the Creative Commons Attribution 4.0 License.
the Creative Commons Attribution 4.0 License.
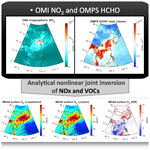
An inversion of NOx and non-methane volatile organic compound (NMVOC) emissions using satellite observations during the KORUS-AQ campaign and implications for surface ozone over East Asia
Amir H. Souri
Caroline R. Nowlan
Gonzalo González Abad
Donald R. Blake
Alan Fried
Andrew J. Weinheimer
Armin Wisthaler
Jung-Hun Woo
Qiang Zhang
Christopher E. Chan Miller
Xiong Liu
Kelly Chance
The absence of up-to-date emissions has been a major impediment to accurately simulating aspects of atmospheric chemistry and to precisely quantifying the impact of changes in emissions on air pollution. Hence, a nonlinear joint analytical inversion (Gauss–Newton method) of both volatile organic compounds (VOCs) and nitrogen oxide (NOx) emissions is made by exploiting the Smithsonian Astrophysical Observatory (SAO) Ozone Mapping and Profiler Suite Nadir Mapper (OMPS-NM) formaldehyde (HCHO) and the National Aeronautics and Space Administration (NASA) Ozone Monitoring Instrument (OMI) tropospheric nitrogen dioxide (NO2) columns during the Korea–United States Air Quality (KORUS-AQ) campaign over East Asia in May–June 2016. Effects of the chemical feedback of NOx and VOCs on both NO2 and HCHO are implicitly included by iteratively optimizing the inversion. Emission uncertainties are greatly narrowed (averaging kernels > 0.8, which is the mathematical presentation of the partition of information gained from the satellite observations with respect to the prior knowledge) over medium- to high-emitting areas such as cities and dense vegetation. The prior amount of total NOx emissions is mainly dictated by values reported in the MIX-Asia 2010 inventory. After the inversion we conclude that there is a decline in emissions (before, after, change) for China (87.94±44.09 Gg d−1, 68.00±15.94 Gg d−1, −23 %), North China Plain (NCP) (27.96±13.49 Gg d−1, 19.05±2.50 Gg d−1, −32 %), Pearl River Delta (PRD) (4.23±1.78 Gg d−1, 2.70±0.32 Gg d−1, −36 %), Yangtze River Delta (YRD) (9.84±4.68 Gg d−1, 5.77±0.51 Gg d−1, −41 %), Taiwan (1.26±0.57 Gg d−1, 0.97±0.33 Gg d−1, −23 %), and Malaysia (2.89±2.77 Gg d−1, 2.25±1.34 Gg d−1, −22 %), all of which have effectively implemented various stringent regulations. In contrast, South Korea (2.71±1.34 Gg d−1, 2.95±0.58 Gg d−1, +9 %) and Japan (3.53±1.71 Gg d−1, 3.96±1.04 Gg d−1, +12 %) are experiencing an increase in NOx emissions, potentially due to an increased number of diesel vehicles and new thermal power plants. We revisit the well-documented positive bias (by a factor of 2 to 3) of MEGAN v2.1 (Model of Emissions of Gases and Aerosols from Nature) in terms of biogenic VOC emissions in the tropics. The inversion, however, suggests a larger growth of VOCs (mainly anthropogenic) over NCP (25 %) than previously reported (6 %) relative to 2010. The spatial variation in both the magnitude and sign of NOx and VOC emissions results in nonlinear responses of ozone production and loss. Due to a simultaneous decrease and increase in NOx∕VOC over NCP and YRD, we observe a ∼53 % reduction in the ratio of the chemical loss of NOx (LNOx) to the chemical loss of ROx (RO2+HO2) over the surface transitioning toward NOx-sensitive regimes, which in turn reduces and increases the afternoon chemical loss and production of ozone through NO2+OH (−0.42 ppbv h−1)∕HO2 (and RO2)+NO (+0.31 ppbv h−1). Conversely, a combined decrease in NOx and VOC emissions in Taiwan, Malaysia, and southern China suppresses the formation of ozone. Simulations using the updated emissions indicate increases in maximum daily 8 h average (MDA8) surface ozone over China (0.62 ppbv), NCP (4.56 ppbv), and YRD (5.25 ppbv), suggesting that emission control strategies on VOCs should be prioritized to curb ozone production rates in these regions. Taiwan, Malaysia, and PRD stand out as regions undergoing lower MDA8 ozone levels resulting from the NOx reductions occurring predominantly in NOx-sensitive regimes.
- Article
(13879 KB) - Full-text XML
-
Supplement
(2887 KB) - BibTeX
- EndNote
The study of ozone (O3) formation within the troposphere in East Asia is of global importance. This significant pollutant is not confined to the source, as it spreads hemispherically through the air, affecting background concentrations as far away as the US. A study by Lin et al. (2017) provided modeling evidence of enhancements in springtime surface ozone levels (+0.5 ppbv yr−1) in the western US in 1980–2014 solely due to the tripling of Asian anthropogenic emissions over the period. As more studies have provided information on the impact of ozone pollution on both human health and crop yields, Chinese governmental regulatory agencies have begun to take action on cutting the amount of NOx (NO+NO2) emissions since 2011–2012 (Gu et al., 2013; Reuter et al., 2014; Krotkov et al., 2016; de Foy et al., 2016; Souri et al., 2017a); however, no effective policy on volatile organic compound (VOC) emissions had been put into effect prior to 2016 (Stavrakou et al., 2017; Souri et al., 2017a; Shen et al., 2019; M. Li et al., 2019), with the exception of the Pearl River Delta (PRD) (Zhong et al., 2013). In addition to China, a number of governments including those of Malaysia and Taiwan have put a great deal of effort into shifting their energy pattern from consuming fossil fuels to renewable sources (Trappey el al., 2012; Chua and Oh, 2011). On the other hand, using satellite observations, Irie et al. (2016) and Souri et al. (2017a) revealed a systematic hiatus in the reduction of NOx over South Korea and Japan, potentially due to increases in the number of diesel vehicles and new thermal power plants built to compensate for the collapse of the Fukushima nuclear power plant in 2011. Therefore, it is interesting to quantify to what extent these policies have impacted ozone pollution.
Unraveling the origin of ozone is complicated by a number of factors encompassing the nonlinearity of ozone formation to its sources, primarily from NOx and VOCs. Therefore, to be able to quantify the impact of recent emission changes, we have developed a top-down estimate of relevant emission inventories using well-characterized satellite observations. There are a myriad of studies focusing on optimizing bottom-up anthropogenic and biogenic emissions using satellites observations, which provide high spatial coverage, in conjunction with chemical transport models for VOCs (e.g., Palmer et al., 2003; Shim et al., 2005; Curci et al., 2010; Stavrakou et al., 2009, 2011), and NOx (e.g., Martin et al., 2003; Chai et al., 2009; Miyazaki et al., 2017; Souri et al., 2016a, 2017a, 2018). Most inverse modeling studies do not consider both NO2 and formaldehyde (HCHO) satellite-based observations to perform a joint inversion. It has been shown that VOC and NOx emissions can affect the production or loss of each other (Marais et al., 2012; Wolfe et al. 2016; Valin et al., 2016; Souri et al., 2020a). Consequently, a joint method that incorporates both species while minimizing the uncertainties in their emissions is better suited to address this problem. Dealing with this tangled relationship between VOC–NO2 and NOx–HCHO requires an iteratively nonlinear inversion framework to incrementally consider the relationships derived from a chemical transport model. Here we will provide an optimal estimate of NOx and VOC emissions during the KORUS-AQ campaign using the Smithsonian Astrophysical Observatory (SAO) Ozone Mapping and Profiler Suite Nadir Mapper (OMPS-NM) HCHO and the National Aeronautics and Space Administration (NASA) Ozone Monitoring Instrument (OMI) NO2 retrievals, whose accuracy and precisions are characterized against rich observations collected during the campaign. Having a top-down constraint on both emissions permits a more precise quantification of the impact of the recent emission changes on different chemical pathways pertaining to ozone formation and loss.
2.1 Remote sensing measurements
2.1.1 OMPS HCHO
OMPS-NM onboard the Suomi National Polar-orbiting Partnership (Suomi NPP) is a UV-backscattered radiation spectrometer launched in October 2011 (Flynn et al., 2014). Its revisit time is the same as other NASA A-Train satellites, including Aura at approximately 13:30 local time at the Equator in ascending mode. OMPS-NM covers 300–380 nm with a resolution of 1 nm full-width at half-maximum (FWHM). The sensor has a 340×740 pixel charge-coupled device (CCD) array measuring the UV spectra at a spatial resolution of 50×50 km2 at nadir. The HCHO retrieval has been fully described in González Abad et al. (2015, 2016). Briefly, OMPS HCHO slant columns are fit using direct radiance fitting (Chance, 1998) in the spectral range 327.7–356.5 nm. The spectral fit requires a reference spectrum as a function of the cross-track position as it attempts to determine the number of molecules with respect to a reference (i.e., a differential spectrum fitting). To account for this, we use earthshine radiances over a relatively pristine area in the remote Pacific Ocean within −30 to +30 latitude. An upgrade to this reference correction is the use of daily HCHO profiles over monthly mean climatological ones from simulations done by the GEOS-Chem chemical transport model. On average, this leads to a 4 % difference in HCHO total columns with respect to using the monthly mean climatological values (Supplement Fig. S1). The scattering weights describing the sensitivity of the light path through a simulated atmosphere are calculated using VLIDORT (Spurr, 2006). The shape factors used for calculating air mass factors (AMFs) are derived from a regional chemical transport model (discussed later) that is used for carrying out the inversion in the present study. We remove unqualified pixels based on cloud fraction < 40 %, solar zenith angle < 65∘, and a main quality flag provided in the data. We oversample the HCHO columns for the period of May–June 2016 using a Cressman spatial interpolator with a 1∘ radius of influence.
2.1.2 OMI tropospheric NO2
We use NASA OMI tropospheric NO2 (version 3.1) level 2 data, whose retrieval is made in the violet–blue (402–465 nm) due to strong absorption of the molecule in this wavelength range (Levelt et al., 2018). The sensor has a nadir spatial resolution of 13×24 km2, which can extend to 40×160 km2 at the edge of scan lines. A more comprehensive description of the retrieval and the uncertainty associated with the data can be found in Krotkov et al. (2017) and Choi et al. (2020). We remove bad pixels based on cloud fraction < 20 %, solar zenith angle < 65∘ (without the row anomaly), a vertical column density (VCD) quality flag of 0, and terrain reflectivity < 30 %. Similar to the OMPS HCHO, we recalculate AMFs by using shape factors from the chemical transport model used in this study. We oversample the OMI granules using the Cressman interpolator with a 0.25∘ radius of influence.
2.2 Model simulation
To be able to simulate the atmospheric composition, and to perform analytical inverse modeling, we set up a 27 km grid resolution regional chemical transport model using the Community Multiscale Air Quality Modeling System (CMAQ) model (v5.2.1; https://doi.org/10.5281/zenodo.1212601) (Byun and Schere, 2006) that consists of 328×323 grids covering China, Japan, South Korea, Taiwan, and some portions of Russia, India, and South Asia (Fig. 1). The time period covered by the simulation is from April to June 2016. We use the month of April for spin-up. The anthropogenic emissions are based on the monthly MIX-Asia 2010 inventory (Li et al., 2017) in the CB05 mechanism. The anthropogenic emissions are mainly grouped into three different sectors, namely mobile, point, and residential (area) sources. We apply a diurnal scale to the mobile sectors used in the National Emission Inventory (NEI) 2011 emission platform to represent the first-order approximation of traffic patterns. We include biomass burning emissions from the Fire Inventory from NCAR (FINN) v1.6 inventory (Wiedinmyer et al., 2011) and consider the plume rise parametrization used in the GEOS-Chem model (i.e., 60 % of emissions are distributed uniformly in the planetary boundary layer – PBL). We use the offline Model of Emissions of Gases and Aerosols from Nature (MEGAN) v2.1 model (Guenther et al., 2012) following the high-resolution inputs described in Souri et al. (2017). The diurnally varying lateral chemical conditions are simulated by GEOS-Chem v10 (Bey et al., 2001) using the full chemistry mechanism (NOx–Ox–HC–Aer–Br) spun up for a year. With regard to weather modeling, we use the Weather Research and Forecasting (WRF) model v3.9.1 (Skamarock et al., 2008) at the same resolution as that of the CMAQ (∼27 km), but with a wider grid (342×337) and 28 vertical pressure sigma levels. The lateral boundary conditions and the grid nudging inputs are from the global Final (FNL) 0.25∘ resolution model. The major configurations for the WRF-CMAQ model are summarized in Tables 1 and 2.
2.3 Inverse modeling
We attempt to improve our high-dimensional imperfect numerical representation of atmospheric compounds using the well-characterized NO2 and HCHO columns from satellites. We perform an analytical inversion using the WRF-CMAQ model to constrain the relevant bottom-up emission estimation (Souri et al., 2016a, 2017a, 2018). The inversion seeks to solve the following cost function under the assumptions that (i) both observation and emission error covariances follow Gaussian probability density functions with a zero bias, (ii) the observation and emission error covariances are independent, and (iii) the relationship between observations and emissions is not grossly nonlinear:
where x is the inversion estimate (a posteriori) given two sources of data, a priori (xa) and observation (y). So and Se are the error covariance matrices of the observation (instrument) and emission. F is the forward model (here WRF-CMAQ) to project the emissions onto columns. The first term of Eq. (1) attempts to reduce the distance between observations and the simulated columns. The second term incorporates some prior understanding and expectation of the true state of the emissions. The weight of each term is dictated by its covariance matrix. If Se is large compared to So, the a posteriori will be independent of the prior knowledge and, conversely, if So dominates, the final solution will consist mostly of the a priori.
Following the Gauss–Newton method described in Rodgers (2000), we iteratively derive (i.e., i is the index of iteration) the posterior emissions by
where G is the Kalman gain,
and Ki=K(xi) is the Jacobian matrix calculated explicitly from the model (discussed later). The covariance matrix of the a posteriori is calculated by
where is the Jacobian from the ith iteration. Here we iterate Eq. (2) three times. The averaging kernels (A) are given by
The inversion system is complicated by the commonly overlooked fact that observations are biased. For instance, Souri et al. (2018) found that airborne remote sensing observations were high relative to surface Pandora measurements. The overestimation of the VCDs was problematic, since it could have been propagated in the inversion, inducing a bias in the top-down estimation. The authors partly mitigated it by constraining the MODIS albedo, which was assumed to be responsible for the bias. Attempts to reduce the bias resulting from coarse profiles from a global model in calculating gas shape profiles were made by recalculating the shape factors using those from higher-spatial-resolution regional models in other studies (e.g., Souri et al., 2016a; Laughner et al., 2018). For this study, we use abundant observations from the KORUS-AQ campaign and follow the intercomparison platform proposed by Zhu et al. (2016, 2020) using aircraft observations to be able to mitigate the biases in HCHO columns. Based on the corrected global model as a benchmark (Fig. S2), we scale up all OMPS HCHO columns by 20 %. To mitigate the potential biases in OMI NO2, we exclusively followed the values reported over the KORUS-AQ period in Choi et al. (2020). We increase the NO2 concentration uniformly by 33.9 % (see Table A3 in their paper).
We calculate the covariance matrix of observations using the column uncertainty variable provided in the satellite datasets and consider them to be random errors associated with spectrum fitting. We consider 25 % random errors for the air mass factors. Therefore, these values (as random errors) are significantly lowered by oversampling the data over the course of 2 months. In addition to that, we consider a fixed error for all pixels due to variability that exists in the applied bias correction (3.61×1015 molec. cm−2 for NO2 and 4.62×1015 molec. cm−2 for HCHO). This error is based on the root mean square error (RMSE) obtained from the mentioned studies used for removing biases. Despite the fact that we do not account for non-diagonal elements of the covariance matrices, the incremental updates of G adjusted by both NO2 and HCHO observations should better translate the covariance matrices into the emission space.
To increase the degree of freedom for the optimization, we combine all sector emissions including anthropogenic, biomass burning, and biogenic emissions for NOx and VOCs. Therefore, we use the following formula to estimate the variance of the a priori:
where f denotes the fraction of the emission sector with respect to the total emissions, and σ is the standard deviation of each sector category, which is calculated from the average of each sector to a relative error listed in Table 3.
For the same purpose (enhancing the amount of information gained from satellite observations) and to increase computational speed, we reduce the dimension of the state vectors (emissions) by aggregating them. However, grouping emissions into certain zones could also introduce another type of uncertainty, known as the aggregation error. We choose optimally aggregated zones by running the inversion multiple times, each with a certain selection of state vectors (Turner and Jacob, 2015). As in our previous study in Souri et al. (2018), we use the Gaussian model mixture (GMM) method to cluster emissions into certain zones that share roughly similar features and investigate which combinations will lead to a minimum of the sum of aggregation and smoothing errors.
In order to create the K matrix, one must estimate the impact of changes in emissions for each of the aggregated zones on the concentrations of a target compound, which is calculated using the CMAQ direct decoupled method (DDM) (Dunker et al., 1989; Cohan et al., 2005). For instance, the first row and column of K denoting the response of the first grid cell to a zonal emission can be obtained by
where is the DDM output in molecules per square centimeter (molec. cm−2) for the first row and column. It explains the resultant change in the NO2 column by changing one unit of total NOx emissions. The same concept will be applied to HCHO and VOC emissions. We do not consider the transport between zones due to computational burdens; therefore, we assume that the HCHO and NO2 columns are mostly confined to their sources in the 2-month averages. The advantage of using CMAQ DDM to estimate the sensitivity lies in the fact that it calculates the local gradient, which better represents the nonlinear relationship between the emissions and the columns (Souri et al., 2017a, 2018); this, in turn, reduces the number of iterations.
It is essential to first evaluate some key meteorological variables because large errors in the weather can complicate the inversion (e.g., Liu et al. 2017). In order to validate the performance of the WRF model in terms of a number of meteorological variables, including surface temperature, relative humidity, and winds, we use more than 1100 surface measurements from integrated surface database (ISD) stations (https://www.ncdc.noaa.gov/isd, last access: 12 September 2019) over the domain in May–June 2016. Table 4 lists the comparison of the model and the observations for the mentioned variables. Our model demonstrates a very low bias (0.6 ∘C) with regard to surface temperature. We find a reasonable correspondence in terms of relative humidity, indicating a fair water vapor budget in the model. The largest discrepancy between the model and observations with respect to temperature and humidity occurs in grid cells that are in the proximity of the boundary conditions (not shown). Concerning the wind components, the deviation of the model from the observations is smaller than results obtained in a relatively flat area like Houston in Souri et al. (2016a, b).
Table 4Statistics for surface temperature, relative humidity, and wind. Corr – correlation; RMSE – root mean square error; MAE – mean absolute error; MB – mean bias; O – observation; M – model; O_M – observed mean; M_M – model mean; SD – standard deviation. Units for RMSE, MAE, MB/O_M, M_M, O_SD, and M_SD: degrees Celsius for temperature, percentage for relative humidity, and meters per second for wind.
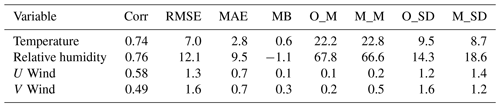
Prior to updating the emissions, we find it necessary to shed light on the spatial distribution of tropospheric NO2 and HCHO columns from both observations and the model, as well as their potential differences relative to their key precursors' emissions. Subsequently, we report the results from the inverse modeling and the uncertainty associated with the top-down estimation; moreover, we wish to assess how much information is gained from utilizing satellite observations via the calculation of averaging kernels. Finally, observations are used to verify, to some extent, the accuracy of our top-down emission estimations.
4.1 NOx
The first row in Fig. 2 illustrates tropospheric NO2 columns from the regional model, OMI (using adjusted AMF and bias-corrected), and the natural logarithmic ratio of both quantities in May–June 2016 at ∼13:30 LST over Asia. The second row depicts daily mean values of dominant sources of NOx, namely as biogenic, anthropogenic, and biomass burning emissions (that are subject to change after the inversion). A high degree of correlation between anthropogenic NOx emissions and NO2 columns implies the predominant production of NO2 from the anthropogenic sources (Logan, 1983). We find a reasonable two-dimensional Pearson correlation (r=0.73) between the modeled and the observed columns. Generally, the WRF-CMAQ largely underestimated (56 %, molec. cm−2) tropospheric NO2 columns with respect to those of OMI over the entire domain. Intuitively segregating the domain into high-emission areas (NOx > 10 t d−1) and low ones (NOx < 10 t d−1) allows for a better understanding of the discrepancy between the model and the observations. In the high NOx areas, the model tends to overestimate tropospheric NO2 columns by 73 % (3.71×1015 molec. cm−2), whereas for the low NOx regions, the model shows a substantial underestimation by 68 % ( molec. cm−2). Such a conflicting bias is confirmed by the contour map of the natural logarithm ratio of OMI to the model in Fig. 2. The large overestimation of the model in terms of NO2 over polluted areas is explained by stringent regulations enacted in various countries in Asia; for instance, Chinese regulatory agencies have recently taken aggressive actions to cut anthropogenic NOx emissions by implementing selective catalytic reductions in power plants, closing a number of coal power plants, and implementing policies on transportation (Zhang et al., 2012; Liu et al., 2016; Reuter et al., 2014; de Foy et al., 2016; Krotkov et al., 2016; Souri et al., 2017a). The highest positive bias in the model is observed over Shanxi Province in China, home to coal production, underscoring the effectiveness of the emission standards at controlling NOx emissions. Likewise, we observe a positive bias in the model over major cities in Japan and South Korea, but the magnitude of the reduction over these cities is substantially smaller than what we observe in China.
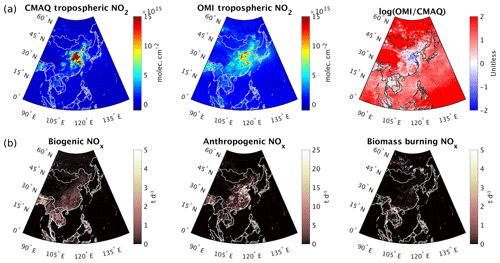
Figure 2(a) Tropospheric NO2 columns from the WRF-CMAQ model, OMI (using adjusted AMFs based on the shape factors derived from the model and bias-corrected following Choi et al., 2020), and the natural logarithmic ratio of OMI ∕ CMAQ during May–June 2016 at ∼13:30 LST. (b) The major sources of NOx emissions in the region including biogenic (soil) emissions simulated by MEGAN, anthropogenic emissions estimated by MIX-Asia (2010), and biomass burning emissions made by FINN. The emissions are the daily mean values based on the emissions in May–June.
The underestimation of the model in the low NOx regions is related to a number of factors, such as the following: (i) the widely reported underestimation of soil (biogenic) NOx emissions due to a lack of precise knowledge of fertilizer use, soil biota, and/or canopy interactions (Jaeglé, et al., 2005; Hudman et al., 2010; Souri et al., 2016a); (ii) the underestimation of the upper-troposphere NO2 due to non-surface emissions (aviation, lightning) or errors in the vertical mixing or moist convection (e.g., Souri et al., 2018); and (iii) a possible overprediction of the lifetime of organic nitrates diminishing background NO2 levels (Canty et al., 2015). Addressing the second issue requires a very high-resolution model with explicit resolving microphysics and large eddy simulations, and the last problem requires more experimental studies to improve organic nitrate chemistry (Romer Present et al., 2020). In this study, we attempt to mitigate the discrepancy between the model and the satellite observations solely by adjusting the relevant emissions. Accordingly, future improvements in physical–chemical processes of models will inevitably offset top-down emission estimates.
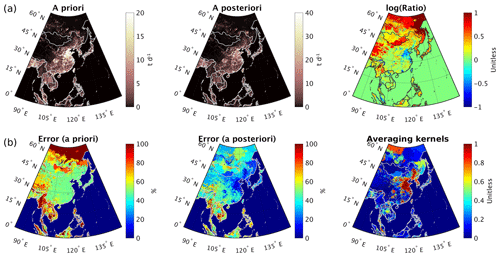
Figure 3(a) Total NOx emissions (i.e., the a priori) constrained by the satellite observations (i.e., the a posteriori) in May–June 2016 and the natural logarithmic ratio of the a posteriori to the a priori. (b) The errors in the a priori based on Table 3, the errors in the top-down estimation, and the averaging kernels (AKs) obtained from the estimation.
The first row in Fig. 3 shows the a priori, the a posteriori, and their ratios in terms of the total NOx emissions in May–June 2016. We observe that the ratios are highly correlated with those of OMI ∕ CMAQ shown in Fig. 2, suggesting that the inversion attempts to reduce the distance between the model and the observations. Major reductions occur over China. The enhancements in NOx emissions are commonly found in rural areas, especially over grasslands located in western–central China and Mongolia. The changes in NOx emissions over South Korea and Japan are positive (Irie et al., 2016; Souri et al., 2017a), mainly due to rapid increases in the number of diesel cars in South Korea and thermal power plants built as a substitution for the Fukushima nuclear plant in Japan. This is especially the case for Japan for which we observe a larger enhancement in total NOx emissions (12 %). The second row in Fig. 3 depicts the relative errors in the a priori, the a posteriori, and averaging kernels (AKs). Relative errors in the a priori are mostly confined to values close to 50 % in polluted areas. They increase further, up to 100 %, in areas experiencing relatively large contributions from biomass burning or biogenic (soil) emissions. Encouragingly, OMI tropospheric NO2 columns in conjunction with the solid mathematical inversion method (Rodgers, 2000) greatly reduce the uncertainties associated with the emissions in polluted areas; we observe AKs close to 1 over major cities or industrial areas. We see the lowest values in AKs over rural areas due to weaker signal-to-noise ratios from the sensor. Therefore, it is desirable but very difficult to improve the model using the sensor with respect to NOx chemistry and emissions in remote areas, evident in the low values of AKs. Table 5 lists the magnitude of the total NOx emissions in several regions (refer to Fig. 1) before and after carrying out the inversion. If we assume that the dominant source of NOx emissions is anthropogenic, the most successful countries at cutting emissions (before, after) are China (87.94±44.09 and 68.00±15.94 Gg d−1), Taiwan (1.26±0.57 and 0.97±0.33 Gg d−1), and Malaysia (2.89±2.77 and 2.25±1.34 Gg d−1). All three countries have successfully implemented regulations to reduce anthropogenic emissions since 2010–2011 (Zhang et al., 2012; Trappey el al., 2012; Chua and Oh, 2011). The uncertainty associated with the top-down estimate improves considerably. The largest reduction in the uncertainty of the emissions is observed over China, a response to a strong signal from OMI.
Table 5NOx emissions before and after carrying out the inversion using OMI ∕ OMPS for different countries in May–June 2016.
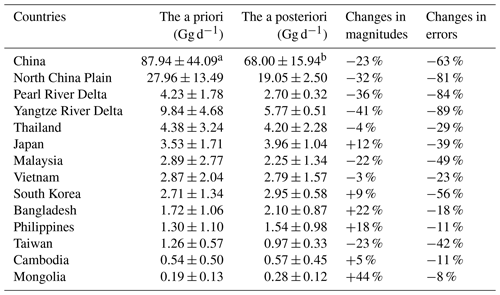
a The errors in the a priori are estimated from Eq. (6). b The errors in the a posteriori are calculated by Eq. (4).
An interesting observation lies in the discrepancy between the natural logarithm ratio of OMI ∕ CMAQ (Fig. 2) and that of the a posteriori to the a priori over the North China Plain (NCP), suggesting that using a bulk ratio (Martin et al., 2003) cannot fully account for possible chemical feedback. The natural logarithm ratio of OMI ∕ CMAQ is consistently lower than the changes in the emission. Two reasons contribute to this effect: (i) as NOx emissions decrease in NOx-saturated areas (i.e., the dominant sink of radicals is through NO2+OH), OH levels essentially increase, resulting in a shorter lifetime of NO2; therefore, to reduce NO2 concentrations, a substantial reduction in NOx (suggested by OMI ∕ CMAQ) is unnecessary, coinciding with results from the inverse modeling. Also, (ii) the CMAQ DDM (Fig. S3) suggests that NO2 columns decrease due to increasing VOC emissions over the region; accordingly, the cross-relationship between NO2 concentrations and VOC emissions partly adds to the discrepancy. It is because of the chemical feedback that recent studies have attempted to enhance the capability of inverse modeling by iteratively adjusting relevant emissions (e.g., Cooper et al., 2017; C. Li et al., 2019). Likewise, our iterative nonlinear inversion shows a superior performance over traditional bulk ratio methods, in part because it incrementally considers the chemical feedback.
To assess the resulting changes in the tropospheric NO2 columns after the inversion, and to validate our results, we compare the simulated values using the a priori and the a posteriori with OMI in Fig. 4. We observe a 64 % reduction in the tropospheric NO2 columns on average over NCP despite only a 32 % reduction in the total NOx emissions over the region, a result of the chemical feedback. The two-dimensional Pearson correlation between the simulation using the a posteriori and OMI increases from 73 % (using the a priori) to 83 %. Both datasets now are in a better agreement as far as the magnitude goes. However, we do not see a significant change in the background values in the new simulation compared to those of OMI due to less certain column observations.
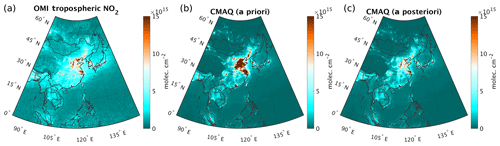
Figure 4(a–c) Tropospheric NO2 columns from OMI, WRF-CMAQ simulated with the prior emissions, and the same model but with the top-down emissions constrained by OMI ∕ OMPS in May–June 2016.
To further validate the results, we compare the NO2 data from the NCAR four-channel chemiluminescence instrument onboard the DC-8 aircraft during the campaign (Fig. S4). These data are not interfered with by the NOz family. The aircraft collected the data over the Korean Peninsula on around 23 d in May–June 2016, covering various altitudes and hours (https://www-air.larc.nasa.gov/cgi-bin/ArcView/korusaq, last access: 8 December 2019). We observe an underestimation of NO2 at the near-surface levels (< 900 hPa) by 19 % (DC-8 =4.50 ppbv, CMAQ =3.67 ppbv). The updated emissions increase the near-surface levels over the Korean Peninsula, which in turn reduces the bias to 11 % (CMAQ =4.02 ppbv).
4.2 VOC
A comparison between HCHO columns from the model and OMPS along with the major sources of VOCs in May–June 2016 is depicted in Fig. 5. Anthropogenic VOCs are emitted from various sources such as solvent use and the mobile and chemical industries (Liu et al., 2008a, b). A reasonable correlation (r=0.78) between the model and OMPS suggests good confidence in the location of emissions. However, the magnitude of HCHO columns between the two datasets strongly disagrees, especially over the tropics where biogenic emissions are large. A myriad of studies have reported a largely positive bias (by a factor of 2–3) associated with isoprene emissions estimated by MEGAN using satellite measurements over the tropics (e.g., Millet et al., 2008; Stavrakou et al., 2009; Marais et al., 2012; Bauwens et al., 2016). To compound, Stavrakou et al. (2011) found a large overestimation in methanol emissions from the same model that can further preclude the accurate estimation of the yield of HCHO. As a response to the overestimation of the biogenic VOCs by MEGAN, we observe a largely positive bias in the simulated HCHO columns ranging from 50 % over the south of China to ∼400 % over Malaysia and Indonesia. As we move away from the hotspot of the biogenic emissions in lower latitudes, the positive bias of the model declines, ultimately turning into a negative bias at higher latitudes. OMPS HCHO columns suggest that the concentration of HCHO over NCP and Yangtze River Delta (YRD) is comparable to those over the tropics, suggesting that the anthropogenic emissions over NCP are the dominant source of HCHO (Souri et al., 2017a; Jin and Holloway, 2015). We do not see a significant deviation in the model from the observations over this region, indicating that no noticeable efforts to control VOC emissions in NCP and YRD have been made, which is very likely due to the fact that the recent regulations over China have overlooked cutting emissions from several industrial sectors (Liu et al., 2016) prior to 2016 (M. Li et al., 2019). For instance, Stavrakou et al. (2017) reported ∼6 % increases in anthropogenic VOC emissions over China from 2010 to 2014. The underestimation of the model with respect to OMPS lines up with results reported by Souri et al. (2017a) and Shen et al. (2019). We observe both underestimated and overestimated values in the simulated HCHO columns over areas in South Korea and Japan. The underestimation of HCHO in the model over regions with low VOCs (such as Mongolia and the Pacific Ocean) can be due to either missing sources or the incapability of the WRF-CMAQ to account for moist convective transport.
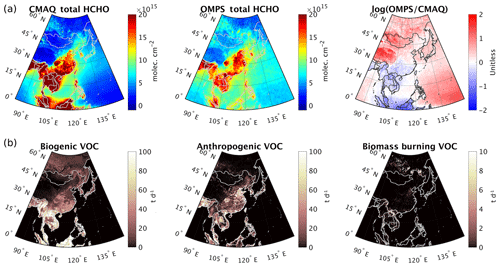
Figure 5(a) HCHO total columns from the WRF-CMAQ model, OMPS (using adjusted AMFs based on the shape factors derived from the model and bias-corrected following the method proposed in Zhu et al., 2020), and the natural logarithmic ratio of OMPS ∕ CMAQ during May–June 2016 at ∼13:30 LST. (b) The major sources of VOC emissions in the area including biogenic emissions simulated by MEGAN, anthropogenic emissions estimated by MIX-Asia (2010), and biomass burning emissions made by FINN. The emissions are the daily mean values based on the emissions in May–June. The VOC emissions only add up those compounds that are included in the CB05 mechanism.
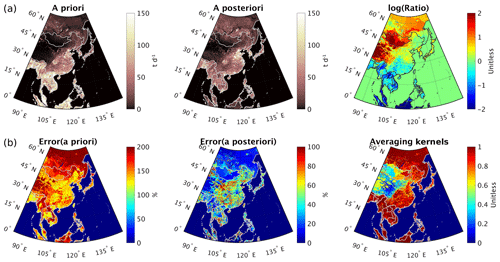
Figure 6(a) Total VOC emissions (i.e., the a priori) constrained by the satellite observations (i.e., the a posteriori) in May–June 2016 and the natural logarithmic ratio of the a posteriori to the a priori. (b) The errors in the a priori based on Table 3, the errors in the top-down estimation, and the averaging kernels (AKs) obtained from the estimation.
Figure 6 illustrates the total VOC emissions before and after the inversion along with their errors. Immediately apparent is the large reduction of VOC emissions in the tropics and subtropics due to the overestimation of isoprene from MEGAN v2.1. In contrast, enhancements of the emissions are evident at higher latitudes. We observe that the dominantly anthropogenic VOC emissions over NCP increase (∼25 %) after the adjustment. Despite the presence of vegetation over Japan and South Korea, we do not see that the emissions are severely overestimated. Hence, the overestimation of isoprene emissions is more pronounced in the tropics, possibly because of an overestimation in the emission factors used for specific plants. Nevertheless, a nontrivial oversight in models could be an insufficient representation of both HOx chemistry and dry deposition in forest canopies (Millet et al., 2008); as a result, the net amount of HCHO in the atmosphere over forest areas is higher than it should be if removal through either a chemical loss or faster dry deposition was considered.
Owing to the fact that we assume anthropogenic VOC emissions to be less uncertain relative to other sectors, the errors in the a priori are smaller in populated areas. We observe that OMPS HCHO columns are able to significantly reduce the uncertainty associated with the total VOC emissions over areas showing a strong HCHO signal (> 1016 molec. cm−2). Over clean areas, it is the other way around; we see less confidence in our top-down estimate (AK < 0.4) in areas such as Tibet and Mongolia.
We then compare the simulated HCHO column using two different emission inventories with those of OMPS in Fig. 7. We observe a substantial improvement in both the spatial structure and the magnitude of simulated HCHO columns using the a posteriori with respect to OMPS. The two-dimensional Pearson correlation increases from 0.78 to 0.91 after applying the adjustments to the emissions. In response to the increases in the total VOC emissions over the NCP, we observe ∼11 % enhancements in the simulated HCHO total columns. The updated emissions lead to a reduction in HCHO total columns as large as 70 % in the tropics.
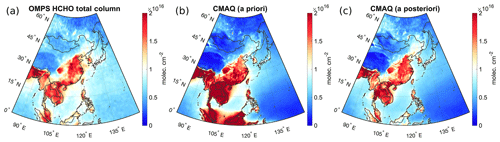
Figure 7(a–c) HCHO total columns from OMPS, the WRF-CMAQ simulated with the prior emissions, and the same model but with the top-down emissions constrained by the satellites in May–June 2016.
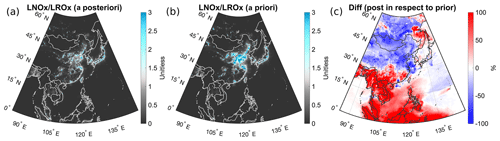
Figure 8(a–c) Ratio of LNOx∕LROx simulated by the posterior emissions, the prior, and their relative differences at 12:00–18:00 CST, averaged over May–June 2016 over the surface.
Validation of the model in terms of VOCs is not a straightforward task because the chemical mechanism used for our model has lumped several VOC species such as terminal or internal olefin or paraffin, only a handful of which were measured during the campaign. Also, the MIX-Asia inventory estimates the anthropogenic emissions for a selected number of VOCs in the CB05 mechanism. Here, we focus only on six compounds including isoprene, HCHO, ethene, ethane, acetaldehyde, and methanol, whose emissions are adjusted (with the same factor) based on satellite measurements. The comparison of the simulated values with the DC-8 measurements showed a noticeable mitigation in the discrepancy between two datasets at lower boundaries (< 900 hPa) in terms of isoprene (Fig. S5), ethane (Fig. S6), ethene (Fig. S7), and acetaldehyde (Fig. S8). Surprisingly, we observe a large underestimation of methanol over the Korean Peninsula by a factor of 10 (Fig. S9). The same tendency was observed in other regions in Wells et al. (2014) (see Fig. 8 in the paper). Our inversion obviously fails at mitigating the bias as there is not much direct constraint from the satellite observations on this compound. Wells et al. (2014) and Hu et al. (2011) demonstrated that methanol can be a secondary source of HCHO up to 10 %–20 % in midlatitudes in warm seasons. We tend to underestimate HCHO concentrations (by 15 %) in the lower atmosphere (< 900 hPa) after using the a posteriori over the Korean Peninsula (Fig. S10).
The results we have generated can be further exploited to elucidate changes in the ozone production rates, P(O3), due to having the constrained NOx and VOC emissions. We calculate P(O3) by subtracting the ozone loss driven by HOx (HO+HO2), reaction with several VOCs (i.e., alkenes and isoprene), the formation of HNO3, and O3 photolysis followed by the reaction of O(1D) with water vapor from the ozone formation via the removal of NO through HO2 or RO2:
Since P(O3) is a nonlinear function of NOx and VOC emissions, it is advantageous to look at the ratio of the chemical loss of NOx to that of ROx (RO2+HO2), a robust indicator pinpointing underlying drivers for the ROx cycle. LROx is defined through the sum of primarily radical–radical reactions:
LNOx mainly occurs via the NO2+OH reaction:
Typically, a value of LNOx∕LROx ∼2.7 defines the transition line between VOC-sensitive and NOx-sensitive regimes (Schroeder et al., 2017; Souri et al., 2020a).
Figure 8 depicts a contour map of LNOx∕ROx ratios over the surface before and after the inversion. As expected, the larger ratios are confined within major cities or industrial areas due to abundant NOx emissions. The hotspot of VOC-sensitive regimes is located in NCP and YRD. Also of interest in Fig. 8 is that advection renders a major fraction of the Yellow Sea (the sea connecting China to Korea) VOC-sensitive. Using the a posteriori leads to precipitous changes in the chemical regimes. As a result of a large reduction in the isoprene emissions in both the tropics and subtropics, we observe a shift toward VOC-limited, though the values of LNOx∕ROx are still too far from the transition line (i.e., ≪2.7). The substantial reduction in NOx emissions and an increase in VOC emissions over NCP and YRD go hand in hand for transitioning towards an NOx-sensitive regime. The ratios over South Korea and Japan are found to be variable and somehow in sync with the changes in NOx emissions.
The resultant changes in the LNOx∕LROx ratios shed some light on ozone sensitivity with respect to its major precursors, but P(O3) is also dependent on the absolute values of emissions, the degree of reactivity of VOCs, and the abundance of radicals. Here we use the integrated reaction rates (IRRs) to determine the most influential reactions pertaining to ozone loss and production at the surface. We focus on 12:00 to 18:00 China standard time (CST). Figure 9 shows the differences in the major pathways for the loss and formation of ozone at the surface within the time window. The differences are computed based on the subtraction of the simulation with the a posteriori from that with the a priori. In Fig. 9 we see a strong degree of correlation between changes in the magnitude of P(O3) through the HO2+NO reaction with those of NOx emissions (Fig. 3), whereas changes in the magnitude of P(O3) via the RO2+NO reaction are primarily on par with those of VOC emissions (Fig. 6). We observe P(O3) increases through HO2+NO and RO2+NO reactions in Japan, South Korea, Myanmar, and the Philippines because of increases in NOx emissions in NOx-sensitive regions. The simultaneous decrease in NOx and VOC in PRD and Taiwan causes the production of ozone via the same pathways to reduce it.
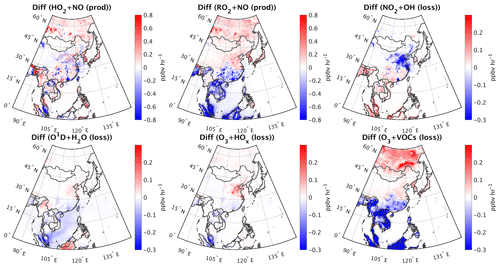
Figure 9Differences between the simulations with the updated emissions and the default ones of six major pathways of ozone production and loss over the surface. The time period is May–June 2016, 12:00–18:00 CST.
Normally, in VOC-rich environments, a reduction in VOC emissions boosts OH concentrations (Fig. S11). Consequently, we observe an enhancement of NO2+OH reaction in the tropics and subtopics. A substantial reduction in the chemical loss of ozone through NO2+OH over NCP and YRD arises from a considerable decrease in NOx emissions and an increase in OH (due to chemical feedback of NOx). In response to an increase in HOx concentrations over NCP (Figs. S11–S12), we observe an enhancement of ozone loss through O3+HOx. The ozone photolysis (O1D+H2O) is majorly driven by photolysis and water vapor mixing ratios, both of which are roughly constant in both simulations; accordingly, the difference map of O1D+H2O is mainly reflecting changes in ozone concentrations (shown later). Interestingly, we observe a large reduction in the loss of ozone through reaction with VOCs at lower latitudes. This is essentially because of the reduction in ISOP+O3, a VOC that prevails in those latitudes. Despite a much slower reaction rate for ISOP+O3 compared to ISOP+OH and ISOP + hv (Karl et al. 2004), this specific chemical pathway can be important as a way to oxidize isoprene and form HOx in forests (Paulson and Orlando, 1996).
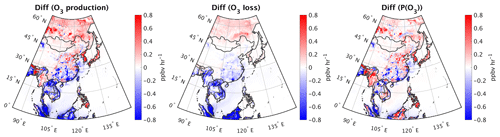
Figure 10Changes in the major chemical pathways of ozone production and loss over the surface, as well as the net ozone production P(O3) after updating the emissions. The time period is May–June 2016, 12:00-18:00 CST.
Figure 10 sums the differences of all mentioned chemical pathways involved in the formation and loss of surface ozone at 12:00–16:00 CST. Because of a complex nonlinear relationship between P(O3) and its precursors, we observe large variability in both the sign and the amplitude of P(O3). On average, changes in O3 production dominate over changes in O3 sinks except in Malaysia, which underwent a significant reduction in isoprene emissions, thus slowing down the ISOP + O3 reaction. In general, the differences in P(O3) follow the changes in the NOx emissions depending on which chemical regimes prevail.
Much of the above analysis is based on ozone production rates; however, various parameters encompassing dry deposition, vertical diffusion, and advection can also affect ozone concentrations. Therefore, we further compute the difference between the simulated maximum daily 8 h average (MDA8) surface ozone levels before and after the inversion depicted in Fig. 11. For comparison, we also over-plot the Chinese air quality monitoring network observations (https://quotsoft.net/air/, last access: 2 June 2020) to have a general grasp of the performance of the model before and after adjusting the emissions. We see a striking correlation between P(O3) (right panel in Fig. 10) and MDA8 surface ozone, indicating that the selected chemical pathways in this study can explain ozone changes. Nonetheless, the transport obviously plays a vital role in the spatial variability associated with the differences of surface ozone (e.g., Souri et al., 2016b). Figure 11 suggests a significant enhancement of ozone over NCP (∼4.56 ppbv, +5.6 %) and YRD (5.2 ppbv, +6.8 %) due to simultaneous decreases and increases in NOx∕VOCs, which is in agreement with K. Li et al. (2019). On the other hand, reductions in NOx mitigate ozone pollution in PRD (−5.4 %), Malaysia (−5.6 %), and Taiwan (−11.6 %). Table 6 lists the simulated MDA8 surface ozone levels for several regions before and after updating the emissions. Increases in MDA8 ozone over NCP and YRD overshadow decreases in southern China, resulting in a 1.1 % enhancement of ozone over China. This provides strong evidence that regulations on cutting VOC emissions should not be ignored. The largest reduction and increase in MDA8 ozone are found over Taiwan and YRD, respectively. Comparisons with surface observations show that the model generally captured the ozone spatial distributions; however, it tends to largely overpredict MDA8 surface ozone (∼7 ppbv). This tendency has been well-documented in other studies (e.g., Travis et al., 2016; Souri et al., 2017b; Lu et al., 2019). The updated simulation with the top-down emission partly reduces this overestimation in southern regions of China, while it further exacerbates the overestimation in the northern parts. No doubt much of this stems from the fact that the preexisting biases associated with the model (beyond emissions such as vertical mixing and cloud optical thickness) mask any potential improvement expected from the constrained emissions. Because of this, in addition to adjusting relevant emissions, a direct assimilation of ozone concentrations should complementarily be exploited (e.g., Miyazaki et al., 2019) to bolster the capability of the model to simulate ozone.
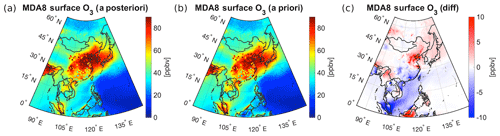
Figure 11Simulated MDA8 surface ozone using the updated emissions constrained by OMI and OMPS observations (a), the default ones (b), and their difference (c) in May–June 2016. We over-plot surface MDA8 ozone values (circles) from the Chinese air quality monitoring network (https://quotsoft.net/air/, last access: 2 June 2020).
In this paper we have focused on providing a top-down constraint on both volatile organic compound (VOC) and nitrogen oxide (NOx) emissions using a combination of the error-characterized Smithsonian Astrophysical Observatory (SAO) Ozone Mapping and Profile Suite Nadir Mapper (OMPS-NM) formaldehyde (HCHO) and National Aeronautics and Space Administration (NASA) Ozone Monitoring Instrument (OMI) nitrogen dioxide (NO2) retrievals during the Korean and United States (KORUS) campaign over East Asia in May–June 2016. Here, we include biogenic, biomass burning, and anthropogenic emissions from MEGAN, FINN, and the MIX-Asia 2010 inventory, respectively. A key point is that by considering the satellite observations together, we not only implicitly take the chemical feedback between HCHO–NOx and NO2–VOC into account by iteratively optimizing analytical nonlinear inversion, but we also quantify the impact of recent changes in emissions (since 2010) on surface ozone pollution.
Concerning total NOx emissions, the inversion estimate suggests a substantial reduction over China (−23 %), North China Plain (NCP) (−32 %), Pearl River Delta (PRD) (−36 %), Yangtze River Delta (YRD) (−41 %), Taiwan (−23 %), and Malaysia (−22 %) with respect to the values reported in the prior emissions mostly dictated by the MIX-Asia 2010 inventory. In essence these values reflect recent actions to lower emissions in those countries (Zhang et al., 2012; Trappey el al., 2012; Chua and Oh, 2011). The analytical inversion also paves the way for estimating the averaging kernels (AKs), thereby indicating the amount of information acquired from satellites for the top-down estimation. We observe AKs > 0.8 over major polluted areas, indicating that OMI is able to improve the emission estimates over medium- to high-emitting regions. Conversely, AKs are found to be small over pristine areas, suggesting that little information can be gained from the satellite over rural areas given retrieval errors. In line with the studies of Irie et al. (2016) and Souri et al. (2017a), we observe a growth in the total NOx emissions in Japan (+12 %) and South Korea (+9 %), which are partially explained by the new construction of thermal power plants in Japan and an upward trend in the number of diesel vehicles in South Korea.
MEGAN v2.1 estimates too much isoprene emission in the tropics and subtropics, a picture that emerges from the latitudinal dependence of the posterior VOC emissions on the prior ones. It is readily apparent from the top-down constrained VOC emissions that the prevailing anthropogenic VOC emissions in NCP are underestimated by 25 %, a direction that is in agreement with studies by Souri et al. (2017a) and Shen et al. (2019). We find that OMPS HCHO columns can greatly reduce the uncertainty associated with the total VOC emissions (AKs > 0.8) over regions having a moderate to strong signal (> 1016 molec. cm−2).
Large spatial variability associated with both NOx and VOC results in great oscillation in chemical condition regimes (i.e., NOx-sensitive or VOC-sensitive). Due to a considerable reduction and increase in NOx∕VOC emissions in NCP and YRD, respectively, we observe a large increase (53 %) in the ratio of the chemical loss of NOx (LNOx) to the chemical loss of ROx (RO2+HO2), shifting the regions towards NOx-sensitive. As a result, a substantial reduction in the afternoon NO2+OH reaction rate (a major loss of O3) and an increase in afternoon NO+HO2 and RO2+NO (a major production pathway for O3) are observed, leading to enhancements of the simulated maximum daily 8 h average (MDA8) surface ozone concentrations by ∼5 ppbv. Therefore, additional regulations on VOC emissions should be implemented to battle ozone pollution in those areas. On the other hand, being predominantly in NOx-sensitive regimes favors regions including Taiwan, Malaysia, and PRD to benefit from reductions in NOx, resulting in noticeable decreases in simulated MDA8 surface ozone levels. The comparison of simulated ozone before and after adjusting emissions and Chinese surface air quality observations reveals a large systematic positive bias (∼7 ppbv), which hinders attaining benefits from a more accurate ozone production rate due to the observationally constrained NOx∕VOC ratios. This highlights the need to explicitly deal with other underlying issues in the model (e.g., Travis et al., 2016) to be able to properly simulate surface ozone.
It has taken many years to develop satellite-based gas retrievals and weather and chemical transport models accurate enough to enable observationally based estimates of emissions with reasonable confidence and quantified uncertainty, as well as to produce credible top-down emission inventories over certain areas. However, it is essential to improve certain aspects to be able to narrow the range of uncertainty associated with the estimation, such as the following: (i) parameterizing the spatiotemporally varying bias of satellite gas retrievals; (ii) improving the knowledge of prior errors in bottom-up emissions; (iii) propagating model parameter errors such as PBL, radiation, and winds to the final output (e.g., Rodgers, 2000); and (iv) due to intertwined chemical feedback between various chemical compounds, inverse modeling needs to properly incorporate all available information (beyond HCHO and NO2) considering the cross-relationship either explicitly or implicitly. Despite these limitations, this research demonstrated that a joint inversion of NOx and VOC emissions using well-characterized observations significantly improved the simulation of HCHO and NO2 columns, permitting an observationally constrained quantification of the response of ozone production rates to emission changes.
The top-down emission inventories estimated from this study can be found at: https://doi.org/10.17632/8s4jscy93m.1 (Souri et al., 2020b).
The supplement related to this article is available online at: https://doi.org/10.5194/acp-20-9837-2020-supplement.
AHS designed the research, analyzed the data, conducted the inverse modeling and atmospheric modeling (for CMAQ, GEOS-Chem, WRF, and MEGAN), made all figures, and wrote the paper. CRN, GG, CECM, XL, and KC retrieved OMPS HCHO columns and conceived the study. LZ validated OMPS HCHO. DRB, AF, AJW, and AW measured different compounds during the campaign. JW and QZ provided the MIX-Asia inventory. All authors contributed to discussions and edited the paper.
The authors declare that they have no conflict of interest.
We are thankful for funding from the NASA Aura Science Team (no. NNX17AH47G), NASA Science of Terra, Aqua, and Suomi NPP (no. 80NSSC18K0691), NASA Making Earth System Data Records for Use in Research Environments (no. 80NSSC18M0091), and the NOAA AC4 program (no. NA18OAR4310108). We acknowledge the publicly available WRF, CMAQ, and GEOS-Chem models, as well as KORUS-AQ data that make this study possible. The simulations were run on the Smithsonian Institution High-Performance Cluster (SI/HPC) (https://doi.org/10.25572/SIHPC). Methanol and acetaldehyde measurements aboard the NASA DC-8 during KORUS-AQ were supported by the Austrian Federal Ministry for Transport, Innovation and Technology (bmvit) through the Austrian Space Applications Programme (ASAP) of the Austrian Research Promotion Agency (FFG). The PTR-MS instrument team (Philipp Eichler, Lisa Kaser, Tomas Mikoviny, Markus Müller) is acknowledged for providing the data used in this study.
This research has been supported by NASA (grant nos. NNX17AH47G, 80NSSC18K0691, and 80NSSC18M0091) and NOAA (grant no. NA18OAR4310108).
This paper was edited by Bryan N. Duncan and reviewed by two anonymous referees.
Bauwens, M., Stavrakou, T., Müller, J.-F., De Smedt, I., Van Roozendael, M., van der Werf, G. R., Wiedinmyer, C., Kaiser, J. W., Sindelarova, K., and Guenther, A.: Nine years of global hydrocarbon emissions based on source inversion of OMI formaldehyde observations, Atmos. Chem. Phys., 16, 10133–10158, https://doi.org/10.5194/acp-16-10133-2016, 2016.
Bey, I., Jacob, D. J., Yantosca, R. M., Logan, J. A., Field, B. D., Fiore, A. M., Li, Q., Liu, H. Y., Mickley, L. J., and Schultz, M. G.: Global modeling of tropospheric chemistry with assimilated meteorology: Model description and evaluation, J. Geophys. Res.-Atmos., 106, 23073–23095, https://doi.org/10.1029/2001JD000807, 2001.
Byun, D. and Schere, K. L.: Review of the Governing Equations, Computational Algorithms, and Other Components of the Models-3 Community Multiscale Air Quality (CMAQ) Modeling System, Appl. Mech. Rev, 59, 51–77, https://doi.org/10.1115/1.2128636, 2006.
Canty, T. P., Hembeck, L., Vinciguerra, T. P., Anderson, D. C., Goldberg, D. L., Carpenter, S. F., Allen, D. J., Loughner, C. P., Salawitch, R. J., and Dickerson, R. R.: Ozone and NOx chemistry in the eastern US: evaluation of CMAQ/CB05 with satellite (OMI) data, Atmos. Chem. Phys., 15, 10965–10982, https://doi.org/10.5194/acp-15-10965-2015, 2015.
Chai, T., Carmichael, G. R., Tang, Y., Sandu, A., Heckel, A., Richter, A., and Burrows, J. P.: Regional NOx emission inversion through a four-dimensional variational approach using SCIAMACHY tropospheric NO2 column observations, Atmos. Environ., 43, 5046–5055, https://doi.org/10.1016/j.atmosenv.2009.06.052, 2009.
Chance, K.: Analysis of BrO measurements from the Global Ozone Monitoring Experiment, Geophys. Res. Lett., 25, 3335–3338, https://doi.org/10.1029/98GL52359, 1998.
Choi, S., Lamsal, L. N., Follette-Cook, M., Joiner, J., Krotkov, N. A., Swartz, W. H., Pickering, K. E., Loughner, C. P., Appel, W., Pfister, G., Saide, P. E., Cohen, R. C., Weinheimer, A. J., and Herman, J. R.: Assessment of NO2 observations during DISCOVER-AQ and KORUS-AQ field campaigns, Atmos. Meas. Tech., 13, 2523–2546, https://doi.org/10.5194/amt-13-2523-2020, 2020.
Chua, S. C. and Oh, T. H.: Green progress and prospect in Malaysia, Renewable and Sustainable Energy Reviews, 15, 2850–2861, https://doi.org/10.1016/j.rser.2011.03.008, 2011.
Cohan, D. S., Hakami, A., Hu, Y., and Russell, A. G.: Nonlinear Response of Ozone to Emissions: Source Apportionment and Sensitivity Analysis, Environ. Sci. Technol., 39, 6739–6748, https://doi.org/10.1021/es048664m, 2005.
Cooper, M., Martin, R. V., Padmanabhan, A., and Henze, D. K.: Comparing mass balance and adjoint methods for inverse modeling of nitrogen dioxide columns for global nitrogen oxide emissions, J. Geophys. Res.-Atmos., 122, 4718–4734, https://doi.org/10.1002/2016JD025985, 2017.
Curci, G., Palmer, P. I., Kurosu, T. P., Chance, K., and Visconti, G.: Estimating European volatile organic compound emissions using satellite observations of formaldehyde from the Ozone Monitoring Instrument, Atmos. Chem. Phys., 10, 11501–11517, https://doi.org/10.5194/acp-10-11501-2010, 2010.
de Foy, B., Lu, Z., and Streets, D. G.: Satellite NO 2 retrievals suggest China has exceeded its NOx reduction goals from the twelfth Five-Year Plan, Sci. Rep.-UK, 6, 1–9, https://doi.org/10.1038/srep35912, 2016.
Dunker, A. M.: The decoupled direct method for calculating sensitivity coefficients in chemical kinetics, J. Chem. Phys., 81, 2385–2393, https://doi.org/10.1063/1.447938, 1984.
Flynn, L., Long, C., Wu, X., Evans, R., Beck, C. T., Petropavlovskikh, I., McConville, G., Yu, W., Zhang, Z., Niu, J., Beach, E., Hao, Y., Pan, C., Sen, B., Novicki, M., Zhou, S., and Seftor, C.: Performance of the Ozone Mapping and Profiler Suite (OMPS) products, J. Geophys. Res.-Atmos., 119, 6181–6195, https://doi.org/10.1002/2013JD020467, 2014.
González Abad, G., Liu, X., Chance, K., Wang, H., Kurosu, T. P., and Suleiman, R.: Updated Smithsonian Astrophysical Observatory Ozone Monitoring Instrument (SAO OMI) formaldehyde retrieval, Atmos. Meas. Tech., 8, 19–32, https://doi.org/10.5194/amt-8-19-2015, 2015.
González Abad, G., Vasilkov, A., Seftor, C., Liu, X., and Chance, K.: Smithsonian Astrophysical Observatory Ozone Mapping and Profiler Suite (SAO OMPS) formaldehyde retrieval, Atmos. Meas. Tech., 9, 2797–2812, https://doi.org/10.5194/amt-9-2797-2016, 2016.
Gu, D., Wang, Y., Smeltzer, C., and Liu, Z.: Reduction in NOx Emission Trends over China: Regional and Seasonal Variations, Environ. Sci. Technol., 47, 12912–12919, https://doi.org/10.1021/es401727e, 2013.
Guenther, A. B., Jiang, X., Heald, C. L., Sakulyanontvittaya, T., Duhl, T., Emmons, L. K., and Wang, X.: The Model of Emissions of Gases and Aerosols from Nature version 2.1 (MEGAN2.1): an extended and updated framework for modeling biogenic emissions, Geosci. Model Dev., 5, 1471–1492, https://doi.org/10.5194/gmd-5-1471-2012, 2012.
Hu, L., Millet, D. B., Mohr, M. J., Wells, K. C., Griffis, T. J., and Helmig, D.: Sources and seasonality of atmospheric methanol based on tall tower measurements in the US Upper Midwest, Atmos. Chem. Phys., 11, 11145–11156, https://doi.org/10.5194/acp-11-11145-2011, 2011.
Hudman, R. C., Russell, A. R., Valin, L. C., and Cohen, R. C.: Interannual variability in soil nitric oxide emissions over the United States as viewed from space, Atmos. Chem. Phys., 10, 9943–9952, https://doi.org/10.5194/acp-10-9943-2010, 2010.
Irie, H., Muto, T., Itahashi, S., Kurokawa, J., and Uno, I.: Turnaround of Tropospheric Nitrogen Dioxide Pollution Trends in China, Japan, and South Korea, Sola, 12, 170–174, https://doi.org/10.2151/sola.2016-035, 2016.
Jaeglé, L., Steinberger, L., Martin, R. V., and Chance, K.: Global partitioning of NOx sources using satellite observations: Relative roles of fossil fuel combustion, biomass burning and soil emissions, Faraday Discuss., 130, 407–423, https://doi.org/10.1039/B502128F, 2005.
Jin, X. and Holloway, T.: Spatial and temporal variability of ozone sensitivity over China observed from the Ozone Monitoring Instrument, J. Geophys. Res.-Atmos., 120, 7229–7246, https://doi.org/10.1002/2015JD023250, 2015.
Karl, M., Brauers, T., Dorn, H.-P., Holland, F., Komenda, M., Poppe, D., Rohrer, F., Rupp, L., Schaub, A., and Wahner, A.: Kinetic Study of the OH-isoprene and O3-isoprene reaction in the atmosphere simulation chamber, SAPHIR, Geophys. Res. Lett., 31, L05117, https://doi.org/10.1029/2003GL019189, 2004.
Krotkov, N. A., Lamsal, L. N., Celarier, E. A., Swartz, W. H., Marchenko, S. V., Bucsela, E. J., Chan, K. L., Wenig, M., and Zara, M.: The version 3 OMI NO2 standard product, Atmos. Meas. Tech., 10, 3133–3149, https://doi.org/10.5194/amt-10-3133-2017, 2017.
Krotkov, N. A., McLinden, C. A., Li, C., Lamsal, L. N., Celarier, E. A., Marchenko, S. V., Swartz, W. H., Bucsela, E. J., Joiner, J., Duncan, B. N., Boersma, K. F., Veefkind, J. P., Levelt, P. F., Fioletov, V. E., Dickerson, R. R., He, H., Lu, Z., and Streets, D. G.: Aura OMI observations of regional SO2 and NO2 pollution changes from 2005 to 2015, Atmos. Chem. Phys., 16, 4605–4629, https://doi.org/10.5194/acp-16-4605-2016, 2016.
Laughner, J. L., Zhu, Q., and Cohen, R. C.: The Berkeley High Resolution Tropospheric NO2 product, Earth Syst. Sci. Data, 10, 2069–2095, https://doi.org/10.5194/essd-10-2069-2018, 2018.
Levelt, P. F., Joiner, J., Tamminen, J., Veefkind, J. P., Bhartia, P. K., Stein Zweers, D. C., Duncan, B. N., Streets, D. G., Eskes, H., van der A, R., McLinden, C., Fioletov, V., Carn, S., de Laat, J., DeLand, M., Marchenko, S., McPeters, R., Ziemke, J., Fu, D., Liu, X., Pickering, K., Apituley, A., González Abad, G., Arola, A., Boersma, F., Chan Miller, C., Chance, K., de Graaf, M., Hakkarainen, J., Hassinen, S., Ialongo, I., Kleipool, Q., Krotkov, N., Li, C., Lamsal, L., Newman, P., Nowlan, C., Suleiman, R., Tilstra, L. G., Torres, O., Wang, H., and Wargan, K.: The Ozone Monitoring Instrument: overview of 14 years in space, Atmos. Chem. Phys., 18, 5699–5745, https://doi.org/10.5194/acp-18-5699-2018, 2018.
Li, C., Martin, R. V., Shephard, M. W., Cady-Pereira, K., Cooper, M. J., Kaiser, J., Lee, C. J., Zhang, L., and Henze, D. K.: Assessing the Iterative Finite Difference Mass Balance and 4D-Var Methods to Derive Ammonia Emissions Over North America Using Synthetic Observations, J. Geophys. Res.-Atmos., 124, 4222–4236, https://doi.org/10.1029/2018JD030183, 2019.
Li, K., Jacob, D. J., Liao, H., Shen, L., Zhang, Q., and Bates, K. H.: Anthropogenic drivers of 2013–2017 trends in summer surface ozone in China, P. Natl. Acad. Sci. USA, 116, 422–427, https://doi.org/10.1073/pnas.1812168116, 2019.
Li, M., Zhang, Q., Zheng, B., Tong, D., Lei, Y., Liu, F., Hong, C., Kang, S., Yan, L., Zhang, Y., Bo, Y., Su, H., Cheng, Y., and He, K.: Persistent growth of anthropogenic non-methane volatile organic compound (NMVOC) emissions in China during 1990–2017: drivers, speciation and ozone formation potential, Atmos. Chem. Phys., 19, 8897–8913, https://doi.org/10.5194/acp-19-8897-2019, 2019.
Lin, M., Horowitz, L. W., Payton, R., Fiore, A. M., and Tonnesen, G.: US surface ozone trends and extremes from 1980 to 2014: quantifying the roles of rising Asian emissions, domestic controls, wildfires, and climate, Atmos. Chem. Phys., 17, 2943–2970, https://doi.org/10.5194/acp-17-2943-2017, 2017.
Liu, F., Zhang, Q., van der A, R. J., Zheng, B., Tong, D., Yan, L., Zheng, Y., and He, K.: Recent reduction in NOx emissions over China: synthesis of satellite observations and emission inventories, Environ. Res. Lett., 11, 114002, https://doi.org/10.1088/1748-9326/11/11/114002, 2016.
Liu, X., Mizzi, A. P., Anderson, J. L., Fung, I. Y., and Cohen, R. C.: Assimilation of satellite NO2 observations at high spatial resolution using OSSEs, Atmos. Chem. Phys., 17, 7067–7081, https://doi.org/10.5194/acp-17-7067-2017, 2017.
Liu, Y., Shao, M., Lu, S., Chang, C.-C., Wang, J.-L., and Fu, L.: Source apportionment of ambient volatile organic compounds in the Pearl River Delta, China: Part II, Atmos. Environ., 42, 6261–6274, https://doi.org/10.1016/j.atmosenv.2008.02.027, 2008a.
Liu, Y., Shao, M., Fu, L., Lu, S., Zeng, L., and Tang, D.: Source profiles of volatile organic compounds (VOCs) measured in China: Part I, Atmos. Environ., 42, 6247–6260, https://doi.org/10.1016/j.atmosenv.2008.01.070, 2008b.
Logan, J. A.: Nitrogen oxides in the troposphere: Global and regional budgets, J. Geophys. Res.-Oceans, 88, 10785–10807, https://doi.org/10.1029/JC088iC15p10785, 1983.
Lu, X., Zhang, L., Chen, Y., Zhou, M., Zheng, B., Li, K., Liu, Y., Lin, J., Fu, T.-M., and Zhang, Q.: Exploring 2016–2017 surface ozone pollution over China: source contributions and meteorological influences, Atmos. Chem. Phys., 19, 8339–8361, https://doi.org/10.5194/acp-19-8339-2019, 2019.
Marais, E. A., Jacob, D. J., Kurosu, T. P., Chance, K., Murphy, J. G., Reeves, C., Mills, G., Casadio, S., Millet, D. B., Barkley, M. P., Paulot, F., and Mao, J.: Isoprene emissions in Africa inferred from OMI observations of formaldehyde columns, Atmos. Chem. Phys., 12, 6219–6235, https://doi.org/10.5194/acp-12-6219-2012, 2012.
Martin, R. V., Jacob, D. J., Chance, K., Kurosu, T. P., Palmer, P. I., and Evans, M. J.: Global inventory of nitrogen oxide emissions constrained by space-based observations of NO2 columns, J. Geophys. Res.-Atmos., 108, 4537, https://doi.org/10.1029/2003JD003453, 2003.
Millet, D. B., Jacob, D. J., Boersma, K. F., Fu, T.-M., Kurosu, T. P., Chance, K., Heald, C. L., and Guenther, A.: Spatial distribution of isoprene emissions from North America derived from formaldehyde column measurements by the OMI satellite sensor, J. Geophys. Res.-Atmos., 113, D02307, https://doi.org/10.1029/2007JD008950, 2008.
Miyazaki, K., Eskes, H., Sudo, K., Boersma, K. F., Bowman, K., and Kanaya, Y.: Decadal changes in global surface NOx emissions from multi-constituent satellite data assimilation, Atmos. Chem. Phys., 17, 807–837, https://doi.org/10.5194/acp-17-807-2017, 2017.
Miyazaki, K., Sekiya, T., Fu, D., Bowman, K. W., Kulawik, S. S., Sudo, K., Walker, T., Kanaya, Y., Takigawa, M., Ogochi, K., Eskes, H., Boersma, K. F., Thompson, A. M., Gaubert, B., Barre, J., and Emmons, L. K.: Balance of Emission and Dynamical Controls on Ozone During the Korea-United States Air Quality Campaign From Multiconstituent Satellite Data Assimilation, J. Geophys. Res.-Atmos., 124, 387–413, https://doi.org/10.1029/2018JD028912, 2019.
Palmer, P. I., Jacob, D. J., Fiore, A. M., Martin, R. V., Chance, K., and Kurosu, T. P.: Mapping isoprene emissions over North America using formaldehyde column observations from space, J. Geophys. Res.-Atmos., 108, 4180, https://doi.org/10.1029/2002JD002153, 2003.
Paulson, S. E. and Orlando, J. J.: The reactions of ozone with alkenes: An important source of HOx in the boundary layer, Geophys. Res. Lett., 23, 3727–3730, https://doi.org/10.1029/96GL03477, 1996.
Reuter, M., Buchwitz, M., Hilboll, A., Richter, A., Schneising, O., Hilker, M., Heymann, J., Bovensmann, H., and Burrows, J. P.: Decreasing emissions of NOx relative to CO2 in East Asia inferred from satellite observations, Nat. Geosci., 7, 792–795, https://doi.org/10.1038/ngeo2257, 2014.
Rodgers, C. D.: Inverse Methods for Atmospheric Sounding: Theory and Practice, WORLD SCIENTIFIC, Oxford, 2000.
Romer Present, P. S., Zare, A., and Cohen, R. C.: The changing role of organic nitrates in the removal and transport of NOx, Atmos. Chem. Phys., 20, 267–279, https://doi.org/10.5194/acp-20-267-2020, 2020.
Schroeder, J. R., Crawford, J. H., Fried, A., Walega, J., Weinheimer, A., Wisthaler, A., Müller, M., Mikoviny, T., Chen, G., Shook, M., Blake, D. R., and Tonnesen, G. S.: New insights into the column CH2O∕NO2 ratio as an indicator of near-surface ozone sensitivity, J. Geophys. Res.-Atmos., 122, 8885–8907, https://doi.org/10.1002/2017JD026781, 2017.
Shen, L., Jacob, D. J., Zhu, L., Zhang, Q., Zheng, B., Sulprizio, M. P., Li, K., Smedt, I. D., Abad, G. G., Cao, H., Fu, T.-M., and Liao, H.: The 2005–2016 Trends of Formaldehyde Columns Over China Observed by Satellites: Increasing Anthropogenic Emissions of Volatile Organic Compounds and Decreasing Agricultural Fire Emissions, Geophys. Res. Lett., 46, 4468–4475, https://doi.org/10.1029/2019GL082172, 2019.
Shim, C., Wang, Y., Choi, Y., Palmer, P. I., Abbot, D. S., and Chance, K.: Constraining global isoprene emissions with Global Ozone Monitoring Experiment (GOME) formaldehyde column measurements, J. Geophys. Res.-Atmos., 110, D24301, https://doi.org/10.1029/2004JD005629, 2005.
Skamarock, W. C. and Klemp, J. B.: A time-split nonhydrostatic atmospheric model for weather research and forecasting applications, J. Comput. Phys., 227, 3465–3485, https://doi.org/10.1016/j.jcp.2007.01.037, 2008.
Souri, A. H., Choi, Y., Jeon, W., Li, X., Pan, S., Diao, L., and Westenbarger, D. A.: Constraining NOx emissions using satellite NO2 measurements during 2013 DISCOVER-AQ Texas campaign, Atmos. Environ., 131, 371–381, https://doi.org/10.1016/j.atmosenv.2016.02.020, 2016a.
Souri, A. H., Choi, Y., Li, X., Kotsakis, A., and Jiang, X.: A 15-year climatology of wind pattern impacts on surface ozone in Houston, Texas, Atmos. Res., 174–175, 124–134, https://doi.org/10.1016/j.atmosres.2016.02.007, 2016b.
Souri, A. H., Choi, Y., Jeon, W., Woo, J.-H., Zhang, Q., and Kurokawa, J.: Remote sensing evidence of decadal changes in major tropospheric ozone precursors over East Asia, J. Geophys. Res.-Atmos., 122, 2474–2492, https://doi.org/10.1002/2016JD025663, 2017a.
Souri, A. H., Choi, Y., Jeon, W., Kochanski, A. K., Diao, L., Mandel, J., Bhave, P. V., and Pan, S.: Quantifying the Impact of Biomass Burning Emissions on Major Inorganic Aerosols and Their Precursors in the U.S., J. Geophys. Res.-Atmos., 122, 12020–12041, https://doi.org/10.1002/2017JD026788, 2017b.
Souri, A. H., Choi, Y., Pan, S., Curci, G., Nowlan, C. R., Janz, S. J., Kowalewski, M. G., Liu, J., Herman, J. R., and Weinheimer, A. J.: First Top-Down Estimates of Anthropogenic NOx Emissions Using High-Resolution Airborne Remote Sensing Observations, J. Geophys. Res.-Atmos., 123, 3269–3284, https://doi.org/10.1002/2017JD028009, 2018.
Souri, A. H., Nowlan, C. R., Wolfe, G. M., Lamsal, L. N., Chan Miller, C. E., Abad, G. G., Janz, S. J., Fried, A., Blake, D. R., Weinheimer, A. J., Diskin, G. S., Liu, X., and Chance, K.: Revisiting the effectiveness of HCHO∕NO2 ratios for inferring ozone sensitivity to its precursors using high resolution airborne remote sensing observations in a high ozone episode during the KORUS-AQ campaign, Atmos. Environ., 224, 117341, https://doi.org/10.1016/j.atmosenv.2020.117341, 2020a.
Souri, A. H., Nowlan, C. R., González Abad, G., Zhu, L., Blake, D. R., Fried, A., Weinheimer, A. J., Wisthaler, A., Woo, J.-H., Zhang, Q., Chan Miller, C. E., Liu, X. and Chance, K.: Data outputs from the joint inversion study, Mendeley Data, https://doi.org/10.17632/8s4jscy93m.1, 2020b.
Spurr, R. J. D.: VLIDORT: A linearized pseudo-spherical vector discrete ordinate radiative transfer code for forward model and retrieval studies in multilayer multiple scattering media, J. Quant. Spectrosc. Ra., 102, 316–342, https://doi.org/10.1016/j.jqsrt.2006.05.005, 2006.
Stavrakou, T., Müller, J.-F., De Smedt, I., Van Roozendael, M., van der Werf, G. R., Giglio, L., and Guenther, A.: Global emissions of non-methane hydrocarbons deduced from SCIAMACHY formaldehyde columns through 2003–2006, Atmos. Chem. Phys., 9, 3663–3679, https://doi.org/10.5194/acp-9-3663-2009, 2009.
Stavrakou, T., Guenther, A., Razavi, A., Clarisse, L., Clerbaux, C., Coheur, P.-F., Hurtmans, D., Karagulian, F., De Mazière, M., Vigouroux, C., Amelynck, C., Schoon, N., Laffineur, Q., Heinesch, B., Aubinet, M., Rinsland, C., and Müller, J.-F.: First space-based derivation of the global atmospheric methanol emission fluxes, Atmos. Chem. Phys., 11, 4873–4898, https://doi.org/10.5194/acp-11-4873-2011, 2011.
Stavrakou, T., Müller, J.-F., Bauwens, M., and De Smedt, I.: Sources and Long-Term Trends of Ozone Precursors to Asian Pollution, in Air Pollution in Eastern Asia: An Integrated Perspective, edited by: Bouarar, I., Wang, X., and Brasseur, G. P., Springer International Publishing, Cham, 167–189, 2017.
Trappey, A. J. C., Trappey, C., Hsiao, C. T., Ou, J. J. R., Li, S. J., and Chen, K. W. P.: An evaluation model for low carbon island policy: The case of Taiwan's green transportation policy, Energ. Policy, 45, 510–515, https://doi.org/10.1016/j.enpol.2012.02.063, 2012.
Travis, K. R., Jacob, D. J., Fisher, J. A., Kim, P. S., Marais, E. A., Zhu, L., Yu, K., Miller, C. C., Yantosca, R. M., Sulprizio, M. P., Thompson, A. M., Wennberg, P. O., Crounse, J. D., St. Clair, J. M., Cohen, R. C., Laughner, J. L., Dibb, J. E., Hall, S. R., Ullmann, K., Wolfe, G. M., Pollack, I. B., Peischl, J., Neuman, J. A., and Zhou, X.: Why do models overestimate surface ozone in the Southeast United States?, Atmos. Chem. Phys., 16, 13561–13577, https://doi.org/10.5194/acp-16-13561-2016, 2016.
Turner, A. J. and Jacob, D. J.: Balancing aggregation and smoothing errors in inverse models, Atmos. Chem. Phys., 15, 7039–7048, https://doi.org/10.5194/acp-15-7039-2015, 2015.
Valin, L. C., Fiore, A. M., Chance, K., and Abad, G. G.: The role of OH production in interpreting the variability of CH2O columns in the southeast U.S., J. Geophys. Res.-Atmos., 121, 478–493, https://doi.org/10.1002/2015JD024012, 2016.
Wells, K. C., Millet, D. B., Cady-Pereira, K. E., Shephard, M. W., Henze, D. K., Bousserez, N., Apel, E. C., de Gouw, J., Warneke, C., and Singh, H. B.: Quantifying global terrestrial methanol emissions using observations from the TES satellite sensor, Atmos. Chem. Phys., 14, 2555–2570, https://doi.org/10.5194/acp-14-2555-2014, 2014.
Wiedinmyer, C., Akagi, S. K., Yokelson, R. J., Emmons, L. K., Al-Saadi, J. A., Orlando, J. J., and Soja, A. J.: The Fire INventory from NCAR (FINN): a high resolution global model to estimate the emissions from open burning, Geosci. Model Dev., 4, 625–641, https://doi.org/10.5194/gmd-4-625-2011, 2011.
Wolfe, G. M., Kaiser, J., Hanisco, T. F., Keutsch, F. N., de Gouw, J. A., Gilman, J. B., Graus, M., Hatch, C. D., Holloway, J., Horowitz, L. W., Lee, B. H., Lerner, B. M., Lopez-Hilifiker, F., Mao, J., Marvin, M. R., Peischl, J., Pollack, I. B., Roberts, J. M., Ryerson, T. B., Thornton, J. A., Veres, P. R., and Warneke, C.: Formaldehyde production from isoprene oxidation across NOx regimes, Atmos. Chem. Phys., 16, 2597–2610, https://doi.org/10.5194/acp-16-2597-2016, 2016.
Zhang, Q., He, K., and Huo, H.: Cleaning China's air, Nature, 484, 161–162, https://doi.org/10.1038/484161a, 2012.
Zhong, L., Louie, P. K. K., Zheng, J., Yuan, Z., Yue, D., Ho, J. W. K., and Lau, A. K. H.: Science–policy interplay: Air quality management in the Pearl River Delta region and Hong Kong, Atmos. Environ., 76, 3–10, https://doi.org/10.1016/j.atmosenv.2013.03.012, 2013.
Zhu, L., Jacob, D. J., Kim, P. S., Fisher, J. A., Yu, K., Travis, K. R., Mickley, L. J., Yantosca, R. M., Sulprizio, M. P., De Smedt, I., González Abad, G., Chance, K., Li, C., Ferrare, R., Fried, A., Hair, J. W., Hanisco, T. F., Richter, D., Jo Scarino, A., Walega, J., Weibring, P., and Wolfe, G. M.: Observing atmospheric formaldehyde (HCHO) from space: validation and intercomparison of six retrievals from four satellites (OMI, GOME2A, GOME2B, OMPS) with SEAC4RS aircraft observations over the southeast US, Atmos. Chem. Phys., 16, 13477–13490, https://doi.org/10.5194/acp-16-13477-2016, 2016.
Zhu, L., González Abad, G., Nowlan, C. R., Chan Miller, C., Chance, K., Apel, E. C., DiGangi, J. P., Fried, A., Hanisco, T. F., Hornbrook, R. S., Hu, L., Kaiser, J., Keutsch, F. N., Permar, W., St. Clair, J. M., and Wolfe, G. M.: Validation of satellite formaldehyde (HCHO) retrievals using observations from 12 aircraft campaigns, Atmos. Chem. Phys. Discuss., https://doi.org/10.5194/acp-2019-1117, in review, 2020.
- Abstract
- Introduction
- Measurements, modeling, and method
- Validation of the model in terms of meteorology
- Comparison to satellites and providing top-down emissions
- Implications for surface ozone
- Summary
- Data availability
- Author contributions
- Competing interests
- Acknowledgements
- Financial support
- Review statement
- References
- Supplement
- Abstract
- Introduction
- Measurements, modeling, and method
- Validation of the model in terms of meteorology
- Comparison to satellites and providing top-down emissions
- Implications for surface ozone
- Summary
- Data availability
- Author contributions
- Competing interests
- Acknowledgements
- Financial support
- Review statement
- References
- Supplement