the Creative Commons Attribution 4.0 License.
the Creative Commons Attribution 4.0 License.
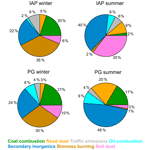
Insight into PM2.5 sources by applying positive matrix factorization (PMF) at urban and rural sites of Beijing
Deepchandra Srivastava
Jingsha Xu
Tuan V. Vu
Di Liu
Linjie Li
Pingqing Fu
Siqi Hou
Natalia Moreno Palmerola
This study presents the source apportionment of PM2.5 performed by positive matrix factorization (PMF) on data presented here which were collected at urban (Institute of Atmospheric Physics – IAP) and rural (Pinggu – PG) sites in Beijing as part of the Atmospheric Pollution and Human Health in a Chinese megacity (APHH-Beijing) field campaigns. The campaigns were carried out from 9 November to 11 December 2016 and from 22 May to 24 June 2017. The PMF analysis included both organic and inorganic species, and a seven-factor output provided the most reasonable solution for the PM2.5 source apportionment. These factors are interpreted as traffic emissions, biomass burning, road dust, soil dust, coal combustion, oil combustion, and secondary inorganics. Major contributors to PM2.5 mass were secondary inorganics (IAP: 22 %; PG: 24 %), biomass burning (IAP: 36 %; PG: 30 %), and coal combustion (IAP: 20 %; PG: 21 %) sources during the winter period at both sites. Secondary inorganics (48 %), road dust (20 %), and coal combustion (17 %) showed the highest contribution during summer at PG, while PM2.5 particles were mainly composed of soil dust (35 %) and secondary inorganics (40 %) at IAP. Despite this, factors that were resolved based on metal signatures were not fully resolved and indicate a mixing of two or more sources. PMF results were also compared with sources resolved from another receptor model (i.e. chemical mass balance – CMB) and PMF performed on other measurements (i.e. online and offline aerosol mass spectrometry, AMS) and showed good agreement for some but not all sources. The biomass burning factor in PMF may contain aged aerosols as a good correlation was observed between biomass burning and oxygenated fractions (r2= 0.6–0.7) from AMS. The PMF failed to resolve some sources identified by the CMB and AMS and appears to overestimate the dust sources. A comparison with earlier PMF source apportionment studies from the Beijing area highlights the very divergent findings from application of this method.
- Article
(3847 KB) - Full-text XML
-
Supplement
(1666 KB) - BibTeX
- EndNote
Atmospheric particulate matter (PM) is composed of various chemical components and can affect air quality (and consequently human health), visibility, and ecosystems (Boucher et al., 2013; Heal et al., 2012). Through absorption and scattering of solar radiation and by affecting clouds, PM also has a major impact on the climate and thus the hydrological cycle. PM with an aerodynamic diameter less than 2.5 µm (PM2.5) is given special attention due to its adverse effects on human health as it can penetrate deep into human lungs when inhaled. Several recent studies have indicated that many adverse health outcomes, such as respiratory and cardiovascular morbidity and mortality, are related to long-term exposure to PM (Lu et al., 2021; Wang et al., 2016; Xing et al., 2016; Xie et al., 2019). In addition, over a million premature deaths per year are reported in China due to poor air quality (GBD MAPS Working Group, 2016). Beijing, the capital city of China, is a megacity with approximately 21 million inhabitants that are regularly exposed to severe haze events. For example, 77 pollution episodes (defined as 2 or more consecutive days where the average PM2.5 concentration exceeds 75 µg m−3) were observed between April 2013 and March 2015 (Batterman et al., 2016). PM2.5 concentrations have reached 1000 µg m−3 in some heavily polluted areas of Beijing (Ji et al., 2014). In addition, a study compared the number of cases of acute cardiovascular, cerebrovascular, and respiratory diseases in the Beijing Emergency Center and haze data from Beijing Observatory between 2006 and 2013. Their results showed a rising trend, highlighting that the average number of cases per day for all three diseases was higher on hazy days than on non-hazy days (Zhang et al., 2015). Therefore, major control measures were implemented to reduce PM2.5 pollution in Beijing (Vu et al., 2019). Recently, one-third of Chinese cities in 2020 were kept under lockdown to prevent the transmission of the COVID-19 virus, which strictly curtailed personal mobility and economic activities. The lockdown led to an improvement in air quality and managed to bring down the levels of PM2.5. Despite these improvements, PM2.5 concentrations during the lockdown periods remained higher than the World Health Organization recommendations, suggesting much more effort is needed (He et al., 2020; Le et al., 2020; Shi et al., 2021). A quantitative source apportionment provides key information to support such efforts.
Receptor models are widely used for source apportionment of PM2.5. These methods include positive matrix factorization (PMF) (Paatero, 1997; Paatero and Tapper, 1994), principal component analysis (PCA) (Lee and Hieu, 2011), chemical mass balance (CMB) (Watson et al., 1990), and UNMIX (Herrera Murillo et al., 2012). Among these methods, PMF is a widely used multivariate method that can resolve the dominant positive factors without prior knowledge of sources. Previous PMF studies, based on high-resolution aerosol mass spectrometer data, have provided valuable information on the sources of PM in urban Beijing and its surrounding areas (Huang et al., 2010; Sun et al., 2010, 2013; Zhang et al., 2013, 2014, 2015, 2016, 2017; Hu et al., 2016; Qiu et al., 2019). However, the factors that influence haze formation and related sources remain unclear due to its inherent complexity (Tie et al., 2017; Sun et al., 2014). Filter-based PMF studies provide a valuable tool for identifying sources of airborne particles, by utilizing size-resolved chemical information (Li et al., 2019; Ma et al., 2017a; Tian et al., 2016; Yu et al., 2013; Song et al., 2007, 2006). These source apportionment studies have predominantly used OC (organic carbon), EC (elemental carbon), water-soluble ions, and metals as the input data matrix to explore the co-variances between species and their associated sources, but to the best of our knowledge, the use of organic markers in PMF has not been explored extensively in Beijing. The use of organic molecular markers in PMF has enhanced our understanding of the PM fraction as they can be source specific (Shrivastava et al., 2007; Jaeckels et al., 2007; Zhang et al., 2009; Wang et al., 2012; Srimuruganandam and Shiva Nagendra, 2012; Schembari et al., 2014; Laing et al., 2015; Waked et al., 2014; Srivastava et al., 2018) and could potentially offer a clearer link between factors and sources.
This study presents the results obtained from the PMF model applied to a filter-based dataset collected in the Beijing metropolitan area at two sites, urban and rural. The study provides source apportionment results from both urban and rural locations in Beijing, including their temporal and spatial variations. In addition, the study also presents a short summary of previously published filter-based studies conducted in the Beijing metropolitan area and their major outcomes. A comparison of the present PMF results was also made with other source apportionment approaches or applications of PMF to other datasets, with an aim of discussing the existing PM sources in the Beijing metropolitan area, including focussing on the strengths and weaknesses of the source apportionment approach employed.
Details about the sampling site, measurements, sample collection, and analytical procedures are reported elsewhere (Shi et al., 2019; Xu et al., 2021; Wu et al., 2020), and hence only the essential information is presented in this section.
2.1 Sampling site and sample collection
The PM2.5 sampling was conducted simultaneously at the urban and rural sites from 9 November to 12 December 2016 and from 22 May to 24 June 2017 as part of the Atmospheric Pollution and Human Health in a Chinese megacity (APHH-Beijing) field campaigns (Shi et al., 2019) (Fig. S1). The urban sampling site (116.39∘ E, 39.98∘ N) – the Institute of Atmospheric Physics (IAP) of the Chinese Academy of Sciences in Beijing – represents a typical condition of central Beijing: there are various roads nearby, including a highway road approximately 200 m away. The rural Pinggu site (PG) (40.17∘ N, 117.05∘ E) is located in Xibaidian. This site is approximately 60 km to the north-east of the Beijing city centre and about 4 km north-west of the Pinggu town centre. The site is surrounded by trees and farmland. In addition, residents mainly use coal and biomass for heating and cooking in individual homes.
Twenty-four-hour PM2.5 samples were collected every day on pre-baked quartz filters (Pallflex, 20×25 cm) and 47 mm polytetrafluoroethylene (PTFE) filters (flow rate of 15.0 L min−1) using high-volume (Tisch, USA, flow rate of 1.1 m3 min−1) and medium-volume (Thermo Scientific Partisol 2025i) air samplers. Field blanks were also collected during the sampling campaign at both sites. The quartz filters were then analysed for organic tracers, OC EC, and ion species. PTFE filters were used for the determination of PM2.5 mass and metals. Details on preparation and conservation of these filter samples have already been reported elsewhere (Wu et al., 2020; Xu et al., 2021).
Real-time composition of non-refractory PM1 particles (NR-PM1) was measured using an Aerodyne aerosol mass spectrometer (AMS) at a time resolution of 2.5 min. The operational details on the AMS measurements have been given elsewhere (Xu et al., 2019). In addition, the measurements of gaseous species such as O3, CO, NO, NO2, and SO2 were performed using gas analysers. The meteorological parameters, including temperature (T), relative humidity (RH), wind speed (WS), and wind direction (WD), were also measured at both sites.
2.2 Analytical procedure
In total, 62 and 72 chemical species were quantified in each PM2.5 sample from IAP and PG, respectively. This included EC OC, 36 organic tracers, 7 major inorganic ions (Na+, K+, Ca2+, NH, Cl−, NO, and SO), and 17 metallic elements (V, Cr, Co, Mn, Ni, Cu, Zn, As, Br, Sr, Ag, Cd, Sn, Sb, Ba, Hg, and Pb) at IAP. Similarly, the identified species at PG included EC OC, 51 organic tracers, 7 major inorganic ions, and 12 metallic elements (V, Cr, Co, Mn, Ni, Cu, Zn, As, Sr, Sb, Ba, and Pb). EC and OC measurements were performed using a Sunset lab analyser (model RT-4) and a DRI multi-wavelength thermal–optical carbon (model 2015) analyser based on the EUSAAR2 (European Supersites for Atmospheric Aerosol Research) transmittance protocol at both sites, IAP and PG, respectively, following the procedure explained by Paraskevopoulou et al. (2014). Major inorganic ions and metallic elements were analysed using an ion chromatograph (Dionex, Sunnyvale, CA, USA) and inductively coupled plasma-mass spectrometer (ICP-MS) at both sites, respectively. Major crustal elements including Al, Si, Ca, Ti, and Fe were determined by an X-ray fluorescence spectrometer (XRF).
Organic tracers at IAP included 11 n-alkanes C24-C34, 2 hopanes, 17 polycyclic aromatic hydrocarbons (PAHs), 3 anhydrous sugars (levoglucosan, mannosan, galactosan), 2 fatty acids (palmitic acid, stearic acid), and cholesterol. These organic tracers were analysed by gas chromatography–mass spectrometry (GC–MS, Agilent 7890A GC plus 5975C mass-selective detector) coupled with a DB-5MS column (30 m × 0.25 mm × 0.25 µm) following the protocol explained in Xu et al. (2020). At PG, organic tracers were analysed based on the method reported by Wu et al. (2020) using GC/MS (Agilent GC-6890N plus MSD-5973N) coupled with an HP-5MS column (30 m × 0.25 mm × 0.25 µm). This included quantification of similar species (12 n-alkanes C24-C35, 9 hopanes, 22 PAHs, 3 anhydrous sugars (levoglucosan, mannosan, galactosan), 4 fatty acids (palmitic acid, stearic acid, linoleic acid, oleic acid), and cholesterol) with few additional ones. Recoveries for the identified organic tracers ranged from 70 % to 100 % and from 80 % to 110 % at IAP and PG, respectively. Field blanks were also analysed as part of quality control and demonstrated very low contamination (< 5 %).
In addition, one or two punches of PM2.5 filter sample were also analysed offline using AMS to investigate the water-soluble OA (WSOA) mass spectra following the procedure explained previously (Qiu et al., 2019).
2.3 Positive matrix factorization
Detailed information on the receptor modelling methods used within this study can be found elsewhere (Paatero and Tapper, 1994; Hopke, 2016). PMF is a multivariate factor analysis tool and based on a weighted least-squares fit, where the weights are derived from the analytical uncertainty. The best model solution was obtained by minimizing residuals obtained between modelled and observed input species concentrations. Estimation of analytical uncertainties for the filter-based measurements was calculated using Eq. (1) (Polissar et al., 1998).
where LDj is the detection limit for compound j and EFj is the error fraction for compound j. The detection limits of all compounds used in the PMF model are given in Table S1 (Supplement). The U.S. Environmental Protection Agency (US-EPA) PMF 5.0 software was used in this work to perform the source apportionment.
2.3.1 Selection of the input data
The selection of species used as input data for the PMF analysis is important and can significantly influence the model results (Lim et al., 2010). The following set of criteria was used for the selection of the input species: signal-to-noise ratio (S N) (Paatero and Hopke, 2003), major PM chemical species, compounds with maximum data points above the detection limit, and those being considered specific markers of a given source (e.g. levoglucosan, picene) (Oros and Simoneit, 2000; Simoneit, 1999) were selected. These steps were taken to limit the input data matrix according to the total number of samples (n= 133); some species were also not included if they belonged to a single source and correlated with another marker of this source. A total of 31 species were used in the model (PM2.5, OC, EC, K+, Na+, Ca2+, NH, NO, SO, Cl−, Ti, V, Mn, Ni, Zn, Pb, Cu, Fe, Al, C26, C29, C31, 17α(H)-22,29,30-trisnorhopane,17β(H),21α(H)-30-norhopane,chrysene, benzo(b)fluoranthene, benzo(a)pyrene, picene, coronene, levoglucosan, and stearic acid). The concentration of PM2.5 was included as a total variable in the model (with large uncertainties) to directly determine the source contributions to the daily mass concentrations.
2.3.2 Selection of the final solution
As recommended, a detailed evaluation of factor profiles, temporal trends, fractional contribution of major species to each factor, and correlations with external tracers were investigated carefully to select the appropriate number of factors.
A few constraints were also applied to the base run to obtain clearer chemical factor profiles in the final solution. The general framework for applying constraints to PMF solutions has already been discussed elsewhere (Amato et al., 2009; Amato and Hopke, 2012). The changes in the Q values were considered here as a diagnostic parameter to provide insight into the rotation of factors. All model runs were carefully monitored by examining the Q values obtained in the robust mode. To limit change in the Q value, only “soft-pulling” constraints were applied. The change in Q robust was < 1 %, which is acceptable as per PMF guidelines (< 5 %) (Norris et al., 2014). Finally, three criteria were used to select the optimal solution, including correlation coefficient (r) between the measured and modelled species, bootstrap, and t test (two-tailed paired t test) performed on the base and constraint runs, as explained previously (Srivastava et al., 2018).
2.4 Back trajectories and geographical origins
The geographical origin of selected identified sources and pollutants was investigated using concentration-weighted trajectory (CWT), non-parametric wind regression (NWR), and cluster analysis methods. NWR combines ambient concentrations with co-located measurements of wind direction and speed and highlights wind sectors that are associated with high measured concentrations (Henry et al., 2009). The general principle is to smooth the data over a fine grid, so that a weighted concentration could be estimated by any wind direction (ϕ)/wind speed (v) couple, where the weighing coefficients are determined through Gaussian-like functions. CWT and cluster analysis assess the potential transport of pollution over a large geographical scale (Polissar et al., 2001). These approaches combine atmospheric concentrations measured at the receptor site with back trajectories and residence time information and help to geographically evaluate air parcels responsible for high concentrations. For this purpose, hourly 24 h back trajectories arriving at 200 m above sea level were calculated from the PC-based version of HYSPLIT v4.1 (Stein et al., 2015; Draxler, 1999). NWR, CWT calculations, and cluster analysis were performed using the ZeFir Igor package (Petit et al., 2017).
2.5 Other receptor modelling approaches
Sources were also resolved at both sites separately using another receptor model known as CMB as well as PMF performed on high-resolution AMS data collected at IAP. Details on sources resolved using these approaches are reported elsewhere (Wu et al., 2020; Xu et al., 2021; Sun et al., 2020).
Briefly, CMB is based on a linear least-squares approach and accounts for uncertainties in both source profiles and ambient measurements to apportion the sources of OC. The US EPA CMB8.2 software was used for this purpose at both sites. The source profiles applied in the model were taken from local studies to better represent the sources, including profiles of straw burning (Zhang et al., 2007), wood burning (Wang et al., 2009), gasoline and diesel vehicles (Cai et al., 2017), industrial and residential coal combustion (Zhang et al., 2008), and cooking (Zhao et al., 2015). Only the source profile for vegetative detritus (Rogge et al., 1993; Wang et al., 2009) was not available from local studies. The selected fitting species included EC, anhydrous sugar (levoglucosan), fatty acids, PAHs, hopanes, and alkanes.
3.1 Overview of PM sources in Beijing based on the current source apportionment study
A seven-factor output provided the most reasonable solution for the PM2.5 source apportionment performed on the combined dataset from IAP and PG (Figs. 1 and 2).
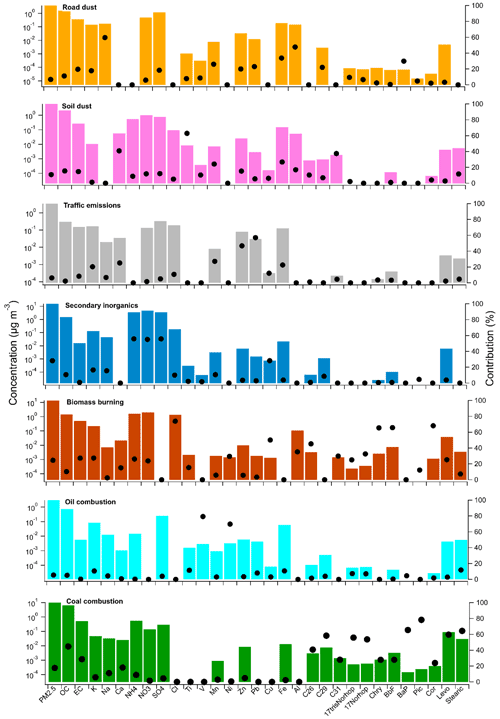
Figure 1Chemical profiles for the identified factors at IAP and PG. The bars show the composition profile (left axis) and the dots the Explained Variation (right axis).
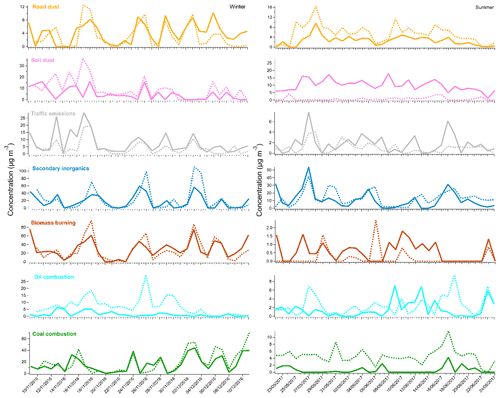
Figure 2Temporal variation of the identified factors at IAP and PG. Solid and broken lines represent IAP and PG, respectively.
Based on the factor profiles, we identified traffic emissions, biomass burning, road dust, soil dust, coal combustion, oil combustion, and secondary inorganics. For the same dataset, solutions with six sources were less explanatory and some factors were mixed. Conversely, an increase in the number of factors led to the split of meaningful factor profiles. In the final solution, the comparison of the reconstructed PM2.5 contributions from all sources with measured PM2.5 concentrations for different seasons at both sites showed good mass closure (r2= 0.61–0.91, slope = 0.99–1.12, p<0.05, ODR – orthogonal distance regression). A low r2 (0.61) value was observed for the summer period at IAP (Fig. S2). This may be due to the inability of PMF to model low concentrations observed for sources such as biomass burning and coal combustion during the summer. In addition, most of the species showed good agreement with measured concentrations (Table S2). Bootstrapping on the final solution showed stable results with more than 95 out of 100 bootstrap-mapped factors (Table S3). Finally, no significant difference (p>0.05) was observed in the factor chemical profiles between the base and constrained runs (Table S4).
Overall, secondary inorganics, biomass burning, and coal combustion sources were the main contributors to the total PM2.5 mass during winter (Fig. 3). These sources accounted for 22 %, 36 %, 20 % and 24 %, 30 %, 21 % of PM2.5 mass at IAP and PG, respectively. Secondary inorganics, road dust, and coal combustion showed the highest contribution during summer at PG, while PM2.5 particles were mainly composed of soil dust and secondary inorganics at IAP. Identified aerosol sources, factor profiles, and temporal evolutions are discussed below. Note that PMF was carried out on the combined datasets and thus only provides a single set of factor profiles for both sites. Similar to previous studies (Li et al., 2019; Ma et al., 2017a; Tian et al., 2016; Yu et al., 2013; Liu et al., 2019; Zhang et al., 2013), neither secondary organic aerosol nor cooking emissions were identified and, given the good mass closure, they must be present within other source categories.
3.1.1 Coal combustion
Coal combustion was identified based on it accounting for a high proportion of PAHs (27 %–78 %), especially picene (78 %) as a specific marker of coal combustion (Oros and Simoneit, 2000), together with significant amounts of OC (45 %) and EC (29 %) (Fig. 1). This factor also made a substantial contribution to n-alkanes (28 %–58 %), stearic acid (64 %), and hopanes (53 %–56 %), as these compounds are also abundant in coal smoke (Bi et al., 2008; Zhang et al., 2008; Oros and Simoneit, 2000; Guo et al., 2015).
The coal combustion factor accounted for 20 % of the PM mass (16.0 µg m−3) at the urban site IAP during winter and followed typical seasonal variations. The contributions of this source to PM2.5 mass were broadly similar (21 % vs. 17 %, Fig. 3) at PG during both seasons, while the average concentrations were higher in winter than summer (19.4 µg m−3 > 4.6 µg m−3). Due to a lack of infrastructure at the rural site PG, the residents still used coal for cooking and heating purposes at the time of sampling (Shi et al., 2019). There is a reduction in coal usage for heating due to elevated temperatures in the summertime, leading to low levels of this factor at IAP. However, the similar contribution at the rural site could be linked to consistent cooking activities throughout the year (Fig. 2) (Shi et al., 2019; Tao et al., 2018). These results were in good agreement with previous observations reported at the same urban site (18 %) (Ma et al., 2017a; Tian et al., 2016). In addition, similar contributions were also observed at other urban locations around Beijing (Wang et al., 2008; Liu et al., 2019).
This factor also included significant contributions from levoglucosan (60 %). Levoglucosan, a major pyrolysis product of cellulose, has been proposed as a molecular marker of biomass burning aerosols (Simoneit, 1999). A study conducted in China suggested that residential coal combustion can also contribute significantly to levoglucosan emissions, based on both source testing and ambient measurements (Yan et al., 2018). Therefore, it is expected that the contribution of levoglucosan is probably linked to residential coal use for cooking in this case. It is possible that the high contribution of levoglucosan could also be linked to model bias as the PMF model only provides average factor profiles for both sites irrespective of their nature (rural vs. urban) and different sampling periods (summer vs. winter).
High concentrations of this source and levoglucosan were observed at low wind speeds (Fig. S3), indicating the significant role of local activities. This was further supported by NWR and CWT analyses, which also showed the influence of local activities during the winter period at both sites. Higher levels of this source were observed at the rural site PG (19.4 µg m−3 vs. 16.0 µg m−3 at the urban site). However, a south-westerly flow was dominant during summer and could be related to transport of air masses from Hebei province, where a large number of industries operate.
3.1.2 Oil combustion
The oil combustion factor profile included high contributions to V (79 %) and Ni (70 %) (see Fig. 1). V and Ni are widely used markers for oil combustion in residential, commercial, and industrial applications (Viana et al., 2008; Mazzei et al., 2008; Pant et al., 2015; Huang et al., 2021). The V Ni ratio obtained in this study was 0.9, close to the previously obtained ratio for residual oil used in power plants (Swietlicki and Krejci, 1996). Results suggest this source might be attributed to residual oil combustion linked to industrial activities as a large number of highly polluting industries are still located in the Beijing neighbourhood (Li et al., 2019). CWT and NWR analysis suggested the influence of regional transport at both sites, highlighting the dominance of south-westerly and south-easterly flows during the winter and summer at both sites (Fig. S4).
The source did not show any seasonal pattern (Fig. 2) and accounted for 2 % (1.4 µg m−3) and 6 % (1.6 µg m−3) at IAP and 8 % (7.1 µg m−3) and 9 % (2.1 µg m−3) at PG of the PM2.5 mass during winter and summer, respectively (Fig. 3). The contribution of this source to the PM mass was within a similar range to the previous study conducted at the nearby urban site (contribution 4.7 %) (Li et al., 2019), which also found a high proportion of V attributed to the identified source.
3.1.3 Biomass burning
The biomass burning factor was characterized by high contributions to Cl− (74 %), K+ (27 %), and levoglucosan (25 %) (Fig. 1). This factor also made significant contributions to PAHs (Chry: 66 %, B[b]F: 66 %, and Cor: 68 %) and followed a clear seasonal variation with a higher contribution in winter (Fig. 2). It accounted for 36 % (29.0 µg m−3) and 30 % (27.3 µg m−3) of the PM2.5 mass during the wintertime at IAP and PG, respectively (Fig. 3), while the contribution during the summertime was extremely low. This was expected due to elevated temperature during the summer period and reduction in biomass burning activities. In addition, NO (24 %) and NH (24 %) also contributed significantly to the biomass burning factor. Biomass burning is an important natural source of NH3 (Zhou et al., 2020) which rapidly reacts with HNO3 to form NH4NO3 aerosols. The presence of NH4NO3 aerosols in biomass burning plumes has also been reported previously (Paulot et al., 2017; Zhao et al., 2020).
It was unexpected to observe a low contribution of levoglucosan, a known biomass burning marker, to this factor. However, model bias and the contribution of other relevant sources to levoglucosan could have caused such observations, as discussed above. K+ is also produced from the combustion of wood lignin and has been used extensively as an inorganic tracer to apportion biomass burning contributions to ambient aerosol (Zhang et al., 2010; Lee et al., 2008a). However, the contribution of K+ to this factor was relatively low, possibly because K+ also has other sources, such as soil dust (Duvall et al., 2008). Cl− can be emitted from both coal combustion and biomass burning, especially during the cold period in Beijing (Sun et al., 2006). It is also important to note that high Cl− levels observed in this factor could be associated with coal combustion (Wang et al., 2008). If we consider this, high Cl− levels related to the coal combustion factor should have also shown a significant contribution to PM mass during the summertime at the rural site (PG) as residents near the rural site mostly use coal and biomass for cooking activities as discussed above, but they do not. Results suggest this factor can be attributed mainly to biomass burning aerosols in the Beijing metropolitan area, but some influence of coal combustion signals cannot be ignored. Back-trajectory analysis also confirmed the local origin of this source during the wintertime at both sites (Fig. S5).
The source contribution reported in the present study was higher than that found in earlier studies in Beijing (11 %–20 %) (Li et al., 2019; Ma et al., 2017a; Tian et al., 2016; Yu et al., 2013; Song et al., 2007, 2006; Liu et al., 2019), suggesting some inclusion of coal burning. As both these sources follow a similar typical seasonal variation, i.e. high concentration during the cold period, it makes their separation difficult due to correlation.
3.1.4 Secondary inorganics
Secondary inorganics were typically characterized by high contributions to NO, SO, and NH (55 %, 56 %, and 56 % of the total species mass, respectively) (Fig. 1). This factor showed a temporal variation, with remarkably high concentrations observed during the period of high RH and low ozone concentration in the winter (Fig. S6). The heterogeneous reactions on pre-existing particles in the polluted environment under high-RH and low-ozone conditions have been shown to play a key role in the formation of secondary aerosols compared to gas-phase photochemical processes (Sun et al., 2013; Niu et al., 2016; Ma et al., 2017b). Therefore, aqueous-phase processes may be the major formation pathway for secondary inorganic aerosols in Beijing during the study period. Additionally, the factor showed a similar contribution (22 %–24 %) to PM mass in winter at both sites. This value is lower than the value reported by Liu et al. (2019) at the other urban location (44 %) in Beijing as a part of the same APHH-Beijing campaign, although it should be noted that the sampling site and dates of sampling differed. We also noticed the source profile reported by Liu et al. (2019) contained the majority of all measured secondary inorganic species (> 70 %) as well as 20 % of OC, while the factor identified in the present study only accounted for ∼ 55 % of secondary inorganic species and 11 % of OC with the remaining fractions identified in other factors. Thus, although the identification of the factor was “secondary” in both studies, they do not represent exactly the same source. The modelled difference in the contribution of this factor to PM mass may also be related to the uncertainties of the input species: a filter-based dataset was used in the present study, while Liu et al. (2019) used online measurements.
The highest contribution to the PM mass was observed during the summertime, with average concentrations of 11.1 µg m−3 (40 %) and 13.2 µg m−3 (48 %) at IAP and PG, respectively (Fig. 3).
3.1.5 Traffic emissions
The traffic emissions factor showed relatively high contributions to metallic elements, such as Zn (47 %), Pb (57 %), Mn (27 %), and Fe (22 %) (Fig. 1). Zn is a major additive to lubricant oil. Zn and Fe can also originate from tyre abrasion, brake linings, lubricants, and corrosion of vehicular parts and tailpipe emission (Pant and Harrison, 2012, 2013; Grigoratos and Martini, 2015; Piscitello et al., 2021). As the use of Pb additives in gasoline has been banned since 1997 in Beijing, the observed Pb emissions may be associated with wear (tyre/brake) rather than fuel combustion (Smichowski et al., 2007). These results suggest the contribution of both exhaust and non-exhaust traffic emissions to this factor. Further quantification of different types of non-exhaust emissions are hard to predict as these metal concentrations varies according to several parameters, such as traffic volume and patterns, vehicle fleet characteristics, and the climate and geology of the region (Duong and Lee, 2011).
Traffic sources accounted for 9 % and 6 % of PM2.5 mass during the wintertime and summertime at IAP (Fig. 3), corresponding to average concentrations of 7.4 µg m−3 and 1.8 µg m−3, respectively. In addition, a low contribution (4 %) was observed at PG during both seasons, as PG experiences a low traffic volume. The contribution of the traffic source to the PM2.5 mass was found to be low compared to other studies conducted in the Beijing area (14 %–20 %) (Li et al., 2019; Tian et al., 2016; Yu et al., 2013; Liu et al., 2019), with the exception of a study by Ma et al. (2017a) where a similar contribution was reported. The observed low contribution was further supported as a recent study also confirms that road traffic remains a dominant source of NOx and primary coarse PM; however, it only accounts for a relatively small fraction of PM2.5 mass at urban locations in Beijing (Harrison et al., 2021). It should be noted that nitrate that can be formed from NOx emitted from road traffic is not included in this factor. Despite the low factor contribution, the resolved chemical profile of this source was consistent with previously identified profiles linked to road traffic emissions in the Beijing area (Ma et al., 2017a; Yu et al., 2013). We noticed that OC and EC contribution in this factor is relatively low, while it may be higher in traffic emissions. However, given the modern gasoline fleet in Beijing (Jing et al., 2016), it is not unexpected to observe low OC and EC contributions. In addition, there was no obvious seasonal variation as expected, though slightly higher concentrations were observed in the cold period, probably due to the typical atmospheric dynamics and consequent poorer dispersion at this time of year.
Metallic elements such as Mn, Fe, and Zn were also used previously to indicate industrial activities (Li et al., 2017; Yu et al., 2013). Back-trajectory analysis reveals the influence of local emissions with a slight regional transport during the winter period at both sites, dominated by north-easterly flow (Fig. S7). Therefore, there is a possibility that these elements could also come from Hebei province, where a large number of smelter industries are located. North-easterly and south-easterly flows were dominant during the summer period at IAP and PG, with possible regional influence. These observations suggest that indeed this factor contains traffic aerosols, though a significant influence of industrial emissions cannot be ruled out.
3.1.6 Road dust
This factor makes a major contribution to crustal species, such as Na+, Al, and Fe (60 %, 48 %, and 34 % of species in this factor, respectively), suggesting this factor may represent the characteristics of a dust-related source as reported previously (Kim and Koh, 2020). Such a high contribution of Na+ in the identified factor was unexpected. Na is a major element of sea salt, sea spray, and marine aerosols (Viana et al., 2008) and has also been found to be enriched in fine particulates from coal combustion (Takuwa et al., 2006). However, the significant influence of marine activities was not expected as Beijing is far away from the sea. It is also notable that a high proportion of Na+ was attributed to road dust in a previous study conducted at the same urban site (Tian et al., 2016), and a crustal source seems likely but has not been confirmed. In addition, the given factor also included significant contributions to Mn, Pb, and Zn (26 %, 23 %, and 20 % of species in this factor, respectively), which are associated with brake and tyre wear as mentioned above (Pant and Harrison, 2012, 2013; Grigoratos and Martini, 2015; Piscitello et al., 2021). High concentrations of Zn and Pb have also been reported for particles emitted from asphalt pavement (Canepari et al., 2008; Sörme et al., 2001). In addition, the ratio of Fe Al observed in the factor chemical profile was 1.26, much higher than the value observed in the earth's crust (0.6), suggesting an anthropogenic origin of some Fe (Sun et al., 2005). This is likely as processes associated with vehicles, such as tyre/brake wear and road abrasion, can contaminate soil with metals, as the urban sampling site is located close to roads, suggesting the resolved factor is likely linked to road dust emissions. These metals (Fe and Al) can also have industrial sources as already reported in the Beijing area (Wang et al., 2008; Tian et al., 2016; Yu et al., 2013; Li et al., 2019). The Beijing–Tianjin–Hebei region is the largest urbanized megalopolis region in northern China and home to many iron and steel-making industries. Fe is a characteristic component of iron and steel industry emissions (Li et al., 2019), while Al may also come from metal processing (Yu et al., 2013). However, disentangling the influence of industrial emissions would require further investigation.
This source also made significant contributions to OC, EC, and SO (11 %–19 %) (Fig. 1) and was consistent with the road dust source profiles observed previously in the Beijing area (Song et al., 2006, 2007; Tian et al., 2016; Yu et al., 2013). This factor accounted for 20 % of the PM2.5 mass during the summertime (5.5 µg m−3), with an exceptionally low contribution (3 %) during the cold period at PG (Fig. 3). However, the factor contribution at IAP was similar during both seasons. In addition, the contribution to PM mass at IAP in this study was similar to that reported by Tian et al. (2016), and the studied urban site in both cases was the same. Crustal dust mass was also estimated based on the concentrations of Al, Si, Fe, Ca, and Ti using the equation below (Chan et al., 1997).
A good correlation was observed between the estimated crustal dust and this factor during both seasons at PG (rural, winter: r2= 0.78, m (slope) = 0.9; summer: r2= 0.94, m= 0.5) and IAP (urban, winter: r2= 0.51, m= 1.3; summer: r2= 0.68, m= 1.2), highlighting that this may also contain a significant fraction of crustal dust (Fig. S8). This suggests that the identified factor is not resolved cleanly and contains a mixed characteristic of road dust and crustal dust.
3.1.7 Soil dust
This factor mainly represents wind-blown soils and was typically characterized by a high contribution to crustal elements, such as Ti (63 %), Ca2+ (41 %), Fe (27 %), and Al (17 %) (Fig. 1). In addition, the contributions to Mn and Zn in the factor profile (Mn = 24 %, Zn = 15 %) suggest that the given source also included resuspended road dust but probably to a lesser extent. This source also showed a significant contribution to n-alkanes (e.g. C29, C31), derived from epicuticular waxes of higher plant biomass (Kolattukudy, 1976; Eglinton et al., 1962), with the highest contribution (37 %) to C31. This suggests the presence of plant-derived organic matter in the soil dust, which is also consistent with a high contribution to OC (15 %).
No clear seasonal variation was observed at PG. However, this factor showed a high contribution (35 %, 9.8 µg m−3) to PM2.5 mass during the summertime at IAP, while the contribution during other seasons at both sites was less than 10 % (Fig. 3). The factor profile resolved here was similar to the profile reported by Ma et al. (2017a) for soil dust, but their soil dust factor only showed a 10 % contribution to PM2.5 mass. In addition, other previous studies (Yu et al., 2013; Zhang et al., 2013) also reported a significant contribution of soil dust to PM2.5 mass, suggesting that soil dust is an important contributor to PM2.5 mass in the Beijing area. It is also expected as Beijing is in a semi-arid region and there are sparsely vegetated surfaces both within and outside the city. This factor also showed good agreement with the crustal fraction estimated from the element masses only during winter at PG (r2= 0.51) and summer at IAP (r2= 0.58). This again highlights the likely mixing of this source with other factors or misattribution. Back-trajectory analysis also indicates the influence of regional transport during the summer period at IAP, dominated by south-easterly–south-westerly flow (Fig. S9) due to high wind speeds (3.6 m s−1). Therefore, there is a possibility that the high contribution is linked to long-range transport in advected air masses. A recent study (Gu et al., 2020) conducted in Beijing showed the high concentrations of more oxidized aerosols during summer due to enhanced photochemical processes; however, such a type of source was not resolved due to a lack of filter-based markers. This suggests the given source may contain some influence from an unidentified/unresolved SOC fraction. Although the most plausible attribution appears to be to soil dust, it is not fully resolved from other sources.
The use of Si in PMF could provide a better understanding of these dust-related sources. However, it is not used in the present PMF input due to a high number of missing data points. The sensitivity of PMF results to the use of Si has also been investigated by adding Si to the input matrix and providing high uncertainty to the missing data. No change was observed in the factor profile and temporal variation of the resolved factors compared to the present one. In addition, we also noticed a good correlation between Si and Al, where Al has been used in PMF (Fig. S10). Several PMF runs were also made with inorganic data only; however, the resolved factors were either mixed or hard to identify. In addition, attempts to improve the PMF results by varying the input species and by analysing data for the IAP and PG sites separately did not offer any advantage.
3.2 Comparison of filter-based PMF results with other receptor modelling approaches on the same dataset
The source apportionment results from PMF were compared with those from CMB on the same filter-based composition data and PMF performed on other measurements, i.e. online AMS (PM1) and offline AMS (PM2.5), to get a deeper insight into the identified PMF factors and their origins (Figs. 4–7). The CMB method resulted in the estimation of eight OC sources (i.e. vegetative detritus, residential coal combustion (CC), industrial CC, cooking, diesel vehicles, gasoline vehicles, biomass burning, other OC), including one secondary factor (other OC) at both sites (IAP and PG). The online AMS datasets allowed the identification of six OA (organic aerosol) – MOOOA (more oxidized oxygenated OA), LOOOA (low more oxidized oxygenated OA), OPOA (oxidized primary OA), BBOA (biomass burning OA), COA (cooking OA), and CCOA (coal combustion OA) – factors during winter at IAP, while analyses of the offline AMS measurements resolved four OA (OOA, BBOA, COA, CCOA) factors.
For these analyses, OC concentrations related to the online/offline AMS OA factors were further calculated by applying OC-to-OA conversion factors specific to each source, i.e. 1.35 for coal combustion organic carbon (Sun et al., 2016), 1.38 for cooking organic carbon, 1.58 for biomass burning organic carbon (Xu et al., 2019), and 1.78 for the oxygenated fraction (Huang et al., 2010), and used to evaluate the OC concentrations of relevant OA factors.
Only OC-equivalent concentrations were used to perform comparisons for all approaches. OC mass closure was also verified at IAP during the wintertime by investigating the relation between OC modelled by online AMS–PMF vs. filter-based PMF (r2= 0.7, slope = 1.17), OC measured vs. OC modelled by filter-based PMF (r2= 0.7, slope = 1.07), OC measured vs. OC modelled by online AMS–PMF (r2= 0.9, slope = 0.92), OC modelled by offline AMS–PMF vs. OC modelled by filter-based PMF (r2= 0.6, slope = 0.75), OC measured vs. OC modelled by offline AMS–PMF (r2= 0.9, slope = 1.41), and OC measured vs. WSOA (offline AMS) (r2= 0.9, slope = 0.85) (Fig. S11). The comparison of OC modelled by PMF and CMB was also investigated at IAP (r2= 0.8, slope = 1.05) and PG (r2= 0.6, slope = 1.78) (Fig. S12). All source apportionment approaches showed fairly good agreement in reconstructing the total OC mass, justifying their direct comparison. In addition, it should be noted that the difference in the sampling size cut-off between online AMS (NR–PM1) and filter measurements (PM2.5) may contribute to the differences observed in the source apportionment results. Therefore, we also compared the relation between NR–PM measured vs. PM measured (r2= 0.96, slope = 0.92) and NR-PM measured vs. PM modelled by filter-based PMF (r2= 0.9, slope = 1.29) (Fig. S13). The agreements observed suggest that most of the PM2.5 mass was accounted for by the PM1 fraction, indicating that the difference in the size cut-off is relatively small.
3.2.1 With CMB results at IAP
Resolved CMB and PMF factors were compared, including data from both seasons at IAP and PG (Fig. 4). A good correlation (r2= 0.6, n= 68, p< 0.05) was observed between biomass burning factors, suggesting that this source was well resolved using both approaches (Fig. 4). However, a slightly higher concentration was reported by the CMB model (2.0 and 1.6 µg m−3 by CMB and PMF, respectively). Individual coal combustion factors (industrial/residential) did not show any significant correlation (r2<0.2) with the coal combustion factor identified using PMF, although the total coal combustion fraction from CMB, the sum of industrial and residential fractions, did show an improved correlation (r2=0.4). Some improvement of the correlation was seen if two outlier data points were removed (see Fig. 4). A likely reason is that PMF did not resolve coal combustion and biomass burning factors well, as both factors presented a strong seasonal pattern with high concentrations during the winter. Another possibility is the difficulty in resolving primary and secondary fractions due to a lack of secondary organic markers used in the study. This was further supported by the fact noted above that the PMF biomass burning factor also contained some signal from coal combustion activities. The sum of coal combustion and biomass burning factors from both approaches showed a good correlation (r2= 0.7, n= 68, p< 0.05), suggesting a common emission pattern (e.g. high in winter and low in summer), making it challenging to resolve them. Factors linked to vehicle emissions did not show any correlation. A weak correlation (r2= 0.3, n= 68, p< 0.05) was observed between other OC from CMB, a proxy for the secondary organic fraction and the PMF secondary inorganic factor. In addition, other OC also weakly correlated with soil dust (r2= 0.22, n= 34, p< 0.05) in summer, suggesting the mixing of an unresolved secondary fraction with the soil dust profile and supporting the hypothesis discussed above. It should be noted that other OC could also contain unresolved primary fractions as PMF results indicated substantial influence of industrial emissions and dust-related sources. However, the source profiles related to industrial emissions and dust were not accounted for in the CMB model (Xu et al., 2021).
3.2.2 With CMB results at PG
The comparison was also made using data from both seasons at PG (Fig. 5). Biomass burning aerosols showed a good correlation for both approaches (r2= 0.7, n= 20, p< 0.05), but a substantially higher concentration was estimated by the CMB model (5.1 and 2.0 µg m−3 by CMB and PMF, respectively). A significant correlation was also seen between traffic-related factors from CMB and PMF (gasoline–CMB vs. traffic: r2= 0.6, n= 20, p< 0.05, diesel–CMB vs. traffic: r2= 0.6, n= 20, p< 0.05), indicating that traffic sources resolved using PMF at the PG site may have included signals from both diesel and gasoline vehicles; however, it was not conclusive at the IAP site, as discussed above. This suggests the traffic source resolved using PMF may contain particles linked to traffic emissions, but the influence of other sources is prominent at IAP and resulted in poor correlation. In addition, for traffic-related factors from CMB, both showed a higher concentration (gasoline–CMB = 0.8 µg m−3, diesel–CMB = 4.5 µg m−3, traffic–PMF = 0.2 µg m−3). As with IAP, no significant correlation was observed between coal combustion factors from both approaches. The sum of coal combustion and biomass burning factors from both approaches also did not present a good correlation (r2= 0.3, n= 20, p< 0.05). This highlights the limitation of these methodologies in apportioning sources when extreme meteorological conditions may lead to high internal mixing of sources. Unfavourable dispersion conditions have been previously observed in the Beijing region during severe haze events in winter (Wang et al., 2014). A high correlation was observed between other OC (CMB) and secondary inorganics (PMF) (r2= 0.7, n= 20, p< 0.05). In addition, other OC also showed a very high correlation with the biomass burning factor resolved from PMF (r2= 0.9, n= 20, p< 0.05). This suggests that the biomass burning factor in PMF may contain a substantial amount of aged aerosols since carbon emitted during biomass burning is in some cases oxygenated and water soluble (Lee et al., 2008b) and is subject to rapid oxidation in the atmosphere.
3.2.3 With online AMS–PMF factors at IAP (winter)
BBOC (biomass burning OC) from PMF–AMS analysis agreed well with that from PMF (r2= 0.7, n= 27, p< 0.05; 4.0 and 3.1 µg m−3 by online AMS and PMF, respectively) (Fig. 6). Coal-combustion-related factors showed a modest correlation (CCOC (coal combustion OC) vs. coal combustion–PMF, r2= 0.4, n= 27, p< 0.05), but the mass concentration of the coal combustion source by PMF (11.3 µg m−3) is significantly higher than by PMF–AMS (CCOC = 4.7 µg m−3). This may partly be due to the different size cut-offs used by these measurements (PM1 for AMS vs. PM2.5). In addition, significant improvement in the correlation was seen if two outlying points were removed (r2= 0.8; see Fig. 6). Oxygenated fractions from AMS, MOOOC (more oxidized oxygenated OC), and LOOOC (low oxidized oxygenated OC) also exhibited a good correlation with secondary inorganics (LOOOC vs. secondary inorganics (r2= 0.6, n= 27, p< 0.05, LOOOC = 2.9 µg m−3, secondary inorganics = 1.6 µg m−3), MOOOC vs. secondary inorganics (r2= 0.7, n= 27, p< 0.05, MOOOC = 4.4 µ m−3)). This was also confirmed by LOOOC and MOOOC showing a good correlation with NO and SO previously (Cao et al., 2017). The formation of both secondary inorganic aerosol and oxygenated organic aerosol is dependent upon largely the same set of oxidant species, notably but not solely the hydroxyl and nitrate radicals. In both cases there are also both homogeneous and heterogeneous (aqueous-phase) pathways, so conditions which promote the formation of oxidized organic aerosol will also favour formation of secondary inorganic aerosol, and hence a correlation is to be expected and is often observed (Hu et al., 2016; Zhang et al., 2011). In addition, both oxygenated fractions were also found to be correlated with biomass burning aerosols (LOOOC vs. biomass burning–PMF: r2= 0.7, n= 27, p< 0.05, MOOOC vs. biomass burning–PMF: r2= 0.6, n= 27, p< 0.05). This further highlights a potentially important role of biomass burning activity in SOA formation at IAP. A good correlation was also observed between OPOC (oxidized primary OC) and secondary inorganics and biomass burning (r2= 0.7, n= 27, p< 0.05).
3.2.4 Offline AMS–PMF factors at IAP (winter)
BBOC from PMF-offline AMS analysis showed a good correlation with that from PMF (r2= 0.6, n= 32, p< 0.05) (Fig. 7), but the mass concentration of BBOC (4.6 µg m−3) is higher than biomass burning (3.1 µg m−3) from PMF. This was also noticed above while comparing with BBOC resolved using online AMS–PMF, suggesting a potential uncertainty in estimating the source contribution from biomass burning. The uncertainty in filter-based PMF analysis could be related to model error. This was further supported as the biomass burning factor also made significant contributions to Ca2+ (15 %), Ni (30 %), Cu (50 %), and Al (35 %), and these species are not necessarily from biomass burning emissions, but they were not resolved by PMF. In addition, the uncertainties linked to PMF–AMS analysis could also contribute. A high correlation was noticed for secondary factors resolved using both approaches (OOC (oxygenated OC) vs. secondary inorganics, r2= 0.8, n= 32, p< 0.05). OOC also showed a good correlation with the biomass burning factor (OOC vs. biomass burning–PMF, r2= 0.7, n= 32, p< 0.05). This supports the hypothesis discussed above on the origin of oxygenated fractions.
Overall, the comparison of filter-based PMF results was in broad agreement with other receptor modelling approaches applied on the same dataset. However, large discrepancies were also observed for some factors/sources. Common sources such as biomass burning and coal combustion were well resolved using all the approaches, with some exceptions observed when using a filter-based PMF approach. This could be linked to internal mixing of sources when the influence of climate and local meteorology on both sources is predominant and makes it challenging to resolve using PMF. Good agreement was also observed between secondary inorganic aerosols and secondary fractions resolved using other approaches. However, sources identified based on metal signatures using PMF indicated some mixing or misattribution. For example, the influence of unresolved SOC on the soil dust profile was observed during summer.
3.3 Comparison with previous PMF source apportionment results in Beijing
In this section an attempt has been made to understand the PM sources identified in the Beijing metropolitan area by previous studies. The goal was here to assess the previous PMF source apportionment results and report any discrepancies noticed in the resolved sources using PMF. This may provide useful insight into sources resolved in the present study and also in exploring the issues associated with filter-based PMF modelling in the Beijing metropolitan area. Details of the studies conducted to evaluate PM sources using a PMF model applied to inorganic and organic markers in the Beijing metropolitan area are presented in Table S5, and the major outcomes are discussed hereafter.
Overall, these previous PMF studies provide insights into PM sources in the Beijing metropolitan area (Li et al., 2019; Ma et al., 2017a; Tian et al., 2016; Yu et al., 2013; Song et al., 2007, 2006; Liu et al., 2019; Wang et al., 2008; Zhang et al., 2013). The major identified sources are dust, traffic emissions, coal combustion, industrial activity, secondary inorganic aerosols, and biomass burning, although there is a general issue of their inability to identify sources such as secondary organic aerosol and cooking emissions, similar to the present study, due to the lack of organic markers used in the PMF model to apportion these sources. However, beyond this, their PMF outcomes were not consistent. Large discrepancies between the sources were seen (Table S5) based on the sources identified as well as their contribution to PM mass concentrations. Several factors could cause these differences, such as the chemical species used as input in the PMF model, the period of the study, identification of sources based on chemical signatures, and changes to the sources with time.
Input species considered within the previous studies were combinations of water-soluble ions, metallic elements, OC, and EC. Similar input species were used in all of these studies, with the exception of the studies by Yu et al. (2013) and Li et al. (2019), who used only metallic elements for the source apportionment. As shown in this study, including organic markers may help to resolve some of the primary sources.
Another important parameter, the chemical species used for identifying sources, was not always consistent. For example, coal combustion was resolved based on high contributions of OC, EC, and Cl present in the factor profile by Zhang et al. (2013), Wang et al. (2008), and Song et al. (2007, 2006), in accordance with source profiles determined in the laboratory (Zheng et al., 2005). High Cl associated with fine aerosols in winter is a distinctive feature in Beijing and even around inland China, which is ascribed to coal combustion (Wang et al., 2008). Contrarily, Tian et al. (2016) identified coal combustion based on a high contribution of OC and EC, while the high contribution of Cl was attributed to a biomass burning source, similarly to another study (Ma et al., 2017a). In other studies (Li et al., 2019; Yu et al., 2013; Liu et al., 2019), coal combustion was resolved based on the presence of metallic elements such as V, Se, Co, Cd, As, and Ni, where V and Ni are widely used markers for oil combustion (Mazzei et al., 2008). High loadings of As and Se have also been reported as a typical source characteristic of coal combustion (Vejahati et al., 2010). Similarly to coal combustion, biomass burning was often characterized using the presence of K (Li et al., 2019; Tian et al., 2016; Yu et al., 2013; Song et al., 2007, 2006; Zhang et al., 2013; Liu et al., 2019; Wang et al., 2008), a typical marker of biomass burning. Farming in Beijing's suburban districts has been extensive in recent years. Burning of the crop remnants and fallen leaves by farmers in autumn and winter results in the enhanced emissions of K. In addition, the contributions of Cl and Na were also considered for the identification of these sources in some cases, depending on the species used within the input (Song et al., 2007, 2006; Tian et al., 2016). This highlights the fact that none of the studies have used organic markers such as picene and levoglucosan, which are very specific to these combustion sources as discussed before, which may cause uncertainty in the resolved sources. However, in the present study the use of organic markers played a key role in the identification of these sources and their better apportionment. Despite this, some issues were observed with these identified sources during winter due to extreme meteorological conditions as well as co-emission of these aerosols at the same time, probably indicative of poor performance of the PMF model under certain conditions.
Other important sources linked to traffic emissions, industrial activities, and dust are commonly resolved among all the studies. The characterization of these sources was predominantly based on the metallic elements. For example, Zn, Cu, and Pb including sometimes EC were most often used to characterize traffic emissions among all previous studies. Both Zn and Cu have been identified within brake linings and tyre fragments (Thorpe and Harrison, 2008), and Pb has been used in the past within gasoline as an anti-knock additive in China (Li et al., 2019). However, Cu and Zn can also serve as indicators for industrial sources (Li et al., 2017; Yu et al., 2013). Other metallic elements (e.g. Sb, Cr, Mn, K, Br, and Ba) were also considered in certain cases to trace traffic emissions (Ma et al., 2017a; Tian et al., 2016; Yu et al., 2013). However, a high contribution of Cr, Mn, and sometimes Fe to the given sources has also been attributed to industrial activities. Both Cr and Cr-containing compounds are widely used in metallurgy, electroplating, pigment, leather, and other industries (Dall'Osto et al., 2013). A previous study found that ferrous metallurgy could emit Mn (Querol et al., 2006). Furthermore, both Fe and Mn are characteristic components of iron and steel industry emissions. In addition, Co, Mg, Al, Ca, Cd, Pb, Tl, Zn, V, Ni, and Cu were also considered for the apportionment of industrial sources (Tian et al., 2016; Yu et al., 2013). Zhang et al. (2013) identified a mixed source of traffic and incineration emissions based on high loadings of Cu, Zn, Cd, Pb, Sb, Sn, Mo, NO, and EC. In the present study, the assignment of road traffic emissions was based on high loadings of Zn and Pb. It was also seen that the given source may contain some influence from industrial activities, as the industrial contribution was not resolved like previous studies and was probably accounted for in other factors. Thus, it is clear that these metals could belong to several sources, and their proper assignment to respective sources is difficult in the complex environment.
The same issue was observed with the assignments of dust type (dust/road dust/soil dust/mineral dust/yellow dust/local dust) sources. Although the dust-type sources were often found to be composed of crustal elements (e.g. Ca, Mg, Si, Ti, Al, and Fe), the attribution of crustal elements to a particular source was not consistent from one study to another previously. The two dust sources (road dust and soil dust) identified in the present study also indicated mixing with other factors.
The identification of the secondary inorganic aerosol factors was often based on the high contribution of water-soluble ions (NO, SO, NH), consistent with other studies (Ma et al., 2017a; Song et al., 2007, 2006; Tian et al., 2016; Liu et al., 2019; Wang et al., 2008; Zhang et al., 2013). These results highlight the role of chemical species used in characterizing source profiles and their influence on the variability noticed in the Beijing metropolitan area. This issue arises because many of these species are not source specific, making it challenging to directly link PMF factors to sources. Pant and Harrison (2012), reviewing receptor modelling studies from India, noted a tendency to attribute metal-rich source profiles to “industry” in a rather casual manner without evidence of local industrial sources.
The change in sources and emissions over the course of time due to stringent emissions regulations could also be considered plausible for the observed variability in the chemical profile and contribution of identified sources. Li et al. (2019) showed that levels of trace metals (V, Cr, Mn, As, Cd, and Pb) decreased by more than 40 % due to the emissions regulations, while crustal elements decreased considerably (4 %–45 %), suggesting emissions from anthropogenic activities were suppressed. A reduction in the contribution of sources such as dust and industrial activity was observed in the present study and another recent study performed by Liu et al. (2019) relative to the previous ones, indicating the effect of regulatory measures on the contribution of identified sources to PM2.5. However, the concentration of the majority of metallic elements (K, Cr, Mn, Fe, Co, Cu, Zn, As, Ag, Cd, and Pb) increased when pollution levels changed from clean days to heavily polluted days. This highlights that specific atmospheric conditions could also play a major role for the observed variability. Another factor is the time of year when these studies were conducted as some of the identified sources (e.g. coal combustion and biomass burning) exhibit typical seasonal patterns. During a low-concentration period, PMF models may have difficulty in resolving sources, leading to mixing of factors.
Overall, the present study provides a view of existing PM2.5 sources in the Beijing metropolitan area by applying the PMF model to a filter-based dataset, which included water-soluble ions, metals, and organic markers. Despite this, factors that were resolved based on metal signatures were not fully resolved and indicate a mixing of different sources. As a part of the same campaign, also discussed above, Liu et al. (2019) used a similar approach by applying PMF on high-resolution (1 h) data, which included OC, EC, ions, and metals, and did not encounter any issue. However, previous filter-based PMF studies conducted in the Beijing region that mostly included ions and metals in their input dataset often showed difficulty in the proper assignment of metals to their respective sources. Even the use of metal signatures from one to another study was not consistent. This highlights that the low temporal resolution of filter data could not capture quickly occurring atmospheric processes in Beijing and may lead to a “blurring” of sources by the long averaging period. Atmospheric circulation and dynamic mechanisms play a key role in persistent haze events in Beijing during the cold period (Wu et al., 2017; Feng et al., 2014). Such events are associated with the high-pollution periods and will offer opportunities for chemical and physical transformation within the aerosol that lead to contravention of the requirement of receptor models for preservation of chemical profiles between source and receptor.
This study presents the outcomes of PMF performed on the combined dataset collected at two sites (IAP and PG) in the Beijing metropolitan area, including their comparison with source apportionment results from other approaches or based on different measurements. The PMF analysis resulted in the identification of seven sources: coal combustion, biomass burning, oil combustion, secondary inorganics, traffic emissions, road dust, and soil dust. These results were in good agreement with previously published source apportionment results made using PMF. However, factors that were resolved based on metal signatures were not fully resolved and indicate an internal mixing of different sources. In particular, soil dust, road dust, and some industrial sources have many elements in common and are very difficult to distinguish.
PMF results were compared with sources resolved from CMB and with PMF performed on other measurements (online AMS, offline AMS). Results showed broad agreement, with some notable exceptions. While this study provides some confirmatory evidence of PM2.5 source apportionment in Beijing, it highlights weaknesses of the PMF method when applied in this locality, and the results should be viewed in the context of studies using other methods such as CMB which appear able to give a more comprehensive view of the key sources affecting air quality. No industrial source profiles were used as inputs to the CMB model reported here, so CMB offers no further insights into possible contributions from industry.
Data supporting this publication are openly available from the UBIRA eData repository at https://doi.org/10.25500/edata.bham.00000721 (Harrison and Srivastava, 2021).
The supplement related to this article is available online at: https://doi.org/10.5194/acp-21-14703-2021-supplement.
This study was conceived by ZS and RMH. DS performed the PMF analysis and wrote the paper with the help of ZS and RMH. TVV and DL conducted the aerosol sampling and laboratory-based chemical analyses. XW and JX conducted the CMB modelling at the PG and IAP sites, respectively. All the authors discussed the paper and approved the final version for publication.
The authors declare that they have no conflict of interest.
Publisher's note: Copernicus Publications remains neutral with regard to jurisdictional claims in published maps and institutional affiliations.
This article is part of the special issue “In-depth study of air pollution sources and processes within Beijing and its surrounding region (APHH-Beijing) (ACP/AMT inter-journal SI)”. It is not associated with a conference.
This research has been supported by the Natural Environment Research Council (grant nos. NE/N007190/1 and NE/S006699/1).
This paper was edited by Mei Zheng and reviewed by two anonymous referees.
Amato, F. and Hopke, P. K.: Source apportionment of the ambient PM2.5 across St. Louis using constrained positive matrix factorization, Atmos. Environ., 46, 329–337, https://doi.org/10.1016/j.atmosenv.2011.09.062, 2012.
Amato, F., Pandolfi, M., Escrig, A., Querol, X., Alastuey, A., Pey, J., Perez, N., and Hopke, P. K.: Quantifying road dust resuspension in urban environment by Multilinear Engine: A comparison with PMF2, Atmos. Environ., 43, 2770–2780, https://doi.org/10.1016/j.atmosenv.2009.02.039, 2009.
Batterman, S., Xu, L., Chen, F., Chen, F., and Zhong, X.: Characteristics of PM(2.5) Concentrations across Beijing during 2013–2015, Atmos. Environ. (Oxford, England: 1994), 145, 104–114, https://doi.org/10.1016/j.atmosenv.2016.08.060, 2016.
Bi, X., Simoneit, B. R. T., Sheng, G., and Fu, J.: Characterization of molecular markers in smoke from residential coal combustion in China, Fuel, 87, 112–119, https://doi.org/10.1016/j.fuel.2007.03.047, 2008.
Boucher, O., Randall, D., Artaxo, P., Bretherton, C., Feingold, G., Forster, P., Kerminen, V.-M., Kondo, Y., Liao, H., and Lohmann, U.: Clouds and aerosols, in: Climate change 2013: the physical science basis, Contribution of Working Group I to the Fifth Assessment Report of the Intergovernmental Panel on Climate Change, Cambridge University Press, 571–657, 2013.
Cai, T., Zhang, Y., Fang, D., Shang, J., Zhang, Y., and Zhang, Y.: Chinese vehicle emissions characteristic testing with small sample size: Results and comparison, Atmos. Pollut. Res., 8, 154–163, https://doi.org/10.1016/j.apr.2016.08.007, 2017.
Canepari, S., Perrino, C., Olivieri, F., and Astolfi, M. L.: Characterisation of the traffic sources of PM through size-segregated sampling, sequential leaching and ICP analysis, Atmos. Environ., 42, 8161–8175, https://doi.org/10.1016/j.atmosenv.2008.07.052, 2008.
Cao, L., Zhu, Q., Huang, X., Deng, J., Chen, J., Hong, Y., Xu, L., and He, L.: Chemical characterization and source apportionment of atmospheric submicron particles on the western coast of Taiwan Strait, China, J. Environ. Sci., 52, 293–304, https://doi.org/10.1016/j.jes.2016.09.018, 2017.
Chan, Y., Simpson, R., McTainsh, G., Vowles, P., Cohen, D., and Bailey, G. J. A. E.: Characterisation of chemical species in PM2.5 and PM10 aerosols in Brisbane, Australia, Atmos. Environ., 31, 3773–3785, 1997.
Dall'Osto, M., Querol, X., Amato, F., Karanasiou, A., Lucarelli, F., Nava, S., Calzolai, G., and Chiari, M.: Hourly elemental concentrations in PM2.5 aerosols sampled simultaneously at urban background and road site during SAPUSS – diurnal variations and PMF receptor modelling, Atmos. Chem. Phys., 13, 4375–4392, https://doi.org/10.5194/acp-13-4375-2013, 2013.
Draxler, R.: Hysplit4 User's Guide, NOAA Tech. Memo. ERL ARL-230, 35 pp. available at: http://www.arl.noaa.gov/documents/reports/hysplit_user_guide.pdf (last access: 13 April 2020), 1999.
Duong, T. T. T. and Lee, B.-K.: Determining contamination level of heavy metals in road dust from busy traffic areas with different characteristics, J. Environ. Manage., 92, 554–562, https://doi.org/10.1016/j.jenvman.2010.09.010, 2011.
Duvall, R. M., Majestic, B. J., Shafer, M. M., Chuang, P. Y., Simoneit, B. R. T., and Schauer, J. J.: The water-soluble fraction of carbon, sulfur, and crustal elements in Asian aerosols and Asian soils, Atmos. Environ., 42, 5872–5884, https://doi.org/10.1016/j.atmosenv.2008.03.028, 2008.
Eglinton, G., Gonzalez, A. G., Hamilton, R. J., and Raphael, R. A.: Hydrocarbon constituents of the wax coatings of plant leaves: A taxonomic survey, Phytochemistry, 1, 89–102, https://doi.org/10.1016/S0031-9422(00)88006-1, 1962.
Feng, X., Li, Q., Zhu, Y., Wang, J., Liang, H., and Xu, R.: Formation and dominant factors of haze pollution over Beijing and its peripheral areas in winter, Atmos. Pollut. Res., 5, 528–538, https://doi.org/10.5094/APR.2014.062, 2014.
GBD MAPS Working Group: Burden of Disease Attributable to Coal-Burning and Other Major Sources of Air Pollution in China, Special Report 20, Health Effects Institute, Boston, MA, 2016.
Grigoratos, T. and Martini, G.: Brake wear particle emissions: a review, Environ. Sci. Pollut. Res., 22, 2491–2504, https://doi.org/10.1007/s11356-014-3696-8, 2015.
Gu, Y., Huang, R.-J., Li, Y., Duan, J., Chen, Q., Hu, W., Zheng, Y., Lin, C., Ni, H., Dai, W., Cao, J., Liu, Q., Chen, Y., Chen, C., Ovadnevaite, J., Ceburnis, D., and O'Dowd, C.: Chemical nature and sources of fine particles in urban Beijing: Seasonality and formation mechanisms, Environ. Int., 140, 105732, https://doi.org/10.1016/j.envint.2020.105732, 2020.
Guo, H., Zhou, J., Wang, L., Zhou, Y., Yuan, J., and Zhao, R.: Seasonal variations and sources of carboxylic acids in PM2.5 in Wuhan, China, Aerosol Air. Qual. Res., 15, 517–528, https://doi.org/10.4209/aaqr.2014.02.0040, 2015.
Harrison, R. M. and Srivastava, D.: Research data supporting “Insight into PM2.5 sources by applying Positive Matrix factorization (PMF) at an urban and rural site of Beijing”, University of Birmingham [data set], https://doi.org/10.25500/edata.bham.00000721, 2021.
Harrison, R. M., Vu, T. V., Jafar, H., and Shi, Z.: More mileage in reducing urban air pollution from road traffic, Environ. Int., 149, 106329, https://doi.org/10.1016/j.envint.2020.106329, 2021.
He, G., Pan, Y., and Tanaka, T.: The short-term impacts of COVID-19 lockdown on urban air pollution in China, Nature Sustainability, 3, 1005–1011, https://doi.org/10.1038/s41893-020-0581-y, 2020.
Heal, M. R., Kumar, P., and Harrison, R. M.: Particles, air quality, policy and health, Chem. Soc. Rev., 41, 6606–6630, 2012.
Henry, R., Norris, G. A., Vedantham, R., and Turner, J. R.: Source Region Identification Using Kernel Smoothing, Environ. Sci. Technol., 43, 4090–4097, https://doi.org/10.1021/es8011723, 2009.
Herrera Murillo, J., Campos Ramos, A., Ángeles García, F., Blanco Jiménez, S., Cárdenas, B., and Mizohata, A.: Chemical composition of PM2. 5 particles in Salamanca, Guanajuato Mexico: Source apportionment with receptor models, Atmos. Res., 107, 31–41, https://doi.org/10.1016/j.atmosres.2011.12.010, 2012.
Hopke, P. K.: Review of receptor modeling methods for source apportionment, J. Air Waste Manage. Assoc., 66, 237–259, https://doi.org/10.1080/10962247.2016.1140693, 2016.
Hu, W., Hu, M., Hu, W., Jimenez, J. L., Yuan, B., Chen, W., Wang, M., Wu, Y., Chen, C., Wang, Z., Peng, J., Zeng, L., and Shao, M.: Chemical composition, sources, and aging process of submicron aerosols in Beijing: Contrast between summer and winter, J. Geophys. Res.-Atmos., 121, 1955–1977, https://doi.org/10.1002/2015jd024020, 2016.
Huang, X., Tang, G., Zhang, J., Liu, B., Liu, C., Zhang, J., Cong, L., Cheng, M., Yan, G., Gao, W., Wang, Y., and Wang, Y.: Characteristics of PM2.5 pollution in Beijing after the improvement of air quality, J. Environ. Sci., 100, 1–10, https://doi.org/10.1016/j.jes.2020.06.004, 2021.
Huang, X.-F., He, L.-Y., Hu, M., Canagaratna, M. R., Sun, Y., Zhang, Q., Zhu, T., Xue, L., Zeng, L.-W., Liu, X.-G., Zhang, Y.-H., Jayne, J. T., Ng, N. L., and Worsnop, D. R.: Highly time-resolved chemical characterization of atmospheric submicron particles during 2008 Beijing Olympic Games using an Aerodyne High-Resolution Aerosol Mass Spectrometer, Atmos. Chem. Phys., 10, 8933–8945, https://doi.org/10.5194/acp-10-8933-2010, 2010.
Jaeckels, J. M., Bae, M.-S., and Schauer, J. J.: Positive matrix factorization (PMF) analysis of molecular marker measurements to quantify the sources of organic aerosols, Environ. Sci. Technol., 41, 5763–5769, 2007.
Ji, D., Li, L., Wang, Y., Zhang, J., Cheng, M., Sun, Y., Liu, Z., Wang, L., Tang, G., and Hu, B. J. A. E.: The heaviest particulate air-pollution episodes occurred in northern China in January, 2013: Insights gained from observation, Atmos. Environ., 92, 546–556, doi.org/10.1016/j.atmosenv.2014.04.048, 2014.
Jing, B., Wu, L., Mao, H., Gong, S., He, J., Zou, C., Song, G., Li, X., and Wu, Z.: Development of a vehicle emission inventory with high temporal–spatial resolution based on NRT traffic data and its impact on air pollution in Beijing – Part 1: Development and evaluation of vehicle emission inventory, Atmos. Chem. Phys., 16, 3161–3170, https://doi.org/10.5194/acp-16-3161-2016, 2016.
Kim, E.-A. and Koh, B.: Utilization of road dust chemical profiles for source identification and human health impact assessment, Sci. Rep., 10, 14259, https://doi.org/10.1038/s41598-020-71180-x, 2020.
Kolattukudy, P. E.: Chemistry and biochemistry of natural waxes, Elsevier Scientific Pub. Co., 1976.
Laing, J. R., Hopke, P. K., Hopke, E. F., Husain, L., Dutkiewicz, V. A., Paatero, J., and Viisanen, Y.: Positive Matrix Factorization of 47 Years of Particle Measurements in Finnish Arctic, Aerosol. Air Qual. Res., 15, 188–207 2015.
Le, T., Wang, Y., Liu, L., Yang, J., Yung, Y. L., Li, G., and Seinfeld, J. H.: Unexpected air pollution with marked emission reductions during the COVID-19 outbreak in China, Science, 369, 702–706, https://doi.org/10.1126/science.abb7431, 2020.
Lee, B.-K. and Hieu, N. T.: Seasonal variation and sources of heavy metals in atmospheric aerosols in a residential area of Ulsan, Korea, Aerosol Air Qual. Res., 11, 679–688, 2011.
Lee, S., Liu, W., Wang, Y., Russell, A. G., and Edgerton, E. S.: Source apportionment of PM2.5: Comparing PMF and CMB results for four ambient monitoring sites in the southeastern United States, Atmos. Environ., 42, 4126–4137, 2008a.
Lee, S., Kim, H. K., Yan, B., Cobb, C. E., Hennigan, C., Nichols, S., Chamber, M., Edgerton, E. S., Jansen, J. J., Hu, Y., Zheng, M., Weber, R. J., and Russell, A. G.: Diagnosis of Aged Prescribed Burning Plumes Impacting an Urban Area, Environ. Sci. Technol., 42, 1438–1444, https://doi.org/10.1021/es7023059, 2008b.
Li, D., Liu, J., Zhang, J., Gui, H., Du, P., Yu, T., Wang, J., Lu, Y., Liu, W., and Cheng, Y.: Identification of long-range transport pathways and potential sources of PM2.5 and PM10 in Beijing from 2014 to 2015, J. Environ. Sci., 56, 214–229, https://doi.org/10.1016/j.jes.2016.06.035, 2017.
Li, M., Liu, Z., Chen, J., Huang, X., Liu, J., Xie, Y., Hu, B., Xu, Z., Zhang, Y., and Wang, Y.: Characteristics and source apportionment of metallic elements in PM2.5 at urban and suburban sites in Beijing: Implication of emission reduction, Atmosphere, 10, 105, https://doi.org/10.3390/atmos10030105, 2019.
Lim, J.-M., Lee, J.-H., Moon, J.-H., Chung, Y.-S., and Kim, K.-H.: Source apportionment of PM10 at a small industrial area using Positive Matrix Factorization, Atmos. Res., 95, 88–100, https://doi.org/10.1016/j.atmosres.2009.08.009, 2010.
Liu, Y., Zheng, M., Yu, M., Cai, X., Du, H., Li, J., Zhou, T., Yan, C., Wang, X., Shi, Z., Harrison, R. M., Zhang, Q., and He, K.: High-time-resolution source apportionment of PM2.5 in Beijing with multiple models, Atmos. Chem. Phys., 19, 6595–6609, https://doi.org/10.5194/acp-19-6595-2019, 2019.
Lu, X., Yuan, D., Chen, Y., and Fung, J. C. H.: Impacts of urbanization and long-term meteorological variations on global PM2.5 and its associated health burden, Environ. Pollut., 270, 116003, https://doi.org/10.1016/j.envpol.2020.116003, 2021.
Ma, Q., Wu, Y., Tao, J., Xia, Y., Liu, X., Zhang, D., Han, Z., Zhang, X., and Zhang, R.: Variations of Chemical composition and source apportionment of PM2.5 during winter haze episodes in Beijing, Aerosol. Air Qual. Res., 17, 2791–2803, https://doi.org/10.4209/aaqr.2017.10.0366, 2017a.
Ma, Q., Wu, Y., Zhang, D., Wang, X., Xia, Y., Liu, X., Tian, P., Han, Z., Xia, X., Wang, Y., and Zhang, R.: Roles of regional transport and heterogeneous reactions in the PM2.5 increase during winter haze episodes in Beijing, Sci. Total Environ., 599–600, 246–253, https://doi.org/10.1016/j.scitotenv.2017.04.193, 2017b.
Mazzei, F., D'Alessandro, A., Lucarelli, F., Nava, S., Prati, P., Valli, G., and Vecchi, R.: Characterization of particulate matter sources in an urban environment, Sci. Total Environ., 401, 81–89, https://doi.org/10.1016/j.scitotenv.2008.03.008, 2008.
Niu, H., Hu, W., Zhang, D., Wu, Z., Guo, S., Pian, W., Cheng, W., and Hu, M.: Variations of fine particle physiochemical properties during a heavy haze episode in the winter of Beijing, Sci. Total Environ., 571, 103–109, https://doi.org/10.1016/j.scitotenv.2016.07.147, 2016.
Norris, G., Duvall, R., Brown, S., and Bai, S.: EPA Positive Matrix Factorization (PMF) 5.0 fundamentals and User Guide Prepared for the US Environmental Protection Agency Office of Research and Development, Washington, DC, DC EPA/600/R-14/108, 2014.
Oros, D. R. and Simoneit, B. R. T.: Identification and emission rates of molecular tracers in coal smoke particulate matter, Fuel, 79, 515–536, https://doi.org/10.1016/S0016-2361(99)00153-2, 2000.
Paatero, P.: Least squares formulation of robust non-negative factor analysis, Chemom. Intell. Lab. Syst., 37, 23–35, https://doi.org/10.1016/S0169-7439(96)00044-5, 1997.
Paatero, P. and Hopke, P. K.: Discarding or downweighting high-noise variables in factor analytic models, Anal. Chim. Acta, 490, 277–289, 2003.
Paatero, P. and Tapper, U.: Positive matrix factorization: A non-negative factor model with optimal utilization of error estimates of data values, Environmetrics, 5, 111–126, 1994.
Pant, P. and Harrison, R. M.: Critical review of receptor modelling for particulate matter: a case study of India, Atmos. Environ., 49, 1–12, 2012.
Pant, P. and Harrison, R. M.: Estimation of the contribution of road traffic emissions to particulate matter concentrations from field measurements: A review, Atmos. Environ., 77, 78–97, https://doi.org/10.1016/j.atmosenv.2013.04.028, 2013.
Pant, P., Shukla, A., Kohl, S. D., Chow, J. C., Watson, J. G., and Harrison, R. M.: Characterization of ambient PM2.5 at a pollution hotspot in New Delhi, India and inference of sources, Atmos. Environ., 109, 178–189, https://doi.org/10.1016/j.atmosenv.2015.02.074, 2015.
Paraskevopoulou, D., Liakakou, E., Gerasopoulos, E., Theodosi, C., and Mihalopoulos, N.: Long-term characterization of organic and elemental carbon in the PM2.5 fraction: the case of Athens, Greece, Atmos. Chem. Phys., 14, 13313–13325, https://doi.org/10.5194/acp-14-13313-2014, 2014.
Paulot, F., Paynter, D., Ginoux, P., Naik, V., Whitburn, S., Van Damme, M., Clarisse, L., Coheur, P.-F., and Horowitz, L. W.: Gas-aerosol partitioning of ammonia in biomass burning plumes: Implications for the interpretation of spaceborne observations of ammonia and the radiative forcing of ammonium nitrate, Geophys. Res. Lett., 44, 8084–8093, https://doi.org/10.1002/2017GL074215, 2017.
Petit, J. E., Favez, O., Albinet, A., and Canonaco, F.: A user-friendly tool for comprehensive evaluation of the geographical origins of atmospheric pollution: Wind and trajectory analyses, Environ. Modell. Softw., 88, 183–187, https://doi.org/10.1016/j.envsoft.2016.11.022, 2017.
Piscitello, A., Bianco, C., Casasso, A., and Sethi, R.: Non-exhaust traffic emissions: Sources, characterization, and mitigation measures, Sci. Total Environ., 766, 144440, https://doi.org/10.1016/j.scitotenv.2020.144440, 2021.
Polissar, A. V., Hopke, P. K., and Poirot, R. L.: Atmospheric aerosol over Vermont: chemical composition and sources, Environ. Sci. Technol., 35, 4604–4621, 2001.
Polissar, A. V., Hopke, P. K., Paatero, P., Malm, W. C., and Sisler, J. F.: Atmospheric aerosol over Alaska: 2. Elemental composition and sources, J. Geophys. Res.-Atmos., 103, 19045–19057, 1998.
Qiu, Y., Xie, Q., Wang, J., Xu, W., Li, L., Wang, Q., Zhao, J., Chen, Y., Chen, Y., Wu, Y., Du, W., Zhou, W., Lee, J., Zhao, C., Ge, X., Fu, P., Wang, Z., Worsnop, D. R., and Sun, Y.: Vertical Characterization and Source Apportionment of Water-Soluble Organic Aerosol with High-resolution Aerosol Mass Spectrometry in Beijing, China, ACS Earth Space Chem., 3, 273–284, https://doi.org/10.1021/acsearthspacechem.8b00155, 2019.
Querol, X., Zhuang, X., Alastuey, A., Viana, M., Lv, W., Wang, Y., López, A., Zhu, Z., Wei, H., and Xu, S.: Speciation and sources of atmospheric aerosols in a highly industrialised emerging mega-city in Central China, J. Environ. Monit., 8, 1049–1059, https://doi.org/10.1039/B608768J, 2006.
Rogge, W. F., Hildemann, L. M., Mazurek, M. A., Cass, G. R., and Simoneit, B. R. T.: Sources of fine organic aerosol. 4. Particulate abrasion products from leaf surfaces of urban plants, Environ. Sci. Technol., 27, 2700–2711, https://doi.org/10.1021/es00049a008, 1993.
Schembari, C., Bove, M. C., Cuccia, E., Cavalli, F., Hjorth, J., Massabò, D., Nava, S., Udisti, R., and Prati, P.: Source apportionment of PM10 in the Western Mediterranean based on observations from a cruise ship, Atmos. Environ., 98, 510–518, https://doi.org/10.1016/j.atmosenv.2014.09.015, 2014.
Shi, Z., Song, C., Liu, B., Lu, G., Xu, J., Van Vu, T., Elliott, R. J. R., Li, W., Bloss, W. J., and Harrison, R. M.: Abrupt but smaller than expected changes in surface air quality attributable to COVID-19 lockdowns, Sci. Adv., 7, eabd6696, https://doi.org/10.1126/sciadv.abd6696, 2021.
Shi, Z., Vu, T., Kotthaus, S., Harrison, R. M., Grimmond, S., Yue, S., Zhu, T., Lee, J., Han, Y., Demuzere, M., Dunmore, R. E., Ren, L., Liu, D., Wang, Y., Wild, O., Allan, J., Acton, W. J., Barlow, J., Barratt, B., Beddows, D., Bloss, W. J., Calzolai, G., Carruthers, D., Carslaw, D. C., Chan, Q., Chatzidiakou, L., Chen, Y., Crilley, L., Coe, H., Dai, T., Doherty, R., Duan, F., Fu, P., Ge, B., Ge, M., Guan, D., Hamilton, J. F., He, K., Heal, M., Heard, D., Hewitt, C. N., Hollaway, M., Hu, M., Ji, D., Jiang, X., Jones, R., Kalberer, M., Kelly, F. J., Kramer, L., Langford, B., Lin, C., Lewis, A. C., Li, J., Li, W., Liu, H., Liu, J., Loh, M., Lu, K., Lucarelli, F., Mann, G., McFiggans, G., Miller, M. R., Mills, G., Monk, P., Nemitz, E., O'Connor, F., Ouyang, B., Palmer, P. I., Percival, C., Popoola, O., Reeves, C., Rickard, A. R., Shao, L., Shi, G., Spracklen, D., Stevenson, D., Sun, Y., Sun, Z., Tao, S., Tong, S., Wang, Q., Wang, W., Wang, X., Wang, X., Wang, Z., Wei, L., Whalley, L., Wu, X., Wu, Z., Xie, P., Yang, F., Zhang, Q., Zhang, Y., Zhang, Y., and Zheng, M.: Introduction to the special issue “In-depth study of air pollution sources and processes within Beijing and its surrounding region (APHH-Beijing)”, Atmos. Chem. Phys., 19, 7519–7546, https://doi.org/10.5194/acp-19-7519-2019, 2019.
Shrivastava, M. K., Subramanian, R., Rogge, W. F., and Robinson, A. L.: Sources of organic aerosol: Positive matrix factorization of molecular marker data and comparison of results from different source apportionment models, Atmos. Environ., 41, 9353–9369, https://doi.org/10.1016/j.atmosenv.2007.09.016, 2007.
Simoneit, B. R.: A review of biomarker compounds as source indicators and tracers for air pollution, Environ. Sci. Pollut. Res., 6, 159–169, 1999.
Smichowski, P., Gómez, D., Frazzoli, C., and Caroli, S.: Traffic-Related Elements in Airborne Particulate Matter, Appl. Spectrosc. Rev., 43, 23–49, https://doi.org/10.1080/05704920701645886, 2007.
Song, Y., Tang, X., Xie, S., Zhang, Y., Wei, Y., Zhang, M., Zeng, L., and Lu, S.: Source apportionment of PM2.5 in Beijing in 2004, J. Hazard. Mater., 146, 124–130, https://doi.org/10.1016/j.jhazmat.2006.11.058, 2007.
Song, Y., Zhang, Y., Xie, S., Zeng, L., Zheng, M., Salmon, L. G., Shao, M., and Slanina, S.: Source apportionment of PM2.5 in Beijing by positive matrix factorization, Atmos. Environ., 40, 1526–1537, https://doi.org/10.1016/j.atmosenv.2005.10.039, 2006.
Sörme, L., Bergbäck, B., and Lohm, U.: Goods in the Anthroposphere as a Metal Emission Source A Case Study of Stockholm, Sweden, Water, Air Soil Poll., 1, 213–227, https://doi.org/10.1023/A:1017516523915, 2001.
Srimuruganandam, B. and Shiva Nagendra, S. M.: Application of positive matrix factorization in characterization of PM10 and PM2.5 emission sources at urban roadside, Chemosphere, 88, 120–130, https://doi.org/10.1016/j.chemosphere.2012.02.083, 2012.
Srivastava, D., Tomaz, S., Favez, O., Lanzafame, G. M., Golly, B., Besombes, J. L., Alleman, L. Y., Jaffrezo, J. L., Jacob, V., Perraudin, E., Villenave, E., and Albinet, A.: Speciation of organic fraction does matter for source apportionment. Part 1: A one-year campaign in Grenoble (France), Sci. Total Environ., 624, 1598–1611, https://doi.org/10.1016/j.scitotenv.2017.12.135, 2018.
Stein, A. F., Draxler, R. R., Rolph, G. D., Stunder, B. J. B., Cohen, M. D., and Ngan, F.: NOAA's HYSPLIT Atmospheric Transport and Dispersion Modeling System, B. Am. Meteorol. Soc., 96, 2059–2077, https://doi.org/10.1175/bams-d-14-00110.1, 2015.
Sun, J., Zhang, Q., Canagaratna, M. R., Zhang, Y., Ng, N. L., Sun, Y., Jayne, J. T., Zhang, X., Zhang, X., and Worsnop, D. R.: Highly time- and size-resolved characterization of submicron aerosol particles in Beijing using an Aerodyne Aerosol Mass Spectrometer, Atmos. Environ., 44, 131–140, https://doi.org/10.1016/j.atmosenv.2009.03.020, 2010.
Sun, Y., Zhuang, G., Tang, A., Wang, Y., and An, Z.: Chemical Characteristics of PM2.5 and PM10 in Haze-Fog Episodes in Beijing, Environ. Sci. Technol., 40, 3148–3155, https://doi.org/10.1021/es051533g, 2006.
Sun, Y., Zhuang, G., Wang, Y., Zhao, X., Li, J., Wang, Z., and An, Z.: Chemical composition of dust storms in Beijing and implications for the mixing of mineral aerosol with pollution aerosol on the pathway, J. Geophys. Chem., 110, D24209, https://doi.org/10.1029/2005jd006054, 2005.
Sun, Y., Jiang, Q., Wang, Z., Fu, P., Li, J., Yang, T., and Yin, Y.: Investigation of the sources and evolution processes of severe haze pollution in Beijing in January 2013, 119, 4380–4398, https://doi.org/10.1002/2014JD021641, 2014.
Sun, Y., Du, W., Fu, P., Wang, Q., Li, J., Ge, X., Zhang, Q., Zhu, C., Ren, L., Xu, W., Zhao, J., Han, T., Worsnop, D. R., and Wang, Z.: Primary and secondary aerosols in Beijing in winter: sources, variations and processes, Atmos. Chem. Phys., 16, 8309–8329, https://doi.org/10.5194/acp-16-8309-2016, 2016.
Sun, Y., He, Y., Kuang, Y., Xu, W., Song, S., Ma, N., Tao, J., Cheng, P., Wu, C., Su, H., Cheng, Y., Xie, C., Chen, C., Lei, L., Qiu, Y., Fu, P., Croteau, P., and Worsnop, D. R.: Chemical differences between PM1 and PM2.5 in Highly polluted environment and implications in air pollution studies, Geophys. Res. Lett., 47, e2019GL086288, https://doi.org/10.1029/2019GL086288, 2020.
Sun, Y. L., Wang, Z. F., Fu, P. Q., Yang, T., Jiang, Q., Dong, H. B., Li, J., and Jia, J. J.: Aerosol composition, sources and processes during wintertime in Beijing, China, Atmos. Chem. Phys., 13, 4577–4592, https://doi.org/10.5194/acp-13-4577-2013, 2013.
Swietlicki, E. and Krejci, R.: Source characterisation of the Central European atmospheric aerosol using multivariate statistical methods, Nucl. Instrum. Methods Phys. Res., 109–110, 519–525, https://doi.org/10.1016/0168-583X(95)01220-6, 1996.
Takuwa, T., Mkilaha, I. S. N., and Naruse, I.: Mechanisms of fine particulates formation with alkali metal compounds during coal combustion, Fuel, 85, 671–678, 2006.
Tao, S., Ru, M. Y., Du, W., Zhu, X., Zhong, Q. R., Li, B. G., Shen, G. F., Pan, X. L., Meng, W. J., Chen, Y. L., Shen, H. Z., Lin, N., Su, S., Zhuo, S. J., Huang, T. B., Xu, Y., Yun, X., Liu, J. F., Wang, X. L., Liu, W. X., Chen, H. F., and Zhu, D. Q.: Quantifying the Rural Residential Energy Transition in China from 1992 to 2012 through a Representative National Survey, Nat. Energy, 3, 567–573, 2018.
Thorpe, A. and Harrison, R. M.: Sources and properties of non-exhaust particulate matter from road traffic: A review, Sci. Total Environ., 400, 270–282, https://doi.org/10.1016/j.scitotenv.2008.06.007, 2008.
Tian, S. L., Pan, Y. P., and Wang, Y. S.: Size-resolved source apportionment of particulate matter in urban Beijing during haze and non-haze episodes, Atmos. Chem. Phys., 16, 1–19, https://doi.org/10.5194/acp-16-1-2016, 2016.
Tie, X., Huang, R.-J., Cao, J., Zhang, Q., Cheng, Y., Su, H., Chang, D., Pöschl, U., Hoffmann, T., Dusek, U., Li, G., Worsnop, D. R., and O'Dowd, C. D.: Severe Pollution in China Amplified by Atmospheric Moisture, Sci. Rep., 7, 15760–15760, https://doi.org/10.1038/s41598-017-15909-1, 2017.
Vejahati, F., Xu, Z., and Gupta, R.: Trace elements in coal: Associations with coal and minerals and their behavior during coal utilization – A review, Fuel, 89, 904–911, https://doi.org/10.1016/j.fuel.2009.06.013, 2010.
Viana, M., Kuhlbusch, T. A. J., Querol, X., Alastuey, A., Harrison, R. M., Hopke, P. K., Winiwarter, W., Vallius, M., Szidat, S., Prévôt, A. S. H., Hueglin, C., Bloemen, H., Wåhlin, P., Vecchi, R., Miranda, A. I., Kasper-Giebl, A., Maenhaut, W., and Hitzenberger, R.: Source apportionment of particulate matter in Europe: A review of methods and results, J. Aerosol Sci., 39, 827–849, https://doi.org/10.1016/j.jaerosci.2008.05.007, 2008.
Vu, T. V., Shi, Z., Cheng, J., Zhang, Q., He, K., Wang, S., and Harrison, R. M.: Assessing the impact of clean air action on air quality trends in Beijing using a machine learning technique, Atmos. Chem. Phys., 19, 11303–11314, https://doi.org/10.5194/acp-19-11303-2019, 2019.
Waked, A., Favez, O., Alleman, L. Y., Piot, C., Petit, J.-E., Delaunay, T., Verlinden, E., Golly, B., Besombes, J.-L., Jaffrezo, J.-L., and Leoz-Garziandia, E.: Source apportionment of PM10 in a north-western Europe regional urban background site (Lens, France) using positive matrix factorization and including primary biogenic emissions, Atmos. Chem. Phys., 14, 3325–3346, https://doi.org/10.5194/acp-14-3325-2014, 2014.
Wang, G., Gu, S., Chen, J., Wu, X., and Yu, J.: Assessment of health and economic effects by PM2.5 pollution in Beijing: a combined exposure–response and computable general equilibrium analysis, Environ. Technol., 37, 3131–3138, https://doi.org/10.1080/09593330.2016.1178332, 2016.
Wang, H., Zhuang, Y., Wang, Y., Sun, Y., Yuan, H., Zhuang, G., and Hao, Z.: Long-term monitoring and source apportionment of PM2.5/PM10 in Beijing, China, J. Environ. Sci., 20, 1323–1327, https://doi.org/10.1016/S1001-0742(08)62228-7, 2008.
Wang, L., Zhang, N., Liu, Z., Sun, Y., Ji, D., and Wang, Y.: The Influence of Climate Factors, Meteorological Conditions, and boundary-layer structure on severe haze pollution in the Beijing-Tianjin-Hebei region during January 2013, Adv. Meteorol., 2014, 685971, https://doi.org/10.1155/2014/685971, 2014.
Wang, Q., Shao, M., Zhang, Y., Wei, Y., Hu, M., and Guo, S.: Source apportionment of fine organic aerosols in Beijing, Atmos. Chem. Phys., 9, 8573–8585, https://doi.org/10.5194/acp-9-8573-2009, 2009.
Wang, Y., Hopke, P. K., Xia, X., Rattigan, O. V., Chalupa, D. C., and Utell, M. J.: Source apportionment of airborne particulate matter using inorganic and organic species as tracers, Atmos. Environ., 55, 525–532, 2012.
Watson, J. G., Robinson, N. F., Chow, J. C., Henry, R. C., Kim, B., Pace, T., Meyer, E. L., and Nguyen, Q.: The USEPA/DRI chemical mass balance receptor model, CMB 7.0, Environ. Soft., 5, 38–49, 1990.
Wu, P., Ding, Y., and Liu, Y.: Atmospheric circulation and dynamic mechanism for persistent haze events in the Beijing–Tianjin–Hebei region, Adv. Atmos. Sci., 34, 429–440, 2017.
Wu, X., Chen, C., Vu, T. V., Liu, D., Baldo, C., Shen, X., Zhang, Q., Cen, K., Zheng, M., He, K., Shi, Z., and Harrison, R. M.: Source apportionment of fine organic carbon (OC) using receptor modelling at a rural site of Beijing: Insight into seasonal and diurnal variation of source contributions, Environ. Pollut., 115078, https://doi.org/10.1016/j.envpol.2020.115078, 2020.
Xie, Y., Dai, H., Zhang, Y., Wu, Y., Hanaoka, T., and Masui, T.: Comparison of health and economic impacts of PM2.5 and ozone pollution in China, Environ. Int., 130, 104881, https://doi.org/10.1016/j.envint.2019.05.075, 2019.
Xing, Y.-F., Xu, Y.-H., Shi, M.-H., and Lian, Y.-X.: The impact of PM2.5 on the human respiratory system, J. Thorac. Dis., 8, E69–E74, https://doi.org/10.3978/j.issn.2072-1439.2016.01.19, 2016.
Xu, J., Liu, D., Wu, X., Vu, T. V., Zhang, Y., Fu, P., Sun, Y., Xu, W., Zheng, B., Harrison, R. M., and Shi, Z.: Source apportionment of fine organic carbon at an urban site of Beijing using a chemical mass balance model, Atmos. Chem. Phys., 21, 7321–7341, https://doi.org/10.5194/acp-21-7321-2021, 2021.
Xu, W., Sun, Y., Wang, Q., Zhao, J., Wang, J., Ge, X., Xie, C., Zhou, W., Du, W., Li, J., Fu, P., Wang, Z., Worsnop, D. R., and Coe, H.: Changes in aerosol chemistry from 2014 to 2016 in winter in Beijing: Insights from high-resolution aerosol mass spectrometry, J. Geophys. Res.-Atmos., 124, 1132–1147, https://doi.org/10.1029/2018JD029245, 2019.
Yan, C., Zheng, M., Sullivan, A. P., Shen, G., Chen, Y., Wang, S., Zhao, B., Cai, S., Desyaterik, Y., Li, X., Zhou, T., Gustafsson, Ö., and Collett, J. L.: Residential coal combustion as a source of levoglucosan in China, Environ. Sci. Technol., 52, 1665–1674, https://doi.org/10.1021/acs.est.7b05858, 2018.
Yu, L., Wang, G., Zhang, R., Zhang, L., Song, Y., Wu, B., Li, X., An, K., and Chu, J.: Characterization and source apportionment of PM2.5 in an urban environment in Beijing, Aerosol Air Qual. Res., 13, 574–583, https://doi.org/10.4209/aaqr.2012.07.0192, 2013.
Zhang, J., Wang, Y., Huang, X., Liu, Z., Ji, D., and Sun, Y.: Characterization of organic aerosols in Beijing using an aerodyne high-resolution aerosol mass spectrometer, Adv. Atmos. Sci., 32, 877–888, https://doi.org/10.1007/s00376-014-4153-9, 2015.
Zhang, J.-J., Cui, M.-M., Fan, D., Zhang, D.-S., Lian, H.-X., Yin, Z.-Y., and Li, J.: Relationship between haze and acute cardiovascular, cerebrovascular, and respiratory diseases in Beijing, Environ. Sci. Pollut. Res., 22, 3920–3925, https://doi.org/10.1007/s11356-014-3644-7, 2015.
Zhang, J. K., Sun, Y., Liu, Z. R., Ji, D. S., Hu, B., Liu, Q., and Wang, Y. S.: Characterization of submicron aerosols during a month of serious pollution in Beijing, 2013, Atmos. Chem. Phys., 14, 2887–2903, https://doi.org/10.5194/acp-14-2887-2014, 2014.
Zhang, J. K., Cheng, M. T., Ji, D. S., Liu, Z. R., Hu, B., Sun, Y., and Wang, Y. S.: Characterization of submicron particles during biomass burning and coal combustion periods in Beijing, China, Sci. Total Environ., 562, 812–821, https://doi.org/10.1016/j.scitotenv.2016.04.015, 2016.
Zhang, R., Jing, J., Tao, J., Hsu, S.-C., Wang, G., Cao, J., Lee, C. S. L., Zhu, L., Chen, Z., Zhao, Y., and Shen, Z.: Chemical characterization and source apportionment of PM2.5 in Beijing: seasonal perspective, Atmos. Chem. Phys., 13, 7053–7074, https://doi.org/10.5194/acp-13-7053-2013, 2013.
Zhang, Q., Jimenez, J. L., Canagaratna, M. R., Ulbrich, I. M., Ng, N. L., Worsnop, D. R., and Sun, Y.: Understanding atmospheric organic aerosols via factor analysis of aerosol mass spectrometry: a review, Anal. Bioanal. Chem., 401, 3045–3067, 2011.
Zhang, X., Hecobian, A., Zheng, M., Frank, N. H., and Weber, R. J.: Biomass burning impact on PM2.5 over the southeastern US during 2007: integrating chemically speciated FRM filter measurements, MODIS fire counts and PMF analysis, Atmos. Chem. Phys., 10, 6839–6853, https://doi.org/10.5194/acp-10-6839-2010, 2010.
Zhang, Y., Sheesley, R. J., Schauer, J. J., Lewandowski, M., Jaoui, M., Offenberg, J. H., Kleindienst, T. E., and Edney, E. O.: Source apportionment of primary and secondary organic aerosols using positive matrix factorization (PMF) of molecular markers, Atmos. Environ., 43, 5567–5574, 2009.
Zhang, Y., Schauer, J. J., Zhang, Y., Zeng, L., Wei, Y., Liu, Y., and Shao, M.: Characteristics of particulate carbon emissions from real-world Chinese coal combustion, Environ. Sci. Technol., 42, 5068–5073, https://doi.org/10.1021/es7022576, 2008.
Zhang, Y., Ren, H., Sun, Y., Cao, F., Chang, Y., Liu, S., Lee, X., Agrios, K., Kawamura, K., Liu, D., Ren, L., Du, W., Wang, Z., Prévôt, A. S. H., Szidat, S., and Fu, P.: High contribution of nonfossil sources to submicrometer organic aerosols in Beijing, China, Environ. Sci. Technol., 51, 7842–7852, https://doi.org/10.1021/acs.est.7b01517, 2017.
Zhang, Y.-X., Min, S., Zhang, Y.-H., Zeng, L.-M., He, L.-Y., Bin, Z., Wei, Y.-J., and Zhu, X.-L.: Source profiles of particulate organic matters emitted from cereal straw burnings, J. Environ. Sci., 19, 167–175, 2007.
Zhao, X., Hu, Q., Wang, X., Ding, X., He, Q., Zhang, Z., Shen, R., Lü, S., Liu, T., Fu, X., and Chen, L.: Composition profiles of organic aerosols from Chinese residential cooking: case study in urban Guangzhou, south China, J. Atmos. Chem., 72, 1–18, https://doi.org/10.1007/s10874-015-9298-0, 2015.
Zhao, Z.-Y., Cao, F., Fan, M.-Y., Zhang, W.-Q., Zhai, X.-Y., Wang, Q., and Zhang, Y.-L.: Coal and biomass burning as major emissions of NOX in Northeast China: Implication from dual isotopes analysis of fine nitrate aerosols, Atmos. Environ., 242, 117762, https://doi.org/10.1016/j.atmosenv.2020.117762, 2020.
Zheng, M., Salmon, L. G., Schauer, J. J., Zeng, L., Kiang, C. S., Zhang, Y., and Cass, G. R.: Seasonal trends in PM2.5 source contributions in Beijing, China, Atmos. Environ., 39, 3967–3976, https://doi.org/10.1016/j.atmosenv.2005.03.036, 2005.
Zhou, Y., Zheng, N., Luo, L., Zhao, J., Qu, L., Guan, H., Xiao, H., Zhang, Z., Tian, J., and Xiao, H.: Biomass burning related ammonia emissions promoted a self-amplifying loop in the urban environment in Kunming (SW China), Atmos. Environ., 253, 118138, https://doi.org/10.1016/j.atmosenv.2020.118138, 2020.