the Creative Commons Attribution 4.0 License.
the Creative Commons Attribution 4.0 License.
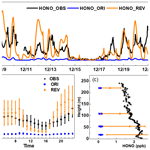
Improving the representation of HONO chemistry in CMAQ and examining its impact on haze over China
Shuping Zhang
Golam Sarwar
Jia Xing
Chaoyang Xue
Arunachalam Sarav
Dian Ding
Haotian Zheng
Yujing Mu
Fengkui Duan
Tao Ma
We compare Community Multiscale Air Quality (CMAQ) model predictions with measured nitrous acid (HONO) concentrations in Beijing, China, for December 2015. The model with the existing HONO chemistry in CMAQ severely underestimates the observed HONO concentrations with a normalized mean bias of −97 %. We revise the HONO chemistry in the model by implementing six additional heterogeneous reactions in the model: the reaction of nitrogen dioxide (NO2) on ground surfaces, the reaction of NO2 on aerosol surfaces, the reaction of NO2 on soot surfaces, the photolysis of aerosol nitrate, the nitric acid displacement reaction, and the hydrochloric acid displacement reaction. The model with the revised chemistry substantially increases HONO predictions and improves the comparison with observed data with a normalized mean bias of −5 %. The photolysis of HONO enhances daytime hydroxyl radical by almost a factor of 2. The enhanced hydroxyl radical concentrations compare favorably with observed data and produce additional sulfate via the reaction with sulfur dioxide, aerosol nitrate via the reaction with nitrogen dioxide, and secondary organic aerosols via the reactions with volatile organic compounds. The additional sulfate stemming from revised HONO chemistry improves the comparison with observed concentration; however, it does not close the gap between model prediction and the observation during polluted days.
- Article
(2339 KB) - Full-text XML
-
Supplement
(616 KB) - BibTeX
- EndNote
China has been suffering from haze pollution (Lelieveld et al., 2015) in which secondary particles contribute more than 70 % to the haze formation (Huang et al., 2014; Quan et al., 2014; Zheng et al., 2015; Guo et al., 2014). However, the mechanism for the formation of high levels of secondary particles is not yet clearly understood, and most current air quality models tend to underestimate particle concentrations compared with observed data in China. Several secondary particle formation pathways have been proposed, such as (1) sulfate (SO) formation via the heterogeneous oxidation of sulfur dioxide (SO2) promoted by hydrogen peroxide (H2O2) and/or (2) nitrogen dioxide (NO2) on mineral dust (He et al., 2014; Huang et al., 2015; Ye et al., 2018), (3) aqueous-phase oxidation of SO2 promoted by NO2 in particle-bound water film (Wang et al., 2016; Li et al., 2017), (4) aqueous-phase oxidation of SO2 by nitrous acid (HONO) produced from the photolysis of aerosol nitrate (NO) in particle-bound water (Wang et al., 2016; Li et al., 2017), and (5) NO formation via efficient hydrolysis of dinitrogen pentoxide (N2O5) on aerosol surfaces (Wang et al., 2017; Kulmala, 2018). However, the gap between the model predictions and observed SO is persistent and still large (Zhang et al., 2019c).
Previous studies suggested that the underestimation of atmospheric oxidation capacity during haze limited the formation of secondary particles (Tsona and Du, 2019; Sun et al., 2013; Gen et al., 2019). As a hydroxyl radical (OH) source, HONO plays an important role in the oxidation of precursors (Kleffmann et al., 2005; Stutz et al., 2002). However, the large underestimation of HONO (up to the parts per billion (ppb) level) is prevalent during haze simulations around the world (Li et al., 2012; Fu et al., 2019; Zhang et al., 2019d). Moreover, HONO underestimation is reported to be highly related to the formation of fine particulate matter (PM2.5) (Wang et al., 2015; Xue et al., 2020), particularly for secondary PM2.5. Compared with summer, HONO concentrations in winter tend to be high when secondary particle underestimation occurs (D. D. Li et al., 2018; Zhang et al., 2019b). The underestimation of HONO may partly explain the phenomenon of insufficient oxidant for the formation of secondary particles during the winter haze (L. Li et al., 2018a, b).
Sarwar et al. (2008) compared Community Multiscale Air Quality (CMAQ) predictions with HONO concentrations measured in Philadelphia, PA, USA, during a summer month (July 2001) and reported that the model with only gas-phase chemistry seriously underestimates observed concentrations. They implemented HONO emissions from motor vehicles, the heterogeneous reaction on the ground and aerosol surfaces, and the photolysis of nitric acid (HNO3) deposited on environmental surfaces, which improved predicted HONO concentrations; however, the underprediction persisted. The model with the revised chemistry enhanced OH and ozone (O3) concentrations. Li et al. (2010) examined the impact of HONO chemistry in Mexico City using the Weather Research and Forecasting model, coupled with chemistry (WRF-Chem). They considered five different HONO reactions: (1) the existing homogeneous reaction between NO (nitric oxide) and OH, (2) the added heterogeneous reaction of NO2 on the aerosol surfaces, (3) the added heterogeneous reaction of NO2 on the ground surfaces, (4) the added heterogeneous reaction of NO2 with semi-volatile organics, and (5) the added heterogeneous reaction of NO2 reaction with freshly emitted soot. The model successfully reproduced observed HONO concentrations in Mexico City during March 2006. The model with the HONO chemistry increased OH, HO2 (hydroperoxyl radical), O3, secondary organic aerosols (SOAs), NO, and ammonium (NH) and improved the comparison with observed data. The enhancements were particularly high in the morning. However, the impact on SO was negligible. Czader et al. (2012) compared CMAQv5.3 predictions with HONO measured during August and September 2006 in Houston, TX, USA, and also reported that the model with the gas phase alone was not sufficient to explain the observed data and predicted concentrations. They added HONO emissions, NO2 hydrolysis, active NO2 chemistry, and conversion of NO2 into HONO on organic materials covered surfaces, which improved model performance for HONO and, subsequently, increased OH and O3 concentrations.
Fu et al. (2019) studied a 5 d episode (4–8 January 2017) in the Pearl River Delta of China during which high levels of particles, O3, and HONO concentrations were measured. They implemented four additional reactions for HONO production into the model: (1) relative-humidity-dependent heterogeneous reaction of NO2, (2) light-dependent heterogeneous reaction of NO2, (3) photolysis of NO3, and (4) photolysis of HNO3 on surfaces. The model with the additional chemistry successfully reproduced measured HONO concentrations, which subsequently enhanced and improved O3 and PM2.5 predictions. Xing et al. (2019) examined the impact of HONO chemistry on SOA in the Beijing–Tianjin–Hebei area (BTH) of China using the WRF-Chem model during 9–26 January 2014. They employed the homogeneous and heterogeneous HONO chemistry of Li et al. (2010) and reported that the HONO chemistry could increase the average SOA concentration by ∼ 46 %. Zhang et al. (2019a) employed the WRF-Chem model to examine the impact of HONO chemistry on OH, HO2, and SOA concentrations in the BTH region during a winter haze period (29 November–3 December 2017). They employed six HONO sources in the model: (1) traffic emissions, (2) soil emissions, (3) biomass burning emissions, (4) indoor emissions, (5) heterogeneous reaction on aerosol surfaces, and (6) heterogeneous reaction on ground surfaces. The model reproduced observed HONO concentrations and substantially elevated OH, HO2, and SOA concentrations.
In this study, we employ the Community Multiscale Air Quality (CMAQ) model to simulate and compare HONO predictions with observed data from the field campaign in Beijing. The field campaign was conducted during 7–22 December 2015, in Beijing, China, during which high concentrations of HONO and aerosols were measured.
2.1 Modeling framework and homogeneous HONO chemistry
The Community Multiscale Air Quality (CMAQv5.3) (USEPA, 2019) (https://www.epa.gov/cmaq, last access: 14 October 2021) was used widely in this study. CMAQv5.3 includes the representation of important atmospheric processes and has been used widely in air quality studies in many countries, including China (Byun and Schere, 2006; Sarwar et al., 2008; Xing et al., 2015). The modeling domain covered China and consisted of 182 × 232 horizontal grid cells with a 27 × 27 km horizontal resolution and 14 vertical layers encompassing the surface to 100 hPa. The first layer height of the model was about 36 m. The static initial and boundary conditions from CMAQv5.3 were used for the study. A 22 d model spin-up period was used to minimize the effect of initial conditions on model predictions. The Carbon Bond 6 (version 3, CB6r3) (Yarwood et al., 2010) chemical mechanism that contain six homogeneous reactions related to HONO (Table 1) was used without any modification. The calculated equilibrium constant in CB6 (Kaiser and Wu, 1977) is similar to reported rate constants by Chan et al. (1976a, b; 5 × 10−20 in CB6 vs. 6 × 10−20 in Chan et al. , 1976a, b). CMAQv5.3 contains a treatment of heterogeneous conversion of NO2 at aerosol and ground surfaces (Sarwar et al., 2008), in which uptake coefficient at aerosol surfaces and area density of ground surfaces were revised in this study (Sect. 2.2). CMAQv5.3 accounts for HONO emissions from motor vehicles as 0.008 × NOx emissions which were kept the same (NOX denotes oxides of nitrogen, NO + NO2). Photolysis rate coefficients (min−1) in CMAQv5.3 (J values) are computed for photodissociation reactions by Eq. (1). Absorption cross-section and quantum yield data suggested by the International Union of Pure and Applied Chemistry (IUPAC) are used for calculating photolysis rate coefficients of HONO (Table 1) (https://iupac-aeris.ipsl.fr/htdocs/datasheets/pdf, last access: 14 October 2021). Absorption cross-section and quantum yield data suggested by the IUPAC for NTR (organic nitrate) are used for calculating photolysis rate coefficients of CRON (nitro-cresol) (Table 1) (https://iupac-aeris.ipsl.fr/htdocs/datasheets/pdf, last access: 14 October 2021).
where F(λ) is the actinic flux (photons cm−2 min−1 nm−1), σi(λ) the absorption cross section for the molecule undergoing photodissociation (cm2 molec.−1), φi(λ) the quantum yield of the photolysis reaction (molecules per photon), and λ the wavelength (nm).
We also instrumented the model with the integrated reaction rate (IRR) option, which enabled the contribution of each reaction to the predicted HONO concentrations to be estimated (Czader et al., 2013). The Sulfur Tracking Model in CMAQv5.3 was used to quantitatively calculate the contribution of each reaction to predicted SO concentration (Mathur et al., 2008).
Table 1Gas-phase chemical reactions related to HONO in CB6r3.
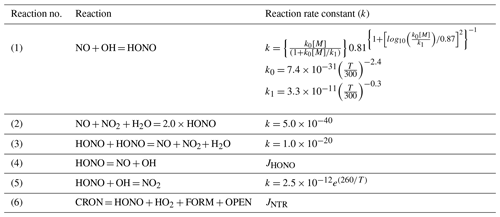
Note the following: NO is nitric oxide; NO2 is nitrogen dioxide; OH is the hydroxyl radical; HO2 is the hydroperoxy radical; H2O is water vapor; HONO is nitrous acid; CRON is nitro-cresol; FORM is formaldehyde; OPEN is the aromatic ring open product; [M] is the total pressure (molecules cm−3); T is air temperature (K); and k is the rate constant. First-order rate constants are given per second (s−1); second-order rate constants are in units of cm3 molec.−1 s−1; and third-order constants are in units of cm6 molec.−2 s−1. CMAQv5.3 converts units of cm molec.−1 s−1 into units of ppm min−1 before solving the system of ordinary differential equations for chemistry. JHONO is the photolysis of HONO, and JNTR is the photolysis of NTR (organic nitrate).
2.2 Heterogeneous HONO chemistry
The heterogeneous formation of HONO has been studied for several decades (Fig. S1). The long research history of HONO heterogeneous reaction can be found in Finlayson-Pitts (2000). The understanding of heterogeneous HONO chemical reactions and parameter method is evolving. Investigators have proposed hydrolysis of NO2 on humid aerosol surfaces, heterogeneous conversion of NO2 on ground surfaces, photolysis of NO, catalytical formation on soot particles, and acid displacement processes in the atmosphere during the past several years (Liu et al., 2014; Karamchandani et al., 2015; Tong et al., 2016; Lu et al., 2018; Xu et al., 2019; Gen et al., 2019; Zhang et al., 2019d; Ye et al., 2016, 2017; Vandenboer et al., 2015; Stemmler et al., 2006). Xue et al. (2020) and Liu et al. (2019) recently measured summertime atmospheric HONO concentrations in a rural area in China and performed simulations using a box model with updated chemical reactions for HONO production published in the literature. They reported that the simulations generally reproduced observed HONO concentrations using the updated HONO chemical reactions. However, the box model did not consider horizontal and vertical transportation, limiting the impact of HONO formation on air quality. We implement these updated chemical reactions into a three-dimensional (3D) air quality model, CMAQv5.3, to examine their impacts on air quality.
Hydrolysis processes on humid aerosol surfaces are an important HONO-producing reaction in the atmosphere (An et al., 2012; Cui et al., 2018). And we use the uptake coefficient (Table 2) employed by Liu et al. (2019) at nighttime (Reaction 7a). The selection criteria and possible ranges of the uptake coefficient are discussed in the Supplement. The reaction on aerosols can be enhanced by light (Zhang et al., 2019b); thus, we use a radiation-dependent uptake coefficient during daytime (Reaction 7b). We use CMAQv5.3-calculated aerosol surface area-to-volume ratio () to calculate the rate constant for the reaction on aerosol surfaces. Heterogeneous conversion of NO2 on ground surfaces has also been studied intensively in the laboratory and field (D. D. Li et al., 2018). Vertical nighttime profile measurements suggest that heterogeneous HONO formation on the ground is the dominant reaction; thus, we also use this reaction. Similar to the heterogeneous reaction on aerosol surfaces, we employ an uptake coefficient used by Liu et al. (2019) for the reaction at night (Reaction 8a) and a radiation-dependent uptake coefficient during daytime (Reaction 8b). Following the suggestions of Vogel et al. (2003), Li et al. (2019), and Liu et al. (2019), we use a value of (, where S′ represents the geometric surface area of the first layer, 1.7 is the effective surface factor per ground surface in first layer, and H is the model's first-layer height) for the surface area-to-volume ratio of the ground () to calculate the rate constant for the reaction on ground surfaces. We also conducted sensitivity analysis using the value of , which is suggested from Voogt and Oke (1997). The result suggests slightly higher concentrations but with similar model performance (details in Fig. S4 in the Supplement).
Ye et al. (2016) proposed that the photolysis of NO can lead to HONO production in the atmosphere and reported that its photolysis rate coefficients can be several hundred times faster than the photolysis rate coefficients of HNO3. Bao et al. (2018) also reported similar photolysis rate coefficients of NO. Fu et al. (2019) used this high photolysis rate coefficient in their study to examine the wintertime HONO production in Hong Kong. However, Romer et al. (2018) reported that such high photolysis rate coefficients of NO are not consistent with observed data over the Yellow Sea and should not be used in air quality models. They suggested that the photolysis rate coefficients of NO in air quality models should be 1 to 30 times the photolysis rate coefficient of HNO3. For photolysis of NO, we use a photolysis rate coefficient of 30 times the photolysis rate coefficient of HNO3 (Reaction 9).
HONO formation on soot particles can be catalytically enhanced in the presence of artificial solar radiation and lead to persistent reactivity of soot over long periods (Monge et al., 2010). The surface of soot particles as a heterogeneous conversion media has been reported by several studies (Monge et al., 2010; Liu et al., 2014; Spataro and Ianniello, 2014; Cui et al., 2018). The reported heterogeneous uptake coefficient on soot ranges from 10−8 to 10−6, with HONO yields ranging between 50 % and 100 % (Spataro et al., 2013). This heterogeneous soot photochemistry potentially may contribute to daytime HONO concentration. We also employ the reaction using the upper limit of the reported uptake coefficient and calculate the HONO formation rate following Spataro et al. (2013) (Reaction 10).
VandenBoer et al. (2013) reported that deposited HONO can react with carbonates or soil at night and, subsequently, be released from the soil during the day by reactions with atmospheric HNO3 and HCl (hydrochloric acid). They suggest that this acid displacement process can contribute to a substantial fraction of daytime HONO. We also use this process (Reactions 11 and 12) and employ a parameter similar to that of Liu et al. (2019), except that we utilize a displacement efficiency of 6 % for HNO3 and 20 % for HCl following VandenBoer et al. (2015). The dry deposition velocities of HNO3 and HCl in CMAQ are calculated using a big-leaf resistance model (Wesely, 1989, 2007). Calculated deposition velocities fall in the reported ranges of values by Jaeglé et al. (2018) (details in the Supplement).
Zhou et al. (2003) reported that HNO3 deposited on environmental surfaces can undergo rapid photolysis, leading to daytime HONO production. Several studies (Sarwar et al., 2008; Fu et al., 2019; Liu et al., 2019) included such a reaction in their models. However, we do not include it because the rate constant has high uncertainty, and it could also pose a problem for performing long-term model simulations. In the long term (annual and multiyear), the deposited amount of HNO3 could accumulate with time, which could continue increasing the HONO production rates with time. Soil can emit HONO and other nitrogen-containing compounds (Su et al., 2011; Oswald et al., 2013). Rasool et al. (2019) implemented these emissions into CMAQv5.3 by using a mechanistic representation of the underlying processes and examined their impacts on air quality over North America. The impacts of HONO emitted from soil are generally low, and we do not include these emissions in this study.
Table 2Heterogeneous HONO reactions used in this study.
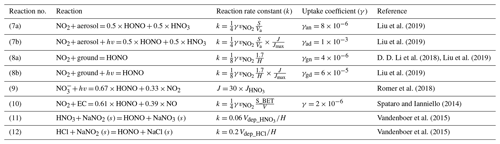
Note the following: k is the first-order rate constant (s−1); γ is the heterogeneous uptake coefficient (−); γan is the nighttime heterogeneous uptake coefficient on aerosol; γad is the daytime heterogeneous uptake coefficient on aerosol; γgn is the nighttime heterogeneous uptake coefficient on ground; γgd is the daytime heterogeneous uptake coefficient on ground; is the density of aerosol surface, is the density of ground surface; v is the mean molecular speed (m s−1); HNO3 is nitric acid; NaNO2 is sodium nitrite; NaCl is sodium chloride; J is the NO2 photolysis rate coefficient; Jmax is the maximum NO2 photolysis rate coefficient; Vdep_HNO3 is the deposition velocity of HNO3 (m s−1); VHCl is the deposition velocity of HCl (m s−1); H is the first-layer height (m); and is the BET surface area-to-volume ratio that we calculate as follows: CMAQv5.3-predicted elemental carbon (EC) (µg m−3) × 1.0 × 10−6 (g µg−1) × 122 m2 g−1; NO is aerosol nitrate; and EC is elemental carbon; s represents the solid phase. Reactions (7a), (7b), (8a), and (8b) are revised from CMAQv5.3, while Reactions (9), (10), (11), and (12) are newly added reactions.
2.3 Simulation cases
We performed two different simulations using CMAQv5.3 for 7–22 December 2015. One simulation denoted by “ORI” used the gas-phase HONO chemistry in CB6r3 along with the heterogeneous hydrolysis of NO2 in CMAQv5.3. The implementation of the heterogeneous hydrolysis of NO2 in CMAQ has previously been described by Sarwar et al. (2008). They accounted for aerosol surface area as well as the ground surface area provided by leaves and building and other structures. Leaf area was estimated using 2 × LAI (LAI is the leaf area index, and H is the surface layer height in the model) while building, and other structure surface areas were estimated using 0.002 × PURB (PURB is the percent urban area of a grid cell in the model). The other simulation denoted by “REV” used the gas-phase HONO chemistry in CB6r3 and the heterogeneous reactions presented in Table 2. For this simulation, we removed the heterogeneous hydrolysis of NO2 in CMAQv5.3 and used the heterogeneous reactions presented in Table 2. Both simulations used the same HONO emissions (Sect. 2.1). We also completed several additional sensitivity simulations as discussed in Sect. 3.0.
We used the ABaCAS national emissions inventory (http://www.abacas-dss.com, last access: 14 October 2021) which resulted in great performance in simulating both NO2 and fine particles (PM2.5). In previous studies, Zhao et al. (2018) and Zheng et al. (2019) used these emissions and reported a normalized mean bias of 4 % for NO2 and −17 % for PM2.5 (normalized mean bias (NMB) = 100 × ∑(Mi-Oi) Oi, Oi is observed HONO concentration, and Mi is the simulated HONO concentration in model; Jaeglé et al., 2018). Meteorological fields for CMAQv5.3 were simulated using the Weather Research and Forecasting model version 3.8 (WRFv3.8) (Skamarock and Klemp, 2008). WRF has consistent parameterization for cloud fraction simulation, as well as other climate models (see Xu and Krueger, 1991, and Xu and Randall, 1996, for a review on these topics). We compared WRF predictions with observed temperature, wind speed, and water vapor mixing ratio in China (Fig. S2). Mean bias (MB) and root mean square error (RMSE) for temperature and wind speed and MB for water vapor mixing ratio meet the benchmark limits suggested by Emery et al. (2001).
2.4 Observation data
A field campaign was conducted during 7–22 December 2015, at the Research Center for Eco-Environmental Sciences (40.01∘ N, 116.35∘ E) to measure atmospheric pollutants and meteorological parameters. Atmospheric concentrations of HONO were measured using a stripping coil (SC) equipped with an ion chromatograph (IC). The details of the instrument have been described elsewhere (Xue et al., 2019). We also completed a statistical analysis of the measurements from the instrument with data from three other methods and concluded that it can provide reliable measurements. The instrument has a minimum detection limit of 4.0 ppt and has been used in several field campaigns (Xue et al., 2019). The concentrations of NO2 and NOx were measured by a nitrogen oxide analyzer (Thermo 42i, Thermo Fisher, USA). Sulfur dioxide (SO2) was measured by a pulsed fluorescence analyzer (Thermo 43i, Thermo Fisher, USA). Fine particles (PM2.5) were measured using an aerosol monitor (TSI, Thermo Fisher TEOM 1405). Relative humidity (RH), temperature, wind speed (WS), wind direction (WD), and other metrological data were measured by an automatic weather-monitoring system. Daily atmospheric SO and NO samples were collected on the roof of a three-story building on the campus of Tsinghua University in Beijing (40.0∘ N, 116.3∘ E) and measured by ion chromatography. Details of the measurements method are described by Ma et al. (2020). The hourly averaged concentrations of the main chemical species of PM2.5 were measured by the Gas and Aerosol Compositions Monitor (IGAC, Fortelice International Co., Taiwan) monitoring system (Feng et al., 2018). The observed vertical HONO concentrations from the study of Meng et al. (2020) were measured in December 2016. The OH measurements in January 2016 were from the study of Tan et al. (2018).
3.1 Comparison of model prediction with observed HONO
Observed HONO concentrations vary with time, ranging between 0.04 and 8 ppb, and contain 11 episodes in which the daily peak concentration exceeds 3.3 ppb (Fig. 1a). The high HONO concentration occurs during low wind speeds (Fig. S2). The average HONO concentration during the period is comparable to the reported values for other cities (Table S1). Predicted HONO concentrations obtained with the ORI case are substantially lower than the observed data. In contrast, predicted HONO concentrations obtained with the REV case are substantially higher than those obtained with the ORI case and generally similar to the observed data at night. The ORI case misses the peak values for all episodes, whereas the REV case captures peak values for most episodes. The observed average concentration during the measurement period is 2.5 ppb; the ORI case only predicts an average concentration of 0.1 ppb, whereas the REV case predicts an average concentration of 2.3 ppb. The NMB of HONO is reduced from −96.5 % with the ORI case to −4.8 % with the REV case.
Consistent with observations at other cities (Bernard et al., 2015; Fu et al., 2019; Platt et al., 1980), the diurnal variation of observed HONO concentrations in Beijing also reveals higher nighttime concentrations than daytime values (Fig. 1b). The predictions with ORI case are an order of magnitude lower than the observed diurnal concentrations. The diurnal variation with the REV case shows a remarkable enhancement of nighttime HONO concentrations to levels similar to the observed concentrations. It also increases daytime concentrations; however, predicted values are substantially lower than the observed data, which suggests that additional processes (Oswald et al., 2013; Romer et al., 2018; Xing et al., 2017) are needed to close the gap between observed and predicted daytime HONO concentrations. Night-time and daytime heterogeneous reaction and other updated reactions contribute to the improvement of HONO diurnal pattern. More detailed analysis about this great enhancement is included in Sect. 3.2. The diurnal pattern of the predicted HONO concentrations with the REV agrees better with the observed diurnal pattern.
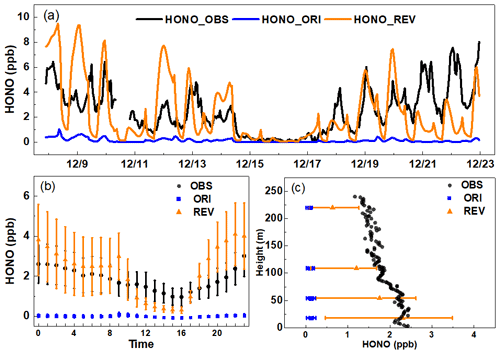
Figure 1A comparison of simulated and observed HONO concentrations in Beijing: (a) time series, (b) diurnal variation, and (c) vertical comparison. Error bars represent 5 %–95 % values of all HONO concentrations.
We compare predicted vertical distribution with observed vertical HONO concentrations (39.97∘ N, 116.38∘ E) from the study of Meng et al. (2020) (Fig. 1c). The measured concentration is the highest at the surface (2.3 ppb), and concentrations decrease with increasing altitude to a value of ∼ 1.2 ppb at ∼ 200 m, which supports the dominant role of the surface HONO production. Predicted HONO levels with ORI case are too small, whereas predictions with the REV agree better with observed data not only at the surface, but also aloft, which provides validity of the simulation results. Consistent with previous HONO vertical concentrations and flux measurements (Vandenboer et al., 2013; D. D. Li et al., 2018), HONO concentration at the surface layer is highest. Model-simulated HONO concentrations (Fig. 1c) show a decreasing trend with height similar to the trend in observation data reported by Meng et al. (2020). Model HONO concentrations at upper layers (above 50 m in Fig. 1c) are slightly underestimated. Model HONO concentrations in these layers are produced mainly by the heterogeneous reaction of NO2 on aerosol surfaces and the reaction of NO + OH. Aerosol indirect effects can reduce the photolysis rate coefficient of HONO (Xing et al., 2017). Decreasing photolysis can improve HONO concentrations in the upper layers in polluted air.
The HONO NO2 ratio is used as an indicator to estimate the efficiency of heterogeneous NO2–HONO conversion (Kleffmann et al., 2006; Li et al., 2012). The observed HONO NO2 ratios ranging between 0.003 and 0.15 are much higher than reported values in the vehicle exhausts (0.001–0.008), which suggests that HONO formation is governed mainly by the secondary production (Kirchstetter et al., 1996; Kurtenbach et al., 2001). The diurnal variations of observed and predicted HONO NO2 ratios are shown in Fig. S3. The predicted HONO NO2 ratios increase substantially with REV compared with the ORI case. The average ratio of HONO NO2 increases from 0.0027 with ORI to 0.053 with REV, which is in agreement with the observed value of 0.055. The NMB of hourly average simulated HONO NO2 ratios at nighttime decreases from −94.4 % with ORI to −34.2 % with REV. The model results suggest that NO2 heterogeneous conversion is the most important reaction for simulating atmospheric HONO concentrations.
According to our detailed literature review in the Methodology section, uncertainties of HONO prediction might be largely associated with four key parameters and inputs, including the uptake coefficient of NO2 at the ground surface, the aerosol nitrate photolysis rate coefficient, the daytime photolysis rate coefficient, and the baseline NOx emissions. Sensitivity analysis was conducted to examine the influences from those parameters, suggesting that HONO concentration could be doubled with different parameters (see the Supplement). In addition, some sources including the photolysis of deposited HNO3, soil emission, and traffic emission could also affect predicted HONO concentration, while the importance of these sources is difficult to quantify. Future studies in improving the accuracy of these parameters are important to reduce the uncertainties of HONO prediction.
3.2 Relative contribution of different HONO reactions
To gain insights into HONO reactions, production rates of different reactions are calculated, and the diurnal variation of the production rates is presented in Fig. S7. The production rates from the heterogeneous reaction on ground surfaces (denoted HONOfrNO2G) are higher during the day than those at night because of the higher rate constant. During nighttime (18:00–05:00), it dominates the HONO production with an average production rate of 1.4 ppb h−1. Similar to HONOfrNO2G, the production rates from the heterogeneous reaction on aerosol surfaces (denoted HONOfrNO2A) are also higher during daytime compared with those at nighttime. It contributes an average production rate of 0.2 ppb h−1 during nighttime. The contribution of other reactions to nighttime HONO production is relatively small (<0.03 ppb h−1). During daytime (6:00–17:00), HONOfrNO2G also dominates the production with an average contribution of 2.05 ppb h−1. HONOfrNO2A is the second most important contributor during daytime, with an average production rate of 0.54 ppb h−1. The photolysis of NO is the third contributor, with an average production of 0.04 ppb h−1. Gas-phase reactions collectively contribute an average production rate of ∼ 0.41 ppb h−1. The NO + OH reaction is the most important gas-phase reaction, producing HONO at an average rate of 0.37 ppb h−1. The average daytime production rates of the acid displacement reactions of HNO3 and HCl are 0.25 and 0.03 ppb h−1, respectively. The contribution of the reaction on elemental carbon (EC) is even smaller (<0.01 ppb h−1). Daytime production from the heterogeneous reaction on ground and aerosol surfaces is greater than the combined production from all other reactions. Although updated daytime reaction rates are higher than at nighttime, accelerated photochemical loss slows down the HONO increase during daytime.
The relative contribution of the chemistry updates to HONO formation (REV) is shown in Fig. 2. HONOfrNO2G is the most important reaction, contributing ∼ 86.2 % of the nighttime HONO production. HONOfrNO2A is the second largest contributor, representing ∼ 12.3 % of the nighttime HONO production. During daytime, HONOfrNO2G contributes ∼ 64.7 % of the HONO production, whereas HONOfrNO2A is the second largest contributor, representing 12.6 % of the HONO production. The daytime HONO production rate from HONOfrNO2A is higher than that at nighttime due to the higher rate constant. Consequently, the relative importance of daytime heterogeneous reaction on aerosol surfaces increases, whereas the relative importance of daytime heterogeneous reaction on ground surfaces decreases. The acid displacement reaction of HNO3 contributes 11 % to daytime HONO formation, and its contribution peaks at 17:00. The average contributions of gas-phase reactions, photolysis of NO, and acid displacement reactions to daytime HONO production are 9.4 %, 1.0 %, and 1.3 %, respectively. Note that the reaction of OH+NO becomes important in the morning (09:00 to 10:00) during which it contributes 17.4 % of the total HONO production. Averaged over the day and night, HONOfrNO2G is the most significant reaction, contributing 75.6 % of the HONO production. HONOfrNO2A is the second largest contributor, representing 12.3 % of the HONO production. The gas-phase reactions and the acid displacement reaction of HNO3 are the third most important contributor, each accounting for 5.6 % of HONO production. Although HONOfrNO2G had a relatively lower uptake coefficient than the aerosol surface reaction, the reaction rate was large because of the greater ground surface area density (0.047 m2 m−3) compared with the aerosol surface area density (0.0014 m2 m−3).
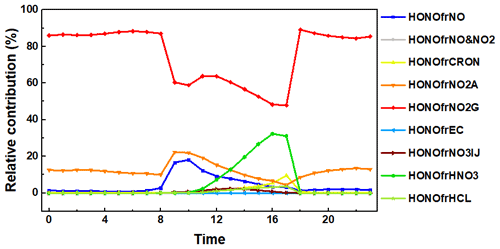
Figure 2Relative contribution of different HONO reactions to near-ground-level HONO concentration in Beijing in December. The production from the NO + OH reaction is denoted as HONOfrNO, the production from the NO + NO2 + H2O reaction is denoted as HONOfrNO&NO2, the production from nitro-cresol is denoted as HONOfrCRON, the production from the heterogeneous reaction on ground surfaces is denoted as HONOfrNO2G, the production from the heterogeneous reaction on aerosol surfaces is denoted as HONOfrNO2A, the production from the reaction of EC is denoted as HONOfrEC, the production from the photolysis of NO is denoted as HONOfrNO3IJ, the production from the acid displacement reaction of HNO3 is denoted as HONOfrHNO3, and the production from acid displacement reaction of HCl is denoted as HONOfrHCL.
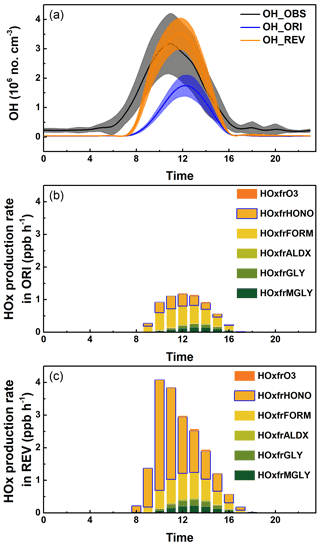
Figure 3(a) A comparison of simulated and observed diurnal variation of OH. Shading in Fig. 3a indicates the range of observation. (b) HOx formation rates from different photolytic reactions with the ORI case and (c) HOx formation rates from different photolytic reactions with the REV case. The production of HOx from the O3 photolysis is denoted as HOxfrO3, the production of HOx from the HONO photolysis is denoted as HOxfrHONO, the production of HOx from the formaldehyde photolysis is denoted as HOxfrFORM, the production of HOx from the higher aldehyde photolysis is denoted as HOxfrALDX, the production of HOx from the glyoxal photolysis is denoted as HOxfrGLY, and the production of HOx from the methyl glyoxal photolysis is denoted as HOxfrMGLY.
3.3 Impacts of HONO chemistry on hydroxyl radical concentration
Enhanced HONO production increases model OH concentration via photolysis. We compare predicted OH concentrations with observed winter data (40.41∘ N, 116.68∘ E) reported by Tan et al. (2018) in Fig. 3a. Observed concentrations are low (∼ 2–3 × 105 no. cm3) at night and rapidly increase in the morning, reaching a peak value of ∼ 3 × 106 no. cm3 at around 11:00, then slowly decrease to the low nightly values. The ORI case underpredicts the observed peak value by a factor of ∼ 2, and the model peak time occurs 1 to 1.5 h after the observed peak time, which is consistent with a previous study in which additional HONO reactions increased OH levels by a factor of >2 (Xue et al., 2020). In addition, the morning enhancement rate with ORI is very low compared with the observed rate. In contrast, the REV case reproduces the observed peak and improves the timing of the peak. The morning enhancement rate also substantially increases and closely tracks the observed enhancement rate. The daily average concentration of OH with REV increases by ∼ 98 % compared with that obtained with ORI. Thus, the REV case successfully captures the morning enhancement rate and the peak and improves the timing of the peak in observed OH data in Beijing. Overall, it captures the observed OH concentration in Beijing much better than the model with the original chemistry. To examine the vertical extent of the impact on OH, predicted OH concentrations with altitude are shown in Fig. S8 (40.0∘ N, 116.3∘ E). Predicted OH concentration with ORI is the lowest at the surface and increases with altitude, primarily because of higher O3 aloft. The REV case increases OH concentration, not only due to the surface HONO, but also aloft. However, the impact on OH decreases with altitude as the HONO production decreases with altitude.
Various photolytic reactions, including the photolysis of O3, HONO, formaldehyde, higher aldehyde, glyoxal, and methyl glyoxal that produces HOx (OH + HO2), are in the model. To understand the relative impacts of these HONO reactions on HOx production, we compare the diurnal production rates of HOx from these reactions in Fig. 3b and c. In the ORI case (Fig. 3b), the production of HOx is relatively small and dominated by the photolysis of HONO and formaldehyde. The photolysis of HONO and formaldehyde starts producing HOx at 09:00, which initiates daytime atmospheric chemistry. From late morning, the production of HOx from glyoxal and methyl glyoxal also contributes to the continuation of daytime atmospheric chemistry. In our simulation, glyoxal and methyl glyoxal originate from the oxidation of aromatics in the atmosphere because isoprene concentration in Beijing is low in winter. Averaged over the entire day, the photolysis of formaldehyde is the largest contributor (0.14 ppb h−1), and the photolysis of HONO is the second largest contributor (0.08 ppb h−1) to the total HOx production rate. The production from O3 and higher aldehyde photolysis is small as their concentrations are low.
In contrast, the HOx production rates in the REV case are much higher than those in the ORI case because of the enhanced formation from HONO (Fig. 3c). The photolysis of HONO produces HOx in the morning, which then kick-starts daytime atmospheric chemistry at 08:00 (1 h earlier than in the ORI case) and continues to play an important role during the entire day. From late morning, the production of HOx from formaldehyde, glyoxal, methyl glyoxal, and higher aldehyde also contributes to the continuation of daytime atmospheric chemistry. The production of HOx from glyoxal, methyl glyoxal, and higher aldehydes plays a larger role compared with that in the ORI case because of higher concentrations produced by the enhanced oxidation of aromatics by higher OH. The photolysis of HONO is the largest contributor (0.5 ppb h−1) to the overall HOx production rate averaged over the entire day, while the photolysis of formaldehyde is the second largest contributor (0.18 ppb h−1). Thus, HONO plays a crucial role in producing OH in the morning, and without updated reactions, the start of daytime atmospheric chemistry is delayed, and the reaction rates are slower; it also plays an important role in atmospheric chemistry throughout the day. Many other photolytic reactions also produce HOx in the model; however, the productions from the other pathways are small and do not affect our calculation; hence, they are not shown in the figure. The daytime underestimation of HONO in Fig.1 may potentially lead to the underestimation of OH concentration; however, the aerosol indirect effect may lower the OH concentration by reducing the rates of HOx formation. Therefore, more accurate HONO simulation needs to consider more complex and significant atmospheric chemical processes.
HONO can affect the daily OH budget greatly (Harris et al., 1982; L. Li et al., 2018b; Lu et al., 2019; Xue et al., 2020; Platt et al., 1980). Our simulations with the additional HONO reactions enhance OH, which in turn increases HO2 by the fast conversion between OH and HO2 radicals (Heard and Pilling, 2003; Lu et al., 2012). The reaction rate of the HO2+ NO reaction increases from 1.8 ppb h−1 in ORI to 3.6 ppb h−1 in REV. This indicates that the HONO chemistry also indirectly promotes the formation of OH by increasing the activity of HO2. This highlights the promoting role of HONO in gas-phase radicals.
Increased OH concentration oxidizes additional volatile organic compounds (VOCs), lowers the concentrations of precursor species, and increases the concentrations of secondary species (Table S3). Enhanced oxidation of VOCs, sulfur dioxide, and NO2 leads to secondary pollutants, including SO, NO, NH, and SOA, which are discussed in the next section.
3.4 Impacts of HONO chemistry on the formation of secondary particles
Daily averaged model-predicted SO, NO, and NH concentrations are compared with observed data in Beijing in Fig. 4. The ORI case captures the observed trend but generally underestimates the observed SO concentrations, whereas the REV case enhances SO concentrations and closes the gap between model predictions and observation data. Over the entire simulation period, the average concentration of SO is increased from 13.3 to 15.8 µg m−3 (19 %). CMAQv5.3 includes six chemical pathways for the conversion of SO2 into SO (Sarwar et al., 2011). These are (1) the gas-phase oxidation of SO2 by OH, aqueous-phase oxidation of S(IV) (the sum of SO2 ⋅ H2O [hydrated SO2], HSO [bisulfite ion] and SO [sulfite ion]) by (2) H2O2 (hydrogen peroxide), (3) O3, (4) PAA (peroxyacetic acid), (5) MHP (methylhydroperoxide), and (6) oxygen catalyzed by iron (Fe[III]) and manganese (Mn[II]). We utilized the sulfate tracking model to examine SO production from these chemical pathways over Beijing. The SO production from the gas-phase oxidation of SO2 by OH in the REV is ∼ 79 % greater than that of the ORI case because of the higher OH concentration from HONO photolysis. SO production from the aqueous-phase oxidation of S(IV) by H2O2 in the ORI is relatively small because the predicted H2O2 concentration is also small in winter. However, the REV case enhances H2O2 concentration, which consequently also increases the SO production from this pathway. The other chemical pathways produce similar concentrations in both models, except the oxygen catalyzed by the Fe[III] and Mn[II] pathway, which produces slightly lower SO production in the REV case because of the competition among different chemical pathways and greater oxidation by the OH initiated pathway.
Table 3Predicted SO concentration in Beijing from different chemical pathways in CMAQv5.3.
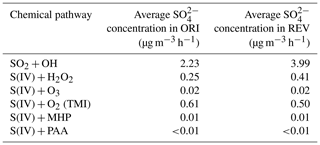
TMI: S(IV) oxidation by oxygen catalyzed by Fe[III] and Mn[II].
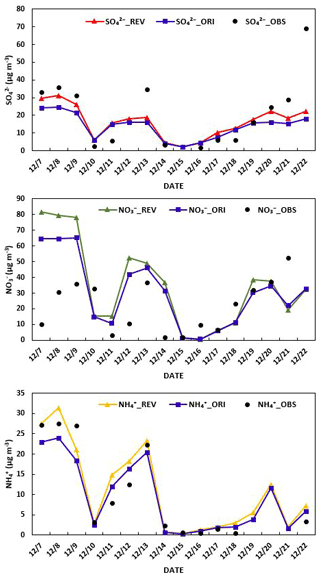
Figure 4A comparison of simulated and observed daily averaged sulfate, nitrate, and ammonium concentration in Beijing.
Additional SO production is needed in the model to close the gap between the model prediction and observed data. Several investigators have proposed other pathways that can generate additional SO production. For example, Gen et al. (2019) conducted laboratory experiments and reported that the photolysis of NO can generate N(III) (HONO ) in aerosol liquid water, which oxidizes S(IV) into SO. Zheng et al. (2020) recently incorporated such a pathway and reported that it can enhance SO production and can explain 15 % to 65 % of the gap between model predictions and observed SO concentrations in China. Shao et al. (2019) implemented several additional heterogeneous SO formation pathways for oxidation of S(IV) in aerosol liquid water and reported that the pathways can enhance SO production by 20 % in China. Wang et al. (2020b) recently reported that S(IV) can be oxidized by HONO and NO2 in cloud and fog to produce SO in China. Other investigators (Wang et al., 2016; Ye et al., 2018) have suggested additional chemical pathways for SO production in China. Additional research is needed to further understand the chemical pathways for SO production in China (Wang et al., 2020b). These pathways are not the focus of this study and, therefore, are not included in our simulations, which leads to the model underpredictions. However, our analysis reveals that the HONO chemistry and the subsequent production of OH can enhance SO production in China so should be included in air quality models.
The ORI case has mixed performance in simulating observed NO (Fig. 4). It overestimates the daily averaged observed NO concentration on some days but captures or underestimates the observed concentrations on the other. The overestimation of winter NO by CMAQ has been reported in previous studies (Yu et al., 2005; Appel et al., 2008). Several reactions contribute to the formation of HNO3 in CMAQv5.3, which then partitions into NO. The heterogeneous hydrolysis of N2O5 is the most important nighttime reaction, and the oxidation of NO2 by OH is the most important daytime reaction forming HNO3. CMAQv5.3 uses the parameterization of Davis et al. (2008) for calculating the uptake coefficient for the heterogeneous hydrolysis of N2O5. It does not include the organic-coating effect (Anttila et al., 2006; Riemer et al., 2009) that can lower the uptake coefficient. Several studies (Brown et al., 2006; McDuffie et al., 2018; Chang et al., 2016; Wang et al., 2020a) have suggested that the parameterizations used in air quality models, including box models, WRF-Chem, and CMAQv5.3, produce higher uptake coefficients than those derived from observation-based studies. These higher uptake coefficients produce high levels of HNO3 and NO in the model. A recent study also suggests that the heterogeneous uptake coefficient in China can be even lower than the values derived over the United States (Wang et al., 2020b). Our current model does not include such a lower uptake coefficient and overpredicts NO concentrations. Our IRR analysis of the ORI case results suggests that 30.3 % of NO (averaged over the entire simulation period in Beijing) is produced via nighttime heterogeneous hydrolysis of N2O5, and 69.7 % is produced via daytime oxidation of NO2 by OH. The revised chemistry further enhances predicted NO, primarily via the enhanced daytime oxidation of NO2. Overall, nighttime heterogeneous hydrolysis of N2O5 contributes 27.6 %, and daytime oxidation of NO2 contributes 72.4 % in the REV case. Consequently, predicted NO concentrations with the revised chemistry further are overestimated on most days.
Because of the increased production of SO and fNO, the average concentration of NH also increased from 11.1 µg m−3 in ORI to 13.1 µg m−3 in REV (Fig. 4). NH formation is promoted by enhancing the neutralization of sulfuric acid and HNO3 by ammonia. The dissolution of the precursor and the ion balance is the main factor for the growth of NH in CMAQv5.3. The overestimation of NO leads to the overestimation of NH (Liu et al., 2020).
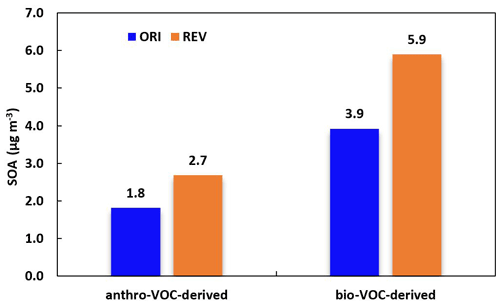
Figure 5Predicted monthly average SOA concentration from anthropogenic VOCs (anthro-VOC-derived) and biogenic VOCs (bio-VOC-derived) in Beijing. Numbers in this figure only include SOA from representative anthropogenic or biogenic VOCs.
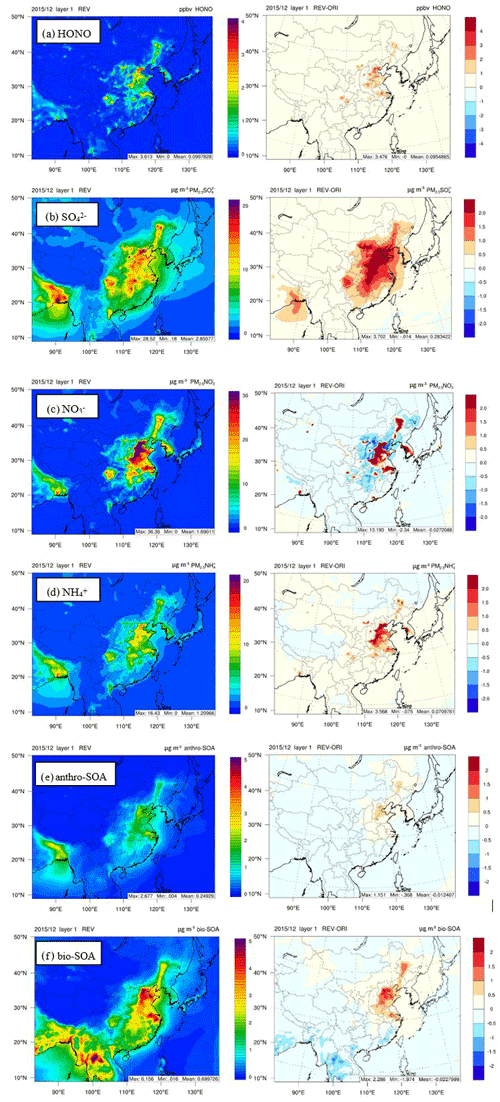
Figure 6Spatial distributions of monthly averaged (a) HONO, (b) sulfate, (c) nitrate, (d) ammonium, (e) anthro-VOC-derived SOA, and (f) and bio-VOC-derived SOA concentrations simulated with REV and the differences (REV−ORI) between the two simulations in December 2015.
CMAQv5.3 has a comprehensive treatment of organic aerosols (Murphy et al., 2017; Pye et al., 2017; Xu et al., 2018), including SOA production from anthropogenic VOCs (anthro-VOC-derived) and biogenic VOCs (bio-VOC-derived); Fig. 5 displays the anthro-VOC-derived and bio-VOC-derived SOA in Beijing. The REV case enhances the concentration of anthro-VOC-derived SOA by 0.9 µg m−3 (50 %) and bio-VOC-derived SOA by 2.0 µg m−3 (51 %). Enhanced OH from additional HONO enhances the oxidation of VOCs (Table S3) and promotes the SOA formation, which is also reported in previous studies. For example, Xing et al. (2019) used the WRF-Chem model to examine the impact of HONO chemistry updates on SOA formation over the BTH region in winter and reported that the heterogeneous HONO production can increase the regional average SOA concentration by 46 %. Zhang et al. (2019b) implemented six additional HONO reactions (traffic, soil, biomass burning and indoor emissions, and heterogeneous reactions on aerosol and ground surfaces) in the WRF-Chem model and reported that it successfully reproduced the observed HONO concentrations in Wangdu. They suggested that the additional HONO reactions can increase 2 to 15 µg m−3 of SOA (meridional mean) in the BTH region on heavy haze days.
3.5 Spatial impacts on selected species
We examine the spatial impacts of the revised HONO chemistry on selected species (HONO, SO, NO, NH, and SOA) in Fig. 6. Predicted average HONO concentrations with ORI are low (<0.18 ppb) over the entire modeling domain. The revised chemistry increases HONO concentrations over the North China Plain (i.e., BTH, Henan, Shandong) by 0.5 to 3.0 ppb. Abundant emissions of NOx in this area result in higher NO2 concentrations, which subsequently enhance HONO concentrations, as the NO2 reaction on ground is the dominated HONO production source (Fig. 2). It also increases HONO in some other urban areas; however, the impacts in most other areas are relatively small. The ORI case predicts higher average SO, NO, and NH concentrations over the North China Plain and the northeast cites. The revised chemistry enhances the average SO by 1 to 3 µg m−3, with the maximum enhancements over the south part of the Hebei province. It increases NO by up to 1.5 µg m−3 and NH by up to 1.1 µg m−3 over the North China Plain. It also slightly decreases NO over the North China Plain. The revised HONO chemistry decreases NO2 concentration while increasing OH concentration. Thus, daytime production of HNO3 from the NO2+ OH pathway depends on the relative magnitude of the changes of the reaction rate and tends to increase the production in high-NOx areas while decreasing it in low-NOx areas. HNO3 partitions into NO; thus, changes in HNO3 production lead to changes in NO concentration. The ORI case predicts the highest anthropogenic SOA (anthro-SOA) and biogenic SOA (bio-SOA) concentrations over northeast China and the North China Plain. The revised model increases anthro-SOA by 0.37 to 1.2 µg m−3 over this area and changes bio-SOA over the North China Plain and the northeast cities by −2.0 to 2.3 µg m−3. Isoprene emissions in some southern cities are relatively higher than in cities in the North China Plain in the model. Glyoxal and methylglyoxal generated from isoprene are oxidized by increased OH from the HONO chemistry. SOA derived from biogenic VOCs, therefore, is reduced in some areas in Guangdong.
The existing HONO chemistry in CMAQv5.3 cannot re-produce the observed winter HONO concentrations in Beijing. Thus, we revised the HONO chemistry in CMAQv5.3 by implementing several heterogeneous HONO formation pathways. Model predictions with the revised chemistry generally agree with observed HONO concentrations, although the model cannot predict the higher observed daytime concentrations. The heterogeneous production on the ground accounts for nearly 75 % of the total HONO production. Enhanced HONO increases daytime OH concentrations, which also agrees well with observed data in Beijing. Predicted OH concentrations with the existing HONO chemistry are lower than observed data almost by a factor of 2. The morning OH enhancement rate is lower than the observed rate, and the timing of the peak is delayed. The revised HONO chemistry improves the morning OH enhancement rate and reproduces the daily peak and the timing of the daily peak. Enhanced OH increases the oxidation rates of SO2, NO2, and VOCs in the atmosphere and produces additional secondary pollutants. The revised HONO chemistry moderately enhances SO concentration in this study. The impact of HONO chemistry on SO concentration is likely to be greater that shown in this article. For example, HONO chemistry enhances NO, which, in turn, can produce additional SO via the photolysis of NO (Zheng et al., 2020). The oxidation of S(IV) by HONO in cloud and fog also can produce additional SO (Wang et al., 2020). Such pathways are not the focus of this study and are not included in the current model. A recent study (Chen et al., 2019) suggests that HONO also can form on snow-covered ground, which can potentially affect wintertime air quality. Thus, a future study incorporating such chemical reactions to comprehensively examine the impact of HONO chemistry on air quality in different seasons and geographical areas is envisioned.
The standard CMAQ (https://zenodo.org/record/3585898, USEPA, 2019) model is available at https://github.com/USEPA/CMAQ, last access: 16 October 2021. The code changes made in this study and the observational data used in this study are available from the corresponding author upon request (honghe@rcees.ac.cn, xingjia@tsinghua.edu.cn, sarwar.golam@epa.gov).
The supplement related to this article is available online at: https://doi.org/10.5194/acp-21-15809-2021-supplement.
SZ initiated the study, carried out analysis, and wrote the initial draft. All authors helped interpret the data, provided feedback, and commented on the manuscript.
The authors declare that they have no conflict of interest.
The views expressed in this paper are those of the authors and do not
necessarily represent the views or policies of the U.S. EPA.
Publisher's note: Copernicus Publications remains neutral with regard to jurisdictional claims in published maps and institutional affiliations.
This work was completed on the “Explorer 100” cluster system of Tsinghua National Laboratory for Information Science and Technology.
This research has been supported by the National Natural Science Foundation of China (grant nos. 41907190, 51861135102, and 41877304) and the Youth Innovation Promotion Association of the Chinese Academy of Sciences (grant no. 2018060). This work was also financially and technically supported by Toyota Motor Corporation and Toyota Central Research and Development Laboratories Inc.
This paper was edited by Lea Hildebrandt Ruiz and reviewed by William Stockwell and two anonymous referees.
An, J., Li, Y., Chen, Y., Li, J., Qu, Y., and Tang, Y.: Enhancements of major aerosol components due to additional HONO sources in the North China Plain and implications for visibility and haze, Adv. Atmos. Sci., 30, 57–66, https://doi.org/10.1007/s00376-012-2016-9, 2012.
Anttila, T., Kiendler-Scharr, A., Tillmann, R., and Mentel, T. F.: On the Reactive Uptake of Gaseous Compounds by Organic-Coated Aqueous Aerosols: Theoretical Analysis and Application to the Heterogeneous Hydrolysis of N2O5, J. Phys. Chem. A, 110, 10435–10443, https://doi.org/10.1021/jp062403c, 2006.
Appel, W., Bhave, P., Gilliland, A., Sarwar, G., and Roselle, S.: Evaluation of the community multiscale air quality (CMAQ) model version 4.5: Sensitivities impacting model performance, Part II – particulate matter, Atmos. Environ., 42, 6057–6066, https://doi.org/10.1016/j.atmosenv.2008.03.036, 2008.
Bao, F. X., Li, M., Zhang, Y., Chen, C. C., and Zhao, J. C.: Photochemical Aging of Beijing Urban PM2.5: HONO Production, Environ. Sci. Technol., 52, 6309–6316, https://doi.org/10.1021/acs.est.8b00538, 2018.
Bernard, F., Cazaunau, M., Grosselin, B., Zhou, B., Zheng, J., Liang, P., Zhang, Y., Ye, X., Daële, V., Mu, Y., Zhang, R., Chen, J.-M., and Mellouki, A.: Measurements of nitrous acid (HONO) in urban area of Shanghai, China, Environ. Sci. Pollut. Res., 23, 5818–5829, https://doi.org/10.1007/s11356-015-5797-4, 2015.
Brown, S. S., Ryerson, T. B., Wollny, A. G., Brock, C. A., Peltier, R., Sullivan, A. P., Weber, R. J., Dubé, W. P., Trainer, M., Meagher, J. F., Fehsenfeld, F. C., and Ravishankara, A. R.: Variability in Nocturnal Nitrogen Oxide Processing and Its Role in Regional Air Quality, Science, 311, 67–70, https://doi.org/10.1126/science.1120120, 2006.
Byun, D. and Schere, K. L.: Review of the governing equations, computational algorithms, and other components of the Models-3 Community Multiscale Air Quality (CMAQ) modeling system, Appl. Mechan. Rev., 59, 51–77, 2006.
Chan, W. H., Nordstrom, R. J., Calvert, J. G., and Shaw, J. H.: Kinetic study of nitrous acid formation and decay reactions in gaseous mixtures of nitrous acid, nitrogen oxide (NO), nitrogen oxide (NO2), water, and nitrogen, Environ. Sci. Technol., 10, 674–682, https://doi.org/10.1021/es60118a007, 1976a.
Chan, W. H., Nordstrom, R. J., Galvert, J. G., and Shaw, J. H.: An IRFTS spectroscopic study of the kinetics and the mechanism of the reactions in the gaseous system, HONO, NO, NO2, H2O, Chem. Phys. Lett., 37, 441–446, https://doi.org/10.1016/0009-2614(76)85010-5, 1976b.
Chang, W. L., Brown, S. S., Stutz, J., Middlebrook, A. M., Bahreini, R., Wagner, N. L., Dubé, W. P., Pollack, I. B., Ryerson, T. B., and Riemer, N.: Evaluating N2O5 heterogeneous hydrolysis parameterizations for CalNex 2010, J. Geophys. Res.-Atmos., 121, 5051–5070, https://doi.org/10.1002/2015JD024737, 2016.
Chen, Q., Edebeli, J., McNamara, S. M., Kulju, K. D., May, N. W., Bertman, S. B., Thanekar, S., Fuentes, J. D., and Pratt, K. A.: HONO, Particulate Nitrite, and Snow Nitrite at a Midlatitude Urban Site during Wintertime, ACS Earth Space Chem., 3, 811–822, https://doi.org/10.1021/acsearthspacechem.9b00023, 2019.
Cui, L. L., Li, R., Zhang, Y. C., Meng, Y., Fu, H. B., and Chen, J. M.: An observational study of nitrous acid (HONO) in Shanghai, China: The aerosol impact on HONO formation during the haze episodes, Sci. Total Environ., 630, 1057–1070, https://doi.org/10.1016/j.scitotenv.2018.02.063, 2018.
Czader, B. H., Rappenglück, B., Percell, P., Byun, D. W., Ngan, F., and Kim, S.: Modeling nitrous acid and its impact on ozone and hydroxyl radical during the Texas Air Quality Study 2006, Atmos. Chem. Phys., 12, 6939–6951, https://doi.org/10.5194/acp-12-6939-2012, 2012.
Czader, B. H., Li, X., and Rappenglueck, B.: CMAQ modeling and analysis of radicals, radical precursors, and chemical transformations, J. Geophys. Res.-Atmos., 118, 11376–311387, https://doi.org/10.1002/jgrd.50807, 2013.
Davis, J. M., Bhave, P. V., and Foley, K. M.: Parameterization of N2O5 reaction probabilities on the surface of particles containing ammonium, sulfate, and nitrate, Atmos. Chem. Phys., 8, 5295–5311, https://doi.org/10.5194/acp-8-5295-2008, 2008.
Emery, C., Tai, E., and Yarwood, G.: Enhanced Meteorological Modeling and Performance Evaluation for Two Texas Ozone Episodes, Final Report, Final Report, Submitted to Texas Natural Resources Conservation Commission, Prepared by ENVIRON, International Corp., Novato, Work Assignment No. 31984-11, 2001.
Feng, T., Bei, N. F., Zhao, S. Y., Wu, J. R., Li, X., Zhang, T., Cao, J. J., Zhou, W. J., and Li, G. H.: Wintertime nitrate formation during haze days in the Guanzhong basin, China: A case study, Environ. Pollut., 243, 1057–1067, https://doi.org/10.1016/j.envpol.2018.09.069, 2018.
Finlayson-Pitts, B. J.: Chemistry of the upper and lower atmosphere theory, experiments and applications, San Diego, California, Academic Press, San Diego, California, 2000.
Fu, X., Wang, T., Zhang, L., Li, Q., Wang, Z., Xia, M., Yun, H., Wang, W., Yu, C., Yue, D., Zhou, Y., Zheng, J., and Han, R.: The significant contribution of HONO to secondary pollutants during a severe winter pollution event in southern China, Atmos. Chem. Phys., 19, 1–14, https://doi.org/10.5194/acp-19-1-2019, 2019.
Gen, M., Zhang, R., Huang, D. D., Li, Y., and Chan, C. K.: Heterogeneous SO2 Oxidation in Sulfate Formation by Photolysis of Particulate Nitrate, Environ. Sci. Technol. Lett., 6, 86–91, https://doi.org/10.1021/acs.estlett.8b00681, 2019.
Guo, S., Hu, M., Zamora, M. L., Peng, J., Shang, D., Zheng, J., Du, Z., Wu, Z., Shao, M., Zeng, L., Molina, M. J., and Zhang, R.: Elucidating severe urban haze formation in China, P. Natl. Acad. Sci. USA, 111, 17373–17378, https://doi.org/10.1073/pnas.1419604111, 2014.
Harris, G. W., Carter, W. P. L., Winer, A. M., Pitts, J. N., Platt, U., and Perner, D.: Observations of nitrous acid in the Los Angeles atmosphere and implications for predictions of ozone-precursor relationships, Environ. Sci. Technol., 16, 414–419, https://doi.org/10.1021/es00101a009, 1982.
He, H., Wang, Y., Ma, Q., Ma, J., Chu, B., Ji, D., Tang, G., Liu, C., Zhang, H., and Hao, J.: Mineral dust and NOx promote the conversion of SO2 to sulfate in heavy pollution days, Sci. Rep., 4, 4172, https://doi.org/10.1038/srep04172, 2014.
Heard, D. E. and Pilling, M. J.: Measurement of OH and HO2 in the Troposphere, Chem. Rev., 103, 5163–5198, https://doi.org/10.1021/cr020522s, 2003.
Huang, L., Zhao, Y., Li, H., and Chen, Z.: Kinetics of Heterogeneous Reaction of Sulfur Dioxide on Authentic Mineral Dust: Effects of Relative Humidity and Hydrogen Peroxide, Environ. Sci. Technol., 49, 10797–10805, https://doi.org/10.1021/acs.est.5b03930, 2015.
Huang, R.-J., Zhang, Y., Bozzetti, C., Ho, K.-F., Cao, J.-J., Han, Y., Daellenbach, K. R., Slowik, J. G., Platt, S. M., and Canonaco, F.: High secondary aerosol contribution to particulate pollution during haze events in China, Nature, 514, 218–222, 2014.
Jaeglé, L., Shah, V., Thornton, J. A., Lopez-Hilfiker, F. D., Lee, B. H., McDuffie, E. E., Fibiger, D., Brown, S. S., Veres, P., Sparks, T. L., Ebben, C. J., Wooldridge, P. J., Kenagy, H. S., Cohen, R. C., Weinheimer, A. J., Campos, T. L., Montzka, D. D., Digangi, J. P., Wolfe, G. M., Hanisco, T., Schroder, J. C., Campuzano-Jost, P., Day, D. A., Jimenez, J. L., Sullivan, A. P., Guo, H., and Weber, R. J.: Nitrogen Oxides Emissions, Chemistry, Deposition, and Export Over the Northeast United States During the WINTER Aircraft Campaign, J. Geophys. Res.-Atmos., 123, 12368–312393, https://doi.org/10.1029/2018JD029133, 2018.
Kaiser, E. W. and Wu, C. H.: A kinetic study of the gas phase formation and decomposition reactions of nitrous acid, J. Phys. Chem., 81, 1701–1706, https://doi.org/10.1021/j100533a001, 1977.
Karamchandani, P., Emery, C., Yarwood, G., Lefer, B., Stutz, J., Couzo, E., and Vizuete, W.: Implementation and refinement of a surface model for heterogeneous HONO formation in a 3-D chemical transport model, Atmos. Environ., 112, 356–368, https://doi.org/10.1016/j.atmosenv.2015.01.046, 2015.
Kirchstetter, T. W., Harley, R. A., and Littlejohn, D.: Measurement of nitrous acid in motor vehicle exhaust, Environ. Sci. Technol., 30, 2843–2849, 1996.
Kleffmann, J., Gavriloaiei, T., Hofzumahaus, A., Holland, F., Koppmann, R., Rupp, L., Schlosser, E., Siese, M., and Wahner, A.: Daytime formation of nitrous acid: A major source of OH radicals in a forest, Geophys. Res. Lett., 32, L05818, https://doi.org/10.1029/2005gl022524, 2005.
Kleffmann, J., Lörzer, J., Wiesen, P., Kern, C., Trick, S., Volkamer, R., Rodenas, M., and Wirtz, K.: Intercomparison of the DOAS and LOPAP techniques for the detection of nitrous acid (HONO), Atmos. Environ., 40, 3640–3652, 2006.
Kulmala, M.: Build a global Earth observatory, Nature, 553, 21–23, https://doi.org/10.1038/d41586-017-08967-y, 2018.
Kurtenbach, R., Becker, K., Gomes, J., Kleffmann, J., Lörzer, J., Spittler, M., Wiesen, P., Ackermann, R., Geyer, A., and Platt, U.: Investigations of emissions and heterogeneous formation of HONO in a road traffic tunnel, Atmos. Environ., 35, 3385–3394, 2001.
Lelieveld, J., Evans, J. S., Fnais, M., Giannadaki, D., and Pozzer, A.: The contribution of outdoor air pollution sources to premature mortality on a global scale, Nature, 525, 367–371, https://doi.org/10.1038/nature15371, 2015.
Li, D. D., Xue, L. K., Wen, L., Wang, X. F., Chen, T. S., Mellouki, A., Chen, J. M., and Wang, W. X.: Characteristics and sources of nitrous acid in an urban atmosphere of northern China: Results from 1-yr continuous observations, Atmos. Environ., 182, 296–306, https://doi.org/10.1016/j.atmosenv.2018.03.033, 2018.
Li, G., Lei, W., Zavala, M., Volkamer, R., Dusanter, S., Stevens, P., and Molina, L. T.: Impacts of HONO sources on the photochemistry in Mexico City during the MCMA-2006/MILAGO Campaign, Atmos. Chem. Phys., 10, 6551–6567, https://doi.org/10.5194/acp-10-6551-2010, 2010.
Li, G., Bei, N., Cao, J., Huang, R., Wu, J., Feng, T., Wang, Y., Liu, S., Zhang, Q., Tie, X., and Molina, L. T.: A possible pathway for rapid growth of sulfate during haze days in China, Atmos. Chem. Phys., 17, 3301–3316, https://doi.org/10.5194/acp-17-3301-2017, 2017.
Li, L., Duan, Z., Li, H., Zhu, C., Henkelman, G., Francisco, J. S., and Zeng, X. C.: Formation of HONO from the NH3-promoted hydrolysis of NO2 dimers in the atmosphere, P. Natl. Acad. Sci. USA, 115, 7236–7241, https://doi.org/10.1073/pnas.1807719115, 2018a.
Li, L. J., Hoffmann, M. R., and Colussi, A. J.: Role of Nitrogen Dioxide in the Production of Sulfate during Chinese Haze-Aerosol Episodes, Environ. Sci. Technol., 52, 2686–2693, https://doi.org/10.1021/acs.est.7b05222, 2018b.
Li, M., Su, H., Li, G., Ma, N., Pöschl, U., and Cheng, Y.: Relative importance of gas uptake on aerosol and ground surfaces characterized by equivalent uptake coefficients, Atmos. Chem. Phys., 19, 10981–11011, https://doi.org/10.5194/acp-19-10981-2019, 2019.
Li, X., Brauers, T., Häseler, R., Bohn, B., Fuchs, H., Hofzumahaus, A., Holland, F., Lou, S., Lu, K. D., Rohrer, F., Hu, M., Zeng, L. M., Zhang, Y. H., Garland, R. M., Su, H., Nowak, A., Wiedensohler, A., Takegawa, N., Shao, M., and Wahner, A.: Exploring the atmospheric chemistry of nitrous acid (HONO) at a rural site in Southern China, Atmos. Chem. Phys., 12, 1497–1513, https://doi.org/10.5194/acp-12-1497-2012, 2012.
Liu, Y., Lu, K., Li, X., Dong, H., Tan, Z., Wang, H., Zou, Q., Wu, Y., Zeng, L., Hu, M., Min, K.-E., Kecorius, S., Wiedensohler, A., and Zhang, Y.: A Comprehensive Model Test of the HONO Sources Constrained to Field Measurements at Rural North China Plain, Environ. Sci. Technol., 53, 3517–3525, https://doi.org/10.1021/acs.est.8b06367, 2019.
Liu, Y., Zhang, Y., Lian, C., Yan, C., Feng, Z., Zheng, F., Fan, X., Chen, Y., Wang, W., Chu, B., Wang, Y., Cai, J., Du, W., Daellenbach, K. R., Kangasluoma, J., Bianchi, F., Kujansuu, J., Petäjä, T., Wang, X., Hu, B., Wang, Y., Ge, M., He, H., and Kulmala, M.: The promotion effect of nitrous acid on aerosol formation in wintertime in Beijing: the possible contribution of traffic-related emissions, Atmos. Chem. Phys., 20, 13023–13040, https://doi.org/10.5194/acp-20-13023-2020, 2020.
Liu, Z., Wang, Y., Costabile, F., Amoroso, A., Zhao, C., Huey, L. G., Stickel, R., Liao, J., and Zhu, T.: Evidence of Aerosols as a Media for Rapid Daytime HONO Production over China, Environ. Sci. Technol., 48, 14386–14391, https://doi.org/10.1021/es504163z, 2014.
Lu, K., Fuchs, H., Hofzumahaus, A., Tan, Z., Wang, H., Zhang, L., Schmitt, S. H., Rohrer, F., Bohn, B., Broch, S., Dong, H., Gkatzelis, G. I., Hohaus, T., Holland, F., Li, X., Liu, Y., Liu, Y., Ma, X., Novelli, A., Schlag, P., Shao, M., Wu, Y., Wu, Z., Zeng, L., Hu, M., Kiendler-Scharr, A., Wahner, A., and Zhang, Y.: Fast Photochemistry in Wintertime Haze: Consequences for Pollution Mitigation Strategies, Environ. Sci. Technol., 53, 10676–10684, https://doi.org/10.1021/acs.est.9b02422, 2019.
Lu, K. D., Rohrer, F., Holland, F., Fuchs, H., Bohn, B., Brauers, T., Chang, C. C., Häseler, R., Hu, M., Kita, K., Kondo, Y., Li, X., Lou, S. R., Nehr, S., Shao, M., Zeng, L. M., Wahner, A., Zhang, Y. H., and Hofzumahaus, A.: Observation and modelling of OH and HO2; concentrations in the Pearl River Delta 2006: a missing OH source in a VOC rich atmosphere, Atmos. Chem. Phys., 12, 1541–1569, https://doi.org/10.5194/acp-12-1541-2012, 2012.
Lu, X. C., Wang, Y. H., Li, J. F., Shen, L., and Fung, J. C. H.: Evidence of heterogeneous HONO formation from aerosols and the regional photochemical impact of this HONO source, Environ. Res. Lett., 13, 114002, https://doi.org/10.1088/1748-9326/aae492, 2018.
Ma, T., Furutani, H., Duan, F., Kimoto, T., Jiang, J. K., Zhang, Q., Xu, X. B., Wang, Y., Gao, J., Geng, G. N., Li, M., Song, S. J., Ma, Y. L., Che, F., Wang, J., Zhu, L. D., Huang, T., Toyoda, M., and He, K. B.: Contribution of hydroxymethanesulfonate (HMS) to severe winter haze in the North China Plain, Atmos. Chem. Phys., 20, 5887–5897, https://doi.org/10.5194/acp-20-5887-2020, 2020.
Mathur, R., Roselle, S., Pouliot, G., and Sarwar, G.: Diagnostic analysis of the three-dimensional sulfur distributions over the eastern United states using the CMAQ model and measurements from the ICARTT field experiment, in: Air Pollution Modeling and Its Application XIX, Springer, 496–504, 2008.
McDuffie, E. E., Fibiger, D. L., Dubé, W. P., Lopez-Hilfiker, F., Lee, B. H., Thornton, J. A., Shah, V., Jaeglé, L., Guo, H., Weber, R. J., Michael Reeves, J., Weinheimer, A. J., Schroder, J. C., Campuzano-Jost, P., Jimenez, J. L., Dibb, J. E., Veres, P., Ebben, C., Sparks, T. L., Wooldridge, P. J., Cohen, R. C., Hornbrook, R. S., Apel, E. C., Campos, T., Hall, S. R., Ullmann, K., and Brown, S. S.: Heterogeneous N2O5 Uptake During Winter: Aircraft Measurements During the 2015 WINTER Campaign and Critical Evaluation of Current Parameterizations, J. Geophys. Res.-Atmos., 123, 4345–4372, https://doi.org/10.1002/2018jd028336, 2018.
Meng, F., Qin, M., Tang, K., Duan, J., Fang, W., Liang, S., Ye, K., Xie, P., Sun, Y., Xie, C., Ye, C., Fu, P., Liu, J., and Liu, W.: High-resolution vertical distribution and sources of HONO and NO2; in the nocturnal boundary layer in urban Beijing, China, Atmos. Chem. Phys., 20, 5071–5092, https://doi.org/10.5194/acp-20-5071-2020, 2020.
Monge, M., D'Anna, B., Mazri, L., Giroir-Fendler, A., Ammann, M., Donaldson, D. J., and George, C.: Light changes the atmospheric reactivity of soot, P. Natl. Acad. Sci. USA, 107, 6605–6609, https://doi.org/10.1073/pnas.0908341107, 2010.
Murphy, B. N., Woody, M. C., Jimenez, J. L., Carlton, A. M. G., Hayes, P. L., Liu, S., Ng, N. L., Russell, L. M., Setyan, A., Xu, L., Young, J., Zaveri, R. A., Zhang, Q., and Pye, H. O. T.: Semivolatile POA and parameterized total combustion SOA in CMAQv5.2: impacts on source strength and partitioning, Atmos. Chem. Phys., 17, 11107–11133, https://doi.org/10.5194/acp-17-11107-2017, 2017.
Oswald, R., Behrendt, T., Ermel, M., Wu, D., Su, H., Cheng, Y., Breuninger, C., Moravek, A., Mougin, E., and Delon, C.: HONO emissions from soil bacteria as a major source of atmospheric reactive nitrogen, Science, 341, 1233–1235, 2013.
Platt, U., Perner, D., Harris, G. W., Winer, A. M., and Pitts, J. N.: Observations of nitrous acid in an urban atmosphere by differential optical absorption, Nature, 285, 312–314, https://doi.org/10.1038/285312a0, 1980.
Pye, H. O. T., Murphy, B. N., Xu, L., Ng, N. L., Carlton, A. G., Guo, H., Weber, R., Vasilakos, P., Appel, K. W., Budisulistiorini, S. H., Surratt, J. D., Nenes, A., Hu, W., Jimenez, J. L., Isaacman-VanWertz, G., Misztal, P. K., and Goldstein, A. H.: On the implications of aerosol liquid water and phase separation for organic aerosol mass, Atmos. Chem. Phys., 17, 343–369, https://doi.org/10.5194/acp-17-343-2017, 2017.
Quan, J., Tie, X., Zhang, Q., Liu, Q., Li, X., Gao, Y., and Zhao, D.: Characteristics of heavy aerosol pollution during the 2012–2013 winter in Beijing, China, Atmos. Environ., 88, 83–89, https://doi.org/10.1016/j.atmosenv.2014.01.058, 2014.
Rasool, Q. Z., Bash, J. O., and Cohan, D. S.: Mechanistic representation of soil nitrogen emissions in the Community Multiscale Air Quality (CMAQ) model v 5.1, Geosci. Model Dev., 12, 849–878, https://doi.org/10.5194/gmd-12-849-2019, 2019.
Riemer, N., Vogel, H., Vogel, B., Anttila, T., Kiendler-Scharr, A., and Mentel, T. F.: Relative importance of organic coatings for the heterogeneous hydrolysis of N2O5 during summer in Europe, J. Geophys. Res.-Atmos., 114, D17307, https://doi.org/10.1029/2008jd011369, 2009.
Romer, P. S., Wooldridge, P. J., Crounse, J. D., Kim, M. J., Wennberg, P. O., Dibb, J. E., Scheuer, E., Blake, D. R., Meinardi, S., Brosius, A. L., Thames, A. B., Miller, D. O., Brune, W. H., Hall, S. R., Ryerson, T. B., and Cohen, R. C.: Constraints on Aerosol Nitrate Photolysis as a Potential Source of HONO and NOx, Environ. Sci. Technol., 52, 13738–13746, https://doi.org/10.1021/acs.est.8b03861, 2018.
Sarwar, G., Roselle, S. J., Mathur, R., Appel, W., Dennis, R. L., and Vogel, B.: A comparison of CMAQ HONO predictions with observations from the northeast oxidant and particle study, Atmos. Environ., 42, 5760–5770, https://doi.org/10.1016/j.atmosenv.2007.12.065, 2008.
Sarwar, G., Fahey, K., Napelenok, S., Roselle, S., and Mathur, R.: Examining the impact of CMAQ model updates on aerosol sulfate predictions, The 10th Annual CMAS Models-3 User's Conference, 24–26 October 2011, Chapel Hill, North Carolina, USA, poster no. 28, available at: https://www.cmascenter.org/conference//2011/slides/sarwar_examining_impact_2011, (last access: 16 October 2021), 2011..
Shao, J., Chen, Q., Wang, Y., Lu, X., He, P., Sun, Y., Shah, V., Martin, R. V., Philip, S., Song, S., Zhao, Y., Xie, Z., Zhang, L., and Alexander, B.: Heterogeneous sulfate aerosol formation mechanisms during wintertime Chinese haze events: air quality model assessment using observations of sulfate oxygen isotopes in Beijing, Atmos. Chem. Phys., 19, 6107–6123, https://doi.org/10.5194/acp-19-6107-2019, 2019.
Skamarock, W. C. and Klemp, J. B.: A time-split nonhydrostatic atmospheric model for weather research and forecasting applications, J. Comput. Phys., 227, 3465–3485, 2008.
Spataro, F. and Ianniello, A.: Sources of atmospheric nitrous acid: State of the science, current research needs, and future prospects, J. Air Waste Manage. Assoc., 64, 1232–1250, https://doi.org/10.1080/10962247.2014.952846, 2014.
Spataro, F., Ianniello, A., Esposito, G., Allegrini, I., Zhu, T., and Hu, M.: Occurrence of atmospheric nitrous acid in the urban area of Beijing (China), Sci. Total Environ., 447, 210–224, https://doi.org/10.1016/j.scitotenv.2012.12.065, 2013.
Stemmler, K., Ammann, M., Donders, C., Kleffmann, J., and George, C.: Photosensitized reduction of nitrogen dioxide on humic acid as a source of nitrous acid, Nature, 440, 195–198, https://doi.org/10.1038/nature04603, 2006.
Stutz, J., Alicke, B., and Neftel, A.: Nitrous acid formation in the urban atmosphere: Gradient measurements of NO2 and HONO over grass in Milan, Italy, J. Geophys. Res.-Atmos., 107, LOP8192, https://doi.org/10.1029/2001JD000390, 2002.
Su, H., Cheng, Y., Oswald, R., Behrendt, T., Trebs, I., Meixner, F. X., Andreae, M. O., Cheng, P., Zhang, Y., and Pöschl, U.: Soil Nitrite as a Source of Atmospheric HONO and OH Radicals, Science, 333, 1616, https://doi.org/10.1126/science.1207687, 2011.
Sun, Y., Wang, Z., Fu, P., Jiang, Q., Yang, T., Li, J., and Ge, X.: The impact of relative humidity on aerosol composition and evolution processes during wintertime in Beijing, China, Atmos. Environ., 77, 927–934, https://doi.org/10.1016/j.atmosenv.2013.06.019, 2013.
Tan, Z., Rohrer, F., Lu, K., Ma, X., Bohn, B., Broch, S., Dong, H., Fuchs, H., Gkatzelis, G. I., Hofzumahaus, A., Holland, F., Li, X., Liu, Y., Liu, Y., Novelli, A., Shao, M., Wang, H., Wu, Y., Zeng, L., Hu, M., Kiendler-Scharr, A., Wahner, A., and Zhang, Y.: Wintertime photochemistry in Beijing: observations of ROx radical concentrations in the North China Plain during the BEST-ONE campaign, Atmos. Chem. Phys., 18, 12391–12411, https://doi.org/10.5194/acp-18-12391-2018, 2018.
Tong, S., Hou, S., Zhang, Y., Chu, B., Liu, Y., He, H., Zhao, P., and Ge, M.: Exploring the nitrous acid (HONO) formation mechanism in winter Beijing: direct emissions and heterogeneous production in urban and suburban areas, Faraday Discuss., 189, 213–230, https://doi.org/10.1039/c5fd00163c, 2016.
Tsona, N. T. and Du, L.: A potential source of atmospheric sulfate from O-induced SO2 oxidation by ozone, Atmos. Chem. Phys., 19, 649–661, https://doi.org/10.5194/acp-19-649-2019, 2019.
USEPA: U.S. Environmental Protection Agency (EPA), Office of Research and Development (ORD): CMAQ (Version 5.3), Zenodo [code], https://zenodo.org/record/3585898, Washington, DC, USA, August 2019.
VandenBoer, T. C., Young, C. J., Talukdar, R. K., Markovic, M. Z., Brown, S. S., Roberts, J. M., and Murphy, J. G.: Nocturnal loss and daytime source of nitrous acid through reactive uptake and displacement, Nat. Geosci., 8, 55–60, https://doi.org/10.1038/ngeo2298, 2015.
VandenBoer, T. C., Brown, S. S., Murphy, J. G., Keene, W. C., Young, C. J., Pszenny, A. A. P., Kim, S., Warneke, C., de Gouw, J. A., Maben, J. R., Wagner, N. L., Riedel, T. P., Thornton, J. A., Wolfe, D. E., Dubé, W. P., Öztürk, F., Brock, C. A., Grossberg, N., Lefer, B., Lerner, B., Middlebrook, A. M., and Roberts, J. M.: Understanding the role of the ground surface in HONO vertical structure: High resolution vertical profiles during NACHTT-11, J. Geophys. Res.-Atmos., 118, 10155–110171, https://doi.org/10.1002/jgrd.50721, 2013.
Vogel, B., Vogel, H., Kleffmann, J., and Kurtenbach, R.: Measured and simulated vertical profiles of nitrous acid – Part II. Model simulations and indications for a photolytic source, Atmos. Environ., 37, 2957–2966, 2003.
Voogt, J. A. and Oke, T. R.: Complete Urban Surface Temperatures, J. Appl. Meteorol., 36, 1117–1132, https://doi.org/10.1175/1520-0450(1997)036<1117:cust>2.0.co;2, 1997.
Wang, G., Zhang, R., Gomez, M. E., Yang, L., Levy Zamora, M., Hu, M., Lin, Y., Peng, J., Guo, S., Meng, J., Li, J., Cheng, C., Hu, T., Ren, Y., Wang, Y., Gao, J., Cao, J., An, Z., Zhou, W., Li, G., Wang, J., Tian, P., Marrero-Ortiz, W., Secrest, J., Du, Z., Zheng, J., Shang, D., Zeng, L., Shao, M., Wang, W., Huang, Y., Wang, Y., Zhu, Y., Li, Y., Hu, J., Pan, B., Cai, L., Cheng, Y., Ji, Y., Zhang, F., Rosenfeld, D., Liss, P. S., Duce, R. A., Kolb, C. E., and Molina, M. J.: Persistent sulfate formation from London Fog to Chinese haze, P. Natl. Acad. Sci. USA, 113, 13630, https://doi.org/10.1073/pnas.1616540113, 2016.
Wang, G., Cheng, S., Wei, W., Yang, X., Wang, X., Jia, J., Lang, J., and Lv, Z.: Characteristics and emission-reduction measures evaluation of PM2.5 during the two major events: APEC and Parade, Sci. Total Environ., 595, 81–92, https://doi.org/10.1016/j.scitotenv.2017.03.231, 2017.
Wang, H., Chen, X., Lu, K., Tan, Z., Ma, X., Wu, Z., Li, X., Liu, Y., Shang, D., Wu, Y., Zeng, L., Hu, M., Schmitt, S., Kiendler-Scharr, A., Wahner, A., and Zhang, Y.: Wintertime N2O5 uptake coefficients over the North China Plain, Sci. Bull., 65, 765–774, https://doi.org/10.1016/j.scib.2020.02.006, 2020a.
Wang, J., Li, J., Ye, J., Zhao, J., Wu, Y., Hu, J., Liu, D., Nie, D., Shen, F., Huang, X., Huang, D. D., Ji, D., Sun, X., Xu, W., Guo, J., Song, S., Qin, Y., Liu, P., Turner, J. R., Lee, H. C., Hwang, S., Liao, H., Martin, S. T., Zhang, Q., Chen, M., Sun, Y., Ge, X., and Jacob, D. J.: Fast sulfate formation from oxidation of SO2 by NO2 and HONO observed in Beijing haze, Nat. Commun., 11, 2844, https://doi.org/10.1038/s41467-020-16683-x, 2020b.
Wang, L. W., Wen, L., Xu, C. H., Chen, J. M., Wang, X. F., Yang, L. X., Wang, W. X., Yang, X., Sui, X., Yao, L., and Zhang, Q. Z.: HONO and its potential source particulate nitrite at an urban site in North China during the cold season, Sci. Total Environ., 538, 93–101, https://doi.org/10.1016/j.scitotenv.2015.08.032, 2015.
Wesely, M.: Parameterization of surface resistances to gaseous dry deposition in regional-scale numerical models, Atmos. Environ., 41, 52–63, 2007.
Wesely, M. L.: Parameterization of surface resistances to gaseous dry deposition in regional-scale numerical models, Atmos. Environ. (1967), 23, 1293–1304, https://doi.org/10.1016/0004-6981(89)90153-4, 1989.
Xing, J., Mathur, R., Pleim, J., Hogrefe, C., Gan, C. M., Wong, D. C., Wei, C., and Wang, J. D.: Air pollution and climate response to aerosol direct radiative effects: A modeling study of decadal trends across the northern hemisphere, J. Geophys. Res.-Atmos., 120, 12221–12236, https://doi.org/10.1002/2015jd023933, 2015.
Xing, J., Wang, J., Mathur, R., Wang, S., Sarwar, G., Pleim, J., Hogrefe, C., Zhang, Y., Jiang, J., Wong, D. C., and Hao, J.: Impacts of aerosol direct effects on tropospheric ozone through changes in atmospheric dynamics and photolysis rates, Atmos. Chem. Phys., 17, 9869–9883, https://doi.org/10.5194/acp-17-9869-2017, 2017.
Xing, L., Wu, J., Elser, M., Tong, S., Liu, S., Li, X., Liu, L., Cao, J., Zhou, J., El-Haddad, I., Huang, R., Ge, M., Tie, X., Prévôt, A. S. H., and Li, G.: Wintertime secondary organic aerosol formation in Beijing–Tianjin–Hebei (BTH): contributions of HONO sources and heterogeneous reactions, Atmos. Chem. Phys., 19, 2343–2359, https://doi.org/10.5194/acp-19-2343-2019, 2019.
Xu, K.-M. and Krueger, S. K.: Evaluation of Cloudiness Parameterizations Using a Cumulus Ensemble Model, Mon. Weather Rev., 119, 342–367, https://doi.org/10.1175/1520-0493(1991)119<0342:eocpua>2.0.co;2, 1991.
Xu, K.-M. and Randall, D. A.: Evaluation of Statistically Based Cloudiness Parameterizations Used in Climate Models, J. Atmos. Sci., 53, 3103–3119, https://doi.org/10.1175/1520-0469(1996)053<3103:eosbcp>2.0.co;2, 1996.
Xu, L., Pye, H. O. T., He, J., Chen, Y., Murphy, B. N., and Ng, N. L.: Experimental and model estimates of the contributions from biogenic monoterpenes and sesquiterpenes to secondary organic aerosol in the southeastern United States, Atmos. Chem. Phys., 18, 12613–12637, https://doi.org/10.5194/acp-18-12613-2018, 2018.
Xu, W., Kuang, Y., Zhao, C., Tao, J., Zhao, G., Bian, Y., Yang, W., Yu, Y., Shen, C., and Liang, L.: NH3-promoted hydrolysis of NO2 induces explosive growth in HONO, Atmos. Chem. Phys., 19, 10557–10570, https://doi.org/10.5194/acp-19-10557-2019, 2019.
Xue, C., Ye, C., Ma, Z., Liu, P., Zhang, Y., Zhang, C., Tang, K., Zhang, W., Zhao, X., Wang, Y., Song, M., Liu, J., Duan, J., Qin, M., Tong, S., Ge, M., and Mu, Y.: Development of stripping coil-ion chromatograph method and intercomparison with CEAS and LOPAP to measure atmospheric HONO, Sci. Total Environ., 646, 187–195, https://doi.org/10.1016/j.scitotenv.2018.07.244, 2019.
Xue, C., Zhang, C., Ye, C., Liu, P., Catoire, V., Krysztofiak, G., Chen, H., Ren, Y., Zhao, X., Wang, J., Zhang, F., Zhang, C., Zhang, J., An, J., Wang, T., Chen, J., Kleffmann, J., Mellouki, A., and Mu, Y.: HONO budget and its role in nitrate formation in the rural North China Plain, Environ. Sci. Technol., 54, 11048–11057, https://doi.org/10.1021/acs.est.0c01832, 2020.
Yarwood, G., Jung, J., Whitten, G. Z., Heo, G., Mellberg, J., and Estes, M.: Updates to the Carbon Bond mechanism for version 6 (CB6), 9th Annual CMAS Conference, Chapel Hill, NC, 11–13, 2010.
Ye, C., Gao, H., Zhang, N., and Zhou, X.: Photolysis of Nitric Acid and Nitrate on Natural and Artificial Surfaces, Environ. Sci. Technol., 50, 3530–3536, https://doi.org/10.1021/acs.est.5b05032, 2016.
Ye, C., Zhang, N., Gao, H., and Zhou, X.: Photolysis of Particulate Nitrate as a Source of HONO and NOx, Environ. Sci. Technol., 51, 6849–6856, https://doi.org/10.1021/acs.est.7b00387, 2017.
Ye, C., Liu, P., Ma, Z., Xue, C., Zhang, C., Zhang, Y., Liu, J., Liu, C., Sun, X., and Mu, Y.: High H2O2 concentrations observed during haze periods during the winter in Beijing: Importance of H2O2 oxidation in sulfate formation, Environ. Sci. Technol. Lett., 5, 757–763, 2018.
Yu, S., Dennis, R., Roselle, S., Nenes, A., Walker, J., Eder, B., Schere, K., Swall, J., and Robarge, W.: An assessment of the ability of three-dimensional air quality models with current thermodynamic equilibrium models to predict aerosol NO, J. Geophys. Res., 110, D07S13, https://doi.org/10.1029/2004JD004718, 2005.
Zhang, J., Chen, J., Xue, C., Chen, H., Zhang, Q., Liu, X., Mu, Y., Guo, Y., Wang, D., Chen, Y., Li, J., Qu, Y., and An, J.: Impacts of six potential HONO sources on HOx budgets and SOA formation during a wintertime heavy haze period in the North China Plain, Sci. Total Environ., 681, 110–123, https://doi.org/10.1016/j.scitotenv.2019.05.100, 2019a.
Zhang, J. W., An, J. L., Qu, Y., Liu, X. G., and Chen, Y.: Impacts of potential HONO sources on the concentrations of oxidants and secondary organic aerosols in the Beijing-Tianjin-Hebei region of China, Sci. Total Environ., 647, 836–852, https://doi.org/10.1016/j.scitotenv.2018.08.030, 2019b.
Zhang, S., Xing, J., Sarwar, G., Ge, Y., He, H., Duan, F., Zhao, Y., He, K., Zhu, L., and Chu, B.: Parameterization of heterogeneous reaction of SO2 to sulfate on dust with coexistence of NH3 and NO2 under different humidity conditions, Atmos. Environ., 208, 133–140, https://doi.org/10.1016/j.atmosenv.2019.04.004, 2019c.
Zhang, W. Q., Tong, S. R., Ge, M. F., An, J. L., Shi, Z. B., Hou, S. Q., Xia, K. H., Qu, Y., Zhang, H. X., Chu, B. W., Sun, Y. L., and He, H.: Variations and sources of nitrous acid (HONO) during a severe pollution episode in Beijing in winter 2016, Sci. Total Environ., 648, 253–262, https://doi.org/10.1016/j.scitotenv.2018.08.133, 2019d.
Zhao, B., Zheng, H., Wang, S., Smith, K. R., Lu, X., Aunan, K., Gu, Y., Wang, Y., Ding, D., Xing, J., Fu, X., Yang, X., Liou, K.-N., and Hao, J.: Change in household fuels dominates the decrease in PM2.5; exposure and premature mortality in China in 2005–2015, P. Natl. Acad. Sci. USA, 115, 12401, https://doi.org/10.1073/pnas.1812955115, 2018.
Zheng, B., Zhang, Q., Zhang, Y., He, K. B., Wang, K., Zheng, G. J., Duan, F. K., Ma, Y. L., and Kimoto, T.: Heterogeneous chemistry: a mechanism missing in current models to explain secondary inorganic aerosol formation during the January 2013 haze episode in North China, Atmos. Chem. Phys., 15, 2031–2049, https://doi.org/10.5194/acp-15-2031-2015, 2015.
Zheng, H., Zhao, B., Wang, S., Wang, T., Ding, D., Chang, X., Liu, K., Xing, J., Dong, Z., Aunan, K., Liu, T., Wu, X., Zhang, S., and Wu, Y.: Transition in source contributions of PM2.5 exposure and associated premature mortality in China during 2005–2015, Environ. Int., 132, 105111, https://doi.org/10.1016/j.envint.2019.105111, 2019.
Zhou, X., Gao, H., He, Y., Huang, G., Bertman, S. B., Civerolo, K., and Schwab, J.: Nitric acid photolysis on surfaces in low-NOx environments: Significant atmospheric implications, Geophys. Res. Lett., 30, 2217, https://doi.org/10.1029/2003gl018620, 2003.