the Creative Commons Attribution 4.0 License.
the Creative Commons Attribution 4.0 License.
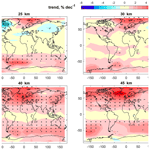
Measurement report: regional trends of stratospheric ozone evaluated using the MErged GRIdded Dataset of Ozone Profiles (MEGRIDOP)
Viktoria F. Sofieva
Monika Szeląg
Johanna Tamminen
Erkki Kyrölä
Doug Degenstein
Chris Roth
Daniel Zawada
Alexei Rozanov
Carlo Arosio
John P. Burrows
Mark Weber
Alexandra Laeng
Gabriele P. Stiller
Thomas von Clarmann
Lucien Froidevaux
Nathaniel Livesey
Michel van Roozendael
Christian Retscher
In this paper, we present the MErged GRIdded Dataset of Ozone Profiles (MEGRIDOP) in the stratosphere with a resolved longitudinal structure, which is derived from data from six limb and occultation satellite instruments: GOMOS, SCIAMACHY and MIPAS on Envisat, OSIRIS on Odin, OMPS on Suomi-NPP, and MLS on Aura. The merged dataset was generated as a contribution to the European Space Agency Climate Change Initiative Ozone project (Ozone_cci). The period of this merged time series of ozone profiles is from late 2001 until the end of 2018.
The monthly mean gridded ozone profile dataset is provided in the altitude range from 10 to 50 km in bins of 10∘ latitude × 20∘ longitude. The merging is performed using deseasonalized anomalies. The created MEGRIDOP dataset can be used for analyses that probe our understanding of stratospheric chemistry and dynamics. To illustrate some possible applications, we created a climatology of ozone profiles with resolved longitudinal structure. We found zonal asymmetry in the climatological ozone profiles at middle and high latitudes associated with the polar vortex. At northern high latitudes, the amplitude of the seasonal cycle also has a longitudinal dependence.
The MEGRIDOP dataset has also been used to evaluate regional vertically resolved ozone trends in the stratosphere, including the polar regions. It is found that stratospheric ozone trends exhibit longitudinal structures at Northern Hemisphere middle and high latitudes, with enhanced trends over Scandinavia and the Atlantic region. This agrees well with previous analyses and might be due to changes in dynamical processes related to the Brewer–Dobson circulation.
- Article
(8233 KB) - Full-text XML
-
Supplement
(966 KB) - BibTeX
- EndNote
Nowadays, the importance of protecting the ozone layer and monitoring its recovery from the effect of ozone depleting substances is well recognized (e.g., Petropavlovskikh et al., 2019; WMO, 2014, 2018). Past analyses have demonstrated that ozone is recovering in the upper stratosphere (e.g., Arosio et al., 2019; Bourassa et al., 2014; Kyrölä et al., 2013; Petropavlovskikh et al., 2019; Sofieva et al., 2017b; Steinbrecht et al., 2017; WMO, 2018). The ozone recovery in the lower stratosphere has not yet been observed, and lower stratospheric ozone trends are the subject of recent controversial discussions (Ball et al., 2018, 2019; Chipperfield et al., 2018).
In the majority of studies of ozone profile trends using satellite observations made in limb-viewing geometry, analyses are performed on zonal mean data. This representation allows ozone trends to be estimated globally. At the same time, such representation provides a sufficiently large amount of experimental data in spatiotemporal bins (usually 10∘ latitude and 1 month) to enable robust estimation of trends. This is especially important for the period before 2001 when long data records are available only from solar occultation instruments having relatively sparse data coverage.
A recent study by Arosio et al. (2019) using the merged SCIAMACHY-OMPS dataset has shown that ozone trends for the period from 2003–2018 have a significant dependence on longitude. Also, total ozone column trends (WMO, 2018 and references therein) have a pronounced zonal structure.
This paper is focused on a new longitudinally resolved merged dataset of ozone profiles in the stratosphere based on several limb and occultation instruments. This new merged dataset is a contribution to the European Space Agency Climate Change Initiative ozone project (Ozone_cci). It can be used in different applications, including the evaluation of regional ozone trends in the stratosphere.
The paper is organized as follows. In Sect. 2, we briefly discuss the satellite data used for creating the merged dataset. Section 3 is dedicated to the methodological aspects of data merging. Examples of ozone distributions are shown in Sect. 4. Section 5 is dedicated to regional trend analysis. A discussion and summary (Sect. 6) conclude the paper.
The MEGRIDOP dataset is a merged and gridded dataset generated using ozone profiles retrieved from several limb and occultation instruments, viz. MIPAS (Michelson Interferometer for Passive Atmospheric Sounding), SCIAMACHY (SCanning Imaging Spectrometer for Atmospheric CHartographY) and GOMOS (Global Ozone Monitoring by occultation of Stars), all on Envisat, OSIRIS (Optical Spectrograph and InfraRed Imaging System) on Odin, OMPS-LP (Ozone Mapping and Profiles Suite – Limb Profiler) on Suomi-NPP, and MLS (Microwave Limb Sounder) on Aura.
These instruments provide high-quality ozone profiles with a good vertical resolution of 2–4 km and a relatively dense spatiotemporal coverage (100–3500 ozone profiles per day with fairly uniform sampling in longitude). The important information about the datasets is collected in Table 1. More information about the datasets from the individual satellite instruments is found in Petropavlovskikh et al. (2019), Sofieva et al. (2017b) and references therein.
For all instruments except MLS, the original ozone profile retrievals are performed on an altitude grid. GOMOS, OSIRIS, SCIAMACHY and OMPS-LP provide number density ozone profiles; therefore this representation (number density on an altitude grid) is used for the merged dataset. For MIPAS, the retrievals are performed in a volume mixing ratio vs. altitude grid. The conversion to number density profiles is performed using temperature profiles retrieved by MIPAS and the pressure profiles provided with the MIPAS ozone data; the latter are constructed from altitude and temperature using one (z, p, T) data point from the ERA-Interim reanalysis (https://www.ecmwf.int/en/forecasts/datasets/reanalysis-datasets/era-interim, last access: 22 April 2021; Dee et al., 2011).
For MLS, retrievals are performed in a mixing ratio on a pressure grid. Similarly to the conversion procedure of MIPAS data, we performed the conversion to number density using the retrieved MLS temperatures, but for altitude–pressure conversion, we used the ERA-Interim reanalysis data. Such a conversion might introduce some uncertainty in the MLS data. For studies of long-term changes, this uncertainty is associated with a potentially imperfect representation of temperature trends in ERA-Interim, which might influence ozone trends. However, since current stratospheric temperature trends (after 2000) are small (Maycock et al., 2018; Steiner et al., 2020), this uncertainty is expected to be small. The MLS ozone profiles data record is stable (Hubert et al., 2016); therefore, including MLS data into the merged dataset is advantageous, especially for the merging method applied in our work (see also below).
For all the instruments, we use the ozone profiles from the updated HARMonized dataset of Ozone profiles (HARMOZ_ALT) developed in the ESA Ozone_cci project (Sofieva et al., 2013), https://climate.esa.int/en/projects/ozone/ (last access: 22 April 2021). HARMOZ consists of the original retrieved ozone profiles from each instrument, which are screened for invalid data by the instrument experts and are presented on a vertical grid (altitude-gridded profiles are used in our paper) and in a common netCDF4 format. Detailed information about the original datasets can be found in Sofieva et al. (2013), and references to the corresponding publications are also collected in Table 1 of our paper.
The method used for creating the MEGRIDOP dataset is similar to that used for the creation of the merged SAGE-CCI-OMPS dataset (Sofieva et al., 2017b). Below we describe and illustrate the merging process.
3.1 Gridded monthly means from individual instruments
First, gridded ozone profile data in each latitude–longitude bin b and at altitude z were created for each individual dataset i and each month t. The mean number density profile in each spatiotemporal bin is . For each instrument, we required more than 10 measurements in each spatiotemporal bin. The uncertainty of the averaged data is approximated by the standard error of the mean (see discussion in Toohey and von Clarmann, 2013 on possible influence of correlations caused by orbital sampling on the standard error of the mean).
The non-uniformity of the sampling pattern can be characterized by the inhomogeneity measure, which is defined as the linear combination of two classical inhomogeneity measures, asymmetry A and entropy E: (Sofieva et al., 2014). The unitless inhomogeneity measure H ranges from 0 to 1 (the more homogeneous, the smaller H is). For our application, we considered the inhomogeneity in time (Htime) as the main contribution to sampling uncertainty.
Examples of gridded datasets at 30 km altitude for individual satellite instruments are shown in Figs. 1 and 2. All instruments show a similar morphology, although biases between individual datasets exist. The coverage is instrument-specific and to some extent time-dependent; the most complete coverage is achieved by MIPAS and MLS. The spatial bins are covered rather uniformly by the data. Examples of the inhomogeneity measure Htime are presented in Fig. S1 in the Supplement. Htime is very close to zero for the instruments with dense sampling (MIPAS, SCIAMACHY, MLS, OMPS). For OSIRIS and GOMOS, H is usually below 0.1 (good homogeneity of the data) with a few exceptions for some months and locations. In this work, the inhomogeneity measure Htime is used for detection of spatial bins with high inhomogeneity of data (see below).
3.2 Seasonal cycle and deseasonalized anomalies
For each instrument i, latitude–longitude bin b and altitude level z, deseasonalized anomalies are computed as:
where is the monthly mean in this spatial bin and is the climatological mean value for the month m. In other words, from each January we removed the mean January value, from each February the mean February value, and so on.
In our computations, we removed values for spatial bins with less than 10 profiles and inhomogeneity Htime larger than 0.9. For all instruments except for OMPS, the seasonal cycle is estimated using the years from 2005–2011. For OMPS, the seasonal cycle is evaluated using data from 2012–2018. Figure 3 illustrates the seasonal cycle at 40 km for all instruments except GOMOS, as the GOMOS data do not cover all months for the considered spatial bins. Although biases are visible, the overall behavior of the seasonal cycle is similar for the different datasets. In the tropics (left panel), small differences in seasonal cycle between two longitude regions, 0–20 and 120–140∘ E, are observed, while at mid-latitudes, all satellite instruments show consistently different seasonal cycles in these two longitude regions.
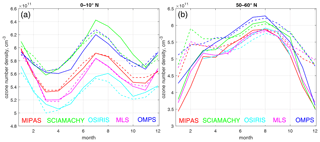
Figure 3Examples of seasonal cycles in the tropics (a) and NH upper stratosphere (b) at 40 km. Solid lines: longitudes 0–20∘ E; dashed lines: longitudes 120–140∘ E. In the tropics, a semi-annual cycle is observed.
For two instruments – MIPAS and MLS – which measure during day and night and thus provide data at all latitudes in all seasons, we compared the relative amplitude of the seasonal cycle at several altitude levels (Fig. 4). As seen from Fig. 4, longitudinal structures in the relative amplitude of the seasonal cycle are observed to be largest in the northern middle and high latitudes, particularly in the middle and upper stratosphere.
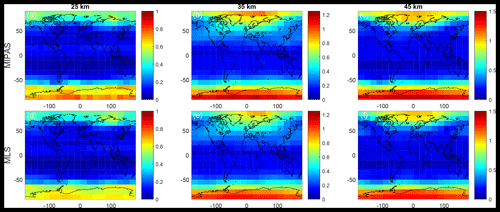
Figure 4Relative amplitude of seasonal cycle at 25 km (a, d), 35 km (b, e) and 45 km (c, f) for MIPAS (a, b, c) and MLS (d, e, f).
The merging of individual datasets was performed on deseasonalized anomalies. The main advantage of using deseasonalized anomalies is that various biases between the individual datasets – e.g., instrumental-specific, or those due to the difference in local time – are automatically removed. The deseasonalization also removes spatial sampling biases if the sampling patterns do not change over time. Details of the applied merging method are presented in the next section.
3.3 Merging the data
The merging method used for creating MEGRIDOP is similar to that used in creating the merged SAGE-CCI-OMPS dataset (Sofieva et al., 2017b). The deseasonalized anomalies of all instruments except OMPS are aligned, as the seasonal cycle was estimated using the same period. First, we offset the OMPS deseasonalized anomalies to the median of the deseasonalized anomalies from all other instruments. These additive offsets are computed using the data from years 2012–2018, and the offsetting procedure is illustrated in Fig. 5. In this figure, we selected a spatial bin where the effect of the offsetting is clearly visible. In many other bins, the offsets are small or negligible. As observed in Fig. 5 (and also below in Fig. 6), the deseasonalized anomalies from individual datasets are in good agreement.
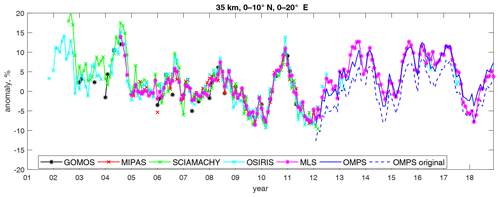
Figure 5Illustration of offsetting the OMPS deseasonalized anomalies. The data are shown for altitude 35 km and the 0–10∘ N, 0–20∘ E bin.
After offsetting OMPS, the merged ozone profiles in each spatiotemporal bin and at each altitude level is obtained from the median of the deseasonalized anomalies corresponding to individual instruments:
The advantage of using the median estimate is that the merged anomaly follows the majority of the data and it is not very sensitive to exclusion or addition of an individual data record in cases where there are several (and consistent) anomaly datasets available. The sensitivity of the dataset and the evaluated trends to the number of instruments was studied in detail for the SAGE-CCI-OMPS dataset, which is created with the same merging algorithm (Sofieva et al., 2017b), and this is also valid for MEGRIDOP.
The uncertainties of the merged deseasonalized anomalies are computed similarly to those used for the merged SAGE-CCI-OMPS dataset (Sofieva et al., 2017b). For each instrument, the uncertainty of the deseasonalized anomalies, σΔi, is estimated via Gaussian error propagation; it is given by
where σi is the uncertainty of the gridded ozone profiles (see Sect. 3.1) and σm,i is the uncertainty of the seasonal cycle ρm,i, which can be estimated via propagation of random uncertainties to the mean value:
where Nm is the number of monthly mean values in a given month m available from all years.
Analogously to Sofieva et al. (2017b), the uncertainties of the merged deseasonalized anomalies are estimated as
where is the anomaly uncertainty of the instrument corresponding to the median value. In cases where there are an even number of measurements, the mean of two neighbors to the median is used. Analogously to uncertainty estimates in the merged SAGE-CCI-OMPS dataset (Sofieva et al., 2017b), the uncertainties given by Eq. (5) can be interpreted as follows. If individual anomalies are significantly different, the uncertainty of the merged anomaly is the uncertainty corresponding to the median value. In cases where several instruments report a similar anomaly (intersecting error bars), this provides more confidence in this anomaly value, and the resulting uncertainty of the merged anomaly is approximated by the second term in Eq. (5).
The deseasonalized anomalies from individual datasets are usually very close to each other so that several values can be typically found within the uncertainty interval of the merged anomaly . This is similar to the approach taken with the SAGE_CCI-OMPS dataset (Sofieva et al., 2017b, Fig. S8).
Examples of deseasonalized anomalies and their estimated uncertainties are displayed in Figs. 6 and 7, respectively.
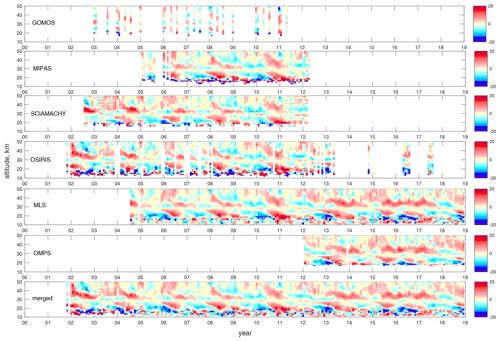
Figure 6An example of deseasonalized anomalies (in %) for individual instruments and the merged dataset in the spatial bin 0–10∘ N, 0–20∘ E.
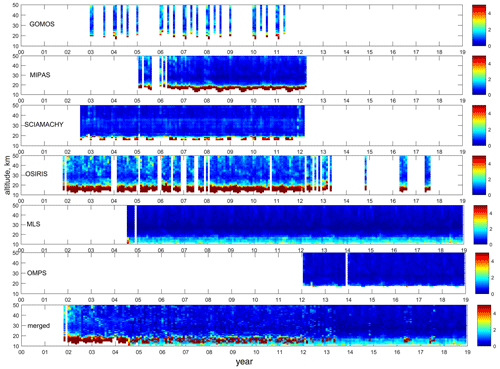
Figure 7An example of uncertainties in deseasonalized anomalies (in %) for individual instruments and the merged dataset in the spatial bin 0–10∘ N, 0–20∘ E.
The average estimated uncertainty of the merged ozone is usually less than 2 % before 2012 and below 1 % after 2012. In the upper troposphere and the lower stratosphere (UTLS), uncertainties are larger than in the stratosphere; they are typically in the range of 2 %–12 % before 2012 and 2 %–6 % after 2012.
The merged deseasonalized anomalies can be directly used for evaluation of ozone trends in the stratosphere. The evaluation of regional ozone trends is discussed in Sect. 5 of our paper. We also created a version of MEGRIDOP in number density through restoration of the seasonal cycle. This was achieved in a manner similar to that applied in creating the merged SAGE-CCI-OMPS dataset (Sofieva et al., 2017b). The best estimates of the amplitude and morphology of the seasonal cycle are provided by MIPAS and MLS, as these two instruments provide global coverage in all seasons. The ozone profiles from OSIRIS and MLS have the smallest biases with respect to ozone soundings (Hubert et al., 2016). For the seasonal cycle of the merged dataset, we computed the mean of MIPAS and MLS seasonal cycles and offset it to the mean of OSIRIS and MLS values (this offset does not depend on season). Using this procedure, the seasonal cycle in the merged dataset has absolute values, which have the smallest biases with respect to the ground-based instruments and a realistic amplitude. An example of a number density MEGRIDOP dataset is shown in Fig. 8.
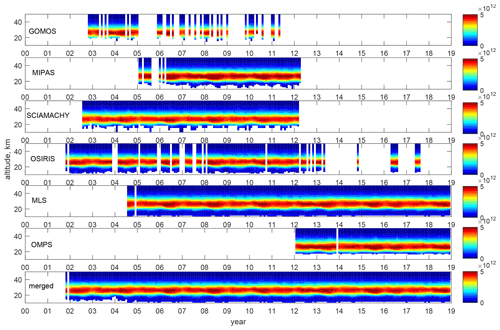
Figure 8An example of number density ozone profiles (in cm−3) for individual instruments and the merged dataset in the spatial bin 0–10∘ N, 0–20∘ E.
The merged dataset allows us to provide a gridded climatology of ozone profiles, i.e., the collection of ozone profiles categorized by calendar month, latitude, longitude and altitude. Figure 9 shows these climatological ozone values for 4 months and at four altitude levels. The polar projections of these distributions are presented in the Supplement (Figs. S2 and S3). As observed in these figures, there is zonal asymmetry associated with the polar vortex in both hemispheres. In other locations, the ozone distributions are rather uniform in longitude.
For evaluation of the regional ozone trends, we exploited the standard approach of multiple linear regression and applied it to the deseasonalized anomalies:
where we model the trend with a simple linear term, QBO30(t) and QBO50(t) are the equatorial winds at 30 and 50 hPa, respectively (http://www.cpc.ncep.noaa.gov/data/indices/, last access: 22 April 2021), F10.7(t) is the monthly average solar 10.7 cm radio flux (https://www.ngdc.noaa.gov/stp/solar/flux.html, last access: 25 April 2021) and ENSO(t) is the 2 month lagged ENSO proxy (https://psl.noaa.gov/enso/mei/, last access: 23 April 2021). The evaluation of trends has been performed for each latitude–longitude bin and for each altitude level separately. Autocorrelations are removed using the Cochrane–Orcutt transformation (Cochrane and Orcutt, 1949).
In our analysis, we consider long-term trends over the years covered by MEGRIDOP and approximate them by a linear function (which describes bulk changes). However, real changes in the atmosphere can be non-linear (Laine et al., 2014): if variations are analyzed on a shorter timescale, they can be different from long-term trends (e.g., Arosio et al., 2019; Chipperfield et al., 2018; Galytska et al., 2019; Strahan et al., 2020). We selected the years after 2003 in order to avoid the influence of a major sudden stratospheric warming in September 2002 on ozone trends at Southern Hemisphere middle and high latitudes (see also the discussion below).
Ozone trends (expressed in percent per decade) estimated at several altitude levels for the years from 2003–2018 are shown in Fig. 10. Figure 11 displays the trends at these altitudes in absolute units, DU km−1 decade−1). In Figs. 10 and 11, black stars indicate the statistically significant trends, i.e., trends different from zero at a 95 % or greater confidence level. The morphology of ozone trends presented in absolute and in relative units look similar. As shown in Figs. 10 and 11, statistically significant trends are observed in the upper stratosphere. A longitudinal structure is clearly visible in the NH mid-latitude trends above 40 km: the trends are significantly larger over Scandinavia and the northern Atlantic Ocean (5 %–6 % decade−1) than over Siberia (∼ 1 % decade−1). The same feature was also observed by Arosio et al. (2019). Enhanced ozone trends over the mid-latitude Atlantic sector are seen in both absolute and relative units, and also at lower altitudes (but the ozone trends are not statistically significant below 40 km).
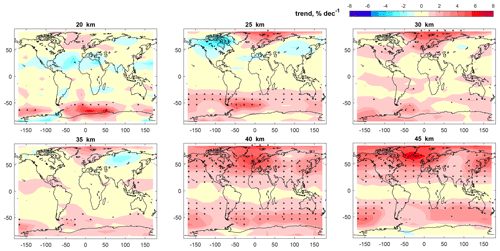
Figure 10Ozone trends (% decade−1) in 2003–2018 for several altitudes. Statistically significant trends are indicated by stars.
We also compared the trends in late 2004–2018, the common measurement period, using MEGRIDOP, only MLS data and the merged SCIAMACHY-OMPS dataset by Arosio et al. (2019). We found that the spatial distributions of ozone trends are similar for the considered datasets (Fig. 12, top). The MEGRIDOP and pure MLS ozone trends in 2004–2018 are similar (as expected, MLS data are used in MEGRIDOP). SCIAMACHY-OMPS trends are somewhat larger, which might be related to the OMPS drift (Kramarova et al., 2018), but within error limits, and the morphology of ozone trends is similar. Specifically interesting is a two-core structure of ozone trends in the NH polar region, and this is seen nearly at all altitude levels (Fig. 12, bottom) for all datasets.
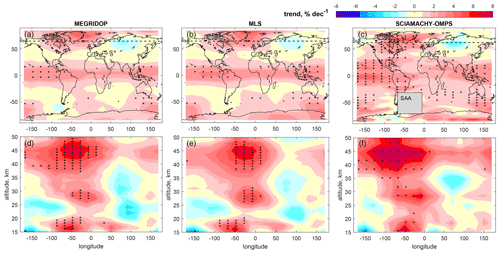
Figure 12(a, b, c) Ozone trends in late 2004–2018 (% decade−1) at 35 km and (d, e, f) longitude–altitude cross section of the ozone trends at ∼ 65∘ N (the latitude is indicated by a dashed line on the top panels). Ozone trends are estimated using the MEGRIDOP (a, d), MLS (b, e) and SCIAMACHY-OMPS datasets (c, f). For the SCIAMACHY-OMPS dataset, ozone trends in the Southern Atlantic Anomaly (SAA) region are not shown because SCIAMACHY data are flagged in this region.
There are several analyses showing that the residual circulation has a pronounced longitudinal two-core structure at Northern Hemisphere high and middle latitudes (e.g., Demirhan Bari et al., 2013; Kozubek et al., 2015). Kozubek et al. (2015) also performed a trend analysis and showed a weakening of the two-core structure, which possibly affects the ozone distribution in the region. Arosio et al. (2019) suggested that this longitudinal structure in the NH mid-latitude ozone trends is due to changes in dynamical processes related to the 3D structure of the Brewer–Dobson circulation. However, the origin of the longitudinal structure of ozone trends requires more detailed investigations in the future, including simulations with chemistry-transport models.
Statistically significant (at 95 % confidence level) positive trends (1 %–2 % decade−1) are also observed at SH mid-latitudes (∼ 40–50∘ S) at 25 km. This is in agreement with other studies of zonally averaged ozone trends (e.g., Arosio et al., 2019; Petropavlovskikh et al., 2019; Sofieva et al., 2017b). In our analysis, there is a zonal asymmetry with larger trends in the sector 50∘ W–10∘ E. At altitudes of 20–25 km, the trend patterns are different in the Northern and Southern Hemispheres.
Comparisons of MEGRIDOP ozone trends at 35 km in Figs. 10 and 12 show larger positive ozone trends in the tropics in the period from 2004–2018 compared to the period from 2003–2018. A pronounced sensitivity of tropical ozone trends at ∼ 35 km to the selection of the period for evaluation of ozone trends has been reported in several papers (e.g., Laine et al., 2014; Arosio et al., 2019; Galytska et al., 2019). As a hypothesis, this might be related to a decadal-scale ozone oscillation resulting from changes in Brewer–Dobson Circulation.
In previous studies (e.g., Petropavlovskikh et al., 2019; Steinbrecht et al., 2017; WMO, 2018), ozone trends have been evaluated at latitudes 60∘ S–60∘ N, i.e., excluding polar regions. In this study, we have made an attempt to also evaluate ozone trends in polar regions. The ozone trends in polar projections are shown in the Supplement.
We found statistically significant positive trends in the NH polar middle stratosphere (25–30 km). In the SH polar regions, the estimated ozone trends are mostly positive, but they are not statistically significant. We found that the estimated trends in the SH polar regions are sensitive to the inclusion of 2002 data into the trend analysis. Quite exceptional (larger) ozone values in 2002 due to a SH major sudden stratospheric warming result in negative, although not statistically significant, ozone trends in the SH polar stratosphere, as expected since 2002 is at the beginning of the time period. If data from 2002 are excluded from the analysis, the estimated trends over Antarctica are not sensitive to the selection of the starting point for the trend analysis. This can be observed, for example, by comparison of ozone trends at 35 km in Fig. 10 (trends for 2003–2018) and Fig. 12 (trends for late 2004 to 2018).
Since natural variability is high in polar regions and the observational period is relatively short, it is quite expected that a simple multiple regression will lead to trend estimates that are not statistically significant. Other methods for trend analysis in polar regions, such as considering seasonal trends (Solomon et al., 2016; Szeląg et al., 2020; Galytska et al., 2019) can be explored in future work. In addition, the relation of winter–spring trends with respect to the position of the polar vortex would be an interesting subject in future studies.
The satellite data quality typically degrades in the UTLS compared to higher levels in the stratosphere. Our merging principle seems to be particularly optimal for the UTLS datasets, as it automatically removes biases, which can be significant in this altitude region. The very large natural ozone variability results in MEGRIDOP trend estimates below 20 km being not statistically significant in most locations.
In this paper, we presented the merged gridded dataset of ozone profiles (MEGRIDOP), which combines ozone data from six limb-viewing satellite instruments. The merged gridded ozone profiles are the monthly means in latitude–longitude bins, and they cover altitudes from 10 to 50 km. This dataset covers the years from 2001–2018 and will be extended regularly in the future.
The merging was performed using aligned deseasonalized anomalies: the merged dataset represents the median of the deseasonalized anomalies from the individual instruments. The merged deseasonalized anomalies can be used directly for evaluation of ozone trends. For other applications, the MEGRIDOP is also available in the form of ozone number density profiles.
The MEGRIDOP dataset can be used in different analyses. As an illustration of one of the possible applications, a climatology of ozone profiles with resolved longitudinal structure has been created. We found zonal asymmetry in the climatological ozone profiles at middle and high latitudes associated with the polar vortex. At northern high latitudes, the amplitude of the seasonal cycle also has a longitudinal dependence.
We evaluated regional ozone trends over the years from 2001–2018 using a multiple linear regression method. Overall, the estimated trends agree well with the trends derived from zonal mean ozone profiles. We found a zonal asymmetry in the upper stratospheric ozone trends at middle and high latitudes in the Northern Hemisphere: the trends are larger over Scandinavia than over Siberia. This feature agrees well with previous analyses and might be due to changes in dynamical processes related to the Brewer–Dobson circulation.
We also estimated regional and vertically resolved ozone trends in the polar regions. As far as we know, this is the first such analysis using limb satellite measurements. We found statistically significant positive trends in the NH polar middle stratosphere (25–30 km). In the SH polar regions, the estimated ozone trends are mostly positive, but they are not statistically significant.
The MEGRIDOP dataset can be used in different analyses. In particular, it can be used for intercomparison of climate data records from ground-based and other satellite measurements and chemistry-transport models in the future.
The dataset is available through open access at https://climate.esa.int/en/projects/ozone/data/ and at ftp://cci_web@ftp-ae.oma.be/esacci (ESA Climate Office, last access: 25 April 2021).
The supplement related to this article is available online at: https://doi.org/10.5194/acp-21-6707-2021-supplement.
VFS designed the study, performed analyses and wrote the major part of the manuscript. MS participated in trend analyses. MS, JT, EK, DD, CR, DZ, AR, CA, JPB, MW, AL, GPS, TvC, LF, NL, MvR and CR provided the data and contributed to analyses and manuscript writing.
Gabriele P. Stiller, Thomas von Clarmann and Michel van Roozendael are editors of ACP, but have not been involved in the evaluation of this paper.
This article is part of the special issue “New developments in atmospheric limb measurements: instruments, methods, and science applications (AMT/ACP inter-journal SI)”.
The work is performed in the framework of the ESA Ozone_cci+ project. The GOMOS ALGOM2s dataset was created in the framework of ESA ALGOM project. The KIT team would like to thank the European Space Agency (ESA) for giving access to MIPAS level-1 data. The SCIAMACHY ozone retrieval was funded in parts by the ESA, the German Academic Exchange Service (DAAD), the German Aerospace Agency (DLR), and the University and State of Bremen. The dataset was calculated with resources provided by the North-German Supercomputing Alliance (HLRN). The study is also a contribution to the German Ministry of Education and Research (BMBF) Synopsis Project. The FMI team thanks the Academy of Finland. The authors thank the Canadian Space Agency. Work at the Jet Propulsion Laboratory, California Institute of Technology, was performed under contract with the National Aeronautics and Space Administration (NASA).
This research has been supported by the European Space Agency (Ozone_cci+ project, contract 4000126562/19/I-NB), the EU Copernicus Climate Change Service for Atmospheric Composition ECVs (contract C3S_312b_Lot2_DLR_2018SC1), the German Academic Exchange Service (DAAD), the German Aerospace Agency (DLR), the University and State of Bremen, the German Federal Ministry for Economic Affairs and Energy (project SEREMISA), the Academy of Finland (the Centre of Excellence of Inverse Modelling and Imaging (decision 336798)), the Canadian Space Agency and the National Aeronautics and Space Administration (Contract 80NM0018D0004).
This paper was edited by Jens-Uwe Grooß and reviewed by two anonymous referees.
Arosio, C., Rozanov, A., Malinina, E., Weber, M., and Burrows, J. P.: Merging of ozone profiles from SCIAMACHY, OMPS and SAGE II observations to study stratospheric ozone changes, Atmos. Meas. Tech., 12, 2423–2444, https://doi.org/10.5194/amt-12-2423-2019, 2019.
Ball, W. T., Alsing, J., Mortlock, D. J., Staehelin, J., Haigh, J. D., Peter, T., Tummon, F., Stübi, R., Stenke, A., Anderson, J., Bourassa, A., Davis, S. M., Degenstein, D., Frith, S., Froidevaux, L., Roth, C., Sofieva, V., Wang, R., Wild, J., Yu, P., Ziemke, J. R., and Rozanov, E. V.: Evidence for a continuous decline in lower stratospheric ozone offsetting ozone layer recovery, Atmos. Chem. Phys., 18, 1379–1394, https://doi.org/10.5194/acp-18-1379-2018, 2018.
Ball, W. T., Alsing, J., Staehelin, J., Davis, S. M., Froidevaux, L., and Peter, T.: Stratospheric ozone trends for 1985–2018: sensitivity to recent large variability, Atmos. Chem. Phys., 19, 12731–12748, https://doi.org/10.5194/acp-19-12731-2019, 2019.
Bourassa, A. E., Degenstein, D. A., Randel, W. J., Zawodny, J. M., Kyrölä, E., McLinden, C. A., Sioris, C. E., and Roth, C. Z.: Trends in stratospheric ozone derived from merged SAGE II and Odin-OSIRIS satellite observations, Atmos. Chem. Phys., 14, 6983–6994, https://doi.org/10.5194/acp-14-6983-2014, 2014.
Bourassa, A. E., Roth, C. Z., Zawada, D. J., Rieger, L. A., McLinden, C. A., and Degenstein, D. A.: Drift-corrected Odin-OSIRIS ozone product: algorithm and updated stratospheric ozone trends, Atmos. Meas. Tech., 11, 489–498, https://doi.org/10.5194/amt-11-489-2018, 2018.
Chipperfield, M. P., Dhomse, S., Hossaini, R., Feng, W., Santee, M. L., Weber, M., Burrows, J. P., Wild, J. D., Loyola, D., and Coldewey-Egbers, M.: On the Cause of Recent Variations in Lower Stratospheric Ozone, Geophys. Res. Lett., 45, 5718–5726, https://doi.org/10.1029/2018GL078071, 2018.
Cochrane, D. and Orcutt, G. H.: Application of Least Squares Regression to Relationships Containing Auto-Correlated Error Terms, J. Am. Stat. Assoc., 44, 32–61, https://doi.org/10.1080/01621459.1949.10483290, 1949.
Dee, D. P., Uppala, S. M., Simmons, A. J., Berrisford, P., Poli, P., Kobayashi, S., Andrae, U., Balmaseda, M. A., Balsamo, G., Bauer, P., Bechtold, P., Beljaars, A. C. M., van de Berg, L., Bidlot, J., Bormann, N., Delsol, C., Dragani, R., Fuentes, M., Geer, A. J., Haimberger, L., Healy, S. B., Hersbach, H., Hólm, E. V, Isaksen, L., Kållberg, P., Köhler, M., Matricardi, M., McNally, A. P., Monge-Sanz, B. M., Morcrette, J.-J., Park, B.-K., Peubey, C., de Rosnay, P., Tavolato, C., Thépaut, J.-N., and Vitart, F.: The ERA-Interim reanalysis: configuration and performance of the data assimilation system, Q. J. Roy. Meteorol. Soc., 137, 553–597, https://doi.org/10.1002/qj.828, 2011.
Degenstein, D. A., Bourassa, A. E., Roth, C. Z., and Llewellyn, E. J.: Limb scatter ozone retrieval from 10 to 60 km using a multiplicative algebraic reconstruction technique, Atmos. Chem. Phys., 9, 6521–6529, https://doi.org/10.5194/acp-9-6521-2009, 2009.
Demirhan Bari, D., Gabriel, A., Körnich, H., and Peters, D. W. H.: The effect of zonal asymmetries in the Brewer-Dobson circulation on ozone and water vapor distributions in the northern middle atmosphere, J. Geophys. Res.-Atmos., 118, 3447–3466, https://doi.org/10.1029/2012JD017709, 2013.
ESA climate office: Ozone data, available at: https://climate.esa.int/en/projects/ozone/data/ and ftp://cci_web@ftp-ae.oma.be/esacci, last access: 25 April 2021.
Galytska, E., Rozanov, A., Chipperfield, M. P., Dhomse, Sandip. S., Weber, M., Arosio, C., Feng, W., and Burrows, J. P.: Dynamically controlled ozone decline in the tropical mid-stratosphere observed by SCIAMACHY, Atmos. Chem. Phys., 19, 767–783, https://doi.org/10.5194/acp-19-767-2019, 2019.
Hubert, D., Lambert, J.-C., Verhoelst, T., Granville, J., Keppens, A., Baray, J.-L., Bourassa, A. E., Cortesi, U., Degenstein, D. A., Froidevaux, L., Godin-Beekmann, S., Hoppel, K. W., Johnson, B. J., Kyrölä, E., Leblanc, T., Lichtenberg, G., Marchand, M., McElroy, C. T., Murtagh, D., Nakane, H., Portafaix, T., Querel, R., Russell III, J. M., Salvador, J., Smit, H. G. J., Stebel, K., Steinbrecht, W., Strawbridge, K. B., Stübi, R., Swart, D. P. J., Taha, G., Tarasick, D. W., Thompson, A. M., Urban, J., van Gijsel, J. A. E., Van Malderen, R., von der Gathen, P., Walker, K. A., Wolfram, E., and Zawodny, J. M.: Ground-based assessment of the bias and long-term stability of 14 limb and occultation ozone profile data records, Atmos. Meas. Tech., 9, 2497–2534, https://doi.org/10.5194/amt-9-2497-2016, 2016.
Jia, J., Rozanov, A., Ladstätter-Weißenmayer, A., and Burrows, J. P.: Global validation of SCIAMACHY limb ozone data (versions 2.9 and 3.0, IUP Bremen) using ozonesonde measurements, Atmos. Meas. Tech., 8, 3369–3383, https://doi.org/10.5194/amt-8-3369-2015, 2015.
Kozubek, M., Krizan, P., and Lastovicka, J.: Northern Hemisphere stratospheric winds in higher midlatitudes: longitudinal distribution and long-term trends, Atmos. Chem. Phys., 15, 2203–2213, https://doi.org/10.5194/acp-15-2203-2015, 2015.
Kramarova, N. A., Bhartia, P. K., Jaross, G., Moy, L., Xu, P., Chen, Z., DeLand, M., Froidevaux, L., Livesey, N., Degenstein, D., Bourassa, A., Walker, K. A., and Sheese, P.: Validation of ozone profile retrievals derived from the OMPS LP version 2.5 algorithm against correlative satellite measurements, Atmos. Meas. Tech., 11, 2837–2861, https://doi.org/10.5194/amt-11-2837-2018, 2018.
Kyrölä, E., Tamminen, J., Sofieva, V., Bertaux, J. L., Hauchecorne, A., Dalaudier, F., Fussen, D., Vanhellemont, F., Fanton d'Andon, O., Barrot, G., Guirlet, M., Mangin, A., Blanot, L., Fehr, T., Saavedra de Miguel, L., and Fraisse, R.: Retrieval of atmospheric parameters from GOMOS data, Atmos. Chem. Phys., 10, 11881–11903, https://doi.org/10.5194/acp-10-11881-2010, 2010.
Kyrölä, E., Laine, M., Sofieva, V., Tamminen, J., Päivärinta, S.-M., Tukiainen, S., Zawodny, J., and Thomason, L.: Combined SAGE II–GOMOS ozone profile data set for 1984–2011 and trend analysis of the vertical distribution of ozone, Atmos. Chem. Phys., 13, 10645–10658, https://doi.org/10.5194/acp-13-10645-2013, 2013.
Laine, M., Latva-Pukkila, N., and Kyrölä, E.: Analysing time-varying trends in stratospheric ozone time series using the state space approach, Atmos. Chem. Phys., 14, 9707–9725, https://doi.org/10.5194/acp-14-9707-2014, 2014.
Livesey, N. J., Read, W. G., Froidevaux, L., Lambert, A., Manney, G. L., Pumphrey, H. C., Santee, M. L., Schwartz, M. J., Wang, S., Cofield, R. E., Cuddy, D. T., Fuller, R. A., Jarnot, R. F., Jiang, J. H., Knosp, B. W., Stek, P. C., Wagner, P. A., and Wu, D. L.: EOS MLS Version 3.3 and 3.4 Level 2 data quality and description document, available at: http://mls.jpl.nasa.gov/data/v3_data_quality_document.pdf (last access: 23 April 2021), 2013.
Maycock, A. C., Randel, W. J., Steiner, A. K., Karpechko, A. Y., Christy, J., Saunders, R., Thompson, D. W. J., Zou, C.-Z., Chrysanthou, A., Luke Abraham, N., Akiyoshi, H., Archibald, A. T., Butchart, N., Chipperfield, M., Dameris, M., Deushi, M., Dhomse, S., Di Genova, G., Jöckel, P., Kinnison, D. E., Kirner, O., Ladstädter, F., Michou, M., Morgenstern, O., O'Connor, F., Oman, L., Pitari, G., Plummer, D. A., Revell, L. E., Rozanov, E., Stenke, A., Visioni, D., Yamashita, Y., and Zeng, G.: Revisiting the Mystery of Recent Stratospheric Temperature Trends, Geophys. Res. Lett., 45, 9919–9933, https://doi.org/10.1029/2018GL078035, 2018.
Petropavlovskikh, I., Godin-Beekmann, S., Hubert, D., Damadeo, R., Hassler, B., and Sofieva, V.: SPARC/IO3C/GAW Report on Long-term Ozone Trends and Uncertainties in the Stratosphere, in: 9th assessment report of the SPARC project, edited by: Kenntner, M. and Ziegele, B., available at: https://elib.dlr.de/126666/ (last access: 28 April 2021), GAW Report No. 241, WCRP Report 17/2018, International Project Office at DLR-IPA, 2019.
Sofieva, V. F., Rahpoe, N., Tamminen, J., Kyrölä, E., Kalakoski, N., Weber, M., Rozanov, A., von Savigny, C., Laeng, A., von Clarmann, T., Stiller, G., Lossow, S., Degenstein, D., Bourassa, A., Adams, C., Roth, C., Lloyd, N., Bernath, P., Hargreaves, R. J., Urban, J., Murtagh, D., Hauchecorne, A., Dalaudier, F., van Roozendael, M., Kalb, N., and Zehner, C.: Harmonized dataset of ozone profiles from satellite limb and occultation measurements, Earth Syst. Sci. Data, 5, 349–363, https://doi.org/10.5194/essd-5-349-2013, 2013.
Sofieva, V. F., Kalakoski, N., Päivärinta, S.-M., Tamminen, J., Laine, M., and Froidevaux, L.: On sampling uncertainty of satellite ozone profile measurements, Atmos. Meas. Tech., 7, 1891–1900, https://doi.org/10.5194/amt-7-1891-2014, 2014.
Sofieva, V. F., Ialongo, I., Hakkarainen, J., Kyrölä, E., Tamminen, J., Laine, M., Hubert, D., Hauchecorne, A., Dalaudier, F., Bertaux, J.-L., Fussen, D., Blanot, L., Barrot, G., and Dehn, A.: Improved GOMOS/Envisat ozone retrievals in the upper troposphere and the lower stratosphere, Atmos. Meas. Tech., 10, 231–246, https://doi.org/10.5194/amt-10-231-2017, 2017a.
Sofieva, V. F., Kyrölä, E., Laine, M., Tamminen, J., Degenstein, D., Bourassa, A., Roth, C., Zawada, D., Weber, M., Rozanov, A., Rahpoe, N., Stiller, G., Laeng, A., von Clarmann, T., Walker, K. A., Sheese, P., Hubert, D., van Roozendael, M., Zehner, C., Damadeo, R., Zawodny, J., Kramarova, N., and Bhartia, P. K.: Merged SAGE II, Ozone_cci and OMPS ozone profile dataset and evaluation of ozone trends in the stratosphere, Atmos. Chem. Phys., 17, 12533–12552, https://doi.org/10.5194/acp-17-12533-2017, 2017b.
Solomon, S., Ivy, D. J., Kinnison, D., Mills, M. J., Neely, R. R., and Schmidt, A.: Emergence of healing in the Antarctic ozone layer, Science, 353, 269–274, https://doi.org/10.1126/science.aae0061, 2016.
Steinbrecht, W., Froidevaux, L., Fuller, R., Wang, R., Anderson, J., Roth, C., Bourassa, A., Degenstein, D., Damadeo, R., Zawodny, J., Frith, S., McPeters, R., Bhartia, P., Wild, J., Long, C., Davis, S., Rosenlof, K., Sofieva, V., Walker, K., Rahpoe, N., Rozanov, A., Weber, M., Laeng, A., von Clarmann, T., Stiller, G., Kramarova, N., Godin-Beekmann, S., Leblanc, T., Querel, R., Swart, D., Boyd, I., Hocke, K., Kämpfer, N., Maillard Barras, E., Moreira, L., Nedoluha, G., Vigouroux, C., Blumenstock, T., Schneider, M., García, O., Jones, N., Mahieu, E., Smale, D., Kotkamp, M., Robinson, J., Petropavlovskikh, I., Harris, N., Hassler, B., Hubert, D., and Tummon, F.: An update on ozone profile trends for the period 2000 to 2016, Atmos. Chem. Phys., 17, 10675–10690, https://doi.org/10.5194/acp-17-10675-2017, 2017.
Steiner, A. K., Ladstädter, F., Randel, W. J., Maycock, A. C., Fu, Q., Claud, C., Gleisner, H., Haimberger, L., Ho, S.-P., Keckhut, P., Leblanc, T., Mears, C., Polvani, L. M., Santer, B. D., Schmidt, T., Sofieva, V., Wing, R., and Zou, C.-Z.: Observed temperature changes in the troposphere and stratosphere from 1979 to 2018, J. Clim., 33, 8165–8194, https://doi.org/10.1175/JCLI-D-19-0998.1, 2020.
Strahan, S. E., Smale, D., Douglass, A. R., Blumenstock, T., Hannigan, J. W., Hase, F., Jones, N. B., Mahieu, E., Notholt, J., Oman, L. D., Ortega, I., Palm, M., Prignon, M., Robinson, J., Schneider, M., Sussmann, R., and Velazco, V. A.: Observed Hemispheric Asymmetry in Stratospheric Transport Trends From 1994 to 2018, Geophys. Res. Lett., 47, e2020GL088567, https://doi.org/10.1029/2020GL088567, 2020.
Szeląg, M. E., Sofieva, V. F., Degenstein, D., Roth, C., Davis, S., and Froidevaux, L.: Seasonal stratospheric ozone trends over 2000–2018 derived from several merged data sets, Atmos. Chem. Phys., 20, 7035–7047, https://doi.org/10.5194/acp-20-7035-2020, 2020.
Toohey, M. and von Clarmann, T.: Climatologies from satellite measurements: the impact of orbital sampling on the standard error of the mean, Atmos. Meas. Tech., 6, 937–948, https://doi.org/10.5194/amt-6-937-2013, 2013.
von Clarmann, T., Glatthor, N., Grabowski, U., Höpfner, M., Kellmann, S., Kiefer, M., Linden, A., Tsidu, G. M., Milz, M., Steck, T., Stiller, G. P., Wang, D. Y., Fischer, H., Funke, B., Gil-López, S., López-Puertas, M., Mengistu Tsidu, G., Milz, M., Steck, T., Stiller, G. P., Wang, D. Y., Fischer, H., Funke, B., Gil-López, S., and López-Puertas, M.: Retrieval of temperature and tangent altitude pointing from limb emission spectra recorded from space by the Michelson Interferometer for Passive Atmospheric Sounding (MIPAS), J. Geophys. Res., 108, 4736, https://doi.org/10.1029/2003JD003602, 2003.
von Clarmann, T., Höpfner, M., Kellmann, S., Linden, A., Chauhan, S., Funke, B., Grabowski, U., Glatthor, N., Kiefer, M., Schieferdecker, T., Stiller, G. P., and Versick, S.: Retrieval of temperature, H2O, O3, HNO3, CH4, N2O, ClONO2 and ClO from MIPAS reduced resolution nominal mode limb emission measurements, Atmos. Meas. Tech., 2, 159–175, https://doi.org/10.5194/amt-2-159-2009, 2009.
WMO (World Meteorological Organization): Scientific Assessment of Ozone Depletion, Global Ozone Research and Monitoring Project-Report No. 52, Geneva, Switzerland, available at: https://www.esrl.noaa.gov/csd/assessments/ozone/ (last access: 23 April 2021), 2014.
WMO (World Meteorological Organization): Scientific Assessment of Ozone Depletion, Global Ozone Research and Monitoring Project-Report No. 58, 588 pp., Geneva, Switzerland, 2018.
Zawada, D. J., Rieger, L. A., Bourassa, A. E., and Degenstein, D. A.: Tomographic retrievals of ozone with the OMPS Limb Profiler: algorithm description and preliminary results, Atmos. Meas. Tech., 11, 2375–2393, https://doi.org/10.5194/amt-11-2375-2018, 2018.