the Creative Commons Attribution 4.0 License.
the Creative Commons Attribution 4.0 License.
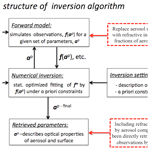
Retrieval of aerosol components directly from satellite and ground-based measurements
Lei Li
Yevgeny Derimian
Gregory L. Schuster
Tatyana Lapyonok
Pavel Litvinov
Fabrice Ducos
David Fuertes
Cheng Chen
Zhengqiang Li
Anton Lopatin
Benjamin Torres
Huizheng Che
This study presents a novel methodology for the remote monitoring of aerosol components over large spatial and temporal domains. The concept is realized within the GRASP (Generalized Retrieval of Aerosol and Surface Properties) algorithm to directly infer aerosol components from the measured radiances. The observed aerosols are assumed to be mixtures of hydrated soluble particles embedded with black carbon, brown carbon, iron oxide, and other (non-absorbing) insoluble inclusions. The complex refractive indices of the dry components are fixed a priori (although the refractive index of the soluble host is allowed to vary with hydration), and the complex refractive indices of the mixture are computed using mixing rules. The volume fractions of these components are derived along with the size distribution and the fraction of spherical particles, as well as the spectral surface reflectance in cases when the satellite data are inverted. The retrieval is implemented as a statistically optimized fit in a continuous space of solutions. This contrasts with most conventional approaches in which the type of aerosol is either associated with a pre-assumed aerosol model that is included in a set of look-up tables, or determined from the analysis of the retrieved aerosol optical parameters (e.g., single scattering albedo, refractive index, among others, provided by the AERONET retrieval algorithm); here, we retrieve the aerosol components explicitly. The approach also bridges directly to the quantities used in global chemical transport models. We first tested the approach with synthetic data to estimate the uncertainty, and then applied it to real ground-based AERONET and spaceborne POLDER/PARASOL observations; thus, the study presents a first attempt to derive aerosol components from satellite observations specifically tied to global chemical transport model quantities. Our results indicate aerosol optical characteristics that are highly consistent with standard products (e.g., R of ∼0.9 for aerosol optical thickness) and demonstrate an ability to separate intrinsic optical properties of fine- and coarse-sized aerosols. We applied our method to POLDER/PARASOL radiances on the global scale and obtained spatial and temporal patterns of the aerosol components that agree well with existing knowledge on aerosol sources and transport features. Finally, we discuss limitations and perspectives of this new technique.
- Article
(11533 KB) -
Supplement
(4042 KB) - BibTeX
- EndNote
Information about atmospheric aerosol chemical composition has a great importance for monitoring and understanding of various aspects of climate and environment. This information can be obtained by laboratory analysis of sampled aerosol. However, in situ measurements require considerable effort and represent only small geographic areas without providing results on a wide spatial and temporal scale. It is known that chemical transport models are able to represent chemical component concentrations with wide spatial and temporal coverage, and this capability has been developed rapidly over the past decade. However, these models can have uncertainties, as they are initialized by gridded emission inventories that presently have substantial uncertainties. For example, the carbon emissions inventories can be uncertain by up to a factor of 2, and this uncertainty is carried forward to the model output (Bond et al., 1998; Cooke et al., 1999; Streets et al., 2001).
Aerosol components are often divided into two categories: strongly light-absorbing components and mainly scattering (non-absorbing) components. The radiative impacts of aerosols at the top of the atmosphere can change from cooling to warming as their optical properties change from highly scattering to highly absorbing (e.g., Haywood and Shine, 1995). There are two kinds of absorbing aerosols that are commonly found in the atmosphere: absorbing carbon and mineral dust that contains iron oxides (Sokolik and Toon, 1999).
Light-absorbing carbon is produced by incomplete combustion, and is an important component of atmospheric aerosol. The complex refractive index of light-absorbing carbon is dependent upon the type of fuel and the combustion conditions (Andreae and Gelencsér, 2006; Schkolnik et al., 2007). The term black carbon (BC) is begrudgingly (and universally) associated with soot carbon in the climate science community, and constitutes the strongest light-absorbing carbon found in the atmosphere (Andreae and Gelencsér, 2006; Bond et al., 2013). Meanwhile, the term brown carbon (BrC) is used to denote organic matter that contains some absorbing organic species, and generally has much greater absorption at near-ultraviolet and blue wavelengths than at red wavelengths (Chen and Bond, 2010; Dinar et al., 2007; Hoffer et al., 2006; Jacobson, 1999; Kanakidou et al., 2005; Kirchstetter et al., 2004; Schnaiter et al., 2006; Sun et al., 2007).
Mineral dust particles can also have a strong spectral signature, with strong absorption at the UV and blue wavelengths when iron oxides are present. Hematite and goethite are different forms of free iron, and they typically appear together (Arimoto et al., 2002; Formenti et al., 2014; Lafon et al., 2006; Shi et al., 2012). The presence of iron in mineral dust particles is known to be important due to its biogeochemical and radiative impacts (Jickells et al., 2005; Mahowald et al., 2005; Sokolik and Toon, 1999). Although the regional distribution of the iron concentration is important for climate studies, it is difficult to obtain as it requires in situ aerosol sampling or the simulation of complex natural processes. In addition, mineral dust particles can be affected by the presence of anthropogenic aerosol particles (e.g., carbonaceous particles produced from biomass burning).
Separating the absorption associated with light-absorbing carbon from the absorption associated with mineral dust (especially iron oxides) is not an obvious task (Derimian et al., 2008), and determination of the relative proportions of BC, BrC, and iron oxides should consider differences in absorption spectral dependence. For instance, Dubovik et al. (2002a) showed that the spectral absorption of carbonaceous aerosol is distinct from that of mineral dust. Schuster et al. (2005) inferred the BC column content from AERONET retrievals by assuming BC is the source of all significant aerosol absorption in the AERONET retrievals. Koven and Fung (2006) retrieved the hematite concentration at dust sites based upon the spectral variability of the imaginary refractive index, whereas Arola et al. (2011) retrieved BrC from AERONET retrievals. Wang et al. (2013) added single scattering albedo as an additional constraint to the approach using the refractive index (Arola et al., 2011; Schuster et al., 2005) and made it feasible to distinguish BC, BrC, and dust simultaneously. Similarly, Li et al. (2015, 2013) investigated the microphysical, optical, and chemical properties of atmospheric aerosols by fitting the AERONET complex refractive indices measured at Beijing and Kanpur. Recently, Schuster et al. (2016a) used the AERONET size distributions and complex refractive indices to retrieve the relative proportion of carbonaceous aerosols (BC and BrC) and free iron minerals (hematite and goethite) in fine- and coarse-mode particles. Nevertheless, all of these methods for retrieving aerosol components rely upon an intermediate retrieval of the refractive index and/or the aerosol absorption optical depth (e.g., one provided by the AERONET operational inversion). Importantly, we also note that these retrievals of aerosol components are only conducted for ground-based remote sensing measurements.
Global satellite observations of aerosol properties provide an opportunity to validate and constrain the model simulations at large spatial and temporal scales (Collins et al., 2001; Liu et al., 2005; Yu et al., 2006, 2004, 2003; J. Zhang et al., 2008). The integration of observations with model results can fill gaps in satellite retrievals and constrain global distributions of aerosol properties to have good agreement with ground-based measurements (Liu et al., 2005; Yu et al., 2006, 2003). In this regard, inverse modeling can be used to reduce large aerosol simulation uncertainties. For instance, several studies (Chen et al., 2018, 2019; Dubovik et al., 2008; Henze et al., 2007) have shown the ability to retrieve global aerosol sources with inverse models that rely upon satellite observations. Therefore, the practice of satellite data fusion into models provides the possibility to improve aerosol simulations of the pre- and post-satellite eras. However, besides the knowledge of the amounts (concentrations) and locations of aerosol emissions, accurate modeling of atmospheric aerosols and their effects also requires information about particle composition. The lack of comprehensive datasets providing multiple constraints for the key parameters employed in models has hindered the improvement of model simulation. Specifically, improving the ability of aerosol component estimation will require the enhancement of remote sensing capabilities to provide aerosol component information on the global scale. The accuracy and specification of the aerosol components as retrieved from satellite observations should respond to the requirements of the aerosol transport models. At the same time, the information content of remote sensing is limited and the main challenge is to identify the aerosol component parameters that can be successfully retrieved by remote sensing measurements, given their sensitivity to the aerosol optical properties and complex refractive index in particular.
The POLDER space instrument (Deschamps et al., 1994; Tanré et al., 2011) is an example of the instruments providing satellite observations that are sensitive to aerosol components. The implementation of multi-wavelength, multi-angle, and polarization measurement capabilities has made it possible to derive particle properties (size, shape, and absorption; Dubovik et al., 2011; Waquet et al., 2013) that are essential for characterizing and estimating aerosol components. This study presents a methodology for the direct retrieval of aerosol components from such measurements. Our methodology is stimulated by the Schuster et al. (2016a, 2009, 2005) works on deriving aerosol component information from ground-based sun/sky photometers of the AERONET network. Here, the idea has evolved and expanded to retrieving the aerosol components from satellite remote sensing observations as well. Namely, we have incorporated an aerosol component module into the Generalized Retrieval of Aerosol and Surface Properties (GRASP) algorithm (Dubovik et al., 2014, 2011). It should be noted that GRASP is a versatile algorithm designed to retrieve an extended set of atmospheric parameters from diverse remote sensing data, including surface, airborne, and satellite observations. Here, we apply GRASP to both ground- and space-based observations, with the primary objective of developing an approach for monitoring aerosol components with extensive spatial and temporal coverage.
The objective of our GRASP/Component approach is to retrieve the aerosol components directly from remote sensing measurements without intermediate retrieval of the complex refractive index, as in previous studies (Arola et al., 2011; Koven and Fung, 2006; Li et al., 2015, 2013; Schuster et al., 2016a, 2009, 2005; Wang et al., 2013). This new approach has a more direct link to the measured radiance field than the “intermediate” approaches; therefore, we expect a reduction in the retrieval uncertainties. The GRASP/Component approach also incorporates an additional constraint on the refractive index spectral variability that is not employed in the conventional retrieval algorithms. Specifically, the spectral variability of aerosol complex refractive index is constrained in the GRASP/Component retrieval by the spectral dependences of the aerosol species used in the algorithm. It is expected that such constraints can improve the retrievals in various situations.
One of the principal difficulties, however, is the identification of an adequate conversion model for linking the refractive index to the aerosol component. An ideal conversion model should cover the entire range of aerosol complex refractive indices and also provide a unique connection between the spectral refractive index and the aerosol component. Therefore, our primary objective focuses on identifying the optimal transformation of chemical and physical aerosol information to optical properties (e.g., refractive index). Once developed, the efficiency of the concept is verified and demonstrated by applying GRASP/Component to ground-based sun/sky photometric measurements, as this type of measurement usually presents a higher sensitivity to aerosol absorption than satellite remote sensing. Finally, the outcome of the GRASP/Component approach is demonstrated with the application of the aerosol component retrieval to multi-angular polarimetric POLDER/PARASOL satellite observations.
It should be noted that the retrieval of the aerosol type has been clearly recognized as an important task by the scientific community and has been addressed in several studies. For example, there are a number of approaches that attempt to identify the type of aerosol via the analysis of optical parameters such as single scattering albedo (SSA), Ångström exponent (AE), absorption AE (AAE), and complex refractive index, etc. Specifically, Russell et al. (2014) relate AERONET- and POLDER-derived optical properties to different aerosol types: urban, dust, marine, biomass burning, and so on. Studies by Chung et al. (2012) and Bahadur et al. (2012) used AERONET optical properties like AE and AAE to separate BC, BrC, and dust into species-specific AAOT (absorption aerosol optical thickness). Schuster et al. (2005, 2009, 2016a), and Li et al. (2015) quantify the relative volume fractions of one or more aerosol species (e.g., BC, BrC, iron oxide, and water) by adjusting the mixture of several components in an aerosol model to fit the AERONET-retrieved refractive indices. However, our new approach differs substantially from all of these methods, as it does not use a retrieval of optical parameters as an intermediate step. Thus, we expect the GRASP/Component approach to provide a stronger link to the radiation field than previous approaches, as well as a fundamentally higher retrieval accuracy.
Moreover, some of the above methods have additional differences and limitations compared with our proposed approach. For example, the Russell et al. (2014) approach is rather qualitative and does not attempt to quantify the relative volume or mass fractions of different species in an aerosol mixture. Chung et al. (2012) and Bahadur et al. (2012) seem to use a technique for separating carbonaceous aerosols from dust that is not fully consistent with the AERONET retrieval assumptions, as discussed by Schuster et al. (2016b).
Also, the look-up table (LUT) approaches employed in most satellite retrievals (Martonchik et al., 1998; Remer et al., 2005; Kahn and Gaitley, 2015; Popp et al., 2016; Hammer et al., 2018; etc.) are designed to search amongst a preselected set of aerosol models (or their mixtures) for a model that provides the best fit to the observations. As the models in a LUT are usually associated with a number of aerosol types (e.g., desert dust, smoke, and urban aerosol, among others), the identification of the model that provides the best fit is often considered as a retrieval of aerosol type/composition. For observations with enhanced sensitivity, such as the Multi-angle Imaging SpectroRadiometer (MISR), a large number of models can be justified in the LUT and the differentiation of the models described by the ensembles of parameters can indeed be rather robust. However, LUT approaches are fundamentally limited to a discrete set of possible solutions, whereas the GRASP/Component approach searches through a continuous space of solutions; thus, the identification of aerosol components with our new methodology is significantly more detailed and elaborate. The proposed approach also bridges directly to the quantities of aerosol compositions used in the global chemical transport models. Specifically, our aerosol component retrievals can satisfy the requirements of chemical transport models (CTM) to constrain their aerosol estimations on a large or global scale. The proposed methodology eliminates two intermediate steps commonly used in comparing satellite results with models. First, the retrieval is carried out directly from measured radiances to components without passing through retrieved optical properties or assumed aerosol models. Second, the methodology allows for matching of CTM components without passing through often ambiguous AOT to mass conversions, e.g., using like mass extinction efficiency. However, we note that the GRASP/Component approach is only possible (1) if there is significant instrument sensitivity to the parameters that are related to aerosol components (i.e., complex refractive index), and (2) if this sensitivity is maintained while other parameters such as the size distribution are adjusted.
GRASP is a highly rigorous and versatile aerosol and surface reflectance retrieval algorithm that is accessible at https://www.grasp-open.com (last acces: 28 October 2019) (Dubovik et al., 2014, 2011). It has been demonstrated that the GRASP algorithm can provide retrieval of a rather complete set of aerosol parameters from multi-angular satellite polarimetry or AERONET-like ground-based observations. The set of retrieved parameters includes size distribution, complex refractive index, information about particle shape, as well as various optical properties such as AOT, single scattering albedo, Ångström exponent, and so on. The values of these parameters are certainly related to aerosol type; however, they do not provide any direct information about possible aerosol type or quantitative indication of various components' presence in the observed aerosol. The identification of the aerosol types present can be obtained by matching the retrieved parameters with preselected classifications based on aerosol components with known properties (e.g., Russell et al., 2014). However, such an approach relies on several intermediate steps based on assumptions of rather different methodological natures; therefore, each step introduces additional uncertainties and even ambiguity (Schuster et al., 2016a; Zhang et al., 2018). The essence of methodological developments in this study is to develop an approach for directly retrieving aerosol components from observations with no intermediate steps and a unique set assumptions.
The goal of the technical developments in this study is to integrate a new conversion model designed to link aerosol components with optical and microphysical characteristics into the standard GRASP inversion procedure. The general logistics is shown in Fig. 1 (modified from Dubovik et al., 2011). The algorithm is divided into several interacting but rather independent modules to enhance its flexibility. The straightforward exchange of limited parameters minimizes the interactions between the modules. The “forward model” and the “numerical inversion” are the two most complex and elaborate modules in the algorithm. The forward model is developed in quite a universal way to quantitatively simulate the measured atmospheric radiation with given surface and aerosol properties. The numerical inversion module (which can be used in various applications, some not even related to atmospheric remote sensing) includes general mathematical operations unrelated to the particular physical nature of the observations. Numerical inversion is implemented as a statistically optimized fitting of observations based upon the multi-term least squares method (LSM), and combines the advantages of a variety of approaches. The module provides transparency and flexibility for developing algorithms that invert passive or active observations to derive several groups of unknown parameters (Dubovik, 2004).
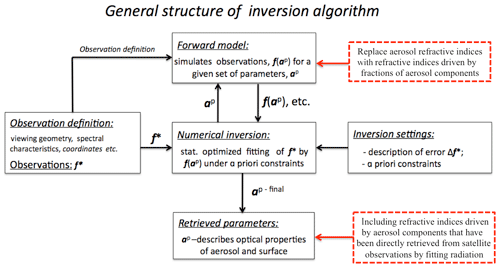
Figure 1The general structure of the GRASP algorithm with the aerosol component conversion model, courtesy of Dubovik et al. (2011). The red dashed frames represent modifications for the component inversion approach. f∗ represents a vector of inverted measurements, aP represents a vector of unknowns at the pth iteration, and f(aP) represents a vector of measurement fit at the pth iteration.
As a consequence of such organization of the algorithm, it can be equally applied (with minimal changes) to invert observations from different satellite sensors or ground-based instruments (Benavent-oltra et al., 2017; Espinosa et al., 2017; Lopatin et al., 2013; Román et al., 2018, 2017; Tsekeri et al., 2017). A full description of the forward model and the numerical inversion algorithm modules can be found in Dubovik et al. (2011). The following sections provide a description of the modifications conducted for realization of the GRASP/Component approach (schematically presented by red dashed frames in Fig. 1).
2.1 Forward model
The formulation of the forward radiative transfer modeling in the presented approach is generally similar to the formulation of the standard GRASP algorithm, where the modeling of the aerosol scattering matrices has been implemented following the ideas described in Dubovik and King (2000) and Dubovik et al. (2006, 2002b). However, we implemented some modifications in the modeling of aerosol single scattering. Namely, the real and imaginary parts of the aerosol complex refractive index are calculated using the fractions of aerosol components and the fixed refractive index of these elements as assumed in the conversion model. Thus, the new component approach uses the same forward model as described in Dubovik et al. (2011), except that aerosol component fractions are iterated in the vector of the retrieved unknowns (instead of the refractive index) and the refractive index is computed a posteriori.
It is worth noting that the aerosol properties in the GRASP algorithm are retrieved simultaneously with the surface reflectance characteristics. The land surface Bidirectional Reflectance Distribution Function (BRDF) in GRASP is described by the kernel-driven Ross–Li model. This model uses a linear combination of three kernels fiso, fvol, and fgeom representing isotropic, volumetric, and geometric optics surface scattering, respectively (Li and Strahler, 1992; Roujean et al., 1992; Wanner et al., 1995). The semiempirical equation by Maignan et al. (2009) is used for the BPDF. The reflective properties of the ocean surface are modeled analogously to earlier POLDER algorithm developments (Deuzé et al., 2001; Herman et al., 2005; Tanré et al., 2011). The Fresnel reflection of the agitated sea surface is taken into account using the Cox and Munk model (Cox and Munk, 1954). The water leaving radiance is nearly isotropic (Voss et al., 2007), and modeling shows that its polarization is negligible (Chami et al., 2001; Chowdhary et al., 2006; Ota et al., 2010). The Fresnel term and the whitecap reflection are taken into account by Lambertian unpolarized reflectance. The whitecap reflectance is driven by the wind speed at the sea surface according to the Koepke model (Koepke, 1984). The seawater reflectance at short wavelengths depends on the properties of oceanic water and can be significant. Thus, in the present model, the wind speed and the magnitude of seawater reflectance at each wavelength are retrieved simultaneously with the atmospheric aerosol properties.
The aerosol and surface characteristics are determined by parameters included in the vector of unknowns and correspondingly they are inferred from observations. Table 1 shows the list of measurements and retrieved parameters from POLDER/PARASOL observations. For the AERONET retrieval the list of parameters is not shown here. However, in principle, it is analogous to POLDER/PARASOL, with the difference that the set of observations is different (i.e., AERONET uses AOT and transmitted total radiances at different wavelengths) and that surface parameters are not retrieved but fixed from the climatology.
2.2 Numerical inversion
The numerical inversion implemented in this study follows the methodology described in the paper of Dubovik et al. (2011). The only difference is that the GRASP/Component approach retrieves the fractions of different aerosol components instead of the spectral dependence of the complex refractive index. Therefore, this section only describes the modifications that are needed to implement the GRASP/Component approach.
GRASP retrieval is designed as a statistically optimized fitting routine and uses multiple a priori constraints. GRASP can implement two different scenarios of satellite retrievals: (i) conventional single-pixel retrieval for processing of satellite images pixel by pixel and (ii) multiple-pixel retrieval for inverting a large group of pixels simultaneously. The multi-pixel approach can be used for POLDER/PARASOL data to improve the consistency of the temporal and spatial variability of retrieved characteristic. The main modifications required for the component approach are related to the definition of a priori constraints. Correspondingly, two types of a priori constraints are reformulated in the component retrieval approach: constraints for single pixel and constraints limiting inter-pixel variability of derived parameters.
2.2.1 Single-pixel observation fitting
For each ith pixel, the retrieval follows a multi-term LSM fitting of joint sets of data combining the observations with a priori constraints defined by the system of equations :
Here, the terms followed by an asterisk represent satellite measurements. For example, denotes a vector of the measurements, fi denotes a vector of the estimations, Δfi denotes a vector of measurement uncertainties, and ai denotes a vector of unknowns in ith pixel. The second expression in Eq. (1) characterizes the a priori smoothness assumptions that constrain the variability of the size distributions and the spectral dependencies of the retrieved surface reflectance parameters. The matrix S includes the coefficients for calculating the mth differences of dV(rj)∕dln r, Frac(i), fiso(λi), fvol(λi), and fgeom(λi). Frac(i) denotes the fraction of the component, dV(rj)∕dln r denotes the values of volume size distribution in Ni size bins ri normalized by Cv and fiso(λi), fvol(λi), fgeom(λi) characterize the property of surface reflectance in the Ross–Li model. The mth differences are numerical equivalents of the mth derivatives. represents a vector of zeros and Δ(Δa) represents a vector of the uncertainties that characterize the deviations of the differences from the zeros. This equation indicates that all of the mth differences are equal to zeros within the uncertainties Δ(Δai). The third expression in Eq. (1) includes the vector of a priori estimates , as well as the vector of the uncertainties ( in a priori estimates of the ith pixel.
The statistically optimized solution of Eq. (1) corresponds to the minimum of the following quadratic form (according to the multi-term LSM):
Following Dubovik et al. (2011), all equations are expressed with weighting matrices W that are defined as (dividing the corresponding covariance matrix C by its first diagonal element ε2); the Lagrange multipliers γa and γΔ are written as and , where , , and represent the first diagonal elements of corresponding covariance matrices Cf, CΔ, and Ca. Thus, in this general formulation, the fractions (Frac(i)) of the aerosol components are presented as unknowns instead of n(λj) and k(λj).
2.2.2 Multiple-pixel observation fitting
In this retrieval regime the fitting for a group of pixels is constrained by the extra a priori limitations on inter-pixel variability of aerosol and/or surface reflectance properties. As the information content of the reflected radiation from a single pixel is sometimes insufficient for a unique retrieval of all unknown parameters, the presented approach can improve the stability of satellite data inversions (Dubovik et al., 2011). The inversion of the multi-pixel observations is a solution for a combined system of equations. For example, a three-pixel system can be defined as follows:
where the subscript “i” () is the pixel index. The total vector of unknowns a is combined by the vectors of unknowns ai of each ith pixel, i.e., . The matrices Sx, Sy, and St include the coefficients for calculating the mth differences of spatial or temporal inter-pixel variability for each retrieved parameter ak that characterizes dV(rj)∕dln r, Frac(i), fiso(λi), fvol(λi), and fgeom(λi). The vectors , and denote vectors of zeros and the vectors Δ(Δxa), Δ(Δya), and Δ(Δta) denote vectors of the uncertainties characterizing the deviations of the differences from the zeros.
The statistically optimized multi-term LSM solution corresponds to the minimum of the following quadratic Ψ(aP):
This is the sum of the corresponding single-pixel forms (first term) and an inter-pixel smoothing component (second term). The smoothness matrix Ωinter in the inter-pixel smoothing term is defined as
Hence, the solution of a multi-pixel system of N pixels is not equivalent to the solution of N independent single pixel systems.
2.2.3 A priori smoothness constraints of fitting
For the framework of deriving aerosol components from the POLDER/GRASP retrieval, the vector ai is composed as
where av, afrac, and asph represent the constituents of the vector a corresponding to dV(ri)∕dln r, Frac(i) and Csph (denotes the fraction of spherical particles). Then ah characterizes the mean altitude of the aerosol layer ha, the element aVc represents the total volume concentration, and av are the logarithms of dV(r)∕dln r, which are normalized by total volume concentration. The three components (abrdf,1, abrdf,2, abrdf,3) are related to the logarithms of the spectrally dependent parameters kiso(λi), kvol(λi), and kgeom(λi) employed in the Ross–Li model. The vector abpdf includes the parameters of the BPDF model. Thus, this work differentiates from Dubovik et al. (2011) by retrieving the volume fractions of the aerosol components (i.e., Frac(i)) instead of the complex refractive index.
There is no evident connection between the retrieved fractions of the aerosol components in each single pixel, so no smoothness constraints are used for afrac. The matrix S for each ith pixel is the same and has the following array structure (Dubovik et al., 2011):
where the corresponding matrices S… have different dimensions and describe differences of different orders. The vectors in Eq. (7) corresponding to afrac, asph, aVc, and ah contain only zeros because no smoothness constraint can be applied to these parameters. The errors Δ(Δa) are assumed to be independent for different components of the vector (Δa)∗, and the smoothness matrix for each ith pixel can be written as
where uses the derivative matrices Si (), Sv, Sbrdf,1, Sbrdf,2, Sbrdf,3, and Sbpdf.
The inter-pixel smoothing term given by Eq. (5) is defined in a very similar way as described by Dubovik et al. (2011); therefore, it is not written here explicitly. Indeed, the spatial and temporal variability of components is very similar to the variability of the refractive index, as both only depend upon the variability of aerosol type.
We note that the above equations apply to the POLDER/GRASP retrievals, but corresponding equations are trivially obtained for the AERONET/GRASP retrievals by excluding parameters describing surface reflectance and aerosol height.
2.3 Model of optical properties of aerosol component
2.3.1 Definition and assumptions
The aerosol refractive index required for the forward calculations (see Fig. 1) is derived by assuming a mixing model and employing fractions of aerosol species; therefore, the retrieval of aerosol components requires the selection of a mixing rule. In our work, we decided to use a simple and widely tested Maxwell Garnett effective medium approximation. Indeed, the choice of the mixing rule is of importance as it can affect the retrieval results. For example, the study of Xie et al. (2014) showed that the Bruggeman approximation was found to be more suitable for the dust case, the Maxwell Garnett for the haze case, and volume average for the clean case. Thus, in order to get an idea about the influence of the mixing rule choice, in our study the retrievals were also produced using the volume-weighted mixing rule. We have not identified a significant influence of the mixing rule choice on the quality of the retrievals in our approach. Moreover, the aerosol optical properties were quite comparable in both cases. The fractions of the elements evidently present some differences due to the differences in the formulation, but were still in reasonable agreement.
The Maxwell Garnett mixing rule has been extensively applied in many studies for the retrieval of aerosol components from ground-based remote sensing measurements (Li et al., 2015, 2013; Schuster et al., 2016a, 2009, 2005; Wang et al., 2013). As Fig. 2 illustrates, the first step in the Maxwell Garnett conversion model is the designation of a “host” and the calculation of the refractive index of the host. In general, the host can be formed by water and soluble inorganic species (e.g., ammonium nitrite, ammonium sulfate, and sea salt). It is well known that inorganic salt particles are mostly hygroscopic and deliquescence in humid air. The phase transition from a solid particle to a saline droplet (host) usually occurs when the relative humidity reaches a specific value, known as the deliquescence point, which is specific to the chemical composition of the aerosol particle (Orr et al., 1958; Tang, 1976; Tang and Munkelwitz, 1993). The refractive indices of hygroscopic aerosols change with the additional amount of water that is absorbed in response to changing relative humidity. These changes in the refractive index, also including the changes in specific density, size, and mass fraction, have been accurately measured as functions of relative humidity (Tang, 1996; Tang and Munkelwitz, 1994, 1991). Schuster et al. (2009) illustrated that the soluble aerosol components (sea salt, ammonium sulfate, ammonium nitrate, and so on) indicate similar refractive indices for similar mixing ratios, even though the dry refractive indices can be quite different. Hence, the aerosol water fraction can be derived from the mixture's real refractive index if the aerosols are known to be one of the common soluble aerosols.
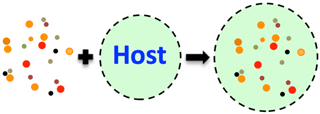
Figure 2Illustrates the general logistics of an effective refractive index calculation using a conversion model that is based upon the Maxwell Garnett effective medium approximation.
In the presented approach, the host is assumed to depend upon the properties and proportions of ammonium nitrate and water (uncertainties due to the selection of ammonium nitrate are evaluated further on). The real refractive index (at the 0.6328 µm wavelength) for a host mixture of ammonium nitrate and water can be expressed as
where X is the weight percent of ammonium nitrate (Tang and Munkelwitz, 1991).
Refractive indices at other wavelengths are spectrally interpolated utilizing measured data (Downing and Williams, 1975; Gosse et al., 1997; Hale and Querry, 1973; Kou et al., 1993; Palmer and Williams, 1974; Tang, 1996; Tang and Munkelwitz, 1991). A detailed description and FORTRAN subroutines for calculating the host complex refractive index is accessible at the GACP (Global Aerosol Climatology Project) website: https://gacp.giss.nasa.gov/data_sets/ (last access: 28 October 2019).
Once the refractive index of the host is determined, the refractive index of the mixture is computed using the Maxwell Garnett equations. The Maxwell Garnett effective medium approximation allows for the computation of the average dielectric function based upon the average electric fields and polarizations of a host matrix with embedded inclusions, and can model insoluble particles suspended in a solution (Bohren and Huffman, 1983; Lesins et al., 2002).
The dielectric functions of aerosols are not typically tabulated in the literature, so they must be computed from the refractive index. Once the dielectric functions are known for the host and its constituents, the Maxwell Garnett dielectric function for a mixture can be calculated. For example, for two types of inclusions in a host, the dielectric function of the mixture can be expressed as follows (Schuster et al., 2005):
where ϵm, ϵ1, and ϵ2 are the complex dielectric functions of the host matrix and inclusions, and f1 and f2 are the volume fractions of the inclusions. If we use the case of f2=0, the corresponding complex refractive index of the mixture can be obtained by Eqs. (11) and (12):
where εr and εi denote the real and imaginary components of the mixture dielectric function, εMG.
The selected refractive indices of inclusions in the Maxwell Garnett effective medium approximation model in this study are shown in Fig. 3. Figure 3 also illustrates the assumption regarding the size-resolved aerosol components presented as an additional constraint. Table 2 shows the description of aerosol components and the complex refractive indices at 0.440 and 0.865 µm of each component employed in the GRASP/Component approach, as well as those used in the uncertainty tests. Our selection of aerosol elements and the size-resolved component results from the examination of a series of sensitivity tests and the stability of the inversion results. The size-resolved component formulation was chosen because a similarity in spectral signatures of some aerosol species meant that they were difficult to distinguish in the observational configuration considered in this study. For instance, brown carbon (BrC) and iron oxides (hematite and goethite) have a similar tendency in spectral absorption; that is, they increase the imaginary refractive index towards ultraviolet wavelengths (Chen and Cahan, 1981; Chen and Bond, 2010; Kerker et al., 1979; Schuster et al., 2016a). At the same time, it is known that carbonaceous absorbing aerosol particles dominate in the fine mode, and mineral dust absorption dominates in the coarse mode. Hence, black carbon (BC) and brown carbon (BrC) are assumed to be the only absorbing insoluble species in the fine mode, and iron oxides are assumed to be the only absorbing insoluble species in the coarse mode. In addition, the fine mode includes non-absorbing insoluble species (FNAI) that represent fine dust or non-absorbing organic carbon (OC), non-absorbing soluble species (FNAS) that represent anthropogenic salts, and aerosol water content (FAWC). The coarse mode includes absorbing insoluble species (CAI), which are mainly iron oxides, but can also include all other absorbing elements. The coarse mode also includes non-absorbing insoluble (CNAI) species that mainly represent the bulk dust material, but can also be non-absorbing insoluble organic carbon particles, non-absorbing soluble species (CNAS) that represent anthropogenic or natural salts (e.g., sea salts), and aerosol water content (CAWC). It should be clarified that the refractive index of only one element is used for each species; however, our tests confirmed that some elements are indistinguishable from an optical point of view, at least for the measurement configurations expected in the scope of the presented algorithm applications. Thus, several of the assumed species in the mixing model elements can be associated with different elements. It should also be mentioned that the maximal fractions for BC and CAI (mainly representing iron oxides) are limited in the algorithm due to the possible range of complex refractive indices in the precomputed kernels of aerosol optical characteristics. That is, the volume fractions of these two highly absorbing species are limited to 10 % for BC and 3 % for CAI in the algorithm. The limitation criteria are based on previous in situ studies (Ganor and Foner, 1996; Guieu et al., 2002; Lafon et al., 2004, 2006; Alfaro et al., 2004; Wagner et al., 2012; Formenti et al., 2014) demonstrating that the volume fraction of free iron in dust particles approximately accounts for 1.4 %–3.25 % (2.8 %–6.5 % by mass as the density is 4.28 g cm−3 for goethite, 5.25 g cm−3 for hematite, and 2.65 g cm−3 for illite, kaolinite, quartz, and calcite; Formenti et al., 2014). The fraction of BC in atmospheric aerosol was generally reported as not exceeding 10 % (Bond et al., 2013). Analysis of our results showed that the introduced maximal values were never reached in the inversion procedure; therefore, the presented limitations should not introduce artificially limited concentrations. It is also notable that the retrievals of aerosol components derived from AERONET measurements by Schuster et al. (2016a) demonstrated that the volume fraction of free iron remains relatively constant in West Africa throughout the year (1.4 %–1.7 %), and the volume fraction of black carbon reaches a peak of 1.0 % for the fine mode during the West African biomass burning season and a peak of 3.0 % for the fine mode in the southern Africa biomass burning season.
Table 2Description of aerosol components and complex refractive indices at 0.440 and 0.865 µm employed in the GRASP components retrieval approach, as well as those used in the uncertainty tests.
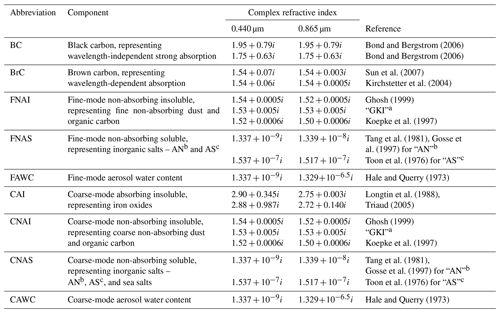
a “GKI” denotes dust composed of a mixture of quartz (Ghosh, 1999), kaolinite (Sokolik and Toon, 1999) and illite (Sokolik and Toon, 1999) with the proportions recalculated from Journet et al. (2014). b “AN” denotes ammonium nitrate, which can be used to create a host in aerosols. c “AS” denotes ammonium sulfate, which is an alternative species for host estimation in aerosols.
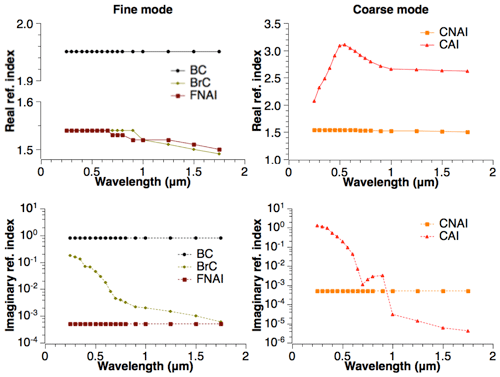
Figure 3The refractive indices of assumed aerosol components embedded in the host of the size-dependent Maxwell Garnett conversion model. The parameters of BC refer to Bond and Bergstrom (2006). The parameters of BrC refer to Sun et al. (2007) and Schuster et al. (2016a). The parameters of fine non-absorbing insoluble (FNAI) and coarse non-absorbing insoluble (CNAI) refer to Ghosh (1999). FNAI represents dust and OC in fine-mode particles, whereas CNAI represents dust in coarse-mode particles. The parameters of coarse absorbing insoluble (CAI) refer to Longtin et al. (1988) representing hematite.
2.3.2 Sensitivity tests
Using the above modifications to the GRASP algorithm described in Dubovik et al. (2011), the aerosol component retrieval approach was tested for the inversion of ground-based AERONET and POLDER/PARASOL satellite observations. For verification of the proposed concept and the algorithm performance, a series of sensitivity tests were conducted using synthetic data. The overall strategy of these tests, as well as of the analysis of the obtained results in the following, was, first, to demonstrate and verify that the GRASP/Component approach matches the standard GRASP retrievals in terms of AOT, AE, and SSA under the predefined component divisions; then, second, if this match is achieved, to demonstrate the possibility of a unique distinction of components in sensitivity tests and to verify agreement with available independent data of the retrieved results.
A comprehensive series of sensitivity tests were mainly conducted using the POLDER/PARASOL observations because, unlike the AERONET retrievals, sensitivity of POLDER/PARASOL observations to the aerosol complex refractive index has not been systematically explored. Thus, first, the POLDER/PARASOL radiances and polarization measurements were simulated using forward calculations. Then, the synthetic measurements were inverted using the GRASP algorithm with the size-dependent aerosol component approach and the Maxwell Garnett mixing model. The tests were conducted for a range of aerosol component fractions for the species described above and a variety of observational configurations such as spectral channels, viewing geometry an so on. Figure 4 presents an example of the assumed and retrieved fractions of aerosol species in the fine and coarse modes. The statistics of the sensitivity test results are presented in Table 3, where we compare assumed and retrieved aerosol parameters (the fractions of aerosol elements, aerosol optical thickness (AOT), single scattering albedo (SSA), and the complex refractive index at 675 nm). The results for other wavelengths are very similar to those presented at 675 nm. In all of the tests conducted, the results demonstrated that, in the framework of the designed model, the use of the size-dependent Maxwell Garnett conversion model allows the algorithm to distinguish amongst the assumed aerosol species, including ammonium nitrate and water in the host.
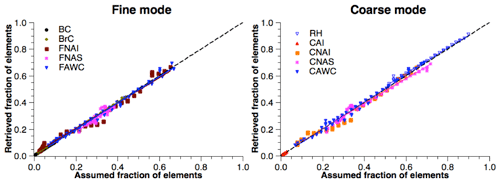
Figure 4Assumed and retrieved fractions of aerosol species resulting from the sensitivity tests of the GRASP/Component retrieval using the Maxwell Garnett mixing model.
Table 3List of statistics for parameters between the assumed and retrieved values in the sensitivity tests of the GRASP component retrieval using the Maxwell Garnett mixing model. The values of the slope (A), intercept (B), correlation coefficient (R), root-mean-square error (RMSE), mean absolute error (MAE), mean relative error (MRE), and standard error deviation (SD) are presented for aerosol components, aerosol optical thickness (AOT), single scattering albedo (SSA), and real (n) and imaginary (k) parts of the complex refractive index in the fine mode (FM) and coarse mode (CM) at 675 nm.
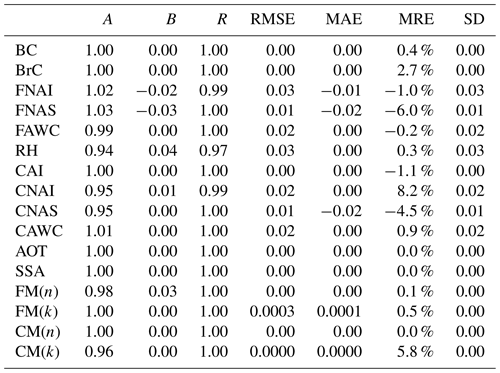
2.3.3 Uncertainty assessment
An important range of variability exists in the refractive indices of the aerosol species reported in the literature. Different assumptions regarding the refractive index of an aerosol species can result in different retrieved fractions of the species proposed in this study. To evaluate a possible range of the retrieved fractions due to uncertain knowledge of the refractive indexes and difficulty selecting one representative value, a series of supplementary calculations were conducted using a range of refractive indices found in the literature. Figure 5 shows the refractive indices employed in the algorithm and those used for the assessment of the uncertainties in the retrieved aerosol fractions. The uncertainty is defined (as a percentage) as the retrieved fraction minus the assumed fraction and is divided by the assumed fraction. The tests are conducted as follows: first, synthetic measurements are created by forward calculations while employing the complex refractive index assumed in the algorithm; second, another complex refractive index is used in the inversion procedure while retrieving the fractions of the aerosol species from the synthetic measurements. Thus, the comparison of the assumed aerosol species fraction in the forward calculations and the retrieved aerosol species fraction in the inversion procedure provides an error assessment due to possible variability of their complex refractive index. The calculations were conducted for all aerosol species that were assumed be embedded in the host of the Maxwell Garnett effective medium approximation. In addition, the tests were also conducted for different fractions of the elements and for different AOT values, reflecting the sensitivity of the retrievals to varying aerosol loading.
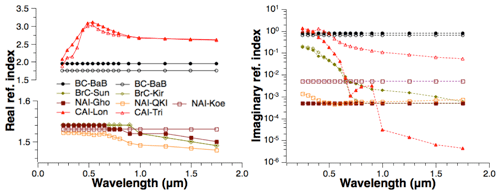
Figure 5Complex refractive index of several aerosol species (BC, BrC, CAI, and NAI) in the host. The values with filled symbols are used in the presented size-dependent Maxwell Garnett conversion model. The values with open symbols are used to replace the corresponding values to test the uncertainties in the aerosol component retrievals. “BaB” represents Bond and Bergstrom (2006); “Sun” represents Sun et al. (2007); “Kir” represents Kirchstetter et al. (2004); “Gho” represents Ghosh (1999); “QKI” represents dust composed of a mixture of quartz (Ghosh, 1999), kaolinite (Sokolik and Toon, 1999), and illite (Sokolik and Toon, 1999), with the proportions of 48 %, 26 %, and 26 %, respectively (Journet et al., 2014); “Koe” represents Koepke et al. (1997); “Lon” represents Longtin et al. (1988); and “Tri” represents Triaud (2005).
An extensive review of BC refractive indices can be found in Bond and Bergstrom (2006), where the recommended imaginary part ranges from 0.63 to 0.79 at visible wavelengths. The spectrally invariant value of 0.79 was adopted in these previous studies (Bond et al., 2013; Bond and Bergstrom, 2006). Based on this literature, we use the spectrally invariant complex refractive index for BC of 1.95+0.79i for our current aerosol component retrievals. We then estimate the uncertainty in the retrieved BC fraction using a BC refractive index of 1.75+0.63i. The results of the uncertainty test for retrieving BC from POLDER/PARASOL are presented in Fig. 6a. As can be seen, the uncertainty strongly depends on the BC fraction and increases when the BC fractions are low. We note that the uncertainty can be large (over 100 %) when the BC fraction is below 0.01 and aerosol loading is weak. However, the uncertainty decreases rapidly and can be 50 % or less for moderate and high aerosol loading (AOT at 440 nm equal to or more than 0.4) and when the BC fraction is above 0.01. Therefore, the estimates should be quite reasonable in cases of large pollution loading.
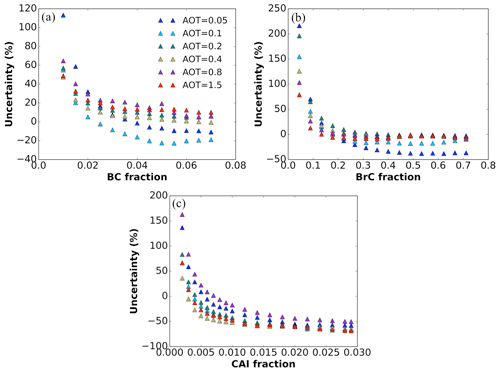
Figure 6Uncertainty in absorbing species retrievals from POLDER/PARASOL attributed to the refractive index variability; uncertainties in the (a) BC, (b) BrC, and (c) CAI fractions.
The refractive index of BrC reported in the literature is variable. For the forward model we employed the BrC refractive index derived from Sun et al. (2007), which was used to retrieve aerosol components from ground-based remote sensing measurements (e.g., Arola et al., 2011; Schuster et al., 2016a). The BrC refractive index, representing carbonaceous particles with light absorption in the blue and ultraviolet spectral regions emitted from biomass combustion (Kirchstetter et al., 2004), was used for the uncertainty estimate. The tests show (Fig. 6b) that the uncertainty in the BrC fraction is more than 100 % when the fractions are below 0.1 and decreases to below 100 % when the BrC fractions are above 0.1 and the aerosol loading is elevated. Note that the uncertainty in the BrC fraction is within 50 % when the fractions are above 0.1, even for very low aerosol loading (AOT =0.05).
Hematite and goethite are the dominant absorbers in the coarse-mode particles. The hematite refractive index was selected for the employed aerosol component mixing model. The literature shows that the hematite refractive indices can also exhibit quite a large range of variability (e.g., see Fig. 5b). Thus, Fig. 6c shows the uncertainties in the retrieved CAI fraction from POLDER/PARASOL associated with the hematite refractive given by Longtin et al. (1988) in the forward calculations and of Triaud (2005) in the inversion. Except the very low fraction of CAI (below 0.005), the uncertainty in CAI fraction is within 50 %.
The insoluble organic carbon and the non-absorbing dust present very similar spectral dependence of the complex refractive index (Ghosh, 1999; Koepke et al., 1997), and it is practically impossible to distinguish between these species in the measurement configurations and the retrieval approach considered in this work. Thus, the non-absorbing insoluble organic carbon and non-absorbing mineral dust are expressed by a non-absorbing insoluble species (NAI). The refractive index for the NAI in the presented algorithm was taken as the dust refractive index in Ghosh (1999). The uncertainty tests for the NAI fraction retrievals are presented for the fine and coarse fractions by replacing the dust refractive index with the refractive indices of dust composed of quartz (Ghosh, 1999), kaolinite (Sokolik and Toon, 1999), and illite (Sokolik and Toon, 1999), with the proportions of 48 %, 26 %, and 26 %, respectively (the proportions are recalculated from Journet et al., 2014; see the legend of Fig. 5 in this paper). The estimated uncertainties for the fine and coarse NAI fractions (FNAI and CNAI) decrease significantly (from 100 % to below 50 %, and vary about the zero) when the NAI fraction is above 0.1 (see Fig. 7a, b).
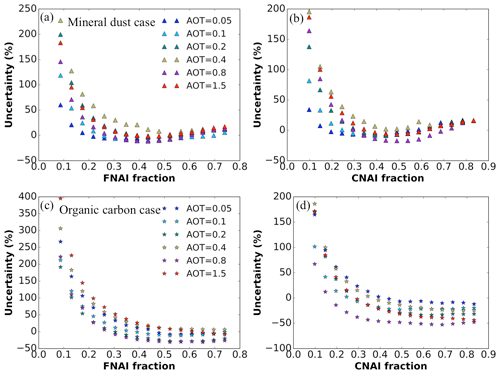
Figure 7Uncertainty in the non-absorbing insoluble particles fraction in the fine (FNAI) and coarse (CNAI) modes attributed to the refractive index variability: (a, b) for mineral dust and (c, d) organic carbon.
The non-absorbing insoluble component can represent not only non-absorbing dust, but also non-absorbing organic carbon, as previously mentioned. Thus, an additional test was conducted in which the dust refractive index (Ghosh, 1999) used in the forward calculations was replaced at the retrievals stage by the refractive index of insoluble organic carbon from Koepke et al. (1997). The corresponding results of the retrieved (in this case) fine and coarse fractions of NAI for POLDER/PARASOL observations are presented in Fig. 7c and d. The variability for each fraction indicates that the choice of the NAI refractive index can cause an uncertainty in the retrieved NAI fraction of less than 100 % for FNAI and less than 50 % for CNAI when the fractions are above 0.1.
Figure 8 shows the uncertainties for the host species fraction (FNAS, CNAS, FAWC, and CAWC), which are attributed to the differences between the refractive indices and hygroscopic properties of ammonium nitrate and ammonium sulfate. The uncertainties are small for FNAS, CNAS, FAWC, and CAWC, particularly when the fractions are more than 0.2.
3.1 Component retrieval from AERONET
AERONET provides measurements that are among the most sensitive data to the aerosol refractive index. In addition, the AOT in AERONET (used in Fig. 9) is the result of direct measurements and not retrieved as in the case of satellite observations. Therefore, the GRASP aerosol component retrieval concept was first tested with real AERONET data to check if the retrieved optical characteristics were consistent with the results of the standard AERONET product.
Figure 9 presents the AERONET measured AOT and Ångström exponent (870 nm/440 nm) and retrieved single scattering albedo (SSA) at 675 nm versus those retrieved using the GRASP/Component approach. Namely, the operational AERONET product is presented versus the derived values from GRASP/Component for three sites on the African continent: Banizoumbou (data for April 2007), Skukuza (data for September 2007), and Ilorin (data for January 2007), which represent (according to the sites location and the considered seasons) a dust, a biomass burning, and a mixture of dust and biomass burning, respectively. It can be seen that the aerosol optical properties are reproduced very well by the GRASP/Component approach not only for the recalculated AOT and its spectral behavior, but also for the SSA. The mean difference in the AOT is about 0.01, which is on the level of the AERONET calibration uncertainty; the difference in the SSA is also well within the expected retrieval uncertainty of 0.03 (Dubovik et al., 2002a). There are no biases observed and the correlation coefficient is nearly 1.0 for AOT and the Ångström exponent.
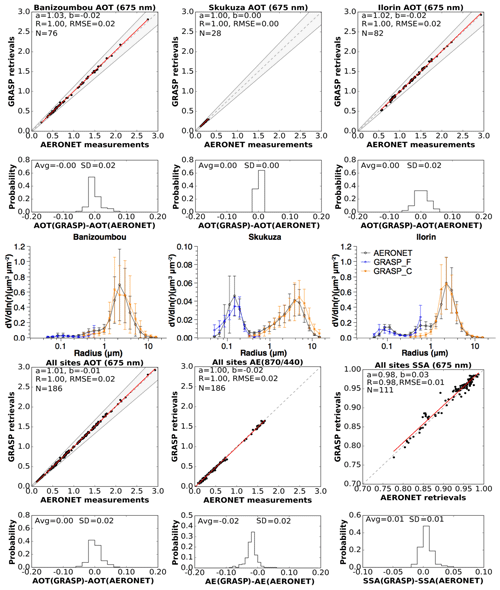
Figure 9The intercomparison of aerosol optical properties derived from sun/sky photometer measurements using the GRASP/Component approach with the corresponding values of the AERONET operational product. The data presented for the Banizoumbou site in April 2007 represent mineral dust aerosol, the data for the Skukuza site in September 2007 represent the biomass burning aerosol, and the data for the Ilorin site in January 2007 represent the mixture of dust and biomass burning.
It should be mentioned here that the fine mode in the presented retrievals is described by 10 bins and the coarse mode is described by 15 bins, which is in contrast to the 22 bins that are used for the entire size distribution in the standard AERONET algorithm (Fig. 9). The component retrieval has the ability to infer different refractive indices for the fine and coarse modes. This is a significant improvement over the standard AERONET and POLDER/PARASOL algorithms, which allow refractive indices to vary with wavelength but not with size. Indeed, the use of fixed spectral dependences of the refractive indices in the GRASP/Component algorithm provides an additional constraint and reduces the number of unknown parameters. Thus, this approach makes the inversion more stable. Nevertheless, the intercomparison of retrievals using the component approach shows full consistency with the operational AERONET product, mainly thanks to an additional physical constraint on the spectral dependence of the refractive index.
In addition to the better characterization of aerosol fine and coarse modes and preserving consistency in the retrievals of optical characteristics, the new approach can also provide insights on aerosol components. For example, Fig. 10 shows the volume fractions of aerosol species retrieved in the fine and coarse modes (panels a–f), and the fractions of the species in the total volume (panels g–i) for the abovementioned African sites. The Banizoumbou site is located near Niamey (Niger) north of the Sahel, the Ilorin site (Nigeria) is located in the Sahel, and the Skukuza site is located in southern Africa. The retrieved aerosol components for Banizoumbou and Ilorin present similarity in terms of abundant dust aerosol. However, contributions of BrC and BC are strong in Ilorin, and the contribution of coarse absorbing insoluble aerosol fraction is strong in Banizoumbou. The southern African site shows different behavior: a strong contribution of the coarse-mode soluble and of the fine-mode non-absorbing insoluble aerosol fractions attributed to water soluble organic carbon and water insoluble organic carbon in the biomass burning region, and a contribution of BC that is almost twice as important as that at the Sahel site . The BrC contribution, however, is about 2 times smaller in southern Africa than that at the Sahel site, which is consistent with AERONET's low spectral dependence for the imaginary index. The SSA is highest in Banizoumbou (0.97 at 675 nm) and is lowest in Skukuza (0.82) because dust and biomass burning aerosols dominate in these two regions, respectively.
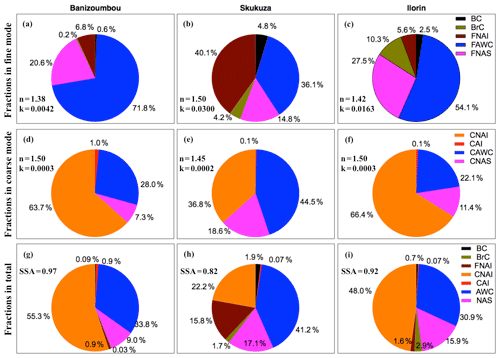
Figure 10Examples of aerosol component retrievals derived from AERONET sun/sky photometer measurements using the GRASP/Component approach. (a, d, g) The mineral dust case at the Banizoumbou site (8 April 2007); (b, e, h) the biomass burning case at the Skukuza site (2 September 2007); and (c, f, i) the mixture of dust and biomass burning at the Ilorin site (25 January 2007). The values of the real part (n) and the imaginary part (k) of the complex refractive index retrieved at 675 nm for the fine and coarse modes, and of SSA at 675 nm derived for an ensemble of aerosol are also indicated in the panels.
3.2 POLDER/PARASOL satellite observations
After testing the aerosol component retrieval approach with the AERONET measurements, the algorithm was applied to the POLDER/PARASOL satellite observations. Figures 11 and 12 as well as Table 4 summarize an intercomparison of the aerosol optical characteristics derived by the GRASP/Component approach applied for POLDER/PARASOL and those of the operational AERONET product. The intercomparison is presented for six sites in Africa and the Middle East (Fig. 11) and for all available AERONET data (Fig. 12) representing the performance for different aerosol types and on the global scale. Because of a limited sensitivity to absorption when the aerosol loading is low, the SSA product is filtered for AOT at 0.440 µm equal to or higher than 0.4 (Dubovik et al., 2002a; Dubovik and King, 2000). The SSA and the Ångström exponent in Fig. 11 are presented for all six sites combined because the dynamic range of the values for each single site is limited by a dominant aerosol type. It should also be noted that in the intercomparison on the global scale (Fig. 12) the correlation for the Ångström exponent was notably better for higher AOT (R of ∼0.6 for all AOTs and of ∼0.8 for AOT equal to or more than 0.2). Better SSA and Ångström exponent retrievals for higher AOTs have, however, also been reported for standard retrievals and other satellite products (de Leeuw et al., 2015; Popp et al., 2016). Nevertheless, the good agreements with respect to AOT (R is generally of ∼0.9 or better), and for the Ångström exponent and SSA (R of ∼0.70–0.80) show that the inversion of POLDER/PARASOL satellite measurements using the component approach is consistent with the ground-based AERONET reference in terms of aerosol optical properties. Analysis of the per site aerosol optical properties retrievals for different aerosol types (Fig. 11) also does not reveal any evident problem. In addition, as illustrated in Fig. 13, the residuals of the GRASP/Component approaches (MG and VW mixing rules) are almost the same as those of standard GRASP. Indeed, the GRASP/Component approaches produce almost the same average residual (2.4±0.9 % for MG and 2.4±1.0 % for VW) as that of the standard GRASP algorithm (2.3±0.9 %), whereas the maximum residual for GRASP/Component (5.0 % for MG and 5.7 % for VW) is smaller than that for standard GRASP (6.6 %); (“±” denotes standard deviation).
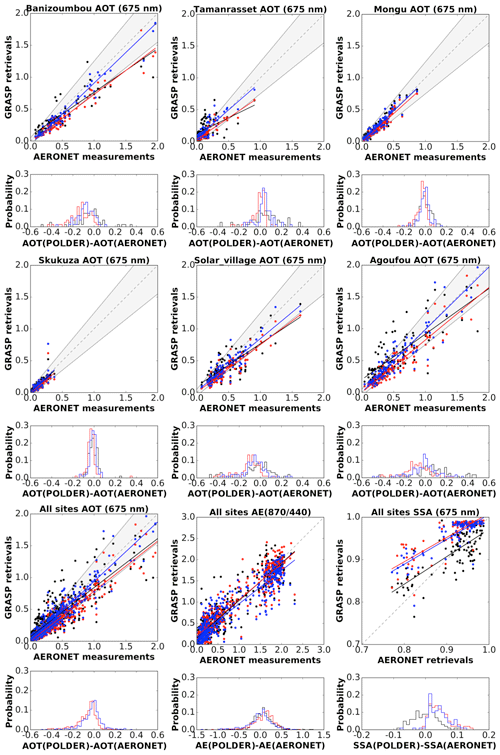
Figure 11Intercomparison of the aerosol optical properties retrieved from POLDER/PARASOL and provided by the AERONET operational product from six AERONET sites located in Africa and the Middle East during the period from 2006 to 2008. Red represents the Maxwell Garnett (MG) mixing model, blue represents the volume-weighted (VW) mixing model, and black represents the standard (ST) GRASP/PARASOL product that does not employ the aerosol component retrievals.
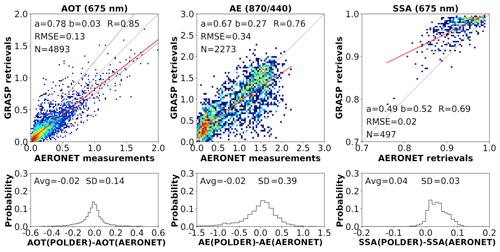
Figure 12Intercomparison of aerosol optical properties retrieved using the POLDER/PARASOL component (MG mixing model) approach and the corresponding operational AERONET products from all globally available sites in 2008.
Table 4The statistics of aerosol parameters in Fig. 10: number of measurements (N), slope (A), intercept (B), correlation coefficient (R), root-mean-square error (RMSE), mean absolute error (MAE), and standard error deviation (SD). The abbreviations used in the table are as follows: GRASP approach – GA, Maxwell Garnett mixing model – MG, volume-weighted mixing model – VW; standard GRASP/PARASOL retrievals without aerosol component mixing model (ST).
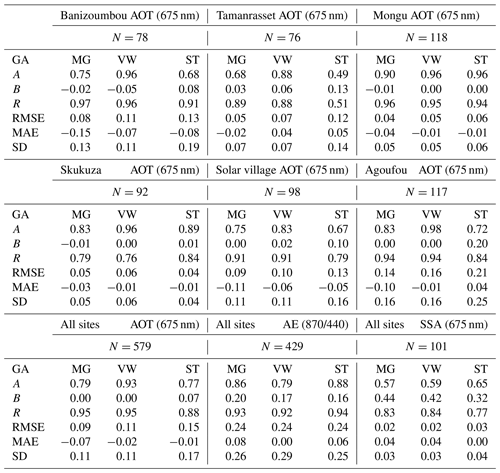
The selected mixing model influence on the retrievals is assessed by a comparison of the results from the Maxwell Garnett effective medium approximation with performance using a simplified volume-weighted (VW) aerosol mixture. Definition of the species constituting the VW mixing model is quite similar to the Maxwell Garnett method, it employs BC, BrC in the fine-mode, absorbing insoluble in the coarse-mode, non-absorbing insoluble, and aerosol water content in both the fine and coarse modes. The tests were conducted in the same manner as for the Maxwell Garnett effective medium approximation. The sensitivity tests revealed that the implementation of the volume-weighted mixing rule yields stable results and that this model can indeed be used for the retrievals. Moreover, the VW model can be preferable in some applications due to its simplicity. Figure 11 and Table 4 illustrate that the GRASP/Component retrievals using the MG and VW mixing models reproduce the aerosol optical properties in a similar fashion. The intercomparison of the standard GRASP/PARASOL retrievals (without the retrieval of the aerosol component) with AERONET is also presented in Fig. 11 and Table 4. It should noted that the results obtained (in the three cases shown) for the AOT and Ångström exponent (AE) from PARASOL using the component approach display comparable and even better correlations with AERONET than the standard GRASP/PARASOL retrieval that derives the spectral refractive indices directly instead of fractions of the aerosol species with fixed refractive indices. This can be considered as confirmation that the constraints adapted in the component approach provide adequate, realistic, and practically useful additional constraints that help to improve satellite retrievals. At the same time, it can be seen that the SSA obtained by standard GRASP/PARASOL correlates better with AERONET than those obtained by the GRASP/PARASOL component approach. Specifically, this GRASP/PARASOL component shows systematically lower absorption than the standard GRASP/PARASOL retrieval. This can be explained by the fact that the complex refractive index in GRASP/Component are constrained by the information (on both magnitudes and spectral tendencies) adapted from the literature, whereas for standard GRASP/PARASOL and operational AERONET products there are no such constraints. As discussed by Dubovik and King (2000) and Dubovik et al. (2011), the standard retrieval approach uses only smoothness constraints on the spectral variability of the complex refractive index. In these regards, tests by Dubovik et al. (2000) demonstrated that in the presence of measurement noise the standard approach tends to generate retrievals with higher values of absorption in a situation with lower aerosol loading (lower AOT). This occurs due to the increased spread of SSAs in situations with a lower aerosol signal. Indeed, due to physical constraints SSA cannot be higher than one; as a result, the appearance of any spread caused by the presence of noise generates lower SSA bias. Such bias has often been discussed by the in situ and modeling community as a rather unfortunate feature of AERONET retrievals (e.g., Schmeisser et al., 2017). Therefore, the slightly higher SSA in the GRASP/Component case can rather be considered a positive effect of the additional constraint. Additional focused analysis should probably be carried out in future, but it can be expected that the slightly higher values in the GRASP/Component case may also be closer on average to what is expected from models because it is tied to similar physical assumptions.
We processed the POLDER/PARASOL observations globally using the aerosol component retrieval algorithm. The results of this processing present the first attempt to assess the measurement-based global distribution and seasonal variability of aerosol components. The data were processed for the year 2008, which provides a notable variety of different aerosol types, including volcanic aerosols from a Hawaiian eruption.
The results are further presented as seasonal means. It should be mentioned, however, that any interpretation of the statistical values should also take the number of available observations into account. Therefore, it is worth presenting the global maps of the number of available cloud-free pixels. Figure 14 shows that the number of the cloud-free pixels over land is significantly higher than over ocean, which can produce a difference in the mean values and create some artificial spatial patterns. In addition, the sensitivity tests and past experience regarding the treatment of remote sensing observations show that the accuracy of the retrievals is low and the sensitivity to the absorbing aerosol and the refractive index variability is particularly limited when the aerosol loading is low. Therefore, it is also worth presenting the global maps of the aerosol optical thickness (Fig. 15), prior to analyzing the aerosol component retrievals. It should also be outlined that despite the fact that the fractions of the elements are the initial retrieval parameters, direct interpretation of the maps of these fractions can be confusing as they do not always correspond to a significant aerosol concentration. For instance, a large fraction of an element retrieved for a size mode where the aerosol volume concentration is very low can have no significant meaning as it does not contribute to the optical signal. Therefore, the columnar volume concentrations of the retrieved elements, and not the fractions, will be presented further. Figures 16 to 21 show the seasonal variabilities of the retrieved columnar aerosol volume concentrations (in mm3 m−2), which denotes the volume concentration in the total atmospheric column with unit surface area) for different aerosol species.
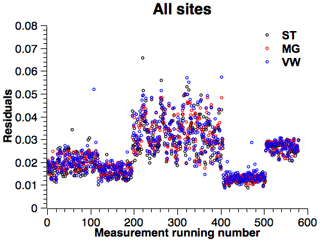
Figure 13Intercomparison of retrieval residuals among different GRASP approaches. Red represents the Maxwell Garnett (MG) mixing model; blue represents the volume-weighted (VW) mixing model; and black represents the standard (ST) GRASP/PARASOL product that does not employ the aerosol component retrievals.
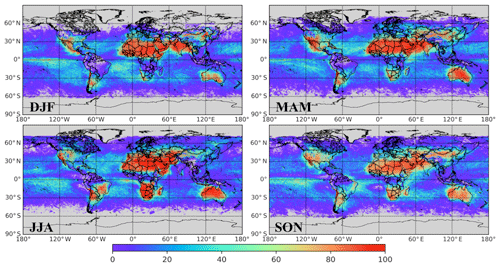
Figure 14Seasonal variability of the number of pixels at a 0.1∘ resolution observed by POLDER/PARASOL satellite over the globe in 2008.
4.1 Black carbon
The retrieved aerosol component shows patterns of biomass burning in the Sahel and southern African regions, expressed by elevated concentrations of BC (Fig. 16). The derived BC concentrations show a pronounced seasonal and spatial variability. The largest concentrations can be observed over the African continent, with Asia, namely India and China, being another noticeable region. The most intensive BC emissions appear during DJF, which is comprised of contributions from the Sahel region, India, and China. Somewhat lower concentrations during SON and JJA are attributed to biomass burning regions in southern Africa. A global minimum of the BC concentrations is observed during MAM. The spatial and seasonal patterns of BC obtained are consistent with the prior knowledge that DJF is a season of intense agricultural burning across the sub-Sahelian region of Africa. BC generated from such agricultural burning can extend for thousands of kilometers from east to west across the continent, as can be seen in Fig. 16. The BC concentration in northern Africa mainly appears over land near the west coast, especially from Senegal south to Gabon at the Equator, and over the Gulf of Guinea, which is attributed to the biomass burning during DJF (e.g., Haywood et al., 2008). The BC observed over the ocean is generally transported from biomass burning areas by prevailing trade winds. The retrievals show that the BC concentration in India and China, which can be rather attributed to anthropogenic activity, is highest during DJF. This result is consistent with a previous study by Li et al. (2015) that also found a maximal BC mass concentration during DJF. The work by Li et al. (2015) is based on the retrieval of aerosol components from AERONET measurements at sites in Beijing and Kanpur and presents a 12-year climatology for the period from 2002 to 2013.
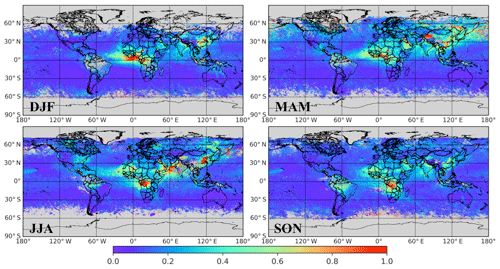
Figure 15Seasonal variability of the AOT at 565 nm in 2008 as retrieved by the GRASP/Component algorithm from POLDER/PARASOL satellite observations.
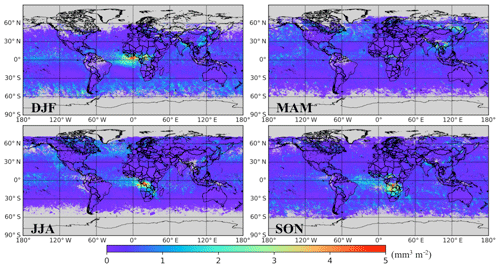
Figure 16Seasonal variability of the BC column volume concentration (mm3 m−2) over the globe in 2008 as retrieved by the GRASP/Component algorithm from POLDER/PARASOL satellite observations.
During JJA and SON, elevated BC concentrations are mostly observed over southern Africa, which is in line with the known African monsoon cycle. The variations of the retrieved BC are consistent with the biomass burning activity progressing from north to south Africa, starting from June, peaking in July–August, and then decreasing in intensity until late October with the end of the dry season (Cahoon et al., 1992; Liousse et al., 1996; Maenhaut et al., 1996; Swap et al., 1996).
It should be noted, however, that the sensitivity to the absorption and, therefore, to the BC signal is limited when the AOT is low. In addition, for very low AOT values the aerosol volume concentrations are also low; thus, the retrieved fractions of the aerosol species are more uncertain. Very low aerosol loading is typical for observations over ocean (Fig. 15); therefore, the appearance of some BC concentrations over ocean should be interpreted with caution.
4.2 Brown carbon
Similar to BC, the observed patterns of BrC (Fig. 17) show seasonal variations, primarily associated with biomass burning in Africa and the contribution of Asian anthropogenic activities. A closer comparison of BrC and BC concentrations reveals, however, that their maximal concentrations are not always co-located. This observation reflects the fact that fresh biomass burning aerosols have a higher BC content than aged aerosols (Abel et al., 2003; Haywood et al., 2003; Reid et al., 1998). During SAFARI 2000, for example, the single scattering albedo increased from 0.84 to 0.90 between smoke close to the source and aged haze 5 h downwind from a large fire (Abel et al., 2003), which is attributed to changes in the aerosol component. Some rapid changes can occur in the relative concentration of particle types with the aging of smoke, and the BC particles gradually become more aggregated with organic and sulfate particles during the aging process (Pósfai et al., 2003). Therefore, the more abundant presence of particles with the spectral absorption signature of BC is reasonable for the areas near biomass burning emissions, whereas particles with a spectrally dependent absorption signature of BrC are generally enriched in downwind regions, which can explain the appearance of BrC concentrations in aerosol particles transported over ocean in Northern Hemisphere.
4.3 Fine-mode non-absorbing soluble
The NAS component is represented by the real part of the refractive index of ammonium nitrate; however, sulfates, sea salt, or aged hydroscopic particles are also included in the NAS component. Figure 18 presents the seasonal means of the NAS retrieved for the fine mode (FNAS). The FNAS volume concentration dominates over China and India, especially during DJF and SON, which can correspond to industrial aerosol and heating activity in megacities with a high population density. The spatial patterns of FNAS also coincide with the patterns of BC in southern Africa, which indicates the presence of the non-absorbing particle fraction in biomass burning emissions (e.g., water soluble organic carbon). Indeed, carbonaceous organic particles can provide a favorable surface for aging processes and sulfate nucleation (Li et al., 2003). Pronounced FNAS particle concentrations are retrieved during JJA over the Mediterranean Sea region, which is in line with prior knowledge regarding the abundant presence of anthropogenic and biogenic sulfate particles in the Mediterranean region (Ganor et al., 2000; Lelieveld et al., 2002; Levin, 2005; Levin et al., 1996). FNAS particles are also retrieved south of the Mediterranean Sea, deep inland over Libya and Egypt. This FNAS component is possible in this area considering persistent north–south and north–east air mass transport in the eastern Mediterranean region governed by a semipermanent low-pressure trough extending from the Persian Gulf in JJA (Bitan and Sa'Aroni, 1992).
4.4 Coarse-mode non-absorbing insoluble
In northern and western Africa, the coarse-mode non-absorbing insoluble component appears all year long with pronounced maximum concentrations from MAM to JJA (Fig. 19), representing the non-absorbing part of mineral dust. A shift in the maximum of this component towards higher latitudes in JJA that corresponds to the northern shift of the intertropical convergence zone is notable. The retrievals also clearly show a “hot spot” of coarse-mode non-absorbing dust over the Bodélé Depression, located between the Tibesti Mountains and Lake Chad, which is known as the most active dust source in the Sahara Desert (Gasse, 2002; Prospero et al., 2002; Washington et al., 2003). This dust source is caused by the coincidence of an extensive source of diatomite sediment and high-velocity winds associated with the Bodélé Low Level Jet (Todd et al., 2007; Washington et al., 2006; Washington and Todd, 2005), with emission peaks during DJF and MAM (Herrmann et al., 1999; Koren and Kaufman, 2004; Todd et al., 2007; Washington and Todd, 2005) that are also distinguishable in the presented retrievals. This CNAI aerosol type also appears over the Middle East, the Arabian Peninsula, and extends over Asia, which is known as the global dust belt. The coarse-mode non-absorbing dust concentration is particularly high over the Arabian Peninsula, central to southern Pakistan, and over the Oman and Arabian seas. Over this region, the maximum dust concentration is observed during MAM and JJA, while the dust concentration substantially decreases during SON and DJF. The higher dust concentration during MAM and JJA is primarily caused by the strong northwesterly winds known as “shamal wind” and dry conditions. The JJA peak is caused by several major sources of dust that have maximum dust activity during JJA, including desert areas in Syria and Iraq where a strong northwesterly shamal wind is blowing (Choobari et al., 2014). The Sistan region can also be distinguished among the high dust concentrations. This region is considered to be a major dust source in southwest Asia (Ginoux et al., 2012; Goudie, 2014; Léon and Legrand, 2003; Middleton, 1986a) which is attributed to the strong, persistent northeasterly winds (Alizadeh Choobari et al., 2013; Middleton, 1986b; Miri et al., 2007). This source can cause frequent dust and sand storms, especially during the June–August period, contributing to the deterioration of air quality (Rashki et al., 2013). In addition, during DJF and SON some elevated CNAI concentrations are observed in Australia (in the Lake Eyre region and the Great Artesian Basin). It should be also noted that some CNAI concentrations are retrieved during the seasons and over the regions in Africa known for biomass burning and over the southeast of the USA. These concentrations indicate the presence of some coarse-mode non-absorbing particles, possibly of organic origin.
4.5 Coarse-mode absorbing insoluble
The coarse-mode absorbing insoluble (CAI) particles, which mainly represent the iron oxides contained in mineral dust, are generally associated with desert regions and with elevated concentrations of CNAI. The high CAI concentrations are observed during MAM and JJA over western Africa and the Arabian Peninsula (Fig. 20). High CAI concentrations are also retrieved over Asia during the same seasons and are quite clearly attributed to the region of the Taklimakan Desert located in northwest China. It is worth noting that the CAI and CNAI maximums do not always coincide, reflecting a different percentage of iron oxides in desert dust that varies depending on the soil mineralogy of the source region. Calculations of the ratio of CAI to CNAI concentrations over the African continent provided values of up to about 0.05, which is consistent with up to 3 %–5 % of iron oxides in desert dust (e.g., Ganor and Foner, 1996; Guieu et al., 2002; Zhang et al., 2003; Lafon et al., 2004).
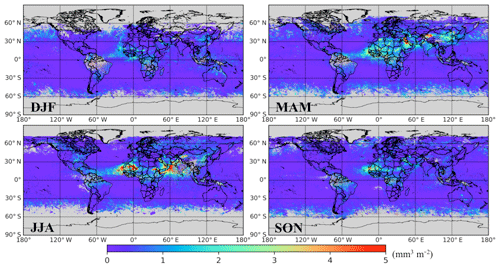
Figure 20Same as Fig. 16, but for coarse-mode absorbing insoluble (CAI, FeOx, and carbonaceous particles).
The high CAI concentrations over western Africa are mainly present over Niger, Mauritania, and near the west coast. This is in line with a study by Formenti et al. (2008) that demonstrates that the higher iron oxide content in Sahelian dust originated from the Sahel belt, while a lower content is observed in the Chad Basin. Lázaro et al. (2008) also reported that the iron oxide content of dust transported to the Canary Islands, near the west coast, tends to have higher values for source areas between 0 and 20∘ N. In addition, high CAI concentrations are also derived over the Arabian Peninsula and the Arabian Sea, which may be attributed to the dust originating from Saudi Arabia, known for the presence of a significant iron content (Krueger et al., 2004). It should be mentioned here that a discontinuity in the retrieved concentrations can be noted between retrievals over land and those over water in the Red Sea and Arabian Sea regions. Given that such a discontinuity does not appear in all coastal regions, but only under particular circumstances, we suppose that there are some physical explanations. For instance, the observed discontinuity corresponds well to the land topography, i.e., the presence of surrounding mountains and the accumulation of aerosol over the Red Sea, the latter of which was observed in a previous study (Brindley et al., 2015). It is also interesting to admit that some coarse-mode absorbing aerosol appears in the regions and seasons associated with biomass burning, whereas elevated concentrations of BrC and BC appear in the fine mode, e.g., in Africa during DJF and SON seasons. This fact may reflect the presence of absorbing carbonaceous material in the coarse mode, which was fitted by refractive index of iron oxide that was assumed to only be an absorbing component of the coarse mode.
4.6 Fine-mode non-absorbing insoluble
Because the fine-mode non-absorbing insoluble component (Fig. 21) can represent both OC and non-absorbing dust, the Ångström exponent can be used as an additional post-retrieval criteria for a better interpretation of this component. For instance, the joint FNAI and Ångström exponent (maps are presented in the Supplement) analysis shows that the particle concentrations derived over western Africa, the Middle East, central Asia, and northwest China mainly reflect the presence of fine-mode non-absorbing dust because they are associated with values of the Ångström exponent that are generally well below one. Specific examples are the concentrations derived over the Bodélé Depression during DJF, the Taklimakan Desert in China during MAM, and the Arabian Peninsula during JJA. However, the elevated FNAI particle concentrations retrieved over southern Africa and South America during JJA and SON, over the eastern part of China and Siberia during JJA, and generally over India, are associated with high Ångström exponent values, and should therefore rather be classified as organic carbon. For example, high OC values in southern China (the Sichuan Basin and the Pearl River Delta region) and urban southern Asia have been confirmed in several previous studies (Decesari et al., 2010; Stone et al., 2010; X. Y. Zhang et al., 2008, 2012). The OC of urban origin in China is enhanced around May to June and in October (Zhang et al., 2012), which may be an explanation for the retrieved high OC concentration during JJA in southern China. Secondary OC (SOC) can also contribute to the total OC concentration (Miyazaki et al., 2006; Weber et al., 2007; X. Y. Zhang et al., 2008, 2005) and be retrieved here as FNAI. Additionally, the elevated OC concentration over South America during SON corresponds well to the known biomass burning season that starts in July and generally peaks in August and September (Duncan et al., 2003).
A plume structure of elevated fine non-absorbing insoluble (Fig. 21) and soluble (Fig. 18) components originating from the Hawaiian Islands in the North Pacific Ocean is also notable. This structure is visible during three seasons from MAM to SON and corresponds to a Hawaiian volcano emission. The material emitted into the atmosphere in this case was not the coarse volcanic ash, but continuous gaseous emissions that can form secondary aerosol during downwind transport (Craddock and Greeley, 2009; Edmonds et al., 2013). Thus, identification of this material using the suggested approach – mixture of components equivalent to ammonium sulfate and fine non-absorbing dust – is quite plausible.
4.7 Aerosol water content and coarse-mode non-absorbing soluble
The algorithm also provides the aerosol water content, which is required to create the host by mixture with the non-absorbing soluble component. As result, the retrieved spatial and temporal patterns of aerosol water content and non-absorbing soluble are very similar. That is, the fine-mode aerosol water content is mainly retrieved in the regions with a high loading of anthropogenic aerosol, similarly to the fine-mode non-absorbing soluble. For instance, the fine-mode aerosol water content can be seen over India and China during SON and DJF, at high latitudes in the Northern Hemisphere, and over Eurasia during SON. Some notable water concentrations are also retrieved over southern Africa during the biomass burning season (JJA), but mainly over ocean, which correspond to visibly transported and likely aged aerosol. The maps of FAWC are presented in the Supplement, as they are very similar to the FNAS that is already presented (Fig. 18).
The retrieved coarse-mode aerosol water content and coarse non-absorbing soluble also present very similar spatial and temporal patterns. However, they are different from the patterns of the fine mode. The concentrations are very low everywhere, except over ocean in the regions associated with high concentrations of the coarse non-absorbing insoluble (dust) component. This feature is associated with dust transported from western Africa and the Arabian Peninsula. These coarse-mode AWC and NAS retrievals require a careful interpretation. First, it should be realized that even a relatively small aerosol water fraction retrieved in the regions of very high aerosol concentration can result in a pronounced volume concentration. In addition, aerosols with low real refractive index, which cannot be fully explained by the assumed dust aerosol model, will be interpreted as a water fraction. For instance, some low water aerosol concentrations erroneously appear over the Bodélé Depression during DJF. The Bodélé dust, however, is known to contain much fossil diatom (Formenti et al., 2008), which would have a different real part of refractive index then the mixture of quartz, kaolinite, and illite assumed in this study. At the same time, possible hygroscopicity of mineral dust, its coating by organics, and its internal mixture with sea salt, have been found in several laboratory and field studies (e.g., Usher et al., 2003; Falkovich et al., 2004; Laskin et al., 2005; Derimian et al., 2017). The fact that a notable aerosol water content is only observed in the retrievals over ocean and not over land, except for retrievals over the Bodélé, also agrees with the hypothesis of the dust hygroscopicity. Therefore, we conclude that, despite this, pronounced water aerosol content in the coarse mode should be questioned and interpreted with caution, and a physical significance of this result should not be excluded. Indeed, this retrieval result may not be fully understood at present, but it was not enforced by any specific assumption or measurement artifact; therefore, it is likely to represent a manifestation of specific physical or chemical transformation of aerosol or properties of dust. In addition to the main feature of CAWC and CNAS described above, the derived maps are presented in the Supplement, along with the maps of FAWC, which are similar to the FNAS that was already presented.
We present a new approach for monitoring atmospheric aerosol components with remote sensing observations. Unlike existing aerosol component retrieval algorithms that interpret an intermediate retrieval of the refractive index, this study utilizes a direct fit of measurements. We demonstrate retrievals of several aerosol components in fine and coarse size modes under the assumption of an internal aerosol mixing rule. The tests using a volume-weighted mixing rule were also conducted and the results compared.
The approach is implemented in a state-of-the-art GRASP algorithm (Dubovik et al., 2014, 2011) designed to process spaceborne and ground-based remote sensing observations. The component module is incorporated in GRASP; thus, the new GRASP/Component version of the code employs mixtures of aerosol components with known refractive indices. This approach also serves as an additional physical constraint on the spectral dependences of the complex refractive index. The component module uses the Maxwell Garnett effective medium approximation (EMA) and is based on the Schuster et al. (2016a, 2009) approach, but assumes independent aerosol mixtures in the fine and coarse modes and the direct fit of radiances instead of an intermediate step of fitting the retrieved refractive indices.
A series of numerical sensitivity tests using synthetic data were conducted to evaluate the component retrieval. Results of the tests showed that the new conversion module allows the retrieval to distinguish amongst several assumed aerosol components. The tests with the new module also show consistency with GRASP tests that are traditionally configured for ground-based AERONET measurements.
We also tested the algorithm with real measurements. Application of the GRASP/Component algorithm to the AERONET sun/sky photometric measurements retrievals of AOT, the Ångström exponent, and SSA presented good agreement with the standard operational AERONET product for sites dominated by dust, biomass burning, and mixtures of dust and biomass burning aerosol. In addition, due to the reduced number of parameters (using six parameters for component retrievals instead of eight parameters for complex refractive index retrievals) and an additional physical constraint on the spectral dependence of the refractive index in the component retrieval, the GRASP/Component approach applied for AERONET can split the characteristics of fine- and coarse-mode aerosol. The GRASP/Component algorithm was also applied for the POLDER/PARASOL satellite observations. An intercomparison of aerosol optical characteristics derived from POLDER/PARASOL using the component approach and those of the AERONET operational product demonstrated a high reliability of the results.
The performance of the aerosol component algorithm has been demonstrated by the application to POLDER/PARASOL observations on the global scale for the year 2008. The spatial and temporal patterns of aerosol component distribution obtained seem to agree well with known physical expectations. For a proper interpretation of the results obtained, it should also be noted that the retrieved aerosol species and their concentrations compose a set of parameters that satisfactorily reproduces the measured radiation field and provides adequate retrieved optical properties of aerosol. At the same time, direct interpretation from a chemical point of view is not always evident or even possible. For instance, as mentioned in Sect. 2, distinguishing some species is not possible for the given configuration of remote sensing measurements. However, the retrieved components still reflect the aerosol microphysics and chemistry, and their variability. One should also remember that, based on the sensitivity tests and experience of aerosol characterization by remote sensing, the accuracy of the retrievals depends on the aerosol loading (the AOT). The accuracy of the absorbing components' retrieval can be primarily affected. Thus, interpretation of all of the patterns obtained requires a more detailed analysis, and we realize that some erroneous component features may be possible. The principal limitations of the presented approach are (i) the lack of sensitivity to absorption species in case of low AOT; (ii) the difficulty of distinguishing between iron oxide and absorbing carbonaceous species (BrC and BC), which is mainly related to the limited number of spectral channels in the shortwave solar spectrum; and (iii) the fact that the non-absorbing insoluble component can include organic material, but also non-absorbing dust. These assumptions can lead to some misinterpretation; for instance, the analysis of the BrC retrievals at some locations reveals that the aerosol absorbing properties attributed to BrC should be attributed to the iron oxides that are present in the fine size fraction. A post-retrieval classification is helpful to resolve the shortcomings. For example, analysis of the Ångström exponent can indicate the dominance of coarse particles of mineral dust origin or fine particles of combustion origin, which can provide more information about the non-absorbing insoluble component.
Nevertheless, the results are encouraging. For example, the derived BC and BrC exhibit a seasonal and spatial variability that is attributed to the known biomass burning season cycle in Africa and the anthropogenic pollution patterns in Asia, in particular India and China. Coarse-mode absorbing (mainly iron oxides) and non-absorbing (mainly dust) insoluble components show a similar seasonal and spatial variability, reaching a peak during MAM and a minimum during SON. It is also noted that the maximums of the iron oxide concentration are not co-located with those of dust, because the elemental and mineralogical components of mineral dust vary depending on the source region. The global dust belt extending from western Africa, through the Middle East to Central Asia is also observed in the component retrieval. GRASP/Component indicates high concentrations of non-absorbing insoluble over the Sahara, the Arabian Peninsula, the Caspian Sea and Aral Sea regions in central Asia, and the Gobi and Taklimakan deserts in China. In addition, dust was also detected over some regions in Australia during DJF and SON.
The component retrieval algorithm demonstrated here using AERONET and POLDER/PARASOL data can also be used for interpreting other observations. That is, the component approach is now incorporated in the GRASP algorithm, which has a generalized input and can be easily modified and adapted to other passive and active remote sensing instruments, for example, the Directional Polarimetric Camera (DPC) launched onboard the GaoFen-5 satellite in the Chinese High-resolution Earth Observation program, which is the first Chinese multi-angle polarized earth observation satellite sensor (Dubovik et al., 2019; Li et al., 2018). Moreover, the proposed aerosol parameterization using components can be helpful not only for retrieving additional information about aerosol components, but also for optimizing retrieval stability.
Additionally, we tested the volume-weighted mixing model, as well as the Maxwell Garnett EMA, to evaluate the sensitivity of our approach to the assumed aerosol EMA. We tested both approaches using our suite of aerosol species (i.e., BC, BrC, coarse-mode absorbing insoluble, and fine- and coarse-mode non-absorbing insoluble). The sensitivity tests revealed that the implementation of the volume-weighted mixing rule also presents stable results that are consistent with the Maxwell Garnett EMA. Thus, the volume-weighted model can also be employed in the GRASP/Component retrieval, and may be preferable in some applications due to its simplicity.
The results of the aerosol component retrieval from AERONET and POLDER/PARASOL satellite measurements demonstrate a potential for constraining global and regional aerosol modeling that would be particularly valuable as no other aerosol component data are often available on a large spatial and temporal scale.
The retrievals can be requested directly from the corresponding author (oleg.dubovik@univ-lille.fr or yevgeny.derimian@univ-lille.fr)
The supplement related to this article is available online at: https://doi.org/10.5194/acp-19-13409-2019-supplement.
LL, OD, YD, and GLS developed the retrieval algorithm, designed and realized the sensitivity and uncertainty tests, and applied the algorithm to the real data. OD suggested the aerosol component retrieval concept with contributions from YD and GLS. TL, PL, AL, FD, DF, and CC contributed to the modifications of the GRASP code and the application to the satellite data. BT and AL contributed to the application to the AERONET data. ZL and HC supported the work and provided expertise on the Asian aerosol observations. LL, YD, and OD wrote the paper with input from all the authors.
The authors declare that they have no conflict of interest.
The authors thank CNES and the ICARE data distribution center for the POLDER/PARASOL data and the entire AERONET team, especially the principal investigators of the AERONET sites used in this study, for their long-term efforts to maintain AERONET observations. The authors acknowledge the suggestions from Mian Chin (NASA GSFC) regarding the connection and comparison with global chemical transport models, and the suggestions from Lorraine A. Remer (UMBC) regarding the interpretation of the results and the uncertainties.
This work is supported by the Labex CaPPA (Laboratory of Excellence – Chemical and Physical Properties of the Atmosphere) project, which is funded by the French National Research Agency through the PIA (Programme d'Investissement d'Avenir) under contract “ANR-11-LABX-0005-01”. This work is also financially supported by the National Science Fund for Distinguished Young Scholars (grant no. 41825011) and the National Key R&D Program of China (grant no. 2016YFA0601901).
This paper was edited by Toshihiko Takemura and reviewed by Lorraine Remer and two anonymous referees.
Abel, S. J., Haywood, J. M., Highwood, E. J., Li, J., and Buseck, P. R.: Evolution of biomass burning aerosol properties from an agricultural fire in southern Africa, Geophys. Res. Lett., 30, 10–13, https://doi.org/10.1029/2003GL017342, 2003.
Alfaro, S., Lafon, S., Rajot, J., Formenti, P., Gaudichet, A., and Maille, M.: Iron oxides and light absorption by pure desert dust: an experimental study, J. Geophys. Res., 109, D08208, https://doi.org/10.1029/2003JD004374, 2004.
Alizadeh Choobari, O., Zawar-Reza, P., and Sturman, A.: Low level jet intensification by mineral dust aerosols, Ann. Geophys., 31, 625–632, https://doi.org/10.5194/angeo-31-625-2013, 2013.
Andreae, M. O. and Gelencsér, A.: Black carbon or brown carbon? The nature of light-absorbing carbonaceous aerosols, Atmos. Chem. Phys., 6, 3131–3148, https://doi.org/10.5194/acp-6-3131-2006, 2006.
Arimoto, R., Balsam, W., and Schloesslin, C.: Visible spectroscopy of aerosol particles collected on filters: Iron-oxide minerals, Atmos. Environ., 36, 89–96, https://doi.org/10.1016/S1352-2310(01)00465-4, 2002.
Arola, A., Schuster, G., Myhre, G., Kazadzis, S., Dey, S., and Tripathi, S. N.: Inferring absorbing organic carbon content from AERONET data, Atmos. Chem. Phys., 11, 215–225, https://doi.org/10.5194/acp-11-215-2011, 2011.
Bahadur, R., Praveen, P., Xu, Y., and Ramanathan, V.: Solar absorption by elemental and brown carbon determined from spectral observations, P. Natl. Acad. Sci. USA, 109, 17366–17371, https://doi.org/10.1073/pnas.1205910109, 2012.
Benavent-Oltra, J. A., Román, R., Granados-Muñoz, M. J., Pérez-Ramírez, D., Ortiz-Amezcua, P., Denjean, C., Lopatin, A., Lyamani, H., Torres, B., Guerrero-Rascado, J. L., Fuertes, D., Dubovik, O., Chaikovsky, A., Olmo, F. J., Mallet, M., and Alados-Arboledas, L.: Comparative assessment of GRASP algorithm for a dust event over Granada (Spain) during ChArMEx-ADRIMED 2013 campaign, Atmos. Meas. Tech., 10, 4439–4457, https://doi.org/10.5194/amt-10-4439-2017, 2017.
Bitan, A. and Sa'Aroni, H.: The horizontal and vertical extension of the Persian Gulf pressure trough, Int. J. Climatol., 12, 733–747, https://doi.org/10.1002/joc.3370120706, 1992.
Bohren, C. F. and Huffman, D. R.: Scattering Coefficients, in: Absorption and Scattering of Light by Small Particles, 1983.
Bond, T. C. and Bergstrom, R. W.: Light Absorption by Carbonaceous Particles: An Investigative Review, Aerosol Sci. Tech., 40, 27–67, https://doi.org/10.1080/02786820500421521, 2006.
Bond, T. C., Charlson, R. J., and Heintzenberg, J.: Quantifying the emission of light-absorbing particles: Measurements tailored to climate studies, Geophys. Res. Lett., 25, 337–340, https://doi.org/10.1029/98GL00039, 1998.
Bond, T. C., Doherty, S. J., Fahey, D. W., Forster, P. M., Berntsen, T., Deangelo, B. J., Flanner, M. G., Ghan, S., Kärcher, B., Koch, D., Kinne, S., Kondo, Y., Quinn, P. K., Sarofim, M. C., Schultz, M. G., Schulz, M., Venkataraman, C., Zhang, H., Zhang, S., Bellouin, N., Guttikunda, S. K., Hopke, P. K., Jacobson, M. Z., Kaiser, J. W., Klimont, Z., Lohmann, U., Schwarz, J. P., Shindell, D., Storelvmo, T., Warren, S. G., and Zender, C. S.: Bounding the role of black carbon in the climate system: A scientific assessment, J. Geophys. Res.-Atmos., 118, 5380–5552, https://doi.org/10.1002/jgrd.50171, 2013.
Brindley, H., Osipov, S., Bantges, R., Smirnov, A., Banks, J., Levy, R., Jish Prakash, P., and Stenchikov, G.: An assessment of the quality of aerosol retrievals over the Red Sea and evaluation of the climatological cloud-free dust direct radiative effect in the region, J. Geophys. Res.-Atmos., 120, 10862–10878, https://doi.org/10.1002/2015JD023282, 2015.
Cahoon, D. R., Stocks, B. J., Levine, J. S., Cofer, W. R., and O'Neill, K. P.: Seasonal distribution of African savanna fires, Nature, 359, 812–815, https://doi.org/10.1038/359812a0, 1992.
Chami, M., Santer, R., and Dilligeard, E.: Radiative transfer model for the computation of radiance and polarization in an ocean–atmosphere system: polarization properties of suspended matter for remote sensing, Appl. Optics, 40, 2398, https://doi.org/10.1038/laban1011-313, 2001.
Chen, C., Dubovik, O., Henze, D. K., Lapyonak, T., Chin, M., Ducos, F., Litvinov, P., Huang, X., and Li, L.: Retrieval of desert dust and carbonaceous aerosol emissions over Africa from POLDER/PARASOL products generated by the GRASP algorithm, Atmos. Chem. Phys., 18, 12551–12580, https://doi.org/10.5194/acp-18-12551-2018, 2018.
Chen, C., Dubovik, O., Henze, D. K., Chin, M., Lapyonok, T., Schuster, G. L., Ducos, F., Fuertes, D., Litvinov, P., Li, L., Lopatin, A., Hu, Q., and Torres, B.: Constraining global aerosol emissions using POLDER/PARASOL satellite remote sensing observations, Atmos. Chem. Phys. Discuss., https://doi.org/10.5194/acp-2019-623, in review, 2019.
Chen, C. T. and Cahan, B. D.: Visible and ultraviolet optical properties of single-crystal and polycrystalline hematite measured by spectroscopic ellipsometry, J. Opt. Soc. Am., 71, 932–934, 1981.
Chen, Y. and Bond, T. C.: Light absorption by organic carbon from wood combustion, Atmos. Chem. Phys., 10, 1773–1787, https://doi.org/10.5194/acp-10-1773-2010, 2010.
Choobari, O. A., Zawar-Reza, P., and Sturman, A.: The global distribution of mineral dust and its impacts on the climate system: A review, Atmos. Res., 138, 152–165, https://doi.org/10.1016/j.atmosres.2013.11.007, 2014.
Chowdhary, J., Cairns, B., and Travis, L. D.: Contribution of water-leaving radiances to multiangle, multispectral polarimetric observations over the open ocean: bio-optical model results for case 1 waters, Appl. Optics, 45, 5542–5567, https://doi.org/10.1364/AO.45.005542, 2006.
Chung, C., Ramanathan, V., and Decremer, D.: Observationally constrained estimates of carbonaceous aerosol radiative forcing, P. Natl. Acad. Sci. USA, 109, 11624–11629, https://doi.org/10.1073/pnas.1203707109, 2012.
Collins, W. D., Rasch, P. J., Eaton, B. E., Khattatov, B. V, Lamarque, J.-F., and Zender, C. S.: Simulating aerosols using a chemical transport model with assimilation of satellite aerosol retrievals: Methodology for INDOEX, J. Geophys. Res.-Atmos., 106, 7313–7336, https://doi.org/10.1029/2000JD900507, 2001.
Cooke, W. F., Liousse, C., Cachier, H., and Feichter, J.: Construction of a fossil fuel emission data set for carbonaceous aerosol and implementation and radiative impact in the ECHAM4 model, J. Geophys. Res.-Atmos., 104, 22137–22162, https://doi.org/10.1029/1999JD900187, 1999.
Cox, C. and Munk, W.: Measurement of the Roughness of the Sea Surface from Photographs of the Sun's Glitter, J. Opt. Soc. Am., 44, 838–850, https://doi.org/10.1364/JOSA.44.000838, 1954.
Craddock, R. A. and Greeley, R.: Minimum estimates of the amount and timing of gases released into the martian atmosphere from volcanic eruptions, Icarus, 204, 512–526, https://doi.org/10.1016/j.icarus.2009.07.026, 2009.
Decesari, S., Facchini, M. C., Carbone, C., Giulianelli, L., Rinaldi, M., Finessi, E., Fuzzi, S., Marinoni, A., Cristofanelli, P., Duchi, R., Bonasoni, P., Vuillermoz, E., Cozic, J., Jaffrezo, J. L., and Laj, P.: Chemical composition of PM10 and PM1 at the high-altitude Himalayan station Nepal Climate Observatory-Pyramid (NCO-P) (5079 m a.s.l.), Atmos. Chem. Phys., 10, 4583–4596, https://doi.org/10.5194/acp-10-4583-2010, 2010.
de Leeuw, G., Holzer-Popp, T., Bevan, S., Davies, W. H., Descloitres, J., Grainger, R. G., Griesfeller, J., Heckel, A., Kinne, S., Klüser, L., Kolmonen, P., Litvinov, P., Martynenko, D., North, P., Ovigneur, B., Pascal, N., Poulsen, C., Ramon, D., Schulz, M., Siddans, R., Sogacheva, L., Tanré, D., Thomas, G. E., Virtanen, T. H., von Hoyningen Huene, W., Vountas, M., and Pinnock, S.: Evaluation of seven European aerosol optical depth retrieval algorithms for climate analysis, Remote Sens. Environ., 162, 295–315, https://doi.org/10.1016/j.rse.2013.04.023, 2015.
Derimian, Y., Karnieli, A., Kaufman, Y. J., Andreae, M. O., Andreae, T. W., Dubovik, O., Maenhaut, W., and Koren, I.: The role of iron and black carbon in aerosol light absorption, Atmos. Chem. Phys., 8, 3623–3637, https://doi.org/10.5194/acp-8-3623-2008, 2008.
Derimian, Y., Choël, M., Rudich, Y., Deboudt, K., Dubovik, O., Laskin, A., Legrand, M., Damiri, B., Koren, I., Unga, F., Moreau, M., Andreae, M. O., and Karnieli, A.: Effect of sea breeze circulation on aerosol mixing state and radiative properties in a desert setting, Atmos. Chem. Phys., 17, 11331–11353, https://doi.org/10.5194/acp-17-11331-2017, 2017.
Deschamps, P. Y., Buriez, J. C., Bréon, F. M., Leroy, M., Podaire, A., Bricaud, A., and Sèze, G.: The POLDER Mission: Instrument Characteristics and Scientific Objectives, IEEE T. Geosci. Remote., 32, 598–615, https://doi.org/10.1109/36.297978, 1994.
Deuzé, J. L., Bréon, F. M., Devaux, C., Goloub, P., Herman, M., Lafrance, B., Maignan, F., Marchand, A., Nadal, F., Perry, G., and Tanré, D.: Remote sensing of aerosols over land surfaces from POLDER-ADEOS-1 polarized measurements, J. Geophys. Res.-Atmos., 106, 4913–4926, https://doi.org/10.1029/2000JD900364, 2001.
Dinar, E., Abo Riziq, A., Spindler, C., Erlick, C., Kiss, G., and Rudich, Y.: The complex refractive index of atmospheric and model humic-like substances (HULIS) retrieved by a cavity ring down aerosol spectrometer (CRD-AS), Faraday Discuss., 137, 279–295, https://doi.org/10.1039/b703111d, 2007.
Downing, H. D. and Williams, D.: Optical constants of water in the infrared, J. Geophys. Res., 80, 1656–1661, https://doi.org/10.1029/JC080i012p01656, 1975.
Dubovik, O.: Optimization of Numerical Inversion in Photopolarimetric Remote Sensing, in: Photopolarimetry in Remote Sensing, Kluwer Academic Publishers, Dordrecht, 65–106, https://doi.org/10.1007/1-4020-2368-5_3, 2004.
Dubovik, O., Holben, B., Eck, T. F., Smirnov, A., Kaufman, Y. J., King, M. D., Tanré, D., and Slutsker, I.: Variability of Absorption and Optical Properties of Key Aerosol Types Observed in Worldwide Locations, J. Atmos. Sci., 59, 590–608, 2002a.
Dubovik, O., Holben, B. N., Lapyonok, T., Sinyuk, A., Mishchenko, M. I., Yang, P., and Slutsker, I.: Non-spherical aerosol retrieval method employing light scattering by spheroids, Geophys. Res. Lett., 29, 541–544, https://doi.org/10.1029/2001GL014506, 2002b.
Dubovik, O., Herman, M., Holdak, A., Lapyonok, T., Tanré, D., Deuzé, J. L., Ducos, F., Sinyuk, A., and Lopatin, A.: Statistically optimized inversion algorithm for enhanced retrieval of aerosol properties from spectral multi-angle polarimetric satellite observations, Atmos. Meas. Tech., 4, 975–1018, https://doi.org/10.5194/amt-4-975-2011, 2011.
Dubovik, O. and King, M. D.: A flexible inversion algorithm for retrieval of aerosol optical properties from Sun and sky radiance measurements, J. Geophys. Res.-Atmos., 105, 20673–20696, https://doi.org/10.1029/2000JD900282, 2000.
Dubovik, O., Sinyuk, A., Lapyonok, T., Holben, B. N., Mishchenko, M., Yang, P., Eck, T. F., Volten, H., Muñoz, O., Veihelmann, B., van der Zande, W. J., Leon, J. F., Sorokin, M., and Slutsker, I.: Application of spheroid models to account for aerosol particle nonsphericity in remote sensing of desert dust, J. Geophys. Res.-Atmos., 111, 1–34, https://doi.org/10.1029/2005JD006619, 2006.
Dubovik, O., Lapyonok, T., Kaufman, Y. J., Chin, M., Ginoux, P., Kahn, R. A., and Sinyuk, A.: Retrieving global aerosol sources from satellites using inverse modeling, Atmos. Chem. Phys., 8, 209–250, https://doi.org/10.5194/acp-8-209-2008, 2008.
Dubovik, O., Lapyonok, T., Litvinov, P., Herman, M., Fuertes, D., Ducos, F., Torres, B., Derimian, Y., Huang, X., Lopatin, A., Chaikovsky, A., Aspetsberger, M., and Federspiel, C.: GRASP: a versatile algorithm for characterizing the atmosphere, SPIE Newsroom, 2–5, https://doi.org/10.1117/2.1201408.005558, 2014.
Dubovik, O., Li, Z., Mishchenko, M. I., Tanré, D., Karol, Y., Bojkov, B., Cairns, B., Diner, D. J., Espinosa, W. R., Goloub, P., Gu, X., Hasekamp, O., Hong, J., Hou, W., Knobelspiesse, K. D., Landgraf, J., Li, L., Litvinov, P., Liu, Y., Lopatin, A., Marbach, T., Maring, H., Martins, V., Meijer, Y., Milinevsky, G., Mukai, S., Parol, F., Qiao, Y., Remer, L., Rietjens, J., Sano, I., Stammes, P., Stamnes, S., Sun, X., Tabary, P., Travis, L. D., Waquet, F., Xu, F., Yan, C., and Yin, D.: Polarimetric remote sensing of atmospheric aerosols: Instruments, methodologies, results, and perspectives, J. Quant. Spectrosc. Ra., 224, 474–511, https://doi.org/10.1016/j.jqsrt.2018.11.024, 2019.
Duncan, B. N., Martin, R. V., Staudt, A. C., Yevich, R., and Logan, J. A.: Interannual and seasonal variability of biomass burning emissions constrained by satellite observations, J. Geophys. Res., 108, 4100, https://doi.org/10.1029/2002JD002378, 2003.
Edmonds, M., Sides, I. R., Swanson, D. A., Werner, C., Martin, R. S., Mather, T. A., Herd, R. A., Jones, R. L., Mead, M. I., Sawyer, G., Roberts, T. J., Sutton, A. J., and Elias, T.: Magma storage, transport and degassing during the 2008-10 summit eruption at Kilauea Volcano, Hawaii, Geochim. Cosmochim. Ac., 123, 284–301, https://doi.org/10.1016/j.gca.2013.05.038, 2013.
Espinosa, W. R., Remer, L. A., Dubovik, O., Ziemba, L., Beyersdorf, A., Orozco, D., Schuster, G., Lapyonok, T., Fuertes, D., and Martins, J. V.: Retrievals of aerosol optical and microphysical properties from Imaging Polar Nephelometer scattering measurements, Atmos. Meas. Tech., 10, 811–824, https://doi.org/10.5194/amt-10-811-2017, 2017.
Falkovich, A. H., Schkolnik, G., Ganor, E., and Rudich, Y.: Adsorption of organic compounds pertinent to urban environments onto mineral dust particles, J. Geophys. Res.-Atmos., 109, D02208, https://doi.org/10.1029/2003jd003919, 2004.
Formenti, P., Rajot, J. L., Desboeufs, K., Caquineau, S., Chevaillier, S., Nava, S., Gaudichet, A., Journet, E., Triquet, S., Alfaro, S., Chiari, M., Haywood, J., Coe, H., and Highwood, E.: Regional variability of the composition of mineral dust from western Africa: Results from the AMMA SOP0/DABEX and DODO field campaigns, J. Geophys. Res.-Atmos., 113, 1–12, https://doi.org/10.1029/2008JD009903, 2008.
Formenti, P., Caquineau, S., Chevaillier, S., Klaver, A., Desboeufs, K., Rajot, J. L., Belin, S., and Briois, V.: Dominance of goethite over hematite in iron oxides of mineral dust from Western Africa: Quantitative partitioning by X-ray absorption spectroscopy, J. Geophys. Res.-Atmos., 119, 12740–12754, https://doi.org/10.1002/2014JD021668, 2014.
Ganor, E. and Foner, H. A.: The mineralogical and chemical properties and the behavior of aeolian Saharan dust over Israel, in: The Impact of Desert Dust Across the Mediterranean, edited by: Guerzoni, S. and Chester, R., Kluwer Academic Publishers, Printed in the Netherlands, 163–172, 1996.
Ganor, E., Foner, H. A., Bingemer, H. G., Udisti, R., and Setter, I.: Biogenic sulphate generation in the Mediterranean Sea and its contribution to the sulphate anomaly in the aerosol over Israel and the Eastern Mediterranean, Atmos. Environ., 34, 3453–3462, https://doi.org/10.1016/S1352-2310(00)00077-7, 2000.
Gasse, F.: Diatom-inferred salinity and carbonate oxygen isotopes in Holocene waterbodies of the western Sahara and Sahel (Africa), Quaternary Sci. Rev., 21, 737–767, https://doi.org/10.1016/S0277-3791(01)00125-1, 2002.
Ghosh, G.: Dispersion-equation coefficients for the refractive index and birefringence of calcite and quartz crystals, Opt. Commun., 163, 95–102, https://doi.org/10.1016/S0030-4018(99)00091-7, 1999.
Ginoux, P., Prospero, J. M., Gill, T. E., Hsu, N. C., and Zhao, M.: Global-scale attribution of anthropogenic and natural dust sources and their emission rates based on MODIS Deep Blue aerosol products, Rev. Geophys., 50, 1–36, https://doi.org/10.1029/2012RG000388, 2012.
Gosse, S. F., Wang, M., Labrie, D., and Chylek, P.: Imaginary part of the refractive index of sulfates and nitrates in the 0.7-2.6-micron spectral region, Appl. Optics, 36, 3622–3634, 1997.
Goudie, A. S.: Desert dust and human health disorders, Environ. Int., 63, 101–113, https://doi.org/10.1016/j.envint.2013.10.011, 2014.
Guieu, C., Loye-Pilot, M. D., Ridame, C., and Thomas, C.: Chemical characterization of the Saharan dust end-member: Some biogeochemical implications for the western Mediterranean Sea, J. Geophys. Res., 107, 4258, https://doi.org/10.1029/2001JD000582, 2002.
Hale, G. M. and Querry, M. R.: Optical Constants of Water in the 200-nm to 200-microm Wavelength Region, Appl. Optics, 12, 555–563, https://doi.org/10.1364/AO.12.000555, 1973.
Hammer, M. S., Martin, R. V., Li, C., Torres, O., Manning, M., and Boys, B. L.: Insight into global trends in aerosol composition from 2005 to 2015 inferred from the OMI Ultraviolet Aerosol Index, Atmos. Chem. Phys., 18, 8097–8112, https://doi.org/10.5194/acp-18-8097-2018, 2018.
Haywood, J. M. and Shine, K. P.: The effect of anthropogenic sulfate and soot aerosol on the clear sky planetary radiation budget, Geophys. Res. Lett., 22, 603–606, https://doi.org/10.1029/95GL00075, 1995.
Haywood, J. M., Osborne, S. R., Francis, P. N., Keil, A., Formenti, P., Andreae, M. O., and Kaye, P. H.: The mean physical and optical properties of regional haze dominated by biomass burning aerosol measured from the C-130 aircraft during SAFARI 2000, J. Geophys. Res.-Atmos., 108, 1–9, https://doi.org/10.1029/2002JD002226, 2003.
Haywood, J. M., Pelon, J., Formenti, P., Bharmal, N., Brooks, M., Capes, G., Chazette, P., Chou, C., Christopher, S., Coe, H., Cuesta, J., Derimian, Y., Desboeufs, K., Greed, G., Harrison, M., Heese, B., Highwood, E. J., Johnson, B., Mallet, M., Marticorena, B., Marsham, J., Milton, S., Myhre, G., Osborne, S. R., Parker, D. J., Rajot, J. L., Schulz, M., Slingo, A., Tanre, D., and Tulet, P.: Overview of the Dust and Biomass-burning Experiment and African Monsoon Multidisciplinary Analysis Special Observing Period-0, J. Geophys. Res.-Atmos., 113, D00C17, https://doi.org/10.1029/2008jd010077, 2008.
Henze, D. K., Hakami, A., and Seinfeld, J. H.: Development of the adjoint of GEOS-Chem, Atmos. Chem. Phys., 7, 2413–2433, https://doi.org/10.5194/acp-7-2413-2007, 2007.
Herman, M., Deuzé, J. L., Marchand, A., Roger, B., and Lallart, P.: Aerosol remote sensing from POLDER/ADEOS over the ocean: Improved retrieval using a nonspherical particle model, J. Geophys. Res.-Atmos., 110, 1–11, https://doi.org/10.1029/2004JD004798, 2005.
Herrmann, L., Stahr, K., and Jahn, R.: The importance of source region identification and their properties for soil-derived dust: The case of Harmattan dust sources for eastern West Africa, Contrib. Atmos. Phys., 72, 141–150, 1999.
Hoffer, A., Gelencsér, A., Guyon, P., Kiss, G., Schmid, O., Frank, G. P., Artaxo, P., and Andreae, M. O.: Optical properties of humic-like substances (HULIS) in biomass-burning aerosols, Atmos. Chem. Phys., 6, 3563–3570, https://doi.org/10.5194/acp-6-3563-2006, 2006.
Jacobson, M. Z.: Isolating nitrated and aromatic aerosols and nitrated aromatic gases as sources of ultraviolet light absorption, J. Geophys. Res.-Atmos., 104, 3527–3542, https://doi.org/10.1029/1998JD100054, 1999.
Jickells, T. D., An, Z. S., Andersen, K. K., Baker, A. R., Bergametti, C., Brooks, N., Cao, J. J., Boyd, P. W., Duce, R. A., Hunter, K. A., Kawahata, H., Kubilay, N., LaRoche, J., Liss, P. S., Mahowald, N., Prospero, J. M., Ridgwell, A. J., Tegen, I., and Torres, R.: Global iron connections between desert dust, ocean biogeochemistry, and climate, Science, 308, 67–71, https://doi.org/10.1126/science.1105959, 2005.
Journet, E., Balkanski, Y., and Harrison, S. P.: A new data set of soil mineralogy for dust-cycle modeling, Atmos. Chem. Phys., 14, 3801–3816, https://doi.org/10.5194/acp-14-3801-2014, 2014.
Kahn, R. A. and Gaitley, B. J.: An analysis of global aerosol type as retrieved by MISR, J. Geophys. Res.-Atmos., 120, 4248–4281, https://doi.org/10.1002/2015JD023322, 2015.
Kanakidou, M., Seinfeld, J. H., Pandis, S. N., Barnes, I., Dentener, F. J., Facchini, M. C., Van Dingenen, R., Ervens, B., Nenes, A., Nielsen, C. J., Swietlicki, E., Putaud, J. P., Balkanski, Y., Fuzzi, S., Horth, J., Moortgat, G. K., Winterhalter, R., Myhre, C. E. L., Tsigaridis, K., Vignati, E., Stephanou, E. G., and Wilson, J.: Organic aerosol and global climate modelling: a review, Atmos. Chem. Phys., 5, 1053–1123, https://doi.org/10.5194/acp-5-1053-2005, 2005.
Kerker, M., Scheiner, P., Cooke, D. D., and Kratohvil, J. P.: Absorption index and color of colloidal hematite, J. Colloid Interf. Sci., 71, 176–187, https://doi.org/10.1016/0021-9797(79)90231-5, 1979.
Kirchstetter, T. W., Novakov, T., and Hobbs, P. V.: Evidence that the spectral dependence of light absorption by aerosols is affected by organic carbon, J. Geophys. Res.-Atmos., 109, D21208, https://doi.org/10.1029/2004JD004999, 2004.
Koepke, P.: Effective reflectance of oceanic whitecaps, Appl. Optics, 23, 1816, https://doi.org/10.1364/AO.23.001816, 1984.
Koepke, P., Hess, M., Schult, I., and Shettle, E. P.: Global Aerosol Data Set, Max-Planck-Institut fur Meteorologie, ISSN: 0937-1060, 1997.
Koren, I. and Kaufman, Y. J.: Direct wind measurements of Saharan dust events from Terra and Aqua satellites, Geophys. Res. Lett., 31, 1–4, https://doi.org/10.1029/2003GL019338, 2004.
Kou, L., Labrie, D., and Chylek, P.: Refractive indices of water and ice in the 0.65 to 2.5 µm spectral range, Appl. Optics, 32, 3531, https://doi.org/10.1364/AO.32.003531, 1993.
Koven, C. D. and Fung, I.: Inferring dust composition from wavelength-dependent absorption in Aerosol Robotic Network (AERONET) data, J. Geophys. Res.-Atmos., 111, D14205, https://doi.org/10.1029/2005JD006678, 2006.
Krueger, B. J., Grassian, V. H., Cowin, J. P., and Laskin, A.: Heterogeneous chemistry of individual mineral dust particles from different dust source regions: The importance of particle mineralogy, Atmos. Environ., 38, 6253–6261, https://doi.org/10.1016/j.atmosenv.2004.07.010, 2004.
Lafon, S., Rajot, J. L., Alfaro, S. C., and Gaudichet, A.: Quantification of iron oxides in desert aerosol, Atmos. Environ., 38, 1211–1218, 2004.
Lafon, S., Sokolik, I. N., Rajot, J. L., Caquincau, S., and Gaudichet, A.: Characterization of iron oxides in mineral dust aerosols: Implications for light absorption, J. Geophys. Res.-Atmos., 111, 1–19, https://doi.org/10.1029/2005JD007016, 2006.
Laskin, A., Iedema, M. J., Ichkovich, A., Graber, E. R., Taraniuk, I., and Rudich, Y.: Direct observation of completely processed calcium carbonate dust particles, Faraday Discuss., 130, 453-468, https://doi.org/10.1039/b417366j, 2005.
Lázaro, F. J., Gutiérrez, L., Barrón, V., and Gelado, M. D.: The speciation of iron in desert dust collected in Gran Canaria (Canary Islands): Combined chemical, magnetic and optical analysis, Atmos. Environ., 42, 8987–8996, https://doi.org/10.1016/j.atmosenv.2008.09.035, 2008.
Lelieveld, J., Berresheim, H., Borrmann, S., Crutzen, P. J., Dentener, F. J., Fischer, H., Feichter, J., Flatau, P. J., Heland, J., Holzinger, R., Korrmann, R., Lawrence, M. G., Levin, Z., Markowicz, K. M., Mihalopoulos, N., Minikin, A., Ramanathan, V., De Reus, M., Roelofs, G. J., Scheeren, H. A., Sciare, J., Schlager, H., Schultz, M., Siegmund, P., Steil, B., Stephanou, E. G., Stier, P., Traub, M., Warneke, C., Williams, J., and Ziereis, H.: Global air pollution crossroads over the Mediterranean, Science, 298, 794–799, https://doi.org/10.1126/science.1075457, 2002.
Léon, J. F. and Legrand, M.: Mineral dust sources in the surroundings of the North Indian Ocean, Geophys. Res. Lett., 30, 1309, https://doi.org/10.1029/2002GL016690, 2003.
Lesins, G., Chylek, P., and Lohmann, U.: A study of internal and external mixing scenarios and its effect on aerosol optical properties and direct radiative forcing, J. Geophys. Res.-Atmos., 107, 4094, https://doi.org/10.1029/2001JD000973, 2002.
Levin, Z.: On the interactions of mineral dust, sea-salt particles, and clouds: A measurement and modeling study from the Mediterranean Israeli Dust Experiment campaign, J. Geophys. Res., 110, D20202, https://doi.org/10.1029/2005JD005810, 2005.
Levin, Z., Ganor, E., and Gladstein, V.: The effects of desert particles coated with sulfate on rain formation in the Eastern Mediterranean, J. Appl. Meteorol., 35, 1511–1523, 1996.
Li, J., Pósfai, M., Hobbs, P. V., and Buseck, P. R.: Individual aerosol particles from biomass burning in southern Africa: 2, Compositions and aging of inorganic particles, J. Geophys. Res.-Atmos., 108, 8484, https://doi.org/10.1029/2002JD002310, 2003.
Li, X. and Strahler, A. H.: Geometric-Optical Bidirectional Reflectance Modeling of the Discrete Crown Vegetation Canopy: Effect of Crown Shape and Mutual Shadowing, IEEE T. Geosci. Remote, 30, 276–292, https://doi.org/10.1109/36.134078, 1992.
Li, Z., Gu, X., Wang, L., Li, D., Xie, Y., Li, K., Dubovik, O., Schuster, G., Goloub, P., Zhang, Y., Li, L., Ma, Y., and Xu, H.: Aerosol physical and chemical properties retrieved from ground-based remote sensing measurements during heavy haze days in Beijing winter, Atmos. Chem. Phys., 13, 10171–10183, https://doi.org/10.5194/acp-13-10171-2013, 2013.
Li, Z., Li, L., Zhang, F., Li, D., Xie, Y., and Xu, H.: Comparison of aerosol properties over Beijing and Kanpur: Optical, physical properties and aerosol component composition retrieved from 12 years ground-based Sun-sky radiometer remote sensing data, J. Geophys. Res.-Atmos., 120, 1520–1535, https://doi.org/10.1002/2014JD022593, 2015.
Li, Z., Hou, W., Hong, J., Zheng, F., Luo, D., Wang, J., Gu, X., and Qiao, Y.: Directional Polarimetric Camera (DPC): Monitoring aerosol spectral optical properties over land from satellite observation, J. Quant. Spectrosc. Ra., 218, 21–37, https://doi.org/10.1016/j.jqsrt.2018.07.003, 2018.
Liousse, C., Penner, J. E., Chuang, C., Walton, J. J., Eddleman, H., and Cachier, H.: A global three-dimensional model study of carbonaceous aerosols, J. Geophys. Res., 101, 19411–19432, https://doi.org/10.1029/95JD03426, 1996.
Liu, H., Pinker, R. T., and Holben, B. N.: A global view of aerosols from merged transport models, satellite, and ground observations, J. Geophys. Res.-Atmos., 110, 1–16, https://doi.org/10.1029/2004JD004695, 2005.
Longtin, D. R., Shettle, E. P., Hummel, J. R., and Pryce, J. D.: A Wind Dependent Desert Aerosol Dust Model: Radiative Properties, Scientific Report No.6, 1988.
Lopatin, A., Dubovik, O., Chaikovsky, A., Goloub, P., Lapyonok, T., Tanré, D., and Litvinov, P.: Enhancement of aerosol characterization using synergy of lidar and sun-photometer coincident observations: the GARRLiC algorithm, Atmos. Meas. Tech., 6, 2065–2088, https://doi.org/10.5194/amt-6-2065-2013, 2013.
Maenhaut, W., Salma, I., Cafmeyer, J., Annegarn, H. J., and Andreae, M. O.: Regional atmospheric aerosol composition and sources in the eastern Transvaal, South Africa, and impact of biomass burning, J. Geophys. Res., 101, 23631, https://doi.org/10.1029/95JD02930, 1996.
Mahowald, N. M., Baker, A. R., Bergametti, G., Brooks, N., Duce, R. A., Jickells, T. D., Kubilay, N., Prospero, J. M., and Tegen, I.: Atmospheric global dust cycle and iron inputs to the ocean, Global Biogeochem. Cy., 19, GB4025, https://doi.org/10.1029/2004GB002402, 2005.
Maignan, F., Bréon, F. M., Fédèle, E., and Bouvier, M.: Polarized reflectances of natural surfaces: Spaceborne measurements and analytical modeling, Remote Sens. Environ., 113, 2642–2650, https://doi.org/10.1016/j.rse.2009.07.022, 2009.
Martonchik, J., Diner, D., Kahn, R., Verstraete, M., Pinty, B., Gordon, H., and Ackerman, T.: Techniques for the retrieval of aerosol properties over land and ocean using multiangle data, IEEE T. Geosci. Remote, 36, 1212–1227, 1998.
Middleton, N. J.: A geography of dust storms in South-West Asia, J. Climatol., 6, 183–196, https://doi.org/10.1002/joc.3370060207, 1986a.
Middleton, N. J.: Dust storms in the Middle East, J. Arid Environ., 10, 83–96, https://doi.org/10.1016/S0140-1963(18)31249-7, 1986b.
Miri, A., Ahmadi, H., Ghanbari, A., and Moghaddamnia, A.: Dust Storms Impacts on Air Pollution and Public Health under Hot and Dry Climate, Int. J. Energy Environ., 2, 101–105, 2007.
Miyazaki, Y., Kondo, Y., Takegawa, N., Komazaki, Y., Fukuda, M., Kawamura, K., Mochida, M., Okuzawa, K., and Weber, R. J.: Time-resolved measurements of water-soluble organic carbon in Tokyo, J. Geophys. Res.-Atmos., 111, 1–12, https://doi.org/10.1029/2006JD007125, 2006.
Orr, C., Hurd, F. K., and Corbett, W. J.: Aerosol size and relative humidity, J. Colloid Sci., 13, 472–482, https://doi.org/10.1016/0095-8522(58)90055-2, 1958.
Ota, Y., Higurashi, A., Nakajima, T., and Yokota, T.: Matrix formulations of radiative transfer including the polarization effect in a coupled atmosphere-ocean system, J. Quant. Spectrosc. Ra., 111, 878–894, https://doi.org/10.1016/j.jqsrt.2009.11.021, 2010.
Palmer, K. F. and Williams, D.: Optical properties of water in the near infrared, J. Opt. Soc. Am., 64, 1107, https://doi.org/10.1364/JOSA.64.001107, 1974.
Popp, T., De Leeuw, G., Bingen, C., Brühl, C., Capelle, V., Chedin, A., Clarisse, L., Dubovik, O., Grainger, R., Griesfeller, J., Heckel, A., Kinne, S., Klüser, L., Kosmale, M., Kolmonen, P., Lelli, L., Litvinov, P., Mei, L., North, P., Pinnock, S., Povey, A., Robert, C., Schulz, M., Sogacheva, L., Stebel, K., Zweers, D. S., Thomas, G., Tilstra, L. G., Vandenbussche, S., Veefkind, P., Vountas, M., and Xue, Y.: Development, production and evaluation of aerosol climate data records from European satellite observations (Aerosol_cci), Remote Sens., 8, 421, https://doi.org/10.3390/rs8050421, 2016.
Pósfai, M., Simonics, R., Li, J., Hobbs, P. V., and Buseck, P. R.: Individual aerosol particles from biomass burning in southern Africa1: Compositions and size distributions of carbonaceous particles, J. Geophys. Res.-Atmos., 108, 8483, https://doi.org/10.1029/2002JD002291, 2003.
Prospero, J. M., Ginoux, P., Torres, O., Nicholson, S. E., and Gill, T. E.: Environmental characterization of global sources of atmospheric soil dust identified with the Nimbus 7 Total Ozone Mapping Spectrometer (TOMS) absorbing aerosol product, Rev. Geophys., 40, 1–31, https://doi.org/10.1029/2000RG000095, 2002.
Rashki, A., Rautenbach, C. J. D. W., Eriksson, P. G., Kaskaoutis, D. G., and Gupta, P.: Temporal changes of particulate concentration in the ambient air over the city of Zahedan, Iran, Air Qual. Atmos. Hlth., 6, 123–135, https://doi.org/10.1007/s11869-011-0152-5, 2013.
Reid, J. S., Hobbs, P. V., Ferek, R. J., Blake, D. R., Martins, J. V., Dunlap, M. R., and Liousse, C.: Physical, chemical, and optical properties of regional hazes dominated by smoke in Brazil, J. Geophys. Res.-Atmos., 103, 32059–32080, https://doi.org/10.1029/98JD00458, 1998.
Remer, L., Kaufman, Y., Tanré, D., Mattoo, S., Chu, D., Martins, J., Li, R., Ichoku, C., Levy, R., Kleidman, R., Eck, T., Vermote, E., and Holben, B.: The MODIS aerosol algorithm, products and validation, J. Atmos. Sci., 62, 947–973, 2005.
Román, R., Torres, B., Fuertes, D., Cachorro, V. E., Dubovik, O., Toledano, C., Cazorla, A., Barreto, A., De Frutos, A., and Alados-arboledas, L.: Remote sensing of lunar aureole with a sky camera?: Adding information in the nocturnal retrieval of aerosol properties with GRASP code, Remote Sens. Environ., 196, 238–252, https://doi.org/10.1016/j.rse.2017.05.013, 2017.
Román, R., Benavent-oltra, J. A., Casquero-vera, J. A., Lopatin, A., Cazorla, A., Lyamani, H., Denjean, C., Fuertes, D., Perez-Ramirez, D., Torres, B., Toledano, C., Dubovik, O., Cachorro, V. E., de Frutos, A. M., Olmo, F. J., and Alados-Arboledas, L.: Retrieval of aerosol profiles combining sunphotometer and ceilometer measurements in GRASP code, Atmos. Res., 204, 161–177, https://doi.org/10.1016/j.atmosres.2018.01.021, 2018.
Roujean, J.-L., Leroy, M., and Deschamps, P.-Y.: A bidirectional reflectance model of the Earth's surface for the correction of remote sensing data, J. Geophys. Res., 97, 20455, https://doi.org/10.1029/92JD01411, 1992.
Russell, P., Kacenelenbogen, M., Livingston, J., Hasekamp, O., Burton, S., Schuster, G., Johnson, M., Knobelspiesse, K., Redemann, J., Ramachandran, S., and Holben, B.: A multiparameter aerosol classification method and its application to retrievals from spaceborne polarimetry, J. Geophys. Res., 119, 9838–9863, https://doi.org/10.1002/2013JD021411, 2014.
Schkolnik, G., Chand, D., Hoffer, A., Andreae, M. O., Erlick, C., Swietlicki, E., and Rudich, Y.: Constraining the density and complex refractive index of elemental and organic carbon in biomass burning aerosol using optical and chemical measurements, Atmos. Environ., 41, 1107–1118, https://doi.org/10.1016/j.atmosenv.2006.09.035, 2007.
Schmeisser, L., Andrews, E., Ogren, J. A., Sheridan, P., Jefferson, A., Sharma, S., Kim, J. E., Sherman, J. P., Sorribas, M., Kalapov, I., Arsov, T., Angelov, C., Mayol-Bracero, O. L., Labuschagne, C., Kim, S.-W., Hoffer, A., Lin, N.-H., Chia, H.-P., Bergin, M., Sun, J., Liu, P., and Wu, H.: Classifying aerosol type using in situ surface spectral aerosol optical properties, Atmos. Chem. Phys., 17, 12097–12120, https://doi.org/10.5194/acp-17-12097-2017, 2017.
Schnaiter, M., Gimmler, M., Llamas, I., Linke, C., Jäger, C., and Mutschke, H.: Strong spectral dependence of light absorption by organic carbon particles formed by propane combustion, Atmos. Chem. Phys., 6, 2981–2990, https://doi.org/10.5194/acp-6-2981-2006, 2006.
Schuster, G. L., Lin, B., and Dubovik, O.: Remote sensing of aerosol water uptake, Geophys. Res. Lett., 36, L03814, https://doi.org/10.1029/2008GL036576, 2009.
Schuster, G. L., Dubovik, O., Holben, B. N., and Clothiaux, E. E.: Inferring black carbon content and specific absorption from Aerosol Robotic Network (AERONET) aerosol retrievals, J. Geophys. Res., 110, D10S17, https://doi.org/10.1029/2004JD004548, 2005.
Schuster, G. L., Dubovik, O., and Arola, A.: Remote sensing of soot carbon – Part 1: Distinguishing different absorbing aerosol species, Atmos. Chem. Phys., 16, 1565–1585, https://doi.org/10.5194/acp-16-1565-2016, 2016a.
Schuster, G. L., Dubovik, O., Arola, A., Eck, T. F., and Holben, B. N.: Remote sensing of soot carbon – Part 2: Understanding the absorption Ångström exponent, Atmos. Chem. Phys., 16, 1587–1602, https://doi.org/10.5194/acp-16-1587-2016, 2016b.
Shi, Z., Krom, M. D., Jickells, T. D., Bonneville, S., Carslaw, K. S., Mihalopoulos, N., Baker, A. R., and Benning, L. G.: Impacts on iron solubility in the mineral dust by processes in the source region and the atmosphere: A review, Aeolian Res., 5, 21–42, https://doi.org/10.1016/j.aeolia.2012.03.001, 2012.
Sokolik, I. N. and Toon, O. B.: Incorporation of mineralogical composition into models of the radiative properties of mineral aerosol from UV to IR wavelengths, J. Geophys. Res.-Atmos., 104, 9423–9444, https://doi.org/10.1029/1998JD200048, 1999.
Stone, E., Schauer, J., Quraishi, T. A., and Mahmood, A.: Chemical characterization and source apportionment of fine and coarse particulate matter in Lahore, Pakistan, Atmos. Environ., 44, 1062–1070, https://doi.org/10.1016/j.atmosenv.2009.12.015, 2010.
Streets, D. G., Gupta, S., Waldhoff, S. T., Wang, M. Q., Bond, T. C., and Yiyun, B.: Black carbon emissions in China, Atmos. Environ., 35, 4281–4296, https://doi.org/10.1016/S1352-2310(01)00179-0, 2001.
Sun, H., Biedermann, L., and Bond, T. C.: Color of brown carbon: A model for ultraviolet and visible light absorption by organic carbon aerosol, Geophys. Res. Lett., 34, L17813, https://doi.org/10.1029/2007GL029797, 2007.
Swap, R., Garstang, M., Macko, S. A., Tyson, P. D., Maenhaut, W., Artaxo, P., Kållberg, P., and Talbot, R.: The long-range transport of southern African aerosols to the tropical South Atlantic, J. Geophys. Res.-Atmos., 101, 23777–23791, https://doi.org/10.1029/95JD01049, 1996.
Tang, I. N.: Chemical and size effects of hygroscopic aerosols on light scattering coefficients, J. Geophys. Res.-Atmos., 101, 19245–19250, https://doi.org/10.1029/96JD03003, 1996.
Tang, I. N.: Phase transformation and growth of aerosol particles composed of mixed salts, J. Aerosol Sci., 7, 7361–7371, https://doi.org/10.1016/0021-8502(76)90022-7, 1976.
Tang, I. N. and Munkelwitz, H. R.: Simultaneous determination of refractive index and density of an evaporating aqueous solution droplet, Aerosol Sci. Tech., 15, 201–207, https://doi.org/10.1080/02786829108959527, 1991.
Tang, I. N. and Munkelwitz, H. R.: Composition and temperature dependence of the deliquescence properties of hygroscopic aerosols, Atmos. Environ., 27, 467–473, https://doi.org/10.1016/0960-1686(93)90204-C, 1993.
Tang, I. N. and Munkelwitz, H. R.: Water activities, densities, and refractive indices of aqueous sulfates and sodium nitrate droplets of atmospheric importance, J. Geophys. Res., 99, 18801–18808, 1994.
Tang, I. N., Wong, W. T., and Munkelwitz, H. R.: The relative importance of atmospheric sulfates and nitrates in visibility reduction, Atmos. Environ., 15, 2463–2471, 1981.
Tanré, D., Bréon, F. M., Deuzé, J. L., Dubovik, O., Ducos, F., François, P., Goloub, P., Herman, M., Lifermann, A., and Waquet, F.: Remote sensing of aerosols by using polarized, directional and spectral measurements within the A-Train: the PARASOL mission, Atmos. Meas. Tech., 4, 1383–1395, https://doi.org/10.5194/amt-4-1383-2011, 2011.
Todd, M. C., Washington, R., Martins, J. V., Dubovik, O., Lizcano, G., M'Bainayel, S., and Engelstaedter, S.: Mineral dust emission from the Bodélé Depression nothern Chad, during BoDEx 2005, J. Geophys. Res.-Atmos., 112, 1–12, https://doi.org/10.1029/2006JD007170, 2007.
Toon, O. B., Pollack, J. B., and Khare, B. N.: The optical constants of several atmospheric aerosol species: atmmonium sulfate, aluminum oxide, and sodium chloride, J. Geophys. Res., 81, 5733–5748, 1976.
Triaud, A. H. M. J.: Earth observation data group: aerosol refractive index archive, available at: http://eodg.atm.ox.ac.uk/ARIA/data?Minerals/Hematite/(Triaud_2005)/hematite_Triaud_2005.ri (last access: 28 October 2019), 2005.
Tsekeri, A., Lopatin, A., Amiridis, V., Marinou, E., Igloffstein, J., Siomos, N., Solomos, S., Kokkalis, P., Engelmann, R., Baars, H., Gratsea, M., Raptis, P. I., Binietoglou, I., Mihalopoulos, N., Kalivitis, N., Kouvarakis, G., Bartsotas, N., Kallos, G., Basart, S., Schuettemeyer, D., Wandinger, U., Ansmann, A., Chaikovsky, A. P., and Dubovik, O.: GARRLiC and LIRIC: strengths and limitations for the characterization of dust and marine particles along with their mixtures, Atmos. Meas. Tech., 10, 4995–5016, https://doi.org/10.5194/amt-10-4995-2017, 2017.
Usher, C. R., Michel, A. E., and Grassian, V. H.: Reactions on mineral dust, Chem. Rev., 103, 4883–4939, https://doi.org/10.1021/cr020657y, 2003.
Voss, K. J., Morel, A., and Antoine, D.: Detailed validation of the bidirectional effect in various Case 1 waters for application to ocean color imagery, Biogeosciences, 4, 781–789, https://doi.org/10.5194/bg-4-781-2007, 2007.
Wagner, R., Ajtai, T., Kandler, K., Lieke, K., Linke, C., Müller, T., Schnaiter, M., and Vragel, M.: Complex refractive indices of Saharan dust samples at visible and near UV wavelengths: a laboratory study, Atmos. Chem. Phys., 12, 2491–2512, https://doi.org/10.5194/acp-12-2491-2012, 2012.
Wang, L., Li, Z., Tian, Q., Ma, Y., Zhang, F., Zhang, Y., Li, D., Li, K., and Li, L.: Estimate of aerosol absorbing components of black carbon, brown carbon, and dust from ground-based remote sensing data of sun-sky radiometers, J. Geophys. Res.-Atmos., 118, 6534–6543, https://doi.org/10.1002/jgrd.50356, 2013.
Wanner, W., Li, X., and Strahler, A.: On the derivation of kernels for kernel-driven models of bidirectional reflectance, J. Geophys. Res., 100, 21077–21089, 1995.
Waquet, F., Cornet, C., Deuzé, J.-L., Dubovik, O., Ducos, F., Goloub, P., Herman, M., Lapyonok, T., Labonnote, L. C., Riedi, J., Tanré, D., Thieuleux, F., and Vanbauce, C.: Retrieval of aerosol microphysical and optical properties above liquid clouds from POLDER/PARASOL polarization measurements, Atmos. Meas. Tech., 6, 991–1016, https://doi.org/10.5194/amt-6-991-2013, 2013.
Washington, R. and Todd, M. C.: Atmospheric controls on mineral dust emission from the Bodélé Depression, Chad: The role of the low level jet, Geophys. Res. Lett., 32, 1–5, https://doi.org/10.1029/2005GL023597, 2005.
Washington, R., Todd, M., Middleton, N. J., and Goudie, A. S.: Dust-storm source areas determined by the total ozone monitoring spectrometer and surface observations, Ann. Assoc. Am. Geogr., 93, 297–313, https://doi.org/10.1111/1467-8306.9302003, 2003.
Washington, R., Todd, M. C., Engelstaedter, S., Mbainayel, S., and Mitchell, F.: Dust and the low-level circulation over the Bodélé Depression, Chad: Observations from BoDEx 2005, J. Geophys. Res.-Atmos., 111, 1–15, https://doi.org/10.1029/2005JD006502, 2006.
Weber, R. J., Sullivan, A. P., Peltier, R. E., Russell, A., Yan, B., Zheng, M., de Grouw, J., Warneke, C., Brock, C., Holloway, J. S., Atlas, E. L., and Edgerton, E.: A study of secondary organic aerosol formation in the anthropogenic-influenced southeastern United States, J. Geophys. Res. Atmos., 112, 1–13, https://doi.org/10.1029/2007JD008408, 2007.
Xie, Y., Li, Z., Li, L., Wang, L., Li, D., Chen, C., Li, K., and Xu, H.: Study on influence of different mixing rules on the aerosol components retrieval from ground-based remote sensing measurements, Atmos. Res., 145, 267–278, https://doi.org/10.1016/j.atmosres.2014.04.006, 2014.
Yu, H., Dickinson, R. E., Chin, M., Kaufman, Y. J., Holben, B. N., Geogdzhayev, I. V., and Mishchenko, M. I.: Annual cycle of global distributions of aerosol optical depth from integration of MODIS retrievals and GOCART model simulations, J. Geophys. Res.-Atmos., 108, 1–14, https://doi.org/10.1029/2002JD002717, 2003.
Yu, H., Dickinson, R. E., Chin, M., Kaufman, Y. J., Zhou, M., Zhou, L., Tian, Y., Dubovik, O., and Holben, B. N.: Direct radiative effect of aerosols as determined from a combination of MODIS retrievals and GOCART simulations, J. Geophys. Res.-Atmos., 109, 1–13, https://doi.org/10.1029/2003JD003914, 2004.
Yu, H., Kaufman, Y. J., Chin, M., Feingold, G., Remer, L. A., Anderson, T. L., Balkanski, Y., Bellouin, N., Boucher, O., Christopher, S., DeCola, P., Kahn, R., Koch, D., Loeb, N., Reddy, M. S., Schulz, M., Takemura, T., and Zhou, M.: A review of measurement-based assessments of the aerosol direct radiative effect and forcing, Atmos. Chem. Phys., 6, 613–666, https://doi.org/10.5194/acp-6-613-2006, 2006.
Zhang, X. Y., Gong, S. L., Shen, Z. X., Mei, F. M., Xi, X. X., Liu, L. C., Zhou, Z. J., Wang, D., Wang, Y. Q., and Cheng, Y.: Characterization of soil dust aerosol in China and its transport and distribution during 2001 ACE-Asia: 1. Network observations, J. Geophys. Res., 108, 4261, https://doi.org/10.1029/2002JD002632, 2003.
Zhang, J., Reid, J. S., Westphal, D. L., Baker, N. L., and Hyer, E. J.: A system for operational aerosol optical depth data assimilation over global oceans, J. Geophys. Res.-Atmos., 113, 1–13, https://doi.org/10.1029/2007JD009065, 2008.
Zhang, X. Y., Wang, Y. Q., Wang, D., Gong, S. L., Arimoto, R., Mao, L. J., and Li, J.: Characterization and sources of regional-scale transported carbonaceous and dust aerosols from different pathways in coastal and sandy land areas of China, J. Geophys. Res.-Atmos., 110, 1–13, https://doi.org/10.1029/2004JD005457, 2005.
Zhang, X. Y., Wang, Y .Q., Zhang, X. C., Guo, W., and Gong, S. L.: Carbonaceous aerosol composition over various regions of China during 2006, J. Geophys. Res.-Atmos., 113, 1–10, https://doi.org/10.1029/2007JD009525, 2008.
Zhang, X. Y., Wang, Y. Q., Niu, T., Zhang, X. C., Gong, S. L., Zhang, Y. M., and Sun, J. Y.: Atmospheric aerosol compositions in China: spatial/temporal variability, chemical signature, regional haze distribution and comparisons with global aerosols, Atmos. Chem. Phys., 12, 779–799, https://doi.org/10.5194/acp-12-779-2012, 2012.
Zhang, Y., Li, Z., Sun, Y., Lv, Y., and Xie, Y.: Estimation of atmospheric columnar organic matter (OM) mass concentration from remote sensing measurements of aerosol spectral refractive indices, Atmos. Environ., 179, 107–117, https://doi.org/10.1016/j.atmosenv.2018.02.010, 2018.