the Creative Commons Attribution 4.0 License.
the Creative Commons Attribution 4.0 License.
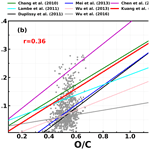
Contrasting effects of secondary organic aerosol formations on organic aerosol hygroscopicity
Biao Xue
Biao Luo
Qicong Song
Wei Chen
Weiwei Hu
Wei Li
Pusheng Zhao
Mingfu Cai
Yuwen Peng
Jipeng Qi
Tiange Li
Sihang Wang
Duohong Chen
Dingli Yue
Min Shao
Water uptake abilities of organic aerosol under sub-saturated conditions play critical roles in direct aerosol radiative effects and atmospheric chemistry; however, field characterizations of the organic aerosol hygroscopicity parameter κOA under sub-saturated conditions remain limited. In this study, a field campaign was conducted to characterize κOA at a relative humidity of 80 % with hourly time resolution for the first time in the Pearl River Delta region of China. Observation results show that, during this campaign, secondary organic aerosol (SOA) dominated total organic aerosol mass (mass fraction > 70 % on average), which provides a unique opportunity to investigate influences of SOA formation on κOA. Results demonstrate that the commonly used organic aerosol oxidation level parameter was weakly correlated with κOA and failed to describe the variations in κOA. However, the variations in κOA were well reproduced by mass fractions of organic aerosol factor resolved based on aerosol mass spectrometer measurements. The more oxygenated organic aerosol (MOOA) factor, exhibiting the highest average (∼ 1) among all organic aerosol factors, was the most important factor driving the increase in κOA and was commonly associated with regional air masses. The less oxygenated organic aerosol (LOOA; average of 0.72) factor revealed strong daytime production, exerting negative effects on κOA. Surprisingly, the aged biomass burning organic aerosol (aBBOA) factor also formed quickly during daytime and shared a similar diurnal pattern with LOOA but had much lower (0.39) and had positive effects on κOA. The correlation coefficient between κOA and mass fractions of aBBOA and MOOA in total organic aerosol mass reached above 0.8. The contrasting effects of LOOA and aBBOA formation on κOA demonstrate that volatile organic compound (VOC) precursors from diverse sources and different SOA formation processes may result in SOA with different chemical composition, functional properties and microphysical structure, consequently exerting distinct influences on κOA and rendering single oxidation level parameters (such as ) unable to capture those differences. Aside from that, distinct effects of aBBOA on κOA were observed during different episodes, suggesting that the hygroscopicity of SOA associated with similar sources might also differ much under different emission and atmospheric conditions. Overall, these results highlight that it is imperative to conduct more research on κOA characterization under different meteorological and source conditions and examine its relationship with VOC precursor profiles and formation pathways to formulate a better characterization and develop more appropriate parameterization approaches in chemical and climate models.
- Article
(6969 KB) -
Supplement
(1151 KB) - BibTeX
- EndNote
Organic aerosol (OA), composed of hundreds to thousands of organic species, is one of the dominant aerosol components in the atmosphere and exerts significant effects on climate and the environment (Jimenez et al., 2009). The water uptake ability of atmospheric organic aerosol plays key roles in aerosol direct radiative effects and aerosol–cloud interactions (Rastak et al., 2017; Liu and Wang, 2010) as well as aerosol liquid water content (Li et al., 2019; Jin et al., 2020) and thus atmospheric chemistry. However, the hygroscopicity parameter κOA that describes the water uptake abilities of organic aerosol remains poorly quantified, and mechanisms behind κOA variations are not well understood (Kuang et al., 2020b). Atmospheric OA is usually composed of both primary and secondary organic aerosol components. Primary OA (POA) is directly emitted from anthropogenic and natural sources such as biomass burning, coal and fossil fuel combustion, cooking, and biogenic emissions, whereas secondary OA (SOA) is typically formed through atmospheric oxidation of volatile organic compounds (VOCs) or aging processes of POA. It is commonly thought that OA becomes more oxidized during its evolution in the atmosphere and will in general be more hygroscopic after aging processes (Jimenez et al., 2009). A few studies have investigated the relationship between κOA and aerosol oxidation state parameters such as ratio or f44 (fraction of 44 in OA measurements of aerosol mass spectrometers). Some results, especially those from laboratory studies, demonstrated that κOA was highly correlated with (Jimenez et al., 2009; Massoli et al., 2010; Kuang et al., 2020a; Zhao et al., 2016; Lambe et al., 2011); however, other research demonstrated that κOA was not or only weakly correlated with (Cerully et al., 2015; Lathem et al., 2013; Yeung et al., 2014; Alfarra et al., 2013). As the research continues, it was revealed that many factors can have significant impacts on κOA, such as different functional groups, carbon chain length, aerosol liquid water content, etc. (Rickards et al., 2013; Suda et al., 2014; Petters et al., 2017; Marsh et al., 2017; Liu et al., 2018). Kuang et al. (2020b) recently reviewed laboratory and field measurements of κOA and concluded that is not enough to parameterize κOA and that additional parameters are needed. Therefore, it is worthwhile and imperative to endeavor on κOA quantifications and parametrizations, especially considering that OA might play more critical roles in the atmospheric environment and climate for decades to come under strict control on anthropogenic emissions.
Most previous studies on κOA focused on laboratory studies, usually investigating κOA of SOA produced from laboratory chamber systems, which might be far different from real atmospheric SOA spectra. Quantifications of κOA based on field measurements remain relatively limited and are also urgently needed to yield complementary information, which in turn might provide guidance for the design of future laboratory studies. It is important to conduct more research on κOA spatiotemporal distributions and examine its relationship with OA profiles to reach a better characterization and give rise to more appropriate parameterization approaches in chemical and climate models. China is a country that has been experiencing severe aerosol pollution and has been undergoing rapid changes under drastic air pollution control measures. However, despite the importance of organic aerosol hygroscopicity, only few studies attempted to quantify κOA based on field measurements (Wu et al., 2016; Li et al., 2019; Hong et al., 2018; Gunthe et al., 2011), mainly focusing on the North China Plain (NCP). The Pearl River Delta (PRD) region is much cleaner than the NCP in terms of particulate matter pollution, suggesting that distinct regions in China are at different stages of air pollution controls (Xu et al., 2020). The composition of PM2.5 (particulate matter with aerodynamic diameter less than 2.5 µm) also differs much among regions; for example, OA and SOA fractions are much higher in the PRD than those in the NCP, and their precursors are also much different (Zhou et al., 2020a). More investigations on κOA based on field studies in regions other than the NCP are urgently required.
In addition, most field studies on κOA only gave an estimate of the average κOA (Gunthe et al., 2011) or an average statistical relationship between κOA and (Wu et al., 2013), only few studies have reported κOA of higher time resolution featuring its diurnal variation characteristics (Deng et al., 2019), and almost no studies have reported κOA with high time resolution. Kuang et al. (2020a) proposed a new method to estimate κOA based on aerosol optical hygroscopicity measurements and bulk aerosol chemical composition measurements, which yielded κOA estimates at hourly time resolution. It revealed that variations in κOA were highly correlated with mass fractions of oxygenated organic aerosol in OA. In this study, the same method was applied to the dataset acquired from field measurements at a background site of the PRD region. High-time-resolution characterization of κOA and aerosol chemical properties was also achieved, which enabled us to dig deeper into the factors other than that drove the variations in κOA and to further elucidate the complexity and possible approaches in parameterizing κOA based on field measurements. We describe details on aerosol measurements and the κOA estimation method in the Measurements and Methodology sections. In the Results and discussions section, we first sketch out the overview of campaign measurements and then discuss the κOA variation characteristics as well as its influencing factors, and in the last part, the complexity regarding κOA parameterization is further demonstrated and elucidated. The summaries are provided in the Conclusions section.
2.1 Sampling site
Physical, optical and chemical properties of ambient aerosol particles as well as meteorological parameters and gas pollutants such as CO, O3 and NOx were continuously measured during autumn (from 30 September to 17 November 2018) at a rural site in Heshan County, Guangdong Province, China. This site was located at a small mountain (22∘42′ N, 112∘55′ E; altitude of 55 m) about 55 km away from the megacity Guangzhou and was surrounded by villages and small residential towns and thus was little influenced by local industrial sources. The location of this site is shown in Fig. S1 in the Supplement. This site is also a supersite operated by the provincial environmental monitoring authority and therefore provides qualified meteorological parameters and pollutant measurements such as PM2.5, CO, O3 and NOx. Acetonitrile was measured by a proton transfer reaction time-of-flight mass spectrometer (PTR-ToF; Ionicon Analytik GmbH, Innsbruck, Austria).
2.2 Aerosol physical property measurements
During this field campaign, instruments were placed in an air-conditioned room. Two inlets were housed on the roof of the three-floor building for aerosol sampling, and both inlets were about 1.8 m above the floor. One of the inlets was a PM10 impactor with a 1.8 m long Nafion drier that lowers the sample relative humidity (RH) down to less than 30 % placed downstream of it. A flow splitter was placed below the drier, and instruments downstream of this splitter included an aerodynamic particle sizer (APS; TSI Inc., Model 3321, flow rate of 5 L min−1), which measured the size distribution of ambient aerosol particles with an aerodynamic diameter of about 600 nm to 20 µm; an AE33 aethalometer (Drinovec et al., 2015) with a flow rate of 5 L min−1, which measured aerosol absorption coefficients at seven wavelengths; and a humidified nephelometer system with a flow rate of about 6 L min−1. The total flow rate of these instruments was about 16 L min−1, which was quite close to the flow rate of 16.7 L min−1 required by the PM10 impactor. Thus, these instruments measured the physical and optical properties of PM10 particles.
The humidified nephelometer system was a laboratory self-assembled one, including two Aurora 3000 nephelometers. One nephelometer measured the aerosol scattering properties (scattering and backscattering coefficients at three wavelengths: 450, 525, 635 nm) at a reference RH (called a dry nephelometer because the sampling RH is lower than 30 %), and another nephelometer measured the aerosol scattering properties under a fixed RH of 80 % (called a wet nephelometer, and the actual sampling RH fluctuates within ± 1 %). Details on the humidifier and control algorithm could be found in Kuang et al. (2020a). To ensure the accuracy of the measured RH in the sensing volume of the wet nephelometer, three Vaisala HMP110 sensors (± 0.2 ∘C and ± 1.7 % for RH between 0 % and 90 %) were used to monitor the RH at different parts of the wet nephelometer. Two sensors were placed at the inlet and outlet of the wet nephelometer, and one was placed in the sensing volume. The water vapor pressures calculated from these three sensors must be strictly consistent with each other (relative difference between any two of the sensors must be less than 2 %). Then the sampling RH of the wet nephelometer was calculated using the averaged water vapor pressure and the sample temperature measured by the sensor placed in the sensing volume of the wet nephelometer.
Another inlet was connected with a PM2.5 impactor (BGI SCC2.354, cut diameter of 2.5 µm with a flow rate of 8 L min−1) and was also equipped with a Nafion drier, lowering the sampling RH down to less than 30 %. Downstream of this inlet were a soot particle aerosol mass spectrometer (SP-AMS; Aerodyne Research, Inc., Billerica, MA, USA) and a scanning mobility particle sizer (SMPS; TSI model 3080), which measured the particle number size distribution (PNSD) ranging from 10 to 760 nm.
2.3 SP-AMS measurements and data analysis
The SP-AMS was deployed to measure the size-resolved chemical composition for submicron aerosol particles. The SP-AMS is a high-resolution time-of-flight aerosol mass spectrometer (HR-ToF-AMS) combining a laser vaporization device, i.e., soot particle (SP) mode. The instrument principle has been provided in previous papers (Canagaratna et al., 2007; Onasch et al., 2012). In brief, the HR-ToF-AMS containing a tungsten vaporizer can provide information of those particulate species vaporized under around 600 ∘C. By adding a Nd:YAG (1064 nm) laser module inside of the HR-ToF-AMS, the vaporizing temperature can increase to around 4000 ∘C, enabling the SP-AMS to detect refractory compositions such as black carbon (BC) and metals. After being vaporized, the gaseous components were ionized via electron impact (70 eV) and then quantitatively measured by a time-of-flight mass spectrometer. The airflow in the AMS was first controlled by the orifice and then focused through the aerodynamic lens of the SP-AMS, and then particles with diameters in the submicron range were detected. During the Heshan campaign, the SP-AMS was located next to an SMPS to minimize the sampling discrepancy. The SP-AMS alternately switched between the V mode (only tungsten vaporizer) and SP mode (laser and tungsten vaporizer). The original time resolution of SP-AMS data was 1 min (per run), and 15 min average values were used in this study to avoid disturbance from mode switching. During the campaign, the calibration system for the SP-AMS was not available, and we used the values of ionization efficiency (IE) and relative ionization efficiency (RIE) of different species from the latest successful calibration. The applied RIEs for default SP-AMS species are 1.1 for nitrate, 4 for ammonium, 1.2 for sulfate, 1.4 for organics and 1.3 for chloride. The composition-dependent collection efficiency (CDCE) was applied to the mentioned species following the instruction of Middlebrook et al. (2012). Refractory BC from SP-AMS was calculated by mass concentration of family Cx ions from the high-resolution mass spectrometer times a scaling factor (8) derived by comparison with equivalent BC mass concentration from AE33. SP-AMS data evaluation was performed by specific software: Squirrel (v1.61) for unit mass resolution and Pika (v1.21) for high resolution based on Igor Pro (v6.37, WaveMetrics, Inc., Oregon, USA). Aside from the good consistency between the aerosol derived from SMPS and SP-AMS components as mentioned in Sect. 3.2 (Fig. S5 in the Supplement), the resulting mass concentrations from SP-AMS were further validated by consistency with the results from external measurements at the same site, e.g., offline PM2.5 filter measurements and online measurements for total PM2.5 mass and individual components using a gas aerosol collection system (GAC) with ion chromatography operated (Figs. S7–S9 in the Supplement).
The source apportionment of organic aerosols (OAs) was performed by positive matrix factorization (PMF) based on high-resolution OA data collected in V mode (only tungsten vaporizer). The principle of PMF has been described in previous papers (Paatero, 1997; Paatero and Tapper, 1994). In this study, PMF using high-resolution AMS data including two matrices (organic ion mass concentrations and their uncertainties) were conducted by an Igor Pro-based panel, i.e., PMF evaluation tool (PET; v2.06, Ulbrich et al., 2009), following the instruction in Ulbrich et al. (2009). Isotopes and ions with > 120 were excluded to minimize the interference from repeatedly calculated uncertainties and noise signals. In total, 454 ions were considered in PMF. After investigating different solutions with factor numbers from 2 to 10, a six-factor solution was chosen based on the best performance shown by PMF quality parameters and the most reasonable source identification. Two primary OA factors were identified including a hydrocarbon-like OA (HOA; containing cooking emissions) and a biomass burning OA (BBOA). The other four factors were related to secondary formation or aging process: (1) more oxygenated OA (MOOA; regional transport), (2) less oxygenated OA (LOOA; related to daytime photochemical formation), (3) nighttime-formed OA (Night-OA) and (4) aged BBOA (aBBOA). The mass spectral profile and time series of OA factors are shown in Fig. S6 in the Supplement, and OA factors with identified sources are discussed in Sect. 4. More detailed discussions on the PMF results will be given elsewhere.
3.1 Aerosol hygroscopicity derivation from aerosol light scattering measurements
The aerosol light scattering enhancement factor , σsp(RH,λ) is the aerosol scattering coefficient at a light wavelength of λ and condition of RH and was only measured at 80 % RH. Thus, the aerosol hygroscopicity parameter κf(RH) was derived from f(80 %,525 nm), and κf(RH) represents a diameter-independent hygroscopicity parameter κ that fits the observed f(80 %,525 nm) best and is solved through an iteration algorithm. Although Kuang et al. (2017) proposed a simple method for deriving κf(RH) based only on measurements of the humidified nephelometer system, in this study, the more traditional method described therein was adopted to retrieve κf(RH), which uses measurements of PNSD as inputs of Mie theory and the κ-Köhler theory. The idea of deriving κf(RH) from aerosol light scattering measurements was first proposed by Chen et al. (2014); however, the physical understanding of κf(RH) was not mathematically interpreted until the study of Kuang et al. (2020a). Briefly, κf(RH) can be approximately understood as the overall hygroscopicity of aerosol particles with aerosol scattering coefficient contribution as the weighting function for size-resolved κ distribution. Results of Kuang et al. (2020a) demonstrated that for typical continental aerosols, κf(RH) represents the overall hygroscopicity of aerosol particles with a dry diameter range between 200 and 800 nm; thus no matter if κf(RH) values were retrieved based on aerosol light scattering enhancement factor measurements downstream of a PM10 or a PM1 impactor, they are almost the same, which was confirmed by direct measurements in Kuang et al. (2020a) (observed average relative difference of about 3.5 %).
3.2 Organic aerosol hygroscopicity derivation based on aerosol chemical composition and optical hygroscopicity measurements
The aerosol hygroscopicity parameter κ can be calculated from aerosol chemical composition measurements (κchem) on the basis of the volume mixing rule; thus the organic aerosol hygroscopicity parameter κOA was usually estimated through closure between measured κ and estimated κ using aerosol chemical measurements. In this study, the size-resolved aerosol chemical compositions of PM1 were measured using the SP-AMS; however, the overall aerosol hygroscopicity was only derived based on aerosol light scattering measurements of PM10 bulk aerosols. Results of Kuang et al. (2020a) demonstrated that κchem calculated based on bulk chemical compositions of PM1 are quite consistent with κf(RH) of PM1 () and therefore also consistent with κf(RH) of PM10 ().
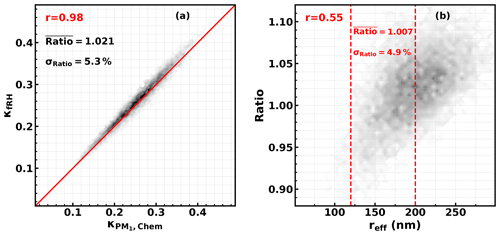
Figure 1Simulated and ; red texts give correlation coefficients, ratio = , reff is the effective radius of the aerosol populations, and dashed red lines show the reff range during the field campaign of this study.
However, simulation results in Kuang et al. (2020a) demonstrated that the ratio between and varies with PNSD and size-resolved κ distributions, and the applicability of this conclusion under varying aerosol chemical compositions and size distributions needs further clarification. Thus, we have designed a simulation experiment to simulate the ratio between and , considered to be wide ranges of aerosol chemical compositions and size distributions. Details of the simulation are introduced in Part 2 of the Supplement. The simulated results are shown in Fig. 1. The results show that the average relative difference was 2.1 ± 5.3 %, which demonstrates that in general can be used to represent under varying atmospheric conditions. The results also show that the ratio is positively correlated with the effective radius of the aerosol population, which means that different levels of bias may exist under different PNSD conditions, and for an effective radius range of this field campaign, the average relative difference is 0.7 ± 4.9 %. Given this, we have further simulated the κf(RH) of PM10 and κchem of PM1 under different PNSDs of this campaign coupled with different size-resolved κ distribution scenarios (as shown in Fig. S4a in the Supplement). As shown in the results in Fig. S4b, and are quite close to each other, and the simulated average relative difference was −0.4±3 %. Thus, was used as the measured in the following discussions.
The SP-AMS measures size-resolved PM1 mass concentrations of , , , Cl− and organic aerosol, thus providing their bulk mass concentrations. A simplified ion pairing scheme was used to derive mass concentrations of different inorganic salts (as listed in Table 1) based on measured bulk ion mass concentrations (Gysel et al., 2007; Wu et al., 2016). Note that the hygroscopicity parameter was measured at RH of 80 %, and the κ values of ammonium sulfate and ammonium nitrate at 80 % RH were predicted using the Extended Aerosol Inorganic Model (E-AIM), whose predictions for ammonium nitrate and ammonium sulfate have been proven to be consistent with laboratory results (Luo et al., 2020; Jing et al., 2018), and those of potassium chloride and ammonium bisulfate were consistent with Liu et al. (2014)
Note that Cl− was coupled with K+ due to the fact that biomass burning events prevailed during this field campaign. The simple volume mixing rule called Zdanovskii–Stokes–Robinson (ZSR) was usually used for κchem calculations; that is, bulk κchem of PM1 can be calculated on the basis of volume fractions of different compounds (Petters and Kreidenweis, 2007) using the following equation:
where κi is the hygroscopicity parameter κ of compound i, and εi is the volume fraction of compound i in the mixture (, Vi and Vtot are volume of compound i and total aerosol volume of PM1). Based on Eq. (2) and Table 1, κchem can be formulated as follows:
where κOA and εOA are κ and volume fraction of entire organic aerosol populations, and κX and εX are κ and volume fraction of aerosol constituents which are beyond the detection ability of the SP-AMS. These unidentified aerosol species in continental regions are likely dust but are still possibly composed of other components such as biogenic primary aerosol. On the basis of current literature reports, dust is nearly hydrophobic and varies a lot, with κ of mineral dust and road dust as well as oil or coal fly ash in the range of 0.01 to 0.08 (Koehler et al., 2009; Peng et al., 2020). In this paper, the unidentified part is assumed as dust, and κX is arbitrarily specified as 0.05. The εX is estimated as the PM1 volume concentration () difference between that measured by the SMPS and calculated from volume concentration summation of chemical compounds listed in Table 1 and volume concentrations of BC and organic aerosol, and the estimated average contribution of εX during this campaign is 13 %, as shown in Fig. S6. In the volume concentration calculations of BC and organic aerosol, a BC density of 1.7 g cm−3 was assumed, and organic aerosol density is calculated based on the density parameterization scheme proposed by Kuwata et al. (2012) using the organic aerosol elemental ratios O:C and H:C measured by the SP-AMS as input parameters. In addition, κBC was set to zero due to the hydrophobic property of BC particles. Then, κOA can be estimated based on measured κchem using the following formula:
The effects of κchem perturbations, aerosol mass concentrations, and κX perturbations on κOA derivations are simulated using the Monte Carlo method for each data point of the κOA time series (1000 cases are randomly produced for each κOA data point), and average effects are summarized in Table 2. The perturbation parameter of κchem is based on the simulation results using PNSDs of this field campaign shown in Fig. S4. The perturbation parameters of aerosol mass concentrations are consistent with Hong et al. (2018), and that of is from Ma et al. (2011). The perturbation parameter of κX is specified based on the fact that κ of dust in general ranges from 0.01 to 0.08. The results show that the accuracy of using to represent mostly affects κOA derivations.
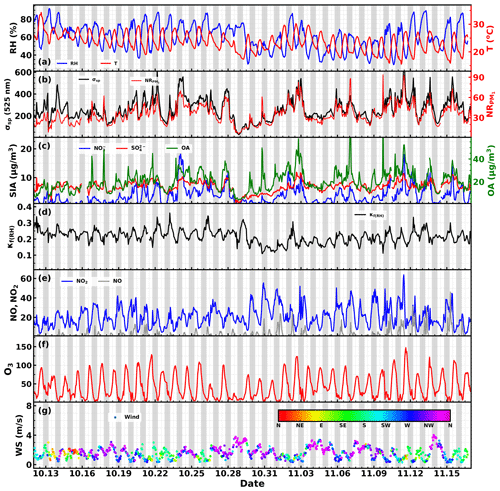
Figure 2Time series of (a) RH and temperature; (b) aerosol scattering coefficient at 525 nm and mass concentrations of PM1 non-refractory components; (c) mass concentrations of sulfate, nitrate and organic aerosol; (d) the hygroscopicity parameter κ retrieved from aerosol light scattering enhancement measurements; (e) NO and NO2 concentrations; (f) O3 concentration; (g) wind speed and direction. Shaded gray areas corresponding to nighttime periods.
4.1 Overview of the campaign data
The time series of meteorological parameters such as wind speed, wind direction, RH and ambient air temperature, aerosol scattering coefficients, aerosol hygroscopicity parameter κf(RH), mass concentrations of aerosol components, and gas pollutant concentrations are shown in Fig. 2. During this campaign, the RH mainly ranged from 50 % to 80 % with an average (± 1σ) of 60 ± 14 %, with the nighttime RH frequently exceeding 70 %, which favors the nighttime aqueous-phase chemistry. Temperatures mainly ranged from 18 to 28 ∘C, with an average (± 1σ) of 23.6 ± 3.3 ∘C, indicating a relatively warm state during this campaign though in the autumn. The aerosol scattering coefficients at 525 nm (σsp,525) shown in Fig. 2b demonstrate that σsp,525 generally ranged between 20 and 600 Mm−1, with an average (± 1σ) of 256 ± 102 Mm−1, indicating moderately polluted conditions during this campaign. The non-refractory mass concentrations of PM1 (NR-PM1) measured by the SP-AMS ranged from 1 to 94 µg m−3, with an average (± 1σ) of 33 ± 14 µg m−3. Nitrate, sulfate, ammonium and organic aerosol contributed on average 19 %, 11 %, 9 % and 58 % to total NR-PM1, which was consistent with the aerosol chemical compositions typically observed in the PRD region featuring organic aerosol as the major constituent of NR-PM1 and higher sulfate concentration than nitrate concentration (Zhou et al., 2020b). However, the NR-PM1 composition profile differed much from those recently observed in urban Guangzhou (Guo et al., 2020), a megacity about 100 km away from Heshan, where sulfate concentrations were on average only slightly higher than nitrate concentrations during autumn and winter seasons of 2017. The large mass contribution of organic aerosol in PM1 resulted in generally moderate ambient aerosol hygroscopicity, with κf(RH) ranging between 0.11 and 0.36 with an average (± 1σ) of 0.22 ± 0.04. The small standard deviation further suggests relatively small variations in aerosol hygroscopicity. Sulfate concentrations showed much less daily and diurnal variations than those of nitrate and organic aerosol, suggesting that the sulfate level was determined by the regional-scale background, while nitrate and organic aerosol concentration was significantly influenced by local production. In particular, the nitrate concentration usually experienced a sharp increase since sunset, and peaks after midnight sometimes even reached beyond sulfate mass concentration. The time series of NO2, NO and O3 concentration are also shown in Fig. 2e and f. NO2 concentration showed distinct diurnal variations and ranged from 3.5 to 64 ppb with an average (± 1σ) of 20.5 ± 10.5 ppb. The NO concentration ranged from almost 0 to 45 ppb with an average (± 1σ) of 2.2 ± 4.5 ppb, indicating generally low concentrations of NO. O3 concentrations ranged from 2 to 147 ppb with an average (± 1σ) of 41.5 ± 31.4 ppb, frequently reaching over 90 ppb in the afternoon, indicating strong daytime photochemistry, and dropped rapidly after sunset towards a very low concentration (usually below 5 ppb) after midnight.
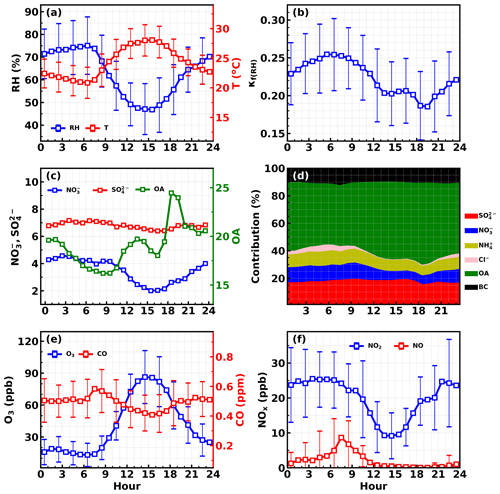
Figure 3Average diurnal variations in (a) RH and T; (b) κf(RH); (c) sulfate, nitrate and organic aerosol; (d) mass fractions of different components; (e) O3 and CO; (f) NO2 and NO.
The average diurnal variations in NO2, NO, O3, CO, aerosol chemical compositions, κf(RH) and meteorological parameters are shown in Fig. 3. O3 concentrations began to increase after sunrise, peaked around 15:00 local time (local time is used throughout the paper) and then began to decrease quickly but drop slower after midnight. Meanwhile, NO concentration began to decrease quickly after sunrise, reached and remained near zero after noontime, and began to slightly increase after 21:00. NO2 concentration increased quickly after 15:00 and reached a plateau after 21:00. Variation characteristics of NO, O3 and NO2 suggest that the relatively low NO concentration resulted in weak titration effects on O3, whereupon typical NO3 chemistry and subsequent N2O5 chemistry might occur, which might contribute to the observed nitrate increase after sunset. However, nitrate concentrations increased quickly after about 16:00 LT and peaked after midnight (about 03:00 LT), indicating that there must be a mechanism responsible for the observed nitrate increase at least before sunset. To dig more into this, the possible pathways of nitrate formation since 16:00 is simulated and discussed in Sect. S3 of the Supplement. The results demonstrate that the repartitioning of HNO3 into the gas and aerosol phase due to the temperature decrease and RH increase can mainly explain the observed nitrate increase. And the strong daytime photochemistry and decrease in NO2 concentration might result in significant production of the gas phase before about 16:00. However, the possible contribution of N2O5 hydrolysis to nitrate formation cannot be excluded.
Under the strong daytime photochemistry and nighttime increase in nitrate, evident diurnal variations in aerosol hygroscopicity were observed. The overall aerosol hygroscopicity variation was generally consistent with the variation pattern of inorganic aerosol fraction in NR-PM1 as shown in Fig. 3d. In detail, the overall variations in nitrate and associated ammonium as well as organic aerosol determine the general hygroscopicity variation pattern: the quick increase in organic aerosol between 16:00 and 19:00 resulted in the quick κf(RH) decrease during this period; since then the general decrease in organic aerosol and increase in nitrate resulted in the increase in κf(RH) until the next morning. The daytime decrease in nitrate and increase in organic aerosol resulted in a κf(RH) decrease before 13:00. Note that sulfate concentration remaining almost constant throughout the day further confirmed the previous statement that local production likely contributed less to sulfate concentration, which can be an indicator of regional air mass status.
These results suggest that typical strong daytime photochemistry and nighttime NO3 chemistry characteristics occurred during this field campaign and played significant roles in diurnal variations in organic aerosol and nitrate, while aged regional air mass determined the sulfate concentration, which provides a good opportunity for investigating how typical daytime photochemistry and nighttime chemistry and aged regional organic aerosol components impact organic aerosol hygroscopicity.
4.2 κOA derivations and its relationship with organic aerosol oxidation state
The organic aerosol hygroscopicity parameter κOA was derived according to the method mentioned in Sect. 3.2, and the results with hourly time resolution are shown in Fig. 4a; κOA revealed daily and diurnal variations and ranged from almost zero to 0.28 with an average (± 1σ) of 0.085 ± 0.05. The relationship between κOA and was further investigated and shown in Fig. 4b. Results demonstrated that κOA and were weakly correlated during this campaign, with most data points falling in the published κOA and relationship band. During this campaign, generally resided in a small range (from about 0.4 to 0.6) with an average (± 1σ) of 0.53 ± 0.06, indicating small variations in , however, featuring drastic variations in κOA. The average diurnal variations in and κOA are shown in Fig. 4c. On average, κOA increased slowly during the nighttime and varied even smaller during most of the daytime. Nevertheless, it experienced a relatively quicker decrease from 17:00 to 19:00, which appeared to be coincident with the quick OA concentration increase as shown in Fig. 3. However, the increased during the period when O3 concentration increased quickly, suggesting that daytime photochemistry drove the OA oxidation during daytime. The key point here is that the diurnal patterns of and κOA differed much from each other, which is why the variation in failed to describe that of κOA.
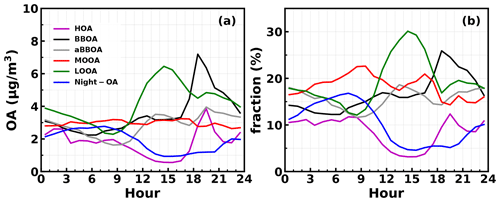
Figure 6Average diurnal variations in mass concentrations (a) and their mass fractions (b) in total OA mass of different PMF OA factors.
The question of which factors were controlling the variations in κOA remains. The relationships between κOA and mass fractions of different PMF OA factors in total OA mass were further investigated and shown in Fig. 5. In general, the average (± 1σ) mass fractions of HOA, BBOA, aBBOA, LOOA, Night-OA and MOOA were 8.7 % (± 7.8 %), 16.5 % (± 8.3 %), 15.9 % (± 10.5 %), 19.1 % (± 10.9 %), 10.4 % (± 6.5 %) and 18.6 % (± 12.2 %), and it means that during this campaign SOA dominates organic aerosol (SOA > 70 %). Two primary OA factors, HOA and BBOA, were related to vehicle exhaust mixed with cooking emissions and to biomass burning emissions, respectively; κOA was negatively correlated with both HOA and BBOA, which is consistent with previous literature reports that primary OA components such as HOA and BBOA are generally hydrophobic (Kuang et al., 2020b). The average diurnal variations in OA PMF factors shown in Fig. 6 demonstrate that both BBOA and HOA peaked near 18:00, which should be associated with the frequently observed biomass burning events and supper cooking in villages near the site. This explained the sharp increase in OA mass and the sharp decrease in κOA near 18:00 as shown in Fig. 4c. However, κOA was also negatively correlated with LOOA (Fig. 5d), whose mass concentration increased rapidly after sunrise and which is likely secondary due to local photochemistry with potential precursors of both biogenic and anthropogenic VOCs considering the observation site is surrounded by small towns and areas with a high percentage of tree cover (Fig. S1). The negative correlation between κOA and LOOA is contradictory with the generally thought that secondary aerosol formation would result in increases in aerosol hygroscopicity. The negative correlation between κOA and LOOA mass fraction explained why failed to describe diurnal variations in κOA: the ratio for LOOA is 0.72, which is only lower than that of MOOA, suggesting that the daytime LOOA formation and decrease in BBOA and HOA mass concentrations drove the increase in daytime , but the κOA did not follow. The κOA was also negatively correlated with Night-OA fraction, which increased during nighttime (Fig. 6). The Night-OA factor was highly correlated with nitrate concentrations (Fig. S5), likely associated with the NO3 nighttime chemistry as discussed in Sect. 4.1. Results of Suda et al. (2014) demonstrated that the addition of an NO3 radical would exert negative impacts on κOA, consistent with the observations shown here. As shown in Fig. 5, κOA was positively correlated with both MOOA and aBBOA, especially with that of MOOA. MOOA was highly correlated with sulfate and showed almost no diurnal variations, indicating that the highly oxygenated ( ∼ 1) MOOA was also more associated with regional air masses. The observed small nighttime increase in κOA could be associated with the slight increase in MOOA mass fraction as shown in Fig. 6b. Similar to LOOA, the aBBOA increased during daytime, which revealed a quick aging process of biomass-burning-related precursors or primary aerosols through photochemistry. Also, the aBBOA factor showed a similar variation trend with ( 151.998; see Fig. S6), which was found as a characteristic ion of a typical aged BBOA component nitrocatechol (Bertrand et al., 2018). However, the resolved average ratio of aBBOA was only 0.39, which is even lower than that of BBOA ( ∼ 0.48), implying that BBOA was likely formed through oxidation of gaseous BBOA precursors rather than the direct oxidation of BBOA. The fact that nitrocatechol is more likely to be contributed by oxidation of gaseous precursors in biomass burning plumes rather than primary biomass burning emissions (Wang et al., 2019) rationalizes this speculation. The similar diurnal characteristics but contrasting effects of LOOA and aBBOA on κOA further explain the weak correlation coefficient between κOA and . However the weak but positive correlation between κOA and should have arose from the much stronger positive correlation between κOA and MOOA mass fractions. LOOA has relatively high , and its abundance usually reaches above that of MOOA during the afternoon; however, its negative effects on κOA were partially compensated by aBBOA, which had lower . In addition, κOA was mostly associated with mass fractions of MOOA with the highest , thus giving rise to the weak but positive relationship between κOA and . As for κOA diurnal variations, daytime increase in aBBOA and LOOA has compensating effects on κOA, and the HOA and Night-OA decrease further complicated its variations.
To test if effects of parameter perturbations on κOA derivations have significant effects on the relationships between κOA and organic aerosol PMF factors, we impose random perturbations on parameters listed in Table 2 in each κOA derivation. The comparison between originally derived κOA and perturbed derivation of κOA results is shown in Fig. S10 in the Supplement. The average difference between derived κOA with and without random errors is 0, and the standard deviation is 0.03. However, the relationships between κOA derived with random errors and organic aerosol PMF factors changed only a little bit, and the results are shown in Fig. S11 in the Supplement.
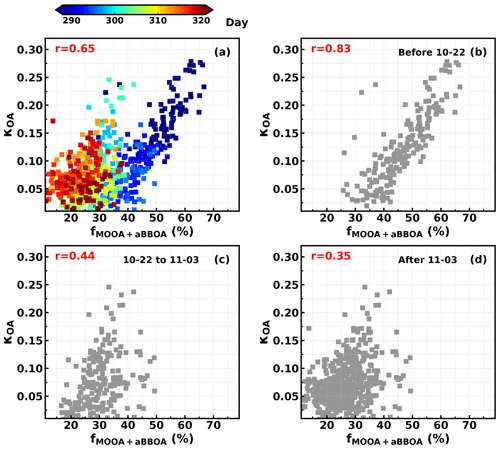
Figure 7Relationships between κOA and fMOOA+aBBOA during (a) the entire observation period, (b) 12 to 22 October, (c) 23 October to 2 November and (d) 3 to 17 November. Colors of scatter points in (a) represent day of the year.
4.3 Discussions on complexity of organic aerosol hygroscopicity parameterizations
As demonstrated in Sect. 4.2, the LOOA factor with higher had negative impacts on κOA, while aBBOA with much lower had positive effects on κOA. These results suggested that is not enough for parameterizing κOA, and the question of what additional parameters are needed or how should they be implemented remains. To further explore this issue, the relationships between κOA and mass fractions of aBBOA + MOOA in total OA mass (fMOOA+aBBOA) were further investigated to manifest the complexity of κOA variations and discuss potential impact factors, with results shown in Fig. 7a. As discussed in Sect. 4.2, both MOOA and aBBOA had positive effects on κOA; however, the relationship between κOA and fMOOA+aBBOA does not yield a higher correlation coefficient than that between κOA and fMOOA, and the results shown in Fig. 7a demonstrate that κOA and fMOOA+aBBOA might have different relationships during different periods. The relationships between κOA and fMOOA+aBBOA during three periods were further investigated and are shown in Fig. 7b–d, which shows that during the first period from 10–12 to 10–22 October, κOA was highly correlated with fMOOA+aBBOA (R = 0.82), with all points falling in a narrow band, suggesting that fMOOA+aBBOA alone could describe the variations in κOA well. However, during the second period (from 23 October to 2 November) and the third period (from 3 to 17 November) the correlation coefficients between κOA and fMOOA+aBBOA were much lower. Obviously, fMOOA+aBBOA during the second and the third period was in general much lower than that during the first period. The time series of κOA and different PMF OA factors are shown in Fig. 8. MOOA displayed relatively small variations during this campaign, highlighting that the regional air mass did not experience tremendous variations and suggesting that changes in other OA factors, especially aBBOA, have resulted in different relationships between κOA and fMOOA+aBBOA. The results in Fig. 8c show that the ratio between aBBOA and BBOA differs much during three periods and declines from the first period to the third period. During the first period, aBBOA was more abundant and was well correlated (R = 0.57) with BBOA. At the same time, aBBOA was positively correlated with HOA (R = 0.49), especially with the cooking emission tracer C6H10O+ (R = 0.60), which could be emitted together with biomass burning emissions, when residents in surrounding villages cooked with biomass fuels. BBOA and aBBOA had comparable levels during the second period; however, aBBOA concentration was much lower than that of BBOA during the third period. It can also be noticed that aBBOA in the second period showed higher correlation with BBOA (R = 0.45) than that in the last period (R = 0.17), which was also the case with cooking emission tracer (R = 0.60 for the second period, 0.36 for the third period). These results suggest that the chemical and physical properties of aBBOA likely changed much within the three periods despite similarities in PMF analysis. Both the primary gas pollutants CO and acetonitrile are highly associated with biomass burning and are often used as indicators of biomass burning events, and the ratio between them can somehow indicate the emission profile changes in biomass burning and thus the primary VOC profile changes. The time series of the ratio between CO and acetonitrile (Fig. 8a) differs much during the three periods, especially for the second and the third period. This difference suggests that although the biomass burning event continued, their emission profiles associated with the burning fuels and conditions likely changed a lot, indicating that aBBOA precursors might have changed during different agricultural activities, thus changing their formation pathways as well as their chemical and physical properties. Other than the aBBOA property changes, changes in OA factor contributions (for example, relative contributions of OA factors other than MOOA and aBBOA) may also impact the relationship between κOA and fMOOA+aBBOA. Also, the chemical and physical properties of Night-OA and LOOA together with the VOC profile may have changed.
In general, the results shown here deliver the following key messages. (1) Although the failed to describe variations in κOA, variations in OA factors that are more related to VOC sources or OA formation pathways could sometimes be found to explain the κOA variations. (2) MOOA, being highly oxygenated and associated with regional air mass, was the most important component that enhanced κOA, which is consistent with current understandings; i.e., organic aerosol aging processes have significant effects on κOA. However, the κOA of secondary organic aerosol does not depend on their (contrary effects of aBBOA and LOOA on κOA). (3) Organic aerosol hygroscopicity of SOA associated with similar sources might differ much under different conditions (effects of aBBOA on κOA differ much during different periods).
These messages might be instructive to the parameterization of κOA in the following ways. (1) We might relate κOA to VOC precursors in laboratory studies, but the laboratory-derived empirical relationship will likely fail in the application of ambient aerosols due to the formation pathway, or the existence of other VOC precursors might result in different chemical properties of ambiently formed SOA, such as functional groups, from the laboratory case. (2) It seems more plausible to find parameters other than ratio to parameterize κOA, which should be independent of sources and associated with the physical properties of OA, such as volatility (Kuwata et al., 2007; Asa-Awuku et al., 2009; Frosch et al., 2013; Kostenidou et al., 2018). Overall, these results further highlighted that κOA parameterizations can be quite difficult and require a lot of future efforts.
In this study, a field campaign was conducted to characterize κOA with high time resolution for the first time at a rural site in the PRD region. The observation results showed that both typical NO3 night chemistry (indicated by extremely low nighttime NO concentration and quick nighttime O3 concentration decrease) and strong daytime photochemical chemistry (indicated by high daytime O3 concentration) prevailed during this field campaign. SOA dominated OA mass (mass fraction > 70 % on average), which provided us with a unique opportunity to investigate influences of SOA formation on variations in organic aerosol hygroscopicity parameter κOA. Six OA factors were resolved by the AMS PMF analysis, including two primary OA factors, HOA and BBOA, and four other secondary OA factors, MOOA, LOOA, aBBOA and Night-OA. The results demonstrated that mass increase in both primary OA factors had negative effects on κOA, which is consistent with current understandings that POA components have quite low hygroscopicity (usually assumed as hydrophilic), while SOA components had distinct effects on κOA. MOOA, with the highest average of 1, was the most important factor that drove the increase in κOA, probably related to regional air mass, and local production contributes little. However, LOOA, with average slightly lower than that of MOOA ( ∼ 0.72), whose mass concentration increased dramatically during daytime due to local production, had negative effects on κOA. Surprisingly, aBBOA, with similar diurnal patterns to that of LOOA, also formed quickly during daytime but displayed much lower (0.39), exerting positive effects on κOA. In addition, κOA revealed weak negative correlation to Night-OA fraction, which increased during nighttime probably due to the NO3 nighttime chemistry. This finding is in general consistent with results of Suda et al. (2014) that the addition of an NO3 radical would exert negative impacts on κOA. As a result, the contrasting effects of LOOA and aBBOA on κOA resulted in the weak correlation coefficient between κOA and ; κOA was mostly associated with mass fractions of MOOA with highest , although its is only a little higher than that of LOOA, which gave rise to the weak but positive relationship between κOA and .
In general, the results presented in this study demonstrate that the failed to describe variations in κOA; however, SOA factors with different VOC sources or from different OA formation pathways might have discrepant influences on the κOA. The contrasting effects of LOOA and aBBOA on κOA demonstrated that VOC precursors from diverse sources and different SOA formation processes may result in SOA with different chemical composition, functional properties and microphysical structure, consequently influencing SOA hygroscopicity. On top of that, the hygroscopicity of SOA associated with similar sources might also differ much during different emission and atmospheric conditions. These results demonstrate that we might relate κOA to VOC precursors in laboratory studies, but the laboratory-derived empirical relationships will likely fail in their application to ambient aerosols due to the more complex formation pathways or the existence of other VOC precursors in the ambient atmosphere and are thus difficult to apply in models. Overall, these results further highlighted that κOA parameterizations are quite complex, and it is important to conduct more research on κOA characterization under different meteorological and source conditions- and examine its relationship with OA and VOC precursor profiles to reach a better characterization and come up with a more appropriate parameterization approach for chemical and climate models.
The data used in this study are available from the corresponding author upon request: Shan Huang (shanhuang_eci@jnu.edu.cn) and Min Shao (mshao@jnu.edu.cn).
The supplement related to this article is available online at: https://doi.org/10.5194/acp-21-10375-2021-supplement.
YK and SH designed the aerosol experiments. YK conceived this research and wrote most of the manuscript, and SH wrote the SPAMS measurements and PMF analysis part. YK, BL and BX conducted aerosol light scattering enhancement factor measurements. QS, WC, WL, SH and WH conducted the SP-AMS measurements, and SH performed the PMF analysis. MC, YK and SH conducted the particle number size distribution measurements. TL and SW performed acetonitrile measurements. MS and BY planned and funded this campaign. YP collected and managed criterial pollutants and meteorological parameters from the Heshan supersite. PZ provided the humidified nephelometer system and contributed to discussions of the scientific findings. DC and DY provided authority of conducting the campaign at the Heshan supersite and gave data availability from the site. All coauthors have contributed to revisions of this paper.
The authors declare that they have no conflict of interest.
This work is supported by the National Natural Science Foundation of China (grant nos. 41805109 and 41807302), National Key Research and Development Program of China (grant nos. 2017YFC0212803, 2016YFC0202206 and 2017YFB0503901), Key-Area Research and Development Program of Guangdong Province (grant no. 2019B110206001), Guangdong Natural Science Foundation (grant no. 2018A030313384), Special Fund Project for Science and Technology Innovation Strategy of Guangdong Province (grant no. 2019B121205004), Guangdong Natural Science Funds for Distinguished Young Scholar (grant no. 2018B030306037), and Guangdong Innovative and Entrepreneurial Research Team Program (grant no. 2016ZT06N263).
This research has been supported by the National Natural Science Foundation of China (grant nos. 41805109 and 41807302), the National Key Research and Development Program of China (grant nos. 2017YFC0212803, 2016YFC0202206, and 2017YFB0503901), the Special Project for Research and Development in Key areas of Guangdong Province (grant no. 2019B110206001), the Natural Science Foundation of Guangdong Province (grant nos. 2018A030313384 and 2018B030306037), the Guangdong Innovative and Entrepreneurial Research Team Program (grant no. 2016ZT06N263), and the Special Fund Project for Science and Technology Innovation Strategy of Guangdong Province (grant no. 2019B121205004).
This paper was edited by Markus Petters and reviewed by two anonymous referees.
Alfarra, M. R., Good, N., Wyche, K. P., Hamilton, J. F., Monks, P. S., Lewis, A. C., and McFiggans, G.: Water uptake is independent of the inferred composition of secondary aerosols derived from multiple biogenic VOCs, Atmos. Chem. Phys., 13, 11769–11789, https://doi.org/10.5194/acp-13-11769-2013, 2013.
Asa-Awuku, A., Engelhart, G. J., Lee, B. H., Pandis, S. N., and Nenes, A.: Relating CCN activity, volatility, and droplet growth kinetics of β-caryophyllene secondary organic aerosol, Atmos. Chem. Phys., 9, 795–812, https://doi.org/10.5194/acp-9-795-2009, 2009.
Bertrand, A., Stefenelli, G., Jen, C. N., Pieber, S. M., Bruns, E. A., Ni, H., Temime-Roussel, B., Slowik, J. G., Goldstein, A. H., El Haddad, I., Baltensperger, U., Prévôt, A. S. H., Wortham, H., and Marchand, N.: Evolution of the chemical fingerprint of biomass burning organic aerosol during aging, Atmos. Chem. Phys., 18, 7607–7624, https://doi.org/10.5194/acp-18-7607-2018, 2018.
Canagaratna, M. R., Jayne, J. T., Jimenez, J. L., Allan, J. D., Alfarra, M. R., Zhang, Q., Onasch, T. B., Drewnick, F., Coe, H., Middlebrook, A., Delia, A., Williams, L. R., Trimborn, A. M., Northway, M. J., DeCarlo, P. F., Kolb, C. E., Davidovits, P., and Worsnop, D. R.: Chemical and microphysical characterization of ambient aerosols with the aerodyne aerosol mass spectrometer, Mass Spectrom. Rev., 26, 185–222, https://doi.org/10.1002/mas.20115, 2007.
Cerully, K. M., Bougiatioti, A., Hite Jr., J. R., Guo, H., Xu, L., Ng, N. L., Weber, R., and Nenes, A.: On the link between hygroscopicity, volatility, and oxidation state of ambient and water-soluble aerosols in the southeastern United States, Atmos. Chem. Phys., 15, 8679–8694, https://doi.org/10.5194/acp-15-8679-2015, 2015.
Chen, J., Zhao, C. S., Ma, N., and Yan, P.: Aerosol hygroscopicity parameter derived from the light scattering enhancement factor measurements in the North China Plain, Atmos. Chem. Phys., 14, 8105–8118, https://doi.org/10.5194/acp-14-8105-2014, 2014.
Deng, Y., Yai, H., Fujinari, H., Kawana, K., Nakayama, T., and Mochida, M.: Diurnal variation and size dependence of the hygroscopicity of organic aerosol at a forest site in Wakayama, Japan: their relationship to CCN concentrations, Atmos. Chem. Phys., 19, 5889–5903, https://doi.org/10.5194/acp-19-5889-2019, 2019.
Drinovec, L., Močnik, G., Zotter, P., Prévôt, A. S. H., Ruckstuhl, C., Coz, E., Rupakheti, M., Sciare, J., Müller, T., Wiedensohler, A., and Hansen, A. D. A.: The “dual-spot” Aethalometer: an improved measurement of aerosol black carbon with real-time loading compensation, Atmos. Meas. Tech., 8, 1965–1979, https://doi.org/10.5194/amt-8-1965-2015, 2015.
Frosch, M., Bilde, M., Nenes, A., Praplan, A. P., Jurányi, Z., Dommen, J., Gysel, M., Weingartner, E., and Baltensperger, U.: CCN activity and volatility of β-caryophyllene secondary organic aerosol, Atmos. Chem. Phys., 13, 2283–2297, https://doi.org/10.5194/acp-13-2283-2013, 2013.
Gunthe, S. S., Rose, D., Su, H., Garland, R. M., Achtert, P., Nowak, A., Wiedensohler, A., Kuwata, M., Takegawa, N., Kondo, Y., Hu, M., Shao, M., Zhu, T., Andreae, M. O., and Pöschl, U.: Cloud condensation nuclei (CCN) from fresh and aged air pollution in the megacity region of Beijing, Atmos. Chem. Phys., 11, 11023–11039, https://doi.org/10.5194/acp-11-11023-2011, 2011.
Guo, J., Zhou, S., Cai, M., Zhao, J., Song, W., Zhao, W., Hu, W., Sun, Y., He, Y., Yang, C., Xu, X., Zhang, Z., Cheng, P., Fan, Q., Hang, J., Fan, S., Wang, X., and Wang, X.: Characterization of submicron particles by time-of-flight aerosol chemical speciation monitor (ToF-ACSM) during wintertime: aerosol composition, sources, and chemical processes in Guangzhou, China, Atmos. Chem. Phys., 20, 7595–7615, https://doi.org/10.5194/acp-20-7595-2020, 2020.
Gysel, M., Crosier, J., Topping, D. O., Whitehead, J. D., Bower, K. N., Cubison, M. J., Williams, P. I., Flynn, M. J., McFiggans, G. B., and Coe, H.: Closure study between chemical composition and hygroscopic growth of aerosol particles during TORCH2, Atmos. Chem. Phys., 7, 6131–6144, https://doi.org/10.5194/acp-7-6131-2007, 2007.
Hong, J., Xu, H., Tan, H., Yin, C., Hao, L., Li, F., Cai, M., Deng, X., Wang, N., Su, H., Cheng, Y., Wang, L., Petäjä, T., and Kerminen, V.-M.: Mixing state and particle hygroscopicity of organic-dominated aerosols over the Pearl River Delta region in China, Atmos. Chem. Phys., 18, 14079–14094, https://doi.org/10.5194/acp-18-14079-2018, 2018.
Jimenez, J. L., Canagaratna, M. R., Donahue, N. M., Prevot, A. S. H., Zhang, Q., Kroll, J. H., DeCarlo, P. F., Allan, J. D., Coe, H., Ng, N. L., Aiken, A. C., Docherty, K. S., Ulbrich, I. M., Grieshop, A. P., Robinson, A. L., Duplissy, J., Smith, J. D., Wilson, K. R., Lanz, V. A., Hueglin, C., Sun, Y. L., Tian, J., Laaksonen, A., Raatikainen, T., Rautiainen, J., Vaattovaara, P., Ehn, M., Kulmala, M., Tomlinson, J. M., Collins, D. R., Cubison, M. J., Dunlea, J., Huffman, J. A., Onasch, T. B., Alfarra, M. R., Williams, P. I., Bower, K., Kondo, Y., Schneider, J., Drewnick, F., Borrmann, S., Weimer, S., Demerjian, K., Salcedo, D., Cottrell, L., Griffin, R., Takami, A., Miyoshi, T., Hatakeyama, S., Shimono, A., Sun, J. Y., Zhang, Y. M., Dzepina, K., Kimmel, J. R., Sueper, D., Jayne, J. T., Herndon, S. C., Trimborn, A. M., Williams, L. R., Wood, E. C., Middlebrook, A. M., Kolb, C. E., Baltensperger, U., and Worsnop, D. R.: Evolution of Organic Aerosols in the Atmosphere, Science, 326, 1525–1529, https://doi.org/10.1126/science.1180353, 2009.
Jin, X., Wang, Y., Li, Z., Zhang, F., Xu, W., Sun, Y., Fan, X., Chen, G., Wu, H., Ren, J., Wang, Q., and Cribb, M.: Significant contribution of organics to aerosol liquid water content in winter in Beijing, China, Atmos. Chem. Phys., 20, 901–914, https://doi.org/10.5194/acp-20-901-2020, 2020.
Jing, B., Wang, Z., Tan, F., Guo, Y., Tong, S., Wang, W., Zhang, Y., and Ge, M.: Hygroscopic behavior of atmospheric aerosols containing nitrate salts and water-soluble organic acids, Atmos. Chem. Phys., 18, 5115–5127, https://doi.org/10.5194/acp-18-5115-2018, 2018.
Koehler, K. A., Kreidenweis, S. M., DeMott, P. J., Petters, M. D., Prenni, A. J., and Carrico, C. M.: Hygroscopicity and cloud droplet activation of mineral dust aerosol, Geophys. Res. Lett., 36, L08805, https://doi.org/10.1029/2009GL037348, 2009.
Kostenidou, E., Karnezi, E., Hite Jr., J. R., Bougiatioti, A., Cerully, K., Xu, L., Ng, N. L., Nenes, A., and Pandis, S. N.: Organic aerosol in the summertime southeastern United States: components and their link to volatility distribution, oxidation state and hygroscopicity, Atmos. Chem. Phys., 18, 5799–5819, https://doi.org/10.5194/acp-18-5799-2018, 2018.
Kuang, Y., Zhao, C., Tao, J., Bian, Y., Ma, N., and Zhao, G.: A novel method for deriving the aerosol hygroscopicity parameter based only on measurements from a humidified nephelometer system, Atmos. Chem. Phys., 17, 6651–6662, https://doi.org/10.5194/acp-17-6651-2017, 2017.
Kuang, Y., He, Y., Xu, W., Zhao, P., Cheng, Y., Zhao, G., Tao, J., Ma, N., Su, H., Zhang, Y., Sun, J., Cheng, P., Yang, W., Zhang, S., Wu, C., Sun, Y., and Zhao, C.: Distinct diurnal variation in organic aerosol hygroscopicity and its relationship with oxygenated organic aerosol, Atmos. Chem. Phys., 20, 865–880, https://doi.org/10.5194/acp-20-865-2020, 2020a.
Kuang, Y., Xu, W., Tao, J., Ma, N., Zhao, C., and Shao, M.: A Review on Laboratory Studies and Field Measurements of Atmospheric Organic Aerosol Hygroscopicity and Its Parameterization Based on Oxidation Levels, Current Pollution Reports, https://doi.org/10.1007/s40726-020-00164-2, 2020b.
Kuwata, M., Kondo, Y., Mochida, M., Takegawa, N., and Kawamura, K.: Dependence of CCN activity of less volatile particles on the amount of coating observed in Tokyo, J. Geophys. Res.-Atmos., 112, D11207, https://doi.org/10.1029/2006JD007758, 2007.
Kuwata, M., Zorn, S. R., and Martin, S. T.: Using Elemental Ratios to Predict the Density of Organic Material Composed of Carbon, Hydrogen, and Oxygen, Environ. Sci. Technol., 46, 787–794, https://doi.org/10.1021/es202525q, 2012.
Lambe, A. T., Onasch, T. B., Massoli, P., Croasdale, D. R., Wright, J. P., Ahern, A. T., Williams, L. R., Worsnop, D. R., Brune, W. H., and Davidovits, P.: Laboratory studies of the chemical composition and cloud condensation nuclei (CCN) activity of secondary organic aerosol (SOA) and oxidized primary organic aerosol (OPOA), Atmos. Chem. Phys., 11, 8913–8928, https://doi.org/10.5194/acp-11-8913-2011, 2011.
Lathem, T. L., Beyersdorf, A. J., Thornhill, K. L., Winstead, E. L., Cubison, M. J., Hecobian, A., Jimenez, J. L., Weber, R. J., Anderson, B. E., and Nenes, A.: Analysis of CCN activity of Arctic aerosol and Canadian biomass burning during summer 2008, Atmos. Chem. Phys., 13, 2735–2756, https://doi.org/10.5194/acp-13-2735-2013, 2013.
Li, X., Song, S., Zhou, W., Hao, J., Worsnop, D. R., and Jiang, J.: Interactions between aerosol organic components and liquid water content during haze episodes in Beijing, Atmos. Chem. Phys., 19, 12163–12174, https://doi.org/10.5194/acp-19-12163-2019, 2019.
Liu, H. J., Zhao, C. S., Nekat, B., Ma, N., Wiedensohler, A., van Pinxteren, D., Spindler, G., Müller, K., and Herrmann, H.: Aerosol hygroscopicity derived from size-segregated chemical composition and its parameterization in the North China Plain, Atmos. Chem. Phys., 14, 2525–2539, https://doi.org/10.5194/acp-14-2525-2014, 2014.
Liu, P., Song, M., Zhao, T., Gunthe, S. S., Ham, S., He, Y., Qin, Y. M., Gong, Z., Amorim, J. C., Bertram, A. K., and Martin, S. T.: Resolving the mechanisms of hygroscopic growth and cloud condensation nuclei activity for organic particulate matter, Nat. Commun., 9, 4076, https://doi.org/10.1038/s41467-018-06622-2, 2018.
Liu, X. and Wang, J.: How important is organic aerosol hygroscopicity to aerosol indirect forcing?, Environ. Res. Lett., 5, 044010, https://doi.org/10.1088/1748-9326/5/4/044010, 2010.
Luo, Q., Hong, J., Xu, H., Han, S., Tan, H., Wang, Q., Tao, J., Ma, N., Cheng, Y., and Su, H.: Hygroscopicity of amino acids and their effect on the water uptake of ammonium sulfate in the mixed aerosol particles, Sci. Total Environ., 734, 139318, https://doi.org/10.1016/j.scitotenv.2020.139318, 2020.
Ma, N., Zhao, C. S., Nowak, A., Müller, T., Pfeifer, S., Cheng, Y. F., Deng, Z. Z., Liu, P. F., Xu, W. Y., Ran, L., Yan, P., Göbel, T., Hallbauer, E., Mildenberger, K., Henning, S., Yu, J., Chen, L. L., Zhou, X. J., Stratmann, F., and Wiedensohler, A.: Aerosol optical properties in the North China Plain during HaChi campaign: an in-situ optical closure study, Atmos. Chem. Phys., 11, 5959–5973, https://doi.org/10.5194/acp-11-5959-2011, 2011.
Marsh, A., Miles, R. E. H., Rovelli, G., Cowling, A. G., Nandy, L., Dutcher, C. S., and Reid, J. P.: Influence of organic compound functionality on aerosol hygroscopicity: dicarboxylic acids, alkyl-substituents, sugars and amino acids, Atmos. Chem. Phys., 17, 5583–5599, https://doi.org/10.5194/acp-17-5583-2017, 2017.
Massoli, P., Lambe, A. T., Ahern, A. T., Williams, L. R., Ehn, M., Mikkilä, J., Canagaratna, M. R., Brune, W. H., Onasch, T. B., Jayne, J. T., Petäjä, T., Kulmala, M., Laaksonen, A., Kolb, C. E., Davidovits, P., and Worsnop, D. R.: Relationship between aerosol oxidation level and hygroscopic properties of laboratory generated secondary organic aerosol (SOA) particles, Geophys. Res. Lett., 37, L24801, https://doi.org/10.1029/2010gl045258, 2010.
Middlebrook, A. M., Bahreini, R., Jimenez, J. L., and Canagaratna, M. R.: Evaluation of Composition-Dependent Collection Efficiencies for the Aerodyne Aerosol Mass Spectrometer using Field Data, Aerosol Sci. Techn., 46, 258–271, https://doi.org/10.1080/02786826.2011.620041, 2012.
Onasch, T. B., Trimborn, A., Fortner, E. C., Jayne, J. T., Kok, G. L., Williams, L. R., Davidovits, P., and Worsnop, D. R.: Soot Particle Aerosol Mass Spectrometer: Development, Validation, and Initial Application, Aerosol Sci. Tech., 46, 804–817, https://doi.org/10.1080/02786826.2012.663948, 2012.
Paatero, P.: Least squares formulation of robust non-negative factor analysis, Chemometr. Intell. Lab., 37, 23–35, https://doi.org/10.1016/S0169-7439(96)00044-5, 1997.
Paatero, P. and Tapper, U.: Positive matrix factorization: A non-negative factor model with optimal utilization of error estimates of data values, Environmetrics, 5, 111–126, https://doi.org/10.1002/env.3170050203, 1994.
Peng, C., Gu, W., Li, R., Lin, Q., Ma, Q., Jia, S., Krishnan, P., Wang, X., and Tang, M.: Large Variations in Hygroscopic Properties of Unconventional Mineral Dust, ACS Earth and Space Chemistry, 4, 1823–1830, https://doi.org/10.1021/acsearthspacechem.0c00219, 2020.
Petters, M. D. and Kreidenweis, S. M.: A single parameter representation of hygroscopic growth and cloud condensation nucleus activity, Atmos. Chem. Phys., 7, 1961–1971, https://doi.org/10.5194/acp-7-1961-2007, 2007.
Petters, S. S., Pagonis, D., Claflin, M. S., Levin, E. J. T., Petters, M. D., Ziemann, P. J., and Kreidenweis, S. M.: Hygroscopicity of Organic Compounds as a Function of Carbon Chain Length and Carboxyl, Hydroperoxy, and Carbonyl Functional Groups, J. Phys. Chem. A, 121, 5164–5174, https://doi.org/10.1021/acs.jpca.7b04114, 2017.
Rastak, N., Pajunoja, A., Acosta Navarro, J. C., Ma, J., Song, M., Partridge, D. G., Kirkevåg, A., Leong, Y., Hu, W. W., Taylor, N. F., Lambe, A., Cerully, K., Bougiatioti, A., Liu, P., Krejci, R., Petäjä, T., Percival, C., Davidovits, P., Worsnop, D. R., Ekman, A. M. L., Nenes, A., Martin, S., Jimenez, J. L., Collins, D. R., Topping, D. O., Bertram, A. K., Zuend, A., Virtanen, A., and Riipinen, I.: Microphysical explanation of the RH-dependent water affinity of biogenic organic aerosol and its importance for climate, Geophys. Res. Lett., 44, 5167–5177, https://doi.org/10.1002/2017gl073056, 2017.
Rickards, A. M. J., Miles, R. E. H., Davies, J. F., Marshall, F. H., and Reid, J. P.: Measurements of the Sensitivity of Aerosol Hygroscopicity and the κ Parameter to the Ratio, J. Phys. Chem. A, 117, 14120–14131, https://doi.org/10.1021/jp407991n, 2013.
Suda, S. R., Petters, M. D., Yeh, G. K., Strollo, C., Matsunaga, A., Faulhaber, A., Ziemann, P. J., Prenni, A. J., Carrico, C. M., Sullivan, R. C., and Kreidenweis, S. M.: Influence of Functional Groups on Organic Aerosol Cloud Condensation Nucleus Activity, Environ. Sci. Technol., 48, 10182–10190, https://doi.org/10.1021/es502147y, 2014.
Ulbrich, I. M., Canagaratna, M. R., Zhang, Q., Worsnop, D. R., and Jimenez, J. L.: Interpretation of organic components from Positive Matrix Factorization of aerosol mass spectrometric data, Atmos. Chem. Phys., 9, 2891–2918, https://doi.org/10.5194/acp-9-2891-2009, 2009.
Wang, Y., Hu, M., Wang, Y., Zheng, J., Shang, D., Yang, Y., Liu, Y., Li, X., Tang, R., Zhu, W., Du, Z., Wu, Y., Guo, S., Wu, Z., Lou, S., Hallquist, M., and Yu, J. Z.: The formation of nitro-aromatic compounds under high NOx and anthropogenic VOC conditions in urban Beijing, China, Atmos. Chem. Phys., 19, 7649–7665, https://doi.org/10.5194/acp-19-7649-2019, 2019.
Wu, Z. J., Poulain, L., Henning, S., Dieckmann, K., Birmili, W., Merkel, M., van Pinxteren, D., Spindler, G., Müller, K., Stratmann, F., Herrmann, H., and Wiedensohler, A.: Relating particle hygroscopicity and CCN activity to chemical composition during the HCCT-2010 field campaign, Atmos. Chem. Phys., 13, 7983–7996, https://doi.org/10.5194/acp-13-7983-2013, 2013.
Wu, Z. J., Zheng, J., Shang, D. J., Du, Z. F., Wu, Y. S., Zeng, L. M., Wiedensohler, A., and Hu, M.: Particle hygroscopicity and its link to chemical composition in the urban atmosphere of Beijing, China, during summertime, Atmos. Chem. Phys., 16, 1123–1138, https://doi.org/10.5194/acp-16-1123-2016, 2016.
Xu, W., Kuang, Y., Bian, Y., Liu, L., Li, F., Wang, Y., Xue, B., Luo, B., Huang, S., Yuan, B., Zhao, P., and Shao, M.: Current Challenges in Visibility Improvement in Southern China, Environ. Sci. Tech. Let., 7, 395–401, https://doi.org/10.1021/acs.estlett.0c00274, 2020.
Yeung, M. C., Lee, B. P., Li, Y. J., and Chan, C. K.: Simultaneous HTDMA and HR-ToF-AMS measurements at the HKUST Supersite in Hong Kong in 2011, J. Geophys. Res.-Atmos., 119, 9864–9883, https://doi.org/10.1002/2013JD021146, 2014.
Zhao, D. F., Buchholz, A., Kortner, B., Schlag, P., Rubach, F., Fuchs, H., Kiendler-Scharr, A., Tillmann, R., Wahner, A., Watne, Å. K., Hallquist, M., Flores, J. M., Rudich, Y., Kristensen, K., Hansen, A. M. K., Glasius, M., Kourtchev, I., Kalberer, M., and Mentel, Th. F.: Cloud condensation nuclei activity, droplet growth kinetics, and hygroscopicity of biogenic and anthropogenic secondary organic aerosol (SOA), Atmos. Chem. Phys., 16, 1105–1121, https://doi.org/10.5194/acp-16-1105-2016, 2016.
Zhou, W., Xu, W., Kim, H., Zhang, Q., Fu, P., Worsnop, D. R., and Sun, Y.: A review of aerosol chemistry in Asia: insights from aerosol mass spectrometer measurements, Environmental Science: Processes & Impacts, 22, 1616–1653, https://doi.org/10.1039/D0EM00212G, 2020a.
Zhou, W., Xu, W., Kim, H., Zhang, Q., Fu, P., Worsnop, D. R., and Sun, Y.: A review of aerosol chemistry in Asia: insights from aerosol mass spectrometer measurements, Environmental Science: Processes & Impacts, 22, 1616–1653, https://doi.org/10.1039/D0EM00212G, 2020b.