the Creative Commons Attribution 4.0 License.
the Creative Commons Attribution 4.0 License.
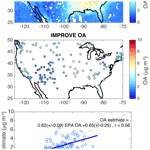
Towards a satellite formaldehyde – in situ hybrid estimate for organic aerosol abundance
Jin Liao
Thomas F. Hanisco
Glenn M. Wolfe
Jason St. Clair
Jose L. Jimenez
Pedro Campuzano-Jost
Benjamin A. Nault
Alan Fried
Eloise A. Marais
Gonzalo Gonzalez Abad
Kelly Chance
Hiren T. Jethva
Thomas B. Ryerson
Carsten Warneke
Armin Wisthaler
Organic aerosol (OA) is one of the main components of the global particulate burden and intimately links natural and anthropogenic emissions with air quality and climate. It is challenging to accurately represent OA in global models. Direct quantification of global OA abundance is not possible with current remote sensing technology; however, it may be possible to exploit correlations of OA with remotely observable quantities to infer OA spatiotemporal distributions. In particular, formaldehyde (HCHO) and OA share common sources via both primary emissions and secondary production from oxidation of volatile organic compounds (VOCs). Here, we examine OA–HCHO correlations using data from summertime airborne campaigns investigating biogenic (NASA SEAC4RS and DC3), biomass burning (NASA SEAC4RS), and anthropogenic conditions (NOAA CalNex and NASA KORUS-AQ). In situ OA correlates well with HCHO (r=0.59–0.97), and the slope and intercept of this relationship depend on the chemical regime. For biogenic and anthropogenic regions, the OA–HCHO slopes are higher in low NOx conditions, because HCHO yields are lower and aerosol yields are likely higher. The OA–HCHO slope of wildfires is over 9 times higher than that for biogenic and anthropogenic sources. The OA–HCHO slope is higher for highly polluted anthropogenic sources (e.g., KORUS-AQ) than less polluted (e.g., CalNex) anthropogenic sources. Near-surface OAs over the continental US are estimated by combining the observed in situ relationships with HCHO column retrievals from NASA's Ozone Monitoring Instrument (OMI). HCHO vertical profiles used in OA estimates are from climatology a priori profiles in the OMI HCHO retrieval or output of specific period from a newer version of GEOS-Chem. Our OA estimates compare well with US EPA IMPROVE data obtained over summer months (e.g., slope =0.60–0.62, r=0.56 for August 2013), with correlation performance comparable to intensively validated GEOS-Chem (e.g., slope =0.57, r=0.56) with IMPROVE OA and superior to the satellite-derived total aerosol extinction (r=0.41) with IMPROVE OA. This indicates that OA estimates are not very sensitive to these HCHO vertical profiles and that a priori profiles from OMI HCHO retrieval have a similar performance to that of the newer model version in estimating OA. Improving the detection limit of satellite HCHO and expanding in situ airborne HCHO and OA coverage in future missions will improve the quality and spatiotemporal coverage of our OA estimates, potentially enabling constraints on global OA distribution.
- Article
(5789 KB) -
Supplement
(2028 KB) - BibTeX
- EndNote
Aerosols are the largest source of uncertainty in climate radiative forcing (IPCC, 2013; Carslaw et al., 2013) and decrease atmospheric visibility and impact human health (Pope, 2002). Organic aerosols (OAs) comprise a large portion (∼50 %) of submicron aerosols (Jimenez et al., 2009; Murphy et al., 2006; Shrivastava et al., 2017), and this fraction will grow with continued decline in SO2 emissions (Attwood et al., 2014; Marais et al., 2017; Ridley et al., 2018). In addition, OAs serve as cloud condensation nuclei (CCN) and affect cloud formation and climate radiative forcing. OA components also have adverse health effects (e.g., Walgraeve et al., 2010) and contribute significantly to regional severe haze events (e.g., Hayes et al., 2013). Finally, because the response of temperature to changes in climate forcing is non-linear (Taylor and Penner, 1994) and the forcing by aerosols has strong regional character (Kiehl and Briegleb, 1993), it is necessary to separate out different climate forcing components to accurately forecast the climate response to changes in forcing.
Despite their importance, it has been challenging to accurately represent OAs in global models. Chemical transport models (CTMs) often underpredict OA (e.g., more than a factor of 2 lower OA near the ground) compared to observations, and model-to-model variability can exceed a factor of 100 in the free troposphere (Tsigaridis et al., 2014; Heald et al., 2008, 2011). Fully explicit mechanisms have attempted to capture the full OA chemical formation mechanisms (e.g., Lee-Taylor et al., 2015), but it is too computationally expensive to apply these mechanisms to OA formation in global CTMs at a useful resolution. For computational efficiency, 3-D models such as GEOS-Chem include direct emissions of primary OA (POA) and represent secondary OA (SOA) formation either by lumping SOA products according to similar hydrocarbon classes (Kim et al., 2015) or based on the volatility of the oxidation products (Pye et al., 2010). Marais et al. (2016) applied an aqueous-phase mechanism for SOA formation from isoprene in GEOS-Chem to reasonably simulate isoprene SOA in the southeastern (SE) US. Schroder et al. (2018) showed GEOS-Chem has a very large underprediction of SOA in the northeastern US dominated by anthropogenic emissions. Accurate emission inventories are also needed to correctly represent volatile organic compounds (VOCs) and NOx () inputs, and these often have biases compared to observational constraints (Kaiser et al., 2018; Travis et al., 2016; Anderson et al., 2014; McDonald et al., 2018).
A quantitative measure of OA from space would be helpful for verifying emissions and aerosol processes in models. However, direct measurements of OA from space are currently unavailable. Aerosol optical depth (AOD) measured by satellite sensors provides a coarse but global picture of total aerosol distributions. The Multi-angle Imaging SpectroRadiometer (MISR) provides aerosol property information such as size, shape, and absorbing properties, which allows retrieving the AOD of a subset of aerosols (Kahn and Gaitley, 2015). Classification algorithms have been developed to speciate different aerosol types (e.g., OA) based on AOD, extinction Ångström exponent, UV aerosol index, and trace gas columns from satellite instruments (Penning de Vries et al., 2015). Here, we aim to provide a quantitative estimation of OA mass concentrations from satellite measurements.
Formaldehyde (HCHO) is one of the few VOCs that can be directly observed from space. Sources emitting POA (e.g., biomass burning; BB) often simultaneously release VOCs. HCHO and SOA are also both produced from emitted VOCs. VOCs, as well as intermediate- and semi-volatile organic compounds (I/SVOCs), are oxidized by hydroxyl radicals (OH) to form peroxy radicals (RO2), which then react with NO, RO2, or hydroperoxy radicals (HO2) or isomerize. These oxidation processes produce HCHO and oxidized organic compounds with low volatility that condense to form SOA (Robinson et al., 2007; Ziemann and Atkinson, 2012). The yield of HCHO and SOA from hydrocarbon oxidation thus depends on the VOC precursors, oxidants (OH, O3, and NO3), RO2 reaction pathway (e.g., NO levels), and pre-existing aerosol abundance and properties (Wolfe et al., 2016; Pye et al., 2010; Marais et al., 2016, 2017; Xu et al., 2016). Moreover, although the lifetime of HCHO (1–3 h) is shorter than OA (1 week), HCHO continues to form from slower-reacting VOCs, as well as from the oxidation of later-generation products. Observations across megacities around the world show that OA formation in polluted/urban areas happens over about 1 day (e.g., DeCarlo et al., 2010; Hodzic and Jimenez, 2011; Hayes et al., 2013, 2015), and HCHO is also significantly formed over this timescale (Nault et al., 2018). In addition, Veefkind et al. (2011) found that satellite AOD correlated with HCHO over the summertime SE US, BB regions, and southeast Asian industrialized regions. This also suggests that OAs share common emission sources and photochemical processes with HCHO and are a major contributor to AOD in the regions above. Marais et al. (2016) further used the relationship between aircraft OA and satellite HCHO to evaluate the GEOS-Chem representation of SOA mass yields from biogenic isoprene in the SE US.
We present an OA surface mass concentration estimate (OA estimate) derived from a combination of satellite HCHO column observations and in situ OA–HCHO relationships. Because the detection limit of satellite HCHO column observations limits the quality of OA estimate, we focus our analyses on summertime when HCHO levels are high. The OA estimate is evaluated against OA measurements at ground sites. A 3-D model GEOS-Chem OA simulation is shown for comparison.
2.1 In situ airborne observations
Figure 1 shows flight tracks with altitudes < 1 km of the field campaigns used in the current study. The Studies of Emissions, Atmospheric Composition, Clouds and Climate Coupling by Regional Surveys (SEAC4RS) mission (Toon et al., 2016; SEAC4RS Science Team, 2013) covered the continental US with a focus on the SE US in August–September 2013. The Deep Convective Clouds and Chemistry Experiment (DC3) (Barth et al., 2015; DC3 Science Team, 2012) surveyed the central and SE US in May–June 2012, targeting isolated deep convective thunderstorms and mesoscale convective systems. The California Research at the Nexus of Air Quality and Climate Change (CalNex) (Ryerson et al., 2013; CalNex Science Team, 2010) investigated the California region in May–June 2010, targeting the Los Angeles (LA) Basin and Central Valley. The Korea–United States Air Quality Study (KORUS-AQ) studied South Korean air quality, sampling many large urban areas in South Korea and continental Asian outflow over the West Sea, in May–June 2016 (KORUS-AQ Science Team, 2016). KORUS-AQ only includes data with longitude < 133∘ E to exclude the transit from the US because it targeted South Korea and the nearby region. These field campaigns were selected as they had recent high-quality in situ HCHO and OA data measured with state-of-the-art instruments and studied summertime regional tropospheric chemical composition.
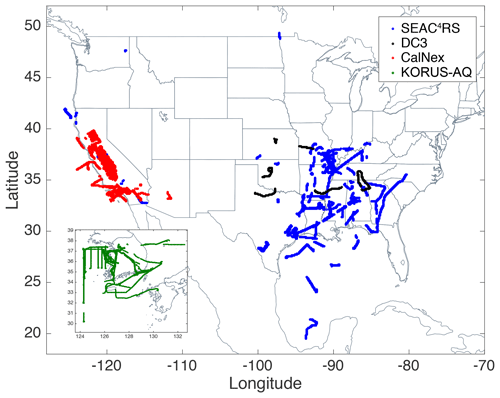
Figure 1Flight tracks of airborne field campaigns SEAC4RS (blue), DC3 (black), CalNex (red), and KORUS-AQ (green) with altitudes (< 1 km), of which in situ OA and HCHO measurements were used.
In situ airborne HCHO observations were acquired by multiple instruments. The DC3 NASA DC-8 payloads featured two HCHO measurements: the NASA In Situ Airborne Formaldehyde (ISAF) (Cazorla et al., 2015) and the Difference Frequency Generation Absorption Spectrometer (DFGAS) (Weibring et al., 2006). The SEAC4RS NASA DC-8 payloads also featured two HCHO measurements: the NASA ISAF and the Compact Atmospheric Multispecies Spectrometer (CAMS) (Richter et al., 2015). HCHO measurements from ISAF were found to be in good agreement with CAMS, with a correlation coefficient of 0.99 and a slope of 1.10 (Zhu et al., 2016). HCHO measurements from ISAF also had a good agreement with DFGAS, with a correlation coefficient of 0.98 and a slope of 1.07. Because ISAF has higher data density, we used ISAF HCHO data for DC3 and SEAC4RS. During KORUS-AQ, CAMS was the only HCHO instrument aboard the DC-8. In CalNex, a proton transfer reaction mass spectrometer (PTR-MS) (Warneke et al., 2011) was used to measure HCHO aboard the NOAA P3 aircraft.
In situ airborne OA from SEAC4RS, DC3, and KORUS-AQ was measured by the University of Colorado high-resolution time-of-flight aerosol mass spectrometer (HR-ToF-AMS; DeCarlo et al., 2006; Dunlea et al., 2009; Canagaratna et al., 2007; Jimenez et al., 2016) and in situ airborne OA from CalNex was measured by the NOAA compact time-of-flight aerosol mass spectrometer (Drewnick et al., 2005; Canagaratna et al., 2007; Bahreini et al., 2012). The OA measurements are from 1 min merge data and converted from µg sm−3 (at 273 K and 1013 mbar) to µg m−3 under local T and P for each data point, to be consistent with HCHO concentrations in µg m−3 or molec cm−3 at local T and P.
Although NO modulates the RO2 lifetime, and thus the production of HCHO and SOA, NO cannot be directly observed via remote sensing. Instead, NO2 can be directly observed in space by satellites, and because NO2 represents typically ∼80 % (e.g., SEAC4RS and KORUS-AQ) of the boundary layer NOx concentrations during the daytime, NO2 can be used as a surrogate for daytime NO concentrations and oxidative conditions around the globe. In situ airborne NO2 was measured by the NOAA chemiluminescence NOyO3 instrument (Ryerson et al., 2001) during SEAC4RS, DC3, and CalNex and by University of Berkeley laser-induced fluorescence NO2 instrument (Day et al., 2002) during KORUS-AQ. SEAC4RS isoprene measurements were from the proton-transfer-reaction mass spectrometer (PTR-MS) (Wisthaler et al., 2002).
2.2 Ground-based OA measurements
Ground-based OA measurements over the US were from the EPA Interagency Monitoring of Protected Visual Environments (IMPROVE) (Malm et al., 1994; Solomon et al., 2014; Hand et al., 2014, 2013; Malm et al., 2017) and Southeastern Aerosol Research and Characterization (SEARCH) (Edgerton et al., 2006) networks. In the IMPROVE network, aerosols were collected on quartz fiber filters and analyzed in the lab by thermal optical reflectance for organic and elemental carbon. The data were reported every 3 days from 1988 to 2014. Monthly averages were used for comparison in this study. IMPROVE OA data over the SE US (east of 70∘ W) in summertime were multiplied by a factor of 1.37 to correct for partial evaporation during filter transport, following the recommendation of a comparison study with SEARCH organic carbon (OC) measurements (Kim et al., 2015; Hand et al., 2013). Although IMPROVE OA corrected for evaporation has potential uncertainties with the constant scaling factor, the IMPROVE measurements have high spatial coverage. SEARCH network (Edgerton et al., 2006; Hidy et al., 2014) OC was determined by the difference between total carbon (TC) detected by a tapered element oscillating microbalance (TEOM) and black carbon (BC) measured by an in situ thermal–optical instrument. This allowed real-time measurement of OC and prevented evaporation during filter transport. Although the SEARCH network only has five sites available, we used observations from this network due to their high accuracy. The IMPROVE and SEARCH network OC measurements were converted to OA by multiplying by a factor of 2.1 based on ground and aircraft observations (Pye et al., 2017; Schroder et al., 2018).
2.3 Satellite measurements
Satellite HCHO column observations were derived from NASA's Ozone Monitoring Instrument (OMI), a UV–visible nadir solar backscatter spectrometer on the Aura satellite (Levelt et al., 2006). Aura passes over the Equator at 13:30 LT, daily. Here, we used the OMI HCHO version 2.0 (collection 3) gridded () retrieval data (Gonzalez Abad et al., 2015) from the Smithsonian Astrophysical Observatory (SAO). Satellite data for HCHO columns were subjected to data quality filters: (1) solar zenith angle lower than 70∘, (2) cloud fraction less than 40 %, and (3) main quality flag and the xtrackquality flag both equal to zero (Harvard-Smithsonian Center for Astrophysics OMI HCHO data product description, 2017). The monthly average HCHO columns were also weighted by the column uncertainties of the pixels. The HCHO retrieval used a priori profiles without aerosol information from the GEOS-Chem model (Gonzalez Abad et al., 2015). Satellite NO2 column observations were also derived from NASA's OMI level 3 data (Lamsal et al., 2014; Krotkov, 2013). Satellite NO2 observations were used to calculate the NOx-related chemical-factor-dependent OA estimate (see Table 2). Satellite AOD observations were acquired from the Moderate Resolution Imaging Spectroradiometer (MODIS) aboard the Aqua satellite, using overpasses at about 13:30 LT. Here, we used Collection 06 (Levy and Hsu, 2015), retrieved using the dark target (DT) and deep blue (DB) algorithms (Levy et al., 2015), monthly average data.
2.4 GEOS-Chem
We used GEOS-Chem (v9-02) at with 47 vertical layers to simulate HCHO and OA globally, the same as that in Marais et al. (2016). GEOS-Chem was driven with meteorological fields from the NASA Global Modeling and Assimilation Office (GMAO). The OA simulation included POA from fires and anthropogenic activity and SOA from the volatility-based reversible partitioning scheme (VBS) of Pye et al. (2010) for anthropogenic, fire, and monoterpene sources, and an irreversible aqueous-phase reactive uptake mechanism for isoprene. The aqueous-phase mechanism was coupled to gas-phase isoprene chemistry and has been extensively validated using surface and aircraft observations of isoprene SOA components in the SE US (Marais et al., 2016). This model version used the fourth-generation Global Fire Emissions Database (GFED4) (Giglio et al., 2013) as a BB emission inventory. The model was driven with Goddard Earth Observing System – Forward Processing (GEOS-FP) meteorology for 2013 and sampled along the SEAC4RS (2013) and KORUS-AQ (2016) flight tracks. The model was also run with a 10 % decrease in biomass burning, biogenic, or anthropogenic emissions as a sensitivity test to evaluate the contributions of different sources to the OA and HCHO budget. Model monthly mean surface layer OA and total column formaldehyde were obtained around the OMI overpass time (12:00–15:00 LT) for 2008–2013 using Modern-Era Retrospective analysis for Research and Applications (MERRA) (Gelaro et al., 2017) meteorology, as GEOS-FP was only available from 2012. This was compared to the OA estimate derived from satellite HCHO.
Global isoprene emissions from the Model of Emissions of Gases and Aerosols from Nature version 2.1 (MEGAN) (Guenther et al., 2006) and satellite NO2 column data were used to calculate an isoprene- and NO2-dependent OA estimate (see Table 2). Global isoprene emissions from MEGAN were implemented in GEOS-Chem and driven with MERRA (MEGAN-MERRA).
2.5 Estimation of surface organic aerosol mass concentrations
An estimate for surface OA mass concentration was calculated based on a simple linear transformation.
Here, ε(i) is the OA estimate for grid cell i (µg m−3), ΩHCHO(i) is the OMI HCHO column density (molec cm−2) in each grid cell (similar resolution to OMI HCHO nadir pixel data), η(i) is the ratio of midday surface layer (∼60 m) HCHO concentrations (molec cm−3) to column concentrations (molec cm−2) from GEOS-Chem, and α(i) and β(i) are the slope and intercept of a linear regression between OA and HCHO from low-altitude (< 1 km) airborne in situ measurements. The in situ to column conversion factor η(i) was similar to that used by Zhu et al. (2017) to convert HCHO columns into surface concentrations. η(i) was derived from the HCHO a priori profiles used in SAO OMI air mass factor (AMF) calculations (GEOS-Chem v9-01-03 climatology) or from GEOS-Chem v9-02, which included an updated isoprene scheme for OA and is the next version of the model (v9-01-03) for a priori profiles used in SAO satellite HCHO retrievals. HCHO a priori profiles were used to be consistent with satellite HCHO retrievals and also to show that the OA estimate can be derived without running a global model separately. The newer version of GEOS-Chem was used to test the sensitivity of OA estimates to the updated version of η. The newer version of GEOS-Chem also allows sampling through the flight tracks of a recent field campaign (SEAC4RS) and examining the factors impacting η with both modeled and measured HCHO profiles. The detailed information about the impact of HCHO profiles on η is provided in Sect. 5.
2.6 Aerosol extinction from satellite measurements
Currently, remote sensing techniques observe aerosols by quantifying AOD. The MISR satellite instrument can estimate a subset of AOD, using constraints on size range, shape, and absorbing properties, but it cannot distinguish OA from other submicron aerosol compounds such as sulfate and nitrate and also requires AOD to be above 0.1. Because MISR estimates a subset of AOD, it is discussed above to verify that we are not neglecting a satellite dataset that has already captured OA AOD. Moreover, OAs account for a large and relatively constant fraction of submicron aerosols in the SE US (Kim et al., 2015; Wagner et al., 2015) and are one of the major submicron aerosol components over the US (Jimenez et al., 2009). Therefore, AOD was converted to extinction to represent OA for comparison:
where Aext is the calculated aerosol extinction (Mm−1), AOD(i) is aerosol optical depth from MODIS (see Sect. 2.3) in each grid cell, and δ(i) (m−1) is the ratio of surface layer OA concentrations (µg m−3, at ambient T and P) to column OA concentrations (µg m−2) from GEOS-Chem multiplied by 106 m Mm−1. The shape of the average vertical profile of OA (OA fraction: 0.54–0.7) was close to that of total aerosol mass over the SE US (Wagner et al., 2015), where a large fraction of the enhanced non-BB aerosol concentrations in summertime over the US are located. Data with BB plume interferences were excluded in the following analysis. The potential contribution of dust and nitrate could alter the shape of the vertical profiles and introduce uncertainties when using OA vertical profiles for other parts of the US. However, the outliers in the aerosol extinction compared to ground OA measurements (see Sect. 6.3) were not located outside of the SE US. Similar vertical profile shapes of OA and submicron particles were also observed in a campaign outside the US over South Korea (Nault et al., 2018). Although OA accounted for ∼40 % of the total submicron particles, the shape of OA and total submicron particles' vertical profiles were nearly identical.
Although OA and HCHO share common VOC emission sources and photochemical processes, their production rates from different emission sources and photochemical conditions vary, as do their loss rates. We found the main factors that modulate OA–HCHO relationships from in situ measurements and discussed them in the following section.
Table 1Linear regression parameters for OA vs. HCHO at low altitudes (< 1 km).
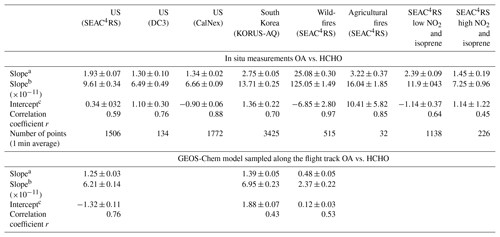
a The unit of the slope is g g−1. b The unit of the slope is pg molec−1. c The unit of the intercept is µg m−3. The uncertainties are 1 standard deviation.
3.1 Regional and source-driven variability
For all regions and/or sources investigated, near-surface in situ OA and HCHO are well correlated. A scatter plot of in situ OA vs. HCHO at low altitudes (< 1 km) from a number of field campaigns (SEAC4RS, DC3, CalNex, and KORUS-AQ) is displayed in Fig. 2. The slopes, intercepts, and correlation coefficients are summarized in Table 1. SEAC4RS, DC3, and CalNex excluded BB data when acetonitrile was > 200 pptv (Hudson et al., 2004). KORUS-AQ used a BB filter with higher acetonitrile (> 500 pptv) because the air masses with moderate acetonitrile enhancement (200–500 pptv) were actually from anthropogenic emissions. This attribution is based on high levels of acetonitrile detected downwind of Seoul and west coastal petrochemical facilities, the slope between acetonitrile and CO being to urban emissions (Warneke et al., 2006), and the concentrations of anthropogenic tracer CHCl3 being high (Warneke et al., 2006). Similar to acetonitrile, another common BB tracer, hydrogen cyanide (HCN), was also enhanced in these air masses. BB data (acetonitrile > 200 pptv) for SEAC4RS were analyzed separately and are in the inset in Fig. 2. Although all CalNex data had a tight correlation, we only included the flight data near the LA Basin to target the area strongly influenced by anthropogenic emissions. In general, the correlation coefficients between in situ OA and HCHO were strong (r=0.59–0.97) (Table 1).
The variety in OA–HCHO regression coefficients among different campaigns reflects the regional and/or source-driven OA–HCHO variability. Considering only the non-biomass burning (non-BB) air masses sampled, OA and HCHO had the tightest correlation for CalNex, because CalNex focused on the LA area (shown in Fig. 2) and Central Valley, while SEAC4RS and DC3 covered a larger area with a potentially larger variety of sources and chemical conditions. Although SEAC4RS and DC3 both sampled the continental US, SEAC4RS had more spatial coverage and sampled more air masses at low altitudes, while DC3 was designed to sample convective outflow air masses and had more data at high altitudes. Although KORUS-AQ covered a much smaller area compared to SEAC4RS, KORUS-AQ data also had a large spread, which may be due to the complicated South Korean anthropogenic sources mixed with transported air masses (e.g., from China) and maybe biogenic sources. OA exhibits a tight correlation with HCHO for both wildfires and agricultural fires during SEAC4RS. This is because the production of HCHO and OA is much higher in BB air masses compared to background. This may also suggest that the emissions of OA and HCHO in these air masses are relatively constant. SEAC4RS data are chosen because the campaign sampled fires and had state-of-the-art, high-quality measurements. More intensive fire sampling is needed to probe the correlation between OA and HCHO across fuel types and environmental conditions.
The different slopes of OA–HCHO among different campaigns also reflect the regional or source-driven OA–HCHO variability. Among the BB, anthropogenic, and biogenic sources, the slopes of OA vs. HCHO for BB air masses were the highest. This is consistent with high POA emission in BB conditions (Heald et al., 2008; Lamarque et al., 2010; Cubison et al., 2011), with low addition of mass due to SOA formation (Cubison et al., 2011; Shrivastava et al., 2017). The slope of OA to HCHO was higher for wildfires than in agricultural fires during SEAC4RS though data were limited (see Table 1). This is consistent with more OA emitted in wildfires than agricultural fires (Liu et al., 2017). The factors driving higher OA to HCHO with wildfires are not clear and may be related to burning conditions and fuels. For the non-BB sources, the slope of OA vs. HCHO was highest for South Korea (KORUS-AQ), which is dominated by heavily polluted anthropogenic sources. During KORUS-AQ, the high OA to HCHO air masses also had high acetonitrile. By the time we sampled, most organic aerosols were secondary (Nault et al., 2018). This indicates that the formation rates of OA and HCHO from different emission sources contribute to the different slopes of OA–HCHO. This also indicates that emission sources with enhanced acetonitrile tend to form more OA relative to HCHO downwind. The slope of OA–HCHO for the LA Basin (California), dominated by relatively clean anthropogenic emissions, was much lower than that for South Korea. The potential difference in the anthropogenic emissions mix could contribute to the different OA–HCHO slopes from the US LA region and South Korean anthropogenic sources (Baker et al., 2008; Na et al., 2002, 2005). The slopes of OA vs. HCHO of SEAC4RS and DC3 dominated by biogenic emissions in the SE US were in between heavily polluted (KORUS-AQ) and clean anthropogenic sources (CalNex). As SEAC4RS had the largest geographic coverage for low-altitude data over the US, the campaign average slope of OA vs. HCHO was used to represent the US region in summer. CalNex LA Basin data were used to represent large cities as case studies.
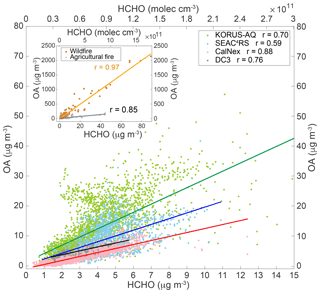
Figure 2Scatter plots of in situ OA (µg m−3) vs. HCHO (µg m−3 or molec cm−3) from SEAC4RS (excluding biomass burning) (blue), DC3 (dark grey), CalNex (pink), and KORUS-AQ (green) low-altitude (< 1 km) data. Inset shows wildfire (brown) and agricultural fire (grey) SEAC4RS data. SEAC4RS biomass burning cases are defined as acetonitrile > 200 pptv. The linear regression fits are shown as the darker lines and correlation coefficients are provided.
Overall, the source-dependent OA–HCHO relationships (Fig. 2) showed higher OA–HCHO slopes of BB and heavily polluted anthropogenic sources with inefficient combustion (e.g., KORUS-AQ) compared to biogenic and relatively clean anthropogenic sources. This indicated that inefficient combustions contribute to the high slopes of OA–HCHO, probably due to both enhanced primary OA and increased formation of SOA. Enhanced pre-existing aerosols such as primary aerosols can provide more surfaces to increase VOC condensation and SOA formation. VOCs co-emitted from heavily polluted anthropogenic sources can also form more SOA. It is possible to extract the factors that govern the different OA–HCHO relationships and potentially have a universal application of the slopes as a function of the factors (e.g., sources and combustion efficiencies).
3.2 Dependence on NOx and VOC speciation
Biogenic and anthropogenic VOCs are oxidized by atmospheric oxidants (e.g., OH as the dominant oxidant) to form RO2. HCHO is produced from the reactions of RO2 with HO2 or NO, with RO2+NO typically producing more HCHO than RO2+HO2 (e.g., Wolfe et al., 2016). RO2 can react with HO2 or NO, or isomerize to form oxidized organic compounds with high molecular weight and low volatility, which condense on existing particles to form SOA. The products of RO2+NO tend to fragment instead of functionalize and often lead to higher volatility compounds (e.g., HCHO) and thus less SOA formation compared to the products of RO2+HO2 (Kroll et al., 2006; Worton et al., 2013). Therefore, with the same VOC, we expect more HCHO and less OA formed at high NO conditions, and vice versa. As mentioned before, NO2 instead of NO is easily measured from space and NO2 typically is ∼80 % of NOx in the boundary layer during the day. Therefore, NO2 is used as a surrogate for the NO levels influencing OA and HCHO production. The yields of HCHO and SOA also depend on VOC speciation (e.g., Lee et al., 2006; Bianchi et al., 2016). Specifically, isoprene has a higher yield of HCHO than most non-alkene VOCs (Dufour et al., 2009).
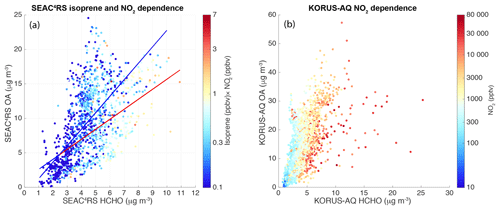
Figure 3(a) A scatter plot of OA vs. HCHO for SEAC4RS non-BB low-altitude data color coded with the product of NO2 and isoprene in log scale. The red and blue lines are the linear regression fits of high (> 0.5) and low (< 0.5) products of NO2 (ppbv) and isoprene (ppbv), respectively. (b) A scatter plot of OA vs. HCHO for KORUS-AQ data color coded by log(NO2).
A scatter plot of OA vs. HCHO for SEAC4RS low-altitude data is shown in Fig. 3a. The data are color coded by the product of in situ isoprene and NO2, attempting to capture time periods strongly influenced by oxidation products of isoprene at high NO conditions. No trends are evident when the data are instead color coded by NO2 or isoprene only. This may be because isoprene (biogenic source) and NO2 (anthropogenic sources) are generally not co-located in the US (Yu et al., 2016) and isoprene is the dominant source of HCHO compared to anthropogenic VOCs in the US (e.g., Millet et al., 2008). This plot shows that, at high NO2 and high isoprene conditions, less OA was formed for each HCHO produced generally. The correlation coefficient of 0.45 for high NO2 and isoprene conditions during SEAC4RS is not very high but still shows significant dependence of the OA–HCHO relationship on the product of NO2 and isoprene, considering that these are ambient data and other factors (e.g., different specific sources) also play a role in determining OA–HCHO relationships. This is consistent with high NO and isoprene conditions promoting HCHO formation over SOA formation. We also looked at the dependence on peroxy acetyl nitrate (PAN), as PAN is a product of the photooxidation of VOCs, including isoprene, in the presence of NO2. The dependence on PAN was not as clear as on the product of NO2 and isoprene.
KORUS-AQ OA vs. HCHO, color coded with NO2, is plotted in Fig. 3b. The OA–HCHO ratio clearly decreased as NO2 levels increased during KORUS-AQ, suggesting that high NO conditions accelerated HCHO formation more than they did SOA production. OA–HCHO relationships do not have dependence on local time of the day (not shown). This further confirms that NOx is an important factor that affects the OA–HCHO relationship. Compared to SEAC4RS, the KORUS-AQ OA–HCHO ratio does not depend on VOCs. This may be consistent with the dominant VOCs being anthropogenic VOCs that are co-located with NO sources. This may also suggest that the anthropogenic VOCs generally have a lower HCHO yield than isoprene does. Because OA and HCHO were tightly correlated during CalNex and DC3, we did not parse for NOx. The NOx range during DC3 low-altitude data was smaller than KORUS-AQ and SEAC4RS. DC3 OA–HCHO relationships only had a slight dependence on NO2 (not shown here), largely due to the limited dataset. The NOx range during CalNex low-altitude data was large. The OA and HCHO correlation during CalNex was very tight and the slope of OA–HCHO did not show clear dependence on NOx, which could be due to the combination of different VOC sources and NOx levels.
In situ OA–HCHO relationships from SEAC4RS low-altitude non-BB (Fig. 4a), KORUS-AQ low-altitude (Fig. 4b), and SEAC4RS BB (Fig. 4c) air masses were compared to GEOS-Chem model simulations (Fig. 4d–f) sampling along the corresponding flight tracks. Similar to the in situ data, GEOS-Chem model simulations also found correlations between OA and HCHO for these three regions, especially for SEAC4RS non-BB. GEOS-Chem was intensively validated with in situ measurements for the SE US (e.g., Marais et al., 2016; Kim et al., 2015). The ratios of the slopes between OA and HCHO for the US (SEAC4RS), South Korea (KORUS-AQ), and wildfire cases (SEAC4RS) from GEOS-Chem were , which was different from the in situ measurements of (Table 1). GEOS-Chem could not capture any wildfires in the US during SEAC4RS, which is probably due to poor representation of the BB emission inventory for US wildfires and also the coarse grid in GEOS-Chem. GEOS-Chem also significantly underpredicted the slope of OA to HCHO for South Korea. We attribute this to a likely underprediction of anthropogenic SOA, which was dominant in South Korea, in GEOS-Chem (Schroder et al., 2018), as well as a different mix of OA and HCHO sources in the US compared to South Korea and representation of these in GEOS-Chem. Although GEOS-Chem contains isoprene chemistry with a focus on the SE US (Marais et al., 2016), there is still room to improve the GEOS-Chem model especially for anthropogenic and BB sources, as well as anthropogenic OA formation mechanisms. For example, in the model, biogenic sources are more important than anthropogenic sources for the OA and HCHO budgets in South Korea, which is not the case from KORUS-AQ in situ measurements. In the model, a 10 % decrease of emissions from biogenic, anthropogenic, and BB sources results in 6 %, 3 %, and 1 % decreases in OA and 2 %, 1 %, and 0 % decreases in HCHO over South Korea in May 2016. However, the in situ airborne field campaign KORUS-AQ found that OA and HCHO were higher near anthropogenic emission sources compared to rural regions. The larger impact of biogenic sources compared to anthropogenic sources on OA and HCHO in the model can be due to both low-biased anthropogenic emission inventories and low-biased anthropogenic SOA. Improving anthropogenic emissions inventories in the models can bring model results closer to observations. Improving anthropogenic SOA, such as implementation of the SIMPLE model, in GEOS-Chem (Hodzic and Jimenez, 2011) can also improve the model results compared to observations. Measurements or measurement-constrained estimation with sufficient spatial and temporal coverage can help to narrow down the key factors (e.g., emission inventories or chemical schemes) in GEOS-Chem to better represent VOCs and OA globally. Furthermore, we did also find that GEOS-Chem could not capture the observed higher slope of OA to HCHO at high altitudes (not shown), which could be due to issues such as transport, OA lifetime, and OA production.
To utilize the derived in situ OA–HCHO relationship, the satellite HCHO columns need to be converted to surface HCHO concentrations. We used a vertical distribution factor η (cm−1) (Sect. 2.5), which is defined as the ratio of surface HCHO concentrations (molec cm−3) to HCHO column (molec cm−2), to estimate surface HCHO concentrations from satellite column measurements. Zhu et al. (2017) used the same vertical distribution factor for their study. The use of this factor is justified by the fact that the derived surface HCHO retained the spatial pattern of the satellite HCHO column and agreed with local surface measurements of HCHO for a multi-year average (Zhu et al., 2017).
We also investigated the main factors affecting the variation of the vertical distribution factor η. Because the factor is determined by HCHO vertical distributions, we examined three typical normalized HCHO vertical distribution profiles with the highest, median, and lowest η values for the SEAC4RS field campaign (Fig. 5). Because the sensitivity of OA estimates to η was investigated with η from different GEOS-Chem versions (Sect. 6.2), we did not compare HCHO vertical profiles from the model to the measurements from a comprehensive set of field campaigns. We chose SEAC4RS to illustrate the main factors impacting the η over the US because SEAC4RS had a larger spatial coverage than DC3 and CalNex. GEOS-Chem can generally capture the vertical profiles of measured HCHO. Boundary layer mixing height and surface emission strength are the dominant factors in determining the fraction of HCHO near the surface. Higher boundary layer mixing height results in lower η for SE US profiles, where there are biogenic sources of HCHO from the surface and HCHO has distinct concentration differences below and above the boundary layer. However, there are exceptions, such as for the profiles over the ocean and the coastal regions. Although the boundary layer is shallow in these regions, a large portion of HCHO resides above the boundary layer, resulting in low η. In these cases, surface emissions of HCHO or precursors are very small, and therefore methane oxidation makes a large contribution to the total HCHO column. High concentrations of HCHO (e.g., in BB plumes) lofted by convection can also impact the vertical profile (Barth et al., 2015), which is not further investigated because OA estimates with BB influences over the US are excluded in current study. Overall, the source intensities and boundary layer mixing height mostly determined the HCHO vertical profiles.
Table 2Cases to estimate OA surface concentrations, based on the choice of slope and intercept from a linear regression relationship between OA and HCHO data found in Table 1.
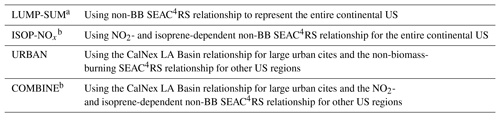
aSEAC4 RS was chosen to represent the entire continental US because it had the largest horizontal and vertical coverage. b In ISOP-NOx and COMBINE, when the product of NO2 column (Sect. 2.3) and surface isoprene emission rate (Sect. 2.4) was above the threshold of 5×1027 molec cm−2 atom C cm−2 s−1, the slope and intercept from SEAC4RS high isoprene and NO2 conditions were used. When the NO2 column isoprene emission product was below that threshold, the slope and intercept from SEAC4RS low isoprene and NO2 conditions were used. The threshold of “isoprene × NO2” was determined by its mean value over the SE US (32–35∘ N, 83–96∘ W). Large urban cities were categorized with high NO2 vertical columns (> 4×1015 molec cm−2) (Tong et al., 2015) based on the satellite NO2 levels over LA. Isoprene emissions instead of concentrations were used because global models use the isoprene emission inventory to simulate isoprene concentrations and the isoprene emission inventory is easier to access. Since isoprene has a short lifetime of up to a few hours (Guenther et al., 2006), the emissions have a similar spatiotemporal distribution to the concentrations.
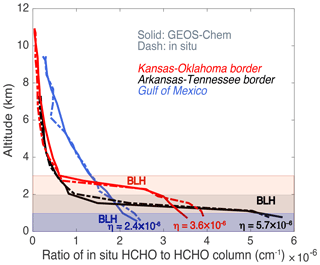
Figure 5Three typical vertical profiles of the ratio of in situ HCHO concentrations (molec cm−3) to integrated HCHO column from the SEAC4RS flight track. These three profiles were located at the Kansas–Oklahoma border (red), Arkansas–Tennessee border (black), and Gulf of Mexico (blue). Solid curves were from GEOS-Chem results and the dashed ones were from ISAF measurements. HCHO columns were integrated HCHO concentrations of these vertical profiles extrapolated from 0 to 10 km, assuming the HCHO values below and above the measured HCHO vertical profiles were the same as the HCHO at the lowest and highest altitudes sampled, respectively. The boundary layer heights (BLHs) of these three profiles are plotted by the shaded areas.
6.1 Variables to construct OA estimate
As mentioned in Sect. 2.5, the OA estimate value in each grid cell was estimated from monthly average satellite HCHO column observation by the linear Eq. (1). Satellite monthly average HCHO column data, ΩHCHO, were converted to surface HCHO concentrations by multiplying by the η(i) factor either from climatology a priori profiles or monthly average HCHO profiles. Surface OA was then estimated by multiplying the derived surface HCHO concentrations by the slope α(i) and adding the intercept β(i). The slope α(i) and intercept β(i) were determined from the linear regression of in situ OA and HCHO from aircraft field campaign data. The relationship between OA and HCHO varies but previous sections demonstrated that we can quantify the surface OA–HCHO relationship by their regions, sources, and chemical conditions (e.g., NOx and isoprene levels). To test the impact of the chosen OA–HCHO relationship on the calculated OA estimate, the OA estimate in the US was calculated using four different methods (see Table 2). The OA estimate was calculated on the monthly timescale, largely because OA estimate is based on OMI HCHO observations, and an uncertainty weighted average for a timescale of about 1 month (Gonzalo et al., 2015; Zhu et al., 2016) is needed to reduce the noise in daily OMI HCHO data. With improved satellite HCHO data from the Tropospheric Monitoring Instrument (TROPOMI), higher time resolution (e.g., weekly average) HCHO data could be useful to estimate OA in the future.
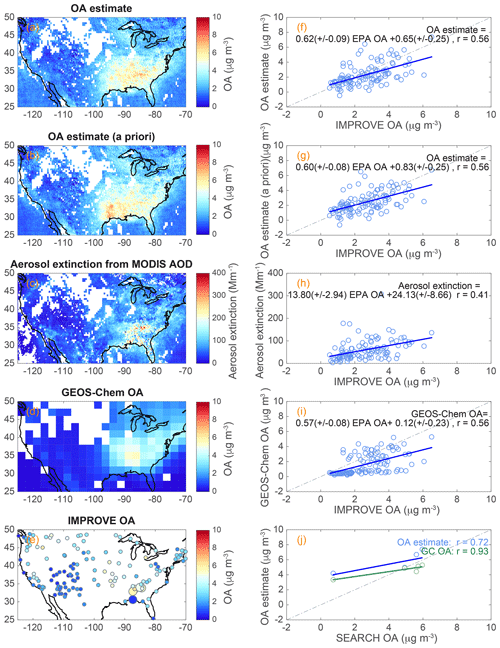
Figure 6(a) The maps of (a) surface OA estimate (LUMP-SUM) with η from GEOS-Chem v9-02, (b) surface OA estimate (LUMP-SUM) with η from a priori profiles, (c) surface aerosol extinction derived from MODIS AOD, (d) GEOS-Chem simulated surface OA, and (e) IMPROVE (small dots) and SEARCH (large dots) network ground sites color coded with OA concentrations for August 2013. The scatter plots of the (f, g) surface OA estimate, (h) surface aerosol extinction derived from MODIS AOD, and (i) surface GEOS-Chem OA vs. IMPROVE network ground sites' OA. IMPROVE sites' OAs were corrected for evaporation. (j) The scatter plots of the surface OA estimate and GEOS-Chem OA vs. SEARCH network ground sites' OA for August 2013. GEOS-Chem OA and the OA estimate did not have good correlations with SEARCH OA for other years (SI). For the scatter plots, linear regressions are shown (blue and green lines) and regression equations and correlation coefficients for the scatter plots are listed. The dashed lines in the scatter plots indicate the 1:1 line. Biomass burning data (UV aerosol index > 1.6) were excluded in all panels.
6.2 OA estimate over the US
The monthly average surface OA estimates over the US in August 2013 using SEAC4RS lump-sum slope and intercept (see Table 2) with different η are shown in Fig. 6a and b. Because BB regions in the US are not covered by smoke continuously during a period of time and it is challenging for satellite retrieval to separate thick BB plumes and clouds without information on the time and location of the burning, thick BB events (OMI UV aerosol index (UVAI) > 1.6) (Torres et al., 2007) were excluded and shown as the blank (white) grid cells in Fig. 6a and b. The same filter was also applied to aerosol extinction and GEOS-Chem OA abundance. To evaluate the representative quality of the OA estimate, OA estimate data were compared to the EPA IMPROVE ground sites' corrected-OA measurements over the US and SEARCH ground sites' OA measurements in the SE US (Sect. 2.2). The locations of IMPROVE and SEARCH sites are displayed in Fig. 6e as small and large dots, respectively. The dot color represents the average OA mass concentrations for August 2013.
Considering the uncertainties in satellite HCHO measurements, in using the campaign lump-sum OA–HCHO relationship to represent spatial resolved OA, in HCHO vertical profiles, and in ground IMPROVE network measurements, the correlation (correlation coefficient r=0.56) between the OA estimate and corrected IMPROVE network measurements (Fig. 6f and g) is reasonably good and indicates that the OA estimate can generally capture the variation of OA loading over the US. First, the correlation coefficient between HCHO SAO retrievals and in situ measurements during SEAC4RS was not high (r=0.24), but this may be partly because they were not sampled at the same time. The uncertainty in HCHO SAO data was likely less than 76 %. Second, the uncertainty in applying a campaign lump-sum OA–HCHO relationship to individual spatial resolved satellite HCHO data to estimate OA induced an uncertainty of 41 % according to the correlation coefficient of OA–HCHO in the field campaign. Third, η in the Fig. 6a OA estimate was from GEOS-Chem v9-02 output for the specific month of August 2013. η in the Fig. 6b OA estimate was from GEOS-Chem v9-01-03 climatology, the same as satellite data a priori profiles. The good correlations of OA estimates with IMPROVE OA indicate that OA estimates are not very sensitive to η from different model versions. The largest difference between the two OA estimates is their concentrations over east Texas. There are no IMPROVE OA measurements in east Texas to evaluate which works better. Fourth, the uncertainties in IMPROVE OA measurements, such as using a constant correction factor to correct the partial evaporation across all SE US sites, and the spatially dependent OA∕OC ratio (Tsigaridis et al., 2014), may also have contributed to the discrepancies between the OA estimate and EPA IMPROVE sites' OA. Therefore, higher quality of satellite HCHO data and refining OA–HCHO relationships will help improve our OA estimate products. These combined with a spatially resolved IMPROVE OA correction factor and OA∕OC ratios will help improve the correlation coefficients between OA estimates and IMPROVE OA.
The linear correlation between the OA estimate and IMPROVE OA measurements yielded a slope of 0.62 or 0.60, indicating that the OA estimate underestimated OA. First, the different data collection time for satellite data, in situ measurements, and ground observations could contribute to the bias. Satellite HCHO data were measured midday, in situ airborne OA and HCHO were measured during the daytime, and IMPROVE network organic carbon was collected day and night. Because ground OAs in the SE US were observed to have little diurnal variation (Xu et al., 2015; Hu et al., 2015), the different sampling time of ground and airborne OAs probably does not have a significant impact on the comparison of OA estimate and IMPROVE OA. Surface HCHO has evident diurnal profiles with the highest concentrations around midday (Kaiser et al., 2016), which could add uncertainties to OA estimate when using inconsistent time ranges of satellite HCHO data measured midday and in situ airborne OA–HCHO relationships measured in the daytime. The SEAC4RS HCHO concentrations were converted to 13:30 LT concentrations according to the average HCHO diurnal profile from the Southern Oxidant and Aerosol Study (SOAS) (Kaiser et al., 2016). The OA–HCHO relationship with HCHO converted to 13:30 LT yielded a slope of 5 % lower than the original OA–HCHO relationship. Second, the potential uncertainty (±30 %) in the OA∕OC ratio could also contribute to the systematic difference because we used OA∕OC of 2.1 and studies (e.g., Pye et al., 2017; Canagaratna et al., 2015) showed that the OA∕OC ratio can range from 1.4 to 2.8. Third, the potential underestimation of HCHO from satellite retrieval (by −37 %) (Zhu et al., 2016) compared to SEAC4RS may be one of the most important reasons that cause the systematic difference (low slope) between the OA estimate and IMPROVE OA according to Eq. (1). Satellite HCHO data corrected by the low bias (by −37 %) (Zhu et al., 2016) will increase our slopes of 0.60–0.62 to be close to the unity.
SEARCH OA data were also used to compare to the OA estimate. The correlation was good for August 2013. Although the SEARCH network OA measurements have better accuracy, the number of SEARCH sites is limited (five sites). The correlation of OA estimate and SEARCH OA varied dramatically in 2008–2013 (Fig. S1 in the Supplement). GEOS-Chem OA did not correlate with SEARCH OA except for the year 2013 (Fig. S1). As the IMPROVE network has more sites and spatial coverage, we used IMPROVE network data as ground OA measurements for comparison in the remainder of the discussion.
6.3 Comparison to aerosol extinction from AOD
To further evaluate the method of using satellite HCHO to derive an OA surface estimate, satellite aerosol measurements were used to approximate surface OA extinction for comparison. Satellite measurements of AOD were converted to surface extinction (see Sect. 2.6). Studies showed that OAs were a dominant component of aerosol mass and extinction during SEAC4RS (Kim et al., 2015; Wagner et al., 2015) and the fractions of OA were relatively constant (interdecile 62 %–74 %) (Wagner et al., 2015). Therefore, AOD variation is expected to generally reflect the OA variation during SEAC4RS. Satellite measurements from MISR can provide more aerosol property information to apportion total AOD to AOD of a subset of aerosols with small to medium size and round shape, which can better capture OA, when AOD is above 0.15 to 0.2 (Kahn and Gaitley, 2015; personal communication with Ralph Kahn, 2018). Because MISR cannot distinguish OA and other submicron aerosol components (e.g., sulfate and nitrate) and would cut off low AOD data which accounted for near half of the data over the US, we used total AOD to derive extinction for our comparison. The AOD-derived extinction map is shown in Fig. 6c, and the scatter plot of AOD-derived extinction and EPA-corrected OA is displayed in Fig. 6h. The same filter of high AI was also applied to AOD-derived extinction to remove BB plumes. Generally, the derived aerosol extinction had a correlation with IMPROVE OA, but the correlation was not as good as for the OA estimate with IMPROVE OA. The high surface aerosol extinctions (> 150 Mm−1) (outliers in the scatter plot) were located in the SE US and therefore were not due to potential contribution of dust and nitrate altering the shape of vertical profiles outside of the SE US. This indicates that the OA estimate derived from HCHO may be better than AOD at representing the concentrations of OA, even for the regions where AOD is dominated by OA (Xu et al., 2015).
6.4 Comparison to GEOS-Chem OA
Surface OA over the US from a GEOS-Chem simulation for August 2013 is shown in Fig. 6d, and the scatter plot of GEOS-Chem OA with IMPROVE OA is in Fig. 6i. Although HCHO vertical profiles from GEOS-Chem were used in OA estimate, the GEOS-Chem simulation had a coarser resolution than the OA estimate. To be comparable to the OA estimate, the scatter plot in Fig. 6i used GEOS-Chem results for the grid squares that overlapped with individual IMPROVE sites. Compared to the OA estimate, GEOS-Chem OA had a similar correlation coefficient with IMPROVE OA. Although the GEOS-Chem OA plot appeared more scattered, there were many GEOS-Chem data points close to zero when IMPROVE OA was low, making the overall correlation coefficient similar to that for the OA estimate. GEOS-Chem underpredicted IMPROVE OA more with a slope of 0.57 compared to the OA estimate. This is consistent with underprediction of anthropogenic OA in Marais et al. (2016).
6.5 OA estimate with different OA–HCHO relationships
OAs were estimated with different OA–HCHO relationships for four cases (Table 2). LUMP-SUM was using the non-BB SEAC4RS campaign lump-sum relationship, the same as shown in Fig. 6a; ISOP-NOx was using non-BB SEAC4RS NO2- and isoprene-dependent relationship; URBAN was using CalNex for large urban cities and SEAC4RS lump-sum for other US regions; and COMBINE was using CalNex for large urban cities and NO2- and isoprene-dependent non-BB SEAC4RS for other US regions. The OA estimates from the four cases (Table 2) were compared to IMPROVE OA and the correlation coefficients are shown in Fig. 7. In general, OA estimate results from the four cases were similar.
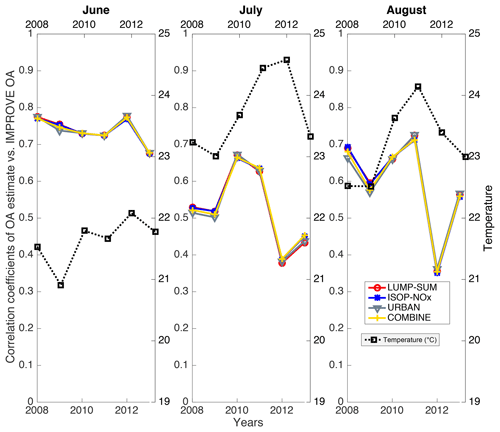
Figure 7The correlation coefficients of the linear regression between the OA estimate from four cases (red, blue, grey, and yellow) vs. EPA-corrected OA from 2008 to 2013 for June, July, and August. The monthly average ambient temperature is in black.
The details about how to implement chemical-factor-dependent OA estimates for the four cases are also provided in Table 2. Including the NO2–isoprene-dependent OA–HCHO relationship (ISOP-NOx case) showed a similar (or slightly worse) correlation between the OA estimate and IMPROVE OA. OMI NO2 column observations were used to represent surface NO2 levels and surface isoprene emissions from MEGAN were used to represent surface isoprene concentrations, assuming that NO2 column observations reflect surface NO2 distributions and isoprene emissions reflect the concentrations of isoprene due to its short lifetime (∼1 h). The detailed implementation is provided in the notes in Table 2. As the in situ data showed a moderate NO2–isoprene-dependent OA–HCHO relationship, we attributed this to the locations of IMPROVE sites in rural regions, the uncertainty in IMPROVE network measurements, the uncertainty in isoprene emissions from MEGAN, or factors (e.g., source-dependent OA–HCHO) that also need to be taken into account when determining the specific OA–HCHO relationship. Satellite OMI NO2 data (at 13:30 LT) were used to represent NO2 levels, big cities were defined as NO2 > 4×1015 molec cm−2, and the CalNex in situ OA–HCHO relationship was applied for big cities. It turned out that only one IMPROVE site (San Gabriel, SAGA1) near LA was affected by high NO2 and led to the insignificant change in URBAN compared to LUMP-SUM. This is not unexpected because IMPROVE sites are in rural regions. The OA estimate in SAGA1 decreased from 1.88 g m−3 from LUMP-SUM to 0.17 g m−3 in URBAN, while the measured OA in IMPROVE SAGA1 was 1.52 g m−3. This may infer that CalNex is not very consistent with SEAC4RS due to different sampling instruments, strategies and seasons. Lowering the NO2 threshold when defining big cities did not help improve the agreement either.
Because separating large urban areas and other regions and applying a simple chemical-regime-dependent in situ OA–HCHO relationship did not improve the agreement between the OA estimate and IMPROVE OA, we used the lump-sum OA–HCHO relationship to derive the OA estimate (shown in Fig. 6). SEAC4RS and DC3 only had a few low-altitude data in the midwest and did not cover the northeast US. The measured OA–HCHO relationship in the midwest did not show significant difference from the SE US. The scatter plots (Fig. 6f and g) of OA estimates and IMPROVE OA do not show outliers for the northeast and midwest. This indicates that using the SEAC4RS lump-sum OA–HCHO relationship can reasonably capture regions outside of the SE US.
6.6 Temporal variation of the agreement between OA estimate and IMPROVE OA
Besides August 2013 (see Fig. 6), the correlations between the OA estimate and IMPROVE OA for the summer months (June–July–August 2008–2013) were also examined and shown in Fig. 7. Generally, the correlation coefficients between the OA estimate and IMPROVE OA were > 0.5 for summer months of the years investigated. The correlation coefficients were generally higher in June compared to July and August. The lower average temperature in June might be related to the higher correlation coefficients. IMPROVE network aerosol samples were transported at ambient temperature in a truck and more organic vapors likely evaporated at higher temperature. The different temperatures and distances from IMPROVE sites to the laboratory may lead to inhomogeneous evaporation among the samples and result in lower correlation coefficients. Although higher temperatures in July and August may also lead to more BB, the average aerosol index over the US was not higher in July (mean: 0.35) and August (mean: 0.36) compared to June (mean: 0.39) for these years. The underlying cause for the lowest correlation coefficients in July and August 2012 is not clear and may be related to the severe drought in 2012 (Seco et al., 2015). The correlation coefficients were also low for the linear regressions (not shown) of IMPROVE OA with both GEOS-Chem OA and AOD-derived extinction. Because the lowest correlation coefficients were consistently observed for multiple OA-related products and not just the OA estimate, we attributed this to uncertainties in the IMPROVE OA measurements or some unknown bias shared by the satellite HCHO, GEOS-Chem OA, and satellite AOD.
6.7 South Korea OA estimate
We attempted to estimate an OA estimate for South Korea using airborne in situ measurements of OA and HCHO from the KORUS-AQ field campaign (KORUS-AQ Science Team, 2016) and SAO OMI HCHO measurements. The National Institute of Environmental Research (NIER) ground sites' OC measurements during KORUS-AQ over South Korea could be used to validate the OA estimate. However, OMI HCHO measurements were below the detection limit (Zhu et al., 2016) in May 2016. Also, there were no OMI data available in June 2016 when airborne measurements and ground sites' OC measurements were available during KORUS-AQ. Because an OA estimate for South Korea could not be well retrieved and validated, it was not presented in this study. Although an OA estimate for South Korea could not be retrieved in the current study, the consistency in the dependence of OA–HCHO relationships on chemical factors (e.g., emission sources, NOx, and altitudes) provides important information for potential application of chemical-factor-dependent OA–HCHO relationships to the geographical domain beyond the continental US, especially with improved satellite HCHO data from TROPOMI.
Because the OA estimate is based on satellite HCHO data, the detection limit of satellite HCHO data affects the quality of the OA estimate. Currently, due to the limited sensitivity of OMI for HCHO, the OA estimate is valid only when high levels of HCHO are present, such as during summertime and near large HCHO sources. With the new TROPOMI satellite instrument and future missions (TEMPO and GEMS), satellite HCHO measurements will have higher spatial and temporal resolutions and lower detection limits. These higher-quality satellite HCHO measurements will improve the quality and spatial and temporal coverage of our OA estimate.
Because the OA estimate uses the relationship of in situ HCHO and OA measurements, the coverage of in situ aircraft field campaigns will impact the OA estimate quality. Currently, in situ airborne measurements of OA and HCHO focus on the continental US. Extending measurements to regions such as African BB, South America, and east Asia, where HCHO and OA have high concentrations, will increase the spatial coverage of the OA estimate product. Ground site measurements of OA with consistent quality control in those regions will also be important for validating the OA estimate.
Improvement of satellite HCHO retrieval during the BB cases will also improve OA estimate quality. BB cases with high UV aerosol index over the US were excluded in the current OA estimate. With improvement in the satellite retrieval of HCHO, we may be able to estimate OA during BB cases over the US. Upcoming field campaigns such as the Fire Influence on Regional and Global Environments Experiment – Air Quality (FIREX-AQ) will provide opportunities to improve the OA estimate in BB cases in the US.
This OA estimate method has limitations in remote regions far away from HCHO sources. Because the lifetimes of HCHO (1–3 h) and OA (1 week) are different, the slopes and intercepts between HCHO and OA are expected to change when air masses are aged (e.g., in remote regions). HCHO is close to being in steady state with production rates roughly equal to loss rates while OA is not in steady state with a lifetime of a week. Therefore, OA can be accumulated relative to HCHO when air masses are aged. OA vs. HCHO from SEAC4RS and KORUS-AQ field campaigns, color coded with altitude as an indicator of air mass age, are plotted in Fig. S2a and b, respectively. A relative depletion of HCHO at high altitudes was observed due to its shorter lifetime. This also suggests that, at remote regions far away from the sources, the ratios of OA and HCHO could be much higher and the relationship between OA and HCHO derived near the sources may no longer apply. On the other hand, the lifetime of 1–3 h for HCHO does not imply that the OA estimate only works within this timescale. HCHO is formed from oxidation of transported gas-phase VOCs, including the oxidation products of the primary emitted VOCs, as well as of the slower-reacting VOCs (e.g., ethane and benzene). Most gas-to-particle oxidation processes that might produce HCHO can last up to 1–2 days (Palm et al., 2018). Figure S3 shows the ratios of OA and HCHO did not change significantly downwind for the Rim Fire plume for about 1 day of aging, which was determined by the distance from the source and the wind speed. A lower photolysis rate of HCHO in the plume can also contribute to this. However, we do not expect the relationship of OA and HCHO to remain past one to two boundary layer ventilation cycles (Palm et al., 2018). Although OA–HCHO relationships depend on air mass age, it does not largely affect our study for monthly average surface OA over the continental US because our OA estimates showed reasonably good agreement with ground sites IMPROVE OA measurements. This also indicates that SOAs are enhanced near the source regions statistically. Nault et al. (2018) also showed the production of HCHO and SOA are similar and plateau around 0.5–1 photochemical days. So, in the near field of emissions and chemistry, the production of these two species is similar; however, outside the near field of emissions and rapid chemistry, the long lifetime of OA vs. the steady state of HCHO would start controlling the slopes and correlations.
We have developed a satellite-based estimate of the surface OA concentration (“OA estimate”) based on in situ observations. This estimate is based on the empirical relationships of in situ OA and HCHO for several regions. OA and HCHO share VOC sources with different yields and lifetimes. Using surface OA and HCHO linear regression slopes and intercepts, we can relate surface HCHO to OA. To estimate the surface HCHO concentration from the satellite HCHO column, we used a vertical distribution factor η from either climatology satellite data a priori profiles or an updated model run for a specific period, which is largely determined by boundary layer height and surface emissions and found to reasonably retrieve surface HCHO from column HCHO.
The OA estimate over the continental US generally correlated well with EPA IMPROVE network OA measurements corrected for partial evaporation, with a biased low slope of 0.62 or 0.60, mostly due to underestimation of HCHO concentrations from the OMI HCHO retrieval. The good correlations are not only for the time during SEAC4RS but also for most summer months over the several years (2008–2013) investigated. Compared to aerosol extinction derived from AOD, the OA estimate had slightly higher correlation coefficients with IMPROVE OA. GEOS-Chem can predict OA with a similar correlation coefficient with IMPROVE OA compared to the OA estimate when GEOS-Chem was intensively validated with in situ measurements for the SE US. Better satellite HCHO data from TROPOMI and future TEMPO and GEMS and extending spatiotemporal coverage of in situ measurements will improve the quality and coverage of the OA estimate.
The OA estimate products, the GEOS-Chem outputs, and satellite HCHO data in this study can be obtained by contacting the corresponding author, Jin Liao (jin.liao@nasa.gov). In situ SEAC4RS data are available at https://doi.org/10.5067/Aircraft/SEAC4RS/Aerosol-TraceGas-Cloud (SEAC4RS Science Team, 2013). DC3 data are available at https://doi.org/10.5067/Aircraft/DC3/DC8/Aerosol-TraceGas (DC3 Science Team, 2012). KORUS-AQ data are available at https://doi.org/10.567/Suborbital/KORUSAQ/DATA01 (KORUS-AQ Science Team, 2016). CalNex data are available at https://www.esrl.noaa.gov/csd/groups/csd7/measurements/2010calnex/ (CalNex Science Team, 2010). Satellite NO2 data are available at https://doi.org/10.5067/Aura/OMI/DATA2017 (Krotkov, 2013). Satellite MODIS AOD data are available at https://ladsweb.nascom.nasa.gov/ (Levy and Hsu, 2015).
The supplement related to this article is available online at: https://doi.org/10.5194/acp-19-2765-2019-supplement.
JL performed the analysis and wrote the paper. TFH directed the research topic and discussed the analysis with JL. TFH, GMW, JSC, AF, and CW provided in situ HCHO measurements. JLJ, PCJ, and BAN provided in situ OA measurements. EAM provided GEOS-Chem model results. GGA and KC provided satellite HCHO data. HTJ provided MODIS AOD data. TBR provided in situ NO2 measurements. AW provided in situ isoprene and acetonitrile measurements. GMW, TFH, JSC, JLJ, BAN, PCJ, EAM, and GGA provided constructive comments to help improve the paper. All authors have reviewed and edited the paper.
The authors declare that they have no conflict of interest.
Jin Liao, Thomas F. Hanisco, Glenn M. Wolfe, and Jason St. Clair were supported by NASA grants NNH15ZDA001N and
NNH10ZDA001N. Benjamin A. Nault, Pedro Campuzano-Jost,
and Jose L. Jimenez were supported by NASA grants NNX15AT96G and
80NSSC18K0630. Armin Wisthaler and PTR-MS measurements during DC3, SEAC4RS, and
KORUS-AQ were supported by the Austrian Federal Ministry for Transport,
Innovation and Technology (bmvit) through the Austrian Space Applications
Programme (ASAP) of the Austrian Research Promotion Agency (FFG). The PTR-MS
instrument team (Philipp Eichler, Lisa Kaiser, Tomas Mikoviny, and Markus Müller) is
acknowledged for their field support. We thank Eric Edgerton for providing the
SEARCH network data.
Edited by: Sally E. Pusede
Reviewed by: two anonymous referees
Anderson, D. C., Loughner, C. P., Diskin, G., Weinheimer, A., Canty, T. P., Salawitch, R. J., Worden, H. M., Fried, A., Mikoviny, T., Wisthaler, A., and Dickerson, R. R.: Measured and modeled CO and NOy in DISCOVER-AQ: An evaluation of emissions and chemistry over the eastern US, Atmos. Environ., 96, 78–87, https://doi.org/10.1016/j.atmosenv.2014.07.004, 2014.
Attwood, A. R., Washenfelder, R. A., Brock, C. A., Hu, W., Baumann, K., Campuzano-Jost, P., Day, D. A., Edgerton, E. S., Murphy, D. M., Palm, B. B., McComiskey, A., Wagner, N. L., de Sa, S. S., Ortega, A., Martin, S. T., Jimenez, J. L., and Brown, S. S.: Trends in sulfate and organic aerosol mass in the Southeast U.S.: Impact on aerosol optical depth and radiative forcing, Geophys. Res. Lett., 41, 7701–7709, https://doi.org/10.1002/2014gl061669, 2014.
Bahreini, R., Middlebrook, A. M., de Gouw, J. A., Warneke, C., Trainer, M., Brock, C. A., Stark, H., Brown, S. S., Dube, W. P., Gilman, J. B., Hall, K., Holloway, J. S., Kuster, W. C., Perring, A. E., Prevot, A. S. H., Schwarz, J. P., Spackman, J. R., Szidat, S., Wagner, N. L., Weber, R. J., Zotter, P., and Parrish, D. D.: Gasoline emissions dominate over diesel in formation of secondary organic aerosol mass, Geophys. Res. Lett., 39, L06805, 10.1029/2011gl050718, 2012.
Baker, A. K., Beyersdorf, A. J., Doezema, L. A., Katzenstein, A., Meinardi, S., Simpson, I. J., Blake, D. R., and Rowland, F. S.: Measurements of nonmethane hydrocarbons in 28 United States cities, Atmos. Environ., 42, 170–182, https://doi.org/10.1016/j.atmosenv.2007.09.007, 2008.
Barth, M. C., Cantrell, C. A., Brune, W. H., Rutledge, S. A., Crawford, J. H., Huntrieser, H., Carey, L. D., MacGorman, D., Weisman, M., Pickering, K. E., Bruning, E., Anderson, B., Apel, E., Biggerstaff, M., Campos, T., Campuzano-Jost, P., Cohen, R., Crounse, J., Day, D. A., Diskin, G., Flocke, F., Fried, A., Garland, C., Heikes, B., Honomichl, S., Hornbrook, R., Huey, L. G., Jimenez, J. L., Lang, T., Lichtenstern, M., Mikoviny, T., Nault, B., O'Sullivan, D., Pan, L. L., Peischl, J., Pollack, I., Richter, D., Riemer, D., Ryerson, T., Schlager, H., St Clair, J., Walega, J., Weibring, P., Weinheimer, A., Wennberg, P., Wisthaler, A., Wooldridge, P. J., and Ziegler, C.: The Deep Convective Clouds and Chemistry (Dc3) Field Campaign, B. Am. Meteorol. Soc., 96, 1281–1309, https://doi.org/10.1175/Bams-D-13-00290.1, 2015.
Bianchi, F., Barmet, P., Stirnweis, L., El Haddad, I., Platt, S. M., Saurer, M., Lötscher, C., Siegwolf, R., Bigi, A., Hoyle, C. R., DeCarlo, P. F., Slowik, J. G., Prévôt, A. S. H., Baltensperger, U., and Dommen, J.: Contribution of methane to aerosol carbon mass, Atmos. Environ., 141, 41–47, https://doi.org/10.1016/j.atmosenv.2016.06.036, 2016.
CalNex Science Team: CalNex NOAA P3 aircraft data archive, available at: https://esrl.noaa.gov/csd/groups/csd7/measurements/2010calnex/P3/DataDownload/ (last access: 18 July 2016), 2010.
Canagaratna, M. R., Jayne, J. T., Jimenez, J. L., Allan, J. D., Alfarra, M. R., Zhang, Q., Onasch, T. B., Drewnick, F., Coe, H., Middlebrook, A., Delia, A., Williams, L. R., Trimborn, A. M., Northway, M. J., DeCarlo, P. F., Kolb, C. E., Davidovits, P., and Worsnop, D. R.: Chemical and microphysical characterization of ambient aerosols with the aerodyne aerosol mass spectrometer, Mass Spectrom. Rev., 26, 185–222, https://doi.org/10.1002/mas.20115, 2007.
Canagaratna, M. R., Jimenez, J. L., Kroll, J. H., Chen, Q., Kessler, S. H., Massoli, P., Hildebrandt Ruiz, L., Fortner, E., Williams, L. R., Wilson, K. R., Surratt, J. D., Donahue, N. M., Jayne, J. T., and Worsnop, D. R.: Elemental ratio measurements of organic compounds using aerosol mass spectrometry: characterization, improved calibration, and implications, Atmos. Chem. Phys., 15, 253–272, https://doi.org/10.5194/acp-15-253-2015, 2015.
Carslaw, K. S., Lee, L. A., Reddington, C. L., Pringle, K. J., Rap, A., Forster, P. M., Mann, G. W., Spracklen, D. V., Woodhouse, M. T., Regayre, L. A., and Pierce, J. R.: Large contribution of natural aerosols to uncertainty in indirect forcing, Nature, 503, 67–71, https://doi.org/10.1038/nature12674, 2013.
Cazorla, M., Wolfe, G. M., Bailey, S. A., Swanson, A. K., Arkinson, H. L., and Hanisco, T. F.: A new airborne laser-induced fluorescence instrument for in situ detection of formaldehyde throughout the troposphere and lower stratosphere, Atmos. Meas. Tech., 8, 541–552, https://doi.org/10.5194/amt-8-541-2015, 2015.
Cubison, M. J., Ortega, A. M., Hayes, P. L., Farmer, D. K., Day, D., Lechner, M. J., Brune, W. H., Apel, E., Diskin, G. S., Fisher, J. A., Fuelberg, H. E., Hecobian, A., Knapp, D. J., Mikoviny, T., Riemer, D., Sachse, G. W., Sessions, W., Weber, R. J., Weinheimer, A. J., Wisthaler, A., and Jimenez, J. L.: Effects of aging on organic aerosol from open biomass burning smoke in aircraft and laboratory studies, Atmos. Chem. Phys., 11, 12049–12064, https://doi.org/10.5194/acp-11-12049-2011, 2011.
Day, D. A., Wooldridge, P. J., Dillon, M. B., Thornton, J. A., and Cohen, R. C.: A thermal dissociation laser-induced fluorescence instrument for in situ detection of NO2, peroxy nitrates, alkyl nitrates, and HNO3, J. Geophys. Res.-Atmos., 107, 4046, https://doi.org/10.1029/2001jd000779, 2002.
DC3 science team: DC3 NASA DC8 aircraft data achive, https://doi.org/10.5067/Aircraft/DC3/DC8/Aerosol-TraceGas, 2012.
DeCarlo, P. F., Kimmel, J. R., Trimborn, A., Northway, M. J., Jayne, J. T., Aiken, A. C., Gonin, M., Fuhrer, K., Horvath, T., Docherty, K. S., Worsnop, D. R., and Jimenez, J. L.: Field-deployable, high-resolution, time-of-flight aerosol mass spectrometer, Anal. Chem., 78, 8281–8289, https://doi.org/10.1021/ac061249n, 2006.
DeCarlo, P. F., Ulbrich, I. M., Crounse, J., de Foy, B., Dunlea, E. J., Aiken, A. C., Knapp, D., Weinheimer, A. J., Campos, T., Wennberg, P. O., and Jimenez, J. L.: Investigation of the sources and processing of organic aerosol over the Central Mexican Plateau from aircraft measurements during MILAGRO, Atmos. Chem. Phys., 10, 5257–5280, https://doi.org/10.5194/acp-10-5257-2010, 2010.
Dufour, G., Wittrock, F., Camredon, M., Beekmann, M., Richter, A., Aumont, B., and Burrows, J. P.: SCIAMACHY formaldehyde observations: constraint for isoprene emission estimates over Europe?, Atmos. Chem. Phys., 9, 1647–1664, https://doi.org/10.5194/acp-9-1647-2009, 2009.
Drewnick, F., Hings, S. S., DeCarlo, P., Jayne, J. T., Gonin, M., Fuhrer, K., Weimer, S., Jimenez, J. L., Demerjian, K. L., Borrmann, S., and Worsnop, D. R.: A new time-of-flight aerosol mass spectrometer (TOF-AMS) – Instrument description and first field deployment, Aerosol. Sci. Tech., 39, 637–658, https://doi.org/10.1080/02786820500182040, 2005.
Dunlea, E. J., DeCarlo, P. F., Aiken, A. C., Kimmel, J. R., Peltier, R. E., Weber, R. J., Tomlinson, J., Collins, D. R., Shinozuka, Y., McNaughton, C. S., Howell, S. G., Clarke, A. D., Emmons, L. K., Apel, E. C., Pfister, G. G., van Donkelaar, A., Martin, R. V., Millet, D. B., Heald, C. L., and Jimenez, J. L.: Evolution of Asian aerosols during transpacific transport in INTEX-B, Atmos. Chem. Phys., 9, 7257–7287, https://doi.org/10.5194/acp-9-7257-2009, 2009.
Edgerton, E. S., Hartsell, B. E., Saylor, R. D., Jansen, J. J., Hansen, D. A., and Hidy, G. M.: The Southeastern Aerosol Research and Characterization Study, part 3: Continuous measurements of fine particulate matter mass and composition, J. Air Waste Manage., 56, 1325–1341, https://doi.org/10.1080/10473289.2006.10464585, 2006.
Gelaro, R., McCarty, W., Suarez, M. J., Todling, R., Molod, A., Takacs, L., Randles, C. A., Darmenov, A., Bosilovich, M. G., Reichle, R., Wargan, K., Coy, L., Cullather, R., Draper, C., Akella, S., Buchard, V., Conaty, A., da Silva, A. M., Gu, W., Kim, G. K., Koster, R., Lucchesi, R., Merkova, D., Nielsen, J. E., Partyka, G., Pawson, S., Putman, W., Rienecker, M., Schubert, S. D., Sienkiewicz, M., and Zhao, B.: The Modern-Era Retrospective Analysis for Research and Applications, Version 2 (MERRA-2), J. Climate, 30, 5419–5454, https://doi.org/10.1175/Jcli-D-16-0758.1, 2017.
Giglio, L., Randerson, J. T., and van der Werf, G. R.: Analysis of daily, monthly, and annual burned area using the fourth-generation global fire emissions database (GFED4): J. Geophys. Res.-Biogeo., 118, 317–328, https://doi.org/10.1002/jgrg.20042, 2013.
González Abad, G., Liu, X., Chance, K., Wang, H., Kurosu, T. P., and Suleiman, R.: Updated Smithsonian Astrophysical Observatory Ozone Monitoring Instrument (SAO OMI) formaldehyde retrieval, Atmos. Meas. Tech., 8, 19–32, https://doi.org/10.5194/amt-8-19-2015, 2015.
Guenther, A., Karl, T., Harley, P., Wiedinmyer, C., Palmer, P. I., and Geron, C.: Estimates of global terrestrial isoprene emissions using MEGAN (Model of Emissions of Gases and Aerosols from Nature), Atmos. Chem. Phys., 6, 3181–3210, https://doi.org/10.5194/acp-6-3181-2006, 2006.
Hand, J. L., Schichtel, B. A., Malm, W. C., and Frank, N. H.: Spatial and Temporal Trends in PM2.5 Organic and Elemental Carbon across the United States, Adv. Meteorol., 2013, 367674, https://doi.org/10.1155/2013/367674, 2013.
Hand, J. L., Schichtel, B. A., Malm, W. C., Pitchford, M., and Frank, N. H.: Spatial and seasonal patterns in urban influence on regional concentrations of speciated aerosols across the United States, J. Geophys. Res.-Atmos., 119, 12832–12849, https://doi.org/10.1002/2014jd022328, 2014.
Harvard-Smithsonian Center for Astrophysics, OMI HCHO data product description: OMHCHO README FILE, available at: https://www.cfa.harvard.edu/atmosphere/Instruments/OMI/PGEReleases/READMEs/OMHCHO_README_v3.0.pdf, last access: 28 January 2017.
Hayes, P. L., Ortega, A. M., Cubison, M. J., Froyd, K. D., Zhao, Y., Cliff, S. S., Hu, W. W., Toohey, D. W., Flynn, J. H., Lefer, B. L., Grossberg, N., Alvarez, S., Rappenglueck, B., Taylor, J. W., Allan, J. D., Holloway, J. S., Gilman, J. B., Kuster, W. C., De Gouw, J. A., Massoli, P., Zhang, X., Liu, J., Weber, R. J., Corrigan, A. L., Russell, L. M., Isaacman, G., Worton, D. R., Kreisberg, N. M., Goldstein, A. H., Thalman, R., Waxman, E. M., Volkamer, R., Lin, Y. H., Surratt, J. D., Kleindienst, T. E., Offenberg, J. H., Dusanter, S., Griffith, S., Stevens, P. S., Brioude, J., Angevine, W. M., and Jimenez, J. L.: Organic aerosol composition and sources in Pasadena, California, during the 2010 CalNex campaign, J. Geophys. Res.-Atmos., 118, 9233–9257, https://doi.org/10.1002/jgrd.50530, 2013.
Hayes, P. L., Carlton, A. G., Baker, K. R., Ahmadov, R., Washenfelder, R. A., Alvarez, S., Rappenglück, B., Gilman, J. B., Kuster, W. C., d e Gouw, J. A., Zotter, P., Prévôt, A. S. H., Szidat, S., Kleindienst, T. E., Offenberg, J. H., Ma, P. K., and Jimenez, J. L.: Modeling the formation and aging of secondary organic aerosols in Los Angeles during CalNex 2010, Atmos. Chem. Phys., 15, 5773–5801, https://doi.org/10.5194/acp-15-5773-2015, 2015.
Heald, C. L., Goldstein, A. H., Allan, J. D., Aiken, A. C., Apel, E., Atlas, E. L., Baker, A. K., Bates, T. S., Beyersdorf, A. J., Blake, D. R., Campos, T., Coe, H., Crounse, J. D., DeCarlo, P. F., de Gouw, J. A., Dunlea, E. J., Flocke, F. M., Fried, A., Goldan, P., Griffin, R. J., Herndon, S. C., Holloway, J. S., Holzinger, R., Jimenez, J. L., Junkermann, W., Kuster, W. C., Lewis, A. C., Meinardi, S., Millet, D. B., Onasch, T., Polidori, A., Quinn, P. K., Riemer, D. D., Roberts, J. M., Salcedo, D., Sive, B., Swanson, A. L., Talbot, R., Warneke, C., Weber, R. J., Weibring, P., Wennberg, P. O., Worsnop, D. R., Wittig, A. E., Zhang, R., Zheng, J., and Zheng, W.: Total observed organic carbon (TOOC) in the atmosphere: a synthesis of North American observations, Atmos. Chem. Phys., 8, 2007–2025, https://doi.org/10.5194/acp-8-2007-2008, 2008.
Heald, C. L., Coe, H., Jimenez, J. L., Weber, R. J., Bahreini, R., Middlebrook, A. M., Russell, L. M., Jolleys, M., Fu, T.-M., Allan, J. D., Bower, K. N., Capes, G., Crosier, J., Morgan, W. T., Robinson, N. H., Williams, P. I., Cubison, M. J., DeCarlo, P. F., and Dunlea, E. J.: Exploring the vertical profile of atmospheric organic aerosol: comparing 17 aircraft field campaigns with a global model, Atmos. Chem. Phys., 11, 12673–12696, https://doi.org/10.5194/acp-11-12673-2011, 2011.
Hidy, G. M., Blanchard, C. L., Baumann, K., Edgerton, E., Tanenbaum, S., Shaw, S., Knipping, E., Tombach, I., Jansen, J., and Walters, J.: Chemical climatology of the southeastern United States, 1999–2013, Atmos. Chem. Phys., 14, 11893–11914, https://doi.org/10.5194/acp-14-11893-2014, 2014.
Hodzic, A. and Jimenez, J. L.: Modeling anthropogenically controlled secondary organic aerosols in a megacity: a simplified framework for global and climate models, Geosci. Model Dev., 4, 901–917, https://doi.org/10.5194/gmd-4-901-2011, 2011.
Hu, W. W., Campuzano-Jost, P., Palm, B. B., Day, D. A., Ortega, A. M., Hayes, P. L., Krechmer, J. E., Chen, Q., Kuwata, M., Liu, Y. J., de Sá, S. S., McKinney, K., Martin, S. T., Hu, M., Budisulistiorini, S. H., Riva, M., Surratt, J. D., St. Clair, J. M., Isaacman-Van Wertz, G., Yee, L. D., Goldstein, A. H., Carbone, S., Brito, J., Artaxo, P., de Gouw, J. A., Koss, A., Wisthaler, A., Mikoviny, T., Karl, T., Kaser, L., Jud, W., Hansel, A., Docherty, K. S., Alexander, M. L., Robinson, N. H., Coe, H., Allan, J. D., Canagaratna, M. R., Paulot, F., and Jimenez, J. L.: Characterization of a real-time tracer for isoprene epoxydiols-derived secondary organic aerosol (IEPOX-SOA) from aerosol mass spectrometer measurements, Atmos. Chem. Phys., 15, 11807–11833, https://doi.org/10.5194/acp-15-11807-2015, 2015.
Hudson, P. K., Murphy, D. M., Cziczo, D. J., Thomson, D. S., de Gouw, J. A., Warneke, C., Holloway, J., Jost, J. R., and Hubler, G.: Biomass-burning particle measurements: Characteristic composition and chemical processing, J. Geophys. Res.-Atmos., 109, D23s27, https://doi.org/10.1029/2003jd004398, 2004.
IPCC 2013: Climate Change 2013: The Physical Science Basis, Contribution of Working Group I to the Fifth Assessment Report of the Intergovernmental Panel on Climate Change, edited by: Stocker, T. F., Qin, D., Plattner, G.-K., Tignor, M., Allen, S. K., Boschung, J., Nauels, A., Xia, Y., Bex, V., and Midgley, P. M., Cambridge University Press, Cambridge, United Kingdom and New York, NY, USA, 1535 pp., https://doi.org/10.1017/CBO9781107415324.
Jimenez, J. L., Canagaratna, M. R., Donahue, N. M., Prevot, A. S. H., Zhang, Q., Kroll, J. H., DeCarlo, P. F., Allan, J. D., Coe, H., Ng, N. L., Aiken, A. C., Docherty, K. S., Ulbrich, I. M., Grieshop, A. P., Robinson, A. L., Duplissy, J., Smith, J. D., Wilson, K. R., Lanz, V. A., Hueglin, C., Sun, Y. L., Tian, J., Laaksonen, A., Raatikainen, T., Rautiainen, J., Vaattovaara, P., Ehn, M., Kulmala, M., Tomlinson, J. M., Collins, D. R., Cubison, M. J., Dunlea, E. J., Huffman, J. A., Onasch, T. B., Alfarra, M. R., Williams, P. I., Bower, K., Kondo, Y., Schneider, J., Drewnick, F., Borrmann, S., Weimer, S., Demerjian, K., Salcedo, D., Cottrell, L., Griffin, R., Takami, A., Miyoshi, T., Hatakeyama, S., Shimono, A., Sun, J. Y., Zhang, Y. M., Dzepina, K., Kimmel, J. R., Sueper, D., Jayne, J. T., Herndon, S. C., Trimborn, A. M., Williams, L. R., Wood, E. C., Middlebrook, A. M., Kolb, C. E., Baltensperger, U., and Worsnop, D. R.: Evolution of Organic Aerosols in the Atmosphere, Science, 326, 1525–1529, https://doi.org/10.1126/science.1180353, 2009.
Jimenez, J. L., Canagaratna, M. R., Drewnick, F., Allan, J. D., Alfarra, M. R., Middlebrook, A. M., Slowik, J. G., Zhang, Q., Coe, H., Jayne, J. T., and Worsnop, D. R.: Comment on “The effects of molecular weight and thermal decomposition on the sensitivity of a thermal desorption aerosol mass spectrometer”, Aerosol. Sci. Tech., 50, I–XV, https://doi.org/10.1080/02786826.2016.1205728, 2016.
Kahn, R. A. and Gaitley, B. J.: An analysis of global aerosol type as retrieved by MISR, J. Geophys. Res.-Atmos., 120, 4248–4281, https://doi.org/10.1002/2015jd023322, 2015.
Kaiser, J., Skog, K. M., Baumann, K., Bertman, S. B., Brown, S. B., Brune, W. H., Crounse, J. D., de Gouw, J. A., Edgerton, E. S., Feiner, P. A., Goldstein, A. H., Koss, A., Misztal, P. K., Nguyen, T. B., Olson, K. F., St. Clair, J. M., Teng, A. P., Toma, S., Wennberg, P. O., Wild, R. J., Zhang, L., and Keutsch, F. N.: Speciation of OH reactivity above the canopy of an isoprene-dominated forest, Atmos. Chem. Phys., 16, 9349–9359, https://doi.org/10.5194/acp-16-9349-2016, 2016.
Kaiser, J., Jacob, D. J., Zhu, L., Travis, K. R., Fisher, J. A., González Abad, G., Zhang, L., Zhang, X., Fried, A., Crounse, J. D., St. Clair, J. M., and Wisthaler, A.: High-resolution inversion of OMI formaldehyde columns to quantify isoprene emission on ecosystem-relevant scales: application to the southeast US, Atmos. Chem. Phys., 18, 5483–5497, https://doi.org/10.5194/acp-18-5483-2018, 2018.
Kiehl, J. T. and Briegleb, B. P.: The Relative Roles of Sulfate Aerosols and Greenhouse Gases in Climate Forcing, Science, 260, 311–314, https://doi.org/10.1126/science.260.5106.311, 1993.
Kim, P. S., Jacob, D. J., Fisher, J. A., Travis, K., Yu, K., Zhu, L., Yantosca, R. M., Sulprizio, M. P., Jimenez, J. L., Campuzano-Jost, P., Froyd, K. D., Liao, J., Hair, J. W., Fenn, M. A., Butler, C. F., Wagner, N. L., Gordon, T. D., Welti, A., Wennberg, P. O., Crounse, J. D., St. Clair, J. M., Teng, A. P., Millet, D. B., Schwarz, J. P., Markovic, M. Z., and Perring, A. E.: Sources, seasonality, and trends of southeast US aerosol: an integrated analysis of surface, aircraft, and satellite observations with the GEOS-Chem chemical transport model, Atmos. Chem. Phys., 15, 10411–10433, https://doi.org/10.5194/acp-15-10411-2015, 2015.
KORUS-AQ science team: KORUS-AQ NASA DC8 aircraft data archive, https://doi.org/10.567/Suborbital/KORUSAQ/DATA01, 2016.
Kroll, J. H., Ng, N. L., Murphy, S. M., Flagan, R. C., and Seinfeld, J. H.: Secondary organic aerosol formation from isoprene photooxidation, Environ. Sci. Technol., 40, 1869–1877, https://doi.org/10.1021/es0524301, 2006.
Krotkov, N. A.: OMI/Aura NO2 Cloud-Screened Total and Tropospheric Column L3 Global Gridded V3, NASA Goddard Space Flight Center, Goddard Earth Sciences Data and Information Services Center (GES DISC), https://doi.org/10.5067/Aura/OMI/DATA3007, 2013.
Lamarque, J.-F., Bond, T. C., Eyring, V., Granier, C., Heil, A., Klimont, Z., Lee, D., Liousse, C., Mieville, A., Owen, B., Schultz, M. G., Shindell, D., Smith, S. J., Stehfest, E., Van Aardenne, J., Cooper, O. R., Kainuma, M., Mahowald, N., McConnell, J. R., Naik, V., Riahi, K., and van Vuuren, D. P.: Historical (1850–2000) gridded anthropogenic and biomass burning emissions of reactive gases and aerosols: methodology and application, Atmos. Chem. Phys., 10, 7017–7039, https://doi.org/10.5194/acp-10-7017-2010, 2010.
Lamsal, L. N., Krotkov, N. A., Celarier, E. A., Swartz, W. H., Pickering, K. E., Bucsela, E. J., Gleason, J. F., Martin, R. V., Philip, S., Irie, H., Cede, A., Herman, J., Weinheimer, A., Szykman, J. J., and Knepp, T. N.: Evaluation of OMI operational standard NO2 column retrievals using in situ and surface-based NO2 observations, Atmos. Chem. Phys., 14, 11587–11609, https://doi.org/10.5194/acp-14-11587-2014, 2014.
Lee, A., Goldstein, A. H., Kroll, J. H., Ng, N. L., Varutbangkul, V., Flagan, R. C., and Seinfeld, J. H.: Gas-phase products and secondary aerosol yields from the photooxidation of 16 different terpenes, J. Geophys. Res.-Atmos., 111, D17305, https://doi.org/10.1029/2006JD007050, 2006.
Lee-Taylor, J., Hodzic, A., Madronich, S., Aumont, B., Camredon, M., and Valorso, R.: Multiday production of condensing organic aerosol mass in urban and forest outflow, Atmos. Chem. Phys., 15, 595–615, https://doi.org/10.5194/acp-15-595-2015, 2015.
Levelt, P. F., Van den Oord, G. H. J., Dobber, M. R., Malkki, A., Visser, H., de Vries, J., Stammes, P., Lundell, J. O. V., and Saari, H.: The Ozone Monitoring Instrument, IEEE T. Geosci. Remote, 44, 1093–1101, https://doi.org/10.1109/Tgrs.2006.872333, 2006.
Levy, R. and Hsu, C.: MODIS Atmosphere L2 Aerosol Product, NASA MODIS Adaptive Processing System, Goddard Space Flight Center, USA, https://doi.org/10.5067/MODIS/MYD04_L2.061, 2015.
Levy, R. C., Munchak, L. A., Mattoo, S., Patadia, F., Remer, L. A., and Holz, R. E.: Towards a long-term global aerosol optical depth record: applying a consistent aerosol retrieval algorithm to MODIS and VIIRS-observed reflectance, Atmos. Meas. Tech., 8, 4083–4110, https://doi.org/10.5194/amt-8-4083-2015, 2015.
Liu, X. X., Huey, L. G., Yokelson, R. J., Selimovic, V., Simpson, I. J., Muller, M., Jimenez, J. L., Campuzano-Jost, P., Beyersdorf, A. J., Blake, D. R., Butterfield, Z., Choi, Y., Crounse, J. D., Day, D. A., Diskin, G. S., Dubey, M. K., Fortner, E., Hanisco, T. F., Hu, W. W., King, L. E., Kleinman, L., Meinardi, S., Mikoviny, T., Onasch, T. B., Palm, B. B., Peischl, J., Pollack, I. B., Ryerson, T. B., Sachse, G. W., Sedlacek, A. J., Shilling, J. E., Springston, S., St Clair, J. M., Tanner, D. J., Teng, A. P., Wennberg, P. O., Wisthaler, A., and Wolfe, G. M.: Airborne measurements of western US wildfire emissions: Comparison with prescribed burning and air quality implications, J. Geophys. Res.-Atmos., 122, 6108–6129, https://doi.org/10.1002/2016jd026315, 2017.
Malm, W. C., Sisler, J. F., Huffman, D., Eldred, R. A., and Cahill, T. A.: Spatial and Seasonal Trends in Particle Concentration and Optical Extinction in the United-States, J. Geophys. Res.-Atmos., 99, 1347–1370, https://doi.org/10.1029/93jd02916, 1994.
Malm, W. C., Schichtel, B. A., Hand, J. L., and Collett, J. L.: Concurrent Temporal and Spatial Trends in Sulfate and Organic Mass Concentrations Measured in the IMPROVE Monitoring Program, J. Geophys. Res.-Atmos., 122, 10341–10355, https://doi.org/10.1002/2017jd026865, 2017.
Marais, E. A., Jacob, D. J., Jimenez, J. L., Campuzano-Jost, P., Day, D. A., Hu, W., Krechmer, J., Zhu, L., Kim, P. S., Miller, C. C., Fisher, J. A., Travis, K., Yu, K., Hanisco, T. F., Wolfe, G. M., Arkinson, H. L., Pye, H. O. T., Froyd, K. D., Liao, J., and McNeill, V. F.: Aqueous-phase mechanism for secondary organic aerosol formation from isoprene: application to the southeast United States and co-benefit of SO2 emission controls, Atmos. Chem. Phys., 16, 1603–1618, https://doi.org/10.5194/acp-16-1603-2016, 2016.
Marais, E. A., Jacob, D. J., Turner, J. R., and Mickley, L. J.: Evidence of 1991-2013 decrease of biogenic secondary organic aerosol in response to SO2 emission controls, Environ. Res. Lett., 12, 054018, https://doi.org/10.1088/1748-9326/aa69c8, 2017.
McDonald, B. C., de Gouw, J. A., Gilman, J. B., Jathar, S. H., Akherati, A., Cappa, C. D., Jimenez, J. L., Lee-Taylor, J., Hayes, P. L., McKeen, S. A., Cui, Y. Y., Kim, S. W., Gentner, D. R., Isaacman-VanWertz, G., Goldstein, A. H., Harley, R. A., Frost, G. J., Roberts, J. M., Ryerson, T. B., and Trainer, M.: Volatile chemical products emerging as largest petrochemical source of urban organic emissions, Science, 359, 760–764, https://doi.org/10.1126/science.aaq0524, 2018.
Millet, D. B., Jacob, D. J., Boersma, K. F., Fu, T. M., Kurosu, T. P., Chance, K., Heald, C. L., and Guenther, A.: Spatial distribution of isoprene emissions from North America derived from formaldehyde column measurements by the OMI satellite sensor, J. Geophys. Res.-Atmos., 113, D02307, https://doi.org/10.1029/2007jd008950, 2008.
Murphy, D. M., Cziczo, D. J., Froyd, K. D., Hudson, P. K., Matthew, B. M., Middlebrook, A. M., Peltier, R. E., Sullivan, A., Thomson, D. S., and Weber, R. J.: Single-particle mass spectrometry of tropospheric aerosol particles, J. Geophys. Res.-Atmos., 111, D23s32, https://doi.org/10.1029/2006jd007340, 2006.
Na, K., Kim, Y. P., and Moon, K. C.: Seasonal variation of the C-2-C-9 hydrocarbons concentrations and compositions emitted from motor vehicles in a Seoul tunnel, Atmos. Environ., 36, 1969–1978, https://doi.org/10.1016/S1352-2310(02)00149-8, 2002.
Na, K., Moon, K. C., and Kim, Y. P.: Source contribution to aromatic VOC concentration and ozone formation potential in the atmosphere of Seoul, Atmos. Environ., 39, 5517–5524, https://doi.org/10.1016/j.atmosenv.2005.06.005, 2005.
Nault, B. A., Campuzano-Jost, P., Day, D. A., Schroder, J. C., Anderson, B., Beyersdorf, A. J., Blake, D. R., Brune, W. H., Choi, Y., Corr, C. A., de Gouw, J. A., Dibb, J., DiGangi, J. P., Diskin, G. S., Fried, A., Huey, L. G., Kim, M. J., Knote, C. J., Lamb, K. D., Lee, T., Park, T., Pusede, S. E., Scheuer, E., Thornhill, K. L., Woo, J.-H., and Jimenez, J. L.: Secondary organic aerosol production from local emissions dominates the organic aerosol budget over Seoul, South Korea, during KORUS-AQ, Atmos. Chem. Phys., 18, 17769–17800, https://doi.org/10.5194/acp-18-17769-2018, 2018.
Palm, B. B., de Sá, S. S., Day, D. A., Campuzano-Jost, P., Hu, W., Seco, R., Sjostedt, S. J., Park, J.-H., Guenther, A. B., Kim, S., Brito, J., Wurm, F., Artaxo, P., Thalman, R., Wang, J., Yee, L. D., Wernis, R., Isaacman-VanWertz, G., Goldstein, A. H., Liu, Y., Springston, S. R., Souza, R., Newburn, M. K., Alexander, M. L., Martin, S. T., and Jimenez, J. L.: Secondary organic aerosol formation from ambient air in an oxidation flow reactor in central Amazonia, Atmos. Chem. Phys., 18, 467–493, https://doi.org/10.5194/acp-18-467-2018, 2018.
Penning de Vries, M. J. M., Beirle, S., Hörmann, C., Kaiser, J. W., Stammes, P., Tilstra, L. G., Tuinder, O. N. E., and Wagner, T.: A global aerosol classification algorithm incorporating multiple satellite data sets of aerosol and trace gas abundances, Atmos. Chem. Phys., 15, 10597–10618, https://doi.org/10.5194/acp-15-10597-2015, 2015.
Pope, C. A., Burnett, R. T., Thun, M. J., Calle, E. E., Krewski, D., Ito, K., and Thurston, G. D.: Lung cancer, cardiopulmonary mortality, and long-term exposure to fine particulate air pollution, JAMA-J. Am. Med. Assoc., 287, 1132–1141, https://doi.org/10.1001/jama.287.9.1132, 2002.
Pye, H. O. T., Chan, A. W. H., Barkley, M. P., and Seinfeld, J. H.: Global modeling of organic aerosol: the importance of reactive nitrogen (NOx and NO3), Atmos. Chem. Phys., 10, 11261–11276, https://doi.org/10.5194/acp-10-11261-2010, 2010.
Pye, H. O. T., Murphy, B. N., Xu, L., Ng, N. L., Carlton, A. G., Guo, H., Weber, R., Vasilakos, P., Appel, K. W., Budisulistiorini, S. H., Surratt, J. D., Nenes, A., Hu, W., Jimenez, J. L., Isaacman-VanWertz, G., Misztal, P. K., and Goldstein, A. H.: On the implications of aerosol liquid water and phase separation for organic aerosol mass, Atmos. Chem. Phys., 17, 343–369, https://doi.org/10.5194/acp-17-343-2017, 2017.
Richter, D., Weibring, P., Walega, J. G., Fried, A., Spuler, S. M., and Taubman, M. S.: Compact highly sensitive multi-species airborne mid-IR spectrometer, Appl. Phys. B-Lasers O., 119, 119–131, https://doi.org/10.1007/s00340-015-6038-8, 2015.
Ridley, D. A., Heald, C. L., Ridley, K. J., and Kroll, J. H.: Causes and consequences of decreasing atmospheric organic aerosol in the United States, P. Natl. Acad. Sci. USA, 115, 290–295, https://doi.org/10.1073/pnas.1700387115, 2018.
Robinson, A. L., Donahue, N. M., Shrivastava, M. K., Weitkamp, E. A., Sage, A. M., Grieshop, A. P., Lane, T. E., Pierce, J. R., and Pandis, S. N.: Rethinking organic aerosols: Semivolatile emissions and photochemical aging, Science, 315, 1259–1262, https://doi.org/10.1126/science.1133061, 2007.
Ryerson, T. B., Trainer, M., Holloway, J. S., Parrish, D. D., Huey, L. G., Sueper, D. T., Frost, G. J., Donnelly, S. G., Schauffler, S., Atlas, E. L., Kuster, W. C., Goldan, P. D., Hubler, G., Meagher, J. F., and Fehsenfeld, F. C.: Observations of ozone formation in power plant plumes and implications for ozone control strategies, Science, 292, 719–723, https://doi.org/10.1126/science.1058113, 2001.
Ryerson, T. B., Andrews, A. E., Angevine, W. M., Bates, T. S., Brock, C. A., Cairns, B., Cohen, R. C., Cooper, O. R., de Gouw, J. A., Fehsenfeld, F. C., Ferrare, R. A., Fischer, M. L., Flagan, R. C., Goldstein, A. H., Hair, J. W., Hardesty, R. M., Hostetler, C. A., Jimenez, J. L., Langford, A. O., McCauley, E., McKeen, S. A., Molina, L. T., Nenes, A., Oltmans, S. J., Parrish, D. D., Pederson, J. R., Pierce, R. B., Prather, K., Quinn, P. K., Seinfeld, J. H., Senff, C. J., Sorooshian, A., Stutz, J., Surratt, J. D., Trainer, M., Volkamer, R., Williams, E. J., and Wofsy, S. C.: The 2010 California Research at the Nexus of Air Quality and Climate Change (CalNex) field study, J. Geophys. Res.-Atmos., 118, 5830–5866, https://doi.org/10.1002/jgrd.50331, 2013.
Schroder, J. C., Campuzano-Jost, P., Day, D. A., Shah, V., Larson, K., Sommers, J. M., Sullivan, A. P., Campos, T., Reeves, J. M., Hills, A., Hornbrook, R. S., Blake, N. J., Scheuer, E., Guo, H., Fibiger, D. L., McDuffie, E. E., Hayes, P. L., Weber, R. J., Dibb, J. E., Apel, E. C., Jaeglé, L., Brown, S. S., Thornton, J. A., and Jimenez, J. L.: Sources and Secondary Production of Organic Aerosols in the Northeastern US during WINTER, J. Geophys. Res.-Atmos., 123, 7771–7796, https://doi.org/10.1029/2018JD028475, 2018.
SEAC4RS Science Team: SEAC4RS NASA DC8 aircraft data archive, https://doi.org/10.5067/Aircraft/SEAC4RS/Aerosol-TraceGas-Cloud, 2013.
Seco, R., Karl, T., Guenther, A., Hosman, K. P., Pallardy, S. G., Gu, L. H., Geron, C., Harley, P., and Kim, S.: Ecosystem-scale volatile organic compound fluxes during an extreme drought in a broadleaf temperate forest of the Missouri Ozarks (central USA), Glob. Change Biol., 21, 3657–3674, https://doi.org/10.1111/gcb.12980, 2015.
Shrivastava, M., Cappa, C. D., Fan, J. W., Goldstein, A. H., Guenther, A. B., Jimenez, J. L., Kuang, C., Laskin, A., Martin, S. T., Ng, N. L., Petaja, T., Pierce, J. R., Rasch, P. J., Roldin, P., Seinfeld, J. H., Shilling, J., Smith, J. N., Thornton, J. A., Volkamer, R., Wang, J., Worsnop, D. R., Zaveri, R. A., Zelenyuk, A., and Zhang, Q.: Recent advances in understanding secondary organic aerosol: Implications for global climate forcing, Rev. Geophys., 55, 509–559, https://doi.org/10.1002/2016rg000540, 2017.
Solomon, P. A., Crumpler, D., Flanagan, J. B., Jayanty, R. K. M., Rickman, E. E., and McDade, C. E.: US National PM2.5 Chemical Speciation Monitoring Networks-CSN and IMPROVE: Description of networks, J. Air Waste Manage., 64, 1410–1438, https://doi.org/10.1080/10962247.2014.956904, 2014.
Taylor, K. E. and Penner, J. E.: Response of the Climate System to Atmospheric Aerosols and Greenhouse Gases, Nature, 369, 734–737, https://doi.org/10.1038/369734a0, 1994.
Tong, D. Q., Lamsal, L., Pan, L., Ding, C., Kim, H., Lee, P., Chai, T. F., Pickering, K. E., and Stajner, I.: Long-term NOx trends over large cities in the United States during the great recession: Comparison of satellite retrievals, ground observations, and emission inventories, Atmos. Environ., 107, 70–84, https://doi.org/10.1016/j.atmosenv.2015.01.035, 2015.
Toon, O. B., Maring, H., Dibb, J., Ferrare, R., Jacob, D. J., Jensen, E. J., Luo, Z. J., Mace, G. G., Pan, L. L., Pfister, L., Rosenlof, K. H., Redemann, J., Reid, J. S., Singh, H. B., Thompson, A. M., Yokelson, R., Minnis, P., Chen, G., Jucks, K. W., and Pszenny, A.: Planning, implementation, and scientific goals of the Studies of Emissions and Atmospheric Composition, Clouds and Climate Coupling by Regional Surveys (SEAC(4)RS) field mission, J. Geophys. Res.-Atmos., 121, 4967–5009, https://doi.org/10.1002/2015jd024297, 2016.
Torres, O., Tanskanen, A., Veihelmann, B., Ahn, C., Braak, R., Bhartia, P. K., Veefkind, P., and Levelt, P.: Aerosols and surface UV products from Ozone Monitoring Instrument observations: An overview, J. Geophys. Res.-Atmos., 112, D24s47, https://doi.org/10.1029/2007jd008809, 2007.
Travis, K. R., Jacob, D. J., Fisher, J. A., Kim, P. S., Marais, E. A., Zhu, L., Yu, K., Miller, C. C., Yantosca, R. M., Sulprizio, M. P., Thompson, A. M., Wennberg, P. O., Crounse, J. D., St. Clair, J. M., Cohen, R. C., Laughner, J. L., Dibb, J. E., Hall, S. R., Ullmann, K., Wolfe, G. M., Pollack, I. B., Peischl, J., Neuman, J. A., and Zhou, X.: Why do models overestimate surface ozone in the Southeast United States?, Atmos. Chem. Phys., 16, 13561–13577, https://doi.org/10.5194/acp-16-13561-2016, 2016.
Tsigaridis, K., Daskalakis, N., Kanakidou, M., Adams, P. J., Artaxo, P., Bahadur, R., Balkanski, Y., Bauer, S. E., Bellouin, N., Benedetti, A., Bergman, T., Berntsen, T. K., Beukes, J. P., Bian, H., Carslaw, K. S., Chin, M., Curci, G., Diehl, T., Easter, R. C., Ghan, S. J., Gong, S. L., Hodzic, A., Hoyle, C. R., Iversen, T., Jathar, S., Jimenez, J. L., Kaiser, J. W., Kirkevåg, A., Koch, D., Kokkola, H., Lee, Y. H., Lin, G., Liu, X., Luo, G., Ma, X., Mann, G. W., Mihalopoulos, N., Morcrette, J.-J., Müller, J.-F., Myhre, G., Myriokefalitakis, S., Ng, N. L., O'Donnell, D., Penner, J. E., Pozzoli, L., Pringle, K. J., Russell, L. M., Schulz, M., Sciare, J., Seland, Ø., Shindell, D. T., Sillman, S., Skeie, R. B., Spracklen, D., Stavrakou, T., Steenrod, S. D., Takemura, T., Tiitta, P., Tilmes, S., Tost, H., van Noije, T., van Zyl, P. G., von Salzen, K., Yu, F., Wang, Z., Wang, Z., Zaveri, R. A., Zhang, H., Zhang, K., Zhang, Q., and Zhang, X.: The AeroCom evaluation and intercomparison of organic aerosol in global models, Atmos. Chem. Phys., 14, 10845–10895, https://doi.org/10.5194/acp-14-10845-2014, 2014.
Veefkind, J. P., Boersma, K. F., Wang, J., Kurosu, T. P., Krotkov, N., Chance, K., and Levelt, P. F.: Global satellite analysis of the relation between aerosols and short-lived trace gases, Atmos. Chem. Phys., 11, 1255–1267, https://doi.org/10.5194/acp-11-1255-2011, 2011.
Wagner, N. L., Brock, C. A., Angevine, W. M., Beyersdorf, A., Campuzano-Jost, P., Day, D., de Gouw, J. A., Diskin, G. S., Gordon, T. D., Graus, M. G., Holloway, J. S., Huey, G., Jimenez, J. L., Lack, D. A., Liao, J., Liu, X., Markovic, M. Z., Middlebrook, A. M., Mikoviny, T., Peischl, J., Perring, A. E., Richardson, M. S., Ryerson, T. B., Schwarz, J. P., Warneke, C., Welti, A., Wisthaler, A., Ziemba, L. D., and Murphy, D. M.: In situ vertical profiles of aerosol extinction, mass, and composition over the southeast United States during SENEX and SEAC4RS: observations of a modest aerosol enhancement aloft, Atmos. Chem. Phys., 15, 7085–7102, https://doi.org/10.5194/acp-15-7085-2015, 2015.
Walgraeve, C., Demeestere, K., Dewulf, J., Zimmermann, R., and Van Langenhove, H.: Oxygenated polycyclic aromatic hydrocarbons in atmospheric particulate matter: Molecular characterization and occurrence, Atmos. Environ., 44, 1831–1846, https://doi.org/10.1016/j.atmosenv.2009.12.004, 2010.
Warneke, C., de Gouw, J. A., Stohl, A., Cooper, O. R., Goldan, P. D., Kuster, W. C., Holloway, J. S., Williams, E. J., Lerner, B. M., McKeen, S. A., Trainer, M., Fehsenfeld, F. C., Atlas, E. L., Donnelly, S. G., Stroud, V., Lueb, A., and Kato, S.: Biomass burning and anthropogenic sources of CO over New England in the summer 2004, J. Geophys. Res.-Atmos., 111, D23s15, https://doi.org/10.1029/2005jd006878, 2006.
Warneke, C., Veres, P., Holloway, J. S., Stutz, J., Tsai, C., Alvarez, S., Rappenglueck, B., Fehsenfeld, F. C., Graus, M., Gilman, J. B., and de Gouw, J. A.: Airborne formaldehyde measurements using PTR-MS: calibration, humidity dependence, inter-comparison and initial results, Atmos. Meas. Tech., 4, 2345–2358, https://doi.org/10.5194/amt-4-2345-2011, 2011.
Weibring, P., Richter, D., Fried, A., Walega, J. G., and Dyroff, C.: Ultra-high-precision mid-IR spectrometer II: system description and spectroscopic performance, Appl. Phys. B-Lasers O., 85, 207–218, https://doi.org/10.1007/s00340-006-2300-4, 2006.
Wisthaler A., Hansel A., Dickerson R. R., and Crutzen P. J.: Organic trace gas measurements by PTR-MS during INDOEX 1999, J. Geophys. Res.-Atmos., 107, 8024, https://doi.org/10.1029/2001JD000576, 2002.
Wolfe, G. M., Kaiser, J., Hanisco, T. F., Keutsch, F. N., de Gouw, J. A., Gilman, J. B., Graus, M., Hatch, C. D., Holloway, J., Horowitz, L. W., Lee, B. H., Lerner, B. M., Lopez-Hilifiker, F., Mao, J., Marvin, M. R., Peischl, J., Pollack, I. B., Roberts, J. M., Ryerson, T. B., Thornton, J. A., Veres, P. R., and Warneke, C.: Formaldehyde production from isoprene oxidation across NOx regimes, Atmos. Chem. Phys., 16, 2597–2610, https://doi.org/10.5194/acp-16-2597-2016, 2016.
Worton, D. R., Surratt, J. D., Lafranchi, B. W., Chan, A. W. H., Zhao, Y., Weber, R., Park, J., Gilman, J. B., de Gouw, J., Park, C., Schade, G., Beaver, M., StClair, J., Crounse, J., Wennberg, P., Wolfe, G., Harrold, S., Thornton, J., Farmer, D. K., Docherty, K. S., Cubison, M. J., Jimenez, J. L., Frossard, A., Russell, L., Kristensen, K., Glasius, M., Mao, J., Ren, X., Brune, W. H., Browne, E., Pusede, S., Cohen, R., Seinfeld, J. H., and Goldstein, A. H.: Observational Insights into Aerosol Formation from Isoprene, Enviro. Sci. Technol., 47, 11403–11413, https://doi.org/10.1021/es4011064, 2013.
Xu, L., Suresh, S., Guo, H., Weber, R. J., and Ng, N. L.: Aerosol characterization over the southeastern United States using high-resolution aerosol mass spectrometry: spatial and seasonal variation of aerosol composition and sources with a focus on organic nitrates, Atmos. Chem. Phys., 15, 7307–7336, https://doi.org/10.5194/acp-15-7307-2015, 2015.
Xu, L., Middlebrook, A. M., Liao, J., de Gouw, J. A., Guo, H. Y., Weber, R. J., Nenes, A., Lopez-Hilfiker, F. D., Lee, B. H., Thornton, J. A., Brock, C. A., Neuman, J. A., Nowak, J. B., Pollack, I. B., Welti, A., Graus, M., Warneke, C., and Ng, N. L.: Enhanced formation of isoprene-derived organic aerosol in sulfur-rich power plant plumes during Southeast Nexus, J. Geophys. Res.-Atmos., 121, 11137–11153, https://doi.org/10.1002/2016jd025156, 2016.
Yu, K., Jacob, D. J., Fisher, J. A., Kim, P. S., Marais, E. A., Miller, C. C., Travis, K. R., Zhu, L., Yantosca, R. M., Sulprizio, M. P., Cohen, R. C., Dibb, J. E., Fried, A., Mikoviny, T., Ryerson, T. B., Wennberg, P. O., and Wisthaler, A.: Sensitivity to grid resolution in the ability of a chemical transport model to simulate observed oxidant chemistry under high-isoprene conditions, Atmos. Chem. Phys., 16, 4369–4378, https://doi.org/10.5194/acp-16-4369-2016, 2016.
Zhu, L., Jacob, D. J., Kim, P. S., Fisher, J. A., Yu, K., Travis, K. R., Mickley, L. J., Yantosca, R. M., Sulprizio, M. P., De Smedt, I., González Abad, G., Chance, K., Li, C., Ferrare, R., Fried, A., Hair, J. W., Hanisco, T. F., Richter, D., Jo Scarino, A., Walega, J., Weibring, P., and Wolfe, G. M.: Observing atmospheric formaldehyde (HCHO) from space: validation and intercomparison of six retrievals from four satellites (OMI, GOME2A, GOME2B, OMPS) with SEAC4RS aircraft observations over the southeast US, Atmos. Chem. Phys., 16, 13477–13490, https://doi.org/10.5194/acp-16-13477-2016, 2016
Zhu, L., Jacob, D. J., Keutsch, F. N., Mickley, L. J., Scheffe, R., Strum, M., Abad, G. G., Chance, K., Yang, K., Rappengluck, B., Millet, D. B., Baasandorj, M., Jaegle, L., and Shah, V.: Formaldehyde (HCHO) As a Hazardous Air Pollutant: Mapping Surface Air Concentrations from Satellite and Inferring Cancer Risks in the United States, Environ. Sci. Technol., 51, 5650–5657, https://doi.org/10.1021/acs.est.7b01356, 2017.
Ziemann, P. J. and Atkinson, R. Kinetics, products, and mechanisms of secondary organic aerosol formation, Chem. Soc. Rev., 41, 6582–6605, https://doi.org/10.1039/C2CS35122F, 2012.
- Abstract
- Introduction
- Methods
- In situ OA–HCHO relationship
- Comparison of OA–HCHO relationships: in situ vs. GEOS-Chem
- Relating satellite HCHO column to surface HCHO concentrations
- Construction of the OA estimate
- Limitations of the OA estimate and future work
- Summary
- Data availability
- Author contributions
- Competing interests
- Acknowledgements
- References
- Supplement
- Abstract
- Introduction
- Methods
- In situ OA–HCHO relationship
- Comparison of OA–HCHO relationships: in situ vs. GEOS-Chem
- Relating satellite HCHO column to surface HCHO concentrations
- Construction of the OA estimate
- Limitations of the OA estimate and future work
- Summary
- Data availability
- Author contributions
- Competing interests
- Acknowledgements
- References
- Supplement