the Creative Commons Attribution 4.0 License.
the Creative Commons Attribution 4.0 License.
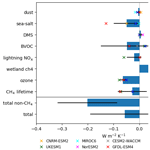
Climate-driven chemistry and aerosol feedbacks in CMIP6 Earth system models
Gillian Thornhill
Dirk Olivié
Ragnhild B. Skeie
Alex Archibald
Susanne Bauer
Ramiro Checa-Garcia
Stephanie Fiedler
Gerd Folberth
Ada Gjermundsen
Larry Horowitz
Jean-Francois Lamarque
Martine Michou
Jane Mulcahy
Pierre Nabat
Vaishali Naik
Fiona M. O'Connor
Fabien Paulot
Michael Schulz
Catherine E. Scott
Roland Séférian
Chris Smith
Toshihiko Takemura
Simone Tilmes
Kostas Tsigaridis
James Weber
Feedbacks play a fundamental role in determining the magnitude of the response of the climate system to external forcing, such as from anthropogenic emissions. The latest generation of Earth system models includes aerosol and chemistry components that interact with each other and with the biosphere. These interactions introduce a complex web of feedbacks that is important to understand and quantify.
This paper addresses multiple pathways for aerosol and chemical feedbacks in Earth system models. These focus on changes in natural emissions (dust, sea salt, dimethyl sulfide, biogenic volatile organic compounds (BVOCs) and lightning) and changes in reaction rates for methane and ozone chemistry. The feedback terms are then given by the sensitivity of a pathway to climate change multiplied by the radiative effect of the change.
We find that the overall climate feedback through chemistry and aerosols is negative in the sixth Coupled Model Intercomparison Project (CMIP6) Earth system models due to increased negative forcing from aerosols in a climate with warmer surface temperatures following a quadrupling of CO2 concentrations. This is principally due to increased emissions of sea salt and BVOCs which are sensitive to climate change and cause strong negative radiative forcings. Increased chemical loss of ozone and methane also contributes to a negative feedback. However, overall methane lifetime is expected to increase in a warmer climate due to increased BVOCs. Increased emissions of methane from wetlands would also offset some of the negative feedbacks. The CMIP6 experimental design did not allow the methane lifetime or methane emission changes to affect climate, so we found a robust negative contribution from interactive aerosols and chemistry to climate sensitivity in CMIP6 Earth system models.
- Article
(1349 KB) - Full-text XML
-
Supplement
(2500 KB) - BibTeX
- EndNote
Climate feedback quantifies the change in the Earth's radiation budget as the surface temperature varies. Overall, this feedback must be negative for a stable climate; i.e. the net radiation budget must decrease as surface temperature increases. The dominant negative feedback comes from increased longwave emissions from a warmer surface (Planck response). Warmer surface temperatures lead to changes in the physical climate system (water vapour, lapse rate, surface albedo, clouds) that further modify the radiation budget, contributing additional positive and negative feedbacks (Sherwood et al., 2020). Earth system models (ESMs) extend the complexity of physical climate models by coupling land and ocean biospheres, atmospheric chemistry and aerosols to the physical climate. Within these models, natural processes, chemical reactions and biological transformations respond to changes in climate, and these processes in turn affect the climate. Therefore, the physical climate system and the biogeochemical cycles are coupled, leading to climate feedbacks that may act to further amplify or dampen the climate response to a climate forcing (Arneth et al., 2010; Ciais et al., 2013; Heinze et al., 2019). The importance of biogeochemical feedbacks has long been recognised for the longer timescales involved in palaeoclimate studies, but the realisation of their relevance in the context of anthropogenic climate change is more recent. A multitude of biogeochemical feedbacks have been identified, but the evaluation of their importance for future climate change remains very limited. A recent review of Earth system feedbacks (Heinze et al., 2019) examined the extensive range of feedbacks possible in an Earth system framework. The largest biogeochemical feedback contribution comes from the carbon cycle (Friedlingstein, 2015). Arneth et al. (2010) considered a range of terrestrial biogeochemical feedbacks interacting with the carbon cycle. O'Connor et al. (2010) reviewed potential feedbacks involving methane. Carslaw et al. (2010) reviewed climate feedbacks involving natural and anthropogenic aerosols. Climate change can impact both the source strength of natural aerosols such as sea salt, dust, biomass burning aerosols or their precursors (dimethyl sulfide (DMS), biogenic volatile organic compounds) and the lifetime of natural and anthropogenic aerosols through changes in transport and dry and wet deposition (Bellouin et al., 2011; Raes et al., 2010). Here, we choose to focus especially on those feedbacks that are mediated through changes in the abundances of reactive gases and aerosols, using data from CMIP6 (Coupled Model Intercomparison Project phase 6) (Eyring et al., 2016) Earth system models that conducted the AerChemMIP (Aerosols and Chemistry Model Intercomparison Project) simulations (Collins et al., 2017).
Note that in this paper we use change in global mean surface temperature as our measure of climate change and for simplicity assume changes in other climate variables are proportional to this. For many of the forcing agents considered here, the forcing pattern varies strongly on regional scales and would be expected to cause larger regional temperature changes than represented by the global mean.
In Sect. 2, we describe the theoretical framework used to diagnose the feedbacks. In Sect. 3, we describe how the different Earth system models implement the biogeochemical processes. Section 4 quantifies the feedbacks as implemented in the models and compares these results with previous modelling and theoretical studies. Section 5 concludes the paper. The Supplement contains further details of the models used and additional figures to support the analysis in Sect. 4.
2.1 Theory
In order to compare climate feedbacks, we need to compare them on a common scale of the change in the top-of-atmosphere radiation balance following a unit warming (in W m−2 K−1) (e.g. Gregory et al., 2009). Following Gregory et al. (2004), the radiative imbalance ΔN from an imposed forcing ΔF is given by
where ΔT is the global mean change in surface temperature and α is the climate feedback parameter (). The total derivative can be split into a set of partial derivatives:
where the αi indicates the individual feedback terms due to a change in a climate variable Ci. For feedbacks involving changes in composition, the ΔCi can represent changes in reactive gas or aerosol burdens or emissions. can then be expressed as ϕiγi, where ϕi is the radiative efficiency of the species per burden (W m−2 Tg−1) or per emission (W m−2 (Tg yr−1)−1), and γi is the change in species burden or emission with climate (Tg K−1 or Tg yr−1 K−1). The radiative efficiencies are based on effective radiative forcing (ERF) (Myhre et al., 2013a) to include rapid adjustments to changes in composition. Since climate change can also affect the atmospheric lifetime of a species, does not necessarily scale with .
2.2 Applying the theory to Earth system models
With Earth system models, the ϕi and γi coefficients can be diagnosed from idealised simulations in which only climate or composition are changed. Here, we use the set of simulations specified under the CMIP6 project (Eyring et al., 2016).
The γi values are diagnosed from a pair of idealised climate change scenarios: a control climate (piControl) where composition is maintained at a level representative of 1850 conditions, and a warmer climate (abrupt-4xCO2) where temperatures have increased following an abrupt quadrupling of CO2. To quantify the sensitivities to this temperature change, we take the 30-year time means from years 121–150 of these simulations for both the surface temperature change and the burden or emission changes. The global mean surface temperature changes are therefore not the same as the model-based equilibrium climate sensitivity (ECS) calculations but are temperatures consistent with the averaging period for the burden or emissions. The γi are calculated from the change in emission or burden divided by the temperature change. For the dust and sea salt (these are the aerosols with single sources), rather than the burden, we diagnose the aerosol optical depth (AOD) change (K−1), where available, as being the quantity most closely related to the radiative forcing (Myhre et al., 2013b). For DMS and organic aerosol emissions, we use the emission change (Tg yr−1 K−1), as changes in aerosol lifetime will also affect AODs from other sources of sulfate and organic aerosol (OA) that we do not have ERF calculations for. For reactive gases, both emissions-based and concentration-based calculations are used. CO2 can have climate effects beyond its global warming; for instance, CO2 directly cools the stratosphere and can affect vegetation with implications for dust and biogenic volatile organic compound (BVOC) emissions. With the AerChemMIP setup, it is not possible to distinguish these adjustments to CO2 concentration from the impacts of surface temperature increase.
The ϕi coefficients for changes in emissions are derived from pairs of the AerChemMIP simulations defined in Collins et al. (2017): piClim-control where composition and climate are maintained at a level representative of 1850 conditions, and experiments piClim-2x (Table 1) in which individual natural emission fluxes are doubled. The climate change in these simulations is restricted by using fixed sea surface temperatures and sea ice cover (Collins et al., 2017) for a 30-year mean of the piControl simulation. The ERFs are determined by the mean difference in top-of-atmosphere radiative fluxes between the piClim-2x and the piClim-control experiments over a 30-year period. The ϕi values are calculated from the ERF divided by either the change in AOD or change in emissions, depending on the units of γi above. The specific simulation variant numbers are listed in Table S2 in the Supplement.
Table 1List of simulations for diagnosing ERFs of natural emitted species. The specified natural emission fluxes are doubled compared to the 1850 control.
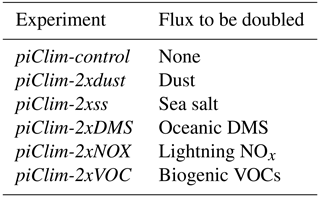
The theoretical framework in Sect. 2.1 is inherently linear, whereas the Earth system may well not be. The climate changes used to diagnose γi are of the order 4–7 K (Table 5), which are much larger than the remaining ∼0.5–1 K goals of the Paris Agreement. The doubled natural emission changes used to diagnose ϕi are larger than the changes found in the 4xCO2 experiments and larger still than those expected from a climate following the Paris goals.
For , the ozone radiative forcing (Tables 10 and 11) is diagnosed from the changes in the 3-D ozone distributions multiplied by a 3-D kernel of ozone radiative efficiencies from Skeie et al. (2020). The uncertainty in radiative transfer modelling was estimated to be only 10 % in Stevenson et al. (2013), but we increase that to 15 % as a conservative estimate comparable to the 14 % radiative modelling uncertainty for methane (Etminan et al., 2016). Radiative modelling uncertainties are negligible compared to the other uncertainties in Sect. 4.
The ESM setups here, even with tropospheric chemistry, still constrain methane to specified concentrations at the surface. This means that any feedbacks mediated through changes in oxidising capacity have a negligible effect on methane. It is, however, possible to diagnose the change in methane that would be expected, if it were not constrained, from the change in its lifetime:
where C is the methane concentration, τ is the total methane lifetime (including loss to soils), and f is the feedback of methane on its own lifetime (Fiore et al., 2009). The effective radiative forcing from the change in concentration is W m−2 ppb−1, calculated using the formula from Etminan et al. (2016) from a methane baseline of 802 ppb representative of 1850 (Myhre et al., 2013a); this is scaled by 1.52 to account for the additional chemical production of ozone (0.4) and stratospheric water vapour (0.12). These values are reduced from 0.5 and 0.15 in Myhre et al. (2013a) (their Sect. 8.SM.11.3.2) as the 25 % increase in radiative efficiency from Etminan et al. (2016) does not affect the ozone or water vapour. This gives 1.11 W m−2 per fractional change in methane lifetime or 0.011 W m−2 %−1. Changes in methane concentration due to changes in emissions ΔE are given by
where τ=9.1 years (Prather et al., 2012), and f=1.34 (Myhre et al., 2013a). mair and are the relative molecular masses of air and methane (28.97 and 16.0).
3.1 Model implementation of aerosols, tropospheric and stratospheric chemistry
We use results from seven Earth system models that contributed simulations under the AerChemMIP piClim-2x experimental setup. All seven models have interactive aerosol schemes and five have interactive stratospheric chemistry of which four also have interactive tropospheric chemistry (Table 2). The level of sophistication of the chemistry can affect the modelled responses to the emissions of reactive gases. For instance, in models without interactive tropospheric chemistry, changes in BVOCs affect only organic aerosols, whereas in models with interactive tropospheric chemistry, they also affect ozone, methane lifetime and potentially the oxidation of other aerosol precursors. For each model, one ensemble member was run for each experiment.
3.2 Model implementation of natural emissions of aerosols and ozone precursors
3.2.1 Land
The land-based natural emissions analysed here are dust, BVOCs and wetland methane (Table 3).
Table 3Levels of complexity of vegetation included in the land-based emissions schemes of dust and BVOCs for the ESMs, including dependence on photosynthetically active radiation (PAR) and leaf area index (LAI).
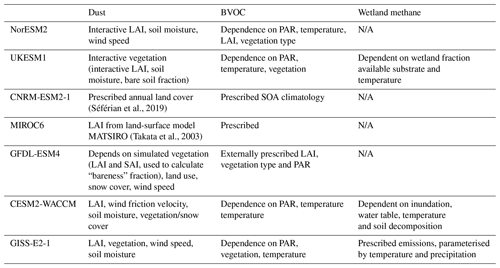
“N/A” signifies that the given diagnostic was not available from that model.
Dust emissions are parameterised as a function of surface wind speeds or wind stress, and account for the amount of bare soil, soil type and aridity (Ackerley et al., 2012; Collins et al., 2011; Evan et al., 2014; Fiedler et al., 2016; Huneeus et al., 2011; Shao et al., 2011; Zender et al., 2004). There is a variation between the models in the sizes considered, whether binned or modal, and the optical properties of the dust particles (Kok et al., 2018; Xie et al., 2018). Table S1 lists the parameterisations for desert-dust aerosol for the contributing models and the simulated dust–aerosol sizes.
BVOC emissions are parameterised as a function of vegetation type and cover, and also temperature and photosynthesis rates (gross primary productivity) (Guenther et al., 1995; Pacifico et al., 2011; Sporre et al., 2019; Unger, 2014). Some parameterisations also include dependence on CO2 concentrations (Pacifico et al., 2012). Models differ in the speciation of the VOCs emitted but typically include isoprene and monoterpenes, with different emission parameterisations for different species. The ability of VOCs to form secondary organic aerosol is typically parameterised as a fixed yield (Mulcahy et al., 2020). For further details, see Table S1 in the Supplement and references therein.
3.2.2 Marine
The ocean emissions analysed here are sea salt, DMS and primary organic aerosols (Table 4).
The air–sea exchange processes for these emissions are parameterised as a function of wind speed and sometimes temperature (Gong, 2003; Jaeglé et al., 2011).
Changes in DMS emissions can be initiated by various factors such as changes in temperature, insolation, depth of the ocean-mixed layer, sea ice extent, wind strength, nutrient recycling or shift in marine ecosystems (Heinze et al., 2019). The DMS fluxes into the atmosphere are prescribed in some models (CNRM-ESM2-1, GFDL-ESM4, MIROC6, CESM2-WACCM) and calculated interactively from ocean biogeochemistry in others (UKESM1, NorESM2). Further details on the current generation of marine biogeochemical models, including the representation of DMS emission scheme, can be found in Séférian et al. (2020). Oceanic organic aerosol emissions are also wind-speed dependent and in addition depend on chlorophyll concentrations generated either from interactive biogeochemistry or observation-based chlorophyll concentrations in models without ocean biogeochemistry components.
3.2.3 Lightning
The models with tropospheric chemistry (UKESM1, GFDL-ESM4, CESM2-WACCM, GISS-E2-1) all include parameterisations of the emission of nitrogen oxides (NOx) from lightning, related to the height of the convective cloud top (Price et al., 1997; Price and Rind, 1992). The lightning frequency depends strongly on the convective cloud-top height, and the ratio of cloud-to-cloud vs. cloud-to-ground lightning depends on the cold cloud thickness (from 0 ∘C to the cloud top). The precise implementation of lighting emissions and their height profile varies between the models.
The feedbacks in this section are all derived from the difference between the piControl and abrupt-4xCO2 CMIP6 experiments. The Earth system models all respond with different levels of climate change, so all climate feedbacks are normalised to the change in global mean surface temperature between abrupt-4xCO2 and piControl for the 30-year period (years 121–150; Table 5) to derive the γi (Sect. 2.1). There is a factor of nearly 2 between the temperature responses of the models. Since this time frame is not long enough for the models to have reached equilibrium (which may take many centuries), these temperatures are not the same as ECS.
Table 5Change in global mean surface temperature following an abrupt quadrupling of CO2 concentrations. The difference between abrupt-4xCO2 and piControl is averaged over the years 121–150. Uncertainties refer to the standard deviation of the interannual variability.

4.1 Aerosol species
4.1.1 Desert dust
The 2xdust perturbation is applied by scaling the parameterisation in the emission scheme. Since changing dust emissions will affect the boundary layer meteorology, the net effect is not an exact doubling of the emissions (Table 6). Four of the six models in AerChemMIP have a negative radiative forcing for doubled dust (Figs. 1a, S2–S4, Table 6). The models all agree on a negative ERF over the oceans close to the source regions. They differ in the sign of the ERF over the deserts themselves, with most (four out of six) showing a positive longwave ERF (Fig. S4). The shortwave ERF is more variable (Fig. S3) and is also affected by any changes in low cloud amount. For CNRM-ESM2-1 and UKESM1, this positive ERF over the deserts outweighs the oceanic negative ERF. The ERF for GFDL-ESM4 is not significantly different from zero. UKESM1 has by far the largest dust emissions (and change from doubling) because it includes particles that are emitted and deposited in the same time step. CNRM-ESM2-1 also includes large particles (up to 20 µm). These models, however, have similar changes in dust AOD compared to the other models, and hence the magnitude of the forcing efficiency per change in AOD (Table 6) is not out of line with the others. MIROC6 has the strongest forcing even with the lowest emissions and smallest change in AOD, thus giving it the largest forcing efficiency per AOD.
Table 6Dust radiative efficiencies by emission and AOD from 2xdust experiments. Changes in emissions and AOD are from abrupt-4xCO2. “Scaled” refers to scaling the 2xdust relations between AOD and emissions by the 4xCO2 changes in emissions. The α values are calculated assuming ERF is proportional to emissions or AOD. Uncertainties for each model are errors in the mean based on interannual variability. Uncertainties in the multi-model results are standard deviation across the models. The multi-model α terms are the average of the individual model α rather than the product of the multi-model ϕ and γ. Multi-model means are not shown for the emissions as some models include coarse particles, whereas others do not.
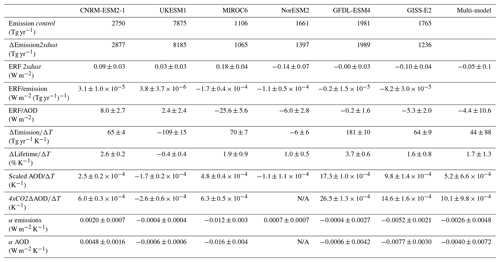
“N/A” signifies that the given diagnostic was not available from that model.
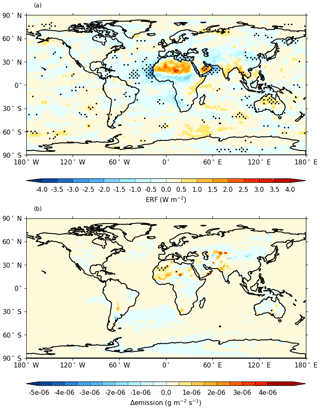
Figure 1Multi-model mean (a) ERF from piClim-2xdust vs. piClim-control, (b) change in dust emissions for abrupt-4xCO2 vs. piControl. Stippling shows areas where the mean changes by more than the standard deviation across models.
The response of dust aerosols to abrupt-4xCO2 (Figs. 1b, S1) is substantially different across the model ensemble. Four models (CNRM-ESM2-1, MIROC6, GFDL-ESM4 and GISS-E2) show an increase in dust emission in a 4xCO2 climate due to increased aridity and near-surface wind speeds, whereas UKESM1 has a decrease in dust emissions with more CO2 due to increased fertilisation of the vegetation (hence less bare soil) paired with decreased near-surface winds. NorESM2 shows near-zero change. The spatial pattern of the opposing response of dust emission to 4xCO2 in the two most extreme models, UKESM1 and CNRM-ESM2-1, is consistent with the responses in near-surface wind speed to 4xCO2 (Fig. S5). These reflect larger increases in mean winds over regions where the mean emission amount is larger for 4xCO2 compared to the pre-industrial climatology. The increase or decrease in winds is also likely to be affected by changes in vegetation in semi-arid regions, e.g., the Sahel.
As well as affecting the emissions, changing climate can also affect the removal of dust through changes in both dry and wet deposition. In all models except UKESM1, the lifetime of dust increases (Table 6). The effect of an increase in lifetime can be seen by comparing the change in AOD. The modelled changes in dust AOD in the abrupt-4xCO2 experiment are a factor of 1.5–2 larger (for those models where lifetime increases) as would be expected assuming a linear scaling with emissions across all size ranges (“scaled AOD” in Table 6).
The climate feedback parameter for dust (α) is given by the product of the radiative efficiencies (ϕ) with the sensitivities to climate (γ). These vary from −0.012 to +0.0020 W m−2 K−1 with a multi-model mean of W m−2 K−1, i.e. consistent with zero. Scaling with AOD change rather than emission change gives a slightly larger magnitude, with a range of −0.016 to +0.0048 W m−2 K−1 and a multi-model mean of W m−2 K−1. Although some models obtain similar feedback terms, this is not necessarily for the same reason. For instance, GFDL-ESM4 and NorESM2 have small feedback terms. NorESM2-LM has a large ERF for doubled dust emissions but a small change in dust emission for 4xCO2, whereas GFDL-ESM4 has a large change in emissions but a small ERF.
Dust–aerosol feedback assessments are a relatively new area of research due to the large uncertainties of climate models in simulating dust aerosols with changes in atmospheric composition. For instance, the spread in model estimates for dust aerosol changes in the 21st century is the largest among wildfires, biogenic SOA and DMS sulfate (Carslaw et al., 2010). Predictions for future dust emission range from an increase (Woodward et al., 2005) to a decrease (Mahowald and Luo, 2003). The modelled feedbacks in Table 6 are smaller in magnitude compared to the theoretical model estimates of −0.04 to +0.02 W m−2 K−1 by Kok et al. (2018).
The model ranges in dust forcing and feedbacks are not surprising in light of past studies that highlight model differences in dust-emitting winds and dust–aerosol parameterisations that contribute to the model diversity in the dust–aerosol loading, optical properties and radiative effects (Ackerley et al., 2012; Evan et al., 2014; Huneeus et al., 2011; Shao et al., 2011; Zender et al., 2004). For instance, the parameterisation of the planetary boundary layer plays an important role in determining the dust loading, forcing and regional feedbacks on winds (Alizadeh Choobari et al., 2012). Influencing factors for regional differences in the dust radiative effects are the surface albedo and aerosol size distribution (Kok et al., 2018; Xie et al., 2018), whereas feedbacks on winds depend also on meteorological factors (Heinold et al., 2008). The substantial model differences in the dust emission response to 4xCO2 paired with corresponding differences in mean 10 m wind speed in this study suggest that also the dust feedback parameter critically relies on accurately simulating atmospheric dynamics. Modelling atmospheric circulation has been identified as a grand challenge in climate research (Bony et al., 2015). Currently, we have no estimate which of the dust feedbacks shown are the most plausible, because convective dust storms are missing in such models, but this dust storm type is believed to be important for north African dust emissions (Heinold et al., 2013). Moreover, natural aerosol–climate feedbacks are thought to depend on the anthropogenic aerosol burden and might therefore be both time-dependent and underestimated in the present-day polluted atmosphere (Spracklen and Rap, 2013). Taken together, we have low confidence in the feedback estimates for dust aerosols to increases in atmospheric concentrations of greenhouse gases.
4.1.2 Sea salt
All models show a strong negative forcing to double sea salt emissions (Figs. 2a, S7, Table 7), although the ERF for MIROC6 is considerably smaller than for the others. The emissions and mass loading for the CNRM-ESM2-1 model are approximately 20 times those of the other models, largely due to including a size bin up to 20 µm. This coarse bin contains a large mass but a lower number of particles, so the AOD change is similar to other models. All models show a similar forcing efficiency per AOD change. All models show an increase in sea salt emissions in the Southern Ocean in 4xCO2 (Figs. 2b, S6) due to increased wind speeds, with a general tendency for decreases elsewhere due to rising temperatures (Jaeglé et al., 2011). The global mean change in emissions is positive in all models except MIROC6 and GISS-E2-1 (where the lower-latitude decreases outweigh the high-latitude increases). For models showing an increased sea salt lifetime in a 4xCO2 climate, the modelled increase in AOD is larger than that expected from scaling the emissions change (“scaled AOD” in Table 7). Although emissions (and the mass burdens) of sea salt decrease in MIROC6 and GISS-E2-1, the AODs increase. The mean feedback is W m−2 K−1 based on emissions and W m−2 K−1 based on the increase in AOD. The signs are consistently negative, except for the emission-based feedbacks for MIROC6 and GISS-E2-1.
Table 7Radiative efficiencies by emission and AOD from 2xss (sea salt). Changes in emissions and AOD are from 4xCO2. “Scaled” refers to scaling the 2xss relations between AOD to emissions by the 4xCO2 changes in emissions. α values are calculated assuming ERF is proportional to emissions or AOD. Uncertainties for each model are errors in the mean based on interannual variability. Uncertainties in the multi-model results are the standard deviation across the models. The multi-model α terms are the average of the individual model α rather than the product of the multi-model ϕ and γ. Multi-model means are not shown for the emissions as these are so variable.
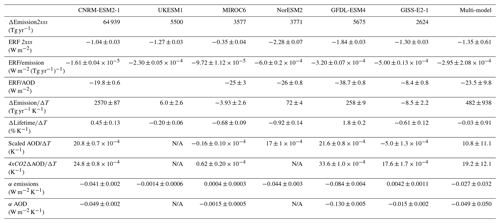
“N/A” signifies that the given diagnostic was not available from that model.
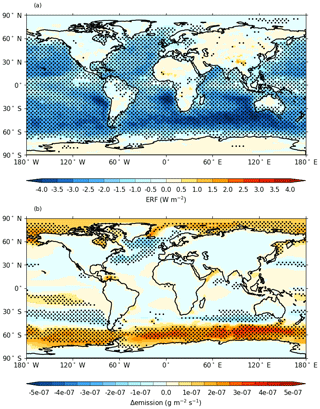
Figure 2Multi-model mean (a) ERF from piClim-2xss vs. piClim-control, (b) change in sea salt emissions for abrupt-4xCO2 vs. piControl. CNRM-ESM2-1 emissions are excluded from the multi-model emissions in panel (b) as they include a coarse bin which dominates. Stippling shows areas where the mean changes by more than the standard deviation across models.
4.1.3 DMS
Four models ran the 2xDMS experiment. Interactive biogeochemistry or interactive DMS emissions are not a prerequisite for the 2xDMS experiment. However, interactive emissions are required to calculate a feedback α; hence, we exclude CNRM-ESM2-1 from Table 8. Two models include interactive ocean biogeochemistry (UKESM1 and NorESM2). The ERF for 2xDMS is negative for all three models that ran this experiment (Figs. 3a, S9, Table 8), though less strongly so for GISS-E2-1. UKESM1 and NorESM2 show a decrease in sulfur emissions in 4xCO2, where the tropical decrease more than compensates for the increase along the edge of the sea ice retreat, whereas GISS-E2-1 shows an increase in overall sulfur emissions. The multi-model mean is shown in Fig. 3b and the individual models in Fig. S8. The multi-model mean emission-based α is slightly positive but consistent with zero. In spite of decreased DMS emissions in UKESM1 and NorESM2, there is an increased sulfur mass in all models in the 4xCO2 simulation due to an increase in the sulfate lifetime of around 2 % K−1. Since this lifetime change applies to all sulfate, not just that from DMS, the radiative efficiency from 2xDMS will not necessarily apply, and we do not calculate an AOD or mass-based feedback, but note that it would be negative.
Table 8Radiative efficiencies by emissions from 2xDMS. Changes in emissions are from the 4xCO2 experiment. α values are calculated assuming ERF is proportional to emissions. Uncertainties for each model are errors in the mean based on interannual variability. Uncertainties in the multi-model results are the standard deviation across the models. The multi-model α terms are the average of the individual model α rather than the product of the multi-model ϕ and γ.
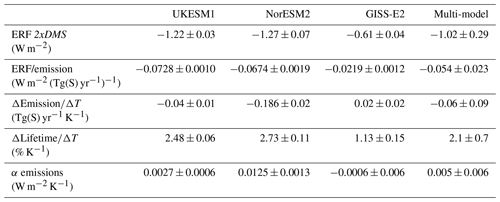
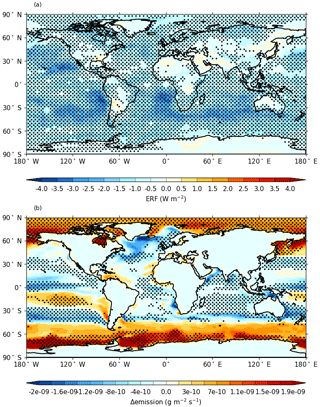
Figure 3Multi-model mean (a) ERF from piClim-2xDMS vs. piClim-control, (b) change in DMS emissions (in g(S)) for abrupt-4xCO2 vs. piControl. Stippling shows areas where the mean changes by more than the standard deviation across models.
DMS is produced by marine biological activity in the ocean, and it is assumed to be the largest natural source of sulfur to the atmosphere. Up to now, there has been no comprehensive model effort to include all the important effects, and therefore the DMS emission strength change under climate change is still uncertain. The slightly positive mean here is in contrast to the −0.02 W m−2 K−1 feedback from AR5 (Ciais et al., 2013) based on results from only one model (HadGEM2-ES).
Modelling studies including ocean biogeochemistry have shown that under climate change, an increased stratification of the ocean at low and midlatitudes leads to a reduction in nutrients supply into the surface ocean and thus a reduction in DMS emissions, whereas at high latitudes, retreat of sea ice can lead to increased biological activity and increase in DMS production (Kloster et al., 2007). Previous models which include ocean biogeochemistry have shown a slight increase in DMS production and emission to the atmosphere in a warming climate (Bopp et al., 2004; Gabric et al., 2004; Gunson et al., 2006; Vallina et al., 2007).
Some more recent studies have included the impact of ocean acidification on ocean DMS production (Schwinger et al., 2017; Six et al., 2013). Both studies used a very similar description of the ocean biogeochemistry and extended it with an observationally based relation between ocean alkalinity and ocean DMS production. Assuming a medium sensitivity of the DMS production on pH, Six et al. (2013) found a global DMS emission decrease by 18 % in 2100 under the Special Report on Emissions Scenarios (SRES) A1B scenario, and Schwinger et al. (2017) found an emission reduction by 31 % in 2200 under the Representative Concentration Pathways (RCP) 8.5 scenario. In addition, recent work has provided evidence for major pathways in the oxidation of DMS in the atmosphere which are not included in any of these ESMs (Berndt et al., 2019; Wu et al., 2015).
4.1.4 Organic aerosol
Biogenic VOC emissions lead to both organic aerosol and ozone production (in those models with tropospheric chemistry). It is therefore necessary to distinguish the two in the ERFs in these models. The ozone stratospheric-temperature-adjusted radiative forcing (SARF) from the ozone changes is diagnosed offline (Sect. 2.1). This is subtracted from the ERF to give the ERF due to aerosols only as shown in Table 9 (ozone is the only non-aerosol forcing agent that varies). For NorESM2, there is no ozone change. The ERF before subtracting the ozone SARF is shown in Fig. 4. These estimated aerosol forcing changes are large (up to −0.69 W m−2). All the ERF-SARF values are negative, apart from UKESM1, which has a large positive forcing from cloud changes (diagnosed from comparing all-sky and clear-sky diagnostics; not shown).
Table 9Non-O3 ERF (subtracting the O3 SARF from Table 10; for NorESM2, there is no O3 change). Radiative efficiencies by emission of BVOC from 2xVOC. Changes in emissions of BVOC are from the 4xCO2 experiment. α values are calculated assuming ERF is proportional to emissions. Uncertainties for each model are errors in the mean based on interannual variability. Uncertainties in the multi-model results are the standard deviation across the models. The multi-model α terms are the average of the individual model α rather than the product of the multi-model ϕ and γ.
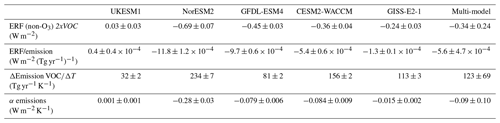
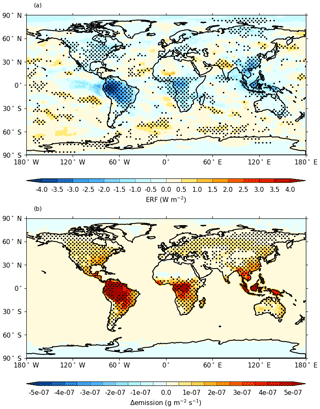
Figure 4Multi-model mean (a) ERF from piClim-2xVOC vs. piClim-control, (b) change in BVOC emissions for abrupt-4xCO2 vs. piControl. Stippling shows areas where the mean changes by more than the standard deviation across models.
In terms of aerosol, there is an increase in OA mass and expected increase in AOD with a very similar spatial pattern when the emission of BVOCs is doubled. The patterns of BVOC increase for the 4xCO2 experiments are much more similar between models (Fig. S10) in terms of pattern and sign than for the previous species (dust, sea salt, DMS), although the magnitude is considerably lower for UKESM1. In the 4xCO2 experiments, these models also simulate an increase in primary organic aerosol emissions from the ocean which adds to the OA mass on top of the effect of BVOC emissions. The feedback factors are negative, apart from UKESM1, and are very strong in some models (NorESM2 with −0.28 W m−2 K−1).
4.2 Ozone and methane feedbacks
4.2.1 Biogenic VOCs
The ozone SARF is diagnosed offline (Sect. 2.1) and shown in Table 10. For all except UKESM1, the magnitude of the ozone forcing is smaller than that for aerosols, leading to a net negative ERF from BVOCs. For UKESM1, the non-ozone forcing is positive (Sect. 4.1.4) and the ozone adds to this. The ozone SARF per Tg VOC emission is similar between the models with CESM2-WACCM slightly lower. The overall feedback is therefore dominated by the variation in the sensitivity of BVOC emissions to climate. This ranges from 0.005 W m−2 K−1 for UKESM1, which has the lowest BVOC increase with climate, to 0.014 W m−2 K−1 for CESM2-WACCM and GISS-ES-1, which have the strongest BVOC response to climate.
Table 10Ozone SARF and radiative efficiencies for 2xVOC emissions. Changes in emissions are from the 4xCO2 experiment. α values are calculated using the ozone SARF. Uncertainties for each model are errors in the mean based on interannual variability and assuming a 15 % uncertainty in the ozone radiative efficiency (Sect. 2.2). Uncertainties in the multi-model results are the standard deviation across the models.
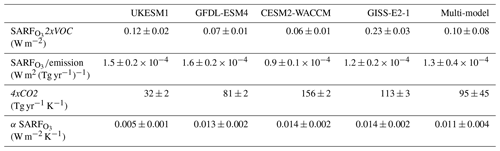
Table 11ERF and ozone SARF radiative efficiencies for 2xNOX lightning NOx emissions. Changes in emissions are from the 4xCO2 experiment. α values are calculated assuming ERF or ozone SARF. Uncertainties for each model are errors in the mean based on interannual variability and assuming a 15 % uncertainty in the ozone radiative efficiency (Sect. 2.2). Uncertainties in the multi-model results are the standard deviation across the models.
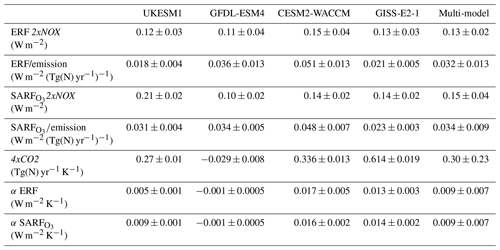
At the multi-model mean level, the cooling associated with an increase in organic aerosol ( W m−2 K−1 – for the four models with chemistry) dominates over the warming associated with an increase in O3 (0.011±0.004 W m−2 K−1), leaving an overall negative feedback.
Using multi-annual simulations of global aerosol, Scott et al. (2018) diagnosed a feedback from biogenic secondary organic aerosol of −0.06 W m−2 K−1 globally and −0.03 W m−2 K−1 when considering only extratropical regions. This global feedback value was composed of a direct aerosol radiative feedback of −0.048 W m−2 K−1 and an indirect aerosol (i.e., cloud albedo) feedback of −0.013 W m−2 K−1. Using observations from 11 sites, Paasonen et al. (2013) estimated an indirect aerosol feedback of −0.01 W m−2 K−1 due to biogenic secondary organic aerosol. The ability of models to account for changes in vegetation has a large effect on the feedback. Sporre et al. (2019) found that interactive vegetation enhanced BVOC emissions by 63 % relative to prescribed vegetation, producing more organic aerosol and an increase in (negative) aerosol forcing.
The level of compensation between increased aerosol forcing and increased ozone is dependent on the model (here positive feedback for GFDL-ESM4, negative for UKESM1 and CESM2-WACCM). Unger (2014) found a positive feedback in NASA GISS ModelE2, whereas Scott et al. (2014) found a negative feedback in HadGEM2-ES.
4.2.2 Lightning NOx
Lightning NOx leads to ozone production and changes in methane lifetime. As for BVOCs (Sect. 4.2.1), ozone radiative kernels are used to quantify the ozone SARF. The ERF and SARF agree for all models except UKESM1 (Table 11), suggesting that there is little effect on aerosols in these models. In UKESM1, NOx is known to increase the formation of new sulfate particles (O'Connor et al., 2020), partially offsetting the positive ozone forcing. The SARF per Tg emission varies by a factor of 2 (0.023 to 0.048 W m2 (Tg(N) yr−1)−1) between the highest and lowest values.
The changes in lightning NOx emissions vary widely across the models, with three showing increases (UKESM1, CESM2-WACCM, GISS-E2-1) but a slight decrease in GFDL-ESM4. Although they all use variations on the cloud-top-height schemes (Sect. 3.2.3), the differences in how this is implemented and how the modelled clouds vary with climate change all affect the emission response. The feedback is positive for the three models with increased lightning (0.009 to 0.016 W m−2 K−1), based on the ozone changes, but slightly negative for GFDL-ESM4 (−0.001 W m−2 K−1). Including the aerosol response to lightning for UKESM1 would reduce its feedback to 0.005 W m−2 K−1, but this seems to be particular to this model.
The ESMs used in CMIP6 all use a cloud-top-height parameterisation of lightning. Such schemes have previously been found to increase lightning production in warmer climates, whereas more sophisticated schemes based on convective updraft mass flux or ice flux show decreases in lightning with temperature (Clark et al., 2017; Finney et al., 2016b, 2018). The result from the Atmospheric Chemistry and Climate Model Intercomparison (ACCMIP) of 0.44 Tg(N) yr−1 K−1 (Finney et al., 2016a) lies within the range of the models with increased lightning under 4xCO2 (0.27 to 0.61 Tg(N) yr−1 K−1).
4.2.3 Methane lifetimes
BVOC and NOx emissions also affect the methane lifetime. Methane does not change in the AerChemMIP experimental setup, but the methane changes that would be expected if methane were allowed to evolve freely can be diagnosed from the change in methane lifetime. The methane lifetime to OH (troposphere and stratosphere) is diagnosed in the models. The losses to chlorine oxidation and soil uptake are assumed to be 11 and 30 Tg yr−1, respectively (Saunois et al., 2020). All models show an increase in methane lifetime with BVOC emissions (0.018–0.035 % (Tg(VOC)yr−1)−1 and a decrease due to lightning NOx emissions (−2.4 % to −6.8 % (Tg(VOC)yr−1)−1 (Table 12). From these, the expected lifetime changes with climate can be deduced from the changes in emissions with temperature. These lifetime changes are then converted to feedbacks using the radiative efficiency (including impacts on ozone and stratospheric water vapour) for methane lifetime changes in Sect. 2.2 (0.011 W m−2 %−1). The feedbacks range from 0.012 to 0.061 W m−2 K−1 for BVOCs and −0.042 to +0.001 W m−2 K−1 for lightning NOx, where the variability is mostly due to the different sensitivities of BVOC or lightning emissions to climate in the models. For BVOC, the methane lifetime feedback is larger than that due to ozone production, thus increasing the overall feedback. For lightning NOx, the methane lifetime feedback is of opposite sign to that from ozone production, with approximate compensation for UKESM1 and GFDL-ESM4 (net 0.002 and 0.000 W m−2 K−1, respectively) and an overall negative lightning feedback from CESM2-WACCM and GISS-E2-1 (−0.009 and −0.028 W m−2 K−1, respectively). For UKESM1, a feedback of −0.004 W m−2 K−1 could be added to the total lightning feedback to account for the increase in sulfate.
Table 12Percentage change in methane lifetime for BVOC and lightning NOx emissions. Estimated change in lifetime following changes in BVOC and NOx emissions from the 4xCO2 experiment. α values are calculated assuming a radiative efficiency of 0.015 W m−2 %−1. Uncertainties for each model assume a 14 % uncertainty in the methane radiative efficiency (Etminan et al., 2016). Uncertainties in the multi-model results are the standard deviation across the models.
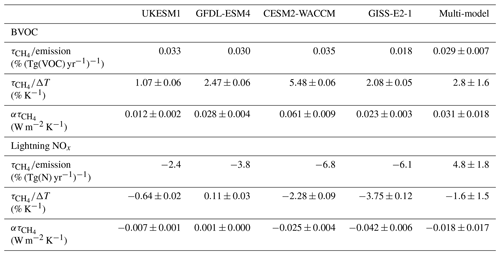
4.2.4 Wetland emissions
Two models diagnosed changes in wetland emissions due to 4xCO2. Although the wetland emissions do not directly affect methane concentrations in the model, changes in emissions can be converted to concentration changes (Sect. 2.2). UKESM1 and CESM2-WACCM, both of which are models with interactive wetland emissions, show strong responses to climate change (Table 13), leading to a feedback of 0.16±0.03 W m−2 K−1.
Wetland emissions are more strongly sensitive to CO2 concentrations than to temperature or precipitation (Melton et al., 2013), so the values presented here are more likely to be “adjustments” to the CO2 rather than feedbacks and hence could be considered part of the CO2 ERF. We find emission increases following quadrupled levels of CO2 of 130 %–160 %. This compares with results from the Wetland and Wetland CH4 Inter-comparison of Models Project (WETCHIMP) of 20 %–160 % following an increase in CO2 of a factor of 2.8 (Melton et al., 2013). The CMIP6 simulation specifications do not include free-running methane concentrations; therefore, the effects of these increased wetland emissions will not be realised in any of the CMIP6 experiments. Outside CMIP6, ESMs are starting to include free-running methane (Ocko et al., 2018), so for these it will be important to understand the effects of changing CO2 and meteorology on wetland emissions.
Table 13Sensitivity of wetland emissions to 4xCO2 in two models. Feedback parameter assuming pre-industrial conditions. Uncertainties for each model assume a 14 % uncertainty in the methane radiative efficiency (Etminan et al., 2016). Uncertainties in the multi-model results are the standard deviation across the models.
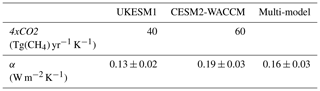
4.2.5 Meteorological drivers
As well as through changes in natural emissions, climate change can affect ozone burden and methane lifetime directly, as the production and loss reactions are sensitive to temperature and water vapour (Johnson et al., 2001). Here, we add the expected changes in ozone SARF and methane lifetime due to changes in BVOCs and lightning NOx from Sect. 4.2.1 and 4.2.2 and compare those to the changes diagnosed from the 4xCO2 experiments (Table 14). Since lightning NOx and BVOCs are the dominant climate-sensitive emissions of (non-methane) species affecting ozone and methane, the residual is then the direct effect of climate. UKESM1, GFDL-ESM4 and GISS-E2-1 all diagnosed ozone changes for the abrupt-4xCO2 experiment (Fig. S12). All three showed decreased tropospheric ozone and increased stratospheric ozone (apart from the tropical lower stratosphere) in the 4xCO2 climate. The ozone SARF (calculated using radiative kernels) is negative, whereas the expected change from lightning NOx and BVOCs would be positive; hence, the residual attributed to meteorological changes is negative.
Table 14Comparison of expected changes in ozone SARF and methane lifetime with that diagnosed from 4xCO2. The residual is given by the difference and is converted to a feedback using radiative efficiencies for methane lifetime.
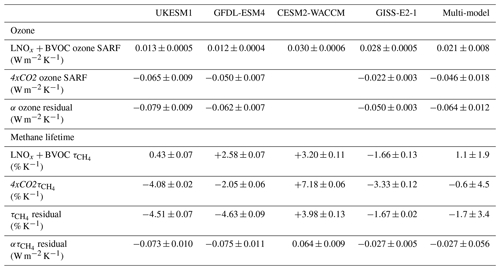
For UKESM1, GFDL-ESM4 and GISS-E2-1, the meteorological changes decrease methane lifetime, leading to an overall decrease in lifetime for the 4xCO2. In CESM2-WACCM, the meteorological changes increase methane lifetime, adding to the strong increase from BVOC emissions. This is surprising since there is no known mechanism whereby temperature and humidity increases can increase the methane lifetime. This could be due to non-linearity, whereby the effect of increased VOCs on methane lifetime is larger than expected from scaling the 2xVOC experiment.
Combining the results from ozone and methane lifetime changes leads to overall feedbacks from temperature of −0.15, −0.14 and −0.08 for UKESM1, GFDL-ESM4 and GISS-E2-1.
The three models showing decreased methane lifetime are in approximate agreement with ACCMIP, which found a sensitivity of % K−1 (Naik et al., 2013; Voulgarakis et al., 2013). ACCMIP found a variation in sign of the ozone feedback amongst models W m−2 for a 1850–2000 change in climate. The ACCMIP models generally did not include stratospheric chemistry, so they either explicitly prescribed the cross-tropopause flux of ozone or imposed a climatology of ozone above the tropopause. The four CMIP6 models here all treat the chemistry seamlessly across the troposphere and stratosphere, so the impact of changes in stratosphere–troposphere exchange (STE) of ozone on the tropospheric column is likely to be different from ACCMIP.
Changes in the stratospheric ozone following a quadrupling of CO2 are driven by cooling temperatures in the stratosphere. This is likely to be due to temperature adjustments to the stratospheric CO2 concentrations and so part of the ERF for CO2 rather than a feedback. Feedbacks and adjustments cannot be distinguished with this experimental setup.
4.3 Overall feedback
The multi-model mean feedbacks are summarised in Table 15 and Fig. 5. The totals assume that feedbacks are additive, which is the basis of the framework in Sect. 2.1. The subsets of model used to generate the multi-model means are different for each process, so the total feedback is a mixture of these different subsets. The largest individual feedbacks are due to the generation of aerosols by BVOCs ( W m−2 K−1) and the emission of methane from wetlands (0.16±0.03 W m−2 K−1). The overall uncertainty is calculated by adding the inter-model uncertainty on each feedback component in quadrature. This is dominated by the uncertainty in the aerosol response to BVOC emissions. Nearly all the feedbacks are negative, mostly because they come from an increase in aerosol emissions with temperature and increased ozone and methane removal with temperature and humidity. For BVOC emissions, the increase in aerosols outweighs the increases in ozone and methane. For lightning NOx, the decrease in methane lifetime outweighs the ozone increase. For wetland, we have attributed all the methane emission changes to temperature, whereas a significant proportion are likely to be an adjustment to CO2 concentrations rather than a feedback (Sect. 4.2.3).
Table 15Feedback parameters of all the aerosol and chemical processes addressed in this study. Uncertainties are the inter-model standard deviations.
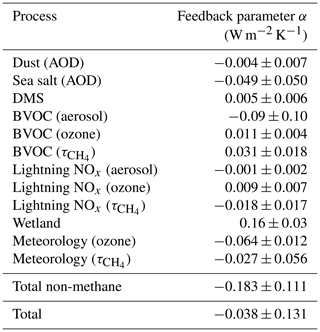
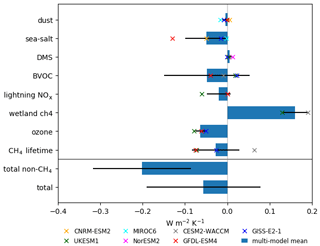
Figure 5Feedback parameters of all the aerosol and chemical processes in Table 15. Multi-model mean and individual models are shown. Uncertainties are the inter-model standard deviations. BVOC and lightning are the sum of aerosol, ozone and methane lifetime effects (points are only shown for models that include all effects). Ozone and CH4 lifetime are the chemical effects (i.e. excluding BVOC and lightning emissions). Non-CH4 is the sum and excludes methane lifetime effects and wetland feedback.
There will be additional systematic uncertainties in the overall feedback term. As described above, the use of a CO2 perturbation to generate the climate change may lead to different feedback sensitivities compared to climate change caused by other forcing agents. There will also be an uncertainty caused by using a pre-industrial baseline atmosphere rather than the present day. We are unable to quantify the likely magnitudes of these systematic uncertainties.
The ESMs that use the abrupt-4xCO2 experiment to quantify the climate sensitivity do not allow methane to vary, so we also quantify the non-methane feedbacks that will be contributing to the diagnosed climate sensitivity in these models. This feedback is significantly negative ( W m−2 K−1), suggesting the climate sensitivity of ESMs might be expected to be lower than for their physical-only counterparts. This analysis (and climate sensitivity in general) is focused on the global mean, but it should be noted that the cooling effects of increased aerosols will be heterogenous and some regions will experience less warming than a global climate sensitivity might suggest.
Earth system models include more processes than physical-only climate models. These models will inherently include additional climate feedbacks and so have a different overall climate feedback (and climate sensitivity) to their physical counterparts. In this study, we consider seven Earth system models (CNRM-ESM2-1, UKESM1, MIROC6, NorESM2, GFDL-ESM4, CESM2-WACCM and GISS-E2-1). In total, six of these (CNRM-ESM2-1, UKESM1, MIROC6, NorESM2, GFDL-ESM4 and GISS-E2-1) participated in the aerosol-related feedback experiments and four (UKESM1, GFDL-ESM4, CESM2-WACCM and GISS-E2-1) in the ozone- and methane-related feedback experiments.
We focus in this study on the responses to an abrupt forcing of quadrupled CO2 concentrations as that is the usual method to diagnose climate feedbacks. By convention, the feedbacks are quantified as a response to temperature (in W m−2 K−1), but they may not necessarily be applicable to drivers of climate change other than CO2 as some of the “feedbacks” may instead be adjustments to CO2 concentrations. It should also be noted that abrupt-4xCO2 feedbacks are based on atmospheric conditions representative of the 1850s and thus may not be applicable to future responses starting from present-day conditions. For many of the forcing agents considered here, the forcing pattern varies strongly on regional scales and would be expected to cause larger regional temperature changes than represented by the global mean. Thus, aerosol-mediated feedbacks may alter the pattern of climate response as well as the magnitude.
Here, we find that the dominant feedbacks are negative; i.e. they act to dampen the response to an imposed forcing. The total feedback, excluding inferred changes in methane, is W m−2 K−1. The increase in organic aerosols from increased emission of VOCs from vegetation makes the largest contribution to both the magnitude of the feedback and its uncertainty ( W m−2 K−1), with increases in sea salt and DMS emissions also contributing.
Contributions from increases in ozone production from biogenic VOCs and lightning NOx are offset by decreased tropospheric ozone lifetime in a warmer climate, leading to an overall negative feedback through ozone. Diagnoses of changes in wetland emissions of methane indicate that if ESMs did allow methane to vary interactively the combined aerosol and chemical feedbacks would be substantially less negative and consistent with zero.
The aerosol and chemistry feedbacks listed here contribute up to the order of −0.2 W m−2 K−1. This is smaller in magnitude than the carbon cycle response to climate (of the order of 0.5 W m−2 K−1; Ciais et al., 2013) or the physical climate feedbacks (of the order of 1–2 W m−2 K−1; Sherwood et al., 2020).
All data from the Earth system models used in this paper are available on the Earth System Grid Federation website and can be downloaded from https://esgf-index1.ceda.ac.uk/search/cmip6-ceda/ (last access: 12 November 2020, ESGF-CEDA, 2020).
The supplement related to this article is available online at: https://doi.org/10.5194/acp-21-1105-2021-supplement.
Manuscript preparation was done by WC, GT, DO, RCG, CES, SF and additional contributions from all co-authors. Model simulations were provided by DO, SB, GF, AG, LH, JFL, MM, JM, PN, VN, FO'C, RS, TT, ST and KT. Analysis was carried out by GT, WC, DO, RBS, AA, SF, RCG and JW.
The authors declare that they have no conflict of interest.
This article is part of the special issue “The Aerosol Chemistry Model Intercomparison Project (AerChemMIP)”. It is not associated with a conference.
Gillian Thornhill, William Collins, Ramiro Checa-Garcia, Martine Michou, Fiona M. O'Connor, Dirk Olivié, Michael Schulz, Ada Gjermundsen, Catherine E. Scott and Martine Michou acknowledge funding received from the European Commission's H2020 Research Infrastructures programme. Catherine E. Scott acknowledges funding from the Natural Environment Research Council. Fiona M. O'Connor, Gerd Folberth and Jane Mulcahy were supported by the Met Office Hadley Centre Climate Programme. Ada Gjermundsen, Dirk Olivié and Michael Schulz were supported by the Research Council of Norway and by Notur/NorStore. Toshihiko Takemura was supported by the Environment Research and Technology Development Fund of the Environmental Restoration and Conservation Agency, Japan; the Japan Society for the Promotion of Science (JSPS) KAKENHI; and the NEC SX supercomputer system of the National Institute for Environmental Studies, Japan.
This research has been supported by the European Commission, H2020 Research Infrastructures (CRESCENDO (grant no. 641816) and CONSTRAIN (grant no. 820829)), the Natural Environment Research Council (grant no. NE/S015396/1), the Met Office Hadley Centre Climate Programme funded by BEIS and Defra (grant no. GA01101), the Research Council of Norway (grant nos. 229771, 285003 and 285013), the Notur/NorStore (grant nos. NN2345K and NS2345K), the Environment Research and Technology Development Fund (grant no. JPMEERF20202F01) of the Environmental Restoration and Conservation Agency, Japan; the JSPS KAKENHI (grant no. JP19H05669), and the NEC SX supercomputer system of the National Institute for Environmental Studies, Japan.
This paper was edited by Holger Tost and reviewed by two anonymous referees.
Ackerley, D., Joshi, M. M., Highwood, E. J., Ryder, C. L., Harrison, M. A. J., Walters, D. N., Milton, S. F., and Strachan, J.: A comparison of two dust uplift schemes within the same general circulation model, Adv. Meteorol., 2012, 260515, https://doi.org/10.1155/2012/260515, 2012.
Alizadeh Choobari, O., Zawar-Reza, P., and Sturman, A.: Feedback between windblown dust and planetary boundary-layer characteristics: Sensitivity to boundary and surface layer parameterizations, Atmos. Environ., 61, 294–304, https://doi.org/10.1016/j.atmosenv.2012.07.038, 2012.
Archibald, A. T., O'Connor, F. M., Abraham, N. L., Archer-Nicholls, S., Chipperfield, M. P., Dalvi, M., Folberth, G. A., Dennison, F., Dhomse, S. S., Griffiths, P. T., Hardacre, C., Hewitt, A. J., Hill, R. S., Johnson, C. E., Keeble, J., Köhler, M. O., Morgenstern, O., Mulcahy, J. P., Ordóñez, C., Pope, R. J., Rumbold, S. T., Russo, M. R., Savage, N. H., Sellar, A., Stringer, M., Turnock, S. T., Wild, O., and Zeng, G.: Description and evaluation of the UKCA stratosphere–troposphere chemistry scheme (StratTrop vn 1.0) implemented in UKESM1, Geosci. Model Dev., 13, 1223–1266, https://doi.org/10.5194/gmd-13-1223-2020, 2020.
Arneth, A., Harrison, S. P., Zaehle, S., Tsigaridis, K., Menon, S., Bartlein, P. J., Feichter, J., Korhola, A., Kulmala, M., O'Donnell, D., Schurgers, G., Sorvari, S., and Vesala, T.: Terrestrial biogeochemical feedbacks in the climate system, Nat. Geosci., 3, 525–532, https://doi.org/10.1038/ngeo905, 2010.
Bauer, S. E., Tsigaridis, K., Faluvegi, G., Kelley, M., Lo, K. K., Miller, R. L., Nazarenko, L., Schmidt, G. A., and Wu, J.: Historical (1850–2014) Aerosol Evolution and Role on Climate Forcing Using the GISS ModelE2.1 Contribution to CMIP6, J. Adv. Model. Earth Sy., 12, e01978, https://doi.org/10.1029/2019ms001978, 2020.
Bellouin, N., Rae, J., Jones, A., Johnson, C., Haywood, J., and Boucher, O.: Aerosol forcing in the Climate Model Intercomparison Project (CMIP5) simulations by HadGEM2-ES and the role of ammonium nitrate, J. Geophys. Res., 116, D20206, https://doi.org/10.1029/2011JD016074, 2011.
Berndt, T., Scholz, W., Mentler, B., Fischer, L., Hoffmann, E. H., Tilgner, A., Hyttinen, N., Prisle, N. L., Hansel, A., and Herrmann, H.: Fast Peroxy Radical Isomerization and OH Recycling in the Reaction of OH Radicals with Dimethyl Sulfide, J. Phys. Chem. Lett., 10, 6478–6483, https://doi.org/10.1021/acs.jpclett.9b02567, 2019.
Bony, S., Stevens, B., Frierson, D. M. W., Jakob, C., Kageyama, M., Pincus, R., Shepherd, T. G., Sherwood, S. C., Siebesma, A. P., Sobel, A. H., Watanabe, M., and Webb, M. J.: Clouds, circulation and climate sensitivity, Nat. Geosci., 8, 261–268, https://doi.org/10.1038/ngeo2398, 2015.
Bopp, L., Boucher, O., Aumont, O., Belviso, S., Dufresne, J. L., Pham, M., and Monfray, P.: Will marine dimethylsulfide emissions amplify or alleviate global warming? A model study, Can. J. Fish. Aquat. Sci., 61, 826–835, 2004.
Carslaw, K. S., Boucher, O., Spracklen, D. V., Mann, G. W., Rae, J. G. L., Woodward, S., and Kulmala, M.: A review of natural aerosol interactions and feedbacks within the Earth system, Atmos. Chem. Phys., 10, 1701–1737, https://doi.org/10.5194/acp-10-1701-2010, 2010.
Ciais, P., Sabine, C., Bala, G., Bopp, L., Brovkin, V., Canadell, J., Chhabra, A., DeFries, R., Galloway, J., Heimann, M., Jones, C., Quéré, C. Le, Myneni, R. B., Piao, S., and Thornton, P.: Carbon and Other Biogeochemical Cycles, in Climate Change 2013: The Physical Science Basis. Contribution of Working Group I to the Fifth Assessment Report of the Intergovernmental Panel on Climate Change, edited by: Stocker, T. F., Qin, D., Plattner, G.-K., Tignor, M., Allen, S. K., Boschung, J., Nauels, A., Xia, Y., Bex, V., and Midgley, P. M., Cambridge University Press, Cambridge, United Kingdom and New York, NY, USA, 465–570, 2013.
Clark, S. K., Ward, D. S., and Mahowald, N. M.: Parameterization-based uncertainty in future lightning flash density, Geophys. Res. Lett., 44, 2893–2901, https://doi.org/10.1002/2017GL073017, 2017.
Collins, W. J., Bellouin, N., Doutriaux-Boucher, M., Gedney, N., Halloran, P., Hinton, T., Hughes, J., Jones, C. D., Joshi, M., Liddicoat, S., Martin, G., O'Connor, F., Rae, J., Senior, C., Sitch, S., Totterdell, I., Wiltshire, A., and Woodward, S.: Development and evaluation of an Earth-System model – HadGEM2, Geosci. Model Dev., 4, 1051–1075, https://doi.org/10.5194/gmd-4-1051-2011, 2011.
Collins, W. J., Lamarque, J.-F., Schulz, M., Boucher, O., Eyring, V., Hegglin, M. I., Maycock, A., Myhre, G., Prather, M., Shindell, D., and Smith, S. J.: AerChemMIP: quantifying the effects of chemistry and aerosols in CMIP6, Geosci. Model Dev., 10, 585–607, https://doi.org/10.5194/gmd-10-585-2017, 2017.
ESGF (Earth System Grid Federation)-CEDA: https://esgf-index1.ceda.ac.uk/search/cmip6-ceda/, last access: 12 November 2020.
Etminan, M., Myhre, G., Highwood, E. J., and Shine, K. P.: Radiative forcing of carbon dioxide, methane, and nitrous oxide: A significant revision of the methane radiative forcing, Geophys. Res. Lett., 43, 12614–12623, https://doi.org/10.1002/2016GL071930, 2016.
Evan, A. T., Flamant, C., Fiedler, S., and Doherty, O.: An analysis of aeolian dust in climate models, Geophys. Res. Lett., 41, 5996–6001, https://doi.org/10.1002/2014GL060545, 2014.
Eyring, V., Bony, S., Meehl, G. A., Senior, C. A., Stevens, B., Stouffer, R. J., and Taylor, K. E.: Overview of the Coupled Model Intercomparison Project Phase 6 (CMIP6) experimental design and organization, Geosci. Model Dev., 9, 1937–1958, https://doi.org/10.5194/gmd-9-1937-2016, 2016.
Fiedler, S., Knippertz, P., Woodward, S., Martin, G. M., Bellouin, N., Ross, A. N., Heinold, B., Schepanski, K., Birch, C. E., and Tegen, I.: A process-based evaluation of dust-emitting winds in the CMIP5 simulation of HadGEM2-ES, Clim. Dynam., 46, 1107–1130, https://doi.org/10.1007/s00382-015-2635-9, 2016.
Finney, D. L., Doherty, R. M., Wild, O., Young, P. J., and Butler, A.: Response of lightning NOx emissions and ozone production to climate change: Insights from the Atmospheric Chemistry and Climate Model Intercomparison Project, Geophys. Res. Lett., 43, 5492–5500, https://doi.org/10.1002/2016GL068825, 2016a.
Finney, D. L., Doherty, R. M., Wild, O., and Abraham, N. L.: The impact of lightning on tropospheric ozone chemistry using a new global lightning parametrisation, Atmos. Chem. Phys., 16, 7507–7522, https://doi.org/10.5194/acp-16-7507-2016, 2016b.
Finney, D. L., Doherty, R. M., Wild, O., Stevenson, D. S., MacKenzie, I. A., and Blyth, A. M.: A projected decrease in lightning under climate change, Nat. Clim. Change, 8, 210–213, https://doi.org/10.1038/s41558-018-0072-6, 2018.
Fiore, A. M., Dentener, F. J., Wild, O., Cuvelier, C., Schultz, M. G., Hess, P., Textor, C., Schulz, M., Doherty, R. M., Horowitz, L. W., MacKenzie, I. A., Sanderson, M. G., Shindell, D. T., Stevenson, D. S., Szopa, S., Van Dingenen, R., Zeng, G., Atherton, C., Bergmann, D., Bey, I., Carmichael, G., Collins, W. J., Duncan, B. N., Faluvegi, G., Folberth, G., Gauss, M., Gong, S., Hauglustaine, D., Holloway, T., Isaksen, I. S. A., Jacob, D. J., Jonson, J. E., Kaminski, J. W., Keating, T. J., Lupu, A., Manner, E., Montanaro, V., Park, R. J., Pitari, G., Pringle, K. J., Pyle, J. A., Schroeder, S., Vivanco, M. G., Wind, P., Wojcik, G., Wu, S., and Zuber, A.: Multimodel estimates of intercontinental source-receptor relationships for ozone pollution, J. Geophys. Res., 114, D04301, https://doi.org/10.1029/2008JD010816, 2009.
Friedlingstein, P.: Carbon cycle feedbacks and future climate change, Philos. T. Roy. Soc. A, 373, 20140421, https://doi.org/10.1098/rsta.2014.0421, 2015.
Gabric, A. J., Simó, R., Cropp, R. A., Hirst, A. C., and Dachs, J.: Modeling estimates of the global emission of dimethylsulfide under enhanced greenhouse conditions, Global Biogeochem. Cy., 18, GB2014, https://doi.org/10.1029/2003GB002183, 2004.
Gettelman, A., Mills, M. J., Kinnison, D. E., Garcia, R. R., Smith, A. K., Marsh, D. R., Tilmes, S., Vitt, F., Bardeen, C. G., McInerney, J., Liu, H.-L., Solomon, S. C., Polvani, L. M., Emmons, L. K., Lamarque, J.-F., Richter, J. H., Glanville, A. S., Bacmeister, J. T., Phillips, A. S., Neale, R. B., Simpson, I. R., DuVivier, A. K., Hodzic, A., and Randel, W. J.: The Whole Atmosphere Community Climate Model Version 6 (WACCM6), J. Geophys. Res.-Atmos., 124, 12380–12403, https://doi.org/10.1029/2019jd030943, 2019.
Gong, S. L.: A parameterization of sea-salt aerosol source function for sub- and super-micron particles, Global Biogeochem. Cy., 17, 1097, https://doi.org/10.1029/2003gb002079, 2003.
Gregory, J. M., Ingram, W. J., Palmer, M. A., Jones, G. S., Stott, P. A., Thorpe, R. B., Lowe, J. A., Johns, T. C., and Williams, K. D.: A new method for diagnosing radiative forcing and climate sensitivity, Geophys. Res. Lett., 31, L03205, https://doi.org/10.1029/2003GL018747, 2004.
Gregory, J. M., Jones, C. D., Cadule, P., and Friedlingstein, P.: Quantifying carbon cycle feedbacks, J. Climate, 22, 5232–5250, https://doi.org/10.1175/2009JCLI2949.1, 2009.
Guenther, A., Hewitt, N. C., Erickson, D., Fall, R., Geron, C., Graedel, T., Harley, P., Klinger, L., Lerdau, M., McKay, W. A., Pierce, T., Scholes, B., Steinbrecher, R., Taalamraju, R., Taylor, J., and Zimmermann, P.: A global model of natural volatile organic compound emissions, J. Geophys. Res., 100, 8873–8892, https://doi.org/10.1029/94JD02950, 1995.
Gunson, J. R., Spall, S. A., Anderson, T. R., Jones, A., Totterdell, I. J., and Woodage, M. J.: Climate sensitivity to ocean dimethylsulphide emissions, Geophys. Res. Lett., 33, L08801, https://doi.org/10.1029/2005GL024982, 2006.
Heinold, B., Tegen, I., Schepanski, K., and Hellmuth, O.: Dust radiative feedback on Saharan boundary layer dynamics and dust mobilization, Geophys. Res. Lett., 35, L20817, https://doi.org/10.1029/2008GL035319, 2008.
Heinold, B., Knippertz, P., Marsham, J. H., Fiedler, S., Dixon, N. S., Schepanski, K., Laurent, B., and Tegen, I.: The role of deep convection and nocturnal low-level jets for dust emission in summertime West Africa: Estimates from convection-permitting simulations, J. Geophys. Res.-Atmos., 118, 4385–4400, https://doi.org/10.1002/jgrd.50402, 2013.
Heinze, C., Eyring, V., Friedlingstein, P., Jones, C., Balkanski, Y., Collins, W., Fichefet, T., Gao, S., Hall, A., Ivanova, D., Knorr, W., Knutti, R., Löw, A., Ponater, M., Schultz, M. G., Schulz, M., Siebesma, P., Teixeira, J., Tselioudis, G., and Vancoppenolle, M.: ESD Reviews: Climate feedbacks in the Earth system and prospects for their evaluation, Earth Syst. Dynam., 10, 379–452, https://doi.org/10.5194/esd-10-379-2019, 2019.
Horowitz, L. W., Naik, V., Paulot, F., Ginoux, P. A., Dunne, J. P., Mao, J., Schnell, J., Chen, X., He, J., John, J. G., Lin, M., Lin, P., Malyshev, S., Paynter, D., Shevliakova, E., and Zhao, M.: The GFDL Global Atmospheric Chemistry-Climate Model AM4.1: Model Description and Simulation Characteristics, J. Adv. Model. Earth Sy., 12, e2019MS002032, https://doi.org/10.1029/2019MS002032, 2020
Huneeus, N., Schulz, M., Balkanski, Y., Griesfeller, J., Prospero, J., Kinne, S., Bauer, S., Boucher, O., Chin, M., Dentener, F., Diehl, T., Easter, R., Fillmore, D., Ghan, S., Ginoux, P., Grini, A., Horowitz, L., Koch, D., Krol, M. C., Landing, W., Liu, X., Mahowald, N., Miller, R., Morcrette, J.-J., Myhre, G., Penner, J., Perlwitz, J., Stier, P., Takemura, T., and Zender, C. S.: Global dust model intercomparison in AeroCom phase I, Atmos. Chem. Phys., 11, 7781–7816, https://doi.org/10.5194/acp-11-7781-2011, 2011.
Jaeglé, L., Quinn, P. K., Bates, T. S., Alexander, B., and Lin, J.-T.: Global distribution of sea salt aerosols: new constraints from in situ and remote sensing observations, Atmos. Chem. Phys., 11, 3137–3157, https://doi.org/10.5194/acp-11-3137-2011, 2011.
Johnson, C. E., Stevenson, D. S., Collins, W. J., and Derwent, R. G.: Role of climate feedback on methane and ozone studied with a coupled ocean-atmosphere-chemistry model, Geophys. Res. Lett., 28, 1723–1726, https://doi.org/10.1029/2000GL011996, 2001.
Kirkevåg, A., Grini, A., Olivié, D., Seland, Ø., Alterskjær, K., Hummel, M., Karset, I. H. H., Lewinschal, A., Liu, X., Makkonen, R., Bethke, I., Griesfeller, J., Schulz, M., and Iversen, T.: A production-tagged aerosol module for Earth system models, OsloAero5.3 – extensions and updates for CAM5.3-Oslo, Geosci. Model Dev., 11, 3945–3982, https://doi.org/10.5194/gmd-11-3945-2018, 2018.
Kloster, S., Six, K. D., Feichter, J., Maier-Reimer, E., Roeckner, E., Wetzel, P., Stier, P., and Esch, M.: Response of dimethylsulfide (DMS) in the ocean and atmosphere to global warming, J. Geophys. Res., 12, G03005, https://doi.org/10.1029/2006JG000224, 2007.
Kok, J. F., Ward, D. S., Mahowald, N. M., and Evan, A. T.: Global and regional importance of the direct dust-climate feedback, Nat. Commun., 9, 241, https://doi.org/10.1038/s41467-017-02620-y, 2018.
Mahowald, N. M. and Luo, C.: A less dusty future?, Geophys. Res. Lett., 30, 1903, https://doi.org/10.1029/2003GL017880, 2003.
Melton, J. R., Wania, R., Hodson, E. L., Poulter, B., Ringeval, B., Spahni, R., Bohn, T., Avis, C. A., Beerling, D. J., Chen, G., Eliseev, A. V., Denisov, S. N., Hopcroft, P. O., Lettenmaier, D. P., Riley, W. J., Singarayer, J. S., Subin, Z. M., Tian, H., Zürcher, S., Brovkin, V., van Bodegom, P. M., Kleinen, T., Yu, Z. C., and Kaplan, J. O.: Present state of global wetland extent and wetland methane modelling: conclusions from a model inter-comparison project (WETCHIMP), Biogeosciences, 10, 753–788, https://doi.org/10.5194/bg-10-753-2013, 2013.
Michou, M., Nabat, P., Saint-Martin, D., Bock, J., Decharme, B., Mallet, M., Roehrig, R., Séférian, R., Sénési, S., and Voldoire, A.: Present-Day and Historical Aerosol and Ozone Characteristics in CNRM CMIP6 Simulations, J. Adv. Model. Earth Sy., 12, e2019MS001816, https://doi.org/10.1029/2019MS001816, 2020.
Mulcahy, J. P., Johnson, C., Jones, C. G., Povey, A. C., Scott, C. E., Sellar, A., Turnock, S. T., Woodhouse, M. T., Abraham, N. L., Andrews, M. B., Bellouin, N., Browse, J., Carslaw, K. S., Dalvi, M., Folberth, G. A., Glover, M., Grosvenor, D. P., Hardacre, C., Hill, R., Johnson, B., Jones, A., Kipling, Z., Mann, G., Mollard, J., O'Connor, F. M., Palmiéri, J., Reddington, C., Rumbold, S. T., Richardson, M., Schutgens, N. A. J., Stier, P., Stringer, M., Tang, Y., Walton, J., Woodward, S., and Yool, A.: Description and evaluation of aerosol in UKESM1 and HadGEM3-GC3.1 CMIP6 historical simulations, Geosci. Model Dev., 13, 6383–6423, https://doi.org/10.5194/gmd-13-6383-2020, 2020.
Myhre, G., Shindell, D., Bréon, F.-M., Collins, W., Fuglestvedt, J., Huang, J., Koch, D., Lamarque, J.-F., Lee, D., Mendoza, B., Nakajima, T., Robock, A., Stephens, G., Takemura, T., and Zhang, H.: Anthropogenic and Natural Radiative Forcing, in: Climate Change 2013: The Physical Science Basis. Contribution of Working Group I to the Fifth Assessment Report of the Intergovernmental Panel on Climate Change, edited by: Stocker, T. F., Qin, D., Plattner, G.-K., Tignor, M., Allen, S. K., Boschung, J., Nauels, A., Xia, Y., Bex, V., and Midgley, P. M., Cambridge University Press, Cambridge, United Kingdom and New York, NY, USA, 659–740, 2013a.
Myhre, G., Samset, B. H., Schulz, M., Balkanski, Y., Bauer, S., Berntsen, T. K., Bian, H., Bellouin, N., Chin, M., Diehl, T., Easter, R. C., Feichter, J., Ghan, S. J., Hauglustaine, D., Iversen, T., Kinne, S., Kirkevåg, A., Lamarque, J.-F., Lin, G., Liu, X., Lund, M. T., Luo, G., Ma, X., van Noije, T., Penner, J. E., Rasch, P. J., Ruiz, A., Seland, ø., Skeie, R. B., Stier, P., Takemura, T., Tsigaridis, K., Wang, P., Wang, Z., Xu, L., Yu, H., Yu, F., Yoon, J.-H., Zhang, K., Zhang, H., and Zhou, C.: Radiative forcing of the direct aerosol effect from AeroCom Phase II simulations, Atmos. Chem. Phys., 13, 1853–1877, https://doi.org/10.5194/acp-13-1853-2013, 2013b.
Naik, V., Voulgarakis, A., Fiore, A. M., Horowitz, L. W., Lamarque, J.-F., Lin, M., Prather, M. J., Young, P. J., Bergmann, D., Cameron-Smith, P. J., Cionni, I., Collins, W. J., Dalsøren, S. B., Doherty, R., Eyring, V., Faluvegi, G., Folberth, G. A., Josse, B., Lee, Y. H., MacKenzie, I. A., Nagashima, T., van Noije, T. P. C., Plummer, D. A., Righi, M., Rumbold, S. T., Skeie, R., Shindell, D. T., Stevenson, D. S., Strode, S., Sudo, K., Szopa, S., and Zeng, G.: Preindustrial to present-day changes in tropospheric hydroxyl radical and methane lifetime from the Atmospheric Chemistry and Climate Model Intercomparison Project (ACCMIP), Atmos. Chem. Phys., 13, 5277–5298, https://doi.org/10.5194/acp-13-5277-20133, 2013.
O'Connor, F. M., Boucher, O., Gedney, N., Jones, C. D., Folberth, G. A., Coppell, R., Friedlingstein, P., Collins, W. J., Chappellaz, J., Ridley, J., and Johnson, C. E.: Possible role of wetlands, permafrost, and methane hydrates in the methane cycle under future climate change: A review, Rev. Geophys., 48, RG4005, https://doi.org/10.1029/2010RG000326, 2010.
O'Connor, F. M., Abraham, N. L., Dalvi, M., Folberth, G., Griffiths, P., Hardacre, C., Johnson, B. T., Kahana, R., Keeble, J., Kim, B., Morgenstern, O., Mulcahy, J. P., Richardson, M. G., Robertson, E., Seo, J., Shim, S., Teixeira, J. C., Turnock, S., Williams, J., Wiltshire, A., and Zeng, G.: Assessment of pre-industrial to present-day anthropogenic climate forcing in UKESM1, Atmos. Chem. Phys. Discuss. [preprint], https://doi.org/10.5194/acp-2019-1152, in review, 2020.
Ocko, I. B., Naik, V., and Paynter, D.: Rapid and reliable assessment of methane impacts on climate, Atmos. Chem. Phys., 18, 15555–15568, https://doi.org/10.5194/acp-18-15555-2018, 2018.
Paasonen, P., Asmi, A., Petäjä, T., Kajos, M. K., Äijälä, M., Junninen, H., Holst, T., Abbatt, J. P. D., Arneth, A., Birmili, W., Van Der Gon, H. D., Hamed, A., Hoffer, A., Laakso, L., Laaksonen, A., Richard Leaitch, W., Plass-Dülmer, C., Pryor, S. C., Räisänen, P., Swietlicki, E., Wiedensohler, A., Worsnop, D. R., Kerminen, V. M., and Kulmala, M.: Warming-induced increase in aerosol number concentration likely to moderate climate change, Nat. Geosci., 6, 438–442, https://doi.org/10.1038/ngeo1800, 2013.
Pacifico, F., Harrison, S. P., Jones, C. D., Arneth, A., Sitch, S., Weedon, G. P., Barkley, M. P., Palmer, P. I., Serça, D., Potosnak, M., Fu, T.-M., Goldstein, A., Bai, J., and Schurgers, G.: Evaluation of a photosynthesis-based biogenic isoprene emission scheme in JULES and simulation of isoprene emissions under present-day climate conditions, Atmos. Chem. Phys., 11, 4371–4389, https://doi.org/10.5194/acp-11-4371-2011, 2011.
Pacifico, F., Folberth, G. A., Jones, C. D., Harrison, S. P., and Collins, W. J.: Sensitivity of biogenic isoprene emissions to past, present, and future environmental conditions and implications for atmospheric chemistry, J. Geophys. Res.-Atmos., 117, D22302, https://doi.org/10.1029/2012JD018276, 2012.
Prather, M. J., Holmes, C. D., and Hsu, J.: Reactive greenhouse gas scenarios: Systematic exploration of uncertainties and the role of atmospheric chemistry, Geophys. Res. Lett., 39, L09803, https://doi.org/10.1029/2012GL051440, 2012.
Price, C. and Rind, D.: A simple lightning parameterization for calculating global lightning distributions, J. Geophys. Res., 97, 9919–9933, https://doi.org/10.1029/92JD00719, 1992.
Price, C., Penner, J., and Prather, M.: NOx from lightning 1. Global distribution based on lightning physics, J. Geophys. Res., 102, 5929–5941, https://doi.org/10.1029/96jd03504, 1997.
Raes, F., Liao, H., Chen, W. T., and Seinfeld, J. H.: Atmospheric chemistry-climate feedbacks, J. Geophys. Res.-Atmos., 115, D12121, https://doi.org/10.1029/2009JD013300, 2010.
Saunois, M., Stavert, A. R., Poulter, B., Bousquet, P., Canadell, J. G., Jackson, R. B., Raymond, P. A., Dlugokencky, E. J., Houweling, S., Patra, P. K., Ciais, P., Arora, V. K., Bastviken, D., Bergamaschi, P., Blake, D. R., Brailsford, G., Bruhwiler, L., Carlson, K. M., Carrol, M., Castaldi, S., Chandra, N., Crevoisier, C., Crill, P. M., Covey, K., Curry, C. L., Etiope, G., Frankenberg, C., Gedney, N., Hegglin, M. I., Höglund-Isaksson, L., Hugelius, G., Ishizawa, M., Ito, A., Janssens-Maenhout, G., Jensen, K. M., Joos, F., Kleinen, T., Krummel, P. B., Langenfelds, R. L., Laruelle, G. G., Liu, L., Machida, T., Maksyutov, S., McDonald, K. C., McNorton, J., Miller, P. A., Melton, J. R., Morino, I., Müller, J., Murguia-Flores, F., Naik, V., Niwa, Y., Noce, S., O'Doherty, S., Parker, R. J., Peng, C., Peng, S., Peters, G. P., Prigent, C., Prinn, R., Ramonet, M., Regnier, P., Riley, W. J., Rosentreter, J. A., Segers, A., Simpson, I. J., Shi, H., Smith, S. J., Steele, L. P., Thornton, B. F., Tian, H., Tohjima, Y., Tubiello, F. N., Tsuruta, A., Viovy, N., Voulgarakis, A., Weber, T. S., van Weele, M., van der Werf, G. R., Weiss, R. F., Worthy, D., Wunch, D., Yin, Y., Yoshida, Y., Zhang, W., Zhang, Z., Zhao, Y., Zheng, B., Zhu, Q., Zhu, Q., and Zhuang, Q.: The Global Methane Budget 2000–2017, Earth Syst. Sci. Data, 12, 1561–1623, https://doi.org/10.5194/essd-12-1561-2020, 2020.
Schwinger, J., Tjiputra, J., Goris, N., Six, K. D., Kirkevåg, A., Seland, Ø., Heinze, C., and Ilyina, T.: Amplification of global warming through pH dependence of DMS production simulated with a fully coupled Earth system model, Biogeosciences, 14, 3633–3648, https://doi.org/10.5194/bg-14-3633-2017, 2017.
Scott, C. E., Rap, A., Spracklen, D. V., Forster, P. M., Carslaw, K. S., Mann, G. W., Pringle, K. J., Kivekäs, N., Kulmala, M., Lihavainen, H., and Tunved, P.: The direct and indirect radiative effects of biogenic secondary organic aerosol, Atmos. Chem. Phys., 14, 447–470, https://doi.org/10.5194/acp-14-447-2014, 2014.
Scott, C. E., Arnold, S. R., Monks, S. A., Asmi, A., Paasonen, P., and Spracklen, D. V.: Substantial large-scale feedbacks between natural aerosols and climate, Nat. Geosci., 11, 44–48, https://doi.org/10.1038/s41561-017-0020-5, 2018.
Séférian, R., Berthet, S., Yool, A., Palmiéri, J., Bopp, L., Tagliabue, A., Kwiatkowski, L., Aumont, O., Christian, J., Dunne, J., Gehlen, M., Ilyina, T., John, J. G., Li, H., Long, M. C., Luo, J. Y., Nakano, H., Romanou, A., Schwinger, J., Stock, C., Santana-Falcón, Y., Takano, Y., Tjiputra, J., Tsujino, H., Watanabe, M., Wu, T., Wu, F., and Yamamoto, A.: Tracking Improvement in Simulated Marine Biogeochemistry Between CMIP5 and CMIP6, Current Climate Change Reports, 6, 95–119, https://doi.org/10.1007/s40641-020-00160-0, 2020.
Seland, Ø., Bentsen, M., Olivié, D., Toniazzo, T., Gjermundsen, A., Graff, L. S., Debernard, J. B., Gupta, A. K., He, Y.-C., Kirkevåg, A., Schwinger, J., Tjiputra, J., Aas, K. S., Bethke, I., Fan, Y., Griesfeller, J., Grini, A., Guo, C., Ilicak, M., Karset, I. H. H., Landgren, O., Liakka, J., Moseid, K. O., Nummelin, A., Spensberger, C., Tang, H., Zhang, Z., Heinze, C., Iversen, T., and Schulz, M.: Overview of the Norwegian Earth System Model (NorESM2) and key climate response of CMIP6 DECK, historical, and scenario simulations, Geosci. Model Dev., 13, 6165–6200, https://doi.org/10.5194/gmd-13-6165-2020, 2020.
Sellar, A. A., Jones, C. G., Mulcahy, J., Tang, Y., Yool, A., Wiltshire, A., O'Connor, F. M., Stringer, M., Hill, R., Palmieri, J., Woodward, S., Mora, L., Kuhlbrodt, T., Rumbold, S., Kelley, D. I., Ellis, R., Johnson, C. E., Walton, J., Abraham, N. L., Andrews, M. B., Andrews, T., Archibald, A. T., Berthou, S., Burke, E., Blockley, E., Carslaw, K., Dalvi, M., Edwards, J., Folberth, G. A., Gedney, N., Griffiths, P. T., Harper, A. B., Hendry, M. A., Hewitt, A. J., Johnson, B., Jones, A., Jones, C. D., Keeble, J., Liddicoat, S., Morgenstern, O., Parker, R. J., Predoi, V., Robertson, E., Siahaan, A., Smith, R. S., Swaminathan, R., Woodhouse, M. T., Zeng, G., and Zerroukat, M.: UKESM1: Description and evaluation of the UK Earth System Model, J. Adv. Model. Earth Syst., 11, 4513–4558, https://doi.org/10.1029/2019ms001739, 2019.
Shao, Y., Wyrwoll, K. H., Chappell, A., Huang, J., Lin, Z., McTainsh, G. H., Mikami, M., Tanaka, T. Y., Wang, X., and Yoon, S.: Dust cycle: An emerging core theme in Earth system science, Aeolian Res., 2, 181–204, https://doi.org/10.1016/j.aeolia.2011.02.001, 2011.
Sherwood, S., Webb, M. J., Annan, J. D., Armour, K. C., Forster, P. M., Hargreaves, J. C., Hegerl, G., Klein, S. A., Marvel, K. D., Rohling, E. J., Watanabe, M., Andrews, T., Braconnot, P., Bretherton, C. S., Foster, G. L., Hausfather, Z., Heydt, A. S. von der, Knutti, R., Mauritsen, T., Norris, J. R., Proistosescu, C., Rugenstein, M., Schmidt, G. A., Tokarska, K. B., and Zelinka, M. D.: An assessment of Earth's climate sensitivity using multiple lines of evidence, Rev. Geophys., 58, e2019RG000678, https://doi.org/10.1029/2019rg000678, 2020.
Six, K. D., Kloster, S., Ilyina, T., Archer, S. D., Zhang, K., and Maier-Reimer, E.: Global warming amplified by reduced sulphur fluxes as a result of ocean acidification, Nat. Clim. Change, 3, 975–978, https://doi.org/10.1038/nclimate1981, 2013.
Skeie, R. B., Myhre, G., Hodnebrog, Ø., Cameron-Smith, P. J., Deushi, M., Hegglin, M. I., Horowitz, L. W., Kramer, R. J., Michou, M., Mills, M. J., Olivié, D. J. L., Connor, F. M. O., Paynter, D., Samset, B. H., Sellar, A., Shindell, D., Takemura, T., Tilmes, S., and Wu, T.: Historical total ozone radiative forcing derived from CMIP6 simulations, npj Clim. Atmos. Sci., 3, 32, https://doi.org/10.1038/s41612-020-00131-0, 2020.
Sporre, M. K., Blichner, S. M., Karset, I. H. H., Makkonen, R., and Berntsen, T. K.: BVOC–aerosol–climate feedbacks investigated using NorESM, Atmos. Chem. Phys., 19, 4763–4782, https://doi.org/10.5194/acp-19-4763-2019, 2019.
Spracklen, D. V. and Rap, A.: Natural aerosol-climate feedbacks suppressed by anthropogenic aerosol, Geophys. Res. Lett., 40, 5316–5319, https://doi.org/10.1002/2013GL057966, 2013.
Stevenson, D. S., Young, P. J., Naik, V., Lamarque, J.-F., Shindell, D. T., Voulgarakis, A., Skeie, R. B., Dalsoren, S. B., Myhre, G., Berntsen, T. K., Folberth, G. A., Rumbold, S. T., Collins, W. J., MacKenzie, I. A., Doherty, R. M., Zeng, G., van Noije, T. P. C., Strunk, A., Bergmann, D., Cameron-Smith, P., Plummer, D. A., Strode, S. A., Horowitz, L., Lee, Y. H., Szopa, S., Sudo, K., Nagashima, T., Josse, B., Cionni, I., Righi, M., Eyring, V., Conley, A., Bowman, K. W., Wild, O., and Archibald, A.: Tropospheric ozone changes, radiative forcing and attribution to emissions in the Atmospheric Chemistry and Climate Model Intercomparison Project (ACCMIP), Atmos. Chem. Phys., 13, 3063–3085, https://doi.org/10.5194/acp-13-3063-2013, 2013.
Tatebe, H., Ogura, T., Nitta, T., Komuro, Y., Ogochi, K., Takemura, T., Sudo, K., Sekiguchi, M., Abe, M., Saito, F., Chikira, M., Watanabe, S., Mori, M., Hirota, N., Kawatani, Y., Mochizuki, T., Yoshimura, K., Takata, K., O'ishi, R., Yamazaki, D., Suzuki, T., Kurogi, M., Kataoka, T., Watanabe, M., and Kimoto, M.: Description and basic evaluation of simulated mean state, internal variability, and climate sensitivity in MIROC6, Geosci. Model Dev., 12, 2727–2765, https://doi.org/10.5194/gmd-12-2727-2019, 2019.
Thornhill, G. D., Collins, W. J., Kramer, R. J., Olivié, D., O'Connor, F., Abraham, N. L., Bauer, S. E., Deushi, M., Emmons, L., Forster, P., Horowitz, L., Johnson, B., Keeble, J., Lamarque, J.-F., Michou, M., Mills, M., Mulcahy, J., Myhre, G., Nabat, P., Naik, V., Oshima, N., Schulz, M., Smith, C., Takemura, T., Tilmes, S., Wu, T., Zeng, G., and Zhang, J.: Effective Radiative forcing from emissions of reactive gases and aerosols – a multimodel comparison, Atmos. Chem. Phys. Discuss. [preprint], https://doi.org/10.5194/acp-2019-1205, in review, 2020.
Unger, N.: On the role of plant volatiles in anthropogenic global climate change, Geophys. Res. Lett., 418563–418569, https://doi.org/10.1002/2014GL061616, 2014.
Vallina, S. M., Simó, R., and Manizza, M.: Weak response of oceanic dimethylsulfide to upper mixing shoaling induced by global warming, P. Natl. Acad. Sci. USA, 104, 16004–16009, https://doi.org/10.1073/pnas.0700843104, 2007.
Voulgarakis, A., Naik, V., Lamarque, J.-F., Shindell, D. T., Young, P. J., Prather, M. J., Wild, O., Field, R. D., Bergmann, D., Cameron-Smith, P., Cionni, I., Collins, W. J., Dalsøren, S. B., Doherty, R. M., Eyring, V., Faluvegi, G., Folberth, G. A., Horowitz, L. W., Josse, B., MacKenzie, I. A., Nagashima, T., Plummer, D. A., Righi, M., Rumbold, S. T., Stevenson, D. S., Strode, S. A., Sudo, K., Szopa, S., and Zeng, G.: Analysis of present day and future OH and methane lifetime in the ACCMIP simulations, Atmos. Chem. Phys., 13, 2563–2587, https://doi.org/10.5194/acp-13-2563-2013, 2013.
Woodward, S., Roberts, D. L., and Betts, R. A.: A simulation of the effect of climate change-induced desertification on mineral dust aerosol, Geophys. Res. Lett., 32, L18810, https://doi.org/10.1029/2005GL023482, 2005.
Wu, R., Wang, S., and Wang, L.: New mechanism for the atmospheric oxidation of dimethyl sulfide. The importance of intramolecular hydrogen shift in a CH3SCH2OO radical, J. Phys. Chem. A, 119, 112–117, https://doi.org/10.1021/jp511616j, 2015.
Xie, X., Liu, X., Che, H., Xie, X., Wang, H., Li, J., Shi, Z., and Liu, Y.: Modeling East Asian Dust and Its Radiative Feedbacks in CAM4-BAM, J. Geophys. Res.-Atmos., 123, 1079–1096, https://doi.org/10.1002/2017JD027343, 2018.
Zender, C. S., Miller, R. L., and Tegen, I.: Quantifying mineral dust mass budgets: Terminology, constraints, and current estimates, EOS, 85, 509–512, https://doi.org/10.1029/2004EO480002, 2004.