the Creative Commons Attribution 4.0 License.
the Creative Commons Attribution 4.0 License.
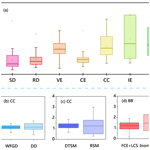
Characteristics of the main primary source profiles of particulate matter across China from 1987 to 2017
Xiaohui Bi
Jianhui Wu
Qing Zhang
Wenhui Zhang
Ruixue Luo
Yuan Cheng
Jiaying Zhang
Lu Wang
Zhuojun Yu
Yufen Zhang
Yingze Tian
Based on published literature and typical profiles from the Nankai University source library, a total of 3326 chemical profiles of the main primary sources of ambient particulate matter (PM) across China from 1987 to 2017 are investigated and reviewed to trace the evolution of their main components and identify the main influencing factors concerning their evolution. In general, the source chemical profiles are varied with respect to their sources and are influenced by different sampling methods. The most complicated profiles are likely attributed to coal combustion (CC) and industrial emissions (IE). The profiles of vehicle emissions (VE) are dominated by organic carbon (OC) and elemental carbon (EC), and vary due to the changing standards of sulfur and additives in gasoline and diesel as well as the sampling methods used. In addition to the sampling methods used, the profiles of biomass burning (BB) and cooking emissions (CE) are also impacted by the different biofuel categories and cooking types, respectively. The variations of the chemical profiles of different sources, and the homogeneity of the subtype source profiles within the same source category are examined using uncertainty analysis and cluster analysis. As a result, a relatively large variation is found in the source profiles of CC, VE, IE, and BB, indicating that these sources urgently require the establishment of local profiles due to their high uncertainties. The results presented highlight the need for further investigation of more specific markers (e.g., isotopes, organic compounds, and gaseous precursors), in addition to routinely measured components, in order to properly discriminate sources. Although the chemical profiles of the main sources have been previously reported in the literature, it should be noted that some of these chemical profiles are currently out of date and need to be updated immediately. Additionally, in the future, specific focus should be placed on the source profile subtypes, especially with respect to local IE in China.
- Article
(5646 KB) - Full-text XML
-
Supplement
(347 KB) - BibTeX
- EndNote
In light of preventing human exposure to high levels of ambient particulate matter (PM), the source apportionment technique is a critical tool that helps with the quantitative recognition of the source contributions of PM and with developing efficient and cost-effective abatement policy. Given the thousands of PM sources in the real world, localized source information is crucial for accurate source identification and contribution estimation. The physical and chemical characterization of primary sources, termed the source profile, is of great importance in the application of receptor models for source apportionment studies, as it characterizes specific sources from a physicochemical point of view which reveals the signatures of source emissions (Watson, 1984; Bi et al., 2007; Simon et al., 2010; Hopke, 2016). As the real-world measurement of source samples is costly and difficult, many studies use factor analytical models (source-unknown models), such as positive matrix factorization (PMF) and principle component analysis (PCA), instead of chemical mass-balance (CMB) models (where source profiles need to be known a priori) to estimate source contributions. However, the measurement of sources is essentially very important fundamental work, as it helps obtain source signatures which subsequently make source identification and apportionment possible. It should be noted that the interpretation of factors deduced from PMF analysis is based on the available source profiles (Shi et al., 2009; Simon et al., 2010; Liu et al., 2017; Hopke, 2016). In addition to source apportionment studies, source profiles also play an important role in calculating source-specific emissions of individual compounds and converting total emissions from sources into the speciated emissions used in air quality models, which can further provide effective strategies for environmental management (Reff et al., 2009; Simon et al., 2010).
In the past few decades, source profiles of PM from a variety of source types have been substantially developed all over the world, especially in US (Simon et al., 2010), Europe (Pernigotti et al., 2016) and East Asia (Liu et al., 2017). The time evolution of source profiles is partly determined by the source apportionment techniques. In general, the receptor model was developed based on the assumption of mass conservation (Winchester and Nifong, 1971; Miller et al., 1972). A mass-balance equation represents that the measured particle mass can be regarded as the linear sum of the mass of all chemical components contributed from several sources (Cooper and Watson, 1980; Watson, 1984). Initially, mass-balance equations were deployed for a couple of specific elements and source types in the US (Miller et al., 1972; Hopke, 2016). Elements, ions, and carbon materials have gradually become the routine chemical species in the source apportionment of PM. With the development of advanced sampling and chemical analysis techniques, more valuable information has been explored to further expand the existing profiles or create new profiles, including data on the following: organic compounds (Schauer and Cass, 2000; Simoneit et al., 1999); isotopic measurement of radiocarbon (Wang et al., 2017), sulfur (Han et al., 2016), and nitrogen (Pan et al., 2016); high-resolution aerosol mass spectra (Zhang et al., 2011); and particle size distribution (Zhou et al., 2004). This information has been proven to provide source specificity capable of being incorporated into receptor models as new markers (Zheng et al., 2002), constraining source contributions (Amato et al., 2009), and developing new models (Ulbrich et al., 2012; Dai et al., 2019). For example, Dai et al. (2019) developed a size-resolved CMB approach for the source apportionment of PM based on the size profiles of sources. This new, valuable information improves the performance of source apportionment models and allows them to obtain more precise and reliable results.
Source profile studies were initially implemented in China in the 1980s (Dai et al., 1987a, b). Over the past 3 decades, hundreds of source profiles have been compiled across the country (Zhao et al., 2006, 2007a, b, 2015a, b; Bi et al., 2007; Kong et al., 2011, 2014; Qi et al., 2015; Wang et al., 2015; Zhang et al., 2015; Pei et al., 2016; Tian et al., 2017; Guo et al., 2017). These profiles cover more than 40 cities and several source types. The main ubiquitous sources of atmospheric PM in China over the past 3 decades can be roughly divided into coal combustion sources (CC – including the coal-fired power plants, coal-fired industrial boilers, and residential coal combustion subtypes), vehicle exhaust (VE – emissions from gasoline and diesel engines), industrial process emissions (IE), biomass burning (BB), cooking emissions (CE), fugitive dust (FD – including the soil fugitive dust, construction dust, and road dust subtypes), and other localized specific sources. These available profiles have filled the knowledge gap with respect to source compositions and have provided effective markers for source apportionment studies. However, the current state of, and potential issues regarding, preexisting primary source profiles of PM in China are still unclear. Therefore, it is time to review the existing source profiles in order to provide the atmospheric research community with more profile knowledge.
In fact, many real-world profiles measured in China have not been published. A database of particulate source profiles founded by the Nankai University contains 2870 profiles that have been measured across China since the 1980s. In this paper, the characteristics and time evolution of the published primary profiles and some typical PM profiles founded by Nankai University are discussed. To collect the potential published data related to source profiles, a two-round literature search covering literature from 1980 to 2018 was carried out. In the first round of the literature search, two authors were responsible for the same source to ensure that every source category was searched twice independently. The search keywords depended on the source category. The following keywords were used individually or in combination for each source: for CC sources – coal combustion, coal burning, coal-fired boiler, coal-fired power plant, residential coal, source profile, chemical profile, and particle composition; for IE – industrial emission, source profile, chemical profile, and particle composition; for VE – vehicle emission, exhaust emission, traffic emission, diesel engine, truck emission, gasoline engine, on-road vehicle, tunnel experiment, chassis dynamometer, portable emission measurement system, source profile, chemical profile, and particle composition; for CE – cooking emission, source profile, chemical profile, and particle composition; for BB – biomass burning, bio-fuel boiler, source profile, chemical profile, and particle composition; and for FD – soil, fugitive dust, crustal material, construction dust, road dust, source profile, chemical profile, and particle composition. Papers and dissertations in Chinese on the China National Knowledge Infrastructure (CNKI) and papers in English on the Web of Science were searched using abovementioned keywords, respectively. Duplicated papers were then double-checked and excluded. Papers on topics related to source profiles but without any information regarding real, measured sources were also excluded. For example, papers that reported source apportionment results from PMF and CMB analyses but did not report local profiles were not taken into account. As a result, a total of 193 papers were collected from these efforts. In the second round of the literature search, the valid papers with available source profile data and detailed source sampling and chemical analysis methods were counted and used in the following analysis. Finally, a total of 456 published source profiles, coupled with the database of source profiles (2870 profiles) founded by the Nankai University were reviewed in this work.
This review is structured as follows: in Sect. 2.1, we summarize the types and the number of particulate source profiles in China that have been published since the 1980s, and review the technological developments in the sampling and chemical analysis methods for source samples. In Sect. 2.2, the characteristics and time evolution of the ubiquitous source profiles in China (CC, VE, IE, BB, CE, and FD) in terms of the marker species of each main source and the effect of various impact factors on source profiles are discussed. In Sect. 2.3, the homogeneity of the sources within the same source category and the heterogeneity between different source categories are further investigated using the coefficient of variation (CV – the standard deviation divided by the mean) and cluster analysis, respectively. In Sect. 3, we summarize the main findings and a few issues relating to the current source profiles, as well as the future requirements for the ongoing development of source profiles in China.
After the abovementioned literature search (of peer-reviewed papers published in international and Chinese journals), a total of 456 published source profiles from across China ranging from the 1980s to the present were collected. In general, all of these profiles were subjectively divided into the previously mentioned six source categories: 81 of them were attributed to CC, 67 to IE, 35 to VE, 98 to FD, 36 to CE, and 139 to BB. With respect to the specific aerodynamic sizes, we obtained a total of 306 PM2.5 profiles, 123 PM10 profiles, and 27 profiles for other sizes. An overview of these profiles is shown in Fig. 1.
These published profiles were also measured in different parts of China. For eastern China, there are 35 published profiles of CC (excluding residential coal combustion), 14 for IE, 14 for VE, 18 for BB, 2 for CE, and 14 for FD; in northern China, there are 16 published profiles for CC, 23 for IE, 9 for VE, 8 for BB, 13 for CE, and 62 for FD; in western China, there are only 20 profiles for CC; in southern China, there are 10 published profiles for VE, 10 for CE, and 5 for FD; in central China, there are 17 published profiles for BB. The profiles of residential coal combustion (RCC) are mainly detected in regions that have obvious residential coal burning activities, such as northern and western China. The different regions of China are defined in Zhu et al. (2018).
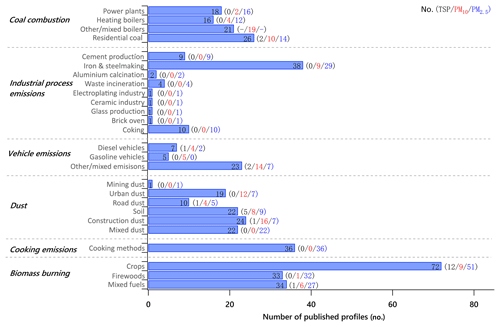
Figure 1Overview of the published source profiles across China. The numbers inside the bars refer to the total number of published profiles for specific source subtypes, whereas the numbers after the bars denote the number of profiles based on measurements of total suspended particles (TSP), PM10, and PM2.5, respectively (see legend).
2.1 Development of sampling and analysis techniques
Sampling for source emissions
Over the past 3 decades, the sampling techniques used in source apportionment research in China have been significantly improved in order to capture the real-world emissions of particles from various complex primary sources. In the 1980s, CC was the predominant source of PM in China (Dai et al., 1987a). The source measurement of CC was mainly performed by collecting dust directly from the precipitators. Source samples of FD were collected from the surfaces of fugitive dust sources (soil, road dust, etc.) (Dai et al., 1987; Qu, 2013). However, sampling methods such as these cannot capture real-world emissions from sources to the ambient air, especially with respect to CC or other emission sources with humid and high-temperature fumes. This is due to the fact that the composition of PM in such fumes appears to change due to physical condensation and chemical reactions during the dispersion process in ambient air. In the 1970s, the dilution tunnel sampling method (DTSM) was originally developed to obtain source samples from vehicle emissions that were close to the real compositions from the sources (Hildemann et al., 1989). Since the 1970s, various dilution tunnels have consequently been developed using different tunnel materials, residence times, dilution ratios, and diameters and effective mixing lengths to collect particles emissions from stationary sources (Houck et al., 1982; Smith et al., 1982; Hildemann, 1989). The development and application of this kind of technique did not take place in China until 2000 (Ge et al., 2001, 2004), although it is widely used nowadays (England et al., 2000; Lind et al., 2003; Ferge et al., 2004; Zhou et al., 2006; Li et al., 2009; Wang et al., 2012).
With respect to fugitive dust, an additional problem is the collection of particle samples with particular aerodynamic sizes from dust samples. In the 1980s–1990s, the Bahco particle size analyzer was used to obtain the size distributions of the source samples (Kauppinen et al., 1991). Due to the low efficiency and potential safety risks involved with the Bahco sampler, a new sampling technique called the resuspension chamber (RSM) was developed in the 1990s by Chow et al. (1994), and has been widely used in China since 2000. This method is capable of obtaining particle samples of certain aerodynamic sizes from dust powder collected from the source in the field. Nowadays, most source samples of fugitive dust in China with a particle aerodynamic size of 2.5 or 10 µm were collected using the RSM (Ho et al., 2003; Zhao et al., 2006). Although the resuspension chamber cannot completely simulate the real environment, it is still currently the best available option for the collection of fugitive dust samples.
Excluding stationary sources, moving sources such as vehicle emissions are gradually becoming the dominant sources in the megacities of China. A variety of measurement methods for vehicle emissions have been developed throughout the world, including direct measurements of exhaust emissions of on-road vehicles and chassis dynamometer testing, portable emission measurement systems, and tunnel experiments.
Regarding biomass burning and RCC, the DTSM has been utilized to measure emissions involved in different combustion methods. For example, indoor or laboratory simulations with fuel burned using a stove or chamber, as well as open burning or field measurements. In addition, biomass fuel can be burned in biofuel boilers, and the industrial use of this type of boiler has recently become more common.
From the previously mentioned published profiles, 65 % of CC profiles, 53 % of IE profiles, 12 % of CE profiles, 43 % of VE profiles, and 37 % of BB profiles were obtained using the DTSM (as shown in Fig. 2).
Chemical analysis
Chemical analysis methods have significantly improved since the 1980s. A typical source profile from literature data collected in China usually contains elements (e.g., Al, As, Ca, Cd, Cr, Cu, Fe, K, Mg, Mn, Na, Pb, and Zn), organic carbon (OC), elemental carbon (EC), and water-soluble ions (WSIs, e.g., Cl−, nitrate (), sulfate (), ammonium (), K+, Na+, Mg2+, and Ca2+). Detailed procedures regarding the establishment of different source profiles are available in previous publications (Chow et al., 1994, 2004; Hou et al., 2008b; Pei et al., 2016).
In China, PM samples collected on Teflon filters have generally been analyzed for elements using an inductively coupled plasma optical emission spectrometer (ICP-OES) or an inductively coupled plasma atomic emission spectrometer (ICP-AES). In recent years, inductively coupled plasma mass spectrometer (ICP-MS) instruments and X-ray fluorescence have also been used, which have a lower threshold/higher accuracy and a quick response time, respectively (Tsai et al., 2004). The total carbon (TC) mass is typically determined using thermal or thermal–optical methods. With the use of a thermal–optical carbon analyzer, there are two widely utilized approaches to dividing OC and EC from TC, known as IMPROVE_A (from the Desert Research Institute – DRI) and NIOSH (method 5040; from the National Institute for Occupational Safety and Health – NIOSH), which are operationally defined by the time–temperature protocols, and the OC–EC split point is determined by optical reflectance/transmittance (Chow et al., 1994, 2004; Ho et al., 2003; Zhang et al., 2007; Phuah et al., 2009). Quartz-fiber filters are normally used for the determination of WSIs via different types of ion chromatography (IC) with high-capacity cation-exchange and anion-exchange columns (Qi et al., 2015).
Organic tracer species, which can be used as indicators of particular sources, play an important role in estimating source contributions. However, most of the source profiles in China are reported using inorganic species, with only a few studies providing information on organic compounds. Organic tracers are of great value in source apportionment studies, as they provide more source-specific information than inorganic species. For example, levoglucosan is a well-known organic tracer for biomass burning (Lee et al., 2008), azaarenes (nitrogen-heterocyclic polycyclic aromatic compounds) are markers of inefficient CC (Junninen et al., 2009; Bandowe et al., 2016), and sterols, monosaccharide anhydrides, and amides are markers of cooking emissions (Schauer et al., 1999, 2002; He et al., 2004; Zhao et al., 2007a, b; Cheng et al., 2016). Furthermore, in order to better discriminate sources, Pb stable isotopes, which are not obviously influenced by ordinary chemical, physical, or biological fractionation processes (Gallon et al., 2005; Cheng and Hu, 2010), were determined using an ICP-MS. Additionally, other isotope measurements, including radiocarbon (Wang et al., 2017), sulfur (Han et al., 2016), and nitrogen (Pan et al., 2016), as well as natural silicon (Lu et al., 2018), have also been recently used as source indicators.
The abovementioned efforts indicate that the reported source profiles were collected using various sampling methods and were also chemically analyzed by different instruments, which makes comparison of the source profiles highly uncertain. It is necessary to establish standards with respect to source sampling, chemical analysis, and QA/QC procedures to ensure the representativeness, validation, and comparability of source profiles collated in China.
2.2 Characteristics and evolution of source profiles
2.2.1 Coal combustion
Coal is the main fuel used in China and is widely used in coal-fired power plants, coal-fired industrial boilers, and residential household stoves; on average, coal accounted for more than 60 % of the total energy consumed in China in 2015 (CESY, 2015). Thus, it is the main cause of air pollution, particularly during the heating season in northern China. The source profiles of CC sources are influenced by many factors, including coal type and property, boiler or stove type and efficiency, burning conditions (burning rate and fuel loading), and decontamination devices (Shen, 2010); therefore, CC appears to be the most complicated of the primary sources. The source profiles of CC in China mainly consist of crustal materials, OC, EC, , and trace metals, indicating the chemical nature of coal burning.
Coal-fired power plants
Using data collected via the same sampling method (DTSM) and from the same boiler type, the characteristics of the source profiles of coal-fired power plants equipped with different dust removal and desulfurization facilities are compared in Fig. 3. OC, EC, and Cl− values in the profiles of electrostatic precipitators (EP) are higher than those in the profiles of electric bag compound dust collectors (EBCC), with average values of 0.0289±0.0342, 0.0036±0.0033, and 0.1403±0.1686 g g−1, respectively. Higher Ca, , and Ca2+ concentrations in the source profiles obtained using EBCC are also found. Comparing data from different desulfurization facilities (Fig. 3), and Ca in PM2.5 profiles from wet flue gas desulfurization (WFGD) are much higher than those from dry desulfurization (DD). It is reported that is converted from SO2 in the flue gas, via a limestone slurry washing reaction, and is then discharged with the fumes (Ma et al., 2015). Ca is also infused in the fumes when flue gas goes through the limestone washing process. OC values in PM2.5 profiles from WFGD are also higher than those from DD, suggesting the possible conversion of gaseous or liquid organics to the particulate state in the limestone slurry. , Na+, and Cl− levels are also higher in the WFGD profiles than in the DD profiles. Hence, the formation mechanism of these species in WFGD requires further investigation.
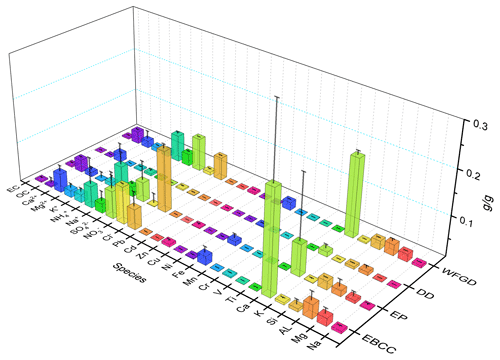
Figure 3Comparison of PM2.5 source profiles collected using different dust removal and desulfurization facilities. WFGD denotes wet flue gas desulfurization, DD denotes dry desulfurization, EP denotes electrostatic precipitators, and EBCC denotes electric bag compound dust collectors. Data from the Nankai University source library were collated.
To evaluate the impact of different sampling methods on the contents of source profiles, measurements using the coal ash RSM and the stack gas DTSM were simultaneously utilized for source sampling at a coal-fired power plant in Wuxi, China. The results of the PM10 source profiles obtained are shown in Fig. 4. For RSM, the crustal elements (Si, Mg, Al, and Ti) are significantly higher than the DTSM values, whereas the fraction of the DTSM measurements is significantly higher than the RSM values, reaching 0.1643 g g−1. V, Cr, Mn, Co, Ni, Cu, Zn, Pb, and other trace metal fractions are strongly enriched in the DTSM measurements, which are 1.7–60.7 times those of the RSM. This suggests that these trace metal elements have a low melting point and are easily liquefied or gasified during combustion, before being condensed on the surface of the particles in the flue or after exiting the flue (where small particles have a large specific surface area and are more prone to enrichment) (Dai et al., 1987a). Similar results have also been previously reported elsewhere (Meij, 1994; Meij and Winkel, 2004; Zhang et al., 2009b).
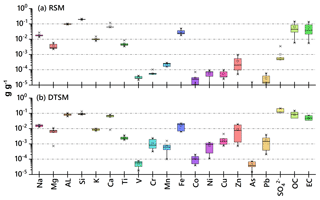
Figure 4Characteristics of chemical profiles for PM10 emitted from a coal-fired power plant obtained using different sampling methods in Wuxi city. RSM and the DTSM denote the resuspension sampling method and the dilution tunnel sampling method, respectively. Data were acquired from the Nankai University source library.
Coal-fired industrial boiler
Coal-fired industrial boilers are used to provide hot water or steam for industry or municipal heating. These boilers consume about 1.1 billion tons of coal annually in China, accounting for 25 % of the total coal consumption, and only have the average capacity of 2.7 MW (ERI, 2013). A comparison of profiles detected in coal-fired power plants and those detected in coal-fired industrial boilers shows that there are substantial differences in these source profiles. Figure 5 shows the differences in the chemical compositions of source profiles between coal-fired industrial boilers with wet desulfurization (IBW) and power plant boilers with wet desulfurization (PPW) using PM samples collected using the same method. Mg, Al, Si, Ca, , , and OC values in the profiles of PPW are higher than those in the profiles of IBW, which likely results from the combustion efficiency and desulfurization efficiency: PPWs are required by the government to operate at highly efficient desulfurization levels, whereas IBWs are less controlled.
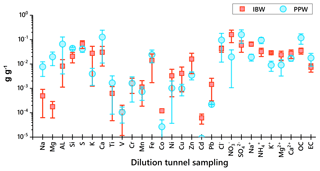
Figure 5The means and standard deviations of chemical species in the source profiles of coal-fired industrial boilers equipped with wet desulfurization devices (IBW) and power plant boilers equipped with wet desulfurization devices (PPW), respectively. Data were collated from the Nankai University source library.
Residential Coal Combustion (RCC)
In 2015, the total amount of coal consumption in mainland China was about 3970.14 Mt with a total of 93.47 Mt of coal consumed in residential sector (CESY, 2015). RCC is an important source of atmospheric PM in rural areas, particularly during the heating season (Duan et al., 2014; Tao et al., 2018; Chen et al., 2004, 2005; Zhang et al., 2007). Contrary to industrial furnaces and boilers, coal burned in household stoves has a significant impact on the indoor and outdoor air quality in terms of its low thermal efficiency, incomplete combustion, and the lack of air pollutant control devices. It has been reported that the emission factors of air pollutants for coal burned in household stoves are more than 2 orders of magnitude higher than those burned in industrial boilers and power plants (Li et al., 2017); thus, pollutants emitted from RCC have caused great concern in recent years.
In general, coals can be classified as anthracite or bituminous coals in the forms of raw chunks or briquettes (Shen, 2015), and they are burned in movable brick or cast-iron stoves that have been used for centuries in China (Shen et al., 2010). There have been many real-world measurements of particle emissions from RCC that have aimed to investigate the nature of these emissions (Chen et al., 2005). Most of these studies have focused on the emission factors rather than the chemical composition of the emissions, as the emission factors of RCC are highly uncertain. The chemical characteristics of RCC profiles vary greatly with respect to the sampling techniques. Three decades ago, Dai et al. (1987a) reported the averaged elemental profiles of 15 RCC particle samples in Tianjin (the samples were collected in 1985), using a Bahco analyzer to cut fly ash (collected from the stack of an RCC stove) into particles with an aerodynamic diameter of less than 12 µm. As expected, this sampling technique resulted in a high fraction of crustal elements in the chemical profile. The resuspension chamber has also been used to cut particle sizes from coal fly ash. However, the particles that are emitted from the stack are not coal fly ash. Thus, the accuracy of RCC source profiles has been improved since the introduction of DTSM in China. As shown in Fig. 6, the fractions of crustal elements (Mg, Al, Si, Ca, and Ti) in the profile measured from coal ash are an order of magnitude higher than those in the RCC profile sampled using the DTSM, whereas the fraction of , , and OC are 2–3 orders of magnitude lower in coal ash.
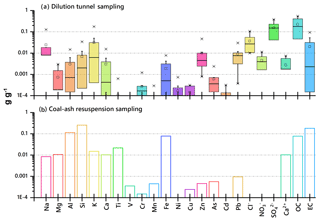
Figure 6RCC profiles of PM2.5 collected using the DTSM with (a) data collected from available published profiles (Ge et al., 2004; Kong, 2014; Liu et al., 2016, 2017; Yan et al., 2017a; Dai et al., 2019), and the coal fly ash RSM with (b) data collected from Wang et al., 2016.
Since the 1990s, much effort has been made at a national level to reduce pollutant emissions from RCC by introducing improved stoves and cleaner fuels, such as the China National Improved Stove Program (Shen et al., 2015). Highly efficient stoves are reported to likely reduce the emission load. However, given the limited available of data, it is not possible to compare the chemical profiles between the less and more efficient stoves in this work. It is also reported that the emission factors of air pollutants from RCC vary widely because of the variations in coal types and properties, stove types, and burning conditions (Shen et al., 2010). As shown in Fig. 7, PM2.5 emissions from the burning of chunk coals have high fractions of OC, EC, , , and , and low fractions of Na, Ca, and K (K+) than the burning of honeycomb briquette coals. Generally, OC and sulfur are the predominate species in PM2.5 emitted from RCC.
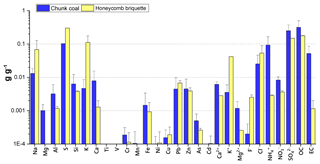
Figure 7RCC profiles of PM2.5 emissions from chunk coal and honeycomb briquette coals. Data were collated from published data (Ge et al., 2004; Kong, 2014; Liu et al., 2016, 2017; Yan et al., 2017b; Dai et al., 2019).
As we mentioned above, there are many factors that affect the profiles of CC sources. Therefore, local CC source profiles should be measured in the specific study area to improve the accuracy and reliability of source apportionment results.
2.2.2 Industrial process emissions
Industrial emissions are one of the most important PM sources in China (Zhu et al., 2018). Particles from industrial emissions are mainly collected using the DTSM (53 %). The source profiles of industrial emissions may be influenced by several key factors, such as the raw materials used in different industrial processes, manufacturing processes, the sampling method used, sample site variability, the control measures taken by different factories, and different operating conditions (Watson and Chow, 2001; Kong et al., 2011; Pant and Harrison, 2012; Guo et al., 2017). There are large differences between the source profiles from different industrial sources. Figure 8 shows the chemical composition of China's main industrial emissions (cement plants, coking plants, and steel plants) (Ma et al., 2015; Qi et al., 2015; Yan et al., 2016; Zhao et al., 2015a). For cement industrial sources, Ca, Al, OC, and are the most abundant species, with average values of more than 0.10 g g−1. For coking industrial sources, Ca2+, Al, and are elevated, whereas OC displays a somewhat notable lower level. For steel industrial sources, the highest fraction species are Fe, Si, K, and , whereas Cl−, Ca2+, EC, and OC show a lower average content of less than 0.0010 g g−1.
In China, there are many different types of industry with different emission characteristics. The source profiles of industrial emissions are currently far from being fully understood. The profiles of some important industrial sources, such as glass melt kilns, non-ferrous smelting, and ceramics, are rarely reported and require further investigation in the future.
2.2.3 Vehicle emissions
Vehicle emissions appear to be the predominant source of ambient PM2.5 in urban areas in China, particularly in megacities like Beijing and Shanghai (Cai et al., 2017b; Cui et al., 2016; Zhang et al., 2015). It has been reported that the contribution of vehicle emissions to PM2.5 is in the range of 5 % to 34 % throughout China based on receptor models (Zhang et al., 2017b). There are many factors affecting vehicle emissions, including fuel types, vehicle types, emission control technologies, operating conditions, engine performance, and sampling methods (Watson et al., 1990; Chen et al., 2017b; Maricq, 2007). The representativeness of the source profiles of vehicle emissions is often controversial. Figure 9 summarizes the PM10 source profiles of different vehicle types obtained using the direct sampling method in China (Chen et al., 2017b). For both diesel and gasoline vehicles, the emission profiles are dominated by OC, EC, , , , Ca, Fe, and Zn. The abundance of EC in diesel vehicle exhaust (particularly in heavy-duty diesel vehicle exhaust) is higher than that in the exhaust from gasoline vehicles, which may be due to the different combustion completion rates between diesel and gasoline on account of the length of the hydrocarbon chains in the fuels (Chen et al., 2017b). As Mn is used in gasoline as an explosion-proof agent, the fraction of Mn in the PM from gasoline vehicle emissions is higher than that in diesel vehicle emissions.
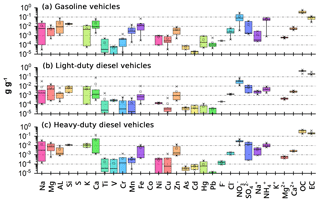
Figure 9Chemical compositions of PM10 source profiles from different vehicle types obtained using the direct sampling method. Data from the Nankai University source library and Chen et al. (2017b) were collated.
Figure S1 summarizes the characteristics of the chemical profiles of PM emitted from vehicles obtained using different sampling methods. Crustal elements (Si, Al, Ca, and Mn) in the chemical profiles obtained using SDSMs (source dominated sampling methods) are higher than those obtained using DSMs (direct sampling methods), which may be due to the influence of suspended road dust. and in chemical profiles obtained using DSM are lower than those obtained using SDSM, probably because their precursors are still in the gaseous state when the samples are collected at a higher temperature using DSM (Kong and Bai, 2013).
The source profiles of vehicle exhaust also vary due to upgrades to the fuel. In China, the oil used in vehicles has been upgraded five times over the past 18 years. The evolution of the fractions of Mn, Pb, and in PM emitted from vehicles over the past 3 decades is shown in Fig. 10. Pb was used as a tracer of gasoline before 2000; however, leaded gasoline was banned in mainland China in 2000, making Pb less prevalent (State Council of China, 1998). The standard value of sulfur in gasoline (for use in cars) was 800 µg g−1 in 2000 and 10 µg g−1 in 2018 (Guo, 2013). The standard value of Mn was 0.018 g L−1 in 2000 and only 0.002 g L−1 in 2018 (Li, 2016). A similar trend is also found with respect to the standard of diesel in China (Zhang et al., 2009a). These changes in the oil standard have definitely affected the evolution of the source profiles of vehicle exhaust emissions. Due to the government's request to stop producing, selling, and using leaded gasoline, the fraction of Pb in vehicle emissions decreased significantly. In 2005, the fraction of Pb in motor vehicle emissions had dropped significantly compared with values from 1985 (Dai et al., 1986; Han et al., 2009). The fraction of Mn also reduced significantly after 2000 (Bi et al., 2007; Han et al., 2009). Similarly, the fraction of in vehicle emissions has shown a significant decreasing trend since 2000, indicating a causal relationship with the reduction of sulfur in gasoline for automotive use in China.
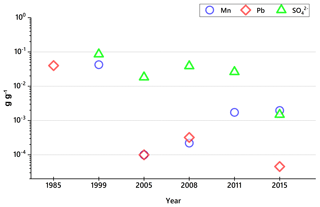
Figure 10Time series of Mn, Pb, and in PM emitted from vehicles. Data were collated from the Nankai University source library, Dai et al. (1986), Zhang et al. (2000), Bi et al. (2007), Han et al. (2009), Zhang et al. (2009a), Guo et al. (2013), and Li et al. (2016).
By comparing the main components of on-road vehicle PM2.5 source profiles derived from local studies and the EPA SPECIATE database, Xia et al. (2017) found that the source profiles of motor vehicles in both China and the US were dominated by OC and EC, although the proportions of these factors were different. In the US, gasoline, ethanol, and methanol are added as aerators, while the oxygen content of gasoline in China is lower than in the US; this is an important reason for the difference in the OC content in the spectrums at home (China) and abroad (Xia et al., 2017). In China, the fraction of is 2.4 times higher than that found in foreign motor vehicles (Wang et al., 2015; Xia et al., 2017), which may be related to the higher sulfur content in the fuels (Guo et al., 2013; Li et al., 2016).
2.2.4 Fugitive dust
Fugitive dust has been founded to be one of the major sources of urban PM (Chow et al., 2003; Kong et al., 2011; Cao et al., 2012; Zhu et al., 2018), especially in the northern cities in China which have dry climates and limited precipitation (Shen et al., 2016; Cao et al., 2008). Urban fugitive dust is not only influenced by soil properties and geographic location, but also by the mixture of various dust-related sources. Therefore, fugitive dust is often referred to as soil dust, road dust, and construction dust (Doskey et al., 1999; Kong et al., 2014). Fugitive dust samples are generally collected using resuspension chambers.
As shown in Fig. 11, the primary species found in soil dust are Si, Al, and Ca, with mass fractions ranging from 0.0500 to 0.2010 g g−1. Si is the predominant species among the detected elements, followed by Al, Fe, Na, and Mg. The main chemical components of road dust are Si, OC, and Ca, with fractions ranging from 0.0712 to 0.0855 g g−1. Al, Fe, and occur in lower concentrations (less than 0.0005 g g−1) in the chemical profiles of road dust. Si, Ca, Al, and Fe are all crustal elements, indicating that soil dust has a greater impact on the composition of road dust. It also shows that OC and values are higher in the source profiles of road dust than they are in the profiles of soil dust, indicating that road dust is also affected by vehicle emissions or coal combustion and other anthropogenic sources (Ma et al., 2015). In general, the total WSIs account for 0.0248–0.0648 g g−1 of the fugitive dust, suggesting that insoluble matter is not the main component of fugitive dust.
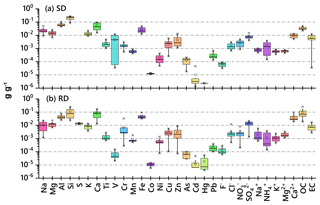
Figure 11Characteristics of chemical profiles for PM emitted from fugitive dust. SD and RD denote soil dust and road dust, respectively. Data were acquired from the Nankai University source library.
Many studies have demonstrated that the ratios of different chemical components can be used as markers for fugitive dust (Alfaro et al., 2003; Arimoto et al., 2004). Kong et al. (2011) found that the Ca ∕ Al ratio of paving road dust affected by construction activities was significantly different from that of soil dust. Zhang et al. (2014) reported that heavy metals such as Zn and Pb were capable of being used as tracers of urban fugitive dust, as the Zn ∕ Al and Pb ∕ Al ratios in urban fugitive dust were 1.5–5 times those found in the Gobi desert and in loess soil samples. The ratio has also been used to compare the relative importance of stationary sources vs. mobile sources. A much higher ratio for road dust was reported by Ho et al. (2003) in Hong Kong, revealing the more important impact of vehicle emissions on the chemical composition of road dust compared with coal combustion.
2.2.5 Biomass burning
Traditionally, China is an agriculture-based country (Bi et al., 2007). As an effective way to eliminate plant residues, direct combustion (by open burning and combustion in domestic stoves) is a predominant and popular practice during the harvest season (Andreae and Merlet, 2001; Ni et al., 2017; Cheng et al., 2013; Li et al., 2014b; Streets et al., 2003). However, this combustion releases a lot of pollutants into the ambient air, and subsequently impacts air quality, human health, and climate (Yao et al., 2017; Chen et al., 2017a). Biofuel burned using boilers is also an important subtype of biomass burning (Tian et al., 2017). Wheat straw, corn stalks, and rice straw represent 80 % of the agricultural combustion in China (Ni et al., 2017), in addition to other fuels such as firewood, soybean, and rape. The biofuel types, sampling procedures, and burning conditions result in large differences in the levels and chemical properties of PM emissions from biomass burning (Tian et al., 2017; Vicente and Alves, 2018).
Biomass is usually burned in three ways in China: open burning (OB), residential stove combustion (RSC), and biofuel boiler burning (BBB). At present, there are two popular ways to measure emissions from biomass burning: the field combustion experiment (FCE) and the laboratory combustion simulation (LCS) (Hays et al., 2005; Li et al., 2014a; Sanchis et al., 2014; De Zarate et al., 2000). Figure 12 summarizes the biomass burning profiles of PM2.5 obtained in China from the three abovementioned burning styles. The samples of biomass boiler exhaust are obtained using the RSM. The main components in the profiles of biomass burning are OC, EC, K+, Cl−, K, and Ca (Fig. 12). The fraction of EC is 4.2 times higher in BBB than in RSC, which is potentially due to the uneven mixing of the air in the biomass boiler that can easily cause straw to burn under anaerobic conditions (Tian et al., 2017). High EC emissions can also occur if high-temperature flame burning conditions are dominant during BBB. In comparison, the oxygen content is sufficient during OB, which leads to relatively higher OC emissions. Furthermore, the fraction of Ca is higher in BBB exhaust than in OB exhaust (Fig. 12). Regarding the emission of specific components from biomass burning, EC emissions from firewood combustion was the highest, which was likely due to the high combustion temperature, the dominance of flame burning conditions, and the higher content of lignin in the wood (Tang et al., 2014), as lignin facilitates the formation of black carbon (Wiinikka and Gebart, 2005).
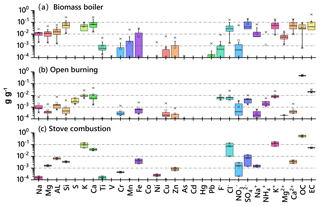
Figure 12Major chemical composition of PM2.5 source profiles of biomass burning. Data were acquired from the Nankai University source library.
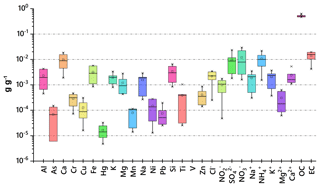
Figure 13PM2.5 chemical profiles of cooking emissions. Data were collated from the Nankai University source library, Zhang et al. (2017a), See et al. (2006), and Taner et al. (2013).
Chen et al. (2007) investigated the particulate emissions from wildland fuels burned in a laboratory combustion facility in the US, and found that the percentage of TC in PM was 63.7 %–100 %, which was higher than values found in China (4.9 %–68 %). K (0.4 %–23.7 %), Cl (0.1 %–9.6 %), and S (0.1 %–2.9 %) were also important components of the remaining PM mass in the US, which differed from Chinese profiles due to the different biomass categories and combustion processes.
2.2.6 Cooking emissions
Due to economic growth, cooking styles and food ingredients in China are becoming increasingly diverse. Since the 1990s, the variety of ingredients and cooking styles have also been influenced by foreign food culture. As China is famous for its diverse food culture, the cooking styles tend to vary by region, or even by city. Thus, cooking is undoubtedly an important local source of ambient particles. Given that there is no ubiquitous source profile for cooking emissions, it is better to measure the real-world source profile of cooking emissions in the specific study area. As one of the essential cooking ingredients in the food and beverage industry, the types of edible oils utilized in China have changed in recent years (Pei et al., 2016). Soybean oil, rapeseed oil, and peanut oil are common edible oils for public dining; however, due to changes in consumer demand, other types of edible oils, such as olive oil, camellia oil, and flaxseed oil, have also been increasingly welcomed by the catering industry. Furthermore, Chinese-style cooking is characterized by high-temperature stir-frying which releases much more OM than western-style cooking (Zhao et al., 2007b).
The chemical nature of PM2.5 emitted from commercial cooking has been investigated in many different studies, with the source profiles varying greatly due to factors such as cooking style, the food cooked, seed oils, and the fuel used (He et al., 2004; Zhao et al., 2007b, 2015b; Hou et al., 2008b; Pei et al., 2016). Robinson et al. (2006) found that the contribution of cooking emissions to OC in PM2.5, calculated using a chemical mass-balance model with different source profiles, yielded a difference of a factor of more than 9.
Previous studies have found that OM accounts for 66.9 % of the total suspended particle (TSP) mass emitted from cooking activities (Zhao et al., 2015b). OC is the major constituent and accounts for 36.2 %–42.9 % of the total mass, whereas the fraction of EC is much lower. Several WSIs measured in fine particles present a relatively lower but noticeable percentage, which comprises about 9.1 %–17.5 % of the total PM2.5 mass (Anwar et al., 2004). Inorganic elements are found to be 7.3 %–12.0 % of the total PM2.5 mass due to their greater presence in cooking oil and raw materials (He et al., 2004).
Figure 13 shows the PM2.5 chemical profiles of cooking emissions including hot pot, Chinese restaurants, barbecues, and cafeterias (See and Balasubramanian, 2006; Taner et al., 2013; Zhang et al., 2017a). Regarding elements, the most abundant element in cooking profiles, on average, is Al, followed by Ca, and Fe. The high levels of Ca and Fe are probably emitted from raw materials and cooking utensils (See and Balasubramanian, 2006; Taner et al., 2013). The high level of Cr, which originates from stainless-steel grills, was observed in a barbecue restaurant (Taner et al., 2013). Overall, OC is the most abundant species in CE profiles.
Organic matter (OM) is the predominant species in PM2.5 emitted from cooking activities (He et al., 2004; Hou et al., 2008a; Pei et al., 2016). Many organic compounds, including n-alkanes, dicarboxylic acids, polycyclic aromatic hydrocarbons (PAHs), saturated fatty acids, and unsaturated fatty acids, have been quantified in the abovementioned studies. Figure 14 shows the fractions of the main organic compounds in the quantified OM emissions from residential cooking (Zhao et al., 2015b) and commercial cooking (Pei et al., 2016). Among the quantified organic compounds, the predominant species are the unsaturated fatty acids (49.4 %–77.8 %), followed by saturated fatty acids (25.1 %–43.8 %).
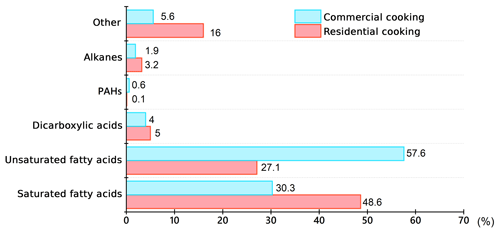
Figure 14Proportions of major organic compounds in quantified OM emission from commercial cooking (Pei et al., 2016) and residential cooking (Zhao et al., 2015b). PAHs refer to polycyclic aromatic hydrocarbons.
In addition, except for biomass burning, many studies have reported that levoglucosan was also founded in the emissions from RCC (Yan et al., 2017b) and a variety of Chinese and western cooking styles (He et al., 2004; Zhao et al., 2007a, b). Furthermore, Pei et al. (2016) found that Italian-style cooking released the smallest amount of monosaccharide anhydrides and the largest amount of cholesterol due to the lower ratio of vegetables to meat compared with Chinese cooking materials. Malay cooking released higher PAH concentrations than Chinese and Indian cooking methods (See et al., 2006). Deep frying emitted more PAHs than other cooking methods due to the higher temperature and the use of more oil during cooking. To our knowledge, molecular markers used for cooking included levoglucosan, galactosan, and cholesterol (He et al., 2004; Zhao et al., 2007a, b), although cholesterol can be regarded as the best marker for meat cooking (Schauer et al., 1999, 2002; Schauer and Cass, 2000).
2.3 Statistical analysis of the source categories
The chemical profile of a given source category is always established from the profiles of several similar sources belonging to the category. Non-negligible uncertainties would be introduced during this process. To evaluate such uncertainties, the coefficient of variation (CV, the standard deviation divided by the mean) is used in this section to further characterize the homogeneity of the sources within the same source category (Fig. 15).
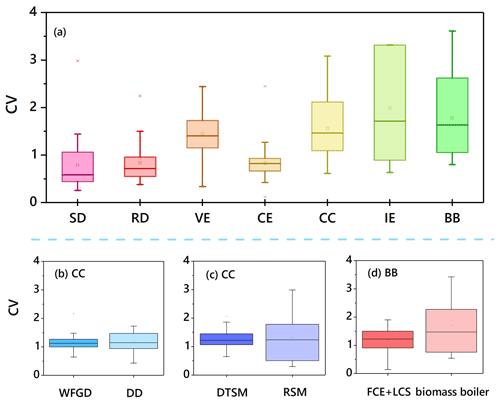
Figure 15Coefficients of variation (CV) calculated for each source category. SD denotes soil dust, RD denotes road dust, VE denotes vehicle emissions, CE denotes cooking emissions, CC denotes coal combustion, IE denotes industrial emissions, BB denotes biomass burning, WFGD denotes wet flue gas desulfurization, DD denotes dry desulfurization, DTSM denotes the dilution tunnel sampling method, RSM denotes the resuspension sampling method, FCE denotes field combustion experiment, and LCS denotes laboratory combustion simulation.
CV values above three (Pernigotti et al., 2016) are observed in CC, IE, and BB, indicating that these source profiles show great variation due to the effects of their influencing factors as described in the preceding sections. The road dust (RD) and soil dust (SD) profiles show less variation, with stable chemical characteristics among the different profiles in the same category. However, the responses of source profiles to various impact factors are different (Fig. 15b–d). For example, the sampling methods have a notable effect on the CC source profile (the variation of coal combustion source profiles obtained by RSM is greater than that obtained using the DTSM), whereas the desulfurization methods have a smaller impact.
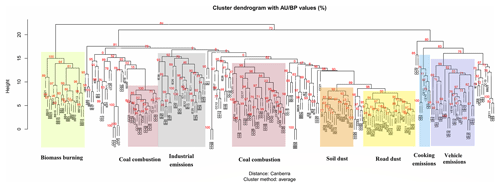
Figure 16Result of the cluster analysis on the collated profiles. AU (approximated unbiased) p values are reported in red as percentages. BP refers to the bootstrap probability.
As source profiles have a local characteristic, it is important and necessary to establish and update local source profiles to reveal the real-world source emission situation (Zhang et al., 2017b; Zhu et al., 2018). However, local source profiles are not always available in developing areas due to factors such as limited funds or lack of instruments. According to the abovementioned statistical results, it can be inferred that the profiles of RD and SD could be used as references for cities in China that do not have local profiles; however, it is still necessary to establish local profiles for industrial emissions, vehicle emissions, coal combustion, and biomass burning.
In order to investigate the similarity of the real-world (measured) source profiles with homogeneous chemical signatures, a cluster analysis was applied to the collated data using the “pvclust” R package (Suzuki and Shimodaira, 2006; Pernigotti et al., 2016). The significance test was performed by resampling the data using the bootstrap method. This function is expected to assign each cluster an approximated unbiased (AU) p value by hierarchic clustering (Shimodaira, 2002). Details regarding the operational steps of this method have been discussed by Pernigotti et al. (2016). The input source profiles involved in the cluster calculation must contain more than two common chemical species, including elements, ions, and OC ∕ EC. In order to reduce the interference from different particle sizes, we used 226 source profiles of PM2.5 for the calculation. The result of the cluster analysis and additional information with respect to the source profiles are shown in Fig. 16 and Table S1. As shown in Fig. 16, clusters are marked if the AU p value ≥90 (values are reported in red). Figure 16 shows that the source profiles are divided into (1) biomass burning, (2) and (4) coal combustion, (3) industrial emissions, (5) soil dust, (6) road dust, (7) cooking emissions, and (8) vehicle emissions. These subjectively measured profiles are successfully classified by an objective approach based on their chemical nature, although some sources are misclassified (Fig. 16). This result indicates that the routinely measured components are not sufficient to distinguish all of the source categories when chemically colinear sources exist. Both the cooking and vehicle emission source profiles are characterized by high OC levels, which causes them to be easily identified as the same source type. The chemical collinearity of the source composition between coal combustion and dust also makes them difficult to distinguish from one another. To solve the chemical colinearity problem between sources, more specific tracers, especially organics, should be further explored.
Chemical profiles of the main PM sources have been established in China since the 1980s. With the development of sampling and analysis techniques, the source profile data set has been gradually enlarged and may be able to reflect the real emissions of the sources to the ambient air. A total of 456 published source profiles, coupled with the database of source profiles (2870 profiles) founded by Nankai University are reviewed in this work. Six source categories including coal combustion, industrial process emissions, vehicle emissions, fugitive dust, biomass burning, and cooking emissions are investigated to characterize the chemical nature of sources and to explore the main factors influencing the chemical composition.
In general, coal combustion is the most complicated source category, as it is influenced by many factors from fuel combustion processes to pollution-control processes. is the predominant species of fine-particle emissions from coal combustion stationary sources equipped with wet flue gas desulfurization devices, and is also the second largest species in fine-particle emissions from RCC. The source profiles of industrial emissions are mainly determined by the components of the industrial products and the pollution-control techniques. Due to the changing standards of gasoline and diesel oil since the 1980s, Pb and Mn are no longer tracers of gasoline vehicle emissions. However, OC and EC have been the dominant species in vehicle emissions since the 1980s, despite the changing standards. The profiles for fugitive dust, including the road dust and soil dust, are characterized by high levels of crustal elements, such as Si, Al, and Ca. The profiles of the biomass burning are determined by the biomass categories and the different combustion phases (smoldering and flaming), with K+ and levoglucosan as the common tracers. With respect to cooking emissions, the source profiles of the emissions from the different cooking types are all dominated by OC.
The results of the uncertainty analysis showed that the relatively large variation in the source profiles for industry emissions, vehicle emissions, coal combustion, and biomass burning, call for the establishment of local profiles for these sources (due to their high uncertainties). In comparison, the profiles of road dust and soil dust showed less variation, suggesting that the profiles of these sources could be utilized as reference profiles for cities in China where local profiles are not available. As source profiles have local characteristics, it is important and necessary to establish local source profiles to reveal the real situation with respect to source emissions, and to immediately update existing profiles.
Cluster analysis results regarding the routinely measured species in the source profiles suggest that some of the sources are difficult to distinguish from others (cooking emissions vs. vehicle emissions); this indicates that more chemical tracers, such as isotopes and organic compounds, should be explored in order to reduce the collinearity among different source profiles. The current source profile database is still missing some important source categories, which have significant impacts on air quality, and is lacking sufficient source profiles, especially with respect to industrial emissions, such as glass melt kilns, nonferrous metal smelting, and brick and tile kilns. Thus, specific focus should be placed on these important, but overlooked, sources in the future.
The chemical composition data for the main sources used in this publication were deposited to the Mendeley data repository and can be freely downloaded from https://doi.org/10.17632/x8dfshjt9j.2 (Bi et al., 2019).
The supplement related to this article is available online at: https://doi.org/10.5194/acp-19-3223-2019-supplement.
XB performed the data analysis and wrote the paper. YF assisted greatly with the paper development and editing. QD contributed to the data analysis. QZ, WZ, RL, YC, JZ, LW, and ZY contributed to the data collection. JW, YZ, and YT provided helpful comments and edits.
The authors declare that they have no conflict of interest.
This work was financially supported by the National Key R&D Program of
China (grant no. 2016YFC0208501) and the Fundamental Research Funds for the
Central Universities of China. The authors thank Jing Ding, Xian Ma, Jiamei
Yang, Tingkun Li, Jinsheng Zhang, Xin Du, Baoshuang Liu, Ming Zhou, and other
students in the research group for their assistance with the source sampling
and post-chemical analyses related to this work.
Edited by: Qiang Zhang
Reviewed by: four anonymous referees
Alfaro, S. C., Gomes, L., Rajot, J. L., Lafon, S., Gaudichet, A., Chatenet, B., Maille, M., Cautenet, G., Lasserre, F., Cachier, H., and Zhang, X. Y.: Chemical and optical characterization of aerosols measured in spring 2002 at the ACE-Asia supersite, Zhenbeitai, China, J. Geophys. Res.-Atmos., 108, 8641, https://doi.org/10.1029/2002jd003214, 2003.
Amato, F., Pandolfi, M., Escrig, A., Querol, X., Alastuey, A., Pey, J., Perez, N., and Hopke, P. K.: Quantifying road dust resuspension in urban environment by Multilinear Engine: a comparison with PMF2, Atmos. Environ., 43, 2770–2780, 2009.
Andreae, M. O. and Merlet, P.: Emission of trace gases and aerosols from biomass burning, Global Biogeochem. Cy., 15, 955–966, https://doi.org/10.1029/2000gb001382, 2001.
Anwar, F., Kazi, T. G., Saleem, R., and Bhanger, M. I.: Rapid determination of some trace metals in several oils and fats, Grasas Aceites, 55, 160–168, 2004.
Arimoto, R., Zhang, X. Y., Huebert, B. J., Kang, C. H., Savoie, D. L., Prospero, J. M., Sage, S. K., Schloesslin, C. A., Khaing, H. M., and Oh, S. N.: Chemical composition of atmospheric aerosols from Zhenbeitai, China, and Gosan, South Korea, during ACE-Asia, J. Geophys. Res.-Atmos., 109, D19S04, https://doi.org/10.1029/2003jd004323, 2004.
Bandowe, B. A. M., Meusel, H., Huang, R., Hoffmann, T., Cao, J., and Ho, K.: Azaarenes in fine particulate matter from the atmosphere of a Chinese megacity, Environ. Sci. Pollut. R., 23, 16025–16036, https://doi.org/10.1007/s11356-016-6740-z, 2016.
Bi, X., Dai, Q., Wu, J.-h., Zhang, Q., Zhang, W., Luo, R., Cheng, Y., Zhang, J., Wang, L., Yu, Z., Zhang, Y., Tian, Y., and Feng, Y.: Data for: chemical compositions of the main source profiles of ambient particulate matter across China, Mendeley Data, v2, https://doi.org/10.17632/x8dfshjt9j.2, 2019.
Bi, X. H., Feng, Y. C., Wu, J. H., Wang, Y. Q., and Zhu, T.: Source apportionment of PM10 in six cities of northern China, Atmos. Environ., 41, 903–912, https://doi.org/10.1016/j.atmosenv.2006.09.033, 2007.
Cai, T. Q., Zhang, Y., Fang, D. Q., Shang, J., Zhang, Y. X., and Zhang, Y. H.: Chinese vehicle emissions characteristic testing with small sample size: Results and comparison, Atmos. Pollut. Res., 8, 154–163, https://doi.org/10.1016/j.apr.2016.08.007, 2017.
Cao, J. J., Chow, J. C., Watson, J. G., Wu, F., Han, Y. M., Jin, Z. D., Shen, Z. X., and An, Z. S.: Size-differentiated source profiles for fugitive dust in the Chinese Loess Plateau, Atmos. Environ., 42, 2261–2275, https://doi.org/10.1016/j.atmosenv.2007.12.041, 2008.
Cao, J. J., Shen, Z. X., Chow, J. C., Watson, J. G., Lee, S. C., Tie, X. X., Ho, K. F., Wang, G. H., and Han, Y. M.: Winter and Summer PM2.5 Chemical Compositions in Fourteen Chinese Cities, J. Air Waste Manage., 62, 1214–1226, https://doi.org/10.1080/10962247.2012.701193, 2012.
Chen, L. W. A., Moosmuller, H., Arnott, W. P., Chow, J. C., Watson, J. G., Susott, R. A., Babbitt, R. E., Wold, C. E., Lincoln, E. N., and Hao, W. M.: Emissions from laboratory combustion of wildland fuels: Emission factors and source profiles, Environ. Sci. Technol., 41, 4317–4325, https://doi.org/10.1021/es062364i, 2007.
Chen, J. M., Li, C. L., Ristovski, Z., Milic, A., Gu, Y. T., Islam, M. S., Wang, S. X., Hao, J. M., Zhang, H. F., He, C. R., Guo, H., Fu, H. B., Miljevic, B., Morawska, L., Thai, P., Fat, L. A. M. Y., Pereira, G., Ding, A. J., Huang, X., and Dumka, U. C.: A review of biomass burning: Emissions and impacts on air quality, health and climate in China, Sci. Total. Environ., 579, 1000–1034, https://doi.org/10.1016/j.scitotenv.2016.11.025, 2017a.
Chen, P. L., Wang, T. J., Dong, M., Kasoar, M., Han, Y., Xie, M., Li, S., Zhuang, B. L., Li, M. M., and Huang, T. N.: Characterization of major natural and anthropogenic source profiles for size-fractionated PM in Yangtze River Delta, Sci. Total Environ., 598, 135–145, https://doi.org/10.1016/j.scitotenv.2017.04.106, 2017b.
Chen, Y. J., Bi, X. H., Mai, B. X., Sheng, G. Y., and Fu, J. M.: Emission characterization of particulate/gaseous phases and size association for polycyclic aromatic hydrocarbons from residential coal combustion, Fuel, 83, 781–790, 2004.
Chen, Y., Sheng, G., Bi, X., Feng, Y., Bixian Mai, A., and Fu, J.: Emission Factors for Carbonaceous Particles and Polycyclic Aromatic Hydrocarbons from Residential Coal Combustion in China, Environ. Sci. Technol., 39, 1861–1867, 2005.
Cheng, H. F. and Hu, Y. A.: Lead (Pb) isotopic fingerprinting and its applications in lead pollution studies in China: A review, Environ. Pollut., 158, 1134–1146, https://doi.org/10.1016/j.envpol.2009.12.028, 2010.
Cheng, S. Y., Wang, G., Lang, J. L., Wen, W., Wang, X. Q., and Yao, S.: Characterization of volatile organic compounds from different cooking emissions, Atmos. Environ., 145, 299–307, https://doi.org/10.1016/j.atmosenv.2016.09.037, 2016.
Cheng, Y., Engling, G., He, K.-B., Duan, F.-K., Ma, Y.-L., Du, Z.-Y., Liu, J.-M., Zheng, M., and Weber, R. J.: Biomass burning contribution to Beijing aerosol, Atmos. Chem. Phys., 13, 7765–7781, https://doi.org/10.5194/acp-13-7765-2013, 2013.
China Energy Statistical Yearbook (CESY), National Bureau of Statistics, China Energy Statistical Yearbook 2015, China Statistics Press, Beijing, China, 2015.
Chow, J. C., Watson, J. G., Houck, J. E., Pritchett, L. C., Rogers, C. F., Frazier, C. A., Egami, R. T., and Ball, B. M.: A laboratory resuspension chamber to measure fugitive dust size distributions and chemical compositions, Atmos. Environ., 28, 3463–3481, 1994.
Chow, J. C., Watson, J. G., Ashbaugh, L. L., and Magliano, K. L.: Similarities and differences in PM10 chemical source profiles for geological dust from the San Joaquin Valley, California, Atmos. Environ., 37, 1317–1340, https://doi.org/10.1016/S1352-2310(02)01021-X, 2003.
Chow, J. C., Watson, J. G., Kuhns, H., Etyemezian, V., Lowenthal, D. H., Crow, D., Kohl, S. D., Engelbrecht, J. P., and Green, M. C.: Source profiles for industrial, mobile, and area sources in the Big Bend Regional Aerosol Visibility and Observational study, Chemosphere, 54, 185–208, https://doi.org/10.1016/j.chemosphere.2003.07.004, 2004.
Cooper, J. A. and Watson, J. G.: Receptor oriented methods of air particulate source apportionment, JAPCA J. Air Waste Ma., 30, 1116–1125, 1980.
Cui, M., Chen, Y. J., Tian, C. G., Zhang, F., Yan, C. Q., and Zheng, M.: Chemical composition of PM2.5 from two tunnels with different vehicular fleet characteristics, Sci. Total Environ., 550, 123–132, https://doi.org/10.1016/j.scitotenv.2016.01.077, 2016.
Dai, Q. L., Bi, X. H., Huangfu, Y. Q., Yang, J. M., Li, T. K., Jahan, Z. K., Song, C. B., Xu, J., Wu, J. H., Zhang, Y. F., and Feng, Y. C.: A size-resolved chemical mass balance (SR-CMB) approach for source apportionment of ambient particulate matter by single element analysis, Atmos. Environ., 197, 45–52, 2019.
Dai, S. G., Zhu, T., Zeng, Y. S., Fu, X. Q., and Miao, Y. M.: Source apportionment for Tianjin urban aerosol in heating season, Chinese Environmental Science, 6, 24–30, 1986 (in Chinese).
Dai, S. G., Zhu, T., Zeng, Y. S., Fu, X. Q., and Liao, Y. M.: Composition characteristics of industrial and residential coal smoke in Tianjin, Environm. Sci., 8, 18–23, 1987a.
Dai, S. G., Zhu, T., Zeng, Y. S., Fu, X. Q., and Miao, Y. M.: Study on the chemical characteristics of industrial and commercial coal in Tianjin, Environm. Sci., 4, 18–23, https://doi.org/10.13227/j.hjkx.1987.04.004, 1987b (in Chinese).
De Zarate, I. O., Ezcurra, A., Lacaux, J. P., and Van Dinh, P.: Emission factor estimates of cereal waste burning in Spain, Atmos. Environ., 34, 3183–3193, 2000.
Doskey, P. V., Fukui, Y., Sultan, M., Al Maghraby, A., and Taher, A.: Source profiles for nonmethane organic compounds in the atmosphere of Cairo, Egypt, J. Air Waste Manage., 49, 814–822, https://doi.org/10.1080/10473289.1999.10463850, 1999.
Duan, X. L., Jiang, Y., Wang, B. B., Zhao, X. G., Shen, G. F., Cao, S. Z., Huang, N., Qian, Y., Chen, Y. T., and Wang, L. M.: Household fuel use for cooking and heating in China: Results from the first Chinese Environmental Exposure-Related Human Activity Patterns Survey (CEERHAPS), Appl. Energ., 136, 692–703, 2014.
Energy Research Institute of China (ERI): Roadmap Study on Achieving Technical Energy Conservation Potential in China's Industrial Sector by 2020, China Scientific Technology Press, Beijing, 2013.
England, G. C., Zielinska, B., and Loos, K.: Characterizing PM2.5 emission profiles for stationary sources: comparison of traditional and dilution sampling techniques, Fuel Process. Technol., 65, 177–188, 2000.
Ferge, T., Maguhn, J., Felber, H., and Zimmermann, R.: Particle collection efficiency and particle re-entrainment of an electrostatic precipitator in a sewage sludge incineration plant, Environ. Sci. Technol., 38, 1545–1553, 2004.
Gallon, C. L., Tessier, A., Gobeil, C., and Beaudin, L.: Sources and chronology of atmospheric lead deposition to a Canadian Shield lake: Inferences from Pb isotopes and PAH profiles, Geochim. Cosmochim. Ac., 69, 3199–3210, https://doi.org/10.1016/j.gca.2005.02.028, 2005.
Ge, S., Bai, Z. P., and Liu, W. L.: Boiler briquette coal versus raw coal: Part I-stack gas emissions, J. Air Waste Manage., 51, 524–533, 2001.
Ge, S., Xu, X., and Chow, J, C.: Emissions of Air Pollutants from Household Stoves: Honeycomb Coal versus Coal Cake, Environ. Sci. Technol., 38, 4612–4618, 2004.
Guo, S.: Status analysis and development suggestions of upgrading gasoline quality in China, Petroleum Products Application Research, Shanghai Institute of Petroleum Commodity Application, Shanghai, 31, 4–11, 2013 (in Chinese).
Guo, Y. Y., Gao, X., Zhu, T. Y., Luo, L., and Zheng, Y.: Chemical profiles of PM emitted from the iron and steel industry in northern China, Atmos. Environ., 150, 187–197, https://doi.org/10.1016/j.atmosenv.2016.11.055, 2017.
Han, B., Feng, Y. C., Bi, X. H., Xue, Y. H., Wu, J. H., Zhu, T., Ding, J. Q., and Du, Y. X.: Source Apportionment of Ambient PM10 in Urban Area of Wuxi City, Research of Environmental Sciences, 22, 37–41, https://doi.org/10.13198/j.res.2009.01.37.hanb.005, 2009 (in Chinese).
Han, X. K., Guo, Q. J., Liu, C. Q., Fu, P. Q., Strauss, H., Yang, J. X., Hu, J., Wei, L. F., Ren, H., Peters, M., Wei, R. F., and Tian, L.Y.: Using stable isotopes to trace sources and formation processes of sulfate aerosols from Beijing, China, Sci. Rep., 6, 29958, https://doi.org/10.1038/srep29958, 2016.
Hays, M. D., Fine, P. M., Geron, C. D., Kleeman, M. J., and Gullett, B. K.: Open burning of agricultural biomass: Physical and chemical properties of particle-phase emissions, Atmos. Environ., 39, 6747–6764, https://doi.org/10.1016/j.atmosenv.2005.07.072, 2005.
He, L. Y., Hu, M., Huang, X. F., Yu, B. D., Zhang, Y. H., and Liu, D. Q.: Measurement of emissions of fine particulate organic matter from Chinese cooking, Atmos. Environ., 38, 6557–6564, https://doi.org/10.1016/j.atmosenv.2004.08.034, 2004.
Hildemann, L. M., Cass, G. R., and Markowski, G. R.: A Dilution Stack Sampler for Collection of Organic Aerosol Emissions: Design, Characterization and Field Tests, Aerosol Sci. Tech., 10, 193–204, 1989.
Ho, K. F., Lee, S. C., Chow, J. C., and Watson, J. G.: Characterization of PM10 and PM2.5 source profiles for fugitive dust in Hong Kong, Atmos. Environ., 37, 1023–1032, 2003.
Hopke, P. K.: Review of receptor modeling methods for sourceapportionment, J. Air Waste Manage., 66, 237–259, https://doi.org/10.1080/10962247.2016.1140693, 2016.
Hou, X. M., Zhuang, G. S., Lin, Y. F., Li, J., Jiang, Y. L., and Fu, J. S.: Emission of fine organic aerosol from traditional charcoal broiling in China, J. Atmos. Chem., 61, 119–131, https://doi.org/10.1007/s10874-009-9128-3, 2008b.
Houck, J. E., Cooper, J. A., and Larson, E. R.: Paper No. 82-61M.2 presented at 75th Annual Meeting of the Air Pollution Control Association, 20–25 June, New Orleans, 1982.
Junninen, H., Monster, J., Rey, M., Cancelinha, J., Douglas, K., Duane, M., Forcina, V., Muller, A., Lagler, F., Marelli, L., Borowiak, A., Niedzialek, J., Paradiz, B., Mira-Salama, D., Jimenez, J., Hansen, U., Astorga, C., Stanczyk, K., Viana, M., Querol, X., Duvall, R. M., Norris, G. A., Tsakovski, S., Wahlin, P., Horak, J., and Larsen, B. R.: Quantifying the Impact of Residential Heating on the Urban Air Quality in a Typical European Coal Combustion Region, Environ. Sci. Technol., 43, 7964–7970, https://doi.org/10.1021/es8032082, 2009.
Kauppinen, E. I., Lind, T. M., Eskelinen, J. J., Jokiniemi, J. K., Maenhaut, W., Royset, O., Vadset, M., Vilokki, H., and Kuivalainen, R.: Aerosols from circulating fluidized bed coal combustion, J. Aerosol Sci., 22, S467–S470, 1991.
Kong, S. F., Ji, Y. Q., Lu, B., Chen, L., Han, B., Li, Z. Y., and Bai, Z. P.: Characterization of PM10 source profiles for fugitive dust in Fushun-a city famous for coal, Atmos. Environ., 45, 5351–5365, https://doi.org/10.1016/j.atmosenv.2011.06.050, 2011.
Kong, S. F. and Bai, Z. P.: Progress on the Composition Profiles for Particulate Matter from Vehicle Emission in Source Apportionment, Environ. Sci. Technol., 10, 26–33, 2013 (in Chinese).
Kong, S. F., Ji, Y. Q., Lu, B., Zhao, X. Y., Han, B., and Bai, Z. P.: Similarities and Differences in PM2.5, PM10 and TSP Chemical Profiles of Fugitive Dust Sources in a Coastal Oilfield City in China, Aerosol Air Qual. Res., 14, 2017-2028, https://doi.org/10.4209/aaqr.2013.06.0226, 2014.
Lee, J. J., Engling, G., Lung, S. C. C., and Lee, K. Y.: Particle size characteristics of levoglucosan in ambient aerosols from rice straw burning, Atmos. Environ., 42, 8300–8308, https://doi.org/10.1016/j.atmosenv.2008.07.047, 2008.
Li, G. H.: Contents of Sulfur and Hydrocarbons in Commercial Available Gasoline and Diesel Oils Sold in China, Journal of University of Chinese Academy of Sciences, 25–28, 2016 (in Chinese).
Li, J., Song, Y., Mao, Y., Mao, Z., Wu, Y., Li, M., Huang, X., He, Q., and Hu, M.: Chemical characteristics and source apportionment of PM2.5 during the harvest season in eastern China's agricultural regions, Atmos. Environ., 92, 442–448, 2014a.
Li, J. F., Song, Y., Mao, Y., Mao, Z. C., Wu, Y. S., Li, M. M., Huang, X., He, Q. C., and Hu, M.: Chemical characteristics and source apportionment of PM2.5 during the harvest season in eastern China's agricultural regions, Atmos. Environ., 92, 442–448, https://doi.org/10.1016/j.atmosenv.2014.04.058, 2014b.
Li, Q., Jiang, J. K., Wang, S. X., Krassi, R., Ryan, M. H., Lidia, M., and Hao, J. M.: Impacts of household coal and biomass combustion on indoor and ambient air quality in China: Current status and implication, Sci. Total Environ., 576, 347–361, 2017.
Li, X., Wang, S., Duan, L., Hao, J., and Nie, Y.: Carbonaceous aerosol emissions from household biofuel combustion in China, Environ. Sci. Technol., 43, 6076–6081, 2009.
Lind, T., Hokkinen, J., Jokiniemi, J. K., Saarikoski, S., and Hillamo, R.: Electrostatic precipitator collection efficiency and trace element emissions from co-combustion of biomass and recovered fuel in fluidized-bed combustion, Environ. Sci. Technol., 37, 2842–2846, 2003.
Liu, B. S., Yang, J. M., Yuan, J., Wang, J., Dai, Q. L., Li, T. K., Bi, X. H., Feng, Y. C., Xiao, Z. M., Zhang, Y. F., and Xu, H.: Source apportionment of atmospheric pollutants based on the online data by using PMF and ME2 models at a megacity, China, Atmos. Res., 185, 22–31, 2017.
Liu, J., Mauzerall, D. L., Chen, Q., Zhang, Q., Song, Y., Peng, W., Klimont, Z., Qiu, X. H., Zhang, S. Q., Hu, M., Lin, W. L., Smith, K. R., and Zhu, T.: Air pollutant emissions from Chinese households: A major and underappreciated ambient pollution source, P. Natl. Acad. Sci. USA, 113, 7756–7761, https://doi.org/10.1073/pnas.1604537113, 2016.
Liu, Y. Y., Zhang, W. J., Bai, Z. P., Yang, W., Zhao, X. Y., Han, B., and Wang, X. H.: China Source Profile Shared Service (CSPSS): The Chinese PM2.5 Database for Source Profiles, Aerosol Air Qual. Res., 17, 1501–1514, https://doi.org/10.4209/aaqr.2016.10.0469, 2017.
Lu, D. W., Liu, Q., Yu, M., Yang, X. Z., Fu, Q., Zhang, X. S., Mu, Y. J., and Jiang, G. B.: Natural Silicon Isotopic Signatures Reveal the Sources of Airborne Fine Particulate Matter, Environ. Sci. Technol., 52, 1088–1095, https://doi.org/10.1021/acs.est.7b06317, 2018.
Ma, Z., Liang, Y., Zhang, J., Zhang, D., Shi, A., Hu, J., Lin, A., Feng, Y., Hu, Y., and Liu, B.: PM2.5 profiles of typical sources in Beijing, Acta Scientiae Circumstantiae, 35, 4043–4052, https://doi.org/10.13671/j.hjkxxb.2015.0584, 2015 (in Chinese).
Maricq, M. M.: Chemical characterization of particulate emissions from diesel engines: A review, J. Aerosol Sci., 38, 1079–1118, https://doi.org/10.1016/j.jaerosci.2007.08.001, 2007.
Meij, R.: Trace element behavior in coal-fired power plants, Fuel Process. Technol., 39, 199–217, 1994.
Meij, R. and Winkel, H. T.: The emissions and environmental impact of PM10 and trace elements from a modern coal-fired power plant equipped with ESP and wet FGD, Fuel Process. Technol., 85, 641–656, https://doi.org/10.1016/j.fuproc.2003.11.012, 2004.
Miller, M. S., Friedlander, S. K., and Hidy, G. M.: A chemical element balance for the Pasadena aerosol, J. Colloid Interf. Sci., 39, 165–176, https://doi.org/10.1016/0021-9797(72)90152-X, 1972.
Ni, H. Y., Tian, J., Wang, X. L., Wang, Q. Y., Han, Y. M., Cao, J. J., Long, X., Chen, L. W. A., Chow, J. C., Watson, J. G., Huang, R. J., and Dusek, U.: PM2.5 emissions and source profiles from open burning of crop residues, Atmos. Environ., 169, 229–237, https://doi.org/10.1016/j.atmosenv.2017.08.063, 2017.
Pan, Y. P., Tian, S. L., Liu, D. W., Fang, Y. T., Zhu, X. Y., Zhang, Q., Zheng, B., Michalski, G., and Wang, Y. S.: Fossil Fuel Combustion-Related Emissions Dominate Atmospheric Ammonia Sources during Severe Haze Episodes: Evidence from 15N-Stable Isotope in Size-Resolved Aerosol Ammonium, Environ. Sci. Technol., 50, 8049–8056, https://doi.org/10.1021/acs.est.6b00634, 2016.
Pant, P. and Harrison, R. M.: Critical review of receptor modelling for particulate matter: A case study of India, Atmos. Environ., 49, 1–12, https://doi.org/10.1016/j.atmosenv.2011.11.060, 2012.
Pei, B., Cui, H. Y., Liu, H., and Yan, N. Q.: Chemical characteristics of fine particulate matter emitted from commercial cooking, Front. Env. Sci. Eng., 10, 559–568, https://doi.org/10.1007/s11783-016-0829-y, 2016.
Pernigotti, D., Belis, C. A., and Spano, L.: SPECIEUROPE: The European data base for PM source profiles, Atmos. Pollut. Res., 7, 307–314, https://doi.org/10.1016/j.apr.2015.10.007, 2016.
Phuah, C. H., Peterson, M. R., Richards, M. H., Turner, J. R., and Dillner, A. M.: A Temperature Calibration Procedure for the Sunset Laboratory Carbon Aerosol Analysis Lab Instrument, Aerosol Sci. Tech., 43, 1013–1021, 2009.
Qi, K., Dai, C. L., Feng, Y., and Yang L.: Establishment and analysis of PM2.5 industrial source profiles in Shijiazhuang City, Hebei, Journal of Industrial Science and Technology, 32, 78–84, 2015 (in Chinese).
Qu, Z.: Setup of the component spectrum for source apportionment of PM2.5 in Urban Atmosphere, Jilin University, Changchun City, China, 2013 (in Chinese).
Reff, A., Bhave, P. V., Simon, H., Pace, T. G., Pouliot, G. A., Mobley, J. D., and Houyoux, M.: Emissions inventory of PM2.5 trace elements across the United States, Environ. Sci. Technol., 43, 5790–5796, 2009.
Robinson, A. L., Subramanian, R., Donahue, N. M., Bernardo-Bricker, A., and Rogge, W. F.: Source apportionment of molecular markers and organic aerosol. 3. Food cooking emissions, Environ. Sci. Technol., 40, 7820–7827, https://doi.org/10.1021/es060781p, 2006.
Sanchis, E., Ferrer, M., Calvet, S., Coscolla, C., Yusa, V., and Cambra-Lopez, M.: Gaseous and particulate emission profiles during controlled rice straw burning, Atmos. Environ., 98, 25–31, https://doi.org/10.1016/j.atmosenv.2014.07.062, 2014.
Schauer, J. J. and Cass, G. R.: Source apportionment of wintertime gas-phase and particle-phase air pollutants using organic compounds as tracers, Environ. Sci. Technol., 34, 1821–1832, https://doi.org/10.1021/es981312t, 2000.
Schauer, J. J., Kleeman, M. J., Cass, G. R., and Simoneit, B. R. T.: Measurement of emissions from air pollution sources. 1. C1 through C29 organic compounds from meat charbroiling, Environ. Sci. Technol., 33, 1566–1577, https://doi.org/10.1021/Es980076j, 1999.
Schauer, J. J., Kleeman, M. J., Cass, G. R., and Simoneit, B. R. T.: Measurement of emissions from air pollution sources. 4. C1-C27 organic compounds from cooking with seed oils, Environ. Sci. Technol., 36, 567–575, https://doi.org/10.1021/es002053m, 2002.
See, S. W. and Balasubramanian, R.: Risk assessment of exposure to indoor aerosols associated with Chinese cooking, Environ. Res., 102, 197–204, https://doi.org/10.1016/j.envres.2005.12.013, 2006.
See, S. W., Karthikeyana, S., and Balasubramanian, R.: Health risk assessment of occupational exposure to particulate-phase polycyclic aromatic hydrocarbons associated with Chinese, Malay and Indian cooking, J. Environ. Monitor., 8, 369–376, https://doi.org/10.1039/b516173h, 2006.
Shen, G. F.: Quantification of emission reduction potentials of primary air pollutants from residential solid fuel combustion by adopting cleaner fuels in China, J. Environ. Sci., 37, 1–7, 2015.
Shen, G. F., Yang, Y. F., Wang, W., Tao, S., Zhu, C., Min, Y. J., Xue, M. A., Ding, J. N., Wang, B., Wang, R., Shen, H. Z., Li, W., Wang, X. L., and Russell, A.G.: Emission Factors of Particulate Matter and Elemental Carbon for Crop Residues and Coals Burned in Typical Household Stoves in China, Environ. Sci. Technol., 44, 7157–7162, 2010.
Shen, G. F., Chen, Y. C., Xue, C. Y., Lin, N., Huang, Y., Shen, H. Z., Wang, Y. L., Li, T. C., Zhang, Y. Y., Su, S., Huangfu, Y. B., Zhang, W. H., Chen, X. F., Liu, G. Q., Liu, W. X., Wang, X. L., Wong, M. H., and Tao, S.: Pollutant Emissions from Improved Coal- and Wood-Fuelled Cookstoves in Rural Households, Environ. Sci. Technol., 49, 6590–6598, 2015.
Shen, Z. X., Sun, J., Cao, J. J., Zhang, L. M., Zhang, Q., Lei, Y. L., Gao, J. J., Huang, R. J., Liu, S. X., Huang, Y., Zhu, C. S., Xu, H. M., Zheng, C. L., Liu, P. P., and Xue, Z. G.: Chemical profiles of urban fugitive dust PM2.5 samples in Northern Chinese cities, Sci. Total Environ., 569, 619–626, https://doi.org/10.1016/j.scitotenv.2016.06.156, 2016.
Shi, G. L., Li, X., Feng, Y. C., Wang, Y. Q., Wu, J. H., Li, J., and Zhu, T.: Combined source apportionment, using positive matrix factorization-chemical mass balance and principal component analysis/multiple linear regression-chemical mass balance models, Atmos. Environ., 43, 2929–2937, 2009.
Shimodaira, H.: An approximately unbiased test of phylogenetic tree selection, Syst. Biol., 51, 492–508, https://doi.org/10.1080/10635150290069913, 2002.
Simon, H., Beck, L., Bhave, P. V., Divita, F., Hsu, Y., Luecken, D., Mobley, J. D., Pouliot, G. A., Reff, A., Sarwar, G., and Strum, M.: The development and uses of EPA's SPECIATE database, Atmos. Pollut. Res., 1, 196–206, https://doi.org/10.5094/Apr.2010.026, 2010.
Simoneit, B. R. T., Schauer, J. J., Nolte, C. G., Oros, D. R., Elias, V. O., Fraser, M. P., Rogge, W. F., and Cass, G. R.: Levoglucosan, a tracer for cellulose in biomass burning and atmospheric particles, Atmos. Environ., 33, 173–182, 1999.
Smith, W. B., Cushing, K. M., Johnson, J. W., Parsons, C. T., Williamson, A. D., and Wilson Jr., R. R.: EPA-600/7-80-036 (PB82-249897), U.S. Environmental Protection Agency, Research Triangle Park, NC, 1982.
State Council: Notice by the State Council of the People's Republic of China on the deadline to stop the use and sales of leaded gasoline for vehicles, No.2 fuyou street, Xicheng district, Beijing, 129, 1998.
Streets, D. G., Yarber, K. F., Woo, J. H., and Carmichael, G. R.: Biomass burning in Asia: Annual and seasonal estimates and atmospheric emissions, Global Biogeochem. Cy., 17, 1099, https://doi.org/10.1029/2003gb002040, 2003.
Suzuki, R. and Shimodaira, H.: Pvclust: an R package for assessing the uncertainty in hierarchical clustering, Bioinformatics, 22, 1540–1542, https://doi.org/10.1093/bioinformatics/btl117, 2006.
Taner, S., Pekey, B., and Pekey, H.: Fine particulate matter in the indoor air of barbeque restaurants: Elemental compositions, sources and health risks, Sci. Total Environ., 454, 79–87, https://doi.org/10.1016/j.scitotenv.2013.03.018, 2013.
Tang, X. B., Huang, C., Lou, S. R., Qiao, L. P., Wang, H. L., Zhou, M., Chen, M. H., Chen, C. H., Wang, Q., Li, G. L., Li, L., Huang, H. Y., and Zhang, G. F.: Emission Factors and PM Chemical Composition Study of Biomass Burning in the Yangtze River Delta Region, Environ. Sci., 35, 1623–1632, https://doi.org/10.13227/j.hjkx.2014.05.001, 2014 (in Chinese).
Tao, S., Ru, M. Y., Du, W., Zhu, X., Zhong, Q. R., Li, B. G., Shen, G. F., Pan, X. L., Meng, W. J., Chen, Y. L., Shen, H. Z., Lin, N., Su, S., Zhuo, S. J., Huang, T. B., Xu, Y., Yun, X., Liu, J. F., Wang, X. L., Liu, W. X., Cheng, H. F., and Zhu, D. Q.: Quantifying the rural residential energy transition in China from 1992 to 2012 through a representative national survey, Nature Energy, 3, 567–573, https://doi.org/10.1038/s41560-018-0158-4, 2018.
Tian, Y. Z., Chen, J. B., Zhang, L. L., Du, X., Wei, J. J., Fan, H., Xu, J., Wang, H. T., Guan, L., Shi, G. L., and Feng, Y. C.: Source profiles and contributions of biofuel combustion for PM2.5, PM10 and their compositions, in a city influenced by biofuel stoves, Chemosphere, 189, 255–264, https://doi.org/10.1016/j.chemosphere.2017.09.044, 2017.
Tsai, J., Owega, S., Evans, G., Jervis, R., Fila, M., Tan, P., and Malpica, O.: Chemical composition and source apportionment of Toronto summertime urban fine aerosol (PM2.5), J. Radioanal. Nucl. Ch., 259, 193–197, 2004.
Ulbrich, I. M., Canagaratna, M. R., Cubison, M. J., Zhang, Q., Ng, N. L., Aiken, A. C., and Jimenez, J. L.: Three-dimensional factorization of size-resolved organic aerosol mass spectra from Mexico City, Atmos. Meas. Tech., 5, 195–224, https://doi.org/10.5194/amt-5-195-2012, 2012.
Vicente, E. D. and Alves, C. A.: An overview of particulate emissions from residential biomass combustion, Atmos. Res., 199, 159–185, https://doi.org/10.1016/j.atmosres.2017.08.027, 2018.
Wang, G., Lang, J. L., Cheng, S. Y., Yao, S., and Wang, X. Q.: Characteristics of PM2.5 and hydrocarbon emitted from heavy-duty diesel vehicle, China Environmental Science, 35, 3581–3587, 2015.
Wang, X. L., Watson, J. G., Chow, J. C., Kohl, S. D., Chen, L. W. A., Sodeman, D. A., Legge, A. H., and Percy, K. E.: Measurement of Real-World Stack Emissions with a Dilution Sampling System, Alberta Oil Sands: Energy, Industry and the Environment, Dev. Environm. Sci., 11, 171–192, 2012.
Wang, X. P., Zong, Z., Tian, C. G., Chen, Y. J., Luo, C. L., Li, J., Zhang, G., and Luo, Y. M.: Combining Positive Matrix Factorization and Radiocarbon Measurements for Source Apportionment of PM2.5 from a National Background Site in North China, Sci. Rep., 7, 10648, https://doi.org/10.1038/s41598-017-10762-8, 2017.
Wang, Z., Guo, J, and Chen, Z.: Analysis of the source componential spectrum of PM2.5 emission in Guiyang, Journal of Safety and Environment, 16, 346–351, 2016.
Watson, J. G.: Overview of receptor model principles, JAPCA J. Air Waste Ma., 34, 619–623, 1984.
Watson, J. G., Chow, J. C., Pritchett, L. C., Houck, J. A., Ragazzi, R. A., and Burns, S.: Chemical source profiles for particulate motor vehicle exhaust under cold and high altitude operating conditions, Sci. Total Environ., 93, 183–190, 1990.
Watson, J. G. and Chow, J. C.: Source characterization of major emission sources in the Imperial and Mexicali Valleys along the US/Mexico border, Sci. Total Environ., 276, 33–47, https://doi.org/10.1016/S0048-9697(01)00770-7, 2001.
Wiinikka, H. and Gebart, R.: The influence of fuel type on particle emissions in combustion of biomass pellets, Combust. Sci. Technol., 177, 741–763, https://doi.org/10.1080/00102200590917257, 2005.
Winchester, J. W. and Nifong, G. D.: Water pollution in Lake Michigan by trace elements from pollution aerosol fallout, Water Air Soil Poll., 1, 50–64, https://doi.org/10.1007/BF00280779, 1971.
Xia, Z. Q., Fan, X. L., Huang, Z. J., Liu, Y. C., Yin, X. H., Ye, X., and Zheng, J. Y.: Comparison of domestic and foreign PM2.5 source profiles and influence on air quality simulation, Research of Environmental Sciences, 30, 359–367, https://doi.org/10.13198/j.issn.1001-6929.2017.01.55, 2017 (in Chinese).
Yan, C. Q., Zheng, M., Bosch, C., Andersson, A., Desyaterik, Y., Sullivan, A. P., Collett, J. L., Zhao, B., Wang, S. X., He, K. B., and Gustafsson, O.: Important fossil source contribution to brown carbon in Beijing during winter, Sci. Rep., 7, 43182, https://doi.org/10.1038/srep43182, 2017a.
Yan, D. J., Liu, S. J., Huang, X. M., Wang, Y., and Xu, Y.: Effects of SO2 and H2O on the SCR activity of the Mn-Ce ∕ TiO2 catalyst at low temperatures, Journal of Safety and Environment, 16, 312–319, https://doi.org/10.13637/j.issn.1009-6094.2016.05.059, 2016.
Yan, Q., Kong, S. F., Liu, H. B., Wang, W., Wu, J., Zheng, M. M., Zheng, S. R., Yang, G. W., Wu, F. Q.: Emission inventory of water soluble ions in fine particles from residential coal burning in China and implication for emission reduction, China Environmental Science, 37, 3708–3721, 2017b.
Yao, H., Song, Y., Liu, M., Archer-Nicholls, S., Lowe, D., McFiggans, G., Xu, T., Du, P., Li, J., Wu, Y., Hu, M., Zhao, C., and Zhu, T.: Direct radiative effect of carbonaceous aerosols from crop residue burning during the summer harvest season in East China, Atmos. Chem. Phys., 17, 5205–5219, https://doi.org/10.5194/acp-17-5205-2017, 2017.
Zhang, D. Y.: Development of gasoline production & realization of unlead gasoline in China, Petroleum Products Application Research, Shanghai Institute of Petroleum Commodity Application, Shanghai, 1–2, 2000 (in Chinese).
Zhang, J. J. and Smith, K. R.: Household air pollution from coal and biomass fuels in China: Measurements, health impacts, and interventions, Environ. Health Persp., 115, 848–855, 2007.
Zhang, J., He, K. B., Ge, Y. S., and Shi, X. Y.: Influence of fuel sulfur on the characterization of PM10 from a diesel engine, Fuel, 88, 504–510, https://doi.org/10.1016/j.fuel.2008.09.001, 2009a.
Zhang, N., Han, B., He, F., Xu, J., Zhao, R. J., Zhang, Y. J., and Bai, Z. P.: Chemical characteristic of PM2.5 emission and inhalational carcinogenic risk of domestic Chinese cooking, Environ. Pollut., 227, 24–30, https://doi.org/10.1016/j.envpol.2017.04.033, 2017a.
Zhang, Q., Jimenez, J. L., Canagaratna, M. R., Ulbrich, I. M., Ng, N. L., Worsnop, D. R., and Sun, Y. L.: Understanding atmospheric organic aerosols via factor analysis of aerosol mass spectrometry: a review, Anal. Bioanal. Chem., 401, 3045–3067, 2011.
Zhang, Q., Shen, Z. X., Cao, J. J., Ho, K. F., Zhang, R. J., Bie, Z. J., Chang, H. R., and Liu, S. X.: Chemical profiles of urban fugitive dust over Xi'an in the south margin of the Loess Plateau, China, Atmos. Pollut. Res., 5, 421–430, https://doi.org/10.5094/Apr.2014.049, 2014.
Zhang, Y., Sheesley, R. J., Schauer, J. J., Lewandowski, M., Jaoui, M., Offenberg, J. H., Kleindienst, T. E., and Edney, E. O.: Source apportionment of primary and secondary organic aerosols using positive matrix factorization (PMF) of molecular markers, Atmos. Environ., 43, 5567–5574, https://doi.org/10.1016/j.atmosenv.2009.02.047, 2009b.
Zhang, Y. J., Cai, J., Wang, S. X., He, K. B., and Zheng, M.: Review of receptor-based source apportionment research of fine particulate matter and its challenges in China, Sci. Total Environ., 586, 917–929, https://doi.org/10.1016/j.scitotenv.2017.02.071, 2017b.
Zhang, Y. X., Shao, M., Zhang, Y. H., Zeng, L. M., He, L. Y., Zhu, B., Wei, Y. J., and Zhu, X. L.: Source profiles of particulate organic matters emitted from cereal straw burnings, J. Environ. Sci.-China, 19, 167–175, https://doi.org/10.1016/S1001-0742(07)60027-8, 2007.
Zhang, Y. Z., Yao, Z. L., Shen, X. B., Liu, H., and He, K. B.: Chemical characterization of PM2.5 emitted from on-road heavy-duty diesel trucks in China, Atmos. Environ., 122, 885–891, https://doi.org/10.1016/j.atmosenv.2015.07.014, 2015.
Zhao, L., Zhang, D., Zhou, Z. E., Ren, L. H., Yin, B. H., and Yuan, R.: A study on emission characteristics of particulate matters from typical industrial combustion sources in Chongqing city, Journal of Environmental Engineering Technology, 5, 447–454, 2015a.
Zhao, P. S., Feng, Y. C., Zhu, T., and Wu, J. H.: Characterizations of resuspended dust in six cities of North China, Atmos. Environ., 40, 5807–5814, 2006.
Zhao, X. Y., Hu, Q. H., Wang, X. M., Ding, X., He, Q. F., Zhang, Z., Shen, R. Q., Lu, S. J., Liu, T. Y., Fu, X. X., and Chen, L. G.: Composition profiles of organic aerosols from Chinese residential cooking: case study in urban Guangzhou, south China, J. Atmos. Chem., 72, 1–18, https://doi.org/10.1007/s10874-015-9298-0, 2015b.
Zhao, Y. L., Hu, M., Slanina, S., and Zhang, Y. H.: The molecular distribution of fine particulate organic matter emitted from Western-style fast food cooking, Atmos. Environ., 41, 8163–8171, https://doi.org/10.1016/j.atmosenv.2007.06.029, 2007a.
Zhao, Y. L., Hu, M., Slanina, S., and Zhang, Y. H.: Chemical compositions of fine particulate organic matter emitted from Chinese cooking, Environ. Sci. Technol., 41, 99–105, https://doi.org/10.1021/es0614518, 2007b.
Zheng, M., Cass, G. R., Schauer, J. J., and Edgerton, E. S.: Source apportionment of PM2.5 in the southeastern United States using solvent-extractable organic compounds as tracers, Environ. Sci. Technol., 36, 2361–2371, 2002.
Zhou, L. M., Kim, E., Hopke, P. K., Stanier, C. O., and Pandis, S.: Advanced factor analysis on Pittsburgh particle size-distribution data, Aerosol Sci. Tech., 38, 118–132, 2004.
Zhou, N., Zeng, L. M., Yu, X. N., Fu, L. L, and Shao, M.: The design and field test of a dilution tunnel for stationary sources, Acta Scientiae Circumstantiae, 26, 764–772, 2006.
Zhu, Y. H., Huang, L., Li, J. Y., Ying, Q., Zhang, H. L., Liu, X. G., Liao, H., Li, N., Liu, Z. X., Mao, Y. H., Fang, H., and Hu, J. L.: Sources of particulate matter in China: Insights from source apportionment studies published in 1987–2017, Environ. Int., 115, 343–357, https://doi.org/10.1016/j.envint.2018.03.037, 2018.