the Creative Commons Attribution 4.0 License.
the Creative Commons Attribution 4.0 License.
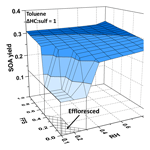
Simulation of SOA formation from the photooxidation of monoalkylbenzenes in the presence of aqueous aerosols containing electrolytes under various NOx levels
Chufan Zhou
Zechen Yu
The formation of secondary organic aerosols (SOAs) from the photooxidation of three monoalkylbenzenes (toluene, ethylbenzene, and n-propylbenzene) in the presence of inorganic seeds (––H2O system) under varying NOx levels has been simulated using the Unified Partitioning Aerosol Phase Reaction (UNIPAR) model. The evolution of the volatility–reactivity distribution (mass-based stoichiometric coefficient, αi) of oxygenated products, which were created by the near-explicit gas kinetic mechanism, was integrated with the model using the parameters linked to the concentrations of HO2 and RO2 radicals. This dynamic distribution was used to estimate the model parameters related to the thermodynamic constants of the products in multiple phases (e.g., the gas phase, organic phase, and inorganic phase) and the reaction rate constants in the aerosol phase. The SOA mass was predicted through the partitioning and aerosol chemistry processes of the oxygenated products in both the organic phase and aqueous solution containing electrolytes, with the assumption of organic–inorganic phase separation. The prediction of the time series SOA mass (12 h), against the aerosol data obtained from an outdoor photochemical smog chamber, was improved by the dynamic αi set compared to the prediction using the fixed αi set. Overall, the effect of an aqueous phase containing electrolytes on SOA yields was more important than that of the NOx level under our simulated conditions or the utilization of the age-driven αi set. Regardless of the NOx conditions, the SOA yields for the three aromatics were significantly higher in the presence of wet electrolytic seeds than those obtained with dry seeds or no seed. When increasing the NOx level, the fraction of organic matter (OM) produced by aqueous reactions to the total OM increased due to the increased formation of relatively volatile organic nitrates and peroxyacyl-nitrate-like products. The predicted partitioning mass fraction increased as the alkyl chain length increased but the organic mass produced via aerosol-phase reactions decreased due to the increased activity coefficient of the organic compounds containing longer alkyl chains. Overall, the lower mass-based SOA yield was seen in the longer alkyl-substituted benzene in both the presence and absence of inorganic-seeded aerosols. However, the difference of mole-based SOA yields of three monoalkylbenzenes becomes small because the highly reactive organic species (i.e., glyoxal) mainly originates from ring opening products without an alkyl side chain. UNIPAR predicted the conversion of hydrophilic, acidic sulfur species to non-electrolytic dialkyl organosulfate (diOS) in the aerosol. Thus, the model predicted the impact of diOS on both hygroscopicity and acidity, which subsequently influenced aerosol growth via aqueous reactions.
- Article
(2376 KB) -
Supplement
(2164 KB) - BibTeX
- EndNote
Anthropogenic volatile organic compounds (VOCs) have significant impacts on urban and regional atmospheric chemistry, despite fewer global emissions compared with biogenic VOCs (McDonald et al., 2018). As an important group of anthropogenic VOCs, aromatic hydrocarbons (HCs) are emitted from automobile exhaust (Zhang et al., 2018) and solvent use (Cheng et al., 2018) and are known to be precursors for secondary organic aerosols (SOAs), which are formed during the process of photooxidation (Seinfeld and Pandis, 2016). In polluted areas (e.g., urban areas in Asia), aromatic HCs occupy 11 % to 25 % of the total nonmethane HC emissions (67.0 Tg in 2010) (Li et al., 2017) and traditionally comprise approximately 15 % of SOA formation (Ait-Helal et al., 2014), which contributes to the urban budget of fine particulate matter (Wood et al., 2010).
SOA formation has attracted substantial interest from scholars because of its vital role in affecting climate change (IPCC, 2015; Seinfeld and Pandis, 2016), urban visibility (Chen et al., 2012; Ren et al., 2018), and health (Requia et al., 2018). The prediction of SOA formation was first fulfilled by a gas–particle partitioning model. The partitioning-based SOA model uses two surrogate products (Odum et al., 1996) or several semivolatile surrogates (e.g., volatility basis set, VBS) (Donahue et al., 2006), with semiempirical parameters (e.g., the product stoichiometric coefficient, α; and gas–particle partitioning coefficient, Kp) for each HC system under a given NOx condition. Due to its simplicity and high efficiency, the partitioning-based model has been widely used in regional and global models. Nonetheless, the models and their predecessors are limited to predict SOAs formed from in-particle chemistry due to the loss of product structures, which govern the reactivity of organic species in the aerosol phase. Overall, regional air quality models have historically underestimated fine particulate matter in summertime (Appel et al., 2017; Huang et al., 2017) due to the lack of in-particle chemistry, particularly in the presence of an aqueous phase containing electrolytes (Ervens et al., 2011; Tsigaridis et al., 2014; Kelly et al., 2018).
A few models have attempted to implement in-particle chemistry into SOA models. For instance, Johnson et al. (2004, 2005) simulated aromatic SOA chamber data, with a modified Kp, to obtain experimentally comparable results, while the delayed simulated SOA mass indicated the occurrence of chemical reactions in the aerosol phase. McNeill et al. (2012) developed the Gas–Aerosol Model for Mechanism Analysis (GAMMA) to predict the formation of SOAs via aqueous-phase chemistry, which was further applied to the production of isoprene SOAs. Im et al. (2014) advanced the Unified Partitioning Aerosol Phase Reaction (UNIPAR) model, which predicted the SOA mass from partitioning processes and aerosol-phase reactions (reactions in both organic and inorganic phases and organosulfate (OS) formation). In that study, toluene and 1,3,5-trimethylbenzene SOAs were modeled using near-explicit products with the organic–inorganic phase separation mode. Beardsley and Jang (2016) extended UNIPAR to simulate isoprene SOAs in the single homogeneously mixed phase (organic–inorganic mixture). Despite the reasonable prediction of SOA masses, UNIPAR faced inaccuracies in predicting time series SOA data due to the use of a fixed (nonage-driven) mass-based stoichiometric coefficient (αi) set.
Age-driven functionalization and fragmentation alter the volatility and reactivity of products and their molecular structures (Donahue et al., 2006; Rudich et al., 2007; Shilling et al., 2007; Hartikainen et al., 2018), which, in turn, varies the in-particle chemistry. Cappa and Wilson (2012) employed tunable parameters to kinetically demonstrate the evolution of SOA mass and the bulk oxygen-to-carbon atomic ratio (O:C ratio) during photochemical aging. However, oligomerization reactions in the aerosol phase were excluded. Donahue et al. (2011) developed a 2D-VBS method, which represented product aging by remapping the volatility and polarity (O : C ratio) of the products in 2-D space. Zhao et al. (2015) reported a discrepancy in the simulated toluene SOAs and α-pinene SOAs within the same 2D-VBS configuration, which may result from the different reactivities of the oxidation products of the precursors in aerosol-phase reactions. In this study, we have attempted to improve the UNIPAR model by using dynamic (age-driven) αi and applying the resulting model to predict the SOA formation of three monoalkylbenzenes (i.e., toluene, ethylbenzene, and n-propylbenzene) under a wide range of environmental conditions (i.e., NOx, temperature, humidity, sunlight, and aerosol acidity). To consider the effect of the aging process on SOA formation, model parameters related to the organic molecular structures (i.e., the molecular weight, MW; and O : C ratio) and the αi set are calculated as the system ages, allowing for the internally dynamic estimation of the activity coefficient of the products (lumping species) in the aqueous phase containing electrolytes. Hence, the model is able to dynamically compute the partitioning coefficient of organics in the inorganic phase (Kin) by reflecting the photochemical evolution of the products in the gas phase and, consequently, improving SOA prediction. Organosulfate (OS), which has been identified in both laboratory and field studies (Hettiyadura et al., 2015; J. Li et al., 2016; Estillore et al., 2016; Chen et al., 2018), is an important chemical species due to its low volatility and ability to modulate the hygroscopicity of sulfate constituents. In the presence of acidic sulfate constituents, UNIPAR also predicts the production of non-electrolytic sulfates (i.e., dialkyl-substituted OS, diOS) and the ensuing modification of aqueous-phase reactions. The feasibility of unified rate constants for aerosol-phase reactions was evaluated by extending the preexisting rate constants, which has been employed for toluene and 1,3,5-trimethylbenzene (Im et al., 2014) and isoprene (Beardsley and Jang, 2016), to the three monoalkylbenzenes in this study.
Table 1Experimental conditions and resulting SOA chamber data from the monoalkylbenzenes photooxidation experiments performed under various NOx conditions with/without inorganic-seeded aerosol in the dual outdoor UF APHOR chambers.
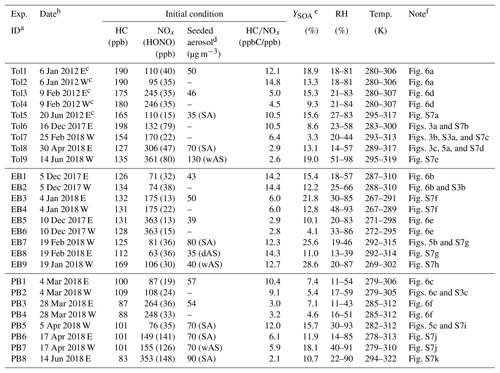
a “Tol”, “EB”, and “PB” represent toluene, ethylbenzene, and n-propylbenzene oxidation experiments, respectively. b “E” or “W” that follows the experiment date represents the east or west chamber for the UF APHOR, respectively. c SOA data obtained from Im et al. (2014). d “SA”, “wAS”, and “dAS” denote directly injected sulfuric-acid-seeded aerosol, wet ammonium-sulfate-seeded aerosol, and dry ammonium-sulfate-seeded aerosol, respectively (dry: RH < ERH; wet: RH > ERH). For those without indication, SO2 (in the unit of ppb) was injected into the chamber to generate sulfuric acidic seeds under the sun light. e SOA yield is estimated using , where ΔOM is formed organic matter and ΔHC is consumed HC. Yield in the table was estimated where SOA mass reached to the maximum over the course of the experiments. f This column denotes in which figures the corresponding data were used. The accuracy of relative humidity (RH) is 5 %. The accuracy of temperature is 0.5 K.
The SOA formation from the photooxidation of monoalkylbenzenes was conducted in the University of Florida Atmospheric PHotochemical Outdoor Reactor (UF APHOR) (Table 1). The concentrations of HCs, trace gasses (Ox, SO2, and O3), inorganic ions, aerosol acidity, and organic carbon (OC) of particles were monitored, as were the meteorological factors (i.e., relative humidity; temperature; and ultraviolet, UV, radiation). The configurations of the chamber and instrumentations were described by Im et al. (2014), J. Li et al. (2016), Beardsley and Jang (2016), Yu et al. (2017), and Jiang et al. (2017). Aerosol acidity ([H+], mol L−1 of aerosol) is monitored using colorimetry integrated with the reflectance UV–visible spectrometer (C-RUV) technique (Li et al., 2015) (Sect. S1 in the Supplement). The diOS concentration (µmol m−3) in an aerosol is estimated by the difference [H+] obtained from ion chromatography (IC) interfaced with a particle-into-liquid sampler (PILS) (Li et al., 2015) and C-RUV method. Each HC was studied under at least two NOx levels (high NOx: HC∕NOx < 5.5; low NOx: HC∕NOx > 5.5) with or without inorganic-seeded aerosols (i.e., sulfuric acid, SA; or ammonium sulfate, AS). HONO was added into the system as a reaction initiator. To investigate the effect of the liquid water content (LWC) on AS-seeded SOA, two RH conditions were applied: (1) dry: RH < efflorescence RH (ERH) of the AS seed; (2) wet: RH > 50 % to prevent crystallization of AS seed. The ratio of organic matter (OM) to OC was experimentally determined to be 1.9 (Table 1, EB4), which was similar to the reported value of 2.0 for a series of toluene–NOx oxidation study (Kleindienst et al., 2007).
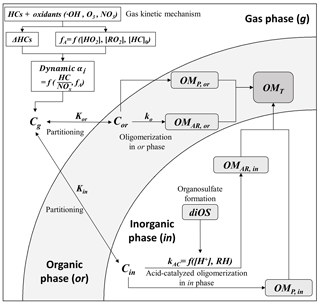
Figure 1Simplified scheme of the UNIPAR model. [HC]0 represent the initial hydrocarbon (HC) concentration. The dynamic mass-based stoichiometric coefficient (dynamic αi), the consumption of HCs (ΔHCs), the concentration of hydroperoxide radical ([HO2]), and the concentration of organic peroxyl radical ([RO2]) are simulated from the gas kinetic mechanism (MCM v3.3.1). The aging scale factor (fA) is represented as a function of [HO2], [RO2], and [HC]0, which is detailed in Sect. 3.1. C and K denote the concentration and the partitioning coefficient of organic compounds, respectively, in gas phase (g), organic phase (or), and inorganic phase (in). kor,i denotes the reaction rate constant of oligomerization of organic compounds in the organic phase. kAC,i denotes the reaction rate constant of acid-catalyzed oligomerization of organic compounds in the inorganic phase and is determined as a function of aerosol acidity ([H+]) and ambient humidity (RH). “OM” represents the concentration of organic matter. Subscripts “AR”, “P”, and “T” indicate OM formed from aerosol-phase reactions, OM formed from the partitioning process, and total OM, respectively. Subscript i represents each lumping species. diOS represents the concentration of organosulfate – dialkyl sulfate (diOS) in this study.
The structure of the UNIPAR model is illustrated in Fig. 1. The simulation of aromatic SOA formation in the aqueous phase containing electrolytes was performed under the assumption of complete organic–inorganic phase separation. Bertram et al. (2011) modeled the separation RH (SRH) in the liquid–liquid phase of the mixture of organic and AS using the bulk O : C ratio. When ambient RH < SRH, the system undergoes organic–inorganic phase separation. The reported O : C ratios of the toluene, ethylbenzene, and n-propylbenzene SOAs were 0.62 (Sato et al., 2012), 0.55 (Sato et al., 2012), and 0.45 (L. J. Li et al., 2016), respectively, which caused the corresponding SRH values to be 65 %, 80 %, and 93 %, respectively. Most RH for active photooxidation of HCs under ambient sunlight were under 65 %, which supported the assumption of organic–inorganic phase separation. In addition, as less soluble oligomers formed in the aerosol phase, an SRH higher than 65 % was more likely to be yielded.
3.1 Atmospheric evolution of lumping species
The gas-phase oxidation of HCs is simulated using the near-explicit gas-phase chemistry mechanism (Master Chemical Mechanism, MCM, v3.3.1) (Jenkin et al., 2012) integrated with the Morpho chemical solver (Jeffries et al., 1998). To represent the polluted urban and clean environments, the gas-phase oxidation is simulated under various NOx levels (HCppbC∕NOxppb = 2–14) for given meteorological conditions (e.g., sunlight, temperature, and RH on 14 June 2018 near the summer solstice, with a clear sky in Gainesville, Florida). The resulting oxygenated products are lumped into 51 species within a 2-D set with eight levels of volatility (1–8: 10−8, 10−6, 10−5, 10−4, 10−3, 10−2, 10−1, and 1 mm Hg) and six levels of aerosol-phase reactivity (very fast: VF, fast: F, medium: M, slow: S, partitioning only: P, and multi-alcohol: MA) plus three additional reactive species (glyoxal, GLY; methylglyoxal, MGLY; and epoxydiols, IEPOX, isoprene products) with their own vapor pressure. The detailed lumping criteria and αi equations are described in Sect. S2 along with the major product structures (Tables S1–S3 in the Supplement).
To simulate age-dependent SOA formation, αi is reconstructed over time by a weighted average method using a pair of gas-phase oxidation compositions with different aging statuses: fresh composition and highly oxidized composition. The weighting factor at time = t is related to an aging scale factor (fA(t)), which is defined as
where [RO2] and [HO2] represent the concentrations (ppb) of RO2 and HO2 radicals, respectively, and [HC]0 represents the initial HC concentration (ppbC). The lower boundary of fA(t) (t = fresh) to determine the fresh αi set is equal to −7.2 at HC∕NOx = 2 (high NOx levels) and −3.7 at HC∕NOx = 14 (low NOx levels) for all three HCs. The upper boundary of fA(t) (t = highly aged) to determine the highly aged αi set is equal to −5.2 and −2.9 under the same high and low NOx levels, respectively. Both the fresh αi and highly aged αi are functions of HC∕NOx. fA(t) is further converted into a fractional aging scale ( ) ranging from 0 (fresh composition) to 1 (highly aged composition) using a weight average method
at each NOx level. Then, αi is dynamically reconstructed based on under varying NOx conditions.
The molecular structures, including O : Ci, MW (MWi), and hydrogen bonding (HBi) parameters, of each species (i) are also dynamically represented by a similar method, as shown in Sects. S3 and S4.
3.2 SOA formation: partitioning
The partitioning coefficient (KP) from the gas (g) phase to the organic (or) phase (Kor,i, m3 µg−1) and from the g phase to the inorganic (in) phase (Kin,i, m3 µg−1) of each species is estimated using the following gas–particle absorption model (Pankow, 1994).
where R represents the gas constant (8.314 J mol−1 K−1). T represents the ambient temperature (K). MWor and MWin represent the average MW (g mol−1) of organic and inorganic aerosols, respectively. represents the subcooled liquid vapor pressure (mm Hg) of a species, i. In the organic phase, we assume that the activity coefficient (γor,i) of a species (i) is unity (Jang and Kamens, 1998). In the inorganic phase, γin,i is semi-empirically predicted by a regression equation, which was fit the theoretical activity coefficients of various organic compounds to RH, fractional sulfate (FS), and molecular structures (i.e., MWi, O : Ci, and HBi). FS is a numerical indicator for inorganic compositions related to aerosol acidity , where [] and [] are the concentration of the total sulfate and the total ammonium, respectively). The theoretical activity coefficients were estimated at a given humidity and an aerosol composition through a thermodynamic model (Aerosol Inorganic-Organic Mixtures Functional Groups Activity Coefficients, AIOMFAC) (Zuend et al., 2011).
The statistical information for Eq. (4) is shown in Sect. S4 and Fig. S1 in the Supplement. The resulting Kor,i and Kin,i are employed to calculate the concentration (µg m−3) of the lumping species in multiple phases (Cg,i, Cor,i, Cin,i, and ).
Schell et al. (2001) developed a partitioning model to predict SOA formation. This model was reconstructed by Cao and Jang (2010) to include OM formed via aerosol-phase reactions (OMAR,i) for a species (i), which is estimated in Sect. 3.3. OM formed during the partitioning process (OMP) is estimated by utilizing the mass balance shown in the following equation.
() is the effective saturation concentration and OM0 represents the concentration (mol m−3) of the preexisting OM. MWoli,i represents the average MW of oligomeric products. Equation (5) is solved via iterations using the globally converging Newton–Raphson method (Press et al., 1992).
3.3 SOA formation: aerosol-phase reactions
The formation of OMAR,i is processed in both the organic and inorganic phases: oligomerization in the organic phase to form OM and oligomerization in the inorganic phase to form OM based on the assumption of a self-dimerization reaction (i.e., second-order reaction) (Odian, 2004) for organic compounds in media. Oligomerization in an aqueous phase can be accelerated under acidic environment (Jang et al., 2002). The oligomerization rate constants (L mol−1 s−1) in the organic phase and inorganic phase are ko,i and kAC,i, respectively, and the kinetic equations for oligomerizations are written as follows.
The bracketed terms in the equations indicate the conversion factors from aerosol-based concentrations ( and : mol L−1) into air-based concentrations (µg m−3). The detailed derivations are shown in Sect. S5 and are illustrated in Fig. S2. ρor and ρin represent the density of the aerosol of organic and inorganic aerosol. ρor was experimentally determined (EB4 in Table 1) to be 1.38 g cm−3, which was similar to the reported value of 1.4 g cm−3 for aromatic SOA (Nakao et al., 2011; Chen et al., 2017; Ng et al., 2007). ρin is obtained from a regression equation through the extended aerosol inorganic model (E-AIM) (Clegg et al., 1998). Due to atmospheric diurnal patterns (high RH at nighttime to low humidity during daytime), it is likely that the RH changes would be based on inorganic aerosol ERH. UNIPAR internally predicts the ERH using the equation derived by Colberg et al. (2003).
kAC,i in Eq. (7) is estimated based on a semiempirical model developed by Jang et al. (2005) as a function of species reactivity (Ri), protonation equilibrium constant (), excess acidity (X), water activity (aw), and proton concentration ([H+]), which are estimated by the E-AIM.
In the organic phase, ko,i is estimated by excluding the X and aw [H+] terms. The formed OMAR can be calculated as a sum of OM and OM for each species assuming that OMAR is irreversibly formed and nonvolatile (Kleindienst et al., 2006; Cao and Jang, 2010).
3.4 Organosulfate formation
In the presence of aqueous acidic sulfate, UNIPAR predicts the formation of diOS ([diOS]model) to compute the change in aerosol hygroscopicity and acidity. At each time step, free electrolytic sulfate ([]free), which is the sulfate that is unassociated with ammonium ([]), is represented as ([] − 0.5 []). []free is then applied to the semiempirical equation tested previously for several SOA systems (Im et al., 2014; Beardsley and Jang, 2016) to predict [diOS]model, as described below:
where fdiOS represents the diOS conversion factor introduced by Im et al. (2014), which was semi-empirically determined to be 0.071 in this study. NdiOS represents the numeric parameter for scaling lumping groups based on the effectiveness of the chemical species to form diOS. For example, the diOS scale factor is 1 for each alcohol and aldehyde group and 2 for each epoxide group (see Tables S1–S3 for functional groups). Then, NdiOS is summed at each time step and applied to Eq. (9).
3.5 Operation of the UNIPAR model
The variables, which include HC consumption (ΔHC), [HO2], [RO2], HC∕NOx, RH, temperature, and the inorganic concentration (i.e., Δ[] and Δ[]), were input to the UNIPAR model every 6 min (Δt=6 min).
4.1 Prediction of SOA mass under the evolution of oxygenated products
As reported in former studies, the kinetic mechanism tends to underestimate the decay of aromatic HCs because of the low prediction of OH radicals (Johnson et al., 2005; Bloss et al., 2005). In this study, the addition of artificial OH radicals varies with the HC∕NOx ratio by fitting the predicted decay of HCs using the kinetic mechanism in the experimental measurements. The time profiles of the decays of the three HCs are shown in Fig. S3 (Sect. S6). When the NOx level is very low, the maximum additional OH radical production rate for monoalkylbenzenes is 2×108 molecules cm3 s−1, which is less than 4×108 (Bloss et al., 2005) but similar to the value reported by Im et al. (2014). When HC∕NOx < 3, no addition of artificial OH radicals is needed for the chamber simulation of the decay of monoalkylbenzenes. For the make-up OH production rate constants of all three HCs under varying NOx, the mathematical weighting equation is written below:
In our model, we assume that the oxidation of products progresses in the gas phase. Lambe et al. (2012) reported that the transition point of n-C10 SOAs from a functionalization-dominant regime to a fragmentation-dominant regime is approximately 3 d (photochemical equivalent age under an atmospheric OH exposure of 1.5×106 molecules cm−3). Under this criterion, we exclude the aging of nonvolatile aerosol products (OMAR). However, the oxidation of aerosol products for longer periods of time may decrease the volatility (George and Abbatt, 2010; Jimenez et al., 2009).
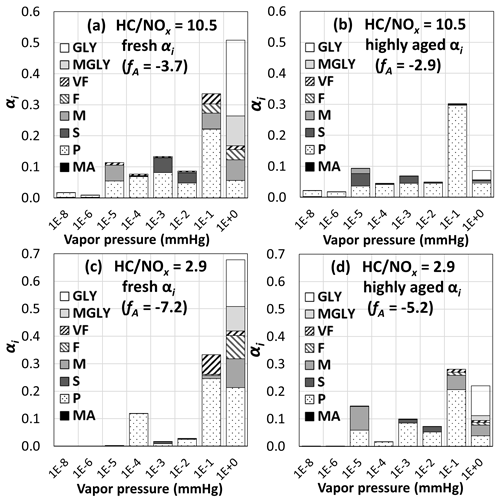
Figure 2The mass-based stoichiometric coefficients (αi) of each lumping species, i, from toluene oxidation (i) under low NOx level (simulation based on the sunlight of Exp. Tol6, HC∕NOx = 10.5, 16 December 2018) at (a) fresh condition and (b) highly aged condition and (ii) under high NOx level (simulation based on the sunlight of Exp. Tol8, HC∕NOx = 2.9, 30 April 2018) at (c) fresh condition and (d) highly aged condition, where fA is the aging scale factor as derived in Eq. (1) in Sect. 3.1. The oxygenated products predicted by the explicit gas kinetic model are lumped as a function of vapor pressure (eight groups: 10−8, 10−6, 10−5, 10−4, 10−3, 10−2, 10−1, and 1 mm Hg) and aerosol-phase reactivity (six groups), i.e., very fast (VF: tricarbonyls and α-hydroxybicarbonyls), fast (F: two epoxides or aldehydes), medium (M: one epoxide or aldehyde), slow (S: ketones), partitioning only (P), and multialcohol (MA). MGLY (methylglyoxal) and GLY (glyoxal) were lumped separately due to the relatively high reactivity.
Figure 2 illustrates the evolution of the volatility–reactivity-based distribution of the mass-based stoichiometric coefficient (αi) of toluene at the two different NOx levels (HC∕NOx = 2.9 and 10.5). Collectively, most αi values at both NOx levels tend to decline as the reaction time lapses (Fig. 2a vs. Fig. 2b; Fig. 2c vs. Fig. 2d) since the evolution of some semivolatile organic compounds (SVOCs) forms very volatile molecules (i.e., CO2, formic acid, and formaldehyde). For example, the αi values of highly reactive carbonyls with high volatility (GLY and MGLY in Table S1 of Sect. S7) are high under the fresh condition and significantly decline as the system ages, because they undergo fast photolysis under sunlight (George et al., 2015; Henry and Donahue, 2012). Consequently, the decay of these highly reactive species leads to the decrease in the production of OMAR. The high NOx level delays the oxidation of gas-phase products. Similar trends in the αi set can be found for ethylbenzene and n-propylbenzene, as shown in Sect. S7 (Tables. S2 and S3, Figs. S4 and S5). The αi of highly reactive species (e.g., GLY, 8VF, 3M, and 5S) decreases by increasing the NOx level due to the suppression of the HOx cycle via the reaction of NO2 with OH radicals. As seen in Fig. 2d, some medium reactivity species – i.e., 2-methyl-4-oxo-3-nitro-2-butenoic acid (3M), 2-methyl-4-oxo-2-butenoic acid (6M), and acetyl-3-oxopropanoate (7M) – start to form as NOx decreases.
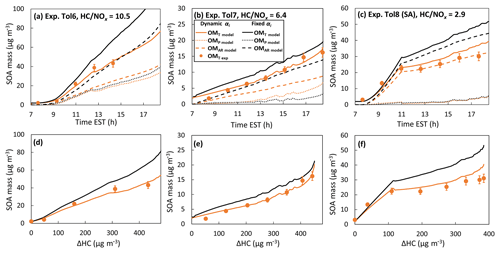
Figure 3Comparison between simulated SOA mass using the fixed αi (αi is obtained when precursor being consumed make up half of the total simulated consumption) and dynamic αi (αi evolving as photooxidation) under (a) low-NOx condition (Exp. Tol6, HC∕NOx = 10.5), (b) moderate-NOx condition (Exp. Tol7, HC∕NOx = 6.4), and (c) high-NOx condition (Exp. Tol8, HC∕NOx = 2.9 with sulfuric acid (SA)-seeded aerosol). (d, e, f) represent the time-dependent SOA growth curve (SOA mass concentration against consumed HC in the unit of µg m−3) corresponding to the experimental conditions of (a, b, c), respectively. The solid circle represents the experimental measurements. The SOA mass is corrected for particle loss to the chamber wall. The experimental conditions are available in Table 1.
In Fig. 3, the comparison between the simulations of SOA formation from toluene oxidation is based on two different αi-reconstruction strategies: dynamic αi and fixed αi. A clear improvement in the prediction of SOA formation is demonstrated when comparing the SOA mass using dynamic αi to that using fixed αi. The aged SOA growth from the three systems (i.e., low NOx level, Fig. 3a and d; moderate NOx level, Fig. 3b and e; and high NOx level with an inorganic seed, Fig. 3c and f) is even smaller than that predicted with the less-aged αi set, which is obtained when precursors being consumed make up half of the total simulated consumption. Our model simulation against the chamber data suggests that while aging may alter aerosol compositions (Fig. 2), it does not always increase SOA yields. Traditionally, the SOA mass has been predicted using fixed thermodynamic parameters (i.e., Kp and αi), which is inadequate when reflecting upon practical scenarios, where oxygenated product distributions vary dynamically with oxidation.
4.2 Effects of aerosol acidity and LWC on SOA formation
In the model, aerosol acidity was expressed using a fractional free sulfate (FFS), which is defined as FFS = ([] − 0.5[])/[]. Humidity can influence both aerosol acidity and LWC, which are the model parameters in UNIPAR. Thus, UNIPAR has the capability to decouple the effect of aerosol acidity and humidity, as shown in Fig. 4 for toluene SOA. The impact of aerosol acidity and humidity on the yields of SOAs derived from ethylbenzene and n-propylbenzene is illustrated in Fig. S6 (Sect. S8). The dramatic difference in SOA yields appears between the RH above ERH and the RH below ERH. The LWC disappears below ERH, and there are no aqueous reactions. For example, the observed SOA yield of ethylbenzene with effloresced AS was significantly smaller than that with wet AS: 11 % (EB8 in Table 1) vs. 30 % (EB9 in Table 1). Kamens et al. (2011) and Liu et al. (2018) reported a significantly lower yield of toluene SOA for dry AS-seeded aerosols compared with its wet counterpart. The partitioning of polar carbonaceous products increases with increasing LWC and, thus, aqueous reactions. In the presence of wet aerosols, SOA yields gradually increase with increasing FFS (increasing acidity) at a given RH due to acid-catalyzed oligomerization. The oxygenated products of toluene are relatively more polar than those of ethylbenzene or propylbenzene and positively attributed to the increase in OMAR.
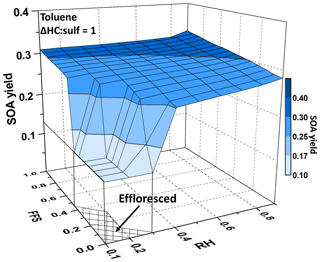
Figure 4Simulated toluene SOA yields (YSOA=ΔOM∕ΔHC at the end of the simulation, where ΔOM is formed organic matter and ΔHC is consumed HC) as a function of relative humidity (RH: 0.1–0.9) and fractional free sulfate (FFS: 0–1), where FFS = ([] − 0.5[])/[], which is another numerical indicator that is used to estimate aerosol acidity ([H+]) in the inorganic thermodynamic model. The RH and FFS are fixed in the simulations. The gas-phase simulations are based on the experimental condition of 14 June 2018 (Exp. Tol9 in Table 1) (initial HC concentration = 5 ppb, HC∕NOx = 2, preexisting OM (OM0) mass concentration = 2 µg m−3, sulfate mass concentration = 20 µg m−3, and the mass ratio of the consumed HC to sulfate (ΔHC : sulf) = 1).
Compared to isoprene SOAs reported by Beardsley and Jang (2016), the impacts of humidity and acidity on the SOA yields of monoalkylbenzenes in this study are relatively weaker above the ERH (Fig. 4), except for the highly acidic conditions under high humidity. In this study, aromatic SOA mass is attributed to a few highly reactive species, such as GLY. Other aromatic oxidation products partitioned in the aerosol phase have moderate solubility and they are slow to react in the aqueous phase. Isoprene products are more hygroscopic than aromatic products and even mixable with an aqueous phase containing electrolytes. The reactions of medium reactivity polar products that formed during isoprene oxidation can be accelerated by an acid catalyst with higher sensitivities to acidity and humidity.
4.3 Organosulfate: simulation vs. measurement
Figure 5 illustrates the time profiles of the predicted concentrations of diOS ([diOS]model) and protons ([H+]) with the measured concentrations of diOS ([diOS]exp), [], and [] for different aromatic HCs under given experimental conditions (Fig. 5a–c). For the three SA-seeded SOA experiments, the fractions of diOS to the total sulfate amount are 0.09, 0.15, and 0.06 for toluene (Exp. Tol8, HC∕NOx = 2.9, FS changing from 0.64 to 0.39), ethylbenzene (Exp. EB7, HC∕NOx = 12.3, FS changing from 0.82 to 0.46), and n-propylbenzene (Exp. PB5, HC∕NOx = 14.4, FS changing from 0.76 to 0.38), respectively. The [diOS]model reasonably agrees with [diOS]exp. The aerosols in Exp. Tol8 and Exp. PB5 show the cessation in diOS formation at approximately 10:00 EST since they became effloresced due to the neutralization of SA with ammonia under the reduction in humidity during the daytime. The diOS fraction in Exp. EB7, which contained wet acidic aerosols, was higher than those in Exp. Tol8 and Exp. PB5, indicating that the acidic condition was favorable for the formation of diOS (Surratt et al., 2010; Lin et al., 2013).
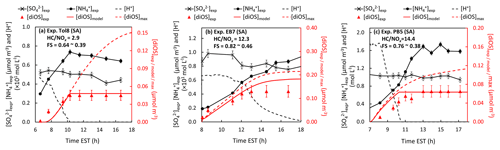
Figure 5Time profiles of measured inorganic sulfate concentration ([]exp), ammonium concentration ([]exp), diOS concentration ([diOS]exp), the predicted proton concentration ([H+]), diOS concentration ([diOS]model), and the maximum diOS concentration ([diOS]max) (assuming there is no ammonia neutralization in the system) for SOA generated from (a) toluene (Exp. Tol8, HC∕NOx = 2.9, OM-to-sulfate mass ratio (OM : sulf) = 1.4), (b) ethylbenzene (Exp. EB7, HC∕NOx = 12.3, OM : sulf = 1.4), and (c) n-propylbenzene (Exp. PB5, HC∕NOx = 14.4, OM : sulf = 0.7). The degree of neutralization is indicated by FS, ranging from 1 (for sulfuric acid) to 0.33 (for ammonium sulfate). “SA” stands for experiment with direct-injection sulfuric-acid-seeded aerosols. The ions and diOS concentrations were corrected for the particle loss to the chamber wall. The experimental conditions are available in Table 1.
Beardsley and Jang (2016) reported that the diOS fraction for isoprene SOAs was 0.26 (HC∕NOx = 32.5, FS changing from 0.69 to 0.47), which was more than that for toluene SOAs, indicating that the oxidation products of isoprene may contain more reactive species to form diOS. For example, IEPOX products in isoprene SOAs are known to be reactive to SA (Budisulistiorini et al., 2017). Additionally, isoprene aerosol products are mixable with aqueous solutions containing electrolytes, and they can more effectively form diOS compared to the aromatic products in liquid–liquid phase separation.
To estimate the potential upper boundary of the concentration of diOS ([diOS]max) in monoalkylbenzene SOA, the aerosol composition was predicted by the model in the presence of SA aerosols (without neutralization with ammonia) under the given experimental conditions shown in Fig. 5. The resulting diOS fractions were 0.29 (OM-to-sulfate mass ratio (OM : sulf) = 1.4), 0.25 (OM : sulf = 1.4), and 0.12 (OM : sulf = 0.7) for toluene, ethylbenzene, and n-propylbenzene, respectively. The aerosol acidity of the ambient aerosol is generally lower than ammonium hydrogen sulfate (AHS), and, thus, the diOS fraction in ambient air would be much lower than the estimated upper boundary. Figure 5 suggests that the change in both aerosol acidity and hygroscopicity by the formation of non-electrolytic sulfate is important to predict SOA mass.
4.4 Effect of NOx on SOA formation in the presence of an aqueous phase containing electrolytes
Figure 6 shows the impact of NOx on the three aromatic SOAs in this study by producing SOAs at two different NOx levels in the presence and absence of SO2. Overall, regardless of the inorganic seed conditions, both the chamber observation and model simulation suggest that increasing the NOx level leads to the decreased formation of SOAs. This trend in the absence of inorganic seed aerosols has also been observed multiple times (Li et al., 2015; Ng et al., 2007; Song et al., 2005). By increasing [NOx], the path of an RO2 radical progresses to the formation of organonitrate and peroxyacyl nitrate (PAN) products, which are less reactive to aerosol-phase reactions. They are relatively volatile and, thus, insignificantly attributed to partitioning SOA mass.
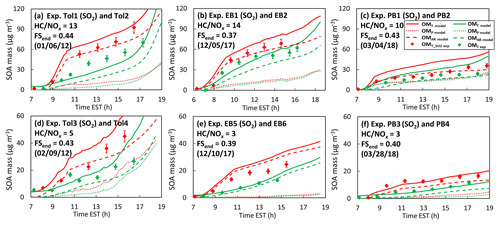
Figure 6Time profiles of measured and modeled SOA mass concentrations (µg m−3) for toluene, ethylbenzene, and n-propylbenzene SOA under low-NOx (a–c)/high-NOx (d–f) conditions in the presence (red color)/absence (green color) of SO2-derived sulfuric-acid-seeded aerosol. Solid, dashed, and dotted lines denote the total organic matter (OMT), the OM from partitioning only (OMP), and the OM from the aerosol-phase reactions (OMAR), respectively. The degree of ammonia neutralization with sulfuric acid is indicated by the FSend, which is the FS at the end of the experimental run. The FSend ranges from 1 (for sulfuric acid) to 0.33 (for ammonium sulfate). The uncertainty associated with experimentally measured OM is about 9 %. The SOA mass was corrected for the particle loss to the chamber wall. The experimental conditions are available in Table 1.
For example, the SOA yields under the low NOx level (HC(ppbC)∕NOx(ppb) = 9.1–14.8, Table 1) in the presence of SO2, with a similar degree of ammonia titration (i.e., similar FS values by the end of the experiments), were higher than those without seeded aerosols: 42 % for toluene (Exp. Tol1, FS = 0.44), 26 % for ethylbenzene (Exp. EB1, FS = 0.37), and 66 % for propylbenzene (Exp. PB1, FS = 0.43). The impact of aerosol acidity was even greater for SOAs produced under a high NOx level (HC(ppbC)∕NOx(ppb) = 2.8–5.0): 65 % for toluene (Exp. Tol3, FS = 0.43), 146 % for ethylbenzene (Exp. EB5, FS = 0.39), and 77 % for propylbenzene (Exp. PB3, FS = 0.40). SOA formation under high-NOx conditions is generally more sensitive to aerosol acidity compared to that at low NOx levels (Fig. 6a–c vs. Fig. 6d–f). The fractions of medium reactivity products are relatively high in high NOx levels, and their reactions in aerosol phase can be accelerated by an acid catalyst. Figure S7 (Sect. S9) also illustrates the simulation of SOA mass against chamber data under varying humidity, NOx levels, and aerosol acidity (Table 1).
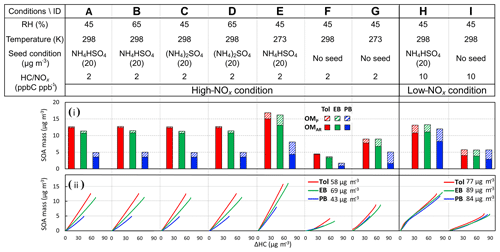
Figure 7The simulated SOA mass (i) for toluene (Tol), ethylbenzene (EB), and n-propylbenzene (PB) under different conditions. The simulation conditions are listed in the top table. The initial concentrations of monoalkylbenzenes, preexisting OM (OM0), NH4HSO4 (AHS)-seeded aerosol, and (NH4)2SO4 (AS)-seeded aerosol are 20 ppb, 2, 20, and 20 µg m−3, respectively. The gas-phase simulation used the sunlight on 14 June 2018 (Exp. Tol9 in Table 1). OMP (diagonal stripes fill) and OMAR (solid fill) represent the organic matter from the partitioning process and aerosol-phase reactions. (ii) shows the time-dependent SOA growth curve for three monoalkylbenzenes under corresponding simulation conditions (top table). The concentrations that follow the legends refer to the mass concentrations of the consumed monoalkyl-substituted benzenes in each simulation under the high or low-NOx conditions.
4.5 Sensitivity of SOA formation to humidity, temperature, aerosol acidity, precursor HCs, and NOx level
Figure 7 illustrates the sensitivity of the SOA mass simulated at relatively low concentration of HCs (20 ppb) (panel i) for three monoalkylbenzenes to important variables – i.e., humidity (Ai vs. Bi for AHS and Ci vs. Di for AS), temperature (Ai vs. Ei for AHS and Fi vs. Gi without inorganic aerosol), aerosol acidity (Ai vs. Ci at RH = 45 % and Bi vs. Di at RH = 65 %), and NOx levels (Ai vs. Hi with AHS-seeded aerosols and Fi vs. Ii without inorganic-seeded aerosols). The most drastic change appears by changing the temperature from 298 K (Ai) to 273 K (Ei). The SOA yield is known to increase by 20 %–150 %, which results from a 10 K decrease in temperature (Sheehan and Bowman, 2001). For all SOAs, noticeable changes are shown between the absence (Fi) and presence (Ai) of wet inorganic seeds, while a minor change appears between wet AHS (Ai) and wet AS (Ci). Within the wet acidic aerosols (Ai vs. Bi and Ci vs. Di), the effect of RH is insignificant in our simulation, as discussed in Sect. 4.2. Although the impact of NOx (Ai vs. Hi and Fi vs. Ii) is less than that of temperature and inorganic seeds, SOA yields are still significantly altered, as discussed in Sect. 4.4.
The panel ii series in Fig. 7 illustrates SOA growth curves under various conditions shown in panel i. Overall, the simulated SOA yields (slopes) increase with a decreased alkyl chain length (toluene > ethylbenzene > n-propylbenzene), which is consistent with our chamber observations (Table 1). Although the decrease in the vapor pressure of products benefits the increases in OMP as the alkyl chain length increases, the increase in the activity coefficient of the organic products containing longer alkyl chains in aqueous phase is unfavorable to the formation of OMAR via aqueous reactions. However, the difference of mole-based SOA yields of three monoalkylbenzenes becomes small because the highly reactive organic species (i.e., glyoxal), which are produced through ring opening reactions without an alkyl side chain, are significantly attributed to OMAR. Fig. 7ii confirms that the effect of an aqueous phase containing electrolytes on SOA yields is more critical than that of the NOx level under our simulated conditions.
4.6 Sensitivity of model prediction to major variables and model uncertainty
To determine the model sensitivity to these parameters, simulations (Exp. Tol9 in Table 1) were performed by increasing/decreasing vapor pressure (Vp), the enthalpy of vaporization (Hvap), γin,i, and kAC,i by factors of 1.5, 1.1, 2, and 2, respectively. The corresponding changes in the SOA mass are −7.2 %/6.8 %, −1.9 %/1.7 %, −8.8 %/4.7 %, and 2.5 %/−3.5 %, respectively. The change in SOA mass from the reference for each simulation is shown in Fig. S8 (Sect. S10).
The uncertainty associated with the group contribution method used for Vp estimation is a factor of 1.45 (Zhao et al., 1999). Hvap has a reported error of 2.6 % (Kolska et al., 2005). γin,i is estimated as a function of O : C, MW, RH, and FS (Eq. ). kAC,i is semi-empirically calculated based on [H+], LWC, and species reactivity (Eq. 8). The E-AIM is performed to estimate the LWC, which is reliable and based on a broadly used water activity dataset (Zhang et al., 2000). Yet, the inorganic thermodynamic models including E-AIM performed inadequately in the prediction of [H+] under low-RH and ammonia-rich conditions (FS < 0.55) (Li and Jang, 2012).
Although most identified toluene products have been included, such as methyl-cyclohexene (3S), 2-methyl-5-nitrophenol (5P), 2-methyl-benzoquinone (6S), 2-methyl-4-oxo-2-butenoic acid (6M), o-cresol (7P), 3-hydroxy-1,3-propandial (7VF), 3-methyl-2(5H)-furanone (8P), MGLY, and GLY (Forstner et al., 1997; Jang and Kamens, 2001; Sato et al., 2007; Gomez Alvarez et al., 2007; Huang et al., 2016), a large number of toluene oxidation mechanisms and involved products remain unstudied. A similar trend can be found in ethylbenzene and propylbenzene. Evidently, the addition of artificial OH radicals in the gas-phase simulation suggests missing mechanisms in the MCM v3.3.1 or an improper branching ratio of reactions. Additionally, the diverse reactions of the RO2 radicals might be oversimplified in the gas-phase simulation by employing surrogate coefficients.
In the model, non-electrolytic diOS was predicted and applied to the prediction of LWC and [H+], which subsequently affect aerosol growth via aqueous reactions. Typically, the monoalkyl sulfate is identified as a product of the esterification of SA with reactive species (Hettiyadura et al., 2015; J. Li et al., 2016; Estillore et al., 2016; Chen et al., 2018). It is possible that monoalkyl sulfates can influence LWC and aerosol acidity differently from sulfuric acid, although they are strongly acidic and hygroscopic. Although Noziere et al. (2010) reported that OS could be produced by the reactions of GLY and sulfate radicals in the presence of aqueous AS under UV light, the amounts of formed monoalkyl OS and their influence on aerosol hygroscopicity are still not clear.
Some other factors in recent investigations, such as organic vapor wall loss and aerosol viscosity, have not been accounted for by the UNIPAR model. The loss of organic vapor to the Teflon chamber wall can compete with the gas–particle partitioning process and the reactions in both the gas phase and aerosol phase to initiate a negative bias in the experimental measurements (Zhang et al., 2014; Mcvay et al., 2014). The modeling of the gas–wall process of semivolatile organic compounds can improve the prediction of SOA mass in regional scales. In addition, an increased aerosol viscosity via aging could modify the diffusivity of the partitioned organic molecules (Abramson et al., 2013) and the reaction rate constant for oligomerization in the aerosol phase.
Despite numerous studies in SOA characterization and formation mechanisms, substantial biases between the simulated and field-measured SOA mass were still found (Hodzic et al., 2016) due to the inadequacy of handling the dynamic multigenerational aging (Jathar et al., 2016) and aqueous reactions of the oxygenated products in the presence of an aqueous phase containing electrolytes (Ervens et al., 2011). In this study, the UNIPAR model addressed those issues using a dynamic age-driven αi set, multiphase partitioning of organic compounds, and in-particle chemistry. Although the utilization of the age-driven αi set improves the time series prediction of SOA mass, as shown in Fig. 3, the photochemical evolution of the gas-phase products via monoalkylbenzene oxidation (Figs. 2, S4, and S5) does not increase the SOA mass, as is commonly suggested. Overall, the effect of an aqueous phase containing electrolytes on SOA formation was more critical than that of the NOx level under our simulated conditions. By adding a wet inorganic seed to the non-seed SOA system, the mass-based SOA yields under high NOx levels increase more than those under low-NOx conditions (Fig. 6 in Sect. 4.4). The vapor pressure of volatile organonitrate and PAN-like species, which are formed at high NOx levels, is not low enough to increase partitioning SOA mass (Fig. 7Aii). Thus, SOA yields decreased by increasing NOx levels. Overall, both simulation and chamber data show that monoalkylbenzene SOA yields increase with a decreased alkyl chain length: toluene > ethylbenzene > n-propylbenzene. This difference is most noticeable in the presence of an inorganic seed at high NOx levels (Fig. 7Aii and Hii) (Colberg et al., 2003).
Due to the pervasiveness and relatively high concentration of toluene in the urban situation, where HC∕NOx < 5.5 and wet inorganic seeds typically exist, the importance of toluene SOAs to the urban SOA burden can increase. The oxidation products from aromatic HCs can also involve cloud condensation nuclei activity due to their high reactivity via heterogeneous chemistry (Molteni et al., 2018), resulting in a change in the properties of clouds and fog and the urban radiation balance (Gordon et al., 2016). The unified aerosol-phase reaction rate constants for three monoalkylbenzenes represent the feasibility of applying the UNIPAR model to more aromatic systems (dialkyl benzenes and trialkyl benzenes) and the complex urban mixture.
The chamber data and simulation results as part of this publication are available upon request.
The supplement related to this article is available online at: https://doi.org/10.5194/acp-19-5719-2019-supplement.
MJ designed the experiments and CZ and ZY carried them out. MJ developed the model, and CZ performed the calculation of model parameters and the simulations. CZ and MJ prepared the manuscript with contributions from ZY.
The authors declare that they have no conflict of interest.
This research was supported by the National Strategic Project-Fine particle of the National Research Foundation of Korea (NRF) funded by the Ministry of Science and ICT (MSIT), the Ministry of Environment (ME), and the Ministry of Health and Welfare (MOHW) (2017M3D8A1090654).
This paper was edited by Christopher Hoyle and reviewed by Kyle Gorkowski and one anonymous referee.
Abramson, E., Imre, D., Beranek, J., Wilson, J., and Zelenyuk, A.: Experimental determination of chemical diffusion within secondary organic aerosol particles, Phys. Chem. Chem. Phys., 15, 2983–2991, https://doi.org/10.1039/c2cp44013j, 2013.
Ait-Helal, W., Borbon, A., Sauvage, S., de Gouw, J. A., Colomb, A., Gros, V., Freutel, F., Crippa, M., Afif, C., Baltensperger, U., Beekmann, M., Doussin, J. F., Durand-Jolibois, R., Fronval, I., Grand, N., Leonardis, T., Lopez, M., Michoud, V., Miet, K., Perrier, S., Prevot, A. S. H., Schneider, J., Siour, G., Zapf, P., and Locoge, N.: Volatile and intermediate volatility organic compounds in suburban Paris: variability, origin and importance for SOA formation, Atmos. Chem. Phys., 14, 10439–10464, https://doi.org/10.5194/acp-14-10439-2014, 2014.
Appel, K. W., Napelenok, S. L., Foley, K. M., Pye, H. O. T., Hogrefe, C., Luecken, D. J., Bash, J. O., Roselle, S. J., Pleim, J. E., Foroutan, H., Hutzell, W. T., Pouliot, G. A., Sarwar, G., Fahey, K. M., Gantt, B., Gilliam, R. C., Heath, N. K., Kang, D. W., Mathur, R., Schwede, D. B., Spero, T. L., Wong, D. C., and Young, J. O.: Description and evaluation of the Community Multiscale Air Quality (CMAQ) modeling system version 5.1, Geosci. Model Dev., 10, 1703–1732, https://doi.org/10.5194/gmd-10-1703-2017, 2017.
Beardsley, R. L. and Jang, M.: Simulating the SOA formation of isoprene from partitioning and aerosol phase reactions in the presence of inorganics, Atmos. Chem. Phys., 16, 5993–6009, https://doi.org/10.5194/acp-16-5993-2016, 2016.
Bertram, A. K., Martin, S. T., Hanna, S. J., Smith, M. L., Bodsworth, A., Chen, Q., Kuwata, M., Liu, A., You, Y., and Zorn, S. R.: Predicting the relative humidities of liquid-liquid phase separation, efflorescence, and deliquescence of mixed particles of ammonium sulfate, organic material, and water using the organic-to-sulfate mass ratio of the particle and the oxygen-to-carbon elemental ratio of the organic component, Atmos. Chem. Phys., 11, 10995–11006, https://doi.org/10.5194/acp-11-10995-2011, 2011.
Bloss, C., Wagner, V., Jenkin, M. E., Volkamer, R., Bloss, W. J., Lee, J. D., Heard, D. E., Wirtz, K., Martin-Reviejo, M., Rea, G., Wenger, J. C., and Pilling, M. J.: Development of a detailed chemical mechanism (MCMv3.1) for the atmospheric oxidation of aromatic hydrocarbons, Atmos. Chem. Phys., 5, 641–664, https://doi.org/10.5194/acp-5-641-2005, 2005.
Budisulistiorini, S. H., Nenes, A., Carlton, A. G., Surratt, J. D., McNeill, V. F., and Pye, H. O. T.: Simulating Aqueous-Phase Isoprene-Epoxydiol (IEPOX) Secondary Organic Aerosol Production During the 2013 Southern Oxidant and Aerosol Study (SOAS), Environ. Sci. Technol., 51, 5026–5034, https://doi.org/10.1021/acs.est.6b05750, 2017.
Cao, G. and Jang, M.: An SOA Model for Toluene Oxidation in the Presence of Inorganic Aerosols, Environ. Sci. Technol., 44, 727–733, https://doi.org/10.1021/es901682r, 2010.
Cappa, C. D. and Wilson, K. R.: Multi-generation gas-phase oxidation, equilibrium partitioning, and the formation and evolution of secondary organic aerosol, Atmos. Chem. Phys., 12, 9505–9528, https://doi.org/10.5194/acp-12-9505-2012, 2012.
Chen, J., Zhao, C. S., Ma, N., Liu, P. F., Gobel, T., Hallbauer, E., Deng, Z. Z., Ran, L., Xu, W. Y., Liang, Z., Liu, H. J., Yan, P., Zhou, X. J., and Wiedensohler, A.: A parameterization of low visibilities for hazy days in the North China Plain, Atmos. Chem. Phys., 12, 4935–4950, https://doi.org/10.5194/acp-12-4935-2012, 2012.
Chen, L. H., Bao, K. J., Li, K. W., Lv, B., Bao, Z. E., Lin, C., Wu, X. C., Zheng, C. H., Gao, X., and Cen, K. F.: Ozone and Secondary Organic Aerosol Formation of Toluene/NOx Irradiations under Complex Pollution Scenarios, Aerosol Air Qual. Res., 17, 1760–1771, https://doi.org/10.4209/aaqr.2017.05.0179, 2017.
Chen, X., Xie, M. J., Hays, M. D., Edgerton, E., Schwede, D., and Walker, J. T.: Characterization of organic nitrogen in aerosols at a forest site in the southern Appalachian Mountains, Atmos. Chem. Phys., 18, 6829–6846, https://doi.org/10.5194/acp-18-6829-2018, 2018.
Cheng, K., Hao, W.-W., Yi, P., Zhang, Y., and Zhang, J.-Y.: Volatile Organic Compounds Emission from Chinese Wood Furniture Coating Industry: Activity-based Emission Factor, Speciation Profiles, and Provincial Emission Inventory, Aerosol Air Qual. Res., 18, 2813–2825, https://doi.org/10.4209/aaqr.2018.02.0044, 2018.
Clegg, S. L., Brimblecombe, P., and Wexler, A. S.: Thermodynamic model of the system H+---NO3−-H2O at tropospheric temperatures, J. Phys. Che. A, 102, 2137–2154, 1998.
Colberg, C. A., Luo, B. P., Wernli, H., Koop, T., and Peter, T.: A novel model to predict the physical state of atmospheric aerosol particles, Atmos. Chem. Phys., 3, 909–924, https://doi.org/10.5194/acp-3-909-2003, 2003.
Donahue, N. M., Robinson, A. L., Stanier, C. O., and Pandis, S. N.: Coupled partitioning, dilution, and chemical aging of semivolatile organics, Environ. Sci. Technol., 40, 2635–2643, https://doi.org/10.1021/es052297c, 2006.
Donahue, N. M., Epstein, S. A., Pandis, S. N., and Robinson, A. L.: A two-dimensional volatility basis set: 1. organic-aerosol mixing thermodynamics, Atmos. Chem. Physics, 11, 3303–3318, https://doi.org/10.5194/acp-11-3303-2011, 2011.
Ervens, B., Turpin, B. J., and Weber, R. J.: Secondary organic aerosol formation in cloud droplets and aqueous particles (aqSOA): a review of laboratory, field and model studies, Atmos. Chem. Phys., 11, 11069–11102, https://doi.org/10.5194/acp-11-11069-2011, 2011.
Estillore, A. D., Hettiyadura, A. P., Qin, Z., Leckrone, E., Wombacher, B., Humphry, T., Stone, E. A., and Grassian, V. H.: Water Uptake and Hygroscopic Growth of Organosulfate Aerosol, Environ. Sci. Technol., 50, 4259–4268, https://doi.org/10.1021/acs.est.5b05014, 2016.
Forstner, H. J. L., Flagan, R. C., and Seinfeld, J. H.: Secondary organic aerosol from the photooxidation of aromatic hydrocarbons: Molecular composition, Environ. Sci. Technol., 31, 1345–1358, https://doi.org/10.1021/Es9605376, 1997.
George, C., Ammann, M., D'Anna, B., Donaldson, D. J., and Nizkorodov, S. A.: Heterogeneous Photochemistry in the Atmosphere, Chem. Rev., 115, 4218–4258, https://doi.org/10.1021/cr500648z, 2015.
George, I. J. and Abbatt, J. P. D.: Heterogeneous oxidation of atmospheric aerosol particles by gas-phase radicals, Nat. Chem., 2, 713–722, https://doi.org/10.1038/nchem.806, 2010.
Gomez Alvarez, E., Viidanoja, J., Munoz, A., Wirtz, K., and Hjorth, J.: Experimental confirmation of the dicarbonyl route in the photo-oxidation of toluene and benzene, Environ. Sci. Technol., 41, 8362–8369, https://doi.org/10.1021/es0713274, 2007.
Gordon, H., Sengupta, K., Rap, A., Duplissy, J., Frege, C., Williamson, C., Heinritzi, M., Simon, M., Yan, C., Almeida, J., Trostl, J., Nieminen, T., Ortega, I. K., Wagner, R., Dunne, E. M., Adamov, A., Amorim, A., Bernhammer, A. K., Bianchi, F., Breitenlechner, M., Brilke, S., Chen, X. M., Craven, J. S., Dias, A., Ehrhart, S., Fischer, L., Flagan, R. C., Franchin, A., Fuchs, C., Guida, R., Hakala, J., Hoyle, C. R., Jokinen, T., Junninen, H., Kangasluoma, J., Kim, J., Kirkby, J., Krapf, M., Kurten, A., Laaksonen, A., Lehtipalo, K., Makhmutov, V., Mathot, S., Molteni, U., Monks, S. A., Onnela, A., Perakyla, O., Piel, F., Petaja, T., Praplanh, A. P., Pringle, K. J., Richards, N. A. D., Rissanen, M. P., Rondo, L., Sarnela, N., Schobesberger, S., Scott, C. E., Seinfeldo, J. H., Sharma, S., Sipila, M., Steiner, G., Stozhkov, Y., Stratmann, F., Tome, A., Virtanen, A., Vogel, A. L., Wagner, A. C., Wagner, P. E., Weingartner, E., Wimmer, D., Winkler, P. M., Ye, P. L., Zhang, X., Hansel, A., Dommen, J., Donahue, N. M., Worsnop, D. R., Baltensperger, U., Kulmala, M., Curtius, J., and Carslaw, K. S.: Reduced anthropogenic aerosol radiative forcing caused by biogenic new particle formation, P. Natl. Acad. Sci. USA, 113, 12053–12058, https://doi.org/10.1073/pnas.1602360113, 2016.
Hartikainen, A., Yli-Pirila, P., Tiitta, P., Leskinen, A., Kortelainen, M., Orasche, J., Schnelle-Kreis, J., Lehtinen, K. E. J., Zimmermann, R., Jokiniemi, J., and Sippula, O.: Volatile Organic Compounds from Logwood Combustion: Emissions and Transformation under Dark and Photochemical Aging Conditions in a Smog Chamber, Environ. Sci. Technol., 52, 4979–4988, https://doi.org/10.1021/acs.est.7b06269, 2018.
Henry, K. M. and Donahue, N. M.: Photochemical Aging of alpha-Pinene Secondary Organic Aerosol: Effects of OH Radical Sources and Photolysis, J. Phys. Chem. A, 116, 5932–5940, https://doi.org/10.1021/jp210288s, 2012.
Hettiyadura, A. P. S., Stone, E. A., Kundu, S., Baker, Z., Geddes, E., Richards, K., and Humphry, T.: Determination of atmospheric organosulfates using HILIC chromatography with MS detection, Atmos. Meas. Tech., 8, 2347–2358, https://doi.org/10.5194/amt-8-2347-2015, 2015.
Hodzic, A., Kasibhatla, P. S., Jo, D. S., Cappa, C. D., Jimenez, J. L., Madronich, S., and Park, R. J.: Rethinking the global secondary organic aerosol (SOA) budget: stronger production, faster removal, shorter lifetime, Atmos. Chem. Phys., 16, 7917–7941, https://doi.org/10.5194/acp-16-7917-2016, 2016.
Huang, J. P., Mcqueen, J., Wilczak, J., Djalalova, I., Stajner, I., Shafran, P., Allured, D., Lee, P., Pan, L., Tong, D., Huang, H. C., Dimego, G., Upadhayay, S., and Monache, L. D.: Improving NOAA NAQFC PM2.5 Predictions with a Bias Correction Approach, Weather Forecast., 32, 407–421, https://doi.org/10.1175/Waf-D-16-0118.1, 2017.
Huang, M. Q., Zhang, J. H., Cai, S. Y., Liao, Y. M., Zhao, W. X., Hu, C. J., Gu, X. J., Fang, L., and Zhang, W. J.: Characterization of particulate products for aging of ethylbenzene secondary organic aerosol in the presence of ammonium sulfate seed aerosol, J. Environ. Sci.-China, 47, 219–229, https://doi.org/10.1016/j.jes.2015.11.033, 2016.
Im, Y., Jang, M., and Beardsley, R. L.: Simulation of aromatic SOA formation using the lumping model integrated with explicit gas-phase kinetic mechanisms and aerosol-phase reactions, Atmos. Chem. Phys., 14, 4013–4027, https://doi.org/10.5194/acp-14-4013-2014, 2014.
IPCC: Climate change 2014: mitigation of climate change, Cambridge University Press, Cambridge, 2015.
Jang, M. and Kamens, R. M.: A thermodynamic approach for modeling partitioning of semivolatile organic compounds on atmospheric particulate matter: Humidity e ffects, Environ. Sci. Technol., 32, 1237–1243, https://doi.org/10.1021/Es970773w, 1998.
Jang, M. and Kamens, R. M.: Characterization of secondary aerosol from the photooxidation of toluene in the presence of NOx and 1-propene, Environ. Sci. Technol., 35, 3626–3639, https://doi.org/10.1021/es010676+, 2001.
Jang, M., Czoschke, N. M., Lee, S., and Kamens, R. M.: Heterogeneous atmospheric aerosol production by acid-catalyzed particle-phase reactions, Science, 298, 814–817, https://doi.org/10.1126/science.1075798, 2002.
Jang, M., Czoschke, N. M., and Northcross, A. L.: Semiempirical model for organic aerosol growth by acid-catalyzed heterogeneous reactions of organic carbonyls, Environ. Sci. Technol., 39, 164–174, https://doi.org/10.1021/es048977h, 2005.
Jathar, S. H., Cappa, C. D., Wexler, A. S., Seinfeld, J. H., and Kleeman, M. J.: Simulating secondary organic aerosol in a regional air quality model using the statistical oxidation model – Part 1: Assessing the influence of constrained multi-generational ageing, Atmos. Chem. Phys., 16, 2309–2322, https://doi.org/10.5194/acp-16-2309-2016, 2016.
Jeffries, H., Gary, M., Kessler, M., and Sexton, K.: Morphecule reaction mechanism, MORPHO, ALLOMORPHIC simulation software, 1998.
Jenkin, M. E., Wyche, K. P., Evans, C. J., Carr, T., Monks, P. S., Alfarra, M. R., Barley, M. H., McFiggans, G. B., Young, J. C., and Rickard, A. R.: Development and chamber evaluation of the MCM v3.2 degradation scheme for beta-caryophyllene, Atmos. Chem. Phys., 12, 5275–5308, https://doi.org/10.5194/acp-12-5275-2012, 2012.
Jiang, H., Jang, M., and Yu, Z. C.: Dithiothreitol activity by particulate oxidizers of SOA produced from photooxidation of hydrocarbons under varied NOx levels, Atmos. Chem. Phys., 17, 9965–9977, https://doi.org/10.5194/acp-17-9965-2017, 2017.
Jimenez, J. L., Canagaratna, M. R., Donahue, N. M., Prevot, A. S. H., Zhang, Q., Kroll, J. H., DeCarlo, P. F., Allan, J. D., Coe, H., Ng, N. L., Aiken, A. C., Docherty, K. S., Ulbrich, I. M., Grieshop, A. P., Robinson, A. L., Duplissy, J., Smith, J. D., Wilson, K. R., Lanz, V. A., Hueglin, C., Sun, Y. L., Tian, J., Laaksonen, A., Raatikainen, T., Rautiainen, J., Vaattovaara, P., Ehn, M., Kulmala, M., Tomlinson, J. M., Collins, D. R., Cubison, M. J., Dunlea, E. J., Huffman, J. A., Onasch, T. B., Alfarra, M. R., Williams, P. I., Bower, K., Kondo, Y., Schneider, J., Drewnick, F., Borrmann, S., Weimer, S., Demerjian, K., Salcedo, D., Cottrell, L., Griffin, R., Takami, A., Miyoshi, T., Hatakeyama, S., Shimono, A., Sun, J. Y., Zhang, Y. M., Dzepina, K., Kimmel, J. R., Sueper, D., Jayne, J. T., Herndon, S. C., Trimborn, A. M., Williams, L. R., Wood, E. C., Middlebrook, A. M., Kolb, C. E., Baltensperger, U., and Worsnop, D. R.: Evolution of Organic Aerosols in the Atmosphere, Science, 326, 1525–1529, https://doi.org/10.1126/science.1180353, 2009.
Johnson, D., Jenkin, M. E., Wirtz, K., and Martin-Reviejo, M.: Simulating the Formation of Secondary Organic Aerosol from the Photooxidation of Toluene, Environ. Chem., 1, 150–165, https://doi.org/10.1071/EN04069, 2004.
Johnson, D., Jenkin, M. E., Wirtz, K., and Martin-Reviejo, M.: Simulating the formation of secondary organic aerosol from the photooxidation of aromatic hydrocarbons, Environ. Chem., 2, 35–48, https://doi.org/10.1071/EN04079, 2005.
Kamens, R. M., Zhang, H. F., Chen, E. H., Zhou, Y., Parikh, H. M., Wilson, R. L., Galloway, K. E., and Rosen, E. P.: Secondary organic aerosol formation from toluene in an atmospheric hydrocarbon mixture: Water and particle seed effects, Atmos. Environ., 45, 2324–2334, https://doi.org/10.1016/j.atmosenv.2010.11.007, 2011.
Kelly, J. M., Doherty, R. M., O'Connor, F. M., and Mann, G. W.: The impact of biogenic, anthropogenic, and biomass burning volatile organic compound emissions on regional and seasonal variations in secondary organic aerosol, Atmos. Chem. Phys., 18, 7393–7422, https://doi.org/10.5194/acp-18-7393-2018, 2018.
Kleindienst, T. E., Edney, E. O., Lewandowski, M., Offenberg, J. H., and Jaoui, M.: Secondary organic carbon and aerosol yields from the irradiations of isoprene and alpha-pinene in the presence of NOx and SO2, Environ. Sci. Technol., 40, 3807–3812, https://doi.org/10.1021/es052446r, 2006.
Kleindienst, T. E., Jaoui, M., Lewandowski, M., Offenberg, J. H., Lewis, C. W., Bhave, P. V., and Edney, E. O.: Estimates of the contributions of biogenic and anthropogenic hydrocarbons to secondary organic aerosol at a southeastern US location, Atmos. Environ., 41, 8288–8300, https://doi.org/10.1016/j.atmosenv.2007.06.045, 2007.
Kolska, Z., Ruzicka, V., and Gani, R.: Estimation of the enthalpy of vaporization and the entropy of vaporization for pure organic compounds at 298.15 K and at normal boiling temperature by a group contribution method, Indust. Eng. Chem. Res., 44, 8436–8454, https://doi.org/10.1021/ie050113x, 2005.
Lambe, A. T., Onasch, T. B., Croasdale, D. R., Wright, J. P., Martin, A. T., Franklin, J. P., Massoli, P., Kroll, J. H., Canagaratna, M. R., Brune, W. H., Worsnop, D. R., and Davidovits, P.: Transitions from Functionalization to Fragmentation Reactions of Laboratory Secondary Organic Aerosol (SOA) Generated from the OH Oxidation of Alkane Precursors, Environ. Sci. Technol., 46, 5430–5437, https://doi.org/10.1021/es300274t, 2012.
Li, J., Jang, M., and Beardsley, R. L.: Dialkylsulfate formation in sulfuric acid-seeded secondary organic aerosol produced using an outdoor chamber under natural sunlight, Environ. Chem., 13, 590–601, 2016.
Li, J. Y. and Jang, M.: Aerosol Acidity Measurement Using Colorimetry Coupled With a Reflectance UV-Visible Spectrometer, Aerosol Sci. Tech., 46, 833–842, https://doi.org/10.1080/02786826.2012.669873, 2012.
Li, L., Tang, P., and Cocker, D. R.: Instantaneous nitric oxide effect on secondary organic aerosol formation from m-xylene photooxidation, Atmos. Environ., 119, 144–155, 2015.
Li, L. J., Tang, P., Nakao, S., and Cocker, D. R.: Impact of molecular structure on secondary organic aerosol formation from aromatic hydrocarbon photooxidation under low-NOx conditions, Atmos. Chem. Phys., 16, 10793–10808, https://doi.org/10.5194/acp-16-10793-2016, 2016.
Li, M., Zhang, Q., Kurokawa, J., Woo, J. H., He, K. B., Lu, Z. F., Ohara, T., Song, Y., Streets, D. G., Carmichael, G. R., Cheng, Y. F., Hong, C. P., Huo, H., Jiang, X. J., Kang, S. C., Liu, F., Su, H., and Zheng, B.: MIX: a mosaic Asian anthropogenic emission inventory under the international collaboration framework of the MICS-Asia and HTAP, Atmos. Chem. Phys., 17, 935–963, https://doi.org/10.5194/acp-17-935-2017, 2017.
Lin, Y. H., Knipping, E. M., Edgerton, E. S., Shaw, S. L., and Surratt, J. D.: Investigating the influences of SO2 and NH3 levels on isoprene-derived secondary organic aerosol formation using conditional sampling approaches, Atmos. Chem. Phys., 13, 8457–8470, https://doi.org/10.5194/acp-13-8457-2013, 2013.
Liu, T. Y., Huang, D. D., Li, Z. J., Liu, Q. Y., Chan, M. N., and Chan, C. K.: Comparison of secondary organic aerosol formation from toluene on initially wet and dry ammonium sulfate particles at moderate relative humidity, Atmos. Chem. Phys., 18, 5677–5689, https://doi.org/10.5194/acp-18-5677-2018, 2018.
McDonald, B. C., de Gouw, J. A., Gilman, J. B., Jathar, S. H., Akherati, A., Cappa, C. D., Jimenez, J. L., Lee-Taylor, J., Hayes, P. L., McKeen, S. A., Cui, Y. Y., Kim, S. W., Gentner, D. R., Isaacman-VanWertz, G., Goldstein, A. H., Harley, R. A., Frost, G. J., Roberts, J. M., Ryerson, T. B., and Trainer, M.: Volatile chemical products emerging as largest petrochemical source of urban organic emissions, Science, 359, 760–764, https://doi.org/10.1126/science.aaq0524, 2018.
McNeill, V. F., Woo, J. L., Kim, D. D., Schwier, A. N., Wannell, N. J., Sumner, A. J., and Barakat, J. M.: Aqueous-Phase Secondary Organic Aerosol and Organosulfate Formation in Atmospheric Aerosols: A Modeling Study, Environ. Sci. Technol., 46, 8075–8081, https://doi.org/10.1021/es3002986, 2012.
Mcvay, R. C., Cappa, C. D., and Seinfeld, J. H.: Vapor-Wall Deposition in Chambers: Theoretical Considerations, Environ. Sci. Technol., 48, 10251–10258, https://doi.org/10.1021/es502170j, 2014.
Molteni, U., Bianchi, F., Klein, F., El Haddad, I., Frege, C., Rossi, M. J., Dommen, J., and Baltensperger, U.: Formation of highly oxygenated organic molecules from aromatic compounds, Atmos. Chem. Phys., 18, 1909–1921, https://doi.org/10.5194/acp-18-1909-2018, 2018.
Nakao, S., Clark, C., Tang, P., Sato, K., and Cocker, D.: Secondary organic aerosol formation from phenolic compounds in the absence of NOx, Atmos. Chem. Phys., 11, 10649–10660, https://doi.org/10.5194/acp-11-10649-2011, 2011.
Ng, N. L., Kroll, J. H., Chan, A. W. H., Chhabra, P. S., Flagan, R. C., and Seinfeld, J. H.: Secondary organic aerosol formation from m-xylene, toluene, and benzene, Atmos. Chem. Phys., 7, 3909–3922, https://doi.org/10.5194/acp-7-3909-2007, 2007.
Noziere, B., Ekstrom, S., Alsberg, T., and Holmstrom, S.: Radical-initiated formation of organosulfates and surfactants in atmospheric aerosols, Geophys. Res. Lett., 37, L05806, https://doi.org/10.1029/2009gl041683, 2010.
Odian, G.: Principles of polymerization, John Wiley & Sons, New York, 2004.
Odum, J. R., Hoffmann, T., Bowman, F., Collins, D., Flagan, R. C., and Seinfeld, J. H.: Gas/particle partitioning and secondary organic aerosol yields, Environ. Sci. Technol., 30, 2580–2585, https://doi.org/10.1021/Es950943+, 1996.
Pankow, J. F.: An Absorption-Model of Gas-Particle Partitioning of Organic-Compounds in the Atmosphere, Atmos. Environ., 28, 185–188, https://doi.org/10.1016/1352-2310(94)90093-0, 1994.
Press, W. H., Teukolsky, S. A., Vetterling, W. T., and Flannery, B. P.: Numerical recipes in Fortran 77: the art of scientific computing, Cambridge University Press, Cambridge, 1992.
Ren, J. Y., Zhang, F., Wang, Y. Y., Collins, D., Fan, X. X., Jin, X. A., Xu, W. Q., Sun, Y. L., Cribb, M., and Li, Z. Q.: Using different assumptions of aerosol mixing state and chemical composition to predict CCN concentrations based on field measurements in urban Beijing, Atmos. Chem. Phys., 18, 6907–6921, https://doi.org/10.5194/acp-18-6907-2018, 2018.
Requia, W. J., Higgins, C. D., Adams, M. D., Mohamed, M., and Koutrakis, P.: The health impacts of weekday traffic: A health risk assessment of PM2.5 emissions during congested periods, Environ. Int., 111, 164–176, https://doi.org/10.1016/j.envint.2017.11.025, 2018.
Rudich, Y., Donahue, N. M., and Mentel, T. F.: Aging of organic aerosol: Bridging the gap between laboratory and field studies, Annu. Rev. Phys. Chem., 58, 321–352, 2007.
Sato, K., Hatakeyama, S., and Imamura, T.: Secondary organic aerosol formation during the photooxidation of toluene: NOx dependence of chemical composition, J. Phys. Chem. A, 111, 9796–9808, https://doi.org/10.1021/jp071419f, 2007.
Sato, K., Takami, A., Kato, Y., Seta, T., Fujitani, Y., Hikida, T., Shimono, A., and Imamura, T.: AMS and LC/MS analyses of SOA from the photooxidation of benzene and 1,3,5-trimethylbenzene in the presence of NOx: effects of chemical structure on SOA aging, Atmos. Chem. Phys., 12, 4667–4682, https://doi.org/10.5194/acp-12-4667-2012, 2012.
Schell, B., Ackermann, I. J., Hass, H., Binkowski, F. S., and Ebel, A.: Modeling the formation of secondary organic aerosol within a comprehensive air quality model system, J. Geophys. Res.-Atmos., 106, 28275–28293, https://doi.org/10.1029/2001jd000384, 2001.
Seinfeld, J. H. and Pandis, S. N.: Atmospheric chemistry and physics: from air pollution to climate change, John Wiley & Sons, New York, 2016.
Sheehan, P. E. and Bowman, F. M.: Estimated effects of temperature on secondary organic aerosol concentrations, Environ. Sci. Technol., 35, 2129–2135, https://doi.org/10.1021/Es001547g, 2001.
Shilling, J. E., King, S. M., Mochida, M., and Martin, S. T.: Mass spectral evidence that small changes in composition caused by oxidative aging processes alter aerosol CCN properties, J. Phys. Chem. A, 111, 3358–3368, https://doi.org/10.1021/jp068822r, 2007.
Song, C., Na, K. S., and Cocker, D. R.: Impact of the hydrocarbon to NOx ratio on secondary organic aerosol formation, Environ. Sci. Technol., 39, 3143–3149, https://doi.org/10.1021/es0493244, 2005.
Surratt, J. D., Chan, A. W. H., Eddingsaas, N. C., Chan, M. N., Loza, C. L., Kwan, A. J., Hersey, S. P., Flagan, R. C., Wennberg, P. O., and Seinfeld, J. H.: Reactive intermediates revealed in secondary organic aerosol formation from isoprene, P. Natl. Acad. Sci. USA, 107, 6640–6645, https://doi.org/10.1073/pnas.0911114107, 2010.
Tsigaridis, K., Daskalakis, N., Kanakidou, M., Adams, P. J., Artaxo, P., Bahadur, R., Balkanski, Y., Bauer, S. E., Bellouin, N., Benedetti, A., Bergman, T., Berntsen, T. K., Beukes, J. P., Bian, H., Carslaw, K. S., Chin, M., Curci, G., Diehl, T., Easter, R. C., Ghan, S. J., Gong, S. L., Hodzic, A., Hoyle, C. R., Iversen, T., Jathar, S., Jimenez, J. L., Kaiser, J. W., Kirkevag, A., Koch, D., Kokkola, H., Lee, Y. H., Lin, G., Liu, X., Luo, G., Ma, X., Mann, G. W., Mihalopoulos, N., Morcrette, J. J., Muller, J. F., Myhre, G., Myriokefalitakis, S., Ng, N. L., O'Donnell, D., Penner, J. E., Pozzoli, L., Pringle, K. J., Russell, L. M., Schulz, M., Sciare, J., Seland, O., Shindell, D. T., Sillman, S., Skeie, R. B., Spracklen, D., Stavrakou, T., Steenrod, S. D., Takemura, T., Tiitta, P., Tilmes, S., Tost, H., van Noije, T., van Zyl, P. G., von Salzen, K., Yu, F., Wang, Z., Wang, Z., Zaveri, R. A., Zhang, H., Zhang, K., Zhang, Q., and Zhang, X.: The AeroCom evaluation and intercomparison of organic aerosol in global models, Atmos. Chem. Phys., 14, 10845–10895, https://doi.org/10.5194/acp-14-10845-2014, 2014.
Wood, E. C., Canagaratna, M. R., Herndon, S. C., Onasch, T. B., Kolb, C. E., Worsnop, D. R., Kroll, J. H., Knighton, W. B., Seila, R., Zavala, M., Molina, L. T., DeCarlo, P. F., Jimenez, J. L., Weinheimer, A. J., Knapp, D. J., Jobson, B. T., Stutz, J., Kuster, W. C., and Williams, E. J.: Investigation of the correlation between odd oxygen and secondary organic aerosol in Mexico City and Houston, Atmos. Chem. Phys., 10, 8947–8968, https://doi.org/10.5194/acp-10-8947-2010, 2010.
Yu, Z. C., Jang, M., and Park, J.: Modeling atmospheric mineral aerosol chemistry to predict heterogeneous photooxidation of SO2, Atmos. Chem. Phys., 17, 10001–10017, https://doi.org/10.5194/acp-17-10001-2017, 2017.
Zhang, X., Cappa, C. D., Jathar, S. H., Mcvay, R. C., Ensberg, J. J., Kleeman, M. J., and Seinfeld, J. H.: Influence of vapor wall loss in laboratory chambers on yields of secondary organic aerosol, P. Natl. Acad. Sci. USA, 111, 5802–5807, https://doi.org/10.1073/pnas.1404727111, 2014.
Zhang, Y., Seigneur, C., Seinfeld, J. H., Jacobson, M., Clegg, S. L., and Binkowski, F. S.: A comparative review of inorganic aerosol thermodynamic equilibrium modules: similarities, differences, and their likely causes, Atmos. Environ., 34, 117–137, https://doi.org/10.1016/S1352-2310(99)00236-8, 2000.
Zhang, Y. L., Yang, W. Q., Simpson, I., Huang, X. Y., Yu, J. Z., Huang, Z. H., Wang, Z. Y., Zhang, Z., Liu, D., Huang, Z. Z., Wang, Y. J., Pei, C. L., Shao, M., Blake, D. R., Zheng, J. Y., Huang, Z. J., and Wang, X. M.: Decadal changes in emissions of volatile organic compounds (VOCs) from on-road vehicles with intensified automobile pollution control: Case study in a busy urban tunnel in south China, Environ. Pollut., 233, 806–819, https://doi.org/10.1016/j.envpol.2017.10.133, 2018.
Zhao, B., Wang, S. X., Donahue, N. M., Chuang, W. N., Hildebrandt Ruiz, L., Ng, N. L., Wang, Y. J., and Hao, J. M.: Evaluation of One-Dimensional and Two-Dimensional Volatility Basis Sets in Simulating the Aging of Secondary Organic Aerosol with Smog-Chamber Experiments, Environ. Sci. Technol., 49, 2245–2254, https://doi.org/10.1021/es5048914, 2015.
Zhao, L. W., Li, P., and Yalkowsky, S. H.: Predicting the entropy of boiling for organic compounds, J. Chem. Inf. Comp. Sci., 39, 1112–1116, https://doi.org/10.1021/Ci990054w, 1999.
Zuend, A., Marcolli, C., Booth, A. M., Lienhard, D. M., Soonsin, V., Krieger, U. K., Topping, D. O., McFiggans, G., Peter, T., and Seinfeld, J. H.: New and extended parameterization of the thermodynamic model AIOMFAC: calculation of activity coefficients for organic-inorganic mixtures containing carboxyl, hydroxyl, carbonyl, ether, ester, alkenyl, alkyl, and aromatic functional groups, Atmos. Chem. Phys., 11, 9155–9206, https://doi.org/10.5194/acp-11-9155-2011, 2011.