the Creative Commons Attribution 4.0 License.
the Creative Commons Attribution 4.0 License.
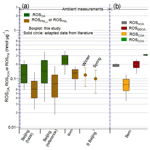
Predominance of secondary organic aerosol to particle-bound reactive oxygen species activity in fine ambient aerosol
Miriam Elser
Ru-Jin Huang
Manuel Krapf
Roman Fröhlich
Deepika Bhattu
Giulia Stefenelli
Peter Zotter
Emily A. Bruns
Simone M. Pieber
Haiyan Ni
Qiyuan Wang
Yichen Wang
Yaqing Zhou
Chunying Chen
Mao Xiao
Jay G. Slowik
Samuel Brown
Laure-Estelle Cassagnes
Kaspar R. Daellenbach
Thomas Nussbaumer
Marianne Geiser
André S. H. Prévôt
Imad El-Haddad
Junji Cao
Urs Baltensperger
Reactive oxygen species (ROS) are believed to contribute to the adverse health effects of aerosols. This may happen by inhaled particle-bound (exogenic) ROS (PB-ROS) or by ROS formed within the respiratory tract by certain aerosol components (endogenic ROS). We investigated the chemical composition of aerosols and their exogenic ROS content at the two contrasting locations Beijing (China) and Bern (Switzerland). We apportioned the ambient organic aerosol to different sources and attributed the observed water-soluble PB-ROS to them. The oxygenated organic aerosol (OOA, a proxy for secondary organic aerosol, SOA) explained the highest fraction of the exogenic ROS concentration variance at both locations. We also characterized primary and secondary aerosol emissions generated from different biogenic and anthropogenic sources in smog chamber experiments. The exogenic PB-ROS content in the OOA from these emission sources was comparable to that in the ambient measurements. Our results imply that SOA from gaseous precursors of different anthropogenic emission sources is a crucial source of water-soluble PB-ROS and should be additionally considered in toxicological and epidemiological studies in an adequate way besides primary emissions. The importance of PB-ROS may be connected to the seasonal trends in health effects of PM reported by epidemiological studies, with elevated incidences of adverse effects in warmer seasons, which are accompanied by more-intense atmospheric oxidation processes.
- Article
(2073 KB) - Full-text XML
-
Supplement
(2187 KB) - BibTeX
- EndNote
Air pollution has been shown to adversely impact human health through cardiovascular and respiratory disorders, which may ultimately lead to a reduction in life expectancy (WHO, 2013a; Pope et al., 2009; Salvi, 2007; Guarnieri and Balmes, 2014; Du et al., 2016; Cohen, 2000; Johnson, 2004). Epidemiological estimates suggest more than 4 million premature deaths per year due to air pollution, with a significant fraction attributed to China and India (Cohen et al., 2017; Lelieveld, 2017). Such analysis is based on relations between total particulate air pollution and health effects to provide exposure-response functions. A significant correlation between fine particle mass concentration and excess mortality was reported in the 1990s in the “Harvard Six-City” study (Dockery et al., 1993) and consistently confirmed later on (e.g., Laden et al., 2006; Lepeule et al., 2012; Beelen et al., 2014).
Since the composition of the atmospheric particulate matter (PM) depends on the sources and their chemical transformation in the atmosphere, an important question is which PM constituents are responsible for the adverse human health effects. Sulfate, organic carbon (OC), and transition metals have been shown to be more prominently associated with adverse health outcomes than other pollutants (Lippmann et al., 2013; Adams et al., 2015; Vedal et al., 2013; Burnett et al., 2000), even though there is no toxicological evidence supporting a causal role (WHO, 2013b). It is generally hypothesized that the adverse health effects caused by PM largely derive from oxidative stress induced by reactive oxygen species (ROS). The mechanism by which oxidative stress can be initiated is either through direct delivery of oxidants contained in PM (exogenous ROS) or by formation of endogenous ROS through introduction of redox-active compounds able to generate ROS in lung cells or by mitochondria as a response to particle exposure. Different acellular assays have been explored to characterize the oxidant activity of PM. For instance, the 2′,7′-dichlorofluorescin (DCFH) and the p-hydroxyphenylacetic acid (POHPAA) assays respond to a range of organic peroxides (Venkatachari and Hopke, 2008; Wang et al., 2011a; King and Weber, 2013; Fuller et al., 2014; Zhou et al., 2018a; Hasson and Paulson, 2003) and are used to measure particle-bound ROS (PB-ROS). Similarly, the assay with 9,10-bis (phenylethynyl) anthracene nitroxide (BPEA-nit) measures the amount of PB-ROS, including radicals and metals such as Cu+ and Fe2+, but not the ROS generated from Fenton chemistry (Miljevic et al., 2010; Hedayat et al., 2016). On the other hand, the dithiothreitol (DTT) assay is strongly sensitive to redox-active components, i.e., transition metals and quinones, and is considered a good estimator of ROS generation in lung cells (Cho et al., 2005; Verma et al., 2012; Charrier and Anastasio, 2012; Fang et al., 2016; Weber et al., 2018), but it is also sensitive to organic peroxides (Wang et al., 2018). The electron spin resonance (ESR) assay measures the capability of PM to induce hydroxyl radicals (Shi et al., 2003). Other assays measure the ability of PM to deplete biological antioxidants such as ascorbic acid (AA), glutathione, and uric acid (Mudway et al., 2004; Fang et al., 2016). Since the different assays capture different fractions of the oxidant activity of PM, a direct comparison of measurements is challenging (Shiraiwa et al., 2017; Fang et al., 2016; Weber et al., 2018; Calas et al., 2018; Perrone et al., 2016; Yang et al., 2014; Janssen et al., 2015). Nonetheless, the ROS generation potential (referred to as oxidative potential, OP) determined using these assays was found to be more strongly associated with emergency department visits for airway and nasal inflammation, asthma, wheezing, and congestive heart failure than PM2.5 (PM with a particle diameter smaller than 2.5 µm) (Bates et al., 2015; Fang et al., 2016; Janssen et al., 2015).
More detailed analyses revealed associations between health effects and specific sources of particulate matter (Lippmann et al., 2013), such as wildfires, traffic, shipping, construction dust, metal sources, and coal and residual oil combustion (Liu et al., 2017; Ostro et al., 2011; Lippmann et al., 2013; Adam et al., 2015). To date, the emission sources governing ROS concentrations especially during haze episodes in developing and emerging countries are poorly constrained. Recent studies attributed the OP of ambient PM to different emission sources by applying source apportionment techniques like positive matrix factorization (PMF). Biomass burning and traffic contributed to the OP measured by DTT in most cases, while contributions by secondary PM components like ammonium sulfate or the more oxidized fraction of organic aerosol (OA) were only found in some studies (Verma et al., 2015; Fang et al., 2016; Bates et al., 2015; Weber et al., 2018). The OP measured by AA was found to correlate with Cu, secondary processes, and traffic (Weber et al., 2018; Fang et al., 2016) but not with biomass burning. The overwhelming majority of these studies are based on offline measurements with the inherent limitations related to filter sampling, including positive and negative artifacts and the loss of short-lived ROS during filter storage (Zhou et al., 2018a).
Here we systematically investigate the links between the chemical nature and sources of atmospheric aerosols and their PB-ROS content, by coupling aerosol mass spectrometry with online PB-ROS measurements, and provide new insights into the sources of reactive oxidants in PM. We combine field data from two distinct environments, i.e., Beijing (China) and Bern (Switzerland), with results from comprehensive laboratory experiments, where we quantified the PB-ROS content in primary and secondary aerosols emitted from traffic, biomass burning, coal burning, and biogenic sources, the most important sources of ambient organic aerosol. We show that PB-ROS, a large fraction of which is not accessible by offline measurements, are generated to a large extent in the atmosphere through oxidation processes.
2.1 Measurement campaigns
We combined the results from two field campaigns and three laboratory studies. One field campaign was conducted in November 2014 at the Institute of Anatomy of the University of Bern (46∘57′02.0′′ N, 7∘26′17.4′′ E), Switzerland, which is located in the city center but not directly affected by heavy traffic. The other field campaign took place in Beijing, China, from January to February 2015 at the National Center for Nanoscience and Technology (39∘59′22.0′′ N, 116∘19′29.7′′ E; 20 m above ground), which is surrounded by university buildings and residential areas. For the laboratory studies, two atmospheric chemistry simulation chambers of the Paul Scherrer Institute (PSI) were used, i.e., the 27 m3 stationary smog chamber (PSI-SSC) and the 7 m3 mobile smog chamber (PSI-MSC). The PSI-SSC is a flexible bag made of fluorinated ethylene propylene (FEP) suspended in a temperature-controlled enclosure, where four xenon arc lamps (4 kW rated power, 1.55×105 lm each (lumens), XBO 4000 W/HS, OSRAM) are used to simulate the solar light spectrum and to mimic natural photochemistry (Paulsen et al., 2005). Before each experiment, humidified pure air with a high ozone concentration (>2 ppmv) was flushed into the chamber for at least 1 h to clean the bag walls with lights on, followed by flushing with dry, pure air for at least 10 h. The PSI-MSC is located inside a temperature-controlled housing flanked by four sets of 10 UV lights (90–100 W, Cleo Performance, Philips) (Platt et al., 2013). In both chambers, OH was formed to a large degree from the photolysis of HONO and its concentration was evaluated by the decay of the marker compound d9-butanol (butanol-D9, 98 %, Sigma-Aldrich) measured by a proton-transfer-reaction mass spectrometer (PTR-MS, Ionicon Analytik GmbH) (Barmet et al., 2012). The gas- and particle-phase primary products that were injected into the chambers were first thoroughly studied before the lights were switched on. Photochemical gas-phase reactions and formation of secondary organic aerosol (SOA) were then initiated by turning on the lights in the smog chambers. The following precursor emission sources were tested in this study:
Wood combustion emissions. In total, we investigated eight combustion devices with different technologies and combustion conditions, including a two-stage combustion updraft pellet boiler, a moving-grate boiler equipped with an electrostatic precipitator, a pellet stove, a two-stage combustion downdraft logwood boiler, two advanced two-stage combustion logwood stoves, and two conventional single-stage combustion logwood stoves. Primary emission measurements from all these devices are reported here. For the secondary wood burning emissions, only the experiments conducted with one of the conventional single-stage combustion logwood stoves and aged in the PSI-MSC are presented here, as it is more representative for the genuine atmospheric aging (with an OH exposure of (2.6–4.8) ×107 molec cm−3 h), compared to the emissions from the other burning devices that were aged in the potential aerosol mass (PAM) chamber (with an OH exposure of (1.1–2.0) ×108 molec cm−3 h). More details of the experimental method as well as the evolution of PB-ROS formation during the aging can be found in our previous publication (Zhou et al., 2018b).
Coal combustion emissions. We combusted three types of Chinese bituminous coal and two types of Chinese anthracite coal in a conventional Chinese household cookstove. For each experiment, 100–300 g of coal was preheated first by a hot honeycomb and anthracite coal until the temperature rose up to ∼600 ∘C, and then emissions were sampled, diluted 100-fold, and injected into the PSI-MSC. One exemplary experiment showing the evolution of ROS and OA formation during the aging of the bituminous coal combustion emissions is presented in Fig. S1 in the Supplement. Aging resulted in a strong increase of the total OA accompanied by formation of ROS reaching 0.6 nmol µg−1 after 2 h.
α-Pinene. α-Pinene (40 ppbv) was injected into the PSI-SSC containing O3 (∼500 ppbv) resulting in rapid formation of SOA. More details about the experimental procedure can be found elsewhere (Krapf et al., 2016). As seen in Fig. S2, the injection of α-pinene resulted in a fast production of OA and ROS, where ROS decreased strongly with time when α-pinene had reacted away, similar to the observed decay of peroxides by Krapf et al. (2016).
2.2 Instrumentation
A suite of online instrumentation was deployed to characterize the chemical and physical properties of gas- and particle-phase emissions in all the campaigns mentioned above.
Reactive oxygen species (ROS) analyzer. An online ROS analyzer based on the 2′,7′-dichlorofluorescin (DCFH) assay was used for the quantification of the water-soluble particle-bound components acting as ROS (PB-ROS). A detailed description of the ROS analyzer is given in our previous publication (Zhou et al., 2018a). Particles were collected at a flow rate of ∼1.7 L min−1, using an aerosol collector, of which the main part is a mist chamber. The oxygen-free ultra-pure water (OF-UPW) was continuously sprayed into the mist chamber and incorporated the collected aerosol particles. The sample extracts were then mixed with the working solution (the DCFH solution) for analysis. The PB-ROS concentration was measured continuously with a response time of 8 min (rise time of signal from 10 % to 90 % of full signal) and was calculated as H2O2 equivalents. An instrument limit of detection of 2 nmol m−3 of sampled air was determined. All tested organic peroxides showed a linear response to DCFH but with different sensitivities, depending on their stability and on neighboring functional groups. This was also observed with the DTT assay for SOA (Wang et al., 2018). Transition metals (i.e., Fe) and quinones (i.e., anthraquinone), which induce redox cycling and are well measured by the DTT assay, do not react or interfere with DCFH when present at typical ambient concentration levels. Thus, we conclude that DCFH measures the capability of particle-borne compounds to act as reactive oxygen species rather than the potential of species to mediate ROS formation (Zhou et al., 2018a). Before the aerosol collector, a honeycomb charcoal denuder was installed in a stainless-steel tube to remove interfering gas-phase compounds. Tests revealed neither interference from NO2 nor ozone for integrated doses up to 2300 ppb h. Denuders were regenerated every day.
Aerosol mass spectrometer (AMS). A field-deployable high-resolution time-of-flight aerosol mass spectrometer (HR-ToF-AMS, Aerodyne Research Inc.) (DeCarlo et al., 2006) was used for the characterization of the nonrefractory aerosol mass in Beijing. Ambient air was sampled through a critical orifice into a recently developed aerodynamic lens, which efficiently transmitted particles between 80 nm and up to at least 3 µm (Williams et al., 2013). Particles were flash-vaporized by impaction on a resistively heated surface (∼600 ∘C) and ionized by electron ionization (70 eV). The mass-to-charge ratios (m∕z) of the resulting fragments were determined by a ToF mass spectrometer. Data were analyzed with the ToF-AMS software SQUIRREL and PIKA. Data were not corrected for lens transmission efficiency. For ambient data, standard relative ionization efficiencies (RIE) were used for organics (RIE = 1.4), nitrate (RIE = 1.1), and chloride (RIE = 1.3). RIE for sulfate and ammonium were experimentally determined to be 1.12 and 3.58, respectively. The composition-dependent collection efficiency (CDCE) was evaluated using the algorithm by Middlebrook et al. (2012). For the laboratory experiments, RIEs of 1.4, 1.3, 1.1, and 1.2 were used for organics, chloride, nitrate, and sulfate, respectively, and experimentally determined as 3.83 for ammonium. In line with past laboratory biomass burning experiments, a CDCE of 1 was used in all wood burning tests.
Aerosol chemical speciation monitor (ACSM). A quadrupole aerosol chemical speciation monitor (Q-ACSM, Aerodyne Research Inc.) was employed to monitor the composition and mass concentration of nonrefractory submicron PM in Bern (Ng et al., 2011b). The Q-ACSM is built upon the same sampling and detection technology as the AMS described above but with reduced complexity (e.g., no particle size measurement), resolution, and performance (Fröhlich et al., 2013). Data were not corrected for lens transmission efficiency. Standard RIEs were used for organics (1.4), nitrate (1.1), and chloride (1.3). RIEs for sulfate and ammonium were experimentally determined to be 0.58 and 4.6, respectively. The CDCE was corrected based on the methodology described by Middlebrook et al. (2012).
Aethalometer. Real-time measurement of the optical absorption (babs) was performed by an Aethalometer at seven different wavelengths. A “next generation” Aethalometer was used (AE33, Magee Scientific, Berkeley, CA, USA), which performed an online correction for possible scattering artifacts of the measured babs (Drinovec et al., 2015). The babs was then converted to an equivalent black carbon (eBC) concentration using the nominal mass absorption cross section (MAC) value of 11.8 m2 g−1 (Zotter et al., 2017).
Gas-phase instrumentation. In the wood combustion PAM chamber experiments, total volatile organic compounds (VOCs) and CH4 were measured with a flame ionization detector (FID) with a nonmethane cutter (model 109A, J.U.M Engineering GmbH), CO and NO were measured with a nondispersive infrared analyzer (Ultramat 23 Siemens), O2 with a paramagnetic oxygen analyzer (Ultramat 23 Siemens), and CO2 with a nondispersive infrared (NDIR) analyzer (model LI-820, LI-COR®). In the smog chamber (SC) aging experiments, CO, CO2, and CH4 were measured with a cavity ring-down spectrometer (G2401, Picarro, Inc.). In all experiments, the composition of nonmethane VOCs was determined by a high-resolution proton-transfer-reaction mass spectrometer (HR-PTR-MS 8000, Ionicon Analytik GmbH).
VACES. A versatile aerosol concentration enrichment system (VACES) was used to enrich the Bern ambient aerosol to increase the ROS concentration above the instrument detection limit. More detailed information on the VACES can be found in Künzi et al. (2015). The aerosol sample was drawn into the VACES at a flow rate of ∼100 L min−1 into a tank with water heated up to ∼30 ∘C. The water-vapor-saturated air stream was then cooled down in a condenser (−2 ∘C) where water droplets with diameters >2 µm formed on the collected aerosol particles. The droplets were then enriched in concentration with a virtual impactor and dried by passing through a diffusion dryer. The concentration enrichment factor CE was calculated as
where Qtot and qmin are the intake and minor flows of the impactor, respectively; ηvi is the collection efficiency; and WL the fractional loss of the impactor (Sioutas et al., 1999). A potential influence of the enrichment process on the PB-ROS concentrations was investigated during the Bern ambient air measurements. We compared offline PB-ROS measurements using filters collected before and after the VACES with the online PB-ROS measurements, which were always done after the VACES. No differences in ROS concentrations were observed between filters taken before and after the VACES compared with the online measurements (Zhou et al., 2018a).
2.3 Statistical analysis
Positive matrix factorization (PMF) and multilinear engine (ME-2). Source apportionment was performed on the organic AMS and ACSM data using PMF as implemented by the multilinear engine (ME-2; Paatero, 1997) and controlled via the interface SoFi coded in Igor WaveMetrics (Source Finder; Canonaco et al., 2013). PMF was developed by Paatero and Tapper (1993, 1994) as a receptor model to apportion the sources on the basis of observations (internal correlations) at the receptor site alone (Viana et al., 2008), as represented by Eq. (2):
Here xij, gik, fkj, and eij are matrix elements of the measurements, factor time series, factor profiles, and residual matrices, respectively, where fik≥0 and gik≥0. The indices i, j, and k correspond to time, m∕z, and a discrete factor, respectively. p denotes the number of factors in the PMF solution determined by the user. The factor profiles remain constant, while their contributions to the matrix elements of the measurements are allowed to vary with time (concentration time series). In PMF, an “objective function” Q as defined in Eq. (3) is iteratively minimized:
where eij is the residual in the ith variable measured in the jth sample and sij represents the corresponding “uncertainty” of eij. The main theoretical limitations of PMF are that (1) source interpretation is relatively subjective and (2) there is an inability to clearly separate covariant sources (Viana et al., 2008) with similar chemical composition, e.g., cooking and traffic.
ME-2 provides a more flexible framework for controlling the solutions of the factor analysis where it permits the imposition of explicit external constraints (Ramadan et al., 2003). Within the a value approach, a priori information (factor profiles or factor time series shown in Fig. S3) of one or more sources can be used as an additional input into the model, using the scalar a to regulate the strength of the constraints:
Here , and fj and gi are the input profiles and the time series, respectively, which can either entirely or partially be constrained by a.
Aethalometer model. The Aethalometer-based source apportionment model developed by Sandradewi et al. (2008) was used to separate the contributions of traffic (TR) and wood burning (WB) to the measured eBC in Bern. The equation used for the model is
Using the power law of the spectral dependence of the absorption, the measured babs at two different wavelengths, and Eq. (6), TR and WB can be apportioned in the following way:
In Eqs. (7)–(10), α is the absorption Ångström exponent for traffic and wood combustion emissions (αTR and αWB, respectively), which have to be assumed a priori. Similar to Fröhlich et al. (2015) and Sciare et al. (2011), the terms αTR=1 and αWB=2 were used, and babs (λ1) and babs(λ2) were chosen to be 470 and 950 nm, respectively. The babs values attributed to the two sources were then converted to eBC using nominal mass absorption cross-section (MAC) values from the instrument manufacturer. In this study, the model was only applied to the Bern data, where the contributions of other combustion emissions to eBC were negligible (Zotter et al., 2017), but not in Beijing, as the contribution of coal combustion is substantial there (Liu et al., 2016; Elser et al., 2016) and large uncertainties regarding the optical properties of eBC emitted from coal combustion still exist.
Bootstrap tests. The bootstrap method, which is an effective approach to partially explore the influence of abnormal experimental conditions (i.e., the haze and reference episodes in Beijing and the presence of outliers), is employed in this study to (1) calculate a Pearson correlation matrix of all the testing factors in Beijing and Bern and (2) test the sensitivity of the multiple linear regression model (MLRM) to the input data as described in Sect. 3.3. This statistical method is based on the creation of replicate inputs perturbing the original data by resampling. This was done by randomly reorganizing the rows of the original time series so that some rows of the original data were present several times, while other rows were removed. Both of the analyses, (1) and (2), were obtained by running the data for 1000 bootstrap replicates.
2.4 Definitions
ROS fraction. In order to study the ROS formation during aging, the secondary ROS content (ROSSOA) was introduced. It describes the number of moles of secondary ROS (ROS) per mass of secondary organic aerosol (SOA) formed during aging and was calculated as
Secondary organic aerosol (SOA) and secondary ROS (ROSS) were calculated by subtracting primary organic aerosol (POA) and primary ROS (ROSP) from the total OA and aged ROS, respectively, assuming ROSP and POA to be lost to the chamber walls at the same rate as eBC but otherwise to remain constant during aging. Although both quantities may not be conserved, a decrease of both did abate their effect on the PB-ROS fraction. Wall loss rates for POA and SOA were assumed to be the same as that of the measured eBC.
f44−SOA. To express the degree of oxygenation of SOA, the fraction of secondary Org44 (corresponding to m∕z 44) in SOA (represented as f44−SOA) is introduced, which was calculated as
where Org44−SOA is the difference of total Org44 and primary Org44 and using the same procedure as for the SOA calculation mentioned above.
The measurements in the city of Bern were performed in November 2014 using a quadrupole aerosol chemical speciation monitor (Q-ACSM), an Aethalometer, and the online ROS analyzer. In Beijing a high-resolution time-of-flight aerosol mass spectrometer (HR-ToF-AMS), an Aethalometer, and the ROS analyzer were used. Visibility was determined by an automatic weather station (MAWS201, Vaisala, Vantaa, Finland) configured with a visibility sensor (Vaisala Model PWD22). Haze periods were defined by a visibility of less than 10 km, while time periods with a visibility above 10 km were termed reference days (China Meteorological Administration, 2010).
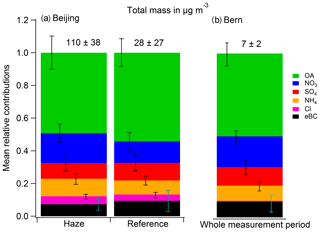
Figure 1Total PM mass concentrations and relative contributions of nonrefractory chemical components plus eBC in (a) Beijing (PM2.5, January–February 2015) and (b) Bern (PM1, November 2014).
3.1 Bulk chemical composition
Figure 1a shows the average contributions of organic aerosol (OA), sulfate (SO4), nitrate (NO3), ammonium (NH4), chloride (Cl), and equivalent black carbon (eBC) to PM2.5 in Beijing during January–February 2015 (time series shown in Fig. S4). During haze events, its sum, considered a proxy for PM2.5, exceeded occasionally values of 200 µg m−3 (average ≈110 µg m−3). On reference days the PM2.5 concentration ranged from 4 to 140 µg m−3 with an average value of 28 µg m−3. OA dominated in both haze and reference days, with a contribution of 49±10 % and 54±8 % to the total mass, respectively. As seen from the average diurnal patterns (Fig. S5), OA, eBC, and Cl increased during the nighttime of the haze days, while NO3 exhibited a maximum in the afternoon during both haze and reference days. During reference days, OA is high during nighttime and additionally showed a significant afternoon peak concurrent with NO3, possibly indicating additional secondary OA formation.
The average concentrations of PM1 in Bern were ∼15 and ∼4 times lower than in Beijing (PM2.5) during haze and reference periods, respectively (Fig. 1, PM1≈0.8 PM2.5 in Bern and Beijing). The relative contributions of the different chemical components in the aerosol from Bern were similar to those from Beijing, except for chloride, which contributed much less to the total mass in Bern than in Beijing. This may be due to hydrogen chloride (HCl) and methyl chloride (CH3Cl) emissions from combustion sources in Beijing, e.g., coal emissions, incinerators, and other industrial sources (Huang et al., 2014; Elser et al., 2016; McCulloch et al., 1999; Yudovich and Ketris, 2006).
3.2 PB-ROS and OA sources
PMF was applied to the organic aerosol mass spectra (shown in Fig. S3) acquired by the ACSM and the AMS to extract the contributions of the different sources (see Sect. 2.3). Advanced error analysis methodologies were deployed to assess the PMF model uncertainties (as shown in Elser et al., 2016). In Beijing, the result representing our data best was a 4-factor solution with 3 unconstrained factors, including oxygenated OA (OOA), a combination of hydrocarbon-like OA from traffic and coal burning emissions (denoted HOA+CCOA), cooking OA (COA), and a constrained biomass burning OA (BBOA) factor. Several tests were performed in the attempt to separate the HOA and CCOA factors, including the constraint of the factor profiles obtained in Elser et al. (2016). However, none of the tested approaches provided a satisfactory separation of these two sources, which were therefore recombined in the final solution. The BBOA factor profile obtained in previous ambient measurements in China by Elser et al. (2016) was constrained varying the a value between 0 and 0.3 in steps of 0.1. In Bern, the solution that represented our data best was a 5-factor solution, with the 4 unconstrained factors OOA, BBOA, HOA, and COA and a fifth constrained factor that could not be attributed to any specific source but is required to properly extract COA in Bern; this factor is referred to as an unidentified factor (Fig. S6a). In order to extract a clean profile of the unidentified factor (to be constrained in the final solution), we studied the changes in the mass spectra of the unidentified factor for an increasing number of factors (Fig. S6b). Based on these changes we constrained the unidentified mass spectra obtained in the 9-, 10-, and 11-factor solutions with a values between 0 and 1 in steps of 0.1.
The factors were identified using their mass spectral fingerprints, and their source assignments were confirmed based on the factor diurnal patterns and their correlation with corresponding marker time series. The OOA profile was characterized by a dominant peak at m∕z 44 and was associated with aged emissions and secondary organic aerosol formation. BBOA showed high contributions of m∕z 29, m∕z 60 (), and m∕z 73 (), associated with cellulose pyrolysis products generated during biomass burning such as levoglucosan (Elser et al., 2016). The HOA+CCOA profile exhibited the typical fragmentation pattern of saturated and unsaturated hydrocarbons (Ng et al., 2011a). The COA profile was characterized by high signals at m∕z 55 (C3H3O+) (Crippa et al., 2013).
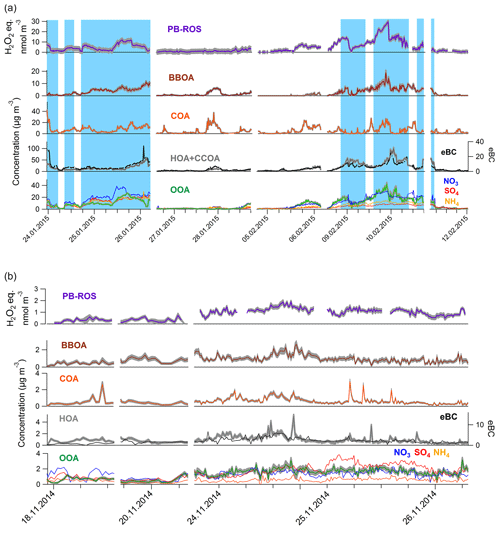
Figure 2Concentrations of PB-ROS, OA components, and eBC during the measurement periods in (a) Beijing and (b) Bern. In Beijing, the periods highlighted with a blue background represent the haze periods (visibility <10 km). The remaining periods were termed reference periods (visibility ≥10 km). The gray bands indicate 1σ errors of the PB-ROS measurements and of our best estimates of the factors.
To explore the correlations between the fine aerosol chemical species, OA sources, and PB-ROS (referred to as testing factors in the following), we calculated a Pearson correlation matrix from 1000 bootstrap tests of those testing factors in Beijing and Bern (see Sect. 2.3 and Fig. S7). The average diurnal patterns of the OA components during the measurement periods in Beijing and Bern are shown in Fig. S8 and the time series of PB-ROS and the different identified source contributions are presented in Fig. 2. Site-specific observations are discussed in the following.
Beijing. As shown in Fig. 2a, PB-ROS as well as all the other aerosol species exhibited a simultaneous increase during the haze periods. Most of the variables had a strong () or moderate () positive Pearson correlation (Fig. S7a), which may be due to the influence of meteorological factors (Chen et al., 2017). HOA+CCOA correlated with eBC (), a tracer for traffic and coal combustion emissions. HOA+CCOA strongly decreased during noon, most likely due to a combined effect of decreased vehicular emissions (e.g., heavy-duty diesel vehicles are only allowed to operate from 22:00 to 06:00 LT) (BTMB, 2004; Lin et al., 2009) and an increase in the mixing height. COA showed clear peaks during lunch (from 12:00 to 13:00 LT) and dinner time (from 18:00 to 23:00 LT) in both haze and reference days, consistent with local emissions from cooking processes. During both episodes, BBOA contributed less than 10 % to the total OA (8.9 % and 6.6 % during haze and reference episodes, respectively), with little diurnal variability. The secondary inorganic components of PM2.5 (SO4, NO3, and NH4; see Fig. S5) followed the same trend as OOA, suggesting that these components were formed through similar atmospheric transformation pathways. The PB-ROS concentration closely followed the trend of OOA with the highest average concentrations occurring during the afternoon and early night.
Bern. In Bern, the Pearson correlation coefficients showed similar features but were generally weaker than those in Beijing (Fig. S7b). The PB-ROS concentrations had moderate to high correlations with OA, SO4, Cl, BBOA, eBC, and OOA () but weak ones with NO3, NH4, COA, and HOA (). HOA increased during the day with a small peak during the morning rush hour (08:00 LT), representing urban traffic behavior. COA was elevated from late morning to early afternoon and again in the evening, reflecting local cooking activities. BBOA showed a clear increase during nighttime, related to residential heating with wood, a well-known aerosol source in Bern (Zotter et al., 2014). We separated eBC into the fossil fuel (eBCTR) and wood burning (eBCWB) fractions according to the method described by Sandradewi et al. (2008) and Zotter et al. (2017). As shown in Fig. S8b, eBCWB had the same diurnal trend as BBOA, characterized by higher nighttime values, while eBCTR showed the opposite trend rather consistent with HOA, confirming the source apportionment results. The secondary inorganic component SO4 correlated with OOA similarly to Beijing (Fig. 2b). These secondary components dominated the PM burden, indicating the importance of atmospheric aging to the chemical composition of the aerosol. PB-ROS levels also followed OOA in Bern (Fig. S8b) with no substantial diurnal trends, except for a slight increase during daytime.
It should be noted that these correlations are not necessarily causative relations. For example, the inorganic species NO3, SO4, and NH4 correlated with each other due to their photochemical production and ammonium salt formation. As we measured the water-soluble PB-ROS concentration in the aerosol phase, eBC is not considered a direct contributor to PB-ROS due to its water-insoluble character but indirectly by catalyzing the formation of oxidants at its surface in the particle. The correlation of PB-ROS with eBC may also be a coincidence caused by the superposition of the two opposite trends of eBCWB and eBCTR. Also, our previous tests showed that inorganic components like NO3 and SO4 did not contribute to the PB-ROS signal in the DCFH assay (Zhou et al., 2018a). Thus, we conclude that the correlations of the secondary inorganics with PB-ROS are a coincidence due to the fact that both are influenced by the atmospheric aging.
3.3 Source contributions to PB-ROS
A multiple linear regression model (MLRM) was applied to identify the dependence of the PB-ROS concentration on the different OA sources. First we fitted the observed PB-ROS data as the dependent variable to all different OA sources obtained from the source apportionment as the predictors (function 1 in Table 1). Secondly, to exclude any influence of the separation of the individual primary sources, we tested the contribution of the total primary emission sources (here combined as primary organic aerosol, ) to the ROS activity in Beijing by running the MLRM with only two variables, POA and OOA (using function 2 in Table 1). To assess the uncertainties related to the modeled ROS content values, we varied the source parameters by using 40 and 315 PMF solutions for Beijing and Bern, respectively. In addition, in each of these 40 (315) MLRM fits we used one set of PB-ROS concentrations generated by randomly varying the observed PB-ROS within the measurement uncertainties determined by the instrument precision and the background subtraction. The outcome of each MLRM fit provides one set of the PB-ROS content of the different OA components. Finally, we also performed 1000 bootstrap runs on the final PMF solution (the average PMF results of all the solutions) to test the sensitivity of the MLRM to the input data, especially in Beijing where model results may be potentially driven by the haze periods. The average regression coefficients obtained from MLRM associated with each independent variable are listed in Table 1. For a better overview, the overall distributions of these regression coefficients are also illustrated as boxplots in Fig. S9.
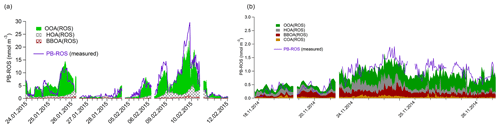
Figure 3Comparison of measured (purple line) and simulated PB-ROS concentrations in (a) Beijing and (b) Bern, where HOA(ROS), COA(ROS), BBOA(ROS), and OOA(ROS) denote the corresponding source contributions to the simulated PB-ROS activity. Note that in Beijing, HOA(ROS) and BBOA(ROS) represent the potential contribution of the HOA and BBOA contributions to PB-ROS, by adding 1 standard deviation to the regression coefficients listed in Table 1 (function 1).
The measured water-soluble PB-ROS time series were fairly well captured by the model at both locations (Fig. 3), with 61 % of the variability explained in Bern and 77 % in Beijing (see Fig. S10 for the normalized frequency distributions of R2). For Beijing, the unexplained variance could be related to the measurement uncertainties, while for Bern the model did not explain the entire variance in the data (22 % of the remaining variability could be related to uncertainty and 17 % remained unexplained). This unexplained part may be related to the variability of the composition or precursors of the OA factors in Bern.
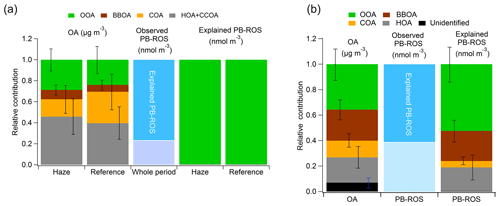
Figure 4Average relative contributions of the OA sources to the observed total OA and to the explained PB-ROS during the measurement period in (a) Beijing and (b) Bern. Data in Beijing are separated for haze and reference periods. The error bars represent the standard deviation of the mean of the whole measurement period.
For Bern, PMF attributed 57 % to the three primary sources BBOA (24 %), HOA (20 %), and COA (13 %) and 36 % to OOA, as shown in Fig. 4b during the whole measurement period (note that the remaining 7 % was attributed to the unidentified factor). However, these OA fractions contributed differently to PB-ROS. OOA was a much higher contributor to the observed PB-ROS (52 % of explained PB-ROS) followed by contributions by BBOA (24 %), HOA (19 %), and a small contribution by COA (5 %). In Beijing, the contribution of OOA to total OA was lower with 25 %. HOA+CCOA was by far the highest primary source (43 %) followed by small contributions by COA (24 %) and BBOA (8 %) during the whole measurement period. Figure 4a shows the contribution of these OA factors during haze and reference days separately. However, concerning ROS, OOA was the main contributor to PB-ROS, while the contributions of the individual primary OA sources to PB-ROS activity were statistically not different from zero within our uncertainties, when these sources were considered individually (Table 1: function 1) or lumped together (Table 1: function 2). We note that this retrieval is dependent on (i) the covariance between factors used as dependent variables and (ii) on the factor relative contributions to the overall ROS activity. In Beijing the OA factors are highly covariant, all increasing during haze. By contrast, in Bern, two regimes were encountered during the measurement period, with the dominance of primary biomass smoke and secondary organic aerosols, during the first and second half of the campaign, respectively. Such variability facilitated the extraction of the contribution of both primary and secondary sources to the ROS activity. The mass spectral profiles also show a higher O:C ratio of OOA in Beijing compared to Bern, while it is the opposite for BBOA (Fig. S3). This might indicate that PMF could have attributed the most oxidized fraction of BBOA to OOA in Beijing. Indeed, other source attribution analyses (Elser et al., 2016; Xu et al., 2019) have obtained higher BBOA fractions of 9 %–20 % and a higher O:C ratio of 0.32–0.39 for BBOA compared to 7±5 % and in this study. In addition, the sources of POA are different at both locations. In Beijing, the contribution of HOA+CCOA, which has a low PB-ROS activity, is much larger than in Bern. By contrast, BBOA, which is ROS active, constitutes a large fraction of POA in Bern.
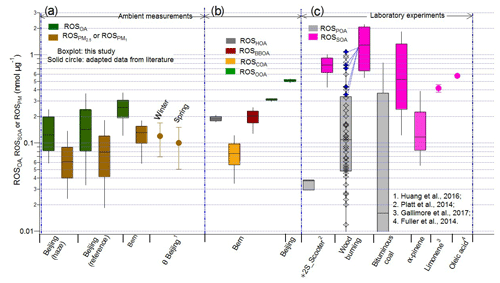
Figure 5Comparison of the ROS content in aerosols from different sources (listed on the x axis) as measured by the DCFH assay. (a) PB-ROS content in OA (ROSOA) and PM (ROS for Beijing and ROS for Bern) at ambient sites. For comparison, the ROS contents for winter and spring in Beijing (represented as θ Beijing) are calculated from the ROS concentrations reported in the literature in equivalent nmol H2O2 m−3 air and then normalized to PM2.5 concentrations obtained from the Embassy of U.S. Department of State (2017); (b) PB-ROS contents of individual source factors in Bern and Beijing; (c) PB-ROS contents in primary (ROSPOA) and secondary organic aerosol (ROSSOA) from wood and coal burning, in SOA from α-pinene (this study, as well as literature data for two-stroke scooters (2S_Scooter; Platt et al., 2014), for SOA from limonene (Gallimore et al., 2017), and oleic acid (Fuller et al., 2014)). The blue points and the dotted blue lines in the wood burning experiment are used to identify the corresponding ROSPOA of the listed ROSSOA. For the ROSPOA of the 2S_Scooter (gasoline emissions), we take only the points right before the lights are turned on from the original data as earlier data points seemed to be still influenced by incomplete mixing of emissions in the chamber.
To further evaluate the contribution of primary emissions to PB-ROS in Beijing, we performed additional MLRM runs where the ratio of ROSSOA∕ROSPOA was constrained between 8 and 18 as shown in the laboratory studies below (Fig. 5). Even though ROSPOA and ROSSOA are highly variable in the laboratory studies, their ratio is not. With this approach the contribution of POA to the overall PB-ROS in Beijing was determined to range between 9 % and 18 %. We note that the constrained and unconstrained models exhibited a statistically similar goodness of fit. This shows that the OOA ROS content is not sensitive to these constraints and suggests that the contribution of POA to the total PB-ROS activity in Beijing does not exceed 18 %. Furthermore, in Fig. 3a we show the potential contribution from primary sources to PB-ROS, which is calculated from the upper limit of their regression coefficients (regression coefficient + 1 standard deviation) listed in Table 1 (function 1). This illustrates that any enhanced contribution by POA could not improve the model.
On average, OA and PB-ROS were driven by different general sources derived from PMF and ME-2 (Fig. 4). For example in Beijing, even though the primary OA sources contributed ∼75 % to the total OA, their lumped contribution to PB-ROS was much lower.
3.4 Comparison of the PB-ROS content of OA from different sources
Figure 5 compiles online measurements of the PB-ROS contents in aerosols measured by the DCFH assay from various ambient and laboratory measurements performed in this study or taken from literature. Ambient measurements are presented as the ROS contents of the total measured aerosol mass (PM2.5 in Beijing and PM1 in Bern, denoted as ROS) or the organic aerosol (OA) fraction alone (ROSOA) as determined from the MLRM. Note that PM2.5∕PM1 is about 1.1–1.3, thus the usage of PM1 in Bern does not much influence a comparison of ROS and ROSOA from PM2.5 measurements. Our values for ROS (0.07±0.04 and 0.09±0.06 nmol µg−1 for “Beijing (haze)” and “Beijing (reference)”, respectively) are in line with other online measurements reported by Huang et al. (2016) for Beijing (0.12±0.05 and 0.10±0.05 nmol µg−1 in winter 2014 and spring 2015, respectively), and the value of ROS (0.13±0.06 nmol µg−1) for Bern is not significantly different from the values in China. Figure 5b indicates the PB-ROS contents attributed to different sources identified in Bern and Beijing, and Fig. 5c summarizes the PB-ROS contents from our own laboratory measurements of different emission sources (see Sect. 2.1), complemented by literature values, i.e., two-stroke scooter emissions (2S_Scooter; Platt et al., 2014), as well as SOA from limonene and oleic acid oxidation (Gallimore et al., 2017; Fuller et al., 2014). The PB-ROS contents in primary emissions (ROSPOA) from two-stroke scooters or wood and coal burning are about 4 to 25 times lower than those in the corresponding SOA samples (ROSSOA; see Sect. 2.4). Results are consistent with the field measurements, where the PB-ROS content in OOA is 2–50 times higher than that in primary emission sources (see Fig. S9 and Table 1). Clearly, the PB-ROS content is enhanced by photochemical reactions, although the enhancement depends on the emitted precursors. SOA from anthropogenic emissions (vehicular exhaust and coal and biomass burning) includes in general a higher ROS activity compared to biogenic SOA from monoterpene precursors. For example, SOA from α-pinene, the most emitted monoterpene compound, has a 2–10 times lower PB-ROS content than SOA from the anthropogenic sources. Thus, despite the widespread dominance of biogenic SOA, especially during summer, SOA formed from anthropogenic precursors might still dominate the PB-ROS burden in the atmosphere. Previously we have shown that the degree of oxygenation and the OA loading do influence the PB-ROS content of aerosols in a specific source (biomass burning) (Zhou et al., 2018b). However, Fig. S11 shows that the variation of PB-ROS in SOA within a specific source is smaller than the differences between the sources and precursors. This indicates that the PB-ROS content of SOA is more driven by the nature of the precursor. As VOCs emitted from wood and coal combustion contain a large fraction of aromatic hydrocarbons (Bruns et al., 2017; Klein et al., 2018), this might indicate that aromatic VOCs yield a more PB-ROS-active aerosol compared to monoterpenes.
Overall, the ROSBBOA derived from the Bern data was in the same range as ROSPOA of wood burning emissions obtained from the laboratory study. The contribution of HOA+CCOA to PB-ROS was found to be negligible for Beijing within the analysis uncertainties (see Table 1), while in laboratory experiments PB-ROS was observed in primary and secondary emissions from bituminous coal but not from anthracite coal. The fairly low and variable PB-ROS content in primary emissions from laboratory studies may explain why we could not derive its contribution to the measured PB-ROS in Beijing. ROSOOA in both Beijing and Bern was about 2 to 4 times lower than ROSSOA of gasoline and wood burning emissions but equal or higher than ROSSOA of coal burning, α-pinene, limonene, and oleic acid. It is reasonable to assume that the ambient OOA was formed from a complex mixture of precursors from various sources like traffic, wood burning, coal burning, and biogenic gases, and therefore the ambient ROSSOA is expected to lie somewhere in the range of these sources.
The ROS content measured with the BPEA-nit assay, which also determines PB-ROS, was in the range of 0.05–0.4 nmol µg−1 for diesel or biodiesel POA showing an increasing trend with the oxygen content of the fuel (Stevanovic et al., 2013; Hedayat et al., 2016), while a higher PB-ROS content (0.2–4 nmol µg−1) was reported in primary aerosols from wood combustion (Miljevic et al., 2010). These concentrations are similar to our values for the scooter and coal POA as well as BBOA. In addition, they also showed that aging of these emissions led to a higher PB-ROS content, which is confirmed by our observations (Stevanovic et al., 2013; Pourkhesalian et al., 2015).
OA, dominated by secondary aerosol, is the largest fraction of PM1 mass worldwide. Using both field and laboratory measurements, we provide compelling evidence that PB-ROS are predominantly secondary. We estimate that while less than 40 % of the OA mass at the urban locations investigated is secondary, this fraction contributes more than 60 % of the total PB-ROS. These findings are corroborated by chamber experiments, showing that atmospheric aging does not only enhance the OA aerosol mass but also results in the formation of 10 times more ROS-active material (nmol µg−1) compared to POA. We show that SOA from complex anthropogenic precursor mixtures is especially ROS active, implying that despite its lower overall contribution to the OA burden compared to biogenic SOA anthropogenic emissions can be an important PB-ROS source. Indeed, epidemiological studies revealed seasonal trends in health effects of PM. Studies in the US, western Europe, Japan, and South Korea, including up to 112 cities and time periods up to 13 years, showed a stronger effect of PM on mortality in spring, summer, or fall compared to winter (Peng et al., 2005; Nawrot et al., 2007; Zanobetti and Schwartz, 2009; Franklin et al., 2008; Yi et al., 2010). Kim et al. (2015) reported the highest overall risk of PM10 for nonaccidental or cardiovascular mortality on extremely hot days. However, in China the effects were highest in summer and winter (Kim et al., 2017; Chen et al., 2013). All in all, except for locations affected by strong haze and specific sources of toxic components (e.g., polycyclic aromatic hydrocarbons (PAHs) and coal combustion in winter in China), a stronger association between PM and mortality was observed at different locations during hotter days, when SOA formation is the most active. Various experiments have also shown that pure SOA does induce adverse effects upon exposure of airway epithelial cells (Arashiro et al., 2016, 2018; Gaschen et al., 2010; Lin et al., 2016; Chowdury et al., 2018; Jiang et al., 2016). Similarly, cellular-based ROS assays showed a strong connection with pure SOA or its fraction in PM (Tuet et al., 2016, 2017a, b; Saffari et al., 2014). Further, Lakey et al. (2016) developed a model to simulate the response of air pollutants including PM on the production rates and concentrations of ROS in the epithelial lining fluid of the human respiratory tract. They found that the OH production rate from SOA in the lung could be as high as the H2O2 production rate from redox-active trace metals and quinones. Others reported that SOA dissolved in water exhibited a high OH radical or H2O2 generation under irradiation (Wang et al., 2011b; Lim and Turpin, 2015; Badali et al., 2015) or a direct formation of OH under dark conditions (Tong et al., 2016). The DCFH assay for exogenous ROS has so far not been applied as tracer for ROS activity and oxidation potential of PM to our knowledge. The most frequently used assay with DTT shows in some cases good associations with oxidized OA and high values in summer, while in other studies better relations with biomass burning and traffic PM components as well as high winter values are observed (Fang et al., 2016; Verma et al., 2014, 2015; Bates et al., 2015). The DTT assay is sensitive to a broad range of components, peroxides, and redox cycling species like transition metals and quinones but may suffer from negative interferences between metals and quinones (Wang et al., 2018). Thus, a combination of measurements may be needed to better assess relations between PM composition, oxidative potential, and possible health effects. The DCFH assay responds to peroxides but not to quinones and transition metals and could thus be helpful to disentangle the various contributions in combination with a DTT assay. It is also known that particles do carry short- and long-lived ROS (Zhou et al., 2018a; Fuller et al., 2014; Krapf et al., 2016), whereby the former are mostly lost when filters are analyzed. A combination of different online and offline methods appears to be more suited for an optimized understanding of the various short- and long-lived ROS. Research on links between PB-ROS quantified by the DCFH assay and adverse biological effects of PM should also be envisaged.
Data related to this article are available at https://doi.org/10.5281/zenodo.3520219 (Zhou and Dommen, 2019).
The supplement related to this article is available online at: https://doi.org/10.5194/acp-19-14703-2019-supplement.
JZ is responsible for the bulk of the conceptualization, measurements, data analysis and writing with contributions from all authors. JZ, JD, UB, MG, RJH, JC, IEH, and ASHP designed the experiments. JZ, JD, MK, RF, and MG carried out the field observation experiment in Bern; JZ, ME, JD, RJH, QW, YW, and YZ carried out the field observation experiment in Beijing. JZ, DB, GS, PZ, EAB, and SB performed the wood combustion experiments; JZ, RJH, SMP, HN, and GS carried out the coal combustion experiments; JZ, MK, and JD carried out the α-pinene ozonolysis experiments. JZ, ME, and RF analyzed the data of field measurements; JZ, GS, DB, and SMP analyzed the data of laboratory experiments; JZ and ME prepared the display items; JZ and JD collected the literature data from ambient and laboratory studies.
The authors declare that they have no conflict of interest.
This research has been supported by the Swiss National Science Foundation (grant nos. CR32I3-140851, CR32I3_166325, 200021L_140590, BSSGI0_155846, and NRP 70 “Energy Turnaround”), the European Union's Horizon 2020 Research and Innovation programme through the EUROCHAMP-2020 Infrastructure Activity under grant agreement no. 730997, the National Natural Science Foundation of China (grant nos. 91644219 and 21661132005), the National Key Research and Development Program of China (grant no. 2017YFC0212701), the China Scholarship Council (grant no. 201307040040), the Sino-Swiss Science Technology Cooperation (SSSTC) project HAZECHINA (Haze pollution in China: Sources and atmospheric evolution of particulate matter, grant no. IZLCZ2_169986), and the Swiss Innovation Agency Innosuisse (SCCER BIOSWEET).
This paper was edited by Xavier Querol and reviewed by Zoran Ristovski and two anonymous referees.
Adam, M., Schikowski, T., Carsin, A. E., Cai, Y., Jacquemin, B., Sanchez, M., Vierkötter, A., Marcon, A., Keidel, D., Sugiri, D., Al Kanani, Z., Nadif, R., Siroux, V., Hardy, R., Kuh, D., Rochat, T., Bridevaux, P.-O., Eeftens, M., Tsai, M.-Y., Villani, S., Phuleria, H. C., Birk, M., Cyrys, J., Cirach, M., de Nazelle, A., Nieuwenhuijsen, M. J., Forsberg, B., de Hoogh, K., Declerq, C., Bono, R., Piccioni, P., Quass, U., Heinrich, J., Jarvis, D., Pin, I., Beelen, R., Hoek, G., Brunekreef, B., Schindler, C., Sunyer, J., Krämer, U., Kauffmann, F., Hansell, A. L., Künzli, N., and Probst-Hensch, N.: Adult lung function and long-term air pollution exposure. ESCAPE: a multicentre cohort study and meta-analysis, Eur. Respir. J., 45, 38–50, https://doi.org/10.1183/09031936.00130014, 2015.
Adams, K., Greenbaum, D. S., Shaikh, R., van Erp, A. M., and Russell, A. G.: Particulate matter components, sources, and health: Systematic approaches to testing effects, J. Air Waste Manag. Assoc., 65, 544–558, https://doi.org/10.1080/10962247.2014.1001884, 2015.
Arashiro, M., Lin, Y.-H., Sexton, K. G., Zhang, Z., Jaspers, I., Fry, R. C., Vizuete, W. G., Gold, A., and Surratt, J. D.: In vitro exposure to isoprene-derived secondary organic aerosol by direct deposition and its effects on COX-2 and IL-8 gene expression, Atmos. Chem. Phys., 16, 14079–14090, https://doi.org/10.5194/acp-16-14079-2016, 2016.
Arashiro, M., Lin, Y.-H., Zhang, Z., Sexton, K. G., Gold, A., Jaspers, I., Fry, R. C., and Surratt, J. D.: Effect of secondary organic aerosol from isoprene-derived hydroxyhydroperoxides on the expression of oxidative stress response genes in human bronchial epithelial cells, Environ. Science: Proc. Imp., 20, 332–339, https://doi.org/10.1039/C7EM00439G, 2018.
Badali, K. M., Zhou, S., Aljawhary, D., Antiñolo, M., Chen, W. J., Lok, A., Mungall, E., Wong, J. P. S., Zhao, R., and Abbatt, J. P. D.: Formation of hydroxyl radicals from photolysis of secondary organic aerosol material, Atmos. Chem. Phys., 15, 7831–7840, https://doi.org/10.5194/acp-15-7831-2015, 2015.
Barmet, P., Dommen, J., DeCarlo, P. F., Tritscher, T., Praplan, A. P., Platt, S. M., Prévôt, A. S. H., Donahue, N. M., and Baltensperger, U.: OH clock determination by proton transfer reaction mass spectrometry at an environmental chamber, Atmos. Meas. Tech., 5, 647–656, https://doi.org/10.5194/amt-5-647-2012, 2012.
Bates, J. T., Weber, R. J., Abrams, J., Verma, V., Fang, T., Klein, M., Strickland, M. J., Sarnat, S. E., Chang, H. H., Mulholland, J. A., Tolbert, P. E., and Russell, A. G.: Reactive oxygen species generation linked to sources of atmospheric particulate matter and cardiorespiratory effects, Environ. Sci. Technol., 49, 13605–13612, https://doi.org/10.1021/acs.est.5b02967, 2015.
Beelen, R., Raaschou-Nielsen, O., Stafoggia, M., Andersen, Z. J., Weinmayr, G., Hoffmann, B., Wolf, K., Samoli, E., Fischer, P., Nieuwenhuijsen, M., Vineis, P., Xun, W. W., Katsouyanni, K., Dimakopoulou, K., Oudin, A., Forsberg, B., Modig, L., Havulinna, A. S., Lanki, T., Turunen, A., Oftedal, B., Nystad, W., Nafstad, P., De Faire, U., Pedersen, N. L., Ostenson, C. G., Fratiglioni, L., Penell, J., Korek, M., Pershagen, G., Eriksen, K. T., Overvad, K., Ellermann, T., Eeftens, M., Peeters, P. H., Meliefste, K., Wang, M., Bueno-de-Mesquita, B., Sugiri, D., Kramer, U., Heinrich, J., de Hoogh, K., Key, T., Peters, A., Hampel, R., Concin, H., Nagel, G., Ineichen, A., Schaffner, E., Probst-Hensch, N., Kunzli, N., Schindler, C., Schikowski, T., Adam, M., Phuleria, H., Vilier, A., Clavel-Chapelon, F., Declercq, C., Grioni, S., Krogh, V., Tsai, M. Y., Ricceri, F., Sacerdote, C., Galassi, C., Migliore, E., Ranzi, A., Cesaroni, G., Badaloni, C., Forastiere, F., Tamayo, I., Amiano, P., Dorronsoro, M., Katsoulis, M., Trichopoulou, A., Brunekreef, B., and Hoek, G.: Effects of long-term exposure to air pollution on natural-cause mortality: an analysis of 22 European cohorts within the multicentre ESCAPE project, Lancet, 383, 785–795, https://doi.org/10.1016/s0140-6736(13)62158-3, 2014.
Bruns, E. A., Slowik, J. G., El Haddad, I., Kilic, D., Klein, F., Dommen, J., Temime-Roussel, B., Marchand, N., Baltensperger, U., and Prévôt, A. S. H.: Characterization of gas-phase organics using proton transfer reaction time-of-flight mass spectrometry: fresh and aged residential wood combustion emissions, Atmos. Chem. Phys., 17, 705–720, https://doi.org/10.5194/acp-17-705-2017, 2017.
BTMB: Beijing Traffic Management Bureau, Beijing, 2004 (in Chinese).
Burnett, R. T., Brook, J., Dann, T., Delocla, C., Philips, O., Cakmak, S., Vincent, R., Goldberg, M. S., and Krewski, D.: Association between particulate- and gas-phase components of urban air pollution and daily mortality in eight Canadian cities, Inhal. Toxicol., 12, 15–39, https://doi.org/10.1080/08958370050164851, 2000.
Calas, A., Uzu, G., Kelly, F. J., Houdier, S., Martins, J. M. F., Thomas, F., Molton, F., Charron, A., Dunster, C., Oliete, A., Jacob, V., Besombes, J.-L., Chevrier, F., and Jaffrezo, J.-L.: Comparison between five acellular oxidative potential measurement assays performed with detailed chemistry on PM10 samples from the city of Chamonix (France), Atmos. Chem. Phys., 18, 7863–7875, https://doi.org/10.5194/acp-18-7863-2018, 2018.
Canonaco, F., Crippa, M., Slowik, J. G., Baltensperger, U., and Prévôt, A. S. H.: SoFi, an IGOR-based interface for the efficient use of the generalized multilinear engine (ME-2) for the source apportionment: ME-2 application to aerosol mass spectrometer data, Atmos. Meas. Tech., 6, 3649–3661, https://doi.org/10.5194/amt-6-3649-2013, 2013.
Charrier, J. G. and Anastasio, C.: On dithiothreitol (DTT) as a measure of oxidative potential for ambient particles: evidence for the importance of soluble transition metals, Atmos. Chem. Phys., 12, 9321–9333, https://doi.org/10.5194/acp-12-9321-2012, 2012.
Chen, R., Peng, R. D., Meng, X., Zhou, Z., Chen, B., and Kan, H.: Seasonal variation in the acute effect of particulate air pollution on mortality in the China Air Pollution and Health Effects Study (CAPES), Sci. Total Environ., 450–451, 259–265, https://doi.org/10.1016/j.scitotenv.2013.02.040, 2013.
Chen, Z., Cai, J., Gao, B., Xu, B., Dai, S., He, B., and Xie, X.: Detecting the causality influence of individual meteorological factors on local PM2.5 concentration in the Jing-Jin-Ji region, Sci. Rep.-UK, 7, 40735, https://doi.org/10.1038/srep40735, 2017.
China Meteorological Administration: Observation and forecasting levels of haze. The State Standard of the People's Republic of China QX/T 113-2010, China Meteorological Press, 2010 (in Chinese).
Cho, A. K., Sioutas, C., Miguel, A. H., Kumagai, Y., Schmitz, D. A., Singh, M., Eiguren-Fernandez, A., and Froines, J. R.: Redox activity of airborne particulate matter at different sites in the Los Angeles Basin, Environ. Res., 99, 40–47, https://doi.org/10.1016/j.envres.2005.01.003, 2005.
Chowdhury, P. H., He, Q., Lasitza Male, T., Brune, W. H., Rudich, Y., and Pardo, M.: Exposure of lung epithelial cells to photochemically aged secondary organic aerosol shows increased toxic effects, Environ. Sci. Technol. Lett., 5, 424–430, https://doi.org/10.1021/acs.estlett.8b00256, 2018.
Cohen, A. J.: Outdoor air pollution and lung cancer, Environ. Health Perspect., 108, 743–750, 2000.
Cohen, A. J., Brauer, M., Burnett, R., Anderson, H. R., Frostad, J., Estep, K., Balakrishnan, K., Brunekreef, B., Dandona, L., Dandona, R., Feigin, V., Freedman, G., Hubbell, B., Jobling, A., Kan, H., Knibbs, L., Liu, Y., Martin, R., Morawska, L., Pope, C. A., 3rd, Shin, H., Straif, K., Shaddick, G., Thomas, M., van Dingenen, R., van Donkelaar, A., Vos, T., Murray, C. J. L., and Forouzanfar, M. H.: Estimates and 25-year trends of the global burden of disease attributable to ambient air pollution: an analysis of data from the Global Burden of Diseases Study 2015, Lancet, 389, 1907–1918, https://doi.org/10.1016/s0140-6736(17)30505-6, 2017.
Crippa, M., El Haddad, I., Slowik, J. G., DeCarlo, P. F., Mohr, C., Heringa, M. F., Chirico, R., Marchand, N., Sciare, J., Baltensperger, U., and Prévôt, A. S. H.: Identification of marine and continental aerosol sources in Paris using high resolution aerosol mass spectrometry, J. Geophys. Res.-Atmos., 118, 1950–1963, https://doi.org/10.1002/jgrd.50151, 2013.
DeCarlo, P. F., Kimmel, J. R., Trimborn, A., Northway, M. J., Jayne, J. T., Aiken, A. C., Gonin, M., Fuhrer, K., Horvath, T., Docherty, K. S., Worsnop, D. R., and Jimenez, J. L.: Field-deployable, high-resolution, time-of-flight aerosol mass spectrometer, Anal. Chem., 78, 8281–8289, https://doi.org/10.1021/ac061249n, 2006.
Dockery, D. W., Pope, C. A., Xu, X., Spengler, J. D., Ware, J. H., Fay, M. E., Ferris, B. G. J., and Speizer, F. E.: An association between air pollution and mortality in six U.S. cities, N. Engl. J. Med., 329, 1753–1759, https://doi.org/10.1056/nejm199312093292401, 1993.
Drinovec, L., Močnik, G., Zotter, P., Prévôt, A. S. H., Ruckstuhl, C., Coz, E., Rupakheti, M., Sciare, J., Müller, T., Wiedensohler, A., and Hansen, A. D. A.: The “dual-spot” Aethalometer: an improved measurement of aerosol black carbon with real-time loading compensation, Atmos. Meas. Tech., 8, 1965–1979, https://doi.org/10.5194/amt-8-1965-2015, 2015.
Du, Y., Xu, X., Chu, M., Guo, Y., and Wang, J.: Air particulate matter and cardiovascular disease: the epidemiological, biomedical and clinical evidence, J. Thoracic Dis., 8, E8–E19, https://doi.org/10.3978/j.issn.2072-1439.2015.11.37, 2016.
Elser, M., Huang, R.-J., Wolf, R., Slowik, J. G., Wang, Q., Canonaco, F., Li, G., Bozzetti, C., Daellenbach, K. R., Huang, Y., Zhang, R., Li, Z., Cao, J., Baltensperger, U., El-Haddad, I., and Prévôt, A. S. H.: New insights into PM2.5 chemical composition and sources in two major cities in China during extreme haze events using aerosol mass spectrometry, Atmos. Chem. Phys., 16, 3207–3225, https://doi.org/10.5194/acp-16-3207-2016, 2016.
Embassy of U.S. Department of State: Mission China, Beijing, available at: http://www.stateair.net/web/historical/1/1.html (note that these data are not fully verified or validated), last access: 1 October 2017.
Fang, T., Verma, V., Bates, J. T., Abrams, J., Klein, M., Strickland, M. J., Sarnat, S. E., Chang, H. H., Mulholland, J. A., Tolbert, P. E., Russell, A. G., and Weber, R. J.: Oxidative potential of ambient water-soluble PM2.5 in the southeastern United States: contrasts in sources and health associations between ascorbic acid (AA) and dithiothreitol (DTT) assays, Atmos. Chem. Phys., 16, 3865–3879, https://doi.org/10.5194/acp-16-3865-2016, 2016.
Franklin, M., Koutrakis, P., and Schwartz, P.: The role of particle composition on the association between PM2.5 and mortality, Epidemiology, 19, 680–689, 2008.
Fröhlich, R., Cubison, M. J., Slowik, J. G., Bukowiecki, N., Prévôt, A. S. H., Baltensperger, U., Schneider, J., Kimmel, J. R., Gonin, M., Rohner, U., Worsnop, D. R., and Jayne, J. T.: The ToF-ACSM: a portable aerosol chemical speciation monitor with TOFMS detection, Atmos. Meas. Tech., 6, 3225–3241, https://doi.org/10.5194/amt-6-3225-2013, 2013.
Fröhlich, R., Crenn, V., Setyan, A., Belis, C. A., Canonaco, F., Favez, O., Riffault, V., Slowik, J. G., Aas, W., Aijälä, M., Alastuey, A., Artiñano, B., Bonnaire, N., Bozzetti, C., Bressi, M., Carbone, C., Coz, E., Croteau, P. L., Cubison, M. J., Esser-Gietl, J. K., Green, D. C., Gros, V., Heikkinen, L., Herrmann, H., Jayne, J. T., Lunder, C. R., Minguillón, M. C., Močnik, G., O'Dowd, C. D., Ovadnevaite, J., Petralia, E., Poulain, L., Priestman, M., Ripoll, A., Sarda-Estève, R., Wiedensohler, A., Baltensperger, U., Sciare, J., and Prévôt, A. S. H.: ACTRIS ACSM intercomparison – Part 2: Intercomparison of ME-2 organic source apportionment results from 15 individual, co-located aerosol mass spectrometers, Atmos. Meas. Tech., 8, 2555–2576, https://doi.org/10.5194/amt-8-2555-2015, 2015.
Fuller, S. J., Wragg, F. P. H., Nutter, J., and Kalberer, M.: Comparison of on-line and off-line methods to quantify reactive oxygen species (ROS) in atmospheric aerosols, Atmos. Environ., 92, 97–103, https://doi.org/10.1016/j.atmosenv.2014.04.006, 2014.
Gallimore, P. J., Mahon, B. M., Wragg, F. P. H., Fuller, S. J., Giorio, C., Kourtchev, I., and Kalberer, M.: Multiphase composition changes and reactive oxygen species formation during limonene oxidation in the new Cambridge Atmospheric Simulation Chamber (CASC), Atmos. Chem. Phys., 17, 9853–9868, https://doi.org/10.5194/acp-17-9853-2017, 2017.
Gaschen, A., Lang, D., Kalberer, M., Savi, M., Geiser, T., Gazdhar, A., Lehr, C.-M., Bur, M., Dommen, J., Baltensperger, U., and Geiser, M.: Cellular responses after exposure of lung cell cultures to secondary organic aerosol particles, Environ. Sci. Technol., 44, 1424–1430, https://doi.org/10.1021/es902261m, 2010.
Guarnieri, M. and Balmes, J. R.: Outdoor air pollution and asthma, Lancet, 383, 1581–1592, https://doi.org/10.1016/S0140-6736(14)60617-6, 2014.
Hasson, A. S. and Paulson, S. E.: An investigation of the relationship between gas-phase and aerosol-borne hydroperoxides in urban air, J. Aerosol Sci., 34, 459–468, https://doi.org/10.1016/S0021-8502(03)00002-8, 2003.
Hedayat, F., Stevanovic, S., Milic, A., Miljevic, B., Nabi, M. N., Zare, A., Bottle, S. E., Brown, R. J., and Ristovski, Z. D.: Influence of oxygen content of the certain types of biodiesels on particulate oxidative potential, Sci. Total Environ., 545–546, 381–388, https://doi.org/10.1016/j.scitotenv.2015.12.036, 2016.
Huang, R.-J., Zhang, Y., Bozzetti, C., Ho, K.-F., Cao, J.-J., Han, Y., Daellenbach, K. R., Slowik, J. G., Platt, S. M., Canonaco, F., Zotter, P., Wolf, R., Pieber, S. M., Bruns, E. A., Crippa, M., Ciarelli, G., Piazzalunga, A., Schwikowski, M., Abbaszade, G., Schnelle-Kreis, J., Zimmermann, R., An, Z., Szidat, S., Baltensperger, U., Haddad, I. E., and Prevot, A. S. H.: High secondary aerosol contribution to particulate pollution during haze events in China, Nature, 514, 218–222, https://doi.org/10.1038/nature13774, 2014.
Huang, W., Zhang, Y., Zhang, Y., Zeng, L., Dong, H., Huo, P., Fang, D., and Schauer, J. J.: Development of an automated sampling-analysis system for simultaneous measurement of reactive oxygen species (ROS) in gas and particle phases: GAC-ROS, Atmos. Environ., 134, 18–26, https://doi.org/10.1016/j.atmosenv.2016.03.038, 2016.
Janssen, N. A. H., Strak, M., Yang, A., Hellack, B., Kelly, F. J., Kuhlbusch, T. A. J., Harrison, R. M., Brunekreef, B., Cassee, F. R., Steenhof, M., and Hoek, G.: Associations between three specific a-cellular measures of the oxidative potential of particulate matter and markers of acute airway and nasal inflammation in healthy volunteers, Occup. Environ. Med., 72, 49–56, https://doi.org/10.1136/oemed-2014-102303, 2015.
Jiang, H., Jang, M., Sabo-Attwood, T., and Robinson, S. E.: Oxidative potential of secondary organic aerosols produced from photooxidation of different hydrocarbons using outdoor chamber 20 under ambient sunlight, Atmos. Environ., 131, 382–389, 2016.
Johnson, R. L.: Relative effects of air pollution on lungs and heart, Circulation, 109, 5–7, https://doi.org/10.1161/01.cir.0000110643.19575.79, 2004.
Kim, S. E., Lim, Y. H., and Kim, H.: Temperature modifies the association between particulate air pollution and mortality: A multi-city study in South Korea, Sci. Total Environ., 524–525, 376–383, https://doi.org/10.1016/j.scitotenv.2015.03.137, 2015.
Kim, S. E., Honda, Y., Hashizume, M., Kan, H., Lim, Y. H., Lee, H., Kim, C. T., Yi, S. M., and Kim, H.: Seasonal analysis of the short-term effects of air pollution on daily mortality in Northeast Asia, Sci. Total Environ., 576, 850–857, https://doi.org/10.1016/j.scitotenv.2016.10.036, 2017.
King, L. E. and Weber, R. J.: Development and testing of an online method to measure ambient fine particulate reactive oxygen species (ROS) based on the 2′,7′-dichlorofluorescin (DCFH) assay, Atmos. Meas. Tech., 6, 1647–1658, https://doi.org/10.5194/amt-6-1647-2013, 2013.
Klein, F., Pieber, S. M., Ni, H., Stefenelli, G., Bertrand, A., Kilic, D., Pospisilova, V., Temime-Roussel, B., Marchand, N., El Haddad, I., Slowik, J. G., Baltensperger, U., Cao, J., Huang, R.-J., and Prévôt, A. S. H.: Characterization of Gas-Phase Organics Using Proton Transfer Reaction Time-of-Flight Mass Spectrometry: Residential Coal Combustion, Environ. Sci. Technol., 52, 2612–2617, https://doi.org/10.1021/acs.est.7b03960, 2018.
Krapf, M., Haddad, I. E., Bruns, E. A., Molteni, U., Daellenbach, K. R., Prévôt, A. S. H., Baltensperger, U., and Dommen, J.: Labile peroxides in secondary organic aerosol, Chem, 1, 603–616, https://doi.org/10.1016/j.chempr.2016.09.007, 2016.
Künzi, L., Krapf, M., Daher, N., Dommen, J., Jeannet, N., Schneider, S., Platt, S., Slowik, J. G., Baumlin, N., Salathe, M., Prévôt, A. S. H., Kalberer, M., Strähl, C., Dümbgen, L., Sioutas, C., Baltensperger, U., and Geiser, M.: Toxicity of aged gasoline exhaust particles to normal and diseased airway epithelia, Sci. Rep.-UK, 5, 11801, https://doi.org/10.1038/srep11801, 2015.
Laden, F., Schwartz, J., Speizer, F. E., and Dockery, D. W.: Reduction in fine particulate air pollution and mortality: Extended follow-up of the Harvard Six Cities study, Am. J. Respir. Crit. Care Med., 173, 667–672, https://doi.org/10.1164/rccm.200503-443OC, 2006.
Lakey, P. S. J., Berkemeier, T., Tong, H., Arangio, A. M., Lucas, K., Pöschl, U., and Shiraiwa, M.: Chemical exposure-response relationship between air pollutants and reactive oxygen species in the human respiratory tract, Sci. Rep.-UK, 6, 32916, https://doi.org/10.1038/srep32916, 2016.
Lelieveld, J.: Clean air in the Anthropocene, Faraday Discuss., 200, 693–703, https://doi.org/10.1039/c7fd90032e, 2017.
Lepeule, J., Laden, F., Dockery, D., and Schwartz, J.: Chronic exposure to fine particles and mortality: an extended follow-up of the Harvard Six Cities study from 1974 to 2009, Environ. Health Perspect., 120, 965–970, https://doi.org/10.1289/ehp.1104660, 2012.
Lim, Y. B. and Turpin, B. J.: Laboratory evidence of organic peroxide and peroxyhemiacetal formation in the aqueous phase and implications for aqueous OH, Atmos. Chem. Phys., 15, 12867–12877, https://doi.org/10.5194/acp-15-12867-2015, 2015.
Lin, P., Hu, M., Deng, Z., Slanina, J., Han, S., Kondo, Y., Takegawa, N., Miyazaki, Y., Zhao, Y., and Sugimoto, N.: Seasonal and diurnal variations of organic carbon in PM2.5 in Beijing and the estimation of secondary organic carbon, J. Geophys. Res.-Atmos., 114, D00G11, https://doi.org/10.1029/2008JD010902, 2009.
Lin, Y.-H., Arashiro, M., Martin, E., Chen, Y., Zhang, Z., Sexton, K. G., Gold, A., Jaspers, I., Fry, R. C., and Surratt, J. D.: Isoprene-derived secondary organic aerosol induces the expression of oxidative stress response genes in human lung cells, Environ. Sci. Technol. Lett., 3, 250–254, https://doi.org/10.1021/acs.estlett.6b00151, 2016.
Lippmann, M., Chen L.-C., Gordon T., Ito K., and Thurston, G. D.: National particle component toxicity (NPACT) initiative: integrated epidemiologic and toxicologic studies of the health effects of particulate matter components, Research Report 177, Health Effects Institute, Boston, 2013.
Liu, J., Han, Y., Tang, X., Zhu, J., and Zhu, T.: Estimating adult mortality attributable to PM2.5 exposure in China with assimilated PM2.5 concentrations based on a ground monitoring network, Sci. Total Environ., 568, 1253–1262, https://doi.org/10.1016/j.scitotenv.2016.05.165, 2016.
Liu, J. C., Wilson, A., Mickley, L. J., Dominici, F., Ebisu, K., Wang, Y., Sulprizio, M. P., Peng, R. D., Yue, X., Son, J.-Y., Anderson, G. B., and Bell, M. L.: Wildfire-specific fine particulate matter and risk of hospital admissions in urban and rural counties, Epidemiology (Cambridge, Mass.), 28, 77–85, https://doi.org/10.1097/EDE.0000000000000556, 2017.
McCulloch, A., Aucott, M. L., Benkovitz, C. M., Graedel, T. E., Kleiman, G., Midgley, P. M., and Li, Y.-F.: Global emissions of hydrogen chloride and chloromethane from coal combustion, incineration and industrial activities: Reactive chlorine emissions inventory, J. Geophys. Res.-Atmos., 104, 8391–8403, https://doi.org/10.1029/1999JD900025, 1999.
Middlebrook, A. M., Bahreini, R., Jimenez, J. L., and Canagaratna, M. R.: Evaluation of composition-dependent collection efficiencies for the Aerodyne aerosol mass spectrometer using field data, Aerosol Sci. Technol., 46, 258–271, https://doi.org/10.1080/02786826.2011.620041, 2012.
Miljevic, B., Heringa, M. F., Keller, A., Meyer, N. K., Good, J., Lauber, A., DeCarlo, P. F., Fairfull-Smith, K. E., Nussbaumer, T., Burtscher, H., Prevot, A. S. H., Baltensperger, U., Bottle, S. E., and Ristovski, Z. D.: Oxidative potential of logwood and pellet burning particles assessed by a novel profluorescent nitroxide probe, Environ. Sci. Technol., 44, 6601–6607, https://doi.org/10.1021/es100963y, 2010.
Mudway, I. S., Stenfors, N., Duggan, S. T., Roxborough, H., Zielinski, H., Marklund, S. L., Blomberg, A., Frew, A. J., Sandstrom, T., and Kelly, F. J.: An in vitro and in vivo investigation of the effects of diesel exhaust on human airway lining fluid antioxidants, Arch. Biochem. Biophys., 423, 200–212, https://doi.org/10.1016/j.abb.2003.12.018, 2004.
Nawrot, T. S., Torfs, R., Fierens, F., De Henauw, S., Hoet, P. H., Van Kersschaever, G., De Backer, G., and Nemery, B.: Stronger associations between daily mortality and fine particulate air pollution in summer than in winter: evidence from a heavily polluted region in western Europe, J. Epidemiol. Commun. Health, 61, 146–149, https://doi.org/10.1136/jech.2005.044263, 2007.
Ng, N. L., Canagaratna, M. R., Jimenez, J. L., Chhabra, P. S., Seinfeld, J. H., and Worsnop, D. R.: Changes in organic aerosol composition with aging inferred from aerosol mass spectra, Atmos. Chem. Phys., 11, 6465–6474, https://doi.org/10.5194/acp-11-6465-2011, 2011a.
Ng, N. L., Herndon, S. C., Trimborn, A., Canagaratna, M. R., Croteau, P. L., Onasch, T. B., Sueper, D., Worsnop, D. R., Zhang, Q., Sun, Y. L., and Jayne, J. T.: An Aerosol Chemical Speciation Monitor (ACSM) for routine monitoring of the composition and mass concentrations of ambient aerosol, Aerosol Sci. Technol., 45, 780–794, https://doi.org/10.1080/02786826.2011.560211, 2011b.
Ostro, B., Tobias, A., Querol, X., Alastuey, A., Amato, F., Pey, J., Pérez, N., and Sunyer, J.: The effects of particulate matter sources on daily mortality: A case-crossover study of Barcelona, Spain, Environ. Health Perspect., 119, 1781–1787, https://doi.org/10.1289/ehp.1103618, 2011.
Paatero, P.: Least squares formulation of robust non-negative factor analysis, Chemom. Intell. Lab. Syst., 37, 23–35, https://doi.org/10.1016/S0169-7439(96)00044-5, 1997.
Paatero, P. and Tapper, U.: Analysis of different modes of factor analysis as least squares fit problems, Chemom. Intell. Lab. Syst., 18, 183–194, https://doi.org/10.1016/0169-7439(93)80055-M, 1993.
Paatero, P. and Tapper, U.: Positive matrix factorization: A non-negative factor model with optimal utilization of error estimates of data values, Environmetrics, 5, 111–126, https://doi.org/10.1002/env.3170050203, 1994.
Paulsen, D., Dommen, J., Kalberer, M., Prévôt, A. S. H., Richter, R., Sax, M., Steinbacher, M., Weingartner, E., and Baltensperger, U.: Secondary organic aerosol formation by irradiation of 1,3,5-trimethylbenzene-NOx-H2O in a new reaction chamber for atmospheric chemistry and physics, Environ. Sci. Technol., 2668–2678, https://doi.org/10.1021/es0489137, 2005.
Peng, R. D., Dominici, F., Pastor-Barriuso, R., Zeger, S. L., and Samet, J. M.: Seasonal analyses of air pollution and mortality in 100 US cities, Am. J. Epidemiol., 161, 585–594, https://doi.org/10.1093/aje/kwi075, 2005.
Perrone, M. G., Zhou, J., Malandrino, M., Sangiorgi, G., Rizzi, C., Ferrero, L., Dommen, J., and Bolzacchini, E.: PM chemical composition and oxidative potential of the soluble fraction of particles at two sites in the urban area of Milan, Northern Italy, Atmos. Environ., 128, 104–113, https://doi.org/10.1016/j.atmosenv.2015.12.040, 2016.
Platt, S. M., El Haddad, I., Zardini, A. A., Clairotte, M., Astorga, C., Wolf, R., Slowik, J. G., Temime-Roussel, B., Marchand, N., Ježek, I., Drinovec, L., Močnik, G., Möhler, O., Richter, R., Barmet, P., Bianchi, F., Baltensperger, U., and Prévôt, A. S. H.: Secondary organic aerosol formation from gasoline vehicle emissions in a new mobile environmental reaction chamber, Atmos. Chem. Phys., 13, 9141–9158, https://doi.org/10.5194/acp-13-9141-2013, 2013.
Platt, S. M., Haddad, I. E., Pieber, S. M., Huang, R. J., Zardini, A. A., Clairotte, M., Suarez-Bertoa, R., Barmet, P., Pfaffenberger, L., Wolf, R., Slowik, J. G., Fuller, S. J., Kalberer, M., Chirico, R., Dommen, J., Astorga, C., Zimmermann, R., Marchand, N., Hellebust, S., Temime-Roussel, B., Baltensperger, U., and Prévôt, A. S. H.: Two-stroke scooters are a dominant source of air pollution in many cities, Nature Comm., 5, 3749, https://doi.org/10.1038/ncomms4749, 2014.
Pope, C. A. I., Ezzati, M., and Dockery, D. W.: Fine-particulate air pollution and life expectancy in the United States, N. Engl. J. Med., 360, 376–386, https://doi.org/10.1056/NEJMsa0805646, 2009.
Pourkhesalian, A. M., Stevanovic, S., Rahman, M. M., Faghihi, E. M., Bottle, S. E., Masri, A. R., Brown, R. J., and Ristovski, Z. D.: Effect of atmospheric aging on volatility and reactive oxygen species of biodiesel exhaust nano-particles, Atmos. Chem. Phys., 15, 9099–9108, https://doi.org/10.5194/acp-15-9099-2015, 2015.
Ramadan, Z., Eickhout, B., Song, X.-H., Buydens, L. M. C., and Hopke, P. K.: Comparison of Positive Matrix Factorization and Multilinear Engine for the source apportionment of particulate pollutants, Chemometr. Intell. Lab. Syst., 66, 15–28, 2003.
Saffari, A., Daher, N., M Shafer, M., J Schauer, J., and Sioutas, C.: Global perspective on the oxidative potential of airborne particulate matter: A synthesis of research findings, Environ. Sci. Technol., 48, 7576–7583, 2014.
Salvi, S.: Health effects of ambient air pollution in children, Paediatr. Respir. Rev., 8, 275–280, https://doi.org/10.1016/j.prrv.2007.08.008, 2007.
Sandradewi, J., Prévôt, A. S. H., Szidat, S., Perron, N., Alfarra, M. R., Lanz, V. A., Weingartner, E., and Baltensperger, U.: Using aerosol light absorption measurements for the quantitative determination of wood burning and traffic emission contributions to particulate matter, Environ. Sci. Technol., 42, 3316–3323, https://doi.org/10.1021/es702253m, 2008.
Sciare, J., d'Argouges, O., Sarda-Estève, R., Gaimoz, C., Dolgorouky, C., Bonnaire, N., Favez, O., Bonsang, B., and Gros, V.: Large contribution of water-insoluble secondary organic aerosols in the region of Paris (France) during wintertime, J. Geophys. Res.-Atmos., 116, D22203, https://doi.org/10.1029/2011JD015756, 2011.
Shi, T., Schins, R. P., Knaapen, A. M., Kuhlbusch, T., Pitz, M., Heinrich, J., and Borm, P. J.: Hydroxyl radical generation by electron paramagnetic resonance as a new method to monitor ambient particulate matter composition, J. Environ. Monit.: JEM, 5, 550–556, 2003.
Shiraiwa, M., Ueda, K., Pozzer, A., Lammel, G., Kampf, C. J., Fushimi, A., Enami, S., Arangio, A. M., Fröhlich-Nowoisky, J., Fujitani, Y., Furuyama, A., Lakey, P. S. J., Lelieveld, J., Lucas, K., Morino, Y., Pöschl, U., Takahama, S., Takami, A., Tong, H., Weber, B., Yoshino, A., and Sato, K.: Aerosol health effects from molecular to global scales, Environ. Sci. Technol., 51, 13545–13567, https://doi.org/10.1021/acs.est.7b04417, 2017.
Sioutas, C., Kim, S., and Chang, M.: Development and evalution of a prototype ultrafine particle concentrator, J. Aerosol Sci., 30, 1001–1017, https://doi.org/10.1016/S0021-8502(98)00769-1, 1999.
Stevanovic, S., Miljevic, B., Surawski, N. C., Fairfull-Smith, K. E., Bottle, S. E., Brown, R., and Ristovski, Z. D.: Influence of oxygenated organic aerosols (OOAs) on the oxidative potential of diesel and biodiesel particulate matter, Environ. Sci. Technol., 47, 7655–7662, https://doi.org/10.1021/es4007433, 2013.
Tong, H., Arangio, A. M., Lakey, P. S. J., Berkemeier, T., Liu, F., Kampf, C. J., Brune, W. H., Pöschl, U., and Shiraiwa, M.: Hydroxyl radicals from secondary organic aerosol decomposition in water, Atmos. Chem. Phys., 16, 1761–1771, https://doi.org/10.5194/acp-16-1761-2016, 2016.
Tuet, W. Y., Fok, S., Verma, V., Tagle Rodriguez, M. S., Grosberg, A., Champion, J. A., and Ng, N. L.: Dose-dependent intracellular reactive oxygen and nitrogen species (ROS/RNS) production from particulate matter exposure: comparison to oxidative potential and chemical composition, Atmos. Environ., 144, 335–344, https://doi.org/10.1016/j.atmosenv.2016.09.005, 2016.
Tuet, W. Y., Chen, Y., Fok, S., Champion, J. A., and Ng, N. L.: Inflammatory responses to secondary organic aerosols (SOA) generated from biogenic and anthropogenic precursors, Atmos. Chem. Phys., 17, 11423–11440, https://doi.org/10.5194/acp-17-11423-2017, 2017a.
Tuet, W. Y., Chen, Y., Fok, S., Gao, D., Weber, R. J., Champion, J. A., and Ng, N. L.: Chemical and cellular oxidant production induced by naphthalene secondary organic aerosol (SOA): effect of redox-active metals and photochemical aging, Sci. Rep.-UK, 7, 15157, https://doi.org/10.1038/s41598-017-15071-8, 2017b.
Vedal, S., Kim, S. Y., Miller, K. A., Fox, J. R., Bergen, S., Gould, T., Kaufman, J. D., Larson, T. V., Sampson, P. D., Sheppard, L., Simpson, C. D., and Szpiro, A. A.: Section 1. NPACTepidemiologic study of components of fine particulate matter and cardiovascular disease in the MESA and WHI-OS cohorts, in: National Particle Component Toxicity (NPACT) Initiative Report on Cardiovascular Effects, Research Report 178, Health Effects Institute, Boston, MA, 9–128, 2013.
Venkatachari, P. and Hopke, P. K.: Development and laboratory testing of an automated monitor for the measurement of atmospheric particle-bound reactive oxygen species (ROS), Aerosol Sci. Technol., 42, 629–635, https://doi.org/10.1080/02786820802227345, 2008.
Verma, V., Rico-Martinez, R., Kotra, N., King, L., Liu, J., Snell, T. W., and Weber, R. J.: Contribution of water-soluble and insoluble components and their hydrophobic/hydrophilic subfractions to the reactive oxygen species-generating potential of fine ambient aerosols, Environ. Sci. Technol., 46, 11384–11392, https://doi.org/10.1021/es302484r, 2012.
Verma, V., Fang, T., Guo, H., King, L., Bates, J. T., Peltier, R. E., Edgerton, E., Russell, A. G., and Weber, R. J.: Reactive oxygen species associated with water-soluble PM2.5 in the southeastern United States: spatiotemporal trends and source apportionment, Atmos. Chem. Phys., 14, 12915–12930, https://doi.org/10.5194/acp-14-12915-2014, 2014.
Verma, V., Fang, T., Xu, L., Peltier, R. E., Russell, A. G., Ng, N. L., and Weber, R. J.: Organic aerosols associated with the generation of reactive oxygen species (ROS) by water-soluble PM2.5, Environ. Sci. Technol., 49, 4646–4656, https://doi.org/10.1021/es505577w, 2015.
Viana, M., Kuhlbusch, T. A. J., Querol, X., Alastuey, A., Harrison, R. M., Hopke, P. K., Winiwarter, W., Vallius, M., Szidat, S., Prévôt, A. S. H., Hueglin, C., Bloemen, H., Wåhlin, P., Vecchi, R., Miranda, A. I., Kasper-Giebl, A., Maenhaut, W., and Hitzenberger, R.: Source apportionment of particulate matter in Europe: A review of methods and results, J. Aerosol Sci., 39, 827–849, https://doi.org/10.1016/j.jaerosci.2008.05.007, 2008.
Wang, S., Ye, J., Soong, R., Wu, B., Yu, L., Simpson, A. J., and Chan, A. W. H.: Relationship between chemical composition and oxidative potential of secondary organic aerosol from polycyclic aromatic hydrocarbons, Atmos. Chem. Phys., 18, 3987–4003, https://doi.org/10.5194/acp-18-3987-2018, 2018.
Wang, Y., Hopke, P. K., Sun, L., Chalupa, D. C., and Utell, M. J.: Laboratory and field testing of an automated atmospheric particle-bound reactive oxygen species sampling-analysis system, J. Toxicol., 2011, 9, https://doi.org/10.1155/2011/419476, 2011a.
Wang, Y., Kim, H., and Paulson, S.: Hydrogen peroxide generation from α- and β-pinene and toluene secondary organic aerosols, Atmos. Environ., 3149–3156, https://doi.org/10.1016/j.atmosenv.2011.02.060, 2011b.
Weber, S., Uzu, G., Calas, A., Chevrier, F., Besombes, J.-L., Charron, A., Salameh, D., Ježek, I., Močnik, G., and Jaffrezo, J.-L.: An apportionment method for the oxidative potential of atmospheric particulate matter sources: application to a one-year study in Chamonix, France, Atmos. Chem. Phys., 18, 9617–9629, https://doi.org/10.5194/acp-18-9617-2018, 2018.
WHO: Health effects of particulate matter. Policy implications for countries in eastern Europe, Caucasus and central Asia, WHO Regional Office for Europe, Copenhagen, Denmark, 2013a.
WHO: Review of evidence on health effects of air pollution-REVIHAAP Project, Technical Report, WHO Regional Office for Europe, Copenhagen, Denmark, 2013b.
Williams, L. R., Gonzalez, L. A., Peck, J., Trimborn, D., McInnis, J., Farrar, M. R., Moore, K. D., Jayne, J. T., Robinson, W. A., Lewis, D. K., Onasch, T. B., Canagaratna, M. R., Trimborn, A., Timko, M. T., Magoon, G., Deng, R., Tang, D., de la Rosa Blanco, E., Prévôt, A. S. H., Smith, K. A., and Worsnop, D. R.: Characterization of an aerodynamic lens for transmitting particles greater than 1 micrometer in diameter into the Aerodyne aerosol mass spectrometer, Atmos. Meas. Tech., 6, 3271–3280, https://doi.org/10.5194/amt-6-3271-2013, 2013.
Xu, W., Sun, Y., Wang, Q., Zhao, J., Wang, J., Ge, X., Xie, C., Zhou, W., Du, W., Li, J., Fu, P., Wang, Z., Worsnop, D. R., and Coe, H.: Changes in aerosol chemistry from 2014 to 2016 in winter in Beijing: Insights from high resolution aerosol mass spectrometry, J. Geophys. Res.-Atmos., 124, 1132–1147, https://doi.org/10.1029/2018JD029245, 2019.
Yang, A., Jedynska, A., Hellack, B., Kooter, I., Hoek, G., Brunekreef, B., Kuhlbusch, T. A. J., Cassee, F. R., and Janssen, N. A. H.: Measurement of the oxidative potential of PM2.5 and its constituents: The effect of extraction solvent and filter type, Atmos. Environ., 83, 35–42, https://doi.org/10.1016/j.atmosenv.2013.10.049, 2014.
Yi, O., Hong, Y. C., and Kim, H.: Seasonal effect of PM10 concentrations on mortality and morbidity in Seoul, Korea: a temperature-matched case-crossover analysis, Environ. Res., 110, 89–95, https://doi.org/10.1016/j.envres.2009.09.009, 2010.
Yudovich, Y. and Ketris, P. M.: Chlorine in coal: A review, Int. J. Coal Geol., 67, 127–144, 2006.
Zanobetti, A. and Schwartz, J.: The effect of fine and coarse particulate air pollution on mortality: a national analysis, Environ. Health Perspect., 117, 898–903, https://doi.org/10.1289/ehp.0800108, 2009.
Zhou, J. and Dommen, J.: Predominance of secondary organic aerosol to particle-bound reactive oxygen species activity in fine ambient aerosol [Data set], Zenodo, https://doi.org/10.5281/zenodo.3520219, 2019.
Zhou, J., Bruns, E. A., Zotter, P., Stefenelli, G., Prévôt, A. S. H., Baltensperger, U., El-Haddad, I., and Dommen, J.: Development, characterization and first deployment of an improved online reactive oxygen species analyzer, Atmos. Meas. Tech., 11, 65–80, https://doi.org/10.5194/amt-11-65-2018, 2018a.
Zhou, J., Zotter, P., Bruns, E. A., Stefenelli, G., Bhattu, D., Brown, S., Bertrand, A., Marchand, N., Lamkaddam, H., Slowik, J. G., Prévôt, A. S. H., Baltensperger, U., Nussbaumer, T., El-Haddad, I., and Dommen, J.: Particle-bound reactive oxygen species (PB-ROS) emissions and formation pathways in residential wood smoke under different combustion and aging conditions, Atmos. Chem. Phys., 18, 6985–7000, https://doi.org/10.5194/acp-18-6985-2018, 2018b.
Zotter, P., Ciobanu, V. G., Zhang, Y. L., El-Haddad, I., Macchia, M., Daellenbach, K. R., Salazar, G. A., Huang, R.-J., Wacker, L., Hueglin, C., Piazzalunga, A., Fermo, P., Schwikowski, M., Baltensperger, U., Szidat, S., and Prévôt, A. S. H.: Radiocarbon analysis of elemental and organic carbon in Switzerland during winter-smog episodes from 2008 to 2012 – Part 1: Source apportionment and spatial variability, Atmos. Chem. Phys., 14, 13551–13570, https://doi.org/10.5194/acp-14-13551-2014, 2014.
Zotter, P., Herich, H., Gysel, M., El-Haddad, I., Zhang, Y., Močnik, G., Hüglin, C., Baltensperger, U., Szidat, S., and Prévôt, A. S. H.: Evaluation of the absorption Ångström exponents for traffic and wood burning in the Aethalometer-based source apportionment using radiocarbon measurements of ambient aerosol, Atmos. Chem. Phys., 17, 4229–4249, https://doi.org/10.5194/acp-17-4229-2017, 2017.