the Creative Commons Attribution 4.0 License.
the Creative Commons Attribution 4.0 License.
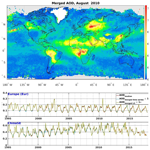
Merging regional and global aerosol optical depth records from major available satellite products
Larisa Sogacheva
Thomas Popp
Andrew M. Sayer
Oleg Dubovik
Michael J. Garay
Andreas Heckel
N. Christina Hsu
Hiren Jethva
Ralph A. Kahn
Pekka Kolmonen
Miriam Kosmale
Gerrit de Leeuw
Robert C. Levy
Pavel Litvinov
Alexei Lyapustin
Peter North
Omar Torres
Antti Arola
Satellite instruments provide a vantage point for studying aerosol loading consistently over different regions of the world. However, the typical lifetime of a single satellite platform is on the order of 5–15 years; thus, for climate studies, the use of multiple satellite sensors should be considered. Discrepancies exist between aerosol optical depth (AOD) products due to differences in their information content, spatial and temporal sampling, calibration, cloud masking, and algorithmic assumptions. Users of satellite-based AOD time-series are confronted with the challenge of choosing an appropriate dataset for the intended application. In this study, 16 monthly AOD products obtained from different satellite sensors and with different algorithms were inter-compared and evaluated against Aerosol Robotic Network (AERONET) monthly AOD. Global and regional analyses indicate that products tend to agree qualitatively on the annual, seasonal and monthly timescales but may be offset in magnitude. Several approaches were then investigated to merge the AOD records from different satellites and create an optimised AOD dataset. With few exceptions, all merging approaches lead to similar results, indicating the robustness and stability of the merged AOD products. We introduce a gridded monthly AOD merged product for the period 1995–2017. We show that the quality of the merged product is as least as good as that of individual products. Optimal agreement of the AOD merged product with AERONET further demonstrates the advantage of merging multiple products. This merged dataset provides a long-term perspective on AOD changes over different regions of the world, and users are encouraged to use this dataset.
- Article
(18918 KB) - Full-text XML
-
Supplement
(15233 KB) - BibTeX
- EndNote
Interactions of atmospheric aerosols with clouds and radiation are the largest source of uncertainty in modelling efforts to quantify current climate and predict climate change (IPCC, 2018). To reduce such uncertainties, we need observations to constrain climate models. However, these observations must be accurately calibrated and validated, have consistent or at least well-characterised uncertainties, and provide adequate temporal and spatial sampling over a long period of time. With their ability to cover the globe systematically, satellites provide this global and temporal perspective. Satellite observations have produced major advances in our understanding of the climate system and its changes, including quantifying the spatio-temporal states of the atmosphere, land and oceans, and aspects of the underlying processes. However, as the typical lifetime of a single satellite platform is on the order of 5–15 years, a single sensor data record may not be long enough to discern a climate signal (WMO, 2017). Moreover, aerosol products from different satellites and algorithms all have limitations regarding their spatial and temporal coverage and vary in their accuracies depending on environmental conditions (aerosol loading and type, surface brightness, and observation geometry), often leading to regional differences (e.g. Li et al., 2014b). Thus, the application of satellite observations for climate change studies requires using products from multiple sources to derive consistent regional conclusions.
The key parameter used for aerosol-related studies to date is the aerosol optical depth (AOD), which is the vertical integral of extinction by aerosol particles through the atmospheric column. Over the last several decades, AOD remote sensing has been performed from space using a wide variety of sensors that have different characteristics, including being passive or active, operating in ultraviolet (UV) to thermal infrared (TIR) spectral regions, being single-view to multi-view, being single-pixel to broad swath, having a sub-kilometre to tens-of-kilometres resolution, being intensity-only or polarimetric, and having different orbits and observation time(s). Table 1 lists the datasets used in the current study, together with key references. Aside from the Earth Polychromatic Imaging Camera (EPIC; orbiting at L1 Lagrange point directly between the Earth and the sun on the Deep Space Climate Observatory (DSCOVR) satellite), all sensors are in polar-orbiting, sun-synchronous low-earth orbits (∼600–800 km). Only a few of these sensors were optimised for accurate aerosol property retrieval, and for many, AOD at one or more visible wavelengths is the only quantitatively reliable aerosol parameter they provide. Table 1 is not exhaustive for available AOD products. Other AOD products such as those from active sensors such as the Cloud-Aerosol Lidar with Orthogonal Polarization (CALIOP) and imaging radiometers on geostationary satellites are not considered here, as they have very different sampling characteristics (e.g. CALIOP profiles a curtain swath, with areas either viewed twice daily and twice during the night during a month or not at all; geostationary sensors sample a constant disc, typical at a frequency of 10 min to 1 h); thus their monthly mean products are conceptually very different from polar-orbiters.
No two datasets provide identical results, whether applying the same algorithm principles to multiple similar sensors (Sayer et al., 2017, 2019; S. Li et al., 2016; Levy et al., 2013) or even between “identical” sensors, such as the Moderate Resolution Imaging Spectroradiometers (MODISs) on Terra and Aqua (Sayer et al., 2015; Levy et al., 2018) for which calibration and time of day differences remain. Using different retrieval algorithms for products retrieved from the same instruments introduces additional discrepancies, such as those found by de Leeuw et al. (2015), Popp et al. (2016) for three Along Track Scanning Radiometer (ATSR) datasets.
Differences can become larger when comparing products from different sensors and algorithms (Kokhanovsky and de Leeuw, 2009; Kinne, 2009; Li et al., 2014b). One other important factor contributing to differences is related to the approach to cloud masking, which affects the pixels selected for processing by retrieval algorithms and propagates into different levels of clear-sky bias in daily and monthly aggregates (Sogacheva et al., 2017; Zhao et al., 2013; Li et al., 2009). Escribano et al. (2017) estimated the impact of choosing different AOD products for a dust emission inversion scheme and concluded that the large spread in aerosol emission flux over the Sahara and Arabian Peninsula is likely associated with differences between satellite datasets. Similarly, Li et al. (2009) concluded that differences in cloud-masking alone could account for most differences among multiple satellite AOD datasets, including several for which different algorithms were applied to data from the same instrument.
There is no single “best” AOD satellite product globally. For example, the MODIS Deep Blue (DB) AOD product shows better performance than MODIS Dark Target (DT) in most regions, besides bright surfaces (i.e. deserts and arid/semi-arid areas) (Wei et al., 2019a). However, despite the differences between satellite products and the fact that none is uniformly most accurate (Sayer et al., 2014; de Leeuw et al., 2015, 2018), the application of statistical techniques such as principal component or maximum covariance analysis (Li et al., 2013, 2014a, b) shows that there are key similarities among the AOD products tested.
Merging multi-sensor AOD products holds the potential to produce a more spatially and temporally complete and accurate AOD picture. With multiple observational datasets available, it is important to examine their consistency in representing aerosol property variability in these dimensions. This is useful for constraining aerosol parameterisations in climate models (Liu et al., 2006), in the study of aerosol climate effects (Chylek et al., 2003; Bellouin et al., 2005) and for verifying global climate models (e.g. Kinne et al., 2003, 2006; Ban-Weiss et al., 2014) in which satellite-retrieved AOD monthly aggregates are used.
However, such an integration into a coherent and consistent climatology is a difficult task (Mishchenko et al., 2007; Li et al., 2009). There are only a few studies where an AOD record was merged from different satellites. Chatterjee et al. (2010) describe a geostatistical data fusion technique that can take advantage of the spatial autocorrelation of AOD distributions retrieved from the Multi-angle Imaging Spectroradiometer (MISR) and MODIS, while making optimal use of all available datasets. Tang et al. (2016) performed a spatio-temporal fusion of satellite AOD products from MODIS and Sea-Viewing Wide Field-of-View Sensor (SeaWiFS) using a Bayesian maximum entropy method for eastern Asia and showed that, in the regions where both MODIS and SeaWiFS have valid observations, the accuracy of the merged AOD is higher than those of the MODIS and SeaWiFS AODs individually. Han et al. (2017) improved the AOD retrieval accuracy by fusing MODIS and CALIOP data. Sogacheva et al. (2018b) combined ATSR and MODIS AOD to study the trends in AOD over China between 1995 and 2017. Naeger et al. (2016) combined daily AOD products from polar-orbiting and geostationary satellites to generate a near-real-time (NRT) daily AOD composite product for a case study of trans-Pacific transport of Asian pollution and dust aerosols in mid-March 2014. J. Li et al. (2016) constructed a monthly mean AOD ensemble by combining monthly AOD anomaly time series from MODIS, MISR, SeaWiFS, Ozone Monitoring Instrument (OMI) and POLarization and Directionality of the Earth's Reflectances (POLDER) and applying an ensemble Kalman filter technique to these multi-sensor and ground-based aerosol observations to reduce uncertainties. Penning de Vries et al. (2015) examined relationships between the monthly mean AOD, Ångström exponent (AE) from MODIS, UV Aerosol Index from the Global Ozone Monitoring Experiment–2 (GOME-2) and trace gas column densities and showed the advantage of using multiple datasets with respect to characterising aerosol type. Boys et al. (2014) combined SeaWiFS and MISR AODs with the GEOS-Chem global model to create and study trends in a 15-year time series of surface particulate matter levels.
A meaningful merge should account for the strengths and limitations of each constituent record. The spread of satellite AOD records also adds to the value of constraining their uncertainty; whereas a lack of diversity among datasets does not mean that they have converged on the true value, the existence of unexplained diversity does imply that they have not.
To assess their consistency, the products should be compared during overlapping periods, because interannual and shorter-term variability in atmospheric aerosols can be significant in some parts of the world (e.g. Lee et al., 2018). In the current study, AOD monthly aggregates from 16 different satellite products were evaluated with ground-based measurements from the Aerosol Robotic Network (AERONET; Holben et al., 1998). Note that, as with all measurements, even the AERONET spectral AOD has limitations as to where it can be informative. For example, AERONET includes ∼450 active stations in 2019, offering far more spatial coverage than in 1993 when the network was founded, yet even now AERONET spatial sampling is particularly limited in remote areas which are often those where aerosol gradients are large, e.g. near sources (e.g. Shi et al., 2011; J. Li et al., 2016).
Based on the comparison with AERONET, we estimate how well the satellite AOD monthly aggregates reproduce the AERONET AOD climatology. We considered areas with different aerosol types, aerosol loading and surface types, which are the dominant factors affecting AOD product quality. This allows users to choose the AOD product of a better quality, depending on the area and research objective. A verification of open-ocean monthly data using the Maritime Aerosol Network (MAN; Smirnov et al., 2009) is not possible in this way, because MAN data are acquired during cruises on ships of opportunity rather than as regular, repeating observations at specific locations.
Different approaches for merging the AOD products (median, weighted according to the evaluation results) are introduced in the current paper. AOD evaluation results are used to merge the L3 gridded monthly AOD data and AOD time series for the period 1995–2017, using different methodologies. The resulting AOD merged products are evaluated against AERONET and compared against one another.
This study grew out of discussions at annual AeroSat (https://aerosat.org, last access: 9 May 2019) meetings about how to move forward on the difficult topic of combining distinct aerosol data records. AeroSat is a grass-roots group of several dozen algorithm developer teams and data users. Meeting in person around once a year in concert with its sibling AeroCom group of aerosol modellers (https://aerocom.mpimet.mpg.de, last access: 9 May 2019) allows an active discussion between data providers and data users to highlight developments, discuss current issues and open questions in the field of satellite aerosol remote sensing and aerosol modelling.
The paper is organised as follows. In Sect. 2, the AOD products and regions of interest are introduced. The main principles and results for the statistical evaluation of individual monthly AOD retrievals are presented in Sect. 3. Alternative methods for merging are discussed in Sect. 4. AOD merged products are introduced, evaluated and inter-compared with individual products in Sect. 5. Annual, seasonal and monthly regional AOD time series are presented and discussed in Sect. 6. A brief summary and conclusion are given in the final section.
2.1 Regions of interest
There are huge regional differences in AOD loading types (composition and optical properties), seasonality and surface reflectance (Holben et al., 2001; Dubovik et al., 2002; Pinty et al., 2011). Retrieval quality (accuracy, precision and coverage) varies considerably as a function of these conditions, as well as whether a retrieval is over land or ocean. Therefore, this study focuses on surface-specific (land or ocean) and regional evaluation of these diverse aerosol products.
In addition to evaluating AOD products AOD over land, over ocean and globally (note that not all sensor–algorithm combinations retrieve over both surfaces), we chose 15 regions that seem likely to represent a sufficient variety of aerosol and surface conditions (Fig. 1 and Table S1 in the Supplement). These include 11 land regions, two ocean regions and one heavily mixed region. The land regions represent Europe (denoted by Eur), Boreal (Bor), northern, eastern and western Asia (AsN, AsE and AsW, respectively), Australia (Aus), northern and southern Africa (AfN and AfS), South America (AmS), and eastern and western Northern America (NAE and NAW). The Atlantic Ocean is represented as two ocean regions, one characterised by Saharan dust outflow over the central Atlantic (AOd) and a second that includes burning outflow over the southern Atlantic (AOb). The mixed region over Indonesia (Ind) includes both land and ocean. Due to documented large changes in AOD during the last 25 years (Sogacheva et al., 2018a, b), we also considered the south-eastern China (ChinaSE) subset of the AsE region.
The main body of the paper focuses on two regions, Europe and ChinaSE, and the big-picture results (global, all land and all ocean). The two regions, Europe and ChinaSE, were chosen because they are often the focus of aerosol studies. Results from the remaining regions are presented in the Supplement.
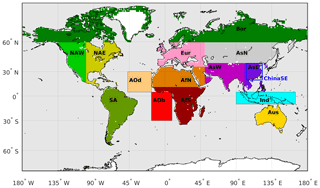
Figure 1Fifteen land and ocean regions defined in this study: Europe (Eur), Boreal (Bor), northern Asia (AsN), eastern Asia (AsE), western Asia (AsW), Australia (Aus), northern Africa (AfN), southern Africa (AfS), South America (SA), eastern North America (NAE), western North America (NAW), Indonesia (Ind), Atlantic Ocean dust outflow (AOd) and Atlantic Ocean biomass burning outflow (AOb). In addition, south-eastern China (ChinaSE), which is part of the AsE region, marked with a blue frame, is considered separately. Land, ocean and global AOD were also considered.
2.2 Instruments, algorithms and AOD products
An overview of the instruments and AOD products included in this study is presented in Table 1. AOD products from the same instruments retrieved with different algorithms are named in the paper with the instrument and retrieval algorithms, e.g. ATSR dual-view (ADV), ATSR Swansea University (SU), Terra Dark Target (DT) & Deep Blue (DB) and Terra MAIAC (multi-angle implementation of atmospheric correction). When both Terra and Aqua are considered, we call them together as MODIS DT&DB or MODIS MAIAC. Note that we used the merged MODIS Dark Target and Deep Blue product (Sayer et al., 2014; denoted “DT&DB”), rather than the results of the individual DB and DT algorithms, as this merged dataset was introduced into the product for similar purposes as the one explored in this work. An ensemble ATSR product (ATSR_ens) was generated from the three ATSR products (ATSR ADV, ATSR SU and ATSR ORAC – ATSR with the optimal retrieval of aerosol and cloud algorithm) in order to combine the strengths of several algorithms and to increase the coverage of the combined product (Kosmale et al., 2020). The ensemble was calculated per pixel as the weighted mean of the individual algorithm values with weights given by the inverse of the individual pixel level uncertainty values. The ensemble algorithm required as a minimum for each pixel to have valid results from at least two of the contributing algorithms. The uncertainties in each algorithm were first corrected in their absolute values to agree on average with the mean error.
For some products, AOD data are available for wavelengths other than 0.55 µm. Specifically, Total Ozone Mapping Spectrometer (TOMS) and OMI products include AOD at 0.50 µm, Advanced Very-High-Resolution Radiometer (AVHRR) NOAA includes AOD at approximately 0.63 µm (with slight variation between the different AVHRR sensors), and EPIC AOD is available at 0.44 µm (in the dataset used in the current study). If the wavelength is not mentioned specifically, 0.55 µm is implicit.
In most cases the official AOD monthly products (typically referred to as Level 3 or L3 data), which correspond to arithmetic means of daily mean data aggregated onto (typically) a 1∘ × 1∘ grid, have been used without further processing. The first exceptions are for AVHRR NOAA and POLDER, which provide very high AOD values poleward of ca. 60∘ and over Hudson Bay (50–70∘ N, 70–95∘ E), respectively. The values are unrealistic, a likely a consequence of cloud and/or sea ice contamination. To eliminate those unrealistic values, AOD values of >0.7 have been removed over the mentioned-above areas. Applying that limit decreased the offset between the AVHRR NOAA product and other products but did not eliminate it (see Sect. S2 in the Supplement for details). Additionally, MISR standard (0.5∘ × 0.5∘ resolution) and AVHRR NOAA (0.1∘ × 0.1∘ resolution) L3 AOD products were aggregated by simple averaging to 1∘ to match the other datasets.
Due to differences in instrument capabilities and swath widths (Table 1), the spatial and temporal data sampling available for calculating monthly averages varies considerably among the satellite products. The ATSR products and MISR have narrow swaths and generally provide only a few days with retrievals per month, whereas most of the rest see the whole planet roughly every day or two so that their coverage is mostly limited by, e.g. the persistence of cloud cover. As mentioned previously, EPIC is a special case, as it provides moving snapshots of the day-lit portion of the Earth, up to several times per day, as distinct from overpasses at only specific local solar equatorial crossing times for the sensors on polar-orbiting satellites. Further, TOMS and OMI have a notably coarser pixel resolution than the others, so their coverage and quality are more sensitive to cloud masking decisions. Some datasets provide measures of internal diversity (e.g. standard deviation), but none currently provides estimates of the monthly aggregate uncertainty against some standard, which would be a combination of (both systematic and random) retrieval uncertainties and sampling limitations. This is an area currently being investigated by AeroSat due to the wide use of L3 products.
For the intercomparison between AOD products, we chose three “reference” years:
-
2000, when the AOD products from TOMS, AVHRR NOAA, SeaWiFS, ATSR-2, MODIS Terra and MISR are available (for the full year, except for MISR and MODIS Terra, which were available from March to December);
-
2008, when the AOD products from Advanced ATSR (AATSR), MODIS Terra and Aqua, MISR, AVHRR NOAA, AVHRR DB/SOAR (Satellite Ocean Aerosol Retrieval), SeaWiFS and POLDER are available; and
-
2017, when the AOD products from MODIS Terra and Aqua, MISR, VIIRS (Visible Infrared Imaging Radiometer Suite) and EPIC are available.
For products with no coverage over ocean (TOMS, OMI and MAIAC products) or land (AVHRR NOAA), global AOD was not considered.
The AOD deviations of the individual products from the median AOD (Figs. S1 and S2 in the Supplement) are discussed in detail in the Supplement (Sect. S2). These show regional differences, even for products retrieved from the same instruments with similar algorithm. Both negative and positive deviations are observed in regions with high AOD; both aerosol optical model assumptions and surface type are also likely to influence the AOD retrieval. High AOD might, in turn, be wrongly screened as cloud, and thus the resulting lack of high AOD retrieval leads to a low bias in monthly AOD. To further reveal differences among the AOD products retrieved with different algorithms and applied to different satellites, the diversity of the satellite annual mean AOD for years 2000, 2008 and 2017 is discussed in Sect. S3 (Figs. S3 and S4). The diversity is lower in 2017, when only MODIS, MISR, EPIC and VIIRS AOD products are available.
3.1 Evaluation of monthly AOD
To evaluate the quality of any AOD product, the verification of the product against more accurate reference measurements, where possible, is obligatory. Ground-based measurements such as those from AERONET (cloud screened and quality assured Version 3 Level 2.0; Giles et al., 2019) provide highly accurate measures of AOD that are widely used as ground truth for the validation of satellite AOD data. Extensive L2 AOD validation has been performed for different aerosol products.
However, climate model evaluation is often performed on monthly scales. Thus, climate analysis begs for evaluation of satellite AOD monthly aggregates (Nabat et al., 2013; Michou et al., 2015; S. Li et al., 2016). Only a few attempts have been made to evaluate AOD monthly aggregates retrieved from satellites (e.g. Li et al., 2014b, Wei et al., 2019b). This is because verification of the L3 monthly aggregate satellite AOD is not a true validation (and note the use of “evaluation” and “verification” here instead of “validation”). AERONET provides AOD at a single point and is not necessarily representative of AOD in a 1∘ × 1∘ grid. While AERONET samples during all cloud-free daylight hours, a given polar-orbiting sensor will only report once per day and at the same time each day (e.g. 13:30 LT for sensors in the A-Train). The possible spatial representativity issues associated with this latter point are a topic of current investigation (e.g. J. Li et al., 2016; Virtanen et al., 2018; Schutgens, 2019). Nevertheless, AERONET's instantaneous AOD uncertainty (around 0.01 in the mid-visible; Eck et al., 1999) is significantly lower than most satellite products, and its temporal sampling is much more complete. As such, it remains a useful source for evaluating these L3 products, and for this purpose we compare AOD monthly aggregates of all available data from both AERONET and each satellite product. Deviations between satellite and AERONET monthly aggregates are expected, e.g. due to differences in satellite spatial and temporal sampling (Sect. 2.2, Table 1), particularly for those satellites with lower coverage.
Results from this comparison have limitations. As mentioned previously, AERONET provides data over certain locations within a grid cell, whereas satellites cover a larger fraction of the area of a grid cell (depending on sampling and cloud cover). So, for example, if AERONET is likely to miss extreme high values (localised plumes missing an AERONET station), that will result in AERONET showing lower AOD than from a satellite. Conversely, if a station happens to be directly under an aerosol plume and the satellite algorithm filters as a cloud, the AERONET value would be higher.
Neither AERONET nor satellite monthly AOD aggregates are true monthly AOD values. When we refer to “AOD monthly aggregate” we mean the daytime, cloud-free AOD monthly aggregated from whatever data are available. How the aggregate is calculated is also important; AOD distributions on monthly scales are often closer to lognormal than normal, which suggests that the arithmetic monthly mean may not be the most appropriate summary metric (O'Neill et al., 2000; Sayer and Knobelspiesse, 2019). The discrepancies between different statistics can be exacerbated when a dataset provides poor sampling of the extreme conditions. Nevertheless, as it is the most widely used statistic within the community and is the standard output of current L3 products, monthly means are presented in this analysis. The general framework could be applied to other AOD summary statistics (e.g. monthly median or geometric mean, advocated by Sayer and Knobelspiesse, 2019) if these L3 outputs become more widely available in the future.
In the evaluation exercise, AERONET monthly mean AOD and AE (which describes how AOD depends on wavelength and is sometimes used as a proxy for aerosol type) were calculated from AERONET daily means. AOD verification was performed for all available AERONET monthly data and separately for different aerosol types, which were defined with AOD and AE thresholds. Although these thresholds are subjective, we consider “background aerosol” to be cases where AOD<0.2, “fine-dominated” to be where AOD>0.2 and AE>1, and “coarse-dominated” to be cases where AOD>0.2 and AE<1 (e.g. Eck et al., 1999). This classification has also been used by e.g. Sayer et al. (2018b) and Sogacheva et al. (2018a, b). The annual and seasonal maps of prevailing aerosol type for AERONET locations, calculated from the AERONET data available for the period of 1995–2017, are shown in Fig. S5. Such a classification differentiates major aerosol scenarios. The biomass burning seasons over the Amazon and South Africa are clearly identified by a domination of the fine aerosol particles in JJA (June, July, August) and SON (September, October, November), and the Asian dust transport season in MAM (March, April, May) is clearly coarse dominated. As the deviation of each satellite product from the median has regional components (Figs. S1 and S2). Even though we tried to choose regions with (somewhat) homogeneous aerosol conditions during a given season, AOD conditions (and thus algorithm performance) might vary within the regional AERONET stations, which may represent different aerosol/surface conditions within one study regions, may have different record lengths. To keep similar weighting for each station in a region, we first calculated statistics for each AERONET station separately and then calculated the regional median validation statistics from all available stations.
To reveal how retrieval quality depends on AOD loading, offsets between AERONET AOD and satellite product AOD were estimated for binned AERONET AOD, and the number of observations in each AOD bin is reported. Correlation coefficient (R, Pearson correlation), offset (satellite product−AERONET), root-mean square error (RMSE) and fraction of points that fulfil the Global Climate Observing System (GCOS) uncertainty goals (GE) of the larger of 0.03 or 10 % of AOD (GCOS, 2011) are also reported.
These monthly AOD verification results are used to calculate weights for each satellite dataset in one of the merging approaches later in Sect. 4.2.
3.1.1 Binned offset global evaluation
As an example, AOD-binned evaluation results are shown in Fig. 2. for Terra DT&DB and in Fig. S6 for all products. A general tendency towards positive satellite-retrieved AOD offsets is observed for most products under background conditions. On average, 70 %–80 % of monthly AODs fall into class “background” (AOD≤0.2), so total AOD mean biases are expected to have similar behaviour. TOMS and OMI have the highest positive offsets globally, which is in line with the results from the dataset spatial intercomparison (Sect. S2). Offsets close to 0 for background AOD are observed for the MODIS MAIAC products.
For most products, except MODIS DT&DB, AOD offsets become negative for AOD>0.2 (fine- and coarse-dominated aerosol types) with increasing amplitude (up to 0.2–0.5) towards highest AOD values. MODIS DT&DB show the lowest offsets for . Offsets for VIIRS are close to 0 for AOD<0.5 and reach ca. 30 % of AOD at AOD≈1. For the current MISR standard product, AOD is systematically underestimated for ; this is largely due to treatment of the surface boundary condition at high AOD (Kahn et al., 2010) and is addressed in the research aerosol retrieval algorithm (Garay et al., 2019; Limbacher and Kahn, 2019). Except for TOMS and Terra MAIAC, offsets are smaller for coarse-dominated AOD.
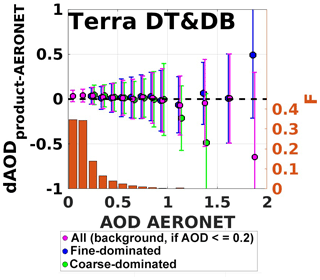
Figure 2Difference between Terra DT&DB and AERONET monthly AOD for selected AOD bins: median bias (circles), bias standard deviation (error bars) for all AOD types (purple), background aerosol (purple; AOD≤0.2), fine-dominated AOD (blue) and coarse-dominated AOD (green) The fraction (F) of points in each bin is represented by orange bars. For all individual products see Fig. S6.
AOD products retrieved from satellites having better coverage show a better agreement with AERONET monthly aggregates. Thus, sampling differences (swath and pixel selection) are critical in evaluation of monthly products, as expected but are not the only factor influencing the evaluation results.
3.1.2 AOD evaluation over selected regions
Due to differences in instrument specifications and retrieval approaches, the performance of retrieval algorithms depends largely on aerosol type, aerosol loading and surface properties at certain locations (e.g. Sayer et al., 2014). In this section we show the evaluation results for AOD products in two selected regions: Europe and ChinaSE (Fig. 3). Results for all regions are shown in Fig. S7. For each region, statistics (R, % of points in GE, offset and RMSE) for all 16 products are combined into one subplot. The merged AOD product M is introduced in Sect. 5.2; evaluation results for that product are summarised in Sect. 5.2.1.
Algorithm performance over Europe is similar for most products, with an R of 0.55–0.65, 45 %–55 % of the pixels in the GE, an offset of 0.05–0.1 and RMSE of ∼0.1. For TOMS and OMI, the performance of each is slightly worse than for other products in Europe. In ChinaSE, the offset (0.1–0.2) and RMSE (0.2–0.3) are considerably higher than in Europe, and fewer pixels fit within the GE (15 %–30 %). This is likely due to a combination of high AOD loading and accompanying high uncertainty in the products, indicated by high variability in aerosol composition and surface properties. In Indonesia and for the biomass burning outflow over the Atlantic, the MODIS and MISR products show a better agreement with AERONET than the ATSR-family products.
Several products which use different surface treatment (ATSR SU, MODIS-family and MISR) show a similarly higher R over AfN, an area of high surface reflectance. However, a high R does not imply that performance is better, only that variations in AOD are captured better. Other statistics (number of pixels within GE, offset and RMSE) in AfN are worse compared with those in Europe.
Overall, no single product has the best statistics for all metrics and regions. Retrievals tend to perform well in areas with darker (more vegetated) surfaces and where aerosol type is less variable over time. In these cases, biases are small and retrieval uncertainties are often better than the GE, tracking temporal AOD variability well but with a tendency to underestimate high-AOD events. In more complex tropical environments, data should be used with greater caution, as there is a greater tendency to underestimate AOD. However, correlation often remains high, suggesting a good ability to identify monthly AOD variations, despite this underestimation.
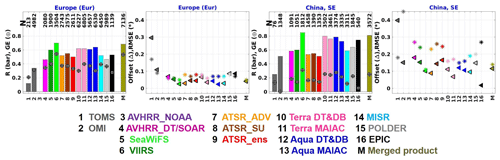
Figure 3AERONET evaluation statistics for Europe and ChinaSE: correlation coefficient R, bar, and fraction of pixels satisfying the GCOS requirements, GE, ⊕; offset (satellite product−AERONET), Δ, and root-mean-square error RMSE, *. Shown for AOD monthly aggregates for each product (1:16; legend for products below the plot) and the L3 merged product (M; approach 2 with RM2 for all aerosol types; for details see Sect. 4.2) with corresponding colours (legend) for the selected regions (as in Fig. 1). N is the number of matches with AERONET. Note, for products that do not provide the global coverage (e.g. no retrieval over oceans), the results are missing. For all studied regions, see Fig. S7.
3.2 AOD time series
In order to move towards consistency in regional and global AOD records derived from multiple satellites using different sensors and retrieval techniques, this section examines annual regional AOD time series obtained from the different products.
Besides the positive offset for TOMS and OMI (Figs. S1, S2, S6 and S7), consistent temporal patterns are observed, and similar interannual AOD variability is tracked by all datasets (Figs. 4 and S8). AOD peaks in Europe in 2002, in ChinaSE in 2006/2007, 2011 and 2014, (possibly related to changes in anthropogenic emissions; Sogacheva et al., 2018a, b). Relative AOD peaks over the Atlantic dust area in 1998, 2012 and 2015 (Peyridieu et al., 2013), and obvious AOD peaks in Indonesia related to the intensive forest fires in 1997, 2002, 2006 and 2015 (Chang et al., 2015; Shi et al., 2019) are clearly seen.
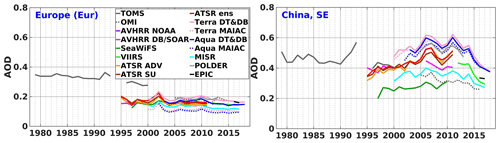
Figure 4Annual AOD time series from different products (see legend) for Europe and ChinaSE. For all selected regions see Fig. S8.
However, significant regional offsets between products exist, which are largest in regions with high aerosol loading. Over ChinaSE, MODIS-family products show higher monthly AOD compared to all others. Over AfN, ATSR SU and ATSR_ens reach higher monthly aggregated AOD than the MODIS-family products, whereas comparisons with AERONET are similar for ATSR and MODIS (with slightly higher RMSE for ATSR by 0.05); differences are likely tied to the small number of stations in this region. A large offset between MODIS and ATSR is revealed over Australia (Fig. S8).
AOD annual cycles for individual products for the year 2008 are discussed in Sect. S8. As in the annual time series (Figs. 4 and S8), the annual AOD cycles are similar between the products (Fig. S9), with more pronounced deviations in areas of high AOD.
Here, 12 AOD products (all available at 0.55 µm) were used to create a merged AOD product for the period of 1995–2017. The temporal availability of the AOD products is shown in Table 2 (counting cases of partial coverage of a dataset during a year as available).
Table 2Availability and coverage of the AOD products for merging for each year in the period 1995–2017. N: annual number of available products.
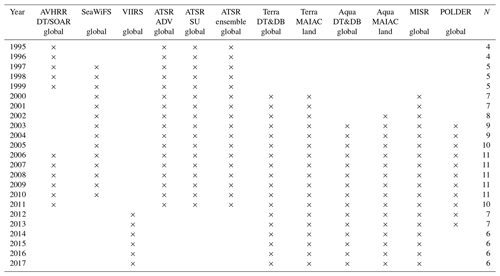
We tested two broad approaches for merging, summarised in Fig. 5. In the first, the median AODs from the available (10 globally and two over land) individual uncorrected and offset-adjusted (shifted to a common value) products were calculated (approach 1, Sect. 4.1 for details). In the second approach, AOD-weighted means were created where the weights for individual products were derived from the evaluation with the AERONET through two different ranking methods (see approach 2 in Sect. 4.2 for details). The same merging scheme was applied to the L3 uncorrected products (Sect. 2.2) and regional time series (Sect. 3.1) yielding 10 merged AOD products and 10 merged regional time series.
To achieve best estimates of the regional AOD by merging multi-sensor monthly AOD data, the systematic and random components of uncertainties within each product should be considered explicitly. However, this cannot yet be done; only some of the L2 products used to create the L3 monthly products contain pixel-level propagated or estimated uncertainties, and their associated propagations to L3 products (together with other contributions from e.g. sampling limitations) have not yet been quantified robustly. The analysis herein therefore represents an initial effort in the absence of a full uncertainty budget. Uncertainties for the chosen merged L3 product (details are discussed in Sect. 5.2.2) were estimated as the root-mean-squared sum of the deviations between the chosen merged product and either the median from the all uncorrected products (approach 1) or each of the other seven merged products (approach 2).
4.1 Approach 1: AOD median for uncorrected and offset-adjusted (shifted) AOD products
The mean (arithmetic average) value, although commonly used in climate studies, is not generally equal to the most frequently occurring value (the mode) and may not reflect the central tendency (the median) of strongly asymmetrical distributions such as those that can be found for AOD (O'Neill et al., 2000; Sayer and Knobelspiesse, 2019). Although the central limit theorem implies that this should be less of an effect when making an estimate of the mean AOD from a cluster of AOD datasets (i.e. a merged time series), in practice this is unlikely to be fully the case because the different datasets are not independent estimates of the underlying AOD field. This is because they are made with sensors and techniques which are not independent (i.e. typically similar spectral/spatial bands and sampling limitations) and may have different bias characteristics. Further, by itself, the mean does not provide any information about how the observations are scattered, whether they are tightly grouped or broadly spread out. Thus, we study the median (which is more robust in the presence outliers which might be caused by a poorly performing algorithm in a certain region) and standard deviations (as a metric of diversity) between the products chosen for merging.
As shown in Sect. 3, the AOD time series of different products display highly consistent temporal patterns, albeit with spatiotemporally varying offsets (Figs. 4, S8 and S9). We use the Terra DT&DB product as a reference to estimate the average offsets between products, because its time period overlaps with each AOD product considered in the current study.
Means and standard deviations of the offsets for all individual products from the Terra DT&DB AOD are shown in Fig. 6 for Europe and ChinaSE and in Fig. S10 for all selected regions. Offset magnitudes and their variations depend on AOD loading; offsets are typically higher for high AOD. Over land, ocean and thus globally, the offset is negative relative to Terra DT&BD for most of the products. This includes Europe and ChinaSE. However, over the bright surface area in northern Africa, AVHRR DT/SOAR, VIIRS, ATSR SU and ATSR ensemble show high (0.05–0.1) positive bias. Also, all ATSR products are biased high in Australia and South America. Thus, the median for the offset-adjusted product is expected to be positive biased. For details, see Sect. 5.1, where evaluation results for the AOD products merged with different approaches are discussed.
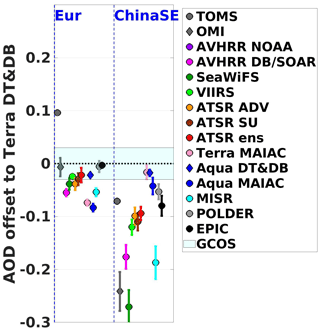
Figure 6Regional annual average AOD offset between each dataset and the Terra DT&DB dataset. GCOS requirement of ±0.03 is shown as a background colour. For all selected regions, see Fig. S10.
With the shifted median merging approach, each AOD product was shifted on a regional basis, based on its regional offset with respect to Terra DT&DB (Sect. 5.2). The median and standard deviation of AOD time series were then derived from these 10 shifted and Terra DT&DB data records.
4.2 Approach 2: weighted AOD
4.2.1 Method
As shown in Sect. 3.1, the products differ in the degree to which each represents the AERONET values on the monthly scale. Our second approach is a weighted mean AOD, where the weights are assigned based on the agreement of each dataset with monthly AERONET averages. This represents an initial attempt to adjust the level of confidence assigned to each product on a regional basis; better-comparing products are given more weight in the calculation of a combined product.
An AOD-weighted mean was calculated, with a ranking approach based on the statistics from the AERONET comparison for AOD: R, bias, RMSE, GE (Figs. 4 and S8) and median bias of the binned AOD in the range [0.45, 1] (Figs. 3 and S7). The last criterion was added to specifically consider algorithm performance for higher AOD.
Two ranking methods were tested. For the first ranking method (RM1) based on best statistics, the 12 products were ranked from 1 (worst) to 12 (best) for each statistic (R, GE, RMSE, bias and binned bias) separately. The five separate ranks were then summed, so the maximum possible rank is . A downside of this method is that when several products have similar statistics small variations in statistics can produce large spread in ranking. Note that no product received a perfect (60) rating.
To overcome this potential downside, the second ranking method (RM2) considers statistics falling into binned ranges (rather than the absolute evaluation statistics). For each statistic, the following windows, [0.5, 1] for R, [0, 0.5] for GE, [0, 0.2] for bias, [0, 0.15] for RMSE and [−0.5, 0] for the binned bias, were divided into 10 bins, and a rank (from 1 to 10) was assigned depending on which bin a particular statistic falls for a particular product. As a result, several algorithms can be ranked equally for certain statistics if their statistics fell within the same bin. For example, if R for three products is between 0.8 and 0.85, all three receive a rank score of 8 for that statistic.
The sum of the five ranks (R, GE, RMSE, bias and binned bias), w, for each product i was calculated and transformed to a weight of each product (as a fraction of total sum for the product from the total sum of ranking for all products) to calculate the AOD-weighted mean, , as follows:
As shown in Sect. 3.1, the performance of the retrieval algorithms often depends on the aerosol conditions (aerosol type and loading; Fig. 2) and surface properties. Accordingly, weights for the different AOD products were calculated separately for each region for different aerosol types (background, fine-dominated or coarse-dominated) separately and “all” aerosol types together considering the corresponding regional statistics from the AERONET comparison. However, aerosol types often change in time and space within the same region (Fig. S5). Thus, those weights for each aerosol type were applied globally to merge both L3 monthly products and time series. As a result, eight merged AOD products were obtained, which include the following: the product of two ranking approaches (RM1 and RM2) and four sets of statistics (all points and the background and the fine- and coarse-dominated subsets).
4.2.2 Ranking results (weights) for individual products
The weighting of the contribution of each product to the merged data product is shown in Fig. 7 (Europe and ChinaSE) and Fig. S11 (all selected regions) for three aerosol types (background, fine-dominated and coarse-dominated) and all aerosol types together (all). With some exceptions (e.g. in AOb, where the RM2 weight of Aqua DT&DB is ca. 15 % higher for coarse-dominated type, and in Australia, where the RM2 weight of SeaWiFS and Aqua MAIAC is 10 %–15 % higher for coarse-dominated type; Fig. S11), the difference in weights obtained with RM1 and RM2, if they exist, does not exceed 5 %–10 %. Thus, the ranking methods RM1 and RM2 introduced in the current study produce similar results. Some products show a better performance for certain aerosol types (Figs. 4 and S4). Thus, the weight of the product depends on which aerosol type is favoured for merging. For example, in Europe VIIRS has lower weight for fine-dominated aerosols, whereas the corresponding weight for ATSR SU is higher for that aerosol type. In ChinaSE, Terra DT&DB performs worse than Terra MAIAC for background aerosols, so for that aerosol type the weight for Terra MAIAC is higher.
As with the results discussed in Sect. 3, none of the algorithms consistently outperforms the others in all regions. There is no clear leader over Europe, a region with low AOD, indicating a similar performance of all algorithms under background conditions. Over land globally, also a region with low AOD, the ranks are similar for EOS (electro-optical-system) sensors and ATSR, with somewhat higher number for VIIRS. Over ocean globally, the ranks are similar for all existing products. One likely reason that the VIIRS and MODIS ranks are often higher is their better coverage, which enables them to better represent AERONET monthly means over land as they sample the variations more fully. However, MODIS is ranked lower over the Atlantic dust region. The lowest ranks are obtained consistently for TOMS, OMI and POLDER, due to their high biases.
Ranks for the different aerosol classes (all, background, fine-dominated and coarse-dominated) are different, which raises another aspect of using multiple products. Over land, MODIS MAIAC often has a higher rank for background AOD, whereas MODIS DT&DB is better for other aerosol types.
As a recap, 10 merged products are created, which include the following: shifted and unshifted medians from approach 1 and eight (two ranking methods times four aerosol type classes) from approach 2. In this section these products are evaluated against AERONET.
5.1 Evaluation of the all merged L3 AOD products with AERONET
Evaluation results (using the same method as in Sect. 3.1) reveal similarities in the accuracy of products merged with different approaches. The AOD binned bias of the merged products (Fig. S12) shows a similarly small deviation from AERONET (±0.03) for AOD<0.5 (positive for AOD<0.3 and negative for ). The offset is slightly higher for the median of the shifted AOD product (approach 1), because as discussed earlier, Terra DT&DB has a positive bias relative to most of the other individual products; this results in slightly elevated AOD compared to the others. For AOD>0.5, where the number of cases is very low, the underestimation increases as AOD increases. As for individual products, the coarse-dominated merged products have the smallest offset with AERONET.
Correlation coefficient, number of the pixels in the GE, offset and RMSE for the AOD merged product are shown in Fig. 8 for Europe and ChinaSE and in Fig. S13 for all regions. The merged products have the best temporal coverage and the number of points used for validation (N) is higher than for any individual product. The correlation coefficients and the number of the pixels matching within the GE are as high as for the one or two best ranked products in the corresponding regions, except for the product merged with approach 2. The offset is close to the average offset, and the RMSE tends to be lowest. Thus, the quality of the merged products, except for the shifted AOD product, is as good as that of the most highly ranked individual AOD products in each region.
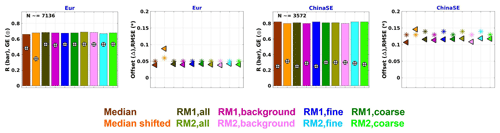
Figure 8AERONET comparison statistics: correlation coefficient R, bar, and fraction of pixels satisfying the GCOS requirements, GE, ⊕; offset, Δ, and root-mean-square error RMSE, *. Shown for AOD products merged with different approaches, median, shifted median, RM1 and RM2 for different aerosol types for Europe and ChinaSE. For all regions, see Fig. S13.
5.2 Final merged product evaluation and intercomparison with individual products
The agreement of the RM1 and RM2 approaches is encouraging, as we can conclude from the big-picture analysis (Sect. 5.1) that the details of the methodology do not matter much. As there is no significant difference in the evaluation results for products merged with approaches 1 and 2, we choose the RM2 approach for all aerosol types as the main merged product. We use this for further intercomparison with individual products to reveal the regional and seasonal differences between the products. If not specifically stated, the merged product mentioned below is the one obtained with RM2 for all aerosol types (RM2 for all).
5.2.1 Summarised evaluation results
The difference between the L3 merged product and the median of all individual products used for merging (Table 2) was calculated for the year 2008 (Fig. 9a, as Fig. S1 for individual products). The difference is within GCOS requirements over both land and ocean (0.009 and 0.007, respectively) and globally (0.008). High latitudes contribute most to the positive bias over oceans, whereas a positive bias is observed over land mostly over bright surfaces.
The evaluation statistics for the L3 merged product against AERONET extracted from Figs. S12 and S13 are combined in Fig. 9b, c, d for all 15 regions, as well as for land, ocean and globally. For most regions, R is between 0.75 and 0.85, 20 %–60 % fall within the GE, and the RMSE and offset are between 0.05 and 0.1, though somewhat higher for the regions with potentially high AOD loading (Indonesia, AOd, AsW and AsE). Statistics for the merged product (M) are also shown in Figs. 3 and S7 for comparisons with individual products.
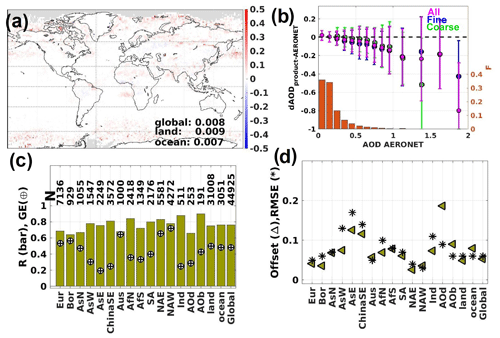
Figure 9(a) L3 merged (approach 2 with RM2 for all) AOD product deviation from the annual median AOD calculated from individual products used for merging (Table 2) for the year 2008 (as Fig. S1 for individual products), (b) L3 monthly merged AOD product evaluation with AERONET: binned AOD bias for all (purple; background (AOD<0.2; purple), fine-dominated (blue) and coarse-dominated (green) aerosol types. (c,d) Regional statistics (c: correlation coefficient R, bar, and fraction of pixels that fulfil the GCOS requirements, GE, circle; d: offset, Δ; RMSE, *).
5.2.2 Uncertainties
Uncertainties (unc, meaning 1−σ of the uncertainty distribution) for the merged L3 products (monthly, seasonal and annual) were estimated as the root-mean-squared sum of the deviations between the chosen merged product M (RM2 for all), the median from the all uncorrected products (approach 1) and each of the other seven merged products (approach 2, with RM1 for all aerosol types and RM1 and RM2 each applied for background, fine-dominated and coarse-dominated particles).
where mi is AOD from alternative merged product i, M is AOD from the chosen merged product (RM2 for all), and N is the number of the alternative merged products. Note that this is a structural uncertainty (i.e. a sensitivity to diversity and decisions in dataset merging) rather than a total uncertainty for the merged product. Seasonal and annual uncertainties for the year 2008 are shown in Fig. 10. These uncertainties show artefacts at regional boundaries because the merging was done according to regional statistics.
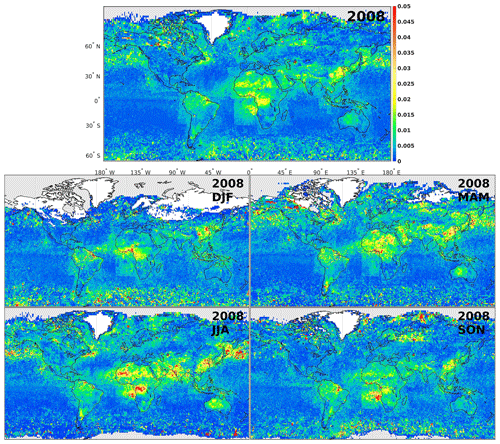
Figure 10Seasonal and annual structural uncertainties between the L3 merged product (M; approach 2 with RM2 for all) and other L3 merged products calculated with the approaches 1 and 2 for the year 2008.
The estimated annual and seasonal structural uncertainties are low, 0.005–0.006 globally. They show seasonal dependence, reaching 0.008 and 0.009 on average over land in MAM and JJA, respectively. The uncertainties are larger (0.01–0.03, on average, up to 0.05) in regions with high AOD (e.g. ChinaSE, India in JJA, AfN in MAM and JJA, AfS in JJA and SON). This means that the uncertainties introduced through the choice of merging strategy often fulfil the requirements calculated by Chylek et al. (2003) for an AOD uncertainty of 0.015 over land and 0.010 over ocean, in order to estimate the direct aerosol radiative effect to within 0.5 W m−2. The fact that this merging uncertainty estimate is smaller than the previously discussed GCOS goal uncertainties implies that reasonable merging method decisions may be of secondary importance in terms of meeting those goals. It is cautioned, though, that since many of the algorithms are susceptible to the same error sources and subject to similar sampling limitations, the uncertainty estimates calculated here are likely to be a lower bound on the true uncertainty in the merged datasets. And it should be remembered that these uncertainties cover only the aspect of choosing the merging method but not the entirety of the uncertainties in the merged datasets versus AERONET.
5.2.3 Spatial and temporal intercomparison with other products
The deviation between individual products and the merged product for the year 2008 is shown in Fig. 11. Among the products used for merging, POLDER has largest positive offset (0.026), and SeaWiFS has the highest largest negative offset (−0.026) on global average. Over land, POLDER has the highest positive offset (0.031); the offsets for ATSR SU and Terra DT&DB are also high (0.024 and 0.023, respectively). The highest negative offsets relative to the merged product are for MAIAC (−0.046 and −0.041 for Terra and Aqua, respectively). Over ocean, POLDER, Terra DT&DB and ATSR ADV are offset high by 0.022–0.024, whereas ATSR SU and SeaWiFS are offset low (−0.030 and −0.027, respectively) compared to the merged AOD product. Most of the observed global, land and ocean AOD offsets (except for Aqua MAIAC over land) are within the GCOS requirement of ±0.03. VIIRS agrees best with the merged product globally (0.003) and over ocean (−0.003); AVHRR DT/SOAR and AQUA DT&DB agree best with the merged product over land, showing opposite-in-sign offsets of −0.011 and 0.009, respectively. Regional biases between the individual products and the merged product are similar to regional biases shown in Fig. 2, where the individual products were compared with median AOD calculated from all individual products available at 0.55 µm.
Regional annual offsets between individual AOD products and the merged AOD product are shown in Fig. S14 (cf. with those for the median AOD product in Figs. 6 and S10). For AsE, which includes ChinaSE and AfN, the AOD offset is higher than 0.03 (GCOS requirement in low-AOD conditions) for some products. However, those areas are characterised by high AOD loading (annual AOD is between 0.4 and 0.8) that is related to e.g. anthropogenic pollution and/or dust events. If the GCOS requirement of 10 % of AOD is also applied here, then most of the offsets are within the GCOS requirements. The highest regional offsets relative to the merged AOD dataset are associated with products which provide AOD at wavelengths other than 0.55 µm – TOMS (0.50 µm), OMI (0.50 µm) and EPIC (0.44 µm) – and thus are not used for merging.
In some regions, AOD offsets between individual products and the merged product show seasonal behaviour (Fig. S15). In ChinaSE, the negative offsets for AVHRR NOAA, SeaWiFS and VIIRS are most pronounced in JJA. In AsW, the ATSR ADV positive offset is higher for that season. In AfN, most products have their largest negative offsets in JJA, whereas ATSR SU and ATSR_ens (which includes the ATSR SU product) have their highest positive biases. In SA, offsets are lower in JJA for all products. In AOb offsets are lower in MAM, and in AOd offsets are lower in SON for all products.
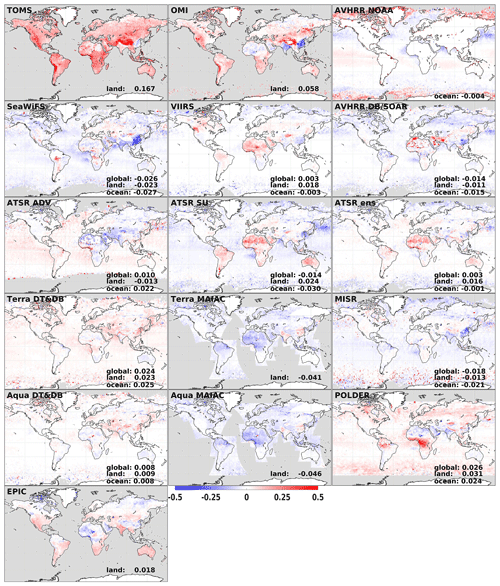
Figure 11AOD deviation of the individual products relative to the merged AOD product for the year 2008. Global, land and ocean AOD mean differences are shown for each product, when available.
As the L3 AOD merged products (Sect. 5), the AOD time series from the individual products (Figs. 4 and S8) were merged, using approach 1 (median for uncorrected AOD) and approach 2 (RM1 and RM2 for different aerosol types). The shifted AOD median (approach 1 for shifted products) has clear limitations when the product chosen as a reference (Terra DT&DB, in our case) deviates considerably from other products over most of the regions (except for Aus, AfN and SA; Fig. S8). Thus, the median for shifted products is not discussed here. However, the median-shifted AOD approach allows an extension of the time series back to 1978–1994, where only the TOMS AOD (over land) and AVHRR NOAA (over ocean) long-term products currently exist and the merging approaches introduced in the current study are not applicable.
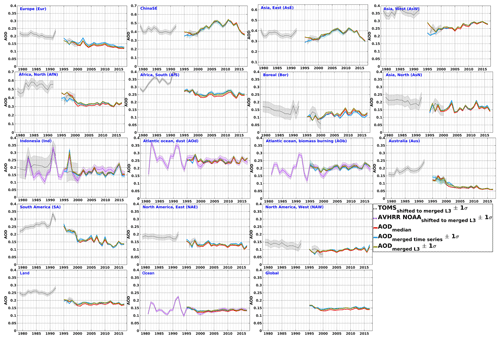
Figure 12Annual AOD time series merged with two different approaches (red and light blue for approaches 1 and 2, respectively) and AOD time series from the L3 merged data (approach 2; olive) for the selected regions. In each, ±1σ of the AOD from all uncorrected AOD products is shown as light blue shadow (often small, thus not visible). TOMS over land and AVHRR NOAA over ocean products shifted to the merged time series are also shown with dashed grey and purple lines, respectively, when available.
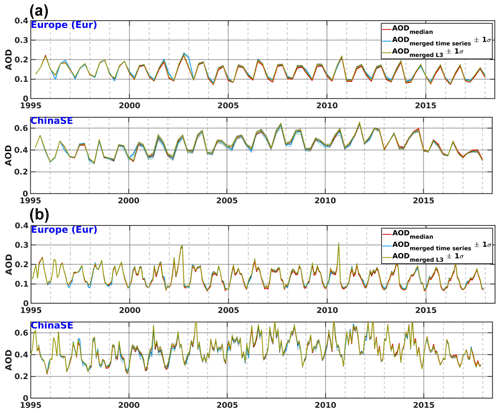
Figure 13 (a) Seasonal and (b) monthly AOD median time series (red), merged time series (blue) and time series from the merged L3 product (olive) for Europe and ChinaSE. AOD±1σ for the merged time series and for the time series from the merged L3 products are shown as light blue and light olive shadows, respectively. Note the different scale. For all selected regions, see Figs. S16 and S17.
The two merging approaches (approach 1 for uncorrected products and approach 2 for weighted AOD) tested here agree well (Fig. 12). The offsets between time series calculated with different approaches are again low (0.004–0.011). Spatial consistency is indicated by high correlation (similar positions of peaks) in AfN and its Atlantic dust outflow region. Interannual variation as well as the standard deviations are highest for regions with the largest AOD, e.g. over ChinaSE (anthropogenic emissions) and Indonesia (biomass burning). The time series of ChinaSE follows the known patterns caused by stepwise regional emission reductions in the last 25 years (Sogacheva et al., 2018b). AOD time series merged with different approaches show a good agreement for all timescales: annual (Fig. 12), seasonal and monthly (Fig. 13a and b, respectively, for Europe and China and Figs. S16 and S17 for all studied regions).
The offsets between the merged time series and time series calculated from the merged L3 product have a regional component and, as, discussed above, depend on the availability of the products (Table 2). The offsets between the time series merged with different approaches (Table 3) are slightly higher for all regions for the periods 1995–1999 and 2012–2017, when fewer products are available for merging (Table 2). The deviation up to 0.05 (AODapproach 1>AODapproach 2) is observed over Indonesia and North America before 2002, when both MODIS satellites become operational. For other regions, the deviation is considerably lower (below 0.03). By adding MISR and both MODIS products in 2000/2002, the offset between the time series is reduced. ATSR products are not available starting in 2012, when the VIIRS product became available. In 1995–1999, the mean offset is similar for all three time series. The offsets are higher for regions with high AOD loading (e.g. Asia and northern Africa, Fig. S18). In 2000–2011 and 2012–2017, the offset is lowest (0.004) between the merged and the median time series, as well as between the merged time series and the time series calculated from the merged L3 product. The agreement in the time series obtained with different approaches supports the conclusion made based on the evaluation results that, for the big-picture analysis of overall trends, details of the methodology do not matter very much.
Annual, seasonal and monthly time series from the merged L3 monthly AOD show slightly higher deviation of both signs compared to the merged time series discussed above. Interestingly, seasonality is observed in the deviation. In AfN, the AOD from the monthly merged L3 is higher in autumn for the period of 1995–1999. In Bor and AsN (Figs. S16 and S17), the deviation is higher in spring for the period of 1997–1999. A possible explanation might be the sparser coverage in those areas (due to restrictions in retrieval algorithms to retrieve bright surfaces, e.g. desert or snow). Regional offsets between the annual, seasonal and monthly AOD merged time series and the time series from the merged L3 monthly product are summarised for three timescales in Fig. S19. The offset is lower for annual data and generally increases with the time resolution. As the previous analysis showed, the offset is bigger in high-AOD regions (e.g. Asia, AfN and SA).
Overall, good agreement exists between the time series calculated using different merging approaches and different orders of the processing steps. There is a general consistency, and similar temporal patterns are observed between the time series merged with two approaches and the time series from merged L3 AOD product, despite small differences, which are more pronounced at the beginning of the period, when less products are available. With only few exceptions, the offsets between the AOD time series calculated with different approaches are within the GCOS requirement of ±0.03 or 10 % of AOD.
A separate study is planned where regional and global trends in this merged AOD L3 product will be analysed.
Table 4Instrument, archive, URL and DOI (last access: 17 February 2020, for all), name and creator of the products used in the current study (if available).
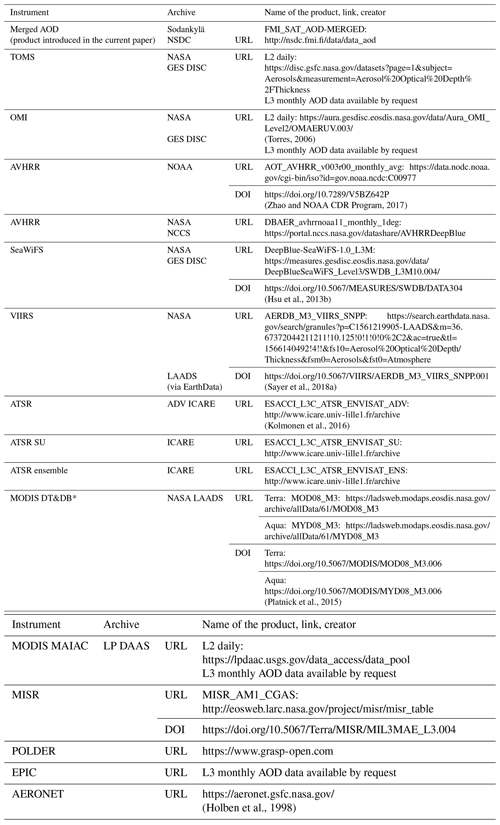
* Additional online documentation at: https://modis-atmos.gsfc.nasa.gov/, https://darktarget.gsfc.nasa.gov/.
This study has analysed the consistency of regional time records of monthly AOD from 16 different satellite products. These were obtained from a wide range of different instruments – TOMS, AVHRR, SeaWiFS, ATSR-2, AATSR, MODIS, MISR, POLDER, VIIRS and EPIC – with largely varying information content and sampling and with different algorithms based on different remote sensing approaches, quality filtering, cloud masking and averaging. Differences between those 16 data records in a set of regions with different characteristics across the globe were demonstrated and verified against a ground-based AERONET monthly mean dataset in order to answer the question how well a satellite dataset can reproduce monthly gridded mean AERONET values in a region.
AOD time series (monthly, seasonal and annual) from the products show a good consistency of temporal patterns but significant regional biases due to all those differences. In many cases the more pronounced differences were between different algorithms applied to the same sensor, rather than between similar algorithms applied to different sensors. This is encouraging in that it implies that algorithmic uncertainties (either retrieval assumptions or pixel selection criteria) can be similar to or larger than sensor ones (e.g. calibration quality and sampling limitations), and as such, refining individual algorithms can still make meaningful steps towards providing better L3 products.
To build an AOD product merged from 12 individual satellite products, two different approaches were introduced and tested. In approach 1, a simple median of the 12 uncorrected and shifted to Terra DT&DR product time records was conducted. In approach 2, the AOD evaluation results (for different aerosol types) against AERONET were used to infer a ranking which was then used to calculate a weighted AOD mean. Two different ranking methods, RM1, simple ranking based on better statistics, and RM2, ranking based on binned statistics, were tested in approach 2. In addition, the order of the processing steps in approach 2 was interchanged (L3 dataset merging or regional merging) to test the stability of the results.
Ten merged L3 AOD monthly products were created and evaluated with AERONET. The evaluation shows that the quality of the merged products (except for one created with the approach 1 for shifted AOD) is as good as that of the most highly ranked individual AOD products in each region. One of the merged products (approach 2 with RM2 for all) was chosen as a final merged product (http://nsdc.fmi.fi/data/data_aod, last access: 20 January 2020), based on slightly better evaluation results. Structural uncertainties for the final merged product were estimated.
All merged regional AOD time series show a very high consistency of temporal patterns and between regions, and the time records with their uncertainties (standard deviations shaded around the median values) clearly illustrate the evolution of regional AOD. With few exceptions all merging methods lead to very similar results, which is reassuring for the usefulness and stability of the merged products.
There are of course caveats to these rather simple and straightforward merging approaches, which do not consider in much detail the differences in sampling and sensitivity to different conditions (e.g. surface brightness or number of independent observables) of the different instruments and algorithms. It is well known that monthly, seasonal or annual gridded mean values can carry large uncertainties, whether inferred from a few ground-based stations meant to represent a full grid cell or from satellite images containing large gaps due to limited swath, clouds or failed retrievals. Pixel-level uncertainties are becoming available for a growing number of satellite products, and it would be highly beneficial if these estimated errors could be propagated consistently to those gridded monthly products. However, this requires deeper insight and new methods to take into account correlation patterns among parts of the uncertainties and to estimate practically the sampling-based uncertainties in light of approximated AOD variability. Altogether, as frequently requested from a user point of view, the stability and consistency of regional, merged AOD time series should be seen as strengthening our confidence in the reliability of satellite-based data records. Recent, ongoing and future work to improve the Level 3 uncertainty budget of the satellite products – as well as assessment of spatio-temporal uncertainties in time-aggregated AERONET data – will benefit the creation and assessment of merged time series. The corresponding time series can be used in regional and global AOD trend analyses and for comparison with (climate and reanalysis) model AOD fields. Aside from the merged dataset itself, some key main outcomes of this research have been a quantification of the diversity between monthly satellite AOD products and their comparability with monthly averages from AERONET and the sensitivity of the merged time series to some sensible decisions which must be made in creating it. Merged AOD product will be extended as satellite missions continue and new data versions are released.
URL and DOI (if available) of the products used in the current study, as well as of the merged AOD product (FMI_SAT_AOD-MERGED), are summarised in Table 4.
The supplement related to this article is available online at: https://doi.org/10.5194/acp-20-2031-2020-supplement.
The exercise on AOD merging has been initiated and widely discussed by the AeroCom/AeroSat community. The work has been performed by LS, who collected data, developed the methodology, performed the analysis and wrote the extended draft of the paper. The evaluation results were widely discussed with the AOD data providers, who coauthor the paper. TP, AMS and RAK also considerably contributed to writing. All authors contributed to reviewing the drafts.
The authors declare that they have no conflict of interest.
The authors thank attendees of AeroCom/AeroSat workshops over the past several years for lively and informative discussions, which helped provide the impetus for and shape this analysis. AeroCom and AeroSat are unfunded community networks which participants contribute to within the remit and constraints of their other aerosol research.
The work presented is partly supported by the Copernicus Climate Change Service (contracts C3S_312a_lot5 and C3S_312b_Lot2) which is funded by the European Union, with support from ESA as part of the Climate Change Initiative (CCI) project Aerosol_cci (ESA-ESRIN projects AO/1-6207/09/I-LG and ESRIN/400010987 4/14/1-NB) and the AirQast 776361 H2020-EO-2017 project.
This paper was edited by Stelios Kazadzis and reviewed by three anonymous referees.
Ban-Weiss, G. A., Jin, L., Bauer, S. E., Bennartz, R., Liu, X., Zhang, K., Ming, Y., Guo, H., and Jiang, J. H.: Evaluating clouds, aerosols, and their interactions in three global climate models using satellite simulators and observations, J. Geophys. Res.-Atmos., 119, 10876–10901, https://doi.org/10.1002/2014JD021722, 2014.
Bellouin, N., Boucher, O., Haywood, J., and Shekar, R. M.: Global estimate of aerosol direct radiative forcing from satellite measurements, Nature, 438, 1138–1141, https://doi.org/10.1038/nature04348, 2005.
Bevan, S., North, P., Los, S., and Grey, W.: A global dataset of atmospheric aerosol optical depth and surface reflectance from AATSR, Remote Sens. Environ., 116, 199–210, 2012.
Boys, B. L., Martin, R. V., van Donkelaar, A., MacDonell, R. J., Hsu, N. C., Cooper, M. J., Yantosca, R. M., Lu, Z., Streets, D. G., Zhang, Q., and Wang, S. W.: Fifteen-year global time series of satellite-derived fine particulate matter, Environ Sci Technol., 48, 11109–11118, 2014.
Chang, C.-H., Hsiao, Y.-L., and Hwang, C.: Evaluating Spatial and Temporal Variations of Aerosol Optical Depth and Biomass Burning over Southeast Asia Based on Satellite Data Products, Aerosol Air Qual. Res., 15, 2625–2640, https://doi.org/10.4209/aaqr.2015.10.0589, 2015.
Chatterjee, A., Michalak, A. M., Kahn, R. A., Paradise, S. R., Braverman, A. J., and Miller, C. E.: A geostatistical data fusion technique for merging remote sensing and ground-based observations of aerosol optical thickness, J. Geophys. Res., 115, D20207, https://doi.org/10.1029/2009JD013765, 2010.
Chylek, P., Henderson, B., and Mishchenko, M.: Aerosol radiative forcing and the accuracy of satellite aerosol optical depth retrieval. J. Geophys. Res., 108, 4764, https://doi.org/10.1029/2003JD004044, 2003.
de Leeuw, G., Holzer-Popp, T., Bevan, S., Davies, W., Descloitres, J., Grainger, R. G., Griesfeller, J., Heckel, A., Kinne, S., Klüser, L., Kolmonen, P., Litvinov, P., Martynenko, D., North, P. J. R., Ovigneur, B., Pascal, N., Poulsen, C., Ramon, D., Schulz, M., Siddans, R., Sogacheva, L., Tanré, D., Thomas, G. E., Virtanen, T. H., von Hoyningen Huene, W., Vountas, M., and Pinnock, S.: Evaluation of seven European aerosol optical depth retrieval algorithms for climate analysis, Remote Sens. Environ., 162, 295–315, https://doi.org/10.1016/j.rse.2013.04.023, 2015.
de Leeuw, G., Sogacheva, L., Rodriguez, E., Kourtidis, K., Georgoulias, A. K., Alexandri, G., Amiridis, V., Proestakis, E., Marinou, E., Xue, Y., and van der A, R.: Two decades of satellite observations of AOD over mainland China using ATSR-2, AATSR and MODIS/Terra: data set evaluation and large-scale patterns, Atmos. Chem. Phys., 18, 1573–1592, https://doi.org/10.5194/acp-18-1573-2018, 2018.
Dubovik, O., Holben, B. N., Eck, T. F., Smirnov, A., Kaufman, Y. J., King, M. D., Tanre, D., and Slutsker, I.: Variability of absorption and optical properties of key aerosol types observed in worldwide locations, J. Atmos. Sci., 59, 590–608, 2002.
Dubovik, O., Herman, M., Holdak, A., Lapyonok, T., Tanré, D., Deuzé, J. L., Ducos, F., Sinyuk, A., and Lopatin, A.: Statistically optimized inversion algorithm for enhanced retrieval of aerosol properties from spectral multi-angle polarimetric satellite observations, Atmos. Meas. Tech., 4, 975–1018, https://doi.org/10.5194/amt-4-975-2011, 2011.
Dubovik, O., Lapyonok, T., Litvinov, P., Herman, M., Fuertes, D., Ducos, F., Lopatin, A., Chaikovsky, A., Torres, B., Derimian, Y., Huang, X., Aspetsberger, M., and Federspiel, C.: GRASP: a versatile algorithm for characterizing the atmosphere, SPIE: Newsroom, https://doi.org/10.1117/2.1201408.005558, 2014.
Dubovik, O., Li, Z., Mishchenko, M. I., Tanre, D., Karol, Y., Bojkov, B., Cairns, B., Diner, D. J., Espinosa, R., Goloub, P., Gu, X., Hasekamp, O., Hong, J., Hou, W., Knobelspiesse, K. D., Landgraf, J., Li, L., Litvinov, P., Liu, Y., Lopatin, A., Marbach, T., Maring, H., Martins, V., Meijer, Y., Milinevsky, G., Mukai, S., Parol, F., Qiao, Y., Remer, L., Rietjens, J., Sano, I., Stammes, P., Stamnes, S., Sun, X., Tabary, P. Travis, L. D., Waquet, F., Xu, F., Yan, C., and Yin, D.: Polarimetric remote sensing of atmospheric aerosols: instruments, methodologies, results, and perspectives, J. Quant. Spectrosc. Ra., 474–511, https://doi.org/10.1016/j.jqsrt.2018.11.024, 2019.
Eck, T. F., Holben, B. N., Reid, J. S., Dubovik, O., Smirnov, A., O'Neill, N. T., Slutsker, I., and Kinne, S.: Wavelength dependence of the optical depth of biomass burning, urban, and desert dust aerosol, J. Geophys. Res., 104, 31333–31350, 1999.
Escribano, J., Boucher, O., Chevallier, F., and Huneeus, N.: Impact of the choice of the satellite aerosol optical depth product in a sub-regional dust emission inversion, Atmos. Chem. Phys., 17, 7111–7126, https://doi.org/10.5194/acp-17-7111-2017, 2017.
Flowerdew, R. J. and Haigh, J. D.: An approximation to improve accuracy in the derivation of surface reflectances from multi-look satellite radiometers, Geophys. Res. Let., 22, 1693–1696, 1995.
Garay, M. J., Kalashnikova, O. V., and Bull, M. A.: Development and assessment of a higher-spatial-resolution (4.4 km) MISR aerosol optical depth product using AERONET-DRAGON data, Atmos. Chem. Phys., 17, 5095–5106, https://doi.org/10.5194/acp-17-5095-2017, 2017.
Garay, M. J., Witek, M. L., Kahn, R. A., Seidel, F. C., Limbacher, J. A., Bull, M. A., Diner, D. J., Hansen, E. G., Kalashnikova, O. V., Lee, H., Nastan, A. M., and Yu, Y.: Introducing the 4.4 km Spatial Resolution MISR Aerosol Product, Atmos. Meas. Tech. Discuss., https://doi.org/10.5194/amt-2019-340, in review, 2019.
GCOS: Systematic observation requirements for satellite-based data products for climate, 2011 update, World Meteorological Organization (WMO) Global Climate Observing System (GCOS) report GCOS-154, available at: https://library.wmo.int/doc_num.php?explnum_id=3710 (last access: 19 June 2019), 2011.
Giles, D. M., Sinyuk, A., Sorokin, M. G., Schafer, J. S., Smirnov, A., Slutsker, I., Eck, T. F., Holben, B. N., Lewis, J. R., Campbell, J. R., Welton, E. J., Korkin, S. V., and Lyapustin, A. I.: Advancements in the Aerosol Robotic Network (AERONET) Version 3 database – automated near-real-time quality control algorithm with improved cloud screening for Sun photometer aerosol optical depth (AOD) measurements, Atmos. Meas. Tech., 12, 169–209, https://doi.org/10.5194/amt-12-169-2019, 2019.
Gupta, P., Levy, R. C., Mattoo, S., Remer, L. A., and Munchak, L. A.: A surface reflectance scheme for retrieving aerosol optical depth over urban surfaces in MODIS Dark Target retrieval algorithm, Atmos. Meas. Tech., 9, 3293–3308, https://doi.org/10.5194/amt-9-3293-2016, 2016.
Han, B., Ding, H., Ma, Y., and Gong, W.: Improving retrieval accuracy for aerosol optical depth by fusion of MODIS and Caliop data, Tehni ki Vjesnik, 24, 791–800, 2017.
Heidinger, A. K., Cao, C., and Sullivan, J.: Using Moderate Resolution Imaging Spectrometer (MODIS) to calibrate Advanced Very High Resolution Radiometer (AVHRR) reflectance channels, J. Geophys. Res., 107, 4702, https://doi.org/10.1029/2001JD002035, 2002.
Holben, B. N., Eck, T. F., Slutsker, I., Tanré, D., Buis, J. P., Setzer, A., Vermote, E., Reagan, J. A., Kaufman, Y., Nakajima, T., Lavenu, F. , Jankowiak, I., and Smirnov, A.: AERONET – A federated instrument network and data archive for aerosol characterization, Remote Sens. Environ., 66, 1–16, 1998.
Holben, B. N., Tanre, D., Smirnow, A., Eck, T. F., Slutsker, I., Abuhassan, N., Newcomb, W. W., Schafer, J. S., Chatenet, B., Lavenu, F., Kaufman, Y. J., Vande Castle, J., Setzer, A., Markham, B., Clark, D., Halthore, R., Karneli, A., O'Neili, N. T., Pietras, C., Pinker, R. T., Vos. K., and Zibord, G.: An Emerging Ground-Based Aerosol Climatology, J. Geophys. Res., 106, 12067–12097, 2001.
Hsu, N. C., Tsay, S. C., King, M. D., and Herman, J. R.: Aerosol properties over bright-reflecting source regions, IEEE T. Geosci. Remote, 42, 557–569, 2004.
Hsu, N. C., Jeong, M.-J., Bettenhausen, C., Sayer, A. M., Hansell, R., Seftor, C. S., Huang, J., and Tsay, S.-C.: Enhanced Deep Blue aerosol retrieval algorithm: The second generation, J. Geophys. Res.-Atmos., 118, 9296–9315, https://doi.org/10.1002/jgrd.50712, 2013a.
Hsu, N. C., Sayer, A. M., Jeong, M.-J., and Bettenhausen, C.: SeaWiFS Deep Blue Aerosol Optical Depth and Angstrom Exponent Monthly Level 3 Data Gridded at 1.0 Degrees V004, Greenbelt, MD, USA, Goddard Earth Sciences Data and Information Services Center (GES DISC), https://doi.org/10.5067/MEASURES/SWDB/DATA304, 2013b.
Hsu, N. C., Lee, J., Sayer, A. M., Carletta, N., Chen, S.-H., Tucker, C. J., and Tsay, S.-C: Retrieving near-global aerosol loading over land and ocean from AVHRR, J. Geophys. Res., 122, 9968–9989, https://doi.org/10.1002/2017JD026932, 2017.
Hsu, N. C., Lee, J., Sayer, A. M., Kim, W., Bettenhausen, C., and Tsay, S.-C.: VIIRS Deep Blue aerosol products over land: Extending the EOS long-term aerosol data records, J. Geophys. Res.-Atmos., 124, 4026–4053, https://doi.org/10.1029/2018JD029688, 2019.
Huang, D., Lyapustin, A., Korkin, S., Wang, Y., Blank, K., and Marshak, A.: The Multi-Angle Implementation of Atmospheric Correction (MAIAC) algorithm for DSCOVR EPIC and initial analysis of data products, Remote. Sens. Environ., in review, 2020.
Ignatov, A. and Stowe, L. L.: Aerosol Retrievals from Individual AVHRR Channels. Part I: Retrieval Algorithm and Transition from Dave to 6S Radiative Transfer Model, J. Atmos. Sci., 59, 313–334, 2002.
IPCC: Summary for Policymakers of IPCC Special Report on Global Warming of 1.5 ∘C approved by governments, available at: https://www.ipcc.ch/site/assets/uploads/2018/11/pr_181008_P48_spm_en.pdf (last access: 30 April 2019), 2018.
Jethva, H. and Torres, O.: Satellite-based evidence of wavelength-dependent aerosol absorption in biomass burning smoke inferred from Ozone Monitoring Instrument, Atmos. Chem. Phys., 11, 10541–10551, https://doi.org/10.5194/acp-11-10541-2011, 2011.
Kahn, R. A., Gaitley, B. J., Garay, M. J., Diner, D. J., Eck, T. F., Smirnov, A., and Holben, B. N.: Multiangle Imaging SpectroRadiometer global aerosol product assessment by comparison with the Aerosol Robotic Network, J. Geophys. Res., 115, D23209, https://doi.org/10.1029/2010JD014601, 2010.
Kinne, S.: Remote sensing data combinations: superior global maps for aerosol optical depth, in: Satellite Aerosol Remote Sensing over Land, Springer, Berlin Heidelberg, 361–381, https://doi.org/10.1007/978-3-540-69397-0_12, 2009.
Kinne, S., Lohmann, U., Feichter, J., Schulz, M., Timmreck, C., Ghan, S., Easter, R., Chin, M., Ginouz, P. , Takemura, T., Tegen, I., Koch, D., Herzog, M., Penner, J., Pitari, G., Holben, B., Eck, T., Smirnov, A., Dubovik, O., Slutsker, I., Tanre, D., Torres, O., Mishchenko, M. Geogdzhayev, I., Chu, D. A., and Kaufman, Y.: Monthly averages of aerosol properties: A global comparison among models, satellite data, and AERONET ground data, J. Geophys. Res., 108, 4634, https://doi.org/10.1029/2001JD001253, 2003.
Kinne, S., Schulz, M., Textor, C., Guibert, S., Balkanski, Y., Bauer, S. E., Berntsen, T., Berglen, T. F., Boucher, O., Chin, M., Collins, W., Dentener, F., Diehl, T., Easter, R., Feichter, J., Fillmore, D., Ghan, S., Ginoux, P., Gong, S., Grini, A., Hendricks, J., Herzog, M., Horowitz, L., Isaksen, I., Iversen, T., Kirkevåg, A., Kloster, S., Koch, D., Kristjansson, J. E., Krol, M., Lauer, A., Lamarque, J. F., Lesins, G., Liu, X., Lohmann, U., Montanaro, V., Myhre, G., Penner, J., Pitari, G., Reddy, S., Seland, O., Stier, P., Takemura, T., and Tie, X.: An AeroCom initial assessment – optical properties in aerosol component modules of global models, Atmos. Chem. Phys., 6, 1815–1834, https://doi.org/10.5194/acp-6-1815-2006, 2006.
Kokhanovsky, A. A. and de Leeuw, G. (Eds.): Satellite Aerosol Remote Sensing Over Land, Springer-Praxis, Berlin, 388 pp., 2009.
Kolmonen, P., Sogacheva, L., Virtanen, T. H., de Leeuw, G., and Kulmala, M.: The ADV/ASV AATSR aerosol retrieval algorithm: current status and presentation of a full-mission AOD data set, Int. J. Digital Earth, 9, 545–561, https://doi.org/10.1080/17538947.2015.1111450, 2016.
Kosmale, M., et al.: in preparation, 2020.
Lee, H., Garay, M. J., Kalashnikova, O. V., Yu, Y., and Gibson, P. B.: How Long should the MISR Record Be when Evaluating Aerosol Optical Depth Climatology in Climate Models?, Remote Sens., 10, 1326, 2018.
Levy, R. C., Mattoo, S., Munchak, L. A., Remer, L. A., Sayer, A. M., Patadia, F., and Hsu, N. C.: The Collection 6 MODIS aerosol products over land and ocean, Atmos. Meas. Tech., 6, 2989–3034, https://doi.org/10.5194/amt-6-2989-2013, 2013.
Levy, R. C., Mattoo, S., Sawyer, V., Shi, Y., Colarco, P. R., Lyapustin, A. I., Wang, Y., and Remer, L. A.: Exploring systematic offsets between aerosol products from the two MODIS sensors, Atmos. Meas. Tech., 11, 4073–4092, https://doi.org/10.5194/amt-11-4073-2018, 2018.
Li, J., Carlson, B. E., and Lacis, A. A.: Application of spectral analysis techniques in the inter-comparison of aerosol data. Part I: An EOF approach to analyze the spatial-temporal variability of aerosol optical depth using multiple remote sensing data sets, J. Geophys. Res.-Atmos., 118, 8640–8648, https://doi.org/10.1002/jgrd.50686, 2013.
Li, J., Carlson, B. E., and Lacis, A. A.: Application of spectral analysis techniques in the inter-comparison of aerosol data. Part II: Using maximum covariance analysis to effectively compare spatio-temporal variability of satellite and AERONET measured aerosol optical depth, J. Geophys. Res.-Atmos., 119, 153–166, https://doi.org/10.1002/2013JD020537, 2014a.
Li, J., Carlson, B. E., and Lacis, A. A.: Application of spectral analysis techniques to the intercomparison of aerosol data – Part 4: Synthesized analysis of multisensor satellite and ground-based AOD measurements using combined maximum covariance analysis, Atmos. Meas. Tech., 7, 2531–2549, https://doi.org/10.5194/amt-7-2531-2014, 2014b.
Li, J., Li, X., Carlson, B. E., Kahn, R. A., Lacis, A. A., Dubovik, O., and Nakajima, T.: Reducing m ultisensor satellite monthly mean aerosol optical depth uncertainty: 1. Objective assessment of current AERONET locations, J. Geophys. Res.-Atmos., 121, 13609–13627, https://doi.org/10.1002/2016JD025469, 2016.
Li, S., Yu, C., Chen, L., Tao, J., Letu, H., Ge, W., Si, Y., and Liu, Y.: Inter-comparison of model-simulated and satellite-retrieved componential aerosol optical depths in China, Atmos. Environ., 141, 320–332, https://doi.org/10.1016/j.atmosenv.2016.06.075, 2016.
Li, Z., Zhao, X., Kahn, R., Mishchenko, M., Remer, L., Lee, K.-H., Wang, M., Laszlo, I., Nakajima, T., and Maring, H.: Uncertainties in satellite remote sensing of aerosols and impact on monitoring its long-term trend: a review and perspective, Ann. Geophys., 27, 2755–2770, https://doi.org/10.5194/angeo-27-2755-2009, 2009.
Limbacher, J. A. and Kahn, R. A.: Updated MISR over- water research aerosol retrieval algorithm – Part 2: A multi-angle aerosol retrieval algorithm for shallow, turbid, oligotrophic, and eutrophic waters, Atmos. Meas. Tech., 12, 675–689, https://doi.org/10.5194/amt-12-675-2019, 2019.
Liu, L., Lacis, A. A., Carlson, B. E., Mishchenko, M. I., and Cairns, B.: Assessing Goddard Institute for Space Studies ModelE aerosol climatology using satellite and ground-based measurements: A comparison study, J. Geophys. Res., 111, D20212, https://doi.org/10.1029/2006JD007334, 2006.
Lyapustin, A., Wang, Y., Korkin, S., and Huang, D.: MODIS Collection 6 MAIAC algorithm, Atmos. Meas. Tech., 11, 5741–5765, https://doi.org/10.5194/amt-11-5741-2018, 2018.
Martonchik, J. V., Kahn, R. A., and Diner, D. J.: Retrieval of Aerosol Properties over Land Using MISR Observations, in: Satellite Aerosol Remote Sensing Over Land, edited by: Kokhanovsky, A. A. and de Leeuw, G., Springer, Berlin, 267–293, 2009.
Michou, M., Nabat, P., and Saint-Martin, D.: Development and basic evaluation of a prognostic aerosol scheme (v1) in the CNRM Climate Model CNRM-CM6, Geosci. Model Dev., 8, 501–531, https://doi.org/10.5194/gmd-8-501-2015, 2015.
Mishchenko, M. I., Geogdzhayev, I. V., Cairns, B., Carlson, B. E., Chowdhary, J., Lacis, A. A., Liu, L., Rossow, W. B., and Travis, L. D.: Past, present, and future of global aerosol climatologies derived from satellite observations: A perspective, J. Quant. Spectrosc. Ra., 106, 325–347, https://doi.org/10.1016/j.jqsrt.2007.01.007, 2007.
Nabat, P., Somot, S., Mallet, M., Chiapello, I., Morcrette, J. J., Solmon, F., Szopa, S., Dulac, F., Collins, W., Ghan, S., Horowitz, L. W., Lamarque, J. F., Lee, Y. H., Naik, V., Nagashima, T., Shindell, D., and Skeie, R.: A 4-D climatology (1979–2009) of the monthly tropospheric aerosol optical depth distribution over the Mediterranean region from a comparative evaluation and blending of remote sensing and model products, Atmos. Meas. Tech., 6, 1287–1314, https://doi.org/10.5194/amt-6-1287-2013, 2013.
Naeger, A. R., Gupta, P., Zavodsky, B. T., and McGrath, K. M.: Monitoring and tracking the trans-Pacific transport of aerosols using multi-satellite aerosol optical depth composites, Atmos. Meas. Tech., 9, 2463–2482, https://doi.org/10.5194/amt-9-2463-2016, 2016.
North, P.: Estimation of aerosol opacity and land surface bidirectional reflectance from ATSR-2 dual-angle imagery: Operational method and validation, J. Geophys. Res., 107, AAC 4-1–AAC 4-10, 2002.
North, P., Briggs, S., Plummer, S., and Settle, J.: Retrieval of land surface bidirectional reflectance and aerosol opacity from ATSR-2 multiangle imagery, IEEE T. Geosci. Remote S., 37, 526–537, 1999.
O'Neill, N. T., Ignatov, A., Holben, B. N., and Eck, T. F.: The lognormal distribution as a reference for reporting aerosol optical depth statistics: Empirical tests using multi-year, multi-site AERONET sun-photometer data, Geophys. Res. Lett., 27, 3333–3336. https://doi.org/10.1029/2000GL011581, 2000.
Penning de Vries, M. J. M., Beirle, S., Hörmann, C., Kaiser, J. W., Stammes, P., Tilstra, L. G., Tuinder, O. N. E., and Wagner, T.: A global aerosol classification algorithm incorporating multiple satellite data sets of aerosol and trace gas abundances, Atmos. Chem. Phys., 15, 10597–10618, https://doi.org/10.5194/acp-15-10597-2015, 2015.
Peyridieu, S., Chédin, A., Capelle, V., Tsamalis, C., Pierangelo, C., Armante, R., Crevoisier, C., Crépeau, L., Siméon, M., Ducos, F., and Scott, N. A.: Characterisation of dust aerosols in the infrared from IASI and comparison with PARASOL, MODIS, MISR, CALIOP, and AERONET observations, Atmos. Chem. Phys., 13, 6065–6082, https://doi.org/10.5194/acp-13-6065-2013, 2013.
Pinty, B., Taberner, M., Haemmerle, V.., Paradise, S. R., Vermote, E., Verstraete, M. M., Gobron, N., and Widlowski, J. L.: Global-Scale Comparison of MISR and MODIS Land Surface Albedos, J. Climate, 24, 732–749, 2011.
Platnick, S., Hubanks, P., Meyer, K., and King, M. D.: MODIS Atmosphere L3 Monthly Product (08_L3), NASA MODIS Adaptive Processing System, Goddard Space Flight Center, https://doi.org/10.5067/MODIS/MOD08_M3.061, 2015a.
Platnick, S., Hubanks, P., Meyer, K., and King, M. D.: MODIS Atmosphere L3 Monthly Product, NASA MODIS Adaptive Processing System, Goddard Space Flight Center, USA, https://doi.org/10.5067/MODIS/MYD08_M3.061, 2015b.
Popp, T., de Leeuw, G., Bingen, C., Brühl, C., Capelle, V., Chedin, A., Clarisse, L., Dubovik, O., Grainger, R., Griesfeller, J., Heckel, A., Kinne, S., Klüser, L., Kosmale, M., Kolmonen, P., Lelli, L., Litvinov, P., Mei, L., North, P., Pinnock, S., Povey, A., Robert, C., Schulz, M., Sogacheva, L., Stebel, K., Stein Zweers, D., Thomas, G., Tilstra, L.G., Vandenbussche, S., Veefkind, P., Vountas, M., and Xue, Y.: Development, Production and Evaluation of Aerosol Climate Data Records from European Satellite Observations (Aerosol_cci), Remote Sens., 8, 421, 2016.
Sayer, A. M. and Knobelspiesse, K. D.: How should we aggregate data? Methods accounting for the numerical distributions, with an assessment of aerosol optical depth, Atmos. Chem. Phys., 19, 15023–15048, https://doi.org/10.5194/acp-19-15023-2019, 2019.
Sayer, A. M., Thomas, G. E., and Grainger, R. G.: A sea surface reflectance model for (A)ATSR, and application to aerosol retrievals, Atmos. Meas. Tech., 3, 813–838, https://doi.org/10.5194/amt-3-813-2010, 2010.
Sayer, A. M., Hsu, N. C., Bettenhausen, C., Ahmad, Z., Holben, B. N., Smirnov, A., Thomas, G. E., and Zhang, J.: SeaWiFS Ocean Aerosol Retrieval (SOAR): Algorithm, validation, and comparison with other data sets, J. Geophys. Res., 117, D03206, https://doi.org/10.1029/2011JD016599, 2012a.
Sayer, A. M., Hsu, N. C., Bettenhausen, C., Jeong, M.-J., Holben, B. N., and Zhang, J.: Global and regional evaluation of over-land spectral aerosol optical depth retrievals from SeaWiFS, Atmos. Meas. Tech., 5, 1761–1778, https://doi.org/10.5194/amt-5-1761-2012, 2012b.
Sayer, A. M., Munchak, L. A., Hsu, N. C., Levy, R. C., Bettenhausen, C., and Jeong, M. J.: MODIS Collection 6 aerosol products: Comparison between Aqua's e-Deep Blue, Dark Target, and ”merged” data sets, and usage recommendations, J. Geophys. Res.-Atmos., 119, 13965–13989, https://doi.org/10.1002/2014jd022453, 2014.
Sayer, A. M., Hsu, N. C., Bettenhausen, C., Jeong, M.-J., and Meister, G.: Effect of MODIS Terra radiometric calibration improvements on Collection 6 Deep Blue aerosol products: Validation and Terra/Aqua consistency, J. Geophys. Res.-Atmos., 120, 12157–12174, https://doi.org/10.1002/2015JD023878, 2015.
Sayer, A. M., Hsu, N. C., Lee, J., Carletta, N., Chen, S.-H., and Smirnov, A.: Evaluation of NASA Deep Blue/SOAR aerosol retrieval algorithms applied to AVHRR measurements, J. Geophys. Res.-Atmos., 122, 9945–9967, https://doi.org/10.1002/2017JD026934, 2017.
Sayer, A. M., Hsu, N. C., Lee, J., Bettenhausen, C., Kim, W. V., and Smirnov, A.: Satellite Ocean Aerosol Retrieval (SOAR) algorithm extension to S-NPP VIIRS as part of the “Deep Blue” aerosol project, J. Geophys. Res.-Atmos., 123, 380–400, https://doi.org/10.1002/2017JD027412, 2018a.
Sayer, A. M., Hsu, N. C., Lee, J., Kim, W. V., Dubovik, O., Dutcher, S. T., Huang, D., Litvinov, P., Lyapustin, A., Tackett, J. L., and Winker, D. M.: Validation of SOAR VIIRS over-water aerosol retrievals and context within the global satellite aerosol data record, J. Geophys. Res.-Atmos., 123, 13496–13526, https://doi.org/10.1029/2018JD029465, 2018b.
Sayer, A. M., Hsu, N. C., Lee, J., Kim, W., and Dutcher, S.: Validation, stability, and consistency of MODIS Collection 6.1 and VIIRS Version 1 Deep Blue aerosol data over land, J. Geophys. Res.-Atmos., 124, 4658–4688, https://doi.org/10.1029/2018JD029598, 2019.
Schutgens, N. A. J.: Site representativity of AERONET and GAW remotely sensed AOT and AAOT observations, Atmos. Chem. Phys. Discuss., https://doi.org/10.5194/acp-2019-767, in review, 2019.
Shi, Y., Zhang, J., Reid, J. S., Hyer, E. J., Eck, T. F., Holben, B. N., and Kahn, R. A.: A critical examination of spatial biases between MODIS and MISR aerosol products – application for potential AERONET deployment, Atmos. Meas. Tech., 4, 2823–2836, https://doi.org/10.5194/amt-4-2823-2011, 2011.
Shi, Y. R., Levy, R. C., Eck, T. F., Fisher, B., Mattoo, S., Remer, L. A., Slutsker, I., and Zhang, J.: Characterizing the 2015 Indonesia fire event using modified MODIS aerosol retrievals, Atmos. Chem. Phys., 19, 259–274, https://doi.org/10.5194/acp-19-259-2019, 2019.
Smirnov, A., Holben, B. N., Slutsker, I., Giles, D. M., Mc-Clain, C. R., Eck, T. F., Sakerin, S. M., Macke, A., Croot, P., Zibordi, G., Quinn, P. K., Sciare, J., Kinne, S., Harvey, M., Smyth, T. J., Piketh, S., Zielinski, T., Proshutinsky, A., Goes, J. I., Nelson, N. B., Larouche, P., Radionov, V. F., Goloub, P., Krishna Moorthy, K., Matarrese, R., Robertson, E. J., and Jourdin, F.: Maritime Aerosol Network as a component of Aerosol Robotic Network, J. Geophys. Res.-Atmos., 114, D06204, https://doi.org/10.1029/2008JD011257, 2009.
Sogacheva, L., Kolmonen, P., Virtanen, T. H., Rodriguez, E., Saponaro, G., and de Leeuw, G.: Post-processing to remove residual clouds from aerosol optical depth retrieved using the Advanced Along Track Scanning Radiometer, Atmos. Meas. Tech., 10, 491–505, https://doi.org/10.5194/amt-10-491-2017, 2017.
Sogacheva, L., de Leeuw, G., Rodriguez, E., Kolmonen, P., Georgoulias, A. K., Alexandri, G., Kourtidis, K., Proestakis, E., Marinou, E., Amiridis, V., Xue, Y., and van der A, R. J.: Spatial and seasonal variations of aerosols over China from two decades of multi-satellite observations – Part 1: ATSR (1995–2011) and MODIS C6.1 (2000–2017), Atmos. Chem. Phys., 18, 11389–11407, https://doi.org/10.5194/acp-18-11389-2018, 2018a.
Sogacheva, L., Rodriguez, E., Kolmonen, P., Virtanen, T. H., Saponaro, G., de Leeuw, G., Georgoulias, A. K., Alexandri, G., Kourtidis, K., and van der A, R. J.: Spatial and seasonal variations of aerosols over China from two decades of multi-satellite observations – Part 2: AOD time series for 1995–2017 combined from ATSR ADV and MODIS C6.1 and AOD tendency estimations, Atmos. Chem. Phys., 18, 16631–16652, https://doi.org/10.5194/acp-18-16631-2018, 2018b.
Tang, Q., Bo, Y., and Zhu, Y.: Spatiotemporal fusion of multiple-satellite aerosol optical depth (AOD) products using Bayesian maximum entropy method, J. Geophys. Res.-Atmos., 121, 4034–4048, https://doi.org/10.1002/2015JD024571, 2016.
Thomas, G. E., Carboni, E., Sayer, A. M., Poulsen, C. A., Siddans, R., and Grainger, R. G.: Oxford-RAL Aerosol and Cloud(ORAC): aerosol retrievals from satellite radiometers, in: Satellite Aerosol Remote Sensing over Land, edited by: Kokhanovsky, A. and de Leeuw, G., Springer Praxis Books, Springer, Berlin,Heidelberg, 193–225, https://doi.org/10.1007/978-3-540-69397-0_7, 2009.
Torres, O. O.: OMI/Aura Near UV Aerosol Optical Depth and Single Scattering Albedo 1-orbit L2 Swath 13×24 km V003, Greenbelt, MD, USA, Goddard Earth Sciences Data and Information Services Center (GES DISC), https://doi.org/10.5067/Aura/OMI/DATA2004, 2006.
Torres, O., Bhartia, P. K., Herman, J. R., Ahmad, Z., and Gleason, J.: Derivation of aerosol properties from satellite measurements of backscattered ultraviolet radiation: Theoretical basis, J. Geophys. Res., 103, 17099–17110, https://doi.org/10.1029/98JD00900, 1998.
Torres, O., Bhartia, P. K., Sinyuk, A., Welton, E. J., and Holben, B.: Total Ozone Mapping Spectrometer measurements of aerosol absorption from space: Comparison to SAFARI 2000 ground-based observations, J. Geophys. Res., 110, D10S18, https://doi.org/10.1029/2004JD004611, 2005.
Torres, O., Tanskanen, A., Veihelmann, B., Ahn, C., Braak, R., Bhartia, P. K., Veefkind, P., and Levelt, P.: Aerosols and surface UV products from Ozone Monitoring Instrument observations: An overview, J. Geophys. Res., 112, D24S47, https://doi.org/10.1029/2007JD008809, 2007.
Torres, O., Ahn, C., and Chen, Z.: Improvements to the OMI near-UV aerosol algorithm using A-train CALIOP and AIRS observations, Atmos. Meas. Tech., 6, 3257–3270, https://doi.org/10.5194/amt-6-3257-2013, 2013.
Torres, O., Bhartia, P. K., Jethva, H., and Ahn, C.: Impact of the ozone monitoring instrument row anomaly on the long-term record of aerosol products, Atmos. Meas. Tech., 11, 2701–2715, https://doi.org/10.5194/amt-11-2701-2018, 2018.
Veefkind, J. P., de Leeuw, G., and Durkee, P. A.: Retrieval of aerosol optical depth over land using two-angle view satellite radiometry during TARFOX, Geophys. Res. Lett., 25, 3135–3138, 1998.
Virtanen, T. H., Kolmonen, P., Sogacheva, L., Rodríguez, E., Saponaro, G., and de Leeuw, G.: Collocation mismatch uncertainties in satellite aerosol retrieval validation, Atmos. Meas. Tech., 11, 925–938, https://doi.org/10.5194/amt-11-925-2018, 2018.
Wei, J., Li, Z., Peng, Y., and Sun, L.: MODIS Collection 6.1 aerosol optical depth products over land and ocean: validation and comparison, Atmos. Environ., 201, 428–440, 2019a.
Wei, J., Peng, Y., Mahmood, R., Sun, L., and Guo, J.: Intercomparison in spatial distributions and temporal trends derived from multi-source satellite aerosol products, Atmos. Chem. Phys., 19, 7183–7207, https://doi.org/10.5194/acp-19-7183-2019, 2019b.
Witek, M. L., Garay, M. J., Diner, D. J., Bull, M. A., and Seidel, F. C.: New approach to the retrieval of AOD and its uncertainty from MISR observations over dark water, Atmos. Meas. Tech., 11, 429–439, https://doi.org/10.5194/amt-11-429-2018, 2018.
WMO: Guidelines on the Calculation of Climate Normals, WMO-No.1203, 2017.
Zhao, T. X. P., Chan, P. K., and Heidinger, A. K.: A global survey of the effect of cloud contamination on the aerosol optical thickness and its long-term trend derived from operational AVHRR satellite observations, J. Geophys. Res.-Atmos., 118, 2849–2857, https://doi.org/10.1002/jgrd.50278, 2013.
Zhao, X. and NOAA CDR Program: NOAA Climate Data Record (CDR) of AVHRR Daily and Monthly Aerosol Optical Thickness (AOT) over Global Oceans, Version 3.0, NOAA National Centers for Environmental Information, https://doi.org/10.7289/V5BZ642P, 2017.
Zhao, X. P., Laszlo, I., Guo, W., Heidinger, A., Cao, C., Jelenak, A., Tarpley, D., and Sullivan, J.: Study of long-term trend in aerosol optical thickness observed from operational AVHRR satellite instrument, J. Geophys. Res., 113, D07201, https://doi.org/10.1029/2007JD009061, 2008.
- Abstract
- Introduction
- Regions of interest, instruments and AOD products
- AOD products intercomparison and evaluation with AERONET
- AOD merging approaches
- Merged L3 AOD products
- Merged AOD time series
- Conclusions
- Data availability
- Author contributions
- Competing interests
- Acknowledgements
- Financial support
- Review statement
- References
- Supplement
- Abstract
- Introduction
- Regions of interest, instruments and AOD products
- AOD products intercomparison and evaluation with AERONET
- AOD merging approaches
- Merged L3 AOD products
- Merged AOD time series
- Conclusions
- Data availability
- Author contributions
- Competing interests
- Acknowledgements
- Financial support
- Review statement
- References
- Supplement