the Creative Commons Attribution 4.0 License.
the Creative Commons Attribution 4.0 License.
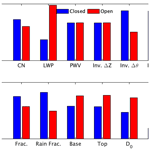
Contrasting characteristics of open- and closed-cellular stratocumulus cloud in the eastern North Atlantic
Virendra P. Ghate
Diana K. Apoznanski
Mary J. Bartholomew
Scott E. Giangrande
Karen L. Johnson
Mandana M. Thieman
Extensive regions of marine boundary layer cloud impact the radiative balance through their significant shortwave albedo while having little impact on outgoing longwave radiation. Despite this importance, these cloud systems remain poorly represented in large-scale models due to difficulty in representing the processes that drive their life cycle and coverage. In particular, the mesoscale organization and cellular structure of marine boundary clouds have important implications for the subsequent cloud feedbacks. In this study, we use long-term (2013–2018) observations from the Atmospheric Radiation Measurement (ARM) Facility's Eastern North Atlantic (ENA) site on Graciosa Island, Azores, Portugal, to identify cloud cases with open- or closed-cellular organization. More than 500 h of each organization type are identified. The ARM observations are combined with reanalysis and satellite products to quantify the cloud, precipitation, aerosol, thermodynamic, and large-scale synoptic characteristics associated with these cloud types. Our analysis shows that both cloud organization populations occur during similar sea surface temperature conditions, but the open-cell cases are distinguished by stronger cold-air advection and large-scale subsidence compared to the closed-cell cases, consistent with their formation during cold-air outbreaks. We also find that the open-cell cases were associated with deeper boundary layers, stronger low-level winds, and higher rain rates compared to their closed-cell counterparts. Finally, raindrops with diameters larger than 1 mm were routinely recorded at the surface during both populations, with a higher number of large drops during the open-cellular cases. The similarities and differences noted herein provide important insights into the environmental and cloud characteristics during varying marine boundary layer cloud mesoscale organization and will be useful for the evaluation of model simulations for ENA marine clouds.
- Article
(6457 KB) - Full-text XML
- BibTeX
- EndNote
This paper has been authored by employees (Michael P. Jensen, Dié Wang, Mary J. Bartholomew, Scott E. Giangrande, Karen L. Johnson) of Brookhaven Science Associates, LLC under contract with the U.S. Department of Energy. The United States Government retains and the publisher, by accepting the article for publication, acknowledges that the United States Government retains a non-exclusive, paid-up, irrevocable, world-wide license to publish or reproduce the published form of this paper, or allow others to do so, for United States Government purposes.
It is well established that a small increase in the global coverage of marine boundary layer (MBL) stratocumulus clouds could offset warming associated with a doubling of CO2 (Hartmann and Short, 1980; Randall et al., 1984; Slingo, 1990). This is because the albedo of MBL clouds is much larger than that of the underlying ocean, generally causing a significant decrease in the amount of solar radiation absorbed in the ocean's mixed layer, with a minimal difference in thermal radiation emitted to space. This large radiative impact coupled with their large areal coverage makes marine stratocumulus clouds an important component of the global energy balance. Bony and Dufresne (2005) have shown that the simulation and response to the changing climate of MBL stratocumulus clouds represents the main source of uncertainty in cloud feedbacks simulated by Earth system models used for predicting the future climate.
MBL stratocumulus clouds are intimately coupled to the turbulence in the boundary layer that is modulated primarily by cloud top radiative cooling, entrainment, precipitation, and surface turbulent fluxes (Wood, 2012). These clouds are known to occur in two distinct mesoscale (20–200 km) organizations known as closed-cellular (unbroken) and open-cellular (broken) stratocumulus (Wood and Hartmann, 2006). These differing modes organize the internal diabatic forcings within the MBL, impacting the low-level cloud fraction, shortwave albedo, and liquid water path driving the localized contribution to the radiative energy balance and water cycle (e.g., Rossow et al., 2002; Savic-Jovcic and Stevens, 2008; Wood et al., 2008, 2016). The key processes responsible for these organizing states have been the subject of much research over the past several decades with the interaction among precipitation and boundary layer dynamics identified as a main driver (e.g., Bretherton et al., 2004; Comstock et al., 2005, 2007; Sharon et al., 2006; Stevens et al., 2005; Savic-Jovcic and Stevens, 2008; Wang and Feingold, 2009 ; Feingold et al., 2010). Aerosols, through their influences on the formation and suppression of drizzle, have also been found to have important implications on MBL cloud organization (e.g., van Zanten and Stevens, 2005; Petters et al., 2006; Mechem et al., 2012; Sharon et al., 2006; Wood et al., 2008; Xue et al., 2008; Wang and Feingold, 2009; Bretherton et al., 2010; Wood et al., 2011). The interplay of these different mechanisms and their relative importance under different regimes remains an area of active research and a needed target for improved understanding and representation in large-scale atmospheric models (Wood et al., 2016; Jensen et al., 2016b).
A number of studies based on observational analyses of field campaign data have investigated the characteristics of cellular MBL cloud fields aiming to define the processes responsible for the organization. Using aircraft observations off the coast of California, Sharon et al. (2006) and Stevens et al. (2005) observed much higher drizzle rates in pockets of open-cellular clouds compared to nearby closed-cellular clouds, hypothesizing that this precipitation was a driving force of organization. Comstock et al. (2005, 2007), using shipboard observations from the East Pacific Investigation of Climate (EPIC) 2001 field campaign (Bretherton et al., 2004), found significantly higher drizzle rates in open-cellular clouds, but they also found that open-cell stratocumulus were associated with deeper, thermodynamically decoupled boundary layers compared to closed-cell stratocumulus. Several studies using observations from the VAMOS Ocean-Cloud-Atmosphere-Land Study (VOCALS) field campaign (Wood et al., 2011) reported that cold pools were common in both open- and closed-cell stratocumulus cloud fields; however, drizzle was stronger and accumulation-mode aerosol concentrations were much lower during open-cellular conditions (Ghate et al., 2013; Wood et al., 2011; Terai and Wood, 2013; Wilbanks et al., 2015). However, Wood et al. (2011) and Terai et al. (2013) found that drizzle rates are not significantly different between open and closed cells, concluding that drizzle, and its associated thermodynamic feedbacks, is not the only factor causing the transition between mesoscale organizations.
The driving mechanisms for changes in the organization of MBL stratocumulus clouds have also been the focus for a number of modeling studies over recent decades. Shao and Randall (1996) perform simulations incorporating increasing complexity of the model analysis for closed-cellular MBL clouds. They found that cloud-top radiative cooling is a primary driver of the boundary layer dynamics and cloud organization. Feingold et al. (2010) combine satellite observations with numerical simulations to show the role of collisions between precipitation-generated cold pools in driving the characteristics of open-cellular cloud organizations and their oscillation between different, weakly stable states. While many studies have noted the importance of drizzle in the transition between organizational cloud states, Yamaguchi and Feingold (2014), through idealized three-dimensional simulations, highlight the importance of the spatial distribution of the precipitation. Feingold et al. (2015) perform a series of idealized cloud-resolving model simulations to investigate the two-way transitions between open- and closed-cellular cloud populations. Their findings reiterate the importance of precipitation in the transition from the closed- to open-cellular states and emphasize that stabilization of the boundary layer due to this precipitation and increased longwave cooling acts as a barrier to cloud formation and recovery to closed-cellular cloud organization.
Within the large decks of marine stratocumulus clouds observed over the eastern subtropical oceans, the transition from closed to open cellular is routinely observed with little diurnal variability (Burleysen and Yuter, 2015) or changes in the large-scale (inversion strength, subsidence, sea surface temperature (SST), etc.) conditions (Muhlbaur et al., 2014). Although the eastern North Atlantic (ENA) broadly falls under the subsiding branch of the Hadley circulation, forcing a boundary layer inversion over a warmer sea surface that leads to shallow convection, it also routinely experiences cold-air outbreaks (McCoy et al., 2017; Lamraoui et al., 2019; Ghate et al., 2020). These cold-air outbreaks also contain open-cellular marine stratocumulus with the transition from the closed- to open-cellular cloud organization happening farther north. Although stratocumulus organized in closed- and open-cellular patterns are routinely observed, the differences between their cloud and environmental characteristics have not been quantified from long-term observations.
In this study, we use observations collected at the Atmospheric Radiation Measurement's (ARM's) ENA site to characterize the aerosol, cloud, precipitation, and thermodynamic conditions during closed- and open-cellular stratocumulus conditions observed in the region. The data and instrumentation at the ENA site are described in Sect. 2. Section 3 describes the methodology for selecting closed- and open-cellular cloud cases. In Sect. 4, we compare and contrast the cloud and environmental characteristics associated with each cloud population. The article is concluded with a summary and discussion in Sect. 5.
The U.S. Department of Energy's ARM (https://www.arm.gov, last access: 20 January 2021) user facility operates a number of surface-based measurement sites around the globe (e.g., Mather and Voyles, 2013). Each of these sites includes a comprehensive suite of instrumentation for continuous remote sensing and in situ measurements of cloud, aerosol, radiation, atmospheric state, and precipitation. Following a successful 2-year deployment of an ARM Mobile Facility (Miller et al., 2016) at Graciosa Island, Azores, Portugal (Wood et al., 2015), the ARM ENA long-term fixed site was established at this location (Fig. 1, 39.09∘ N, 28.03∘ W, 15 m) in October 2013.
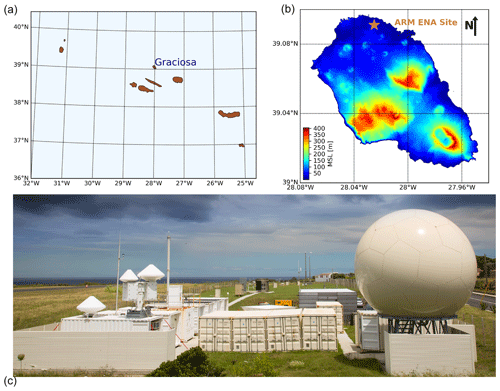
Figure 1(a) Map showing the location of the Azores and Graciosa Island, (b) elevation map of Graciosa Island showing the location of the ARM ENA site on the northern coastline, and (c) photo of the ARM ENA site courtesy of the U.S. Department of Energy ARM user facility.
For this study, we use active and passive remote sensing and in situ observations from the ARM site, combined with satellite observations and reanalysis products to quantify the cloud and environmental characteristics associated with both closed- and open-cellular cloud organization. The Ka-band ARM Zenith Radar (KAZR; Kollias et al., 2007, 2016; Widener et al., 2012) collects profiles of raw Doppler spectra and its first three moments at 2 s and 30 m resolution in co- and cross-polarization modes. Collocated with the KAZR is a ceilometer that records the first three optical cloud base heights at 15 s and 30 m resolution, a micro-pulse lidar (MPL) that records raw backscatter at 7 s and 30 m resolution, and a microwave radiometer (MWR; Morris, 2019; Cadeddu et al., 2013) that records brightness temperatures at 23, 32, and 90 GHz frequencies. The data from the KAZR, MPL, MWR, and ceilometer are combined in the Active Remote Sensing of CLouds (ARSCL) value-added product (ARM, 2015) to produce profiles of noise-filtered moments of Doppler spectra and cloud boundaries (Clothiaux et al., 2000, 2001; Kollias et al., 2005, 2016). The data from the MWR were also used to retrieve column integrated values of liquid water path (LWP) and precipitable water vapor (PWV) at 20 s temporal resolution (ARM, 2014a; Gaustad et al., 2011; Turner et al., 2007). MWR measurements that are flagged as being influenced by rain or PWV measurements that lie beyond 2 standard deviations of the daily mean are removed. Balloon-borne radiosondes are launched twice daily at the site (00:00 and 12:00 UTC) using a Vaisala RS-92, or more recently RS-41, radiosonde (Holdridge et al., 2011; Jensen et al., 2016a) and report profiles of temperature, humidity, pressure, and winds (ARM, 2013). The lower tropospheric stability (LTS), quantified as the difference between the potential temperature at 700 hPa and that at the surface (Klein and Hartmann, 1993), is also calculated using the radiosonde data. Surface raindrop size distributions (DSDs) are measured by a two-dimensional video disdrometer (2DVD; e.g., Kruger and Krajewski, 2002; Bartholomew, 2017; ARM, 2014b). For improved data quality, we apply a fall speed correction and drop size and number thresholds. The DSD parameters are calculated using open-source PyDSD code (Hardin and Guy, 2017). More detailed information regarding the disdrometer processing is described in Wang et al. (2018) and Giangrande et al. (2019). Also present at the site is a surface meteorological station that reports temperature, pressure, humidity, and winds at a 1 min temporal resolution. From the plethora of aerosol instrumentation present at the ENA site, we used the data collected by the condensation particle counter (CPC) that measures the total concentration of aerosol with diameters between 3 and 3000 nm. Visible imagery (0.65 µm) and associated data products available at 30–60 min temporal and 9 km spatial resolution from the Spinning Enhanced Visible and Infrared Imager (SEVIRI) on board the Meteosat-11 satellite were used to characterize cloud conditions around the site. These data were obtained from the Satellite ClOud and Radiation Property retrieval System (SatCORPS) team and NASA Langley Research Center (NASA, 2019a, b).
The data from the National Centers for Environmental Prediction (NCEP) reanalysis available at 2∘ spatial and 6 h temporal resolution was used to characterize the large-scale environmental conditions at the site (Kalnay et al., 1996). Primarily the SST, horizontal wind speed and direction at the surface, and large-scale subsidence as reported by NCEP reanalysis were retrieved. The reanalysis data were also used to force the Hybrid Single Particle Lagrangian Integrated Trajectory (HYSPLIT; Stein et al., 2015; Rolph et al., 2017) model to deduce the location of air parcels before reaching the site. Due to high uncertainty in the reanalysis-reported large-scale vertical air motion, the HYSPLIT simulations were performed at 500 m height assuming isobaric vertical air motion.
The mesoscale cellularity of marine stratocumulus clouds has been previously identified using data from polar orbiting (e.g., Wood and Hartmann, 2006; Jensen et al., 2008; Zhang et al., 2010) and geostationary satellites (Burleysen and Yuter, 2015), through analysis of either retrieved estimates of LWP or cloud top temperatures. As the goal of this study is not to quantify the cellularity but rather to determine the differences between the cloud and environmental characteristics associated with the two mesoscale organizations, we use a more traditional approach of combining data from ground-based and satellite data to select closed- and open-cellular cloud organization cases via visual inspection. To minimize the potential island influences on the observed boundary layer and cloud properties, since the site is located at the northern edge of the island, we focus on cases with dominant boundary layer wind directions from the northern half of the wind rose (e.g., Miller et al., 1998; Rémillard and Tselioudis, 2015). Days that were mostly overcast (minimum 12 h), had relatively uniform (within 500 m) cloud top heights, and had only a single cloud layer below 4 km were selected as closed-cellular cloud organization cases. The SEVIRI images were used to ensure uniform cloud cover within 2∘ north of the ENA site. Days that had relatively uniform cloud top heights of heavily precipitating stratocumulus clouds, had shallow cumulus clouds underneath the precipitating stratocumulus, and had noticeable cellular structure encompassing clear areas (identified from the satellite imagery) with stratocumulus cloud cover lower than 100 % were chosen for open-cellular cloud organization cases. Additionally, cases with any mid-level clouds (4–7 km), that lasted less than 12 h, or were missing any of the ground-based measurements listed above were discarded. In Figs. 2 and 3, we show illustrative examples of the time–height KAZR reflectivity observations and the SEVIRI visible satellite imagery for a closed-cellular and an open-cellular cloud organization case, respectively. Using these criteria, 26 closed-cellular cases (588 h) and 24 open-cellular cases (536 h) were identified from the data collected from October 2015 to December 2018. The list of cases for both open- and closed-cellular stratocumulus clouds is included in Table 1.
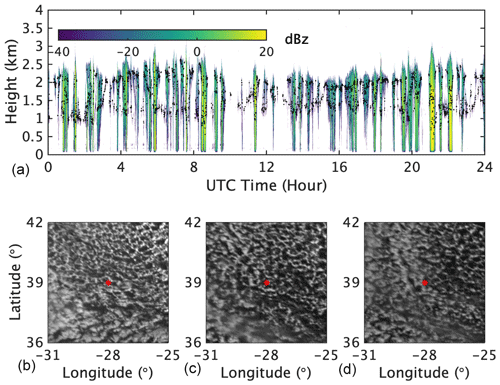
Figure 2Example of open cells from 22 October 2016. (a) Time–height profile of the best estimate radar reflectivity from the ARSCL value-added product. (b) Reflectance at 0.65 µm wavelength from Meteosat observations.
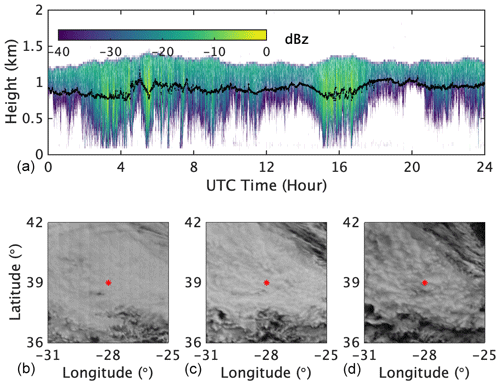
Figure 3Example of closed cells from 4 March 2016. (a) Time–height profile of the best estimate radar reflectivity from the ARSCL value-added product. (b) Reflectance at 0.65 µm wavelength from Meteosat observations.
Table 1List of days and hours if not the whole day, with closed- and open-cellular mesoscale organization at the ARM ENA site.
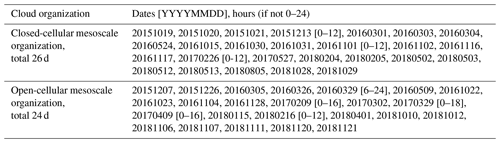
The annual cycle of the synoptic and cloud conditions at the Azores is driven by the interplay of the midlatitude storm track and the subtropical Azorean high-pressure system. During the boreal winter months (December–January–February), the southern displacement of the midlatitude storm track drives an increase in deeper, storm-related cloud systems, and subsidence associated with cold-air outbreaks drives the formation of boundary layer cloudiness (Rémillard and Tselioudis, 2015). During the boreal summer months (June–July–August) subsidence associated with the subtropical Azores high-pressure system drives a maximum in stratocumulus cloud occurrence (Rémillard and Tselioudis, 2015). Most of the cases for both cloud organizations were observed during the transition months, 12 (11) and 9 (8) closed- (open-) cellular cloud organization during September–October–November and March–April–May, respectively (Table 2). There was only one case of closed-cellular and none of open-cellular cloud organization during the summer season. The abundance of open-cellular cases during the transition months is consistent with the climatology presented by McCoy et al. (2017); however the lack of closed-cellular cases during summer months was unexpected. Further scrutiny of the observations during the summer months shows that stratocumulus cloud cases often do not persist through the afternoon hours, lasting for less than 12 h, and are often complicated by embedded cumulus and mid- and multi-level clouds which do not meet our selection criteria for closed-cellular cloud organization cases.
To quantify, compare, and contrast the cloud characteristics and associated environment for closed- and open-cellular cloud organizations at the ENA site, we determine the mean and standard deviation of a number of important large-scale meteorological; environmental thermodynamic; and cloud, precipitation and aerosol variables over the defined populations of cloud organization cases. From these statistical measures we present composite visualizations of the relevant cloud and explanatory environmental parameters.
Table 3Mean and standard deviations of important bulk parameters during closed- and open-cellular conditions.
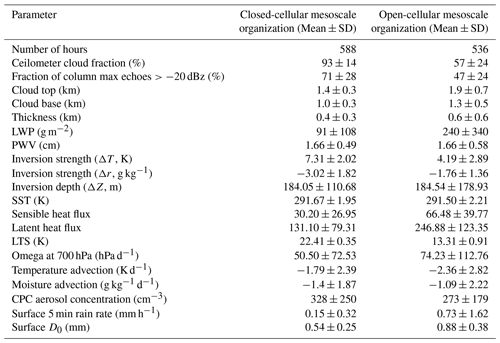
Beginning at the largest scale, we first composite the large-scale meteorological variables (SST, winds, subsidence) from NCEP reanalysis output for each group of cloud organization cases. Figure 4 combines these composited variables with 24 h back trajectories, originating at a height of 500 m, for all the cloud cases in each population. Both closed- and open-cellular cloud organization cases show a similar SST at the ENA site and, as expected based on our selection criteria, a significant northerly component to the surface wind direction but with stronger winds for the open-cellular cases. The closed-cellular cases essentially occur under a classic Azorean surface high-pressure system with weaker winds, resulting in noticeable variability in the originating locations of the 24 h back trajectories. On the other hand, the open-cell cases form under relatively stronger cold-air advection (see also Table 3), i.e., cold-air outbreaks, with the large majority of the back trajectories originating from the Greenland region.
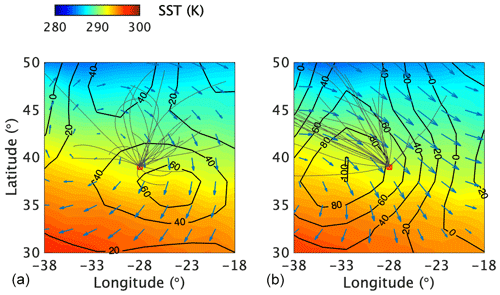
Figure 4Averaged sea surface temperature (K) (colors), winds (arrows) and 700 hPa large-scale subsidence (hPa d−1) (contours) for closed-cell (a) and open-cell (b) cases from NCEP. The thin gray lines indicate the location of parcels 24 h prior to reaching the site. The ARM ENA site is indicated by the red square.
Next, we investigate the differences in the boundary layer thermodynamic structure between the groups of open- and closed-cellular cloud cases. Using twice-daily (nominally 11:00 and 23:00 UTC) radiosonde profiles, we calculate the lower tropospheric profiles of potential temperature, water vapor mixing ratio, and the zonal and meridional components of the horizontal wind. These profiles are used to compile composites for the closed- and open-cellular cloud cases (Fig. 5). For these composite profiles, the height coordinate is normalized by the height of the base of the subsidence inversion to avoid smoothing of the boundary layer thermodynamic structure (Augstein et al., 1974; Mahrt, 1976; Albrecht et al., 1995). We note a distinct difference showing a higher inversion-base height (approx. 2 km compared to approx. 1 km), identified from both the potential temperature and water vapor mixing ratio profiles, for the open-cellular cases which manifest in a higher cloud-top height (approx. 1.9 km compared to 1.4 km); see Table 3. The composite sounding shows that the closed-cellular cases tend to occur in a more well-mixed, moister boundary layer compared to open-cellular cases. This is consistent with our expectations as the organization in closed-cellular cases is driven by relatively stronger cloud-top radiative cooling (e.g., Shao and Randall, 1996) and occurs under shallow boundary layer depths with less precipitation. Consistent with Fig. 4, the radiosonde profiles also show composite winds near the surface from the north-northwest, as expected from our selection criteria, for both groups of cloud organization cases, with significantly stronger wind speeds for the open-cellular cases. When the composites are broken down by the radiosonde launch time (Fig. 6), a difference in the time evolution becomes apparent, with open-cellular cases showing greater variability as a function of time with stabilization in the boundary layer (i.e., a greater increase in potential temperature with height) and a moistening of the free troposphere (above 2 km). This time evolution of the thermodynamic structure is due to a combination of the diurnal cycle and advection. Given that the mean advective tendencies show cold- and dry-air advection for both cloud organization populations, we would expect advection to drive cooling and drying with time for each cloud organization population. Figure 6 shows small to little increase in the temperature and a moistening of the boundary layer with time for both open- and closed-cellular cases. This suggests that the local diurnal cycle plays the more significant role in the evolution of boundary layer thermodynamics. The difference in the mean sensible and latent heat fluxes between open- and closed-cellular cases is consistent with the changes in thermodynamic structure showing larger fluxes, and so larger increases in temperature and moisture for the open-cellular cases. Both open- and closed-cellular cases show a moistening of the boundary layer with time, with the change being more pronounced for the open-cellular cases.
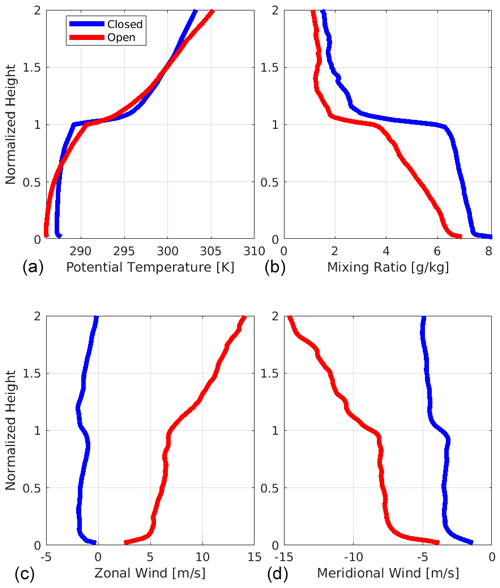
Figure 5Composite profiles of radiosonde reported (a) potential temperature (K), (b) water vapor mixing ratio (g kg−1), and (c) zonal and (d) meridional wind speed (m s−1) for closed- (blue) and open-cellular (red) cloud populations. The height coordinate is normalized by the boundary layer depth.
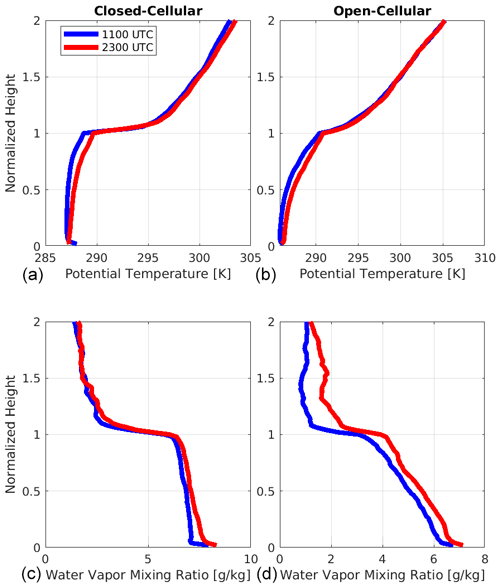
Figure 6Comparison of composite profiles of radiosonde reported (a, b) potential temperature (K) and (c, d) water vapor mixing ratio (g kg−1) for closed cell (a, c) and open cell (b, d) at 11:00 UTC (blue) and 23:00 UTC (red). The height coordinate is normalized by the boundary layer depth.
The most obvious signature of MBL cloud mesoscale organization is the horizontal and vertical distribution of cloud occurrence. Figure 7 shows a two-dimensional histogram for KAZR reflectivity and the vertical profile of cloud occurrence (Xie et al., 2010) for each cloud population. The closed-cellular cases have shallower cloud layers with lower radar reflectivity while the open-cellular cases show smaller cloud frequency of occurrence in the main cloud layer, but deeper cloud layers with overall higher values of radar reflectivity. This is qualitatively consistent with single (or a few) case radar observations of transitions between open- and closed-cellular cases from previous studies (e.g., Stevens et al., 2005; Comstock et al., 2007; Wood et al., 2011) and provides confidence in the visual inspection selection procedure that we used.
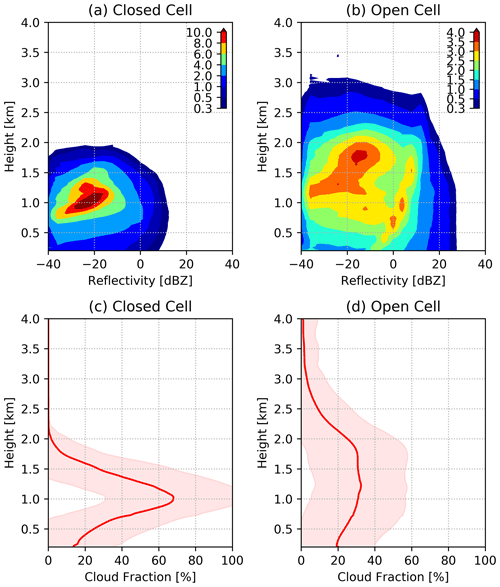
Figure 7Contoured frequency by altitude diagrams (CFAD) of radar reflectivity for (a) open-cell and (b) closed-cell cases. Vertical profiles of hourly cloud fraction from ARSCL for (c) open-cell and closed-cell cases. Lines are means; shadings are 1σ standard deviations.
Given this confirmation of our selection criteria, we calculate the mean and standard deviation for a number of descriptive and potentially explanatory variables for the closed- and open-cell cloud populations (Table 3). There are several noteworthy differences between the populations.
-
Regarding the thermodynamic structure of the MBL, closed-cellular cases occur during times of stronger mean inversions (regardless of the thermodynamic variable used to quantify the inversion strength), but with a similar physical inversion depth. The lower tropospheric stability (LTS; Klein and Hartmann, 1993) is significantly stronger for the closed-cellular cases despite a similar mean SST.
-
The mean subsidence rate (quantified as the value of the large-scale vertical velocity, omega, at the 700 hPa pressure level) is larger, by a factor of 50 % for the open-cellular cases. This greater subsidence rate is likely the result of post-frontal circulations during cold-air outbreak conditions. The deeper boundary layers for the open-cellular cases must be maintained by a combination of increased turbulence (Ghate et al., 2019, 2020) generated by the stronger wind speeds and significantly larger surface sensible and latent heat fluxes compared to closed-cell cases (Kazil et al., 2014; Wang et al., 2010), a consequence of the smaller cloud fractions and thus greater surface downwelling radiative fluxes.
-
When precipitation reaches the surface, and thus is measured by the surface disdrometer, the mean surface rainfall rate is significantly higher, and mean raindrop sizes are significantly larger for the open-cellular cases.
-
Mean aerosol concentrations, as estimated from the CPC, are significantly larger for the closed-cell cases This is consistent with the larger rainfall rates and related increase in precipitation scavenging (Zheng et al., 2018).
-
The mean cloud-top height and cloud-base height are lower, with an accompanying smaller cloud thickness for the closed-cellular cases compared to the open-cellular cases. Correspondingly, mean LWP, when clouds are present, is twice as large for the open-cellular cases compared to the closed-cellular cases. Also, the PWV is identical for the two organizations with slightly more variation for the open-cellular cases.
-
The cloud fraction, defined as the frequency of occurrence of cloud below 4 km in the column above the ceilometer (Wu et al., 2014), is greater for the closed-cellular cases compared to the open-cellular cases.
-
Both open- and closed-cellular populations show mean cold- and dry-air advection, with the open-cellular cases showing stronger cold-air advection and the closed-cellular cases showing stronger dry-air advection.
To further explore the characteristics of the closed- and open-cellular cloud organization populations, the probability density function (PDF) of LWP, PWV, cloud top height, cloud depth, cloud fraction, and echo fraction are examined in Fig. 8. Both the open- and closed-cellular cloud cases exhibit a bimodal distribution of LWP (Fig. 8a), with the local maximum being more clearly separated for the open-cellular cases. This bimodal distribution is consistent with contributions from drizzling and non-drizzling clouds and higher precipitation rates and associated LWP for the open-cellular cases. The two cloud populations show very little difference in the PWV (Fig. 8b) with the closed-cellular cloud cases showing a more well-defined peak at the mode values, consistent with the similarity in the bulk properties presented in Table 3. Both cloud organization populations show a peak cloud-top height (Fig. 8c) at 1.2 km; however, the open-cellular cases show a stronger peak around 2 km, consistent with the bimodal structure in LWP (Fig. 8a) where higher cloud-top heights are associated with larger LWP and precipitating clouds. A comparison of the observed cloud depths (Fig. 8d) is consistent with the LWP and cloud-top height differences showing the open-cellular cloud organization with an extended tail towards larger cloud thicknesses compared to the closed-cellular cloud population. Finally, the cloud fraction (Fig. 8e, ceilometer-observed frequency of occurrence) and echo fraction (Fig. 8f, includes observed drizzle but will exclude the thinnest cloud) of open-cellular cloud cases suggest large variability in cloud coverage with a mode around 50 % compared to much greater coverage for closed-cellular cases. In summary, the open-cellular cloud organization cases have more varied cloud top heights, thickness, and cloud fraction, while the closed-cellular cloud organization cases are overcast with more uniform cloud cover and thickness.
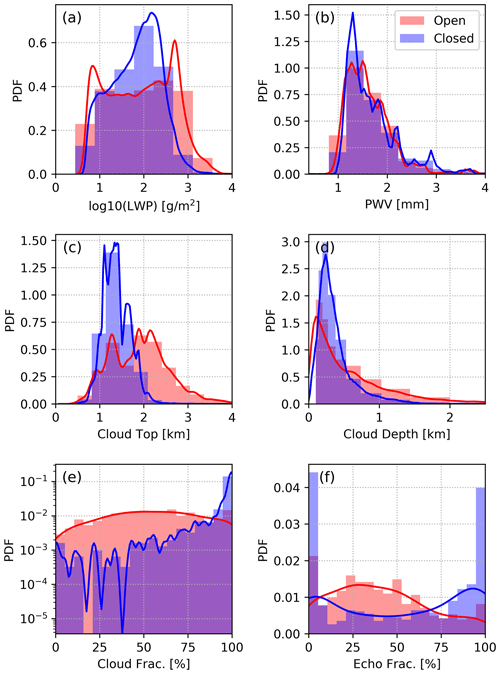
Figure 8Histograms of (a) LWP, (b) PWV, (c) cloud top height, (d) cloud depth, (e) ceilometer cloud fraction (note: logarithmic scale on vertical axis), and (f) KAZR echo fraction for open- (red) and closed-cellular (blue) mesoscale organizations. The lines are the probability density functions (PDFs) calculated using Gaussian kernel density estimation.
The diurnal variation in composite cloud macro-physical properties within the two cloud populations is shown in Fig. 9. As expected from the results shown in Table 3 and Fig. 7, the composite total cloud fraction (Fig. 9a) for closed-cell cases is greater than that for open-cell cases through the entire diurnal cycle. Closed-cell cases are nearly overcast with little variation in total cloud fraction during nighttime and early morning hours (nominally 22:00–10:00 UTC) with a subsequent decrease, but greater variability, during the daytime. Similarly, open-cell cases show relatively smaller total cloud fraction during the afternoon–evening hours (nominally 16:00–23:00 UTC), compared to the overnight and morning hours, but with similar variability over the population across the diurnal cycle. These diurnal cycles in cloud fraction, with decreased low cloud coverage during the afternoon hours, are broadly consistent with previous studies in the Azores region (Miller et al., 1998; Rémillard et al., 2012). Also consistent with Table 3, the mean cloud depth (or thickness) (Fig. 9b) for open-cell cases is larger than that for closed-cell cases; however the variability among the open-cell cases is much greater than the closed-cell cases. This is consistent with the regular occurrence of a mixture of deeper, precipitating cumulus and stratocumulus in the open-cell cases. For the LWP and PWV (Fig. 9c, d), the open-cell cases exhibit much greater variability, with the mean values generally being slightly larger during the nighttime and early morning hours. The hourly mean values, of both LWP and PWV, tend to converge during the daytime hours consistent with the decrease in physical cloud thickness (Fig. 9b).
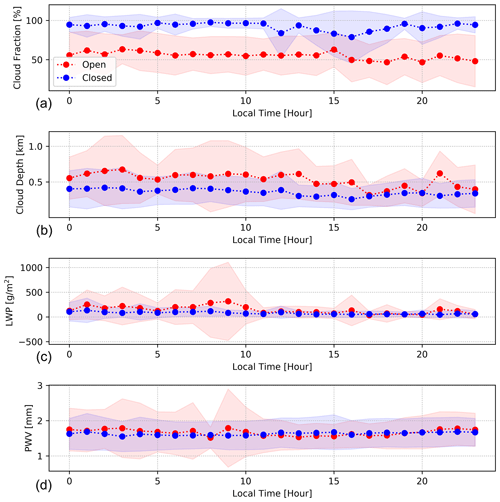
Figure 9Diurnal cycle of the mean and standard deviation of the (a) 1-D cloud fraction (%), (b) cloud depth (or thickness) (km), (c) liquid water path (g m−2) and (d) precipitable water vapor (mm) for open-cell (red) and closed-cell (blue) cases. The markers represent the mean values, and the shadings represent the 1σ standard deviations.
Histograms of surface rainfall DSD characteristics show distinct differences between closed- and open-cellular cloud populations (Fig. 10). Open-cellular cloud cases tend to have larger median drop sizes, liquid water contents (LWCs) and rain rates compared to closed-cellular cases, while the closed-cellular cases have somewhat larger numbers of droplets based on the intercept of the DSD. This result is consistent with the results in Fig. 8 and Table 3 showing that the open-cellular cloud organization cases tend to have larger cloud thickness, higher cloud-top heights and larger LWPs compared to the closed-cellular cloud organization cases. It is also important to note that raindrops larger than 1 mm in diameter are often observed in both closed- and open-cell cases. This is an important threshold for millimeter cloud radar observations (Lehrmitte, 2002; Giangrande et al., 2010) as the Rayleigh approximation becomes invalid, and non-Rayleigh scattering must be considered when interpreting the observed radar reflectivity factor.
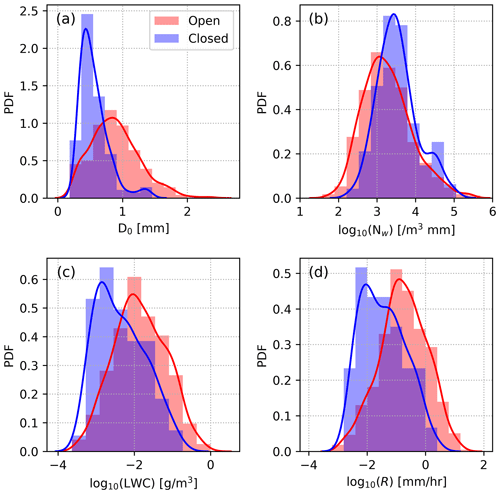
Figure 10Comparison histograms of precipitation drop size distribution parameters: (a) median volume drop size (D0), (b) DSD intercept parameter (Nw), (c) liquid water content and (d) rain rate (RR) for open- and closed-cell cases. The lines are the probability density functions (PDFs) calculated using Gaussian kernel density estimation.
MBL stratocumulus clouds often exist in two distinct states of mesoscale organization: closed and open cellular. The different cloud organization populations differ significantly in their low-level cloud fraction, shortwave albedo and LWP. A number of studies over recent decades have identified the importance of aerosols, drizzle and their impacts on boundary layer dynamics in determining the cellular organization of clouds within the MBL. However, the interplay of these different mechanisms and their relative importance under different regimes remain an area of active research, and advances in our understanding are necessary for improved representation in large-scale atmospheric models (Wood et al., 2016; Jensen et al., 2016b). Using long-term observations from the ARM ENA site, we have compiled composite cloud, precipitation and thermodynamic properties over a carefully selected population of open- and closed-cellular MBL cloud cases. Important highlights of this analysis include the following.
-
Both open- and closed-cellular cloud organization cases occur during similar SST conditions; however, open-cellular cases are distinguished by stronger cold-air advection and subsidence compared to closed-cellular cases, consistent with the open-cellular cases forming during cold-air outbreaks.
-
The open-cellular clouds were associated with deeper boundary layers, stronger winds, higher rain rates, and stronger large-scale subsidence compared to their closed-cellular counterparts.
-
Raindrops with a diameter larger than 1 mm were routinely recorded at the surface during both organizations, with a higher number of large drops during open-cellular cloud cases.
To distinctly summarize the similarities and differences in the environmental and cloud properties during the two mesoscale cloud organization populations, we normalized the data using the average of the samples available for both closed- and open-cellular cloud cases. Figure 11 shows the mean of the normalized data for a number of important boundary layer and cloud properties. These results comparing the surface condensation nuclei (CN), PWV and depth of the boundary layer inversion (Inv. ΔZ) show very little difference between the two populations (difference in normalized values less than 0.2). More significant differences are shown in all the other variables. The LWP and rain rate, which are expected to co-vary (e.g., Wood, 2005; Zuidema et al., 2005; Geoffroy et al., 2008; Serpetzoglou et al., 2008; Kubar et al., 2009; Rémillard et al., 2012), exhibit the largest normalized differences of greater than 0.8. The boundary layer inversion strength, in terms of the jump in either potential temperature (Inv. Δθ) or water vapor mixing ratio (Inv. Δr), was stronger during closed-cellular cases. Although the rain fraction was higher for the closed-cellular cloud cases, due to their greater coverage, they had significantly lower rain rates compared to the open-cellular cloud cases. In addition to providing insights into the environmental and cloud characteristics during these two mesoscale cloud organization populations, the similarities and differences noted here can be used to evaluate model simulations of the two cloud organizational states.
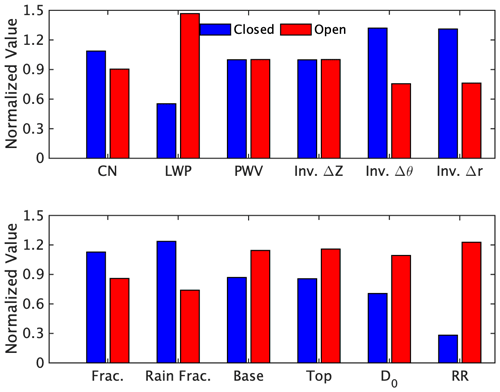
Figure 11Normalized values of boundary layer and cloud properties for closed-cellular (blue) and open-cellular (red) organizations.
Follow-on research activities are aimed at exploring differences among the internal properties of the marine boundary layer such as dynamics and microphysics for the closed- and open-cell cloud populations. In addition, another research avenue is the use of satellite and reanalysis data to investigate the evolution of the cloud and boundary layer properties along the parcel trajectories for both cloud populations.
All ARM ENA datasets are available from the ARM data archive (https://www.arm.gov/data, last access: 20 January 2020; https://doi.org/10.5439/1021460, ARM, 2013; https://doi.org/10.5439/1393437, 2014a; https://doi.org/10.5439/1025316, ARM, 2014b; https://doi.org/10.5439/1393437, ARM, 2015). Meteosat data were obtained from the Satellite ClOud and Radiation Property (SatCORPS) team at NASA Langley Research Center: https://satcorps.larc.nasa.gov/prod/exp/amf_azores_reprocessed/visst-pixel-netcdf (NASA, 2019a) and https://search.earthdata.nasa.gov/search?q5CER_GEO_Ed4_MET10 (NASA, 2019b). The 1 ∘ global reanalysis data are available from http://www.esrl.noaa.gov/psd/data/gridded/data.ncep.reanalysis.html (National Centers for Environmental Prediction et al., 2019).
MPJ and VPG contributed project design and management, data analysis and interpretation, and lead writing responsibilities. DW contributed data analysis and visualization, writing, reviewing, and editing. DKA contributed data analysis and visualization. SEG contributed to interpretation of results, writing, reviewing, and editing. MJB, KJL, and MMT contributed to data preparation and analysis, writing, reviewing, and editing.
The authors declare that they have no conflict of interest.
Publisher’s note: Copernicus Publications remains neutral with regard to jurisdictional claims in published maps and institutional affiliations.
This article is part of the special issue “Marine aerosols, trace gases, and clouds over the North Atlantic (ACP/AMT inter-journal SI)”. It is not associated with a conference.
Participation of Michael P. Jensen, Die Wang, and Scott E. Giangrande was supported by the U.S. DOE Atmospheric System Research Program. Contributions from Mary J. Bartholomew and Karen L. Johnson were supported by the U.S. DOE Atmospheric Radiation Measurement Facility. Participation of Diana K. Apoznanski was supported by the U.S. DOE, Office of Science, Office of Workforce Development for Teachers and Scientists under the Science Undergraduate Laboratory Internships program. Virendra P. Ghate was supported by the National Science Foundation through the University of Chicago and the U.S. DOE Atmospheric System Research program. The METEOSAT-10/11 SatCORPS data were obtained from the NASA Langley Research Center Atmospheric Science Data Center and the NASA Langley Research SatCORPS team. Satellite analyses were supported by the NASA CERES project and by the DOE ARM program.
The research has been supported by the U.S. Department of Energy via grant numbers DE-SC0012704, DE-AC02-06CH11357, DE-SC0013896, and 89243119SSC000035 and by the National Science Foundation grant number AGS-1445831.
This paper was edited by Zhanqing Li and reviewed by three anonymous referees.
Albrecht, B. A., Jensen, M. P., and Syrett, W.J.: Marine boundary layer structure and fractional cloudiness, J. Geophys. Res., 100, 14209–14222, https://doi.org/10.1029/95JD00827,1995.
Atmospheric Radiation Measurement (ARM) user facility: Balloon-borne Sounding System (SONDEWNPN). 2013-09-28 to 2020-12-07, updated hourly, Eastern North Atlantic (ENA) Graciosa Island, Azores, Portugal (C1), compiled by: Keeler, E., Coulter, R., Kyrouac, J., and Holdridge, D., ARM Data Center [data set], https://doi.org/10.5439/1021460, 2013.
Atmospheric Radiation Measurement (ARM) user facility: MWR Retrievals with MWRRET Version 2 (MWRRET2TURN). 2014-05-01 to 2019-10-30, updated hourly, Eastern North Atlantic (ENA) Graciosa Island, Azores, Portugal (C1). ARM Data Center [data set], https://doi.org/10.5439/1393437, 2014a.
Atmospheric Radiation Measurement (ARM) user facility: Video Disdrometer (VDISDROPS). 2014-10-31 to 2020-12-04, updated hourly, Eastern North Atlantic (ENA) Graciosa Island, Azores, Portugal (C1), compiled by: Wang, D., and Bartholomew, M., ARM Data Center [data set], https://doi.org/10.5439/1025316, 2014b.
Atmospheric Radiation Measurement (ARM) user facility: Active Remote Sensing of Clouds (ARSCL) product using Ka-band ARM Zenith Radars, 2015-07-17 to 2019-12-19, updated hourly, Eastern North Atlantic (ENA) Graciosa Island, Azores, Portugal (C1), compiled by: Johnson, K., and Scott, T., ARM Data Center [data set], https://doi.org/10.5439/1393437, 2015.
Augstein, E., Schmidt, H., and Ostapoff, F.: The vertical structure of the atmospheric planetary boundary layer in undisturbed trade winds over the Atlantic Ocean, Bound.-Lay. Meteorol., 6, 129–150, 1974.
Bartholomew, M. J.: Two-dimensional video disdrometer (VDIS) instrument handbook, DOE/SC-ARM-TR-111, U. S. Department of Energy, Office of Science, Office of Biological and Environmental Research, Atmospheric Radiation Measurement Facility. Germantown, MD, 2017.
Bony, S. and Dufresne, J.-L.: Marine boundary layer clouds at the heart of the tropical cloud feedback uncertainties in climate models, Geophys. Res. Lett., 32, L20806, https://doi.org/10.1029/2005GL023851, 2005.
Bretherton, C. S., Uttal, T., Fairall, C. W., Yuter, S. E., Weller, R. A., Baumgardner, D., Comstock, K., and Wood, R.: The EPIC 2001 stratocumulus study, B. Am. Meteorol. Soc., 85, 967–977, 2004.
Bretherton, C. S., Wood, R., George, R. C., Leon, D., Allen, G., and Zheng, X.: Southeast Pacific stratocumulus clouds, precipitation and boundary layer structure sampled along 20∘ S during VOCALS-REx, Atmos. Chem. Phys., 10, 10639–10654, https://doi.org/10.5194/acp-10-10639-2010, 2010.
Burleyson, C. D. and Yuter, S. E.: Patterns of diurnal marine stratocumulus cloud faction variability, J. Appl. Meteor. Clim., 54, 847–866, https://doi.org/10.1175/JAMC-D-14-0178.1, 2015.
Cadeddu, M. P., Liljegren, J. C., and Turner, D. D.: The Atmospheric radiation measurement (ARM) program network of microwave radiometers: instrumentation, data, and retrievals, Atmos. Meas. Tech., 6, 2359–2372, https://doi.org/10.5194/amt-6-2359-2013, 2013.
Clothiaux, E. E., Ackerman, T. P., Mace, G. G., Moran, K. P., Marchand, R. T., Miller, M. A., and Martner, B. E.: Objective determination of cloud heights and radar reflectivities using a combination of active remote sensors at the ARM CART sites, J. Appl. Meteor., 39, 645–665, 2000.
Clothiaux, E. E., Miller, M. A., Perez, R. C., Turner, D. D., Moran, K. P., Martner, B. E., Ackerman, T. P., Mace, G. G., Marchand, R. T., Widener, K. B., Rodriguez, D. J., Uttal, T., Mather, J. H., Flynn, C. J., Gaustad, K. L., and Ermold, B.: The ARM Millimeter Wave Cloud Radars (MMCR2) and the Active Remote Sensing of Clouds (ARSCL) Value-Added Product (VAP), DOE Tech. Memo ARM VAP-002.1, U. S. Department of Energy, Office of Science, Office of Biological and Environmental Research, Atmospheric Radiation Measurement Facility. Germantown, MD, 2001.
Comstock, K. K., Bretherton C. S., and Yuter, S. E.: Mesoscale variability and drizzle in Southeast Pacific stratocumulus, J. Atmos. Soc., 62, 3792–3807, 2005.
Comstock, K. K., Yuter, S. E., Wood, R., and Bretherton, C. S.: The three-dimensional structure and kinematics of drizzling stratocumulus, Mon. Weather Rev., 135, 3767–3784, 2007.
Feingold, G., Koren, I., Wang, H., Xue, H., and Brewer, W. A.: Precipitation-generated oscillations in open cellular cloud fields, Nature, 466, 849–852, https://doi.org/10.1038/nature09314, 2010.
Feingold, G., Koren, I., Yamaguchi, T., and Kazil, J.: On the reversibility of transitions between closed and open cellular convection, Atmos. Chem. Phys., 15, 7351–7367, https://doi.org/10.5194/acp-15-7351-2015, 2015.
Gaustad, K. L., Turner, D. D., and McFarlane, S. A.: MWRRET Value-Added Product: The Retrieval of Liquid Water Path and Precipitable Water Vapor from Microwave Radiometer (MWR) Data Sets, ARM-TR-081.2, 10 pp., U. S. Department of Energy, Office of Science, Office of Biological and Environmental Research, Atmospheric Radiation Measurement Facility. Germantown, MD, 2011.
Geoffroy, O., Brenguier, J.-L., and Sandu, I.: Relationship between drizzle rate, liquid water path and droplet concentration at the scale of a stratocumulus cloud system, Atmos. Chem. Phys., 8, 4641–4654, https://doi.org/10.5194/acp-8-4641-2008, 2008.
Ghate, V. P. and Cadeddu, M. P.: Drizzle and turbulence below closed cellular marine stratocumulus clouds, J. Geophys. Res., 124, 5724–5737, https://doi.org/10.1029/2018JD030141, 2019.
Ghate, V. P., Albrecht, B. A., Miller, M. A., Brewer, A., and Fairall, C. W.: Turbulence and radiation in a stratocumulus topped marine boundary layer: A case study from VOCALS ReX, J. Appl. Meteor. Climatol., 53, 117–135, 2013.
Ghate, V. P., Cadeddu, M. P., and Wood, R.: Drizzle, turbulence, and density currents below post cold frontal open cellular marine stratocumulus clouds, J. Geophys. Res., 125, e2019JD031586, https://doi.org/10.1029/2019JD031586, 2020.
Giangrande, S. E., Luke, E. P., and Kollias, P.: Automated retrievals of precipitation parameters using non-Rayleigh scattering at 95 GHz, J. Atmos. Ocean. Tech., 27, 1490–1503, 2010.
Giangrande, S. E., Wang, D., Bartholomew, M. J., Jensen, M. P., Mechem, D. B., Hardin, J., and Wood, R.: Midlatitude oceanic cloud precipitation properties as sampled by the ARM Eastern North Atlantic Observatory, J. Geophys. Res.-Atmos., 124, 4741–4760, https://doi.org/10.1029/2018JD029667, 2019.
Hardin, J. and Guy, G.: PyDisdrometer v1.0 (v1.0), Zenodo [code], https://doi.org/10.5281/zenodo.9991, 2017.
Hartmann, D. L. and Short, D. A.: On the use of earth radiation budget statistics for studies of clouds and climate, J. Atmos. Sci., 37, 1233–1250, 1980.
Holdridge, D., Prell, J., Ritsche, M., and Coulter, R.: Balloon-Borne Sounding System (SONDE) Handbook, ARM-TR-029, 27 pp., U. S. Department of Energy, Office of Science, Office of Biological and Environmental Research, Atmospheric Radiation Measurement Facility, Germantown, MD, 2011.
Jensen, M. P., Vogelmann, A. M., Collins, W. D., Zhang, G. J., and Luke, E. P.: Investigations of regional and seasonal variations in marine boundary layer cloud properties from MODIS observations, J. Climate, 21, 4955–4973, 2008.
Jensen, M. P., Holdridge, D. J., Survo, P., Lehtinen, R., Baxter, S., Toto, T., and Johnson, K. L.: Comparison of Vaisala radiosondes RS41 and RS92 at the ARM Southern Great Plains site, Atmos. Meas. Tech., 9, 3115–3129, https://doi.org/10.5194/amt-9-3115-2016, 2016a.
Jensen, M. P., Wang, J., and Wood, R.: Atmospheric System Research Marine Low Clouds Workshop Report, DOE/SC-ASR-16-001, 13 pp., U. S. Department of Energy, Office of Science, Office of Biological and Environmental Research, Atmospheric Radiation Measurement Facility, Germantown, MD, 2016b.
Kalnay, E., Kanamitsu, M., Kistler, R., Collins, W., Deaven, D., Gandin, L., Iredell, M., Saha, S., White, G., Woollen, J., Zhu, Y., Chelliah, M., Ebisuzaki, W., Higgins, W., Janowiak, J., Mo, K. C., Ropelewski, C., Wang, J., Leetmaa, A., Reynolds, R., Jenne, R., and Joseph, D.: The NCEP/NCAR 40-Year Reanalysis Project, Bull. Am. Meteorol. Soc., 77, 437–472, 1996.
Kazil, J., Feingold, G., Wang, H., and Yamaguchi, T.: On the interaction between marine boundary layer cellular cloudiness and surface heat fluxes, Atmos. Chem. Phys., 14, 61–79, https://doi.org/10.5194/acp-14-61-2014, 2014.
Klein, S. A. and Hartmann, D. L.: The Seasonal Cycle of Low Stratiform Clouds, J. Climate, 6, 1587–1606, 1993.
Kollias, P., Clothiaux, E. E., Albrecht, B. A., Miller, M. A., Moran, K. P., and Johnson, K. L.: The Atmospheric Radiation Measurement program cloud profiling radars: An evaluation of signal processing and sampling strategies, J. Atmos. Ocean. Tech., 22, 930–948, https://doi.org/10.1175/JTECH1749.1, 2005.
Kollias, P., Miller, M. A., Luke, E. P., Johnson, K. L., Clothiaux, E. E., Moran, K. P., Widener, K. B., and Albrecht, B. A.: The Atmospheric Radiation Measurement Program cloud profiling radars: Second generation sampling strategies, processing, and cloud data products, J. Atmos. Ocean. Tech., 24, 1199–1214, https://doi.org/10.1175/JTECH2033.1, 2007.
Kollias, P., Clothiaux, E. E., Ackerman, T. P., Albrecht, B. A., Widener, K. B., Moran, K. P., Luke, E. P., Johnson, K. L., Bharwadaj, N., Mead, J. B., Miller, M. A., Verlinde, J., Marchand, R. T., and Mace, G. G.: Development and applications of ARM millimeter-wavelength cloud radars, AMS Meteorol. Mono., 57, 17.1–17.19, https://doi.org/10.1175/AMSMONOGRAPHS-D-15-0037.1, 2016.
Kruger, A. and Krajewski, W. F.: Two-dimensional video disdrometer: A description, J. Atmos. Ocean. Tech., 19, 602–617, 2002.
Kubar, T. L., Hatmann, D. L., and Wood, R.: Understanding the importance of microphysics and macrophysics for warm rain in marine low clouds. Part I: Satellite observations, J. Atmos. Sci., 66, 2953–2972, https://doi.org/10.1175/2009JAS3071.1, 2009.
Lamraoui, F., Booth, J. F., Naud, C. M., Johnson, K. L., and Jensen, M. P.: The interactions between boundary layer and convection schemes in a WRF simulation of post-cold-frontal clouds over the ARM East North Atlantic site, J. Geophys. Res.-Atmos., 124, 4699–4721, https://doi.org/10.1029/2018JD029370, 2019.
Lhermitte, R.: Centimeter and millimeter wavelength radars in meteorology, Lhermitte Publications, Miami, FL, USA, p. 550, 2002.
Mahrt, L.: Mixed-layer moisture structure, Mon. Weather Rev., 104, 1403–1418, 1976.
Mather, J. H. and Voyles, J. W.: The ARM climate research facility: A review of structure and capabilities, Bull. Am. Meteorol. Soc., 94, 377–392, https://doi.org/10.1175/BAMS-D-11-00218.1, 2013.
McCoy, I. L., Wood, R., and Fletcher, J. K.: Identifying meteorological controls on open and closed mesoscale cellular convection associated with marine cold air outbreaks, J. Geophys. Res.-Atmos., 122, 11678–11702, https://doi.org/10.1002/2017JD027031, 2017.
Mechem, D. B., Yuter, S. E., and de Szoeke, S. P.: Thermodynamic and aerosol controls in Southeast Pacific stratocumulus, J. Atmos. Sci., 69, 1250–1266, https://doi.org/10.1175/JAS-D-11-0165.1, 2012.
Miller, M. A., Jensen, M. P., and Clothiaux, E. E.: Observations of diurnal variations in cloud, precipitation and thermodynamic structure in the stratocumulus transition regime and implications for models, J. Atmos. Sci., 55, 2294–2310, 1998.
Miller, M. A., Nitschke, K., Ackerman, T. P., Ferrell, W. R., Hickmon, N., and Ivey, M.: Chapter 9: The ARM Mobile Facilities, AMS Meteorol. Mono., 57, 9.1–9.15, https://doi.org/10.1175/AMSMONOGRAPHS-D-15-0051.1, 2016.
Morris, V. R.: Microwave Radiometer (MWR) Handbook, edited by: Stafford, R., U.S. Department of Energy, DOE/SC-ARM/TR-016, 16 pp., U. S. Department of Energy, Office of Science, Office of Biological and Environmental Research, Atmospheric Radiation Measurement Facility, Germantown, MD, 2019.
Muhlbauer, A., McCoy, I. L., and Wood, R.: Climatology of stratocumulus cloud morphologies: microphysical properties and radiative effects, Atmos. Chem. Phys., 14, 6695–6716, https://doi.org/10.5194/acp-14-6695-2014, 2014.
NASA: SatCORPS METEOSAT10-derived Cloud and Radiative Property Dataset: ENA version 4.2.2, available at: https://satcorps.larc.nasa.gov/prod/exp/amf_azores_reprocessed/visst-pixel-netcdf, last access: 19 February 2019a.
NASA: CER_GEO_Ed4_MET10 pixel-level dataset, version1.0, subset Oct15-May17, NASA Earthdata, available at: https://search.earthdata.nasa.gov/search?q5CER_GEO_Ed4_MET10, last access: 10 February 2019b.
National Centers for Environmental Prediction/National Weather Service/NOAA/U.S. Department of Commerce: NCEP/NCAR Global Reanalysis Products, 1948-continuing, Research Data Archive at NOAA/PSL, available at: http://www.esrl.noaa.gov/psd/data/gridded/data.ncep.reanalysis.html, last access: 20 March 2019.
Petters, M. D., Snider, J. R., Stevens, B., Vali, G., Faloona, I., and Russell, L. M.: Accumulation mode aerosol, pockets of open cells, and particle nucleation in the remote subtropical Pacific marine boundary layer, J. Geophys. Res., 11, D02206, https://doi.org/10.1029/2004JD005694, 2006.
Randall, D. A., Coakley Jr., J. A., Fairall, C. W., Kropfli, R. A., and Lenshow, D. H.: Outlook for Research on Subtropical Marine Stratiform Clouds, B. Am. Meteorol. Soc., 65, 1290–1301, 1984.
Rémillard, J. and Tselioudis, G.: Cloud regime variability over the Azores and its applications to climate model evaluation, J. Climate, 28, 9707–9720, https://doi.org/10.1175/JCLI-D-15-0066.1, 2015.
Rémillard, J., Kollias, P., Luke, E. P., and Wood, R.: Marine boundary layer cloud observations in the Azores, J. Climate, 25, 7381–7398, https://doi.org/10.1175/JCLI-D-11-00610.1, 2012.
Rolph, G. D., Stein, A. F., and Sunder, B. J. B.: Real-time Environmental Applications and Display sYstem: READY, Environ. Model. Softw., 95, 210–228, https://doi.org/10.1016/j.envsoft.2017.06.025, 2017.
Rossow, W. B., Delo, C., and Cairns, B.: Implications of the observed mesoscale variations of clouds for the earth's radiation budget, J. Climate, 15, 557–585, 2002.
Savic-Jovcic, V. and Stevens, B.: The structure and mesoscale organization of precipitating stratocumulus, J. Atmos. Sci., 65, 1587–1605, https://doi.org/10.1175/2007JAS2456.1, 2008.
Serpetzoglou, E., Albrecht, B. A., Kollias, P., and Fairall, C. W.: Boundary layer, cloud, and drizzle variability in the southeast Pacific stratocumulus regime, J. Climate, 21, 6196–6214, 2008.
Shao, Q. and Randall, D. A.: Cloud mesoscale cellular convection driven by cloud-top radiative cooling, J. Atmos. Sci., 53, 2144–2165, 1996.
Sharon, T. M., Albrecht, B. A., Johnson, H. H., Minnis, P., Khaiyer, M. M., Van Reken, T., Seinfeld, J., and Flagan, R.: Aerosol and cloud microphysical characteristics of rifts and gradients in maritime stratocumulus clouds, J. Atmos. Sci., 63, 983–997, 2006.
Slingo, A.: Sensitivity of the Earth's radiation budget to changes in low clouds, Nature, 343, 49–51, https://doi.org/10.1038/343049a0, 1990.
Stein, A. F., Draxler, R. R., Rolph, G. D., Stunder, B. J. B., Cohen, M. D., and Ngan, F.: NOAA's HYSPLIT atmospheric transport and dispersion modelling system, B. Am. Meteorol. Soc., 96, 2059–2077, https://doi.org/10.1175/BAMS-D-14-00110.1, 2015.
Stevens, B., Vali, G., Comstock, K., Wood, R., Van Zanten, M., Austin, P. H., Bretherton, C. S., and Lenschow, D. H.: Pockets of open cells (POCs) and drizzle in marine stratocumulus, B. Am. Meteorol. Soc., 86, 51–57, 2005.
Terai, C. R. and Wood, R.: Aircraft observations of cold pools under marine stratocumulus, Atmos. Chem. Phys., 13, 9899–9914, https://doi.org/10.5194/acp-13-9899-2013, 2013.
Turner, D. D., Clough, S. A., Liljegren, J. C., Clothiaux, E. E., Cady-Pereira, K., and Gaustad, K. L.: Retrieving liquid water path and precipitable water vapor from Atmospheric Radiation Measurement (ARM) microwave radiometers, IEEE T. Geosci. Remote, 45, 3680–3690, https://doi.org/10.1109/TGRS.2007.903703, 2007.
Van Zanten, M. C. and Stevens, B.: Observations of the structure of heavily precipitating marine stratocumulus, J. Atmos. Sci., 62, 4327–4342, 2005.
Wang, D., Giangrande, S. E., Bartholomew, M. J., Hardin, J., Feng, Z., Thalman, R., and Machado, L. A. T.: The Green Ocean: precipitation insights from the GoAmazon2014/5 experiment, Atmos. Chem. Phys., 18, 9121–9145, https://doi.org/10.5194/acp-18-9121-2018, 2018.
Wang, H. and Feingold, G.: Modeling mesoscale cellular structures and drizzle in marine stratocumulus. Part I: Impact of drizzle on the formation and evolution of open cells, J. Atmos. Sci., 66, 3237–3256, 2009.
Wang, H., Feingold, G., Wood, R., and Kazil, J.: Modelling microphysical and meteorological controls on precipitation and cloud cellular structures in Southeast Pacific stratocumulus, Atmos. Chem. Phys., 10, 6347–6362, https://doi.org/10.5194/acp-10-6347-2010, 2010.
Widener, K., Bharadwaj, N., and Johnson, K. L.: Ka-band ARM Zenith Radar (KAZR) Handbook, DOE/SC-ARM/TR-106, 18 pp., U. S. Department of Energy, Office of Science, Office of Biological and Environmental Research, Atmospheric Radiation Measurement Facility, Germantown, MD, available at: https://www.arm.gov/publications/tech_reports/handbooks/kazr_handbook.pdf (last access: 20 January 2021), 2012.
Wilbanks, M. C., Yuter, S. E., de Szoeke, S. P., Bewer, W. A., Miller, M. A., Hill, A. M., and Burleyson, C. D.: Near-surface density currents observed in the Southeast Pacific stratocumulus-topped marine boundary layer, Mon. Weather Rev., 143, 3532–3555, https://doi.org/10.1175/MWR-D-14-00359.s1, 2015.
Wood, R.: Drizzle in stratiform boundary layer clouds. Part I: Vertical and horizontal structure, J. Atmos. Sci., 62, 3011–3033, 2005.
Wood, R.: Stratocumulus clouds, Mon. Weather Rev., 140, 2373–2423, https://doi.org/10.1175/MWR-D-11-00121.1,2012.
Wood, R. and Hartmann, D. L.: Spatial Variability of Liquid Water Path in Marine Low Cloud: The Importance of Mesoscale Cellular Convection, J. Climate, 19, 1748–1764, https://doi.org/10.1175/JCLI3702.1, 2006.
Wood, R., Comstock, K. K., Bretherton, C. S., Cornish, C., Tomlinson, J., Collins, D. R., and Fairall, C.: Open cellular structure in marine stratocumulus streets, J. Geophys. Res., 113, D12207, https://doi.org/10.1029/2007JD009371, 2008.
Wood, R., Bretherton, C. S., Leon, D., Clarke, A. D., Zuidema, P., Allen, G., and Coe, H.: An aircraft case study of the spatial transition from closed to open mesoscale cellular convection over the Southeast Pacific, Atmos. Chem. Phys., 11, 2341–2370, https://doi.org/10.5194/acp-11-2341-2011, 2011.
Wood, R., Wyant, M., Bretherton, C. S., Remilliard, J., Kollias, P., Fletcher, J., Stemmier, J., de Szoeke, S., Yuter, S., Miller, M., Mechem, D., Tselioudis, G., Chiu, J. C., Mann, J. A. L., O'Conor, E. J., Hogan, R. J., Dong, X., Miller, M. A., Ghate, V., Jefferson, A., Min, Q., Minnis, P., Polikonda, R., Albrecht, B., Luke, E., Hannay, C., and Lin, Y.: Clouds, Aerosols, and Precipitation in the Marine Boundary Layer: An ARM Mobile Facility Deployment, B. Am. Meteorol. Soc., 96, 419–440, https://doi.org/10.1175/BAMS-D-13-00180.1, 2015.
Wood, R., Jensen, M. P., Wang, J., Bretherton, C. S., Burrows, S. M., Del Genio, A. D., Fridlind, A. M., Ghan, S. J., Ghate, V. P., Kollias, P., Krueger, S. K., McGraw, R. L., Miller, M. A., Painemal, D., Russell, L. M., Yuter, S. E., and Zuidema, P.: Planning the Next Decade of Coordinated Research to Better Understand and Simulate Marine Low Clouds, B. Am. Meteorol. Soc., 97, 1699–1702, 2016.
Wu, W., Liu, Y., Jensen, M. P., Toto, T., Foster, M. J., and Long, C. N.: A comparison of multiscale variations of decade-long cloud fractions from six different platforms over the Southern Great Plains in the United States, J. Geophys. Res., 119, 3438–3459, https://doi.org/10.1002/2013JD019813, 2014.
Xie, S., McCoy, R., Klein, S., Cederwall, R., Wiscombe, W., Clothiaux, E., Gaustad, K., Horwedel, B., Jensen, M., Johnson, K., Long, C., Mather, J., McCord, R., McFarlane, S., Palanisamy, G., Shi, Y., and Turner, D.: Clouds and More: ARM Climate Modeling Best Estimate data a new data product for climate studies, B. Am. Meteorol. Soc., 91, 13–20, https://doi.org/10.1175/2009BAMS2891.1, 2010.
Xue, H., Feingpld, G., and Stevens, B.: Aerosol effects on clouds, precipitation, and the organization of shallow cumulus convection, J. Atmos. Sci., 65, 392–406, https://doi.org/10.1175/2007JAS2428.1, 2008.
Yamaguchi, T. and Feingold, G.: On the relationship between open cellular convective cloud patterns and the spatial distribution of precipitation, Atmos. Chem. Phys., 15, 1237–1251, https://doi.org/10.5194/acp-15-1237-2015, 2015.
Zhang, G. J., Vogelmann, A. M., Jensen, M. P., Collins, W. D., and Luke, E. P.: Relating satellite observed cloud properties from MODIS to meteorological conditions for marine stratus clouds, J. Climate, 23, 1374–1391, 2010.
Zheng, G., Wang, Y., Aiken, A. C., Gallo, F., Jensen, M. P., Kollias, P., Kuang, C., Luke, E., Springston, S., Uin, J., Wood, R., and Wang, J.: Marine boundary layer aerosol in the eastern North Atlantic: seasonal variations and key controlling processes, Atmos. Chem. Phys., 18, 17615–17635, https://doi.org/10.5194/acp-18-17615-2018, 2018.
Zuidema, P., Westwater, E. R., Fairall, C., and Hazen, D.: Ship-based liquid water path estimates in marine stratocumulus, J. Geophys. Res., 110, D20206, https://doi.org/10.1029/2005JD005833, 2005.
- Abstract
- Copyright statement
- Introduction
- Data and instrumentation
- Identification of closed- and open-cellular cloud organization cases
- Composite characteristics for cloud organization populations
- Summary and conclusions
- Data availability
- Author contributions
- Competing interests
- Disclaimer
- Special issue statement
- Acknowledgements
- Financial support
- Review statement
- References
- Abstract
- Copyright statement
- Introduction
- Data and instrumentation
- Identification of closed- and open-cellular cloud organization cases
- Composite characteristics for cloud organization populations
- Summary and conclusions
- Data availability
- Author contributions
- Competing interests
- Disclaimer
- Special issue statement
- Acknowledgements
- Financial support
- Review statement
- References