the Creative Commons Attribution 4.0 License.
the Creative Commons Attribution 4.0 License.
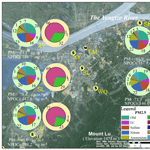
Non-polar organic compounds in autumn and winter aerosols in a typical city of eastern China: size distribution and impact of gas–particle partitioning on PM2.5 source apportionment
Deming Han
Qingyan Fu
Song Gao
Yingge Ma
Liping Qiao
Hao Xu
Shan Liang
Pengfei Cheng
Xiaojia Chen
Yong Zhou
Jian Zhen Yu
Aerosol-associated non-polar organic compounds (NPOCs), including 15 polycyclic aromatic hydrocarbons (PAHs), 30 n-alkanes, 2 iso-alkanes, 5 hopanes and 5 steranes, were identified and quantified in PM2.5 samples using the thermal desorption–gas chromatography–mass spectrometry (TD–GC–MS) method. The samples were mainly collected in autumn and winter in a typical city of eastern China. The total concentrations of NPOCs were 31.7–388.7 ng m−3, and n-alkanes were the most abundant species (67.2 %). The heavy-molecular-weight PAHs (four- and five-ring) contributed 67.9 % of the total PAHs, and the middle-chain-length n-alkanes (C25–C34) were the most abundant (72.3 %) in n-alkanes. PAHs and n-alkanes were mainly distributed in the 0.56–1.00 µm fraction, while ∑ (hopanes + steranes) were associated with the 0.32–1.00 µm fraction, suggesting condensation of combustion products was their important origin. The ratio–ratio plots indicated that NPOCs in the local area were affected by photochemical degradation. To reduce the uncertainty caused by only particle NPOC data for source apportionment, the particle and predicted gaseous-phase NPOCs, incorporated with other PM2.5 compound were used as input data for the positive matrix factorization (PMF) model. Eight factors were extracted for both cases: secondary aerosol formation, vehicle exhaust, industrial emission, coal combustion, biomass burning, ship emission, and dust and light NPOCs. These findings highlight the emissions from different aerosol-associated NPOC origins, which caused different size-specific distributions, photodegradation and gas–particle partitioning, which further affect PM2.5 source apportionment. Considering these effects on organic tracers will help us accurately identify the potential sources of aerosols and then asses the contributions from each source.
- Article
(9600 KB) -
Supplement
(536 KB) - BibTeX
- EndNote
In recent years, severe atmospheric pollution characterized by haze episodes has been a recurring problem in developing countries, affecting visibility, optical radiation and human health (Yadav et al., 2013; Wang et al., 2015; Shen et al., 2015; Sulong et al., 2017). China has experienced numerous severe and long-lasting haze episodes since winter in 2013, which has affected over 600 million local residents and covered a quarter of the country's land area (Huang et al., 2014; Hao and Liu, 2015). In essence, a haze episode is caused by the distribution of particulate matter with different sizes in atmosphere, leading to a decrease in visibility (Xie et al., 2017). Carbonaceous aerosols contain a large amount of particulate matter, accounting for 30–50 % of PM2.5 mass concentrations (Yadav et al., 2013; Wang et al., 2016; Ma et al., 2018). Carbonaceous aerosols have a significant influence on environmental and physical processes, including dry and wet deposition, cloud condensation nucleation and heterogeneous reactions (Feng et al., 2006; Li et al., 2017).
Since carbonaceous aerosols can affect the ambient environment significantly, it is crucial to investigate aerosol-associated organic compounds. Because non-polar organic compounds (NPOCs) can provide specific information on the identification of aerosol sources, they are now of special interest to researchers (Rajput and Sarin, 2014; Wang et al., 2016). n-Alkanes, polycyclic aromatic hydrocarbons (PAHs), hopanes and steranes are four typical NPOC species and are frequently used to apportion sources of ambient particulate matter (Xu et al., 2013; Zhao et al., 2016). n-Alkanes are emitted from natural and anthropogenic activities, including particulate abrasion products from leaf surfaces of vegetation and fossil fuel combustion. Notably, fossil fuel combustion is characterized by the release of C22–C25 n-alkanes, while particulate abrasion products from leaf surfaces are characterized by the predominance of > C29 odd n-alkanes (Yadav et al., 2013). PAHs are mainly emitted from anthropogenic activities, including biomass burning, coal combustion, fossil fuel combustion and industrial processes (Ma et al., 2011; Zhang et al., 2015). Hopanes and steranes are from unburned fossil fuels and lubricant oils, and they are often found in vehicle exhausts, ship emissions and coal combustion emissions.
PAHs are typical semi-volatile organic compounds, which can partition between gas and particle phases in the ambient atmosphere (He and Balasubramanian, 2009; Ma et al., 2011). Recently, research has shown that n-alkanes, hopanes and steranes are also semi-volatile and subject to gas–particle partitioning (Xie et al., 2013, 2014; Wang et al., 2016). Additionally, another crucial factor affecting particle-bound concentrations of NPOCs is their aerodynamic diameter (Wang et al., 2009). The size-specific distributions of compounds are dependent on their physical–chemical properties, gas–particle partitioning and photodegradation (Okonski et al., 2014; X. Chen et al., 2016). Thus, characterization of the size-specific distribution of NPOCs is crucial for understanding their formation, assessing their possible environmental fate and offering proper management (Kleeman et al., 2008; Wang et al., 2009). Although several studies have focused on the size distribution of PAHs, much less attention has been given to other NPOC species (Hien et al., 2007; Wang et al., 2009).
NPOCs are typically assumed to be stable and nonreactive (Feng et al., 2006; Ma et al., 2011). However, recent research has shown that NPOCs can be oxidized by •OH radicals, RO2• radicals and O3 over atmospherically relevant timescales (Xie et al., 2013; Wang et al., 2016). PAHs, n-alkanes, hopanes and steranes can undergo photochemical oxidation, increasing the production of secondary organic aerosol (Robinson et al., 2006; May et al., 2012). For example, PAHs can react with •OH radicals, and through the addition of carbonyl groups to the carbon skeleton the free ends of C–C scission products remain tethered together, which prevents fragmentation and leads to more functional groups on the single product (May et al., 2012). Hence, the low-volatility species which can condense into the particle phase are formed and subsequently undergo oligomerization reactions following condensation.
To develop strategies for controlling atmospheric pollution caused by particulate matter, receptor-based models (e.g., positive matrix factorization, PMF) have been widely applied to quantitatively apportion sources of particulate matter (Wang et al., 2009; Li et al., 2016; Huang et al., 2017). However, the output factors of the receptor model are not necessarily emission sources, because there exist some atmospheric processes like photodegradation or gas–particle partitioning. Considering the gas–particle partitioning of NPOCs, Xie et al. (2013) adopted both gaseous and particulate NPOCs in the PMF model and successfully extracted seven factors. More recently, Wang et al. (2016) used data of NPOCs combined with those of organic/elemental compounds (OC/EC), inorganic compounds and elemental compounds as input for the PMF model, and they found that total (gas + particle)-bound concentrations enabled more reasonable source profiles than the single particle phase.
In this paper, we conducted a comprehensive study on PM2.5-associated NPOCs in a typical city of eastern China. Specifically, we (1) quantified the concentrations of NPOCs (n-alkanes, PAHs, hopanes and steranes) through the thermal desorption–gas chromatography–mass spectrometry (TD–GC–MS) method, (2) determined the size-specific distributions of NPOCs from 0.01 to 18 µm, (3) analyzed the degradation of NPOCs, and (4) explored the gas–particle partitioning of NPOCs and assessed its effects on PM2.5 source apportionment.
2.1 Sampling sites and sample collection
Jiujiang is located at 113∘57′–116∘53′ E and 28∘47′–30∘06′ N with an elevation of 32 m in the Jiangxi Province of eastern China (Fig. 1). It is characterized by a subtropical monsoon climate. Jiujiang is the second largest city in Jiangxi Province, with approximately 4.83 million residents and over 700 000 motor vehicles in 2015. Preliminary statistics indicate that the gross industrial standard coal consumption in Jiujiang amounted to 7.80 million t in 2015. In Jiujiang, the petrochemical industry, which can process approximately 5 million t of crude oil per year, is located at the northeast part of the city and in an upwind direction. In addition, Mount Lu (elevation of 1474 m), located at the south of Jiujiang, blocks the transport of air masses from the North China Plain region to the southern area, leading to the accumulation of particulate matter in the city area, especially in winter seasons.
Five PM2.5 sampling sites were selected for routine air quality measurements in Jiujiang (Table 1), including Shihua (SH), Xiyuan (XY), Shili (SL), Wuqierqi (WQ) and Jiujiangxian (JJ). All PM2.5 filter samples were collected using median-volume air samplers (YH-5, Qingdao, China), at a flow rate of 100 L min−1. Particles were collected on quartz fiber filters (GE Whatman, 1851-090, England, UK) with a diameter of 90 mm, each for a duration of 23 h. Prior to use, these filters were pre-baked at 550 ∘C for 4 h to eliminate residual organic compounds. PM2.5 sampling at these five sites was performed synchronously for five continuous days per month from September to December 2016, in addition to the extensive sampling period (1–16 December). A total of 137 PM2.5 valid samples were collected, as 18 samples were invalid or missing due to the bad weather or power problems during the sampling period.
The sampling site of size-specific aerosols was located at a five-story building of the Jiujiang environmental monitoring station (EM site). Airborne particle samples were collected for 23 h using a nano-micro-orifice uniform deposition impactor (MOUDI) sampler (model 122R, MSP Corp, USA), at an air flow rate of 30 L min−1. Detailed instrument operation, quality assurance and the control method can be found in our previous work (X. Chen et al., 2016; Han et al., 2018). Briefly, this sampler can collect particles within 13 size fractions: 0.01–0.018, 0.018–0.032, 0.032–0.056, 0.056–0.1, 0.1–0.18, 0.18–0.32, 0.32–0.56, 0.56–1.0, 1.0–1.8, 1.8–3.1, 3.1–6.2, 6.2–9.9 and 9.9–18 µm. Prior to sampling, each filter tray was washed with distilled water and ethanol. Two kinds of quartz fiber filters of diameters of 47 and 90 mm were used to collect particles with diameters of 0.056–18.0 and 0.010–0.056 µm, respectively. All the filters were pre-baked at 550 ∘C for 4 h, wrapped in aluminum foil and then sealed in clean polyethylene bags. Leak and flow tests were conducted according to manufacturer's instructions: a leak test was done with a duration of 60 s with a leak rate < 10 Pa s−1 at an initial pressure of 55±5 kPa. The mass concentrations of particulate matter were determined by subtracting the filter weight before and after sampling. A well-calibrated digital balance within a precision of 0.01 mg (Sartorius SE2, Germany) was used. The collected particle samples were stored at controlled temperature (−20 ∘C) and relative humidity until analysis.
2.2 Analysis of aerosol samples using TD–GC–MS
A total of 57 NPOC species (Table S1 and Fig. S1 in the Supplement) were identified by using an in-injection port thermal desorption unit (TDU, Shimadzu, Japan), coupled with gas chromatography–mass spectrometry (GC–MS, QP2010 Plus, Shimadzu, Japan). Compared with the traditional solvent extraction method, the TD–GC–MS method (Ho and Yu, 2004; Ho et al., 2008) has advantages such as solvent and sample filtration, labor saving, and less contamination from solvent impurities. A filter aliquot (1 cm2) from quartz fiber was cut into small pieces on a clean glass dish, and then they were inserted into the TD tubes (CAMSCO, USA). Both sides of the samples were surrounded with pre-baked, silane-treated glass-wool plugs to enhance the cryofocusing of the analytes and prevent heavy and polar compounds from entering the GC column. The internal standards of n-tetracosane d50 (n-C24D50), naphthalene-d8, acenaphthene-d10, phenanthrene-d10, and chrysene-d12 were spiked into each sample through a pipette with a long thin tip. This was done to account for the loss of components from sample filters associated with the instrument instability due to changes in laboratory environmental conditions. After the evaporation of solvent from the internal standard was conducted via air drying for several seconds, the TD tubes were capped and put into a sampler holder.
The sample processing time in TD tube was set to 45 min, and the TD tube was electronically cooled to −10 ∘C. The desorption and interface temperatures were set to 295 and 280 ∘C, respectively. Helium (99.999 %) was used as carrier gas for the thermally desorbed organic compounds, with a gas flow rate of 1.12 mL min−1. GC was used under splitless injection mode, and the initial oven temperature was set to 40 ∘C with an isothermal hold time of 5 min. Stepwise programmed linear temperature ramping included 10 ∘C min−1 to 120 ∘C (held for 2 min), and then 20 ∘C min−1 to 300 ∘C (kept for 20 min). An Rtx-5MS capillary column (Restek, USA; L × I.D. × D.F.: 30 m × 0.25 mm × 0.25 µm) was used to separate desorbed organic compounds. The MS was operated in scan mode with a mass range m∕z of 50–500 and scanned at 0.5 s scan−1 (Ho et al., 2008; Yadav et al., 2013). The ion was produced from electronic impact ionization (EI) at 70 eV and then was separated by a high-performance quadrupole mass filter. Species identification was achieved by comparing the mass spectra and retention times of the chromatographic peak with the corresponding authentic standards.
2.3 Determination of OC/EC and other constituents
OC and EC were analyzed (a round punch of 0.538 cm2) using the thermal-optical-transmittance (TOT) method (NIOSH protocol, Desert Research Institute, USA) (Han et al., 2018). The instrument included a temperature- and atmosphere-controlled oven and a laser of 680 nm wavelength to generate an operational EC/OC split. The instrument was heated stepwise from start to 250 ∘C (60 s), 500 ∘C (60 s), 650 ∘C (60 s) and finally 850 ∘C (90 s) in the helium atmosphere for OC volatilization, and from start to 550 ∘C (45 s), 650 ∘C (60 s), 750 ∘C (60 s) and finally 850 ∘C (80 s) in the helium atmosphere containing 2 % oxygen for EC oxidation.
Elemental compositions, including Na, K, Ca, Mg, P, Fe, Ti, Al, Pb, Cu and Zn, were determined by energy dispersive X-ray fluorescence (ED-XRF) spectrometry (Epsilon 5, the Netherlands). Water-soluble inorganic ions, including cations (Na+, K+, Mg2+, Ca2+, ) and anions (Cl−, and , ), were detected by ion chromatography (IC, ISC-90, Dionex, USA). The detailed experimental procedure of OC/EC, elemental composition and inorganic ions analysis could be found in Li et al. (2017) and Han et al. (2018).
2.4 Quality assurance and quality control
Prior to sampling in each site, the five PM2.5 samplers were calibrated by an environmental monitoring station. PM2.5 samplers were placed on the building rooftop of the Jiujiang environmental monitoring station, each pair within a distance of < 3 m. Field blanks were collected by keeping blank filters in the sampler for the same duration at the sampling site. Additionally, both transport and laboratory blank filters were analyzed, and all the data reported in this study were corrected according to the results.
The NPOCs standards used were the National Institute of Standards and Technology (NIST, USA) Standard Reference Materials (SRM), including SRM 2260A, SRM 1494 and SRM 2266 for 15 PAHs, 30 n-alkanes and 10 hopanes/steranes, respectively. Six-point calibration curves of NPOCs were constructed by adopting different calibration solutions to the field blank filters and loaded into TD tubes. The calibration solutions were 0, 0.05, 0.25, 0.50, 1.0 and 2.0 µg L−1 for PAHs, hopanes and steranes, as well as 0, 0.10, 1.0, 5.0, 10.0 and 20.0 µg L−1 for n-alkanes. The same concentration of internal standards was used for each level of the calibration solution. Ratios of the average peak area values “A” for represented samples “S” to the corresponding internal standard “IS”, namely AS ∕ AIS, and ratios of average concentration “C” for represented samples to the corresponding internal standard (CS ∕ CIS) were generated using Shimadzu software, with the slope of the curve being the RRF (relative response factor). The calibration curves for most target compounds were highly linear (r2 > 0.99), demonstrating the consistency and reproducibility of this method. The standard deviation (S) was calculated by using seven replicates of the second lowest standard solution, and the method detection limit (MDL, MDL = 3.143 × S) was determined at the 99 % confidence level.
Recovery experiments were conducted to improve the desorption of targeted compounds from filters and experimental detection. The analytical recovery was calculated by spiking a known amount of the SRM solution to blank filter, and most compounds were recovered with a recovery efficiency > 90 % except for several light-molecular-weight species. The accuracy of the method was evaluated by the reproducibility of the standard and selected samples were ascertained by processing in quintuplicate; the results suggest the analytical precision was better than 5 %.
2.5 Diagnostic parameters and isomeric ratios
Different diagnostic parameters were adopted in this study to explore natural and anthropogenic contributions. The parameters include the carbon preference index (CPI), the carbon number of the most abundant n-alkane (Cmax), contributions from natural wax n-alkanes (WNA %) and petrogenic n-alkanes (PNA %), higher plant n-alkane average chain length (ACL) and molecular diagnostic ratios (MDRs) for PAHs (Yadav et al., 2013; Zhao et al., 2016).
-
CPI is defined as the ratio of the total concentration of odd n-alkanes to that of even n-alkanes (Eq. 1). It reflects the comparison between natural and anthropogenic contributions.
where i and j represent odd and even carbon numbers, respectively, and C represents the concentrations of carbon n-alkanes.
-
WNA % is calculated as Eq. (2). Note that the negative value of [C] was replaced by zero.
-
Aerosol-associated n-alkanes can originate from plant wax or petroleum combustion, and the percentage of petrogenic n-alkanes (PNA %) can be calculated as
-
Cmax represents the carbon number of the most abundant n-alkane, and it is regarded as the most important indicator of biogenic inputs. In general, Cmax = 31 indicates effects from leaf abrasion products, whereas Cmax = 29 implies effects from road dust, as well as vehicle and industrial emissions.
-
ACL can indicate emissions of n-alkanes from plants which are related to temperature and humidity. It is defined as counted carbon atoms per carbon molecule, depending on the odd n-alkanes from higher plant n-alkanes. ACL can be estimated through Eq. (4) as follows:
-
MDRs for PAH source apportionment include the ANT ∕ PHE (anthracene ∕ phenanthrene) ratio, PYR ∕ FLU (pyrene ∕ fluoranthene) ratio, IcdP ∕ BghiP (indeno[1, 2, 3-cd]pyrene ∕ benzo[ghi]perylene) ratios (namely, the ANT ∕ (ANT + PHE) ratio and FLU ∕ (FLU + PYR) ratio), and IcdP ∕ (IcdP + BghiP). If ANT ∕ (ANT + PHE) < 0.1, petroleum origins are suggested; if the ratio > 0.1, pyrogenic sources are indicated. If FLU ∕ (FLU + PYR) < 0.4, petroleum sources are suggested; if the ratio > 0.4, pyrogenic sources are indicated (Kuang et al., 2011; P. F. Chen et al., 2016). Note that the ratio ranging from 0.4 to 0.5 also suggests fuel combustion. If 0.2 < IcdP ∕ (IcdP + BghiP) < 0.5, fuel combustion is suggested; if the ratio > 0.5, grass, wood and coal combustion should have contributed to particulate matter (P. F. Chen et al., 2016).
2.6 Gas–particle partitioning model
Gas–particle partitioning is an important mechanism that affects the fate and transport of NPOCs (Pankow, 1994; Kim et al., 2011). To understand the partitioning behavior of NPOCs, we evaluated the distribution of NPOCs between gas and particle phases in the atmosphere. The gas–particle coefficient Kp (m3 µg−1) for each compound species was calculated using the following equations:
where F and A represent the concentrations of NPOC in the gas and particle phases (ng m−3), respectively; PM is the measured mass concentration of particulate matter, i.e., PM2.5 in this study (µg m−3); R is the ideal gas constant (8.314 m3 Pa−1 K−1 mol−1); T is the ambient temperature (K); MWOM is the mean molecular weight (g mol−1) and is 200 g mol−1 in this study (Xie et al., 2013); ξOM is the activity coefficient of each compound in the absorbing phase and assumed to be unity in this calculation (Xie et al., 2013); and are subcooled vapor pressures at T and 298 K, respectively (Pa); and ΔH0 is the vaporization enthalpy of the liquid at 298.15 K. The measured and ΔH0 were extracted from previous literature (And and Hanshaw, 2004; Wang et al., 2016).
The total concentration (S) of each NPOC in the gas and particle phases was calculated as Eq. (7):
Also, Junge–Pankow model was further used to investigate gas–particle partitioning. In this model, the ratio (φ) of the concentration of NPOC species in the particle phase to the total NPOC concentration was calculated as follows:
where θ represents the particle surface area per unit volume of air (cm2 cm−3) and c is a constant which depends on the thermodynamics of the adsorption process, molecular weight, and surface properties (Pa cm−1). In this study, c = 17.2 Pa cm−1, and θ is , and (cm2 cm−3) for urban area, rural area and background, respectively (And and Hanshaw, 2004; Xie et al., 2013).
3.1 Abundance of PM2.5 and NPOCs
The statistical summary and the abundance of measured PM2.5 and NPOC species are shown in Fig. 2 and Table S2. The daily average PM2.5 concentration in all sampling sites was 79.3±37.7 µg m−3. The average OC and EC concentrations were 13.8±6.9 and 6.3±2.2 µg m−3, respectively. Organic matter (OM) was estimated to be 1.4 times the OC concentration (Feng et al., 2006; Huang et al., 2014), which was the most abundant component in PM2.5, accounting for 18.8–27.8 % of the total mass in this study. Following OM, , and were also abundant, accounting for 19.9–22.6, 16.4–18.1 and 10.4–13.7 % of PM2.5, respectively.
A total of 57 NPOCs were identified in this study (Table 2), including 30 n-alkanes, 2 iso-alkanes, 15 PAHs, 5 hopanes and 5 steranes. Their daily average concentrations ranged from 31.7 to 388.7 ng m−3 (an average of 155.9±55.4 ng m−3), accounting for 0.4–2.4 % of OM. This was consistent with the measurement results (0.1–4.2 %) of NPOCs in the Pearl River Delta (PRD, China) (Wang et al., 2016) over a 2-year period from 2011 to 2012. Similarly, Zhao et al. (2016) found that the NPOCs varied from 19.8 to 288.2 ng m−3, accounting for 0.8–1.7 % of OM in the South China Sea from September to October 2013. n-Alkanes were the most abundant NPOCs, with a concentration of 105.3±55.1 ng m−3, accounting for 67.2 % of NPOC concentration. PAHs were the second most abundant species, on average accounting for 29.2 % of NPOC concentration. Hopanes and steranes were minor constituents, with average concentrations of 3.6±3.0 and 1.8±1.3 ng m−3, respectively.
When comparing with other NPOCs measurements in China, Li et al. (2013) reported a similar level in that the daily concentration of n-alkanes, PAHs and hopanes were 97.9, 13.5 and 21.5 ng m−3 in Hong Kong in winter, respectively. Xu et al. (2013) measured mean n-alkanes and PAH concentrations of 48.1±20.8 and 19.0±17.5 ng m−3 in urban Guangzhou during the 16th Asian Games, while Feng et al. (2006) found that the daily concentrations of n-alkanes and PAHs ranged from 32.9 to 342.9 and from 7.8 to 151.1 ng m−3 in urban Shanghai in 2002–2003, respectively. Thus, this study finds generally higher PM2.5-associated NPOC concentrations measured in Jiujiang compared to other measurements, which may be due to this study being mainly conducted in the cold season when severe atmospheric pollution episodes (including haze) frequently occurred. In addition, aerosols and NPOCs were transported to Jiujiang from northern China, which has significant amounts of coal burning and industries, through long-range transport of air masses (Han et al., 2018). However, the annual average concentrations of PM10-bound n-alkanes and PAHs in Delhi, India (Yadav et al., 2013), were 4.0 and 8.2 times higher than that in Jiujiang, respectively.
Table 2Comparison of NPOC concentrations between Jiujiang and other areas (ng m−3).
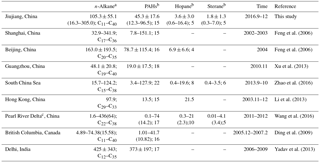
a Mean concentration (concentration range); species.
b Mean concentration (concentration range); number of species.
c Including four cities in the Pearl River Delta: Guangzhou, Dongguang,
Nanhai and Nansha.
3.1.1 PAHs
PAHs are ubiquitous pollutants of the environment. They are originated from natural and anthropogenic sources such as biomass burning, vehicle exhausts, residential heating, waste incineration and industrial emissions. The PM2.5-associated PAHs are shown in Fig. 3a. Their individual species concentrations varied between 0.4 and 5.7 ng m−3. BbF (benzo[b]fluoranthene, 5.7 ng m−3) was the most abundant PAH species, followed by BaA (benz[a]anthracene, 5.6 ng m−3) and BaP (benzo[e]pyrene, 4.2 ng m−3), together accounting for 34.2 % of all PAHs; this was consistent with some previous studies in Guangzhou (Xu et al., 2013). Because of the strong carcinogenic effect of BaP, special attention should be given to it, the level of which was also higher than that indicated by the air quality guideline of the World Health Organization (1 ng m−3).
Due to the vapor-pressure-dependent partitioning, two- and seven-ring PAHs distributed mainly in the gas and particle phases, respectively. However, PAHs with three to six rings appeared in both gas and particle phases through gas–particle partitioning. Moreover, FLU-PYR-CHR (chrysene) and BaA-BaP congeners of the four-ring PAHs often indicate diesel vehicle and biomass combustion (Yadav et al., 2013), respectively, while the five-ring BkF is considered a marker of vehicle tracer. The total percent contribution of four- and five-ring PAHs was 67.9 % in this study, which suggests vehicle exhaust, biomass burning and fossil fuel combustion have mixed effects on local atmospheric pollution.
MDRs of atmospheric PAHs with similar molecular weight have been widely used as a useful tool for aerosol source identification. In this study, the ANT ∕ (PHE + ANT) ratios varied between 0.28 and 0.68 (mean of 0.48), which was greater than 0.1, confirming a strong influence from pyrogenic emissions (Xu et al., 2013; Yadav et al., 2013). Most samples had FLU ∕ (FLU + PYR) ratios of 0.36–0.58, implying combined effects of pyrogenic emission and combustion of fuel, grass, wood and coal (Kuang et al., 2011; P. F. Chen et al., 2016). The average IcdP ∕ (IcdP + BghiP) ratio was 0.57, and in most cases the ratio > 0.50, suggesting significant impacts from the combustion of grass, wood and coal. Despite the fact that MDRs have been consistently used for PAH source identification, it remains a rather rough method and contradictions may occur sometimes. Therefore, more samples should be collected to achieve better results.
3.1.2 n-Alkanes
Unique signatures of n-alkanes have been shown for different sources, including vehicle exhausts, tire-wear particles, road dust, cooking oil, cigarette smoke and particulate abrasion products from leaf epicuticular waxes (Rogge et al., 1994; Ma et al., 2011; Yadav et al., 2013; Zhang et al., 2015). A bimodal distribution of chain lengths of n-alkanes with peaks at C20–C22 and C24–C27 implies vehicle exhaust sources (Zhang et al., 2015). However, a unimodal distribution of chain lengths of > C30 n-alkanes (peak at C37) represents tire-wear particle sources. The distribution pattern characterized by a large proportion of C27–C33 odd n-alkanes suggests vegetation sources.
Our analysis showed that the middle-chain-length n-alkanes (C25–C34) were the most abundant (Fig. 3b), accounting for 72.3 % of the total measured n-alkanes. Feng et al. (2006) and Xu et al. (2013) reported similar findings that C27–C29 n-alkanes dominated the distribution of n-alkanes in three metropolitan cities of China (Beijing, Shanghai and Guangzhou). In addition, a predominance of odd carbon numbered congeners (C25, C27, C29, C31 and C33) was found, with Cmax = 31 in most cases and Cmax = 29 in a few cases (Fig. 3b). The Cmax value in this study suggests that emissions arising from leaf abrasion products contributed to n-alkane concentrations in Jiujiang. However, no obvious odd or even carbon preference was observed for either C11–C14 or C35–C40 n-alkanes.
Plant wax n-alkanes exhibit strong odd or even carbon number predominance, while n-alkanes from fossil fuel combustion do not (Feng et al., 2006). Thus, biogenic n-alkanes should have CPI values greater than unity, whereas anthropogenic n-alkanes should have CPI values close to unity. Furthermore, CPI < 2 is a typical characteristic of urban environment, suggesting major contribution from petrogenic sources, e.g., vehicle exhausts and industrial emissions. The CPI values were 1.00–1.79 (average of 1.29) in this study, implying strong contributions from petrochemical sources, diesel residues and gasoline emissions. Ding et al. (2009) reported a mean CPI value of 1.5 in central British Columbia, Canada, and Xu et al. (2013) reported CPI values of 1.2–1.7 (mean of 1.4) in Guangzhou, China. The mean contribution of plant wax n-alkanes to the total n-alkanes (WNA %) was 17.00±4.41, ranging from 7.9 to 31.31 %. PNA % provides a direct insight into n-alkanes from petrogenic sources. In this study, PNA % was 83±4.41 %, implying that 83 % of n-alkanes were originated from anthropogenic sources. The ACL value varied from 27.45 to 30.95, which was consistent with the calculated result (29.2±0.8) of Delhi in India (Yadav et al., 2013). These small fluctuations may suggest that n-alkane emissions were similar across different sampling sites and displayed a relatively homogeneous distribution in Jiujiang.
3.1.3 Hopanes and steranes
Hopanes and steranes are usually found in crude oil and engine oil, and subsequently in vehicle exhausts from unburned lubricating oil residues. They are regarded as markers of fossil fuel combustion. The concentration profile of hopanes and alkanes is shown in Fig. 3c. Their total concentrations ranged from 1.1 to 20.5 ng m−3, and the concentration of hopanes was approximately 2 times that of steranes. As expected, the total concentrations of hopanes ∕ steranes were 6.7 ∕ 2.5, 3.3 ∕ 1.2 and 1.9 ∕ 1.1 ng m−3 in the petrochemical industry (SH), traffic area (SL) and suburban area (WQ), respectively.
The predominant hopane analogs were C30-αβ-H (αβ-hopane), C31-αβ-S (αβ 22S-homohopane) and C29-αβ-NOR-H (αβ-Norhopane), with concentrations of 1.2±1.3, 0.8±0.7 and 0.8±0.9 ng m−3, respectively. The homohopane index (C31-S ∕ (S + R)) was 0.75, which is much greater than those of diesel (0.49), gasoline vehicle emissions (0.50–0.62) and petroleum (0.6), but a bit smaller than industrial bitumite coal (0.87) (Fraser et al., 2002). This implies vehicle exhausts, petrochemical emissions and coal combustion have all contributed to particle concentrations. The concentration profiles of steranes were similar at different sites, with ααα-20R-EC (ααα-20R24R-ethylcholestane) being the most abundant, followed by ααα-20R-C (ααα-20R-cholestane). This pattern was a bit different from that in Delhi, which was dominated by C29 sterane (Yadav et al., 2013).
3.2 Size-specific distributions
Particulate matter within 13 size fractions was collected. The size-specific distribution of NPOCs was then obtained (Fig. 4). The mean Σ15PAHs (Fig. 4a) in each size fraction ranged from 0.4 ng m−3 in the 0.01–0.018 µm fraction to 5.1 ng m−3 in the 0.56–1.00 µm fraction. A bimodal distribution of the concentrations of PAHs was observed, with peaks in 0.56–1.00 and 9.90–18.0 µm fractions, respectively. PAHs in the 0.56–1.00 µm fraction were the most abundant. This phenomenon could be reasonably explained by the fact that heavy-molecular-weight PAHs tend to be enriched in smaller particles (< 1.4 µm) (Kleeman et al., 2008), which generally originate from gas–particle transformation, adsorption of gaseous PAHs by condensation or coagulation of combustion products on the surface of preexisting particles. However, the light-molecular-weight PAHs are speculated to be adsorbed onto coarse particles, mainly originated from resuspension of soil or dust, plant tissue and growing particles from small diameters. As discussed above, the heavy-molecular-weight PAHs (> four-ring) accounted for 33.7–73.7 % (mean of 50.6 %) of the total PAHs, and the MDRs values of PAH species both confirmed our hypothesis that the condensation or coagulation of combustion products contributed to the size distribution pattern of PAHs. Similarly, Hien et al. (2007) found that PAHs accumulated predominantly in small size fractions (especially < 0.4 µm) in urban aerosols. More recently, Mu et al. (2017) indicated PAHs were strongly correlated with accumulation mode particles (0.05–2.0 µm), and PAHs in this fraction accounted for ∼ 85 % of the total measured PAHs.
The concentrations of n-alkanes (Fig. 4b) in Aitken nuclei (< 0.05 µm), accumulation and coarse mode (> 2.0 µm) particles were 1.7–3.1, 7.0–28.7 and 4.7–6.3 ng m−3, respectively. The concentrations of n-alkanes in individual fractions accounted for 1.5–24.5 % of the total measured n-alkanes in all fractions. n-Alkanes in the 0.56–1.00 µm fraction were the most abundant, whereas n-alkanes in three nano-size fractions accounted for the smallest percentages (1.5–2.5 %). Notably, the concentration of n-alkanes increased with increase in fraction size. After fraction size reached 1.00 µm, however, the concentration of n-alkanes decreased in coarse mode particles. This implies n-alkanes have a tendency to be adsorbed on fine particles. In general, condensation is more likely happen to fine particles because of their large quantity and larger specific surface area (Wang et al., 2009).
The size-specific distribution of hopanes and steranes is illustrated in Fig. 4c. Hopanes and steranes were the most abundant in the following five fractions: 0.56–1.00 (2.9), 0.32–0.56 (2.5), 0.18–0.32 (1.8), 9.9–18 (1.2) and 0.10–0.18 µm (1.1 ng m−3). Approximately 55 % of ∑ (hopanes + steranes) were associated with the 0.10–1.00 µm fraction, which probably was due to their origins from fossil fuel combustion. This result was consistent with that of Kleeman et al. (2008), who found the hopanes and steranes were abundant in ultrafine size fraction during a severe winter pollution episode in Sacramento, USA.
Moreover, our recent research (Han et al., 2018) found that the organic compounds carrier, OC/EC, displayed a unimodal distribution in the fraction of 0.56–1.0 µm among the 13 staged particles. It is also suggested that EC could provide adsorption sites for organic compounds (e.g., NPOCs) due to its large surface area, and it has the catalytic properties for redox chemistry reactions. In fact, the relationship between the concentration of NPOCs and the size particles is highly variable. This suggests that not only source type but also photodegradation and gas–particle partitioning have great influences on the size-specific distribution of NPOCs, which is further discussed in Sect. 3.3 and 3.4, respectively.
3.3 Degradation of organics
Photochemical oxidation has great influences on the mass concentration and size-specific distribution of NPOCs, as well as on their removal and atmospheric fate (May et al., 2012). Photochemical decay could cause the ambient data to be distributed along a line emanating from the source profile in the ratio–ratio plot, with increasing photochemical age (Robinson et al., 2006; Yu et al. 2011). EC shares common origins with PAHs and hopanes but they are subject to photodegradation. In this study, two pairs of EC normalized PAHs and hopanes (Fig. 5), namely IcdP/BghiP and C29-αβ-NOR/C30-αβ-H (αβ-Nnorhopane/αβ-hopane) were adopted to visually compare the distributions of critical marker species and investigate and explore the degradation of NPOCs (Robinson et al., 2006; Wang et al., 2016).
Most of the EC normalized IcdP/BghiP data points were distributed along a line (Fig. 5a), implying ambient PAHs underwent photochemical degradation and were influenced by vehicle emissions and coal combustion. It was reported that the free ends of C–C scission products of PAHs remain tethered together, which prevent fragmentation and help in forming more functional groups from the reactions with OH• radical (Hunter et al., 2014) – ultimately forming the low-volatility species which can condense on the particle phase. There were several deviation points in the lower-left corner, and the values were smaller than the values of the tunnel and vehicle source profile, indicating mixed influence from traffic origins and degradation. Yu et al. (2011) reported a more apparent linear distribution of data sets measured in Hong Kong and PRD, which can be attributed to their single vehicle source type.
In Fig. 5b, most of the data are linearly distributed, implying the photochemical decay of C29-αβ-NOR/C30-αβ-H in this study. Previous research provided evidences that photochemical oxidation alters the molecular-level composition of hopanes (Robinson et al., 2006). OH• radical was expected to be the major source of atmospheric oxidants, and rudimentary calculations suggest that OH• oxidized hopanes on a timescale of a few days, which depended on aerosol size. Moreover, the C29-αβ-NOR/C30-αβ-H ratio–ratio plot also suggests both biomass burning and vehicle emission contributed to hopane concentrations in this study. Robinson et al. (2006) found hopanes were severely depleted in Pittsburgh, USA, and they attributed this phenomenon to regional air mass transport affecting the oxidation of condensed-phase organic compounds.
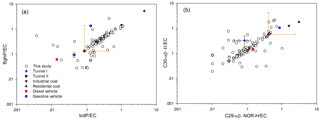
Figure 5Ratio–ratio plots of two pairs of characterized species (IcdP/BghiP and C29-αβ-NOR-H/C30-αβ-H) normalized by EC and published source profiles. (tunnel I: Yu et al., 2011; tunnel II: He et al., 2009; residential coal: Zhang et al., 2008; industrial coal: Zhang et al., 2008; diesel vehicle: Fraser et al., 2002; gasoline vehicle: Fraser et al., 2002).
3.4 Impact of gas–particle partitioning on fine particle source apportionment
3.4.1 Gas–particle partitioning
An important aspect of atmospheric NPOCs is their gas–particle partitioning behavior, which has effects on their fate and size-specific occurrence. The particle-phase fraction (φ) of NPOCs was calculated according to the gas–particle partitioning model (Fig. 6 and Figs. S2 and S3 in the Supplement). The gas-phase fractions of low molecule weight (LMW) PAHs (e.g., FLO, PHE, ANT, FLU and PYR) were rather substantial, and their particle-phase fractions (φ) ranged from 2.4 to 51.3 %. Similarly, the φ values of short-chain C22–C24 n-alkanes varied between 21.2 and 62.5 %, exhibiting an increasing trend with increase in their molecular weight. However, for the heavier-molecular-weight species, the φ values remained greater than 90.0 % for all temperature ranges. The calculated φ values of PAHs and n-alkanes were comparable to those estimated in urban Denver, Chicago and Los Angeles in the USA (Xie et al., 2013) but a bit greater than those in the PRD of China (Wang et al., 2016). The lower fractions of NPOCs in the gas phase in this study compared with that in the PRD was probably because the PRD area is located in a border region between subtropics and tropics. The PRD has higher temperature than the eastern China area, especially in cold winter seasons, and the higher temperature can facilitate the shift of species to gas phase.
3.4.2 PMF source apportionment
Source apportionment analysis involves techniques that can be used to identify source species and their unique contributions, which are critical to making policies for controlling pollution. It is typically assumed the molecular markers are stable in the ambient environment, i.e., being nonreactive and nonvolatile (May et al., 2012). However, as discussed above, many organic markers can be oxidized over atmospherically relevant timescales, as well as partition between gas and particle phases. If the data of NPOCs in the single particle phase are directly used as input for the receptor model, this may confound the aerosol factors.
Additionally, individual organic tracers, elemental species, inorganic ions and OC/EC have been demonstrated to be able to provide source apportionment of aerosols. To explore the impact of gas–particle partitioning on PM2.5 source apportionment, both the single particle phase and the total (gas + particle) NPOCs were incorporated with elemental species, inorganic ions and OC/EC used as input data for the receptor model PMF (detailed description about the PMF model could be seen in Sect. S1 in the Supplement). Results based on the single particle phase and the total phases were denoted by PMFP and PMFT, respectively. A total of 5 to 11 factors were extracted in this study to obtain reasonable results. Finally, it turned out that the results of eight factors gave the most reasonable source profiles (Figs. 7 and S4).
Factor 1 (Fig. 7a) was characterized by a significant presence of Al, Ca, Mg, Ti and Fe, which are regarded as good indicators of dust (including construction dust, geological dust and road dust) (Wang et al., 2015). These elements are the major elements of dust sand, usually accumulated in the coarse mode particles. Geological dust typically contains high concentrations of crustal elements, including Fe and Mn. Hence, this factor was regard as “dust”, with percent contributions of 8.90 and 11.0 % under PMFP and PMFT, respectively.
Factor 2 (Fig. 7b) was characterized by the significant presence of Cu, Mn, Zn, Pb, BkF, BbF, BaF and BaP. Mn, Zn and As are related to emissions from steel production, brick, ceramic and glass-making factories (Li et al., 2016; Sulong et al., 2017). Cu mainly originates from non-ferrous metal production and smelting factories. BkF, BbF and BaP are typical markers of emissions from the coke industry. Several large-scale industrial parks are located in Jiujiang, e.g., Shacheng Industrial Park and Jiujiang Comprehensive Industrial Park in the northern and southern areas, respectively. Therefore, factor 2 was associated with industrial emission.
Factor 3 (Fig. 7c) was characterized by large fractions of high molecular weight (HMW) PAHs (IcdP, BghiP, DahA and COR), as well as relatively high fractions of hopanes and steranes. BghiP and COR are excellent tracers of vehicle exhausts. Hopanes and steranes are related to exhausts from heavy-duty vehicles with diesel engines (Wang et al., 2016). As mentioned above, there were over 700 000 motor vehicles in Jiujiang in 2015, among which about 1/15 were mainly powered by diesel engines. Therefore, factor 3 was identified as vehicle-related exhausts, with percent contributions of 12.5 and 15.0 % under PMFP and PMFT, respectively.
Factor 4 (Fig. 7d) was characterized by the presence of well-documented indicators of secondary aerosol formation, such as , and , with factor fractions of 83.7, 87.4 and 94.1 %, respectively. These secondary products are formed by precursor gases (SO2 and NOx) via oxidation reactions (Wang et al., 2015). They are mainly emitted from biomass burning, coal combustion and vehicles. , and accounted for 19.9–22.3, 16.4–18.1 and 10.4–13.7 % of PM2.5 concentrations, respectively, which were typically derived from the gas–particle conversion process as well as homogeneous and heterogeneous reactions in the urban atmosphere. Furthermore, the similar spatial distribution and contribution in all sites highlight a wide spread of these components. Consequently, factor 4 was identified as secondary aerosol formation.
Factor 5 (Fig. 7e) was characterized by a significant presence of C30–C40 n-alkanes, as well as a relatively significant presence of FLU and PYR. In previous research (Wang et al., 2016), the long-chain n-alkanes (C29–C36) were considered to come from local emissions, especially from coal combustion. PYR and FLU are frequently considered as excellent markers of coal combustion for aerosol source apportionment. Coal is the primary energy source for many industries in China. About 3.1 million t of standard coal are consumed per year by the Jiujiang thermal power plant according to local statistics. Thus, factor 5 was identified as coal burning, with percent contributions of 18.7 and 16.4 % under PMFP and PMFT, respectively.
Factor 6 (Fig. 7f) was characterized by high percentage of Cl− and K+, with some amounts of As, Se, Pb, OC and EC. Cl− and K+ have been widely used as tracers of wood and biomass burning aerosol (Li et al., 2016). In the past, crop straws were disposed of by local farmers in the field by burning, for convenience. Although this has been extensively banned in recent years, several large-scale straw burning sites surrounding this city can still be observed by the China National Satellite Meteorological Center (http://hjj.mep.gov.cn/jgjs, last access: 30 June 2018). Thus, this factor was considered as biomass burning, with percent contributions of 12.7 and 15.7 % under PMFP and PMFT, respectively.
Factor 7 (Fig. 7g) was characterized by high fraction of Ni and V, which are excellent tracers of exhausts from ship and heavy-duty diesel vehicles. In fact, Jiujiang harbor is among the 10 busiest harbors in the Yangtze River, whose port cargo throughput is 59 million t per year. Hence, factor 7 was identified as shipping and diesel exhausts.
Factor 8 (Fig. 7h) was characterized by a high load of short-chain n-alkanes (C22H46, 76.6 %; C23H48, 84.2 %; C24H50, 81.1 %) and LMW PAHs (about 60 % for FLU, PYR, BaA and CHR). These species have several characteristics: most of their particle-phase fractions (φ) were less than 50 %; relatively light molecular weight; strongly temperature-dependent vaporization. These compounds have been interpreted as the light NPOCs factor in previous research (Xie et al., 2013; Wang et al., 2016). The percent contributions of this factor were 3.7 and 5.6 % under PMFP and PMFT, respectively. Additionally, concentrations of the light NPOCs factor showed an increasing trend with increase in temperature, implying the association of this factor with fossil fuel evaporation and biogenic emissions. Hence, this factor was regarded as the light NPOCs factor.
3.4.3 Assessing impacts of gas–particle partitioning on source apportionment
As stated above, using the data of the single particle phase as input data for the PMF model could lead to uncertainty in results, which was related to gas–particle partitioning of NPOCs in the mathematical solution. This influence could be reduced by adding the predicted gaseous NPOC concentrations when the measured gaseous NPOC data were not available. In the present study, the eight extracted factors showed similar source profiles between PMFP and PMFT, which was in sharp contrast with one recent research that found several very volatile NPOCs (like FLU) were quite variable in PMFT, but almost did not appear in PMFP in the PRD (Wang et al., 2016). This difference could probably be due to the fact that the PM2.5 source identification in this study was focused on the period of high-frequency haze episodes (late autumn and winter), with the majority of the predicted NPOCs enriched in the particle phase. Moreover, the NPOCs investigation conducted in the PRD included the hot summer season, which enhanced the uncertainty and variability of predicted light NPOCs.
Despite the fact that the current study could not predict gas-phase NPOCs with high accuracy, the source apportionment result extracted by PMFP and PMFT were comparable. Using the data of total organic compounds in gas–particle phases with other aerosol species as input data for the receptor model provides an excellent tool for PM2.5 source apportionment. However, due to the uncertainty of MWOM, ξOM and values, the gaseous-phase light NPOCs might be overestimated, especially for light NPOCs. Hence, reasonable caution should still be given to the more volatile organic species.
3.5 Limitation and implication
In this work, we confirmed that using the total (gas + particle) NPOCs as input data for the receptor model provides a better source apportionment than using only the particle phase. However, the predicted gas NPOCs from gas–particle partitioning may bring some uncertainty. For example, the partitioning processes are strongly affected by the particle properties (particle size, organic carbon compounds), photodegradation and the prevailing ambient temperature. For size distribution, the PM2.5-associated NPOCs in the 0.56–1.00 µm fraction were the most abundant; our recent study also found OC was primarily distributed in this fraction (Han et al., 2018). Abundant OC would adsorb/absorb large amounts of NPOCs, with the resulting particle-bound NPOCs concentration increasing in this particle size, while the gas-phase oxidation reaction is much faster than heterogeneous reactions in aerosol surface, since the uptake of heterogeneous oxidant is diffusion limited (Robinson et al., 2006; May et al., 2012).
Low temperature promotes NPOCs adsorbing/absorbing onto aerosols, while photochemical degradation of NPOCs is relatively weak in the cold season. Moreover, photochemical reactions would reduce the abundances of organic markers depend on species, significantly altering the relative contribution of different sources extracted by linear source inversion. Compared with the long-term investigation, this study was mainly focused on the cold season, which leads to a relatively high abundance of particle NPOCs with small variation.
The limitation for the PMF model was that it could not identify a potential source without a preexisting tracer. Also, the relative small number of measurements might lead to some uncertainty in source apportionment. In the future, more source tracer data need to be included for the calculation of potential contributions.
NPOCs are typical molecular markers for source identification, which attract researchers' interest worldwide. A total of 57 PM2.5-associated NPOCs including PAHs, n-alkanes, iso-alkanes, hopanes and steranes were identified and quantified using a TD–GC–MS method in a typical city in eastern China. The total concentrations of NPOCs were 31.7–388.7 ng m−3, with n-alkanes being the most abundant species (67.2 %). The heavy-molecular-weight PAHs (four- and five-ring) contributed 67.9 % of the total PAHs, and the middle-chain-length (C25–C34) n-alkanes were the most abundant in n-alkanes.
For size distribution, PAHs and n-alkanes were mainly enriched in the 0.56–1.00 µm fraction, and ∑ (hopanes + steranes) were associated with the 0.32–1.00 µm fraction, implying their similar source of combustion products. The ratio–ratio plots of IcdP/BghiP and C29-αβ-NOR/C30-αβ-H implied that NPOCs in the local area were affected by photochemical degradation. Using single particle (PMFP) and total (particle + gas, PMFT) phase NPOCs as input data for the PMF model, respectively, we successfully extracted eight factors from both cases. The PMFT showed better source profiles than PMFP, and the light NPOCs factor contributed a bit more to PMFT (5.6 %) than to PMFP (3.7 %). This study indicates that NPOCs are useful for aerosol apportionment, and total NPOCs in two phases enable better source profiles than NPOCs in the single particle phase.
The data presented in this article are available from the authors upon request (jpcheng@sjtu.edu.cn)
The supplement related to this article is available online at: https://doi.org/10.5194/acp-18-9375-2018-supplement.
DH mainly conducted the sampling, sample analysis work, as well as manuscript writing and revision. JC provided direct funding and helped with manuscript revision. SEMC and SAES helped with sampling quality control and laboratory analysis, respectively. Other authors helped this work by sampling and analysis.
The authors declare that they have no conflict of interest.
This article is part of the special issue “Regional transport and transformation of air pollution in eastern China”. It is not associated with a conference.
This study was financially supported by the National Natural Science Foundation
of China (no. 21577090 and no. 21777094) and Jiujiang Committee of Science and
Technology (grant no. JXTCJJ2016130099). We thank the Jiujiang Environmental
Protection Agency and Jiujiang environmental monitoring station for coordinating
the sampling process and for their valuable contribution to field
measurements. We appreciate Yajuan Zhou (Instrumental Analysis Center,
Shanghai Jiao Tong University) for her assistance in experimental
analysis.
Edited by: Aijun Ding
Reviewed by: four anonymous referees
And, J. S. C. and Hanshaw, W.: Vapor Pressures and Vaporization Enthalpies of the n-Alkanes from C31 to C38 at T = 298.15 K by Correlation Gas Chromatography, J. Chem. Engineering Data, 49, 620–630, 2004.
Chen, P. F., Li, C. L., Kang, S. C., Rupakheti, M., Panday, A. K., Yan, F. P., Li, Q. L., Zhang, Q. G., Guo, J. M., Rupakheti, D., and Luo, W.: Polycyclic aromatic hydrocarbons (PAHs) in aerosols over the central Himalayas along two south-north transects, Atmos. Chem. Phys. Discuss., https://doi.org/10.5194/acp-2016-71, 2016.
Chen, X., Balasubramanian, R., Zhu, Q., Behera, S. N., Bo, D., Huang, X., Xie, H., and Cheng, J.: Characteristics of atmospheric particulate mercury in size-fractionated particles during haze days in Shanghai, Atmos. Environ., 131, 400–408, 2016.
Ding, L. C., Ke, F., Wang, D. K. W., Dann, T., and Austin, C. C.: A new direct thermal desorption-GC/MS method: Organic speciation of ambient particulate matter collected in Golden, BC, Atmos. Environ., 43, 4894–4902, 2009.
Feng, J., Hu, M., Chan, C. K., Lau, P. S., Fang, M., He, L., and Tang, X.: A comparative study of the organic matter in PM2.5 from three Chinese megacities in three different climatic zones, Atmos. Environ., 40, 3983–3994, 2006.
Fraser, M. P., Lakshmanan, K., Fritz, S. G., and Ubanwa, B.: Variation in composition of fine particulate emissions from heavy-duty diesel vehicles, J. Geophys. Res.-Atmos., 107, ICC 8-1-ICC 8-6, 2002.
Han, D., Zhang, J., Hu, Z., Ma, Y., Duan, Y., Han, Y., and Cheng J.: Particulate mercury in ambient air in Shanghai, China: Size-specific distribution, gas-particle partitioning, and association with carbonaceous composition, Environ. Pollut., 238, 543–553, 2018.
Hao, Y. and Liu, Y. M.: The influential factors of urban PM2.5 concentrations in China: aspatial econometric analysis, J. Clean. Prod., 112, 1443–1453, 2015.
He, J. and Balasubramanian, R.: A study of gas/particle partitioning of SVOCs in the tropical atmosphere of Southeast Asia, Atmos. Environ., 43, 4424–4434, 2009.
He, L. Y., Hu, M., Zhang, Y. H., Huang, X. F., and Yao, T. T.: Fine particle emissions from on-road vehicles in the Zhujiang tunnel, China, Environ. Sci. Technol., 42, 4461–4466, 2008.
Hien, T. T., Le, T. T., Kameda, T., Takenaka, N., and Bandow, H.: Distribution characteristics of polycyclic aromatic hydrocarbons with particle size in urban aerosols at the roadside in Ho Chi Minh City, Vietnam, Atmos. Environ., 41, 1575–1586, 2007.
Ho, S. S. and Yu, J. Z.: In-injection port thermal desorption and subsequent gas chromatography-mass spectrometric analysis of polycyclic aromatic hydrocarbons and n-alkanes in atmospheric aerosol samples, J. Chromatogr. A, 1059, 121–129, 2004.
Ho, S. S. H., Yu, J. Z., Chow, J. C., Zielinska, B., Watson, J. G., Sit, E. H. L., and Schauer, J. J.: Evaluation of an in-injection port thermal desorption-gas chromatography/mass spectrometry method for analysis of non-polar organic compounds in ambient aerosol samples, J. Chromatogr. A, 1200, 217–227, 2008.
Huang, R. J., Zhang, Y., Bozzetti, C., Ho, K. F., Cao, J. J., Han, Y., Daellenbach, K. R., Slowik, J. G., Platt, S. M., and Canonaco, F.: High secondary aerosol contribution to particulate pollution during haze events in China, Nature, 514, 218–222, 2014.
Huang, X., Liu, Z., Liu, J., Hu, B., Wen, T., Tang, G., Zhang, J., Wu, F., Ji, D., Wang, L., and Wang, Y.: Chemical characterization and source identification of PM2.5 at multiple sites in the Beijing-Tianjin-Hebei region, China, Atmos. Chem. Phys., 17, 12941–12962, https://doi.org/10.5194/acp-17-12941-2017, 2017.
Hunter, J. F., Carrasquillo, A. J., Daumit, K. E., and Kroll, J. H.: Secondary organic aerosol formation from acyclic, monocyclic, and polycyclic alkanes, Environ. Sci. Technol., 48, 10227–10234, 2014.
Kim, D. G., Choi, K. I., and Lee, D. H.: Gas-particle partitioning and behavior of dioxin-like PCBs in the urban atmosphere of Gyeonggi-do, South Korea, Atmos. Res., 101, 386–395, 2011.
Kleeman, M. J., Riddle, S. G., and Jakober, C. A.: Size distribution of particle-phase molecular markers during a severe winter pollution episode, Environ. Sci. Technol., 42, 6469–6475, 2008.
Kuang, Y. W., Huang, Z. H., Wen, D. Z., Li, J., and Huang, L. B.: Unravelling airborne polycyclic aromatic hydrocarbons (PAHs) in southern China using tree-rings of 100-yr old Pinus Kwangtungensis, Atmos. Chem. Phys. Discuss., 11, 27359–27382, https://doi.org/10.5194/acpd-11-27359-2011, 2011.
Li, J., Wang, G., Ren, Y., Wang, J., Wu, C., Han, Y., Zhang, L., Cheng, C., and Meng, J.: Identification of chemical compositions and sources of atmospheric aerosols in Xi'an, inland China during two types of haze events, Sci. Total Environ., 566–567, 230–237, 2016.
Li, L., Tan, Q., Zhang, Y., Feng, M., Qu, Y., An, J., and Liu, X.: Characteristics and source apportionment of PM2.5 during persistent extreme haze events in Chengdu, southwest China, Environ. Pollution, 230, 718–729, 2017.
Li, Y. C., Yu, J. Z., Ho, S., Schauer, J. J., Yuan, Z. B., Lau, A. K. H., and Louie, P. K. K.: Chemical characteristics and source apportionment of fine particulate organic carbon in Hong Kong during high particulate matter episodes in winter 2003, Atmos. Res., 120–121, 88–98, 2013.
Ma, W. L., Sun, D. Z., Shen, W. G., Yang, M., Qi, H., Liu, L. Y., Shen, J. M., and Li, Y. F.: Atmospheric concentrations, sources and gas-particle partitioning of PAHs in Beijing after the 29th Olympic Games, Environ. Pollution, 159, 1794–1801, 2011.
Ma, Y., Dai, H., Li, L., Chen, C., Sun, Q., Fan, J., Li, Y., and Huang, T.: A rapid method for screening on organic pollutants in PM2.5 using GCMS combined with compound composer software, Environ. Chem., 37, 188–191, 2018.
May, A. A., Saleh, R., Hennigan, C. J., Donahue, N. M., and Robinson, A. L.: Volatility of organic molecular markers used for source apportionment analysis: measurements and implications for atmospheric lifetime, Environ. Sci. Technol., 46, 12435–12444, 2012.
Mu, L., Peng, L., Liu, X., He, Q., Bai, H., Yan, Y., and Li, Y.: Emission characteristics and size distribution of polycyclic aromatic hydrocarbons from coke production in China, Atmos. Res., 197, 113–120, 2017.
Okonski, K., Degrendele, C., Melymuk, L., Landlová, L., Kukučka, P., Vojta, Š., Kohoutek, J., Čupr, P., and Klánová, J.: Particle Size Distribution of Halogenated Flame Retardants and Implications for Atmospheric Deposition and Transport, Environ. Sci. Technol., 48, 14426–14434, 2014.
Pankow, J. F.: An absorption model of the gas/aerosol partitioning involved in the formation of secondary organic compounds, Atmos. Environ., 28, 189–193, 1994.
Rajput, P. and Sarin, M. M.: Polar and non-polar organic aerosols from large-scale agricultural-waste burning emissions in Northern India: Implications to organic mass-to-organic carbon ratio, Chemosphere, 103, 74–79, 2014.
Robinson, A. L., Donahue, N. M., and Rogge, W. F.: Photochemical oxidation and changes in molecular composition of organic aerosol in the regional context, J. Geophy. Res.-Atmos., 111, 375–402, 2006.
Rogge W. F., Hildemann L. M., and Mazurek M. A.: Sources of fine organic aerosol, 6, Cigaret smoke in the urban atmosphere, Environ. Sci. Technol., 28, 1375–1388, 1994.
Shen, X. J., Sun, J. Y., Zhang, X. Y., Zhang, Y. M., Zhang, L., Che, H. C., Ma, Q. L., Yu, X. M., Yue, Y., and Zhang, Y. W.: Characterization of submicron aerosols and effect on visibility during a severe haze-fog episode in Yangtze River Delta, China, Atmos. Environ., 120, 307–316, 2015.
Sulong, N. A., Latif, M. T., Khan, M. F., Amil, N., Ashfold, M. J., Mia, W., Chan, K. M., and Sahani, M.: Source apportionment and health risk assessment among specific age groups during haze and non-haze episodes in Kuala Lumpur, Malaysia, Sci. Total Environ., 601–602, 556–570, 2017.
Wang, G., Kawamura, K., Xie, M., Hu, S., Gao, S., Cao, J., An, Z., and Wang, Z.: Size-distributions of n-alkanes, PAHs and hopanes and their sources in the urban, mountain and marine atmospheres over East Asia, Atmos. Chem. Phys., 9, 8869–8882, https://doi.org/10.5194/acp-9-8869-2009, 2009.
Wang, Q., Zhuang, G., Huang, K., Liu, T., Deng, C., Xu, J., Lin, Y., Guo, Z., Chen, Y., and Fu, Q.: Probing the severe haze pollution in three typical regions of China: Characteristics, sources and regional impacts, Atmos. Environ., 120, 76–88, 2015.
Wang, Q., Feng, Y., Huang, X. H. H., Griffith, S. M., Zhang, T., Zhang, Q., Wu, D., and Yu, J. Z.: Non-polar organic compounds as PM2.5 source tracers: Investigation of their sources and degradation in the Pearl River Delta, China, J. Geophy. Res.-Atmos, 121, 11862–11879, 2016.
Xie, M., Barsanti, K. C., Hannigan, M. P., Dutton, S. J., and Vedal, S.: Positive matrix factorization of PM2.5 – eliminating the effects of gas/particle partitioning of semivolatile organic compounds, Atmos. Chem. Phys., 13, 7381–7393, https://doi.org/10.5194/acp-13-7381-2013, 2013.
Xie, M., Hannigan, M. P., and Barsanti, K. C.: Impact of Gas/Particle Partitioning of Semivolatile Organic Compounds on Source Apportionment with Positive Matrix Factorization, Environ. Sci. Technol., 48, 9053–9060, 2014.
Xie, Y., Ye, X., Ma, Z., Tao, Y., Wang, R., Zhang, C., Yang, X., Chen, J., and Chen, H.: Insight into winter haze formation mechanisms based on aerosol hygroscopicity and effective density measurements, Atmos. Chem. Phys., 17, 7277–7290, https://doi.org/10.5194/acp-17-7277-2017, 2017.
Xu, H. M., Tao, J., Ho, S. S. H., Ho, K. F., Cao, J. J., Li, N., Chow, J. C., Wang, G. H., Han, Y. M., and Zhang, R. J.: Characteristics of fine particulate non-polar organic compounds in Guangzhou during the 16th Asian Games: Effectiveness of air pollution controls, Atmos. Environ., 76, 94–101, 2013.
Yadav, S., Tandon, A., and Attri, A. K.: Characterization of aerosol associated non-polar organic compounds using TD-GC-MS: a four year study from Delhi, India, J. Hazard. Materials, 252–253, 29–44, 2013.
Yu, J. Z., Huang, X. H., Ho, S. S., and Bian, Q.: Nonpolar organic compounds in fine particles: quantification by thermal desorption-GC/MS and evidence for their significant oxidation in ambient aerosols in Hong Kong, Analytical Bioanalytical Chem., 401, 3125–3139, 2011.
Zhang, Y.-L., Huang, R.-J., El Haddad, I., Ho, K.-F., Cao, J.-J., Han, Y., Zotter, P., Bozzetti, C., Daellenbach, K. R., Canonaco, F., Slowik, J. G., Salazar, G., Schwikowski, M., Schnelle-Kreis, J., Abbaszade, G., Zimmermann, R., Baltensperger, U., Prévôt, A. S. H., and Szidat, S.: Fossil vs. non-fossil sources of fine carbonaceous aerosols in four Chinese cities during the extreme winter haze episode of 2013, Atmos. Chem. Phys., 15, 1299–1312, https://doi.org/10.5194/acp-15-1299-2015, 2015.
Zhao, Y., Zhang, Y., Fu, P., Ho, S. S., Ho, K. F., Liu, F., Zou, S., Wang, S., and Lai, S.: Non-polar organic compounds in marine aerosols over the northern South China Sea: Influence of continental outflow, Chemosphere, 153, 332–339, 2016.