–97, 1962. a
Pitkänen, M. R. A., Mikkonen, S., Lehtinen, K. E. J., Lipponen, A., and Arola, A.: Artificial bias typically neglected in comparisons of uncertain atmospheric data, Geophys. Res. Lett., 43, 10003–10011, https://doi.org/10.1002/2016GL070852, 2016. a
Popp, T., De Leeuw, G., Bingen, C., Brühl, C., Capelle, V., Chedin, A., Clarisse, L., Dubovik, O., Grainger, R., Griesfeller, J., Heckel, A., Kinne, S., Klüser, L., Kosmale, M., Kolmonen, P., Lelli, L., Litvinov, P., Mei, L., North, P., Pinnock, S., Povey, A., Robert, C., Schulz, M., Sogacheva, L., Stebel, K., Zweers, D. S., Thomas, G., Tilstra, L. G., Vandenbussche, S., Veefkind, P., Vountas, M., and Xue, Y.: Development, production and evaluation of aerosol climate data records from European satellite observations (Aerosol_cci), Remote Sens.-Basel, 8, 421–454, https://doi.org/10.3390/rs8050421, 2016. a
Russell, P. B., Kacenelenbogen, M., Livingston, J. M., Hasekamp, O. P., Burton, S. P., Schuster, G. L., Johnson, M. S., Knobelspiesse, K. D., Redemann, J., Ramachandran, S., and Holben, B.: A multiparameter aerosol classification method and its application to retrievals from spaceborne polarimetry, J. Geophys. Res.-Atmos., 11, 9838–9863, https://doi.org/10.1002/2013JD021411, 2014. a
Saide, P. E., Spak, S. N., Pierce, R. B., Otkin, J. A., Schaack, T. K., Heidinger, A. K., Da Silva, A. M., Kacenelenbogen, M., Redemann, J., and Carmichael, G. R.: Central American biomass burning smoke can increase tornado severity in the US, Geophys. Res. Lett., 42, 956–965, https://doi.org/10.1002/2014GL062826, 2015. a
Samset, B. H., Myhre, G., Forster, P. M., Hodnebrog, Andrews, T., Faluvegi, G., Fläschner, D., Kasoar, M., Kharin, V., Kirkevåg, A., Lamarque, J. F., Olivié, D., Richardson, T., Shindell, D., Shine, K. P., Takemura, T., and Voulgarakis, A.: Fast and slow precipitation responses to individual climate forcers: A PDRMIP multimodel study, Geophys. Res. Lett., 43, 2782–2791, https://doi.org/10.1002/2016GL068064, 2016. a
Sayer, A. M., Thomas, G. E., Palmer, P. I., and Grainger, R. G.: Some implications of sampling choices on comparisons between satellite and model aerosol optical depth fields, Atmos. Chem. Phys., 10, 10705–10716, https://doi.org/10.5194/acp-10-10705-2010, 2010. a
Schafer, J. S., Eck, T. F., Holben, B. N., Thornhill, K. L., Anderson, B. E., Sinyuk, A., Giles, D. M., Winstead, E. L., Ziemba, L. D., Beyersdorf, A. J., Kenny, P. R., Smirnov, A., and Slutsker, I.: Intercomparison of aerosol single-scattering albedo derived from AERONET surface radiometers and LARGE in situ aircraft profiles during the 2011 DRAGON-MD and DISCOVER-AQ experiments, J. Geophys. Res.-Atmos., 119, 7439–7452, https://doi.org/10.1002/2013JD021166, 2014. a
Schmeisser, L., Backman, J., Ogren, J. A., Andrews, E., Asmi, E., Starkweather, S., Uttal, T., Fiebig, M., Sharma, S., Eleftheriadis, K., Vratolis, S., Bergin, M., Tunved, P., and Jefferson, A.: Seasonality of aerosol optical properties in the Arctic, Atmos. Chem. Phys., 18, 11599–11622, https://doi.org/10.5194/acp-18-11599-2018, 2018. a
Schmid, B., Michalsky, J., Halthore, R., Beauharnois, M., Harnson, L., Livingston, J., Russell, P., Holben, B., Eck, T., and Smirnov, A.: Comparison of Aerosol Optical Depth from Four Solar Radiometers During the Fall 1997 ARM Intensive Observation Period, Geophys. Res. Lett., 26, 2725–2728, 1999. a
Schutgens, N., Tsyro, S., Gryspeerdt, E., Goto, D., Weigum, N., Schulz, M., and Stier, P.: On the spatio-temporal representativeness of observations, Atmos. Chem. Phys., 17, 9761–9780, https://doi.org/10.5194/acp-17-9761-2017, 2017. a
Schutgens, N., Sayer, A. M., Heckel, A., Hsu, C., Jethva, H., de Leeuw, G., Leonard, P. J. T., Levy, R. C., Lipponen, A., Lyapustin, A., North, P., Popp, T., Poulsen, C., Sawyer, V., Sogacheva, L., Thomas, G., Torres, O., Wang, Y., Kinne, S., Schulz, M., and Stier, P.: An AeroCom–AeroSat study: intercomparison of satellite AOD datasets for aerosol model evaluation, Atmos. Chem. Phys., 20, 12431–12457, https://doi.org/10.5194/acp-20-12431-2020, 2020. a, b, c, d, e, f, g, h, i, j, k, l, m
Schutgens, N., Dubovik, O., Hasekamp, O., Torres, O., Jethva, H., Leonard, P. J. T., Redemann, J., Shinozuka, Y., Balkanski, Y., Bellouin, N., Bergman, T., Bian, H., Curci, G., Kipling, Z., Kirkevåg, A., Kokkola, H., Tronstad Lund, M., Myhre, G., Remy, S., van Noije, T., Olivie, D. J. L., Penner, J. E., Takemura, T., Zhang, K., Zhu, J., Checa-Garcia, R., Liu, X., Matsui, H., Mielonen, T., Neubauer, D., Bieltvedt Skeie, R., Tsyro, S., de Leeuw, G., Kinne, S., Popp, T., Schulz, M., and Stier, P.: AEROCOM/AEROSAT AAOD & SSA study, part II: evaluation of AEROCOM models, in preparation, 2021. a, b
Schutgens, N. A. J.: Site representativity of AERONET and GAW remotely sensed aerosol optical thickness and absorbing aerosol optical thickness observations, Atmos. Chem. Phys., 20, 7473–7488, https://doi.org/10.5194/acp-20-7473-2020, 2020. a, b
Schutgens, N. A. J., Partridge, D. G., and Stier, P.: The importance of temporal collocation for the evaluation of aerosol models with observations, Atmos. Chem. Phys., 16, 1065–1079, https://doi.org/10.5194/acp-16-1065-2016, 2016a. a, b, c
Schutgens, N. A. J., Gryspeerdt, E., Weigum, N., Tsyro, S., Goto, D., Schulz, M., and Stier, P.: Will a perfect model agree with perfect observations? The impact of spatial sampling, Atmos. Chem. Phys., 16, 6335–6353, https://doi.org/10.5194/acp-16-6335-2016, 2016b. a, b
Schwarz, J. P., Spackman, J. R., Gao, R. S., Watts, L. A., Stier, P., Schulz, M., Davis, S. M., Wofsy, S. C., and Fahey, D. W.: Global-scale black carbon profiles observed in the remote atmosphere and compared to models, Geophys. Res. Lett., 37, 5542–5547, https://doi.org/10.1029/2010GL044372, 2010. a
Schwarz, J. P., Samset, B. H., Perring, A. E., Spackman, J. R., Gao, R. S., Stier, P., Schulz, M., Moore, F. L., Ray, E. A., and Fahey, D. W.: Global-scale seasonally resolved black carbon vertical profiles over the Pacific, Geophys. Res. Lett., 40, 5542–5547, https://doi.org/10.1002/2013GL057775, 2013. a
Sinyuk, A., Holben, B. N., Eck, T. F., Giles, D. M., Slutsker, I., Korkin, S., Schafer, J. S., Smirnov, A., Sorokin, M., and Lyapustin, A.: The AERONET Version 3 aerosol retrieval algorithm, associated uncertainties and comparisons to Version 2, Atmos. Meas. Tech., 13, 3375–3411, https://doi.org/10.5194/amt-13-3375-2020, 2020. a, b, c
Smirnov, A., Holben, B. N., Eck, T. F., Dubovik, O., and Slutsker, I.: Cloud-Screening and Quality Control Algorithms for the AERONET Database, Remote Sens. Environ., 73, 337–349, 2000. a
Smith, K. R., Jerrett, M., Anderson, H. R., Burnett, R. T., Stone, V., Derwent, R., Atkinson, R. W., Cohen, A., Shonkoff, S. B., Krewski, D., Pope, C. A., Thun, M. J., and Thurston, G.: Public health benefits of strategies to reduce greenhouse-gas emissions: health implications of short-lived greenhouse pollutants, Lancet, 374, 2091–2103, https://doi.org/10.1016/S0140-6736(09)61716-5, 2009. a
Stap, F. A., Hasekamp, O. P., and Röckmann, T.: Sensitivity of PARASOL multi-angle photopolarimetric aerosol retrievals to cloud contamination, Atmos. Meas. Tech., 8, 1287–1301, https://doi.org/10.5194/amt-8-1287-2015, 2015. a
Swap, R., Garstang, M., Greco, S., Talbot, R., and Kallberg, P.: Saharan dust in the Amazon Basin, Tellus, 44B, 133–149, https://doi.org/10.1034/j.1600-0889.1992.t01-1-00005.x, 1992. a
Taylor, K. E.: Summarizing multiple aspects of model performance in a single diagram, J. Geophys. Res., 106, 7183–7192, 2001. a, b
Tegen, I. and Heinold, B.: Large-Scale Modeling of Absorbing Aerosols and Their Semi-Direct Effects, Atmosphere, 9, 380, https://doi.org/10.3390/atmos9100380, 2018. a
Thomas, J. L., Polashenski, C. M., Soja, A. J., Marelle, L., Casey, K. A., Choi, H. D., Raut, J. C., Wiedinmyer, C., Emmons, L. K., Fast, J. D., Pelon, J., Law, K. S., Flanner, M. G., and Dibb, J. E.: Quantifying black carbon deposition over the Greenland ice sheet from forest fires in Canada, Geophys. Res. Lett., 44, 7965–7974, https://doi.org/10.1002/2017GL073701, 2017. a
Tikhonov, A.: On the solution of incorrectly stated problems and a method of regularization, Dokl. Akad. Nauk SSSR+, 151, 501–504, 1963. a
Torres, B., Dubovik, O., Toledano, C., Berjon, A., Cachorro, V. E., Lapyonok, T., Litvinov, P., and Goloub, P.: Sensitivity of aerosol retrieval to geometrical configuration of ground-based sun/sky radiometer observations, Atmos. Chem. Phys., 14, 847–875, https://doi.org/10.5194/acp-14-847-2014, 2014. a
Torres, O., Tanskanen, A., Veihelmann, B., Ahn, C., Braak, R., Bhartia, P. K., Veefkind, P., and Levelt, P.: Aerosols and surface UV products from Ozone Monitoring Instrument observations: An overview, J. Geophys. Res.-Atmos., 112, D24S47, https://doi.org/10.1029/2007JD008809, 2007. a
Torres, O., Ahn, C., and Chen, Z.: Improvements to the OMI near-UV aerosol algorithm using A-train CALIOP and AIRS observations, Atmos. Meas. Tech., 6, 3257–3270, https://doi.org/10.5194/amt-6-3257-2013, 2013. a
Tosca, M. G., Randerson, J. T., and Zender, C. S.: Global impact of smoke aerosols from landscape fires on climate and the Hadley circulation, Atmos. Chem. Phys., 13, 5227–5241, https://doi.org/10.5194/acp-13-5227-2013, 2013. a
Tsikerdekis, A., Schutgens, N. A. J., and Hasekamp, O. P.: Assimilating aerosol optical properties related to size and absorption from POLDER/PARASOL with an ensemble data assimilation system, Atmos. Chem. Phys., 21, 2637–2674, https://doi.org/10.5194/acp-21-2637-2021, 2021. a, b
Twomey, S.: Pollution and the planetary albedo, Atmos. Environ., 8, 1251–1256, 1974. a
Vink, S. and Measures, C.: The role of dust deposition in determining surface water distributions of Al and Fe in the South West Atlantic, Deep-Sea Res. Pt. II, 48, 2787–2809, https://doi.org/10.1016/S0967-0645(01)00018-2, 2001. a
Virtanen, T. H., Kolmonen, P., Sogacheva, L., Rodríguez, E., Saponaro, G., and de Leeuw, G.: Collocation mismatch uncertainties in satellite aerosol retrieval validation, Atmos. Meas. Tech., 11, 925–938, https://doi.org/10.5194/amt-11-925-2018, 2018. a
Wang, Y., Sartelet, K. N., Bocquet, M., and Chazette, P.: Modelling and assimilation of lidar signals over Greater Paris during the MEGAPOLI summer campaign, Atmos. Chem. Phys., 14, 3511–3532, https://doi.org/10.5194/acp-14-3511-2014, 2014. a
Watson-Parris, D., Schutgens, N., Cook, N., Kipling, Z., Kershaw, P., Gryspeerdt, E., Lawrence, B., and Stier, P.: Community Intercomparison Suite (CIS) v1.4.0: a tool for intercomparing models and observations, Geosci. Model Dev., 9, 3093–3110, https://doi.org/10.5194/gmd-9-3093-2016, 2016. a, b, c
WMO: Systematic observation requirements for satellite-based data products for climate, 2011 Update Supplemental details to the satellite-based component of the “Implementation Plan for the Global Observing System for Climate in Support of the UNFCCC”, Tech. Rep., WMO, Geneva, Switzerland, 2011. a
Wu, L., Hasekamp, O., van Diedenhoven, B., and Cairns, B.: Aerosol retrieval from multiangle, multispectral photopolarimetric measurements: importance of spectral range and angular resolution, Atmos. Meas. Tech., 8, 2625–2638, https://doi.org/10.5194/amt-8-2625-2015, 2015. a
Zhang, Y., Forrister, H., Liu, J., Dibb, J., Anderson, B., Schwarz, J. P., Perring, A. E., Jimenez, J. L., Campuzano-Jost, P., Wang, Y., Nenes, A., and Weber, R. J.: Top-of-atmosphere radiative forcing affected by brown carbon in the upper troposphere, Nat. Geosci., 10, 486–489, https://doi.org/10.1038/ngeo2960, 2017. a
- Abstract
- Introduction
- Datasets
- Collocation and analysis methodology
- A first look at the satellite products
- Evaluation of satellite products with AERONET
- Intercomparison of satellite AAOD and SSA
- Conclusions
- Appendix A: Generic aggregation and collocation
- Code and data availability
- Author contributions
- Competing interests
- Acknowledgements
- Financial support
- Review statement
- References
- Supplement
- Abstract
- Introduction
- Datasets
- Collocation and analysis methodology
- A first look at the satellite products
- Evaluation of satellite products with AERONET
- Intercomparison of satellite AAOD and SSA
- Conclusions
- Appendix A: Generic aggregation and collocation
- Code and data availability
- Author contributions
- Competing interests
- Acknowledgements
- Financial support
- Review statement
- References
- Supplement