the Creative Commons Attribution 4.0 License.
the Creative Commons Attribution 4.0 License.
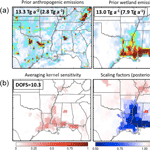
High-resolution inversion of methane emissions in the Southeast US using SEAC4RS aircraft observations of atmospheric methane: anthropogenic and wetland sources
Daniel J. Jacob
Alexander J. Turner
Joannes D. Maasakkers
Melissa P. Sulprizio
A. Anthony Bloom
Arlyn E. Andrews
Debra Wunch
We use observations of boundary layer methane from the SEAC4RS aircraft campaign over the Southeast US in August–September 2013 to estimate methane emissions in that region through an inverse analysis with up to (25×25 km2) resolution and with full error characterization. The Southeast US is a major source region for methane including large contributions from oil and gas production and wetlands. Our inversion uses state-of-the-art emission inventories as prior estimates, including a gridded version of the anthropogenic EPA Greenhouse Gas Inventory and the mean of the WetCHARTs ensemble for wetlands. Inversion results are independently verified by comparison with surface (NOAA∕ESRL) and column (TCCON) methane observations. Our posterior estimates for the Southeast US are 12.8±0.9 Tg a−1 for anthropogenic sources (no significant change from the gridded EPA inventory) and 9.4±0.8 Tg a−1 for wetlands (27 % decrease from the mean in the WetCHARTs ensemble). The largest source of error in the WetCHARTs wetlands ensemble is the land cover map specification of wetland areal extent. Our results support the accuracy of the EPA anthropogenic inventory on a regional scale but there are significant local discrepancies for oil and gas production fields, suggesting that emission factors are more variable than assumed in the EPA inventory.
- Article
(993 KB) - Full-text XML
- BibTeX
- EndNote
Methane is an important greenhouse gas (Myhre et al., 2013). Individual countries must report their national emissions to the United Nations Framework Convention on Climate Change (UNFCCC; United Nation, 1992). Observations of atmospheric methane reviewed by Brandt et al. (2014) have implied that the US national inventory reported by the Environmental Protection Agency (EPA) may be greatly underestimated. Here we use aircraft observations from the NASA SEAC4RS aircraft campaign over the Southeast US (Toon et al., 2016), together with a newly gridded version of the EPA inventory (Maasakkers et al., 2016), in a fine-resolution inversion with detailed error characterization to better quantify methane emissions over this major source region.
The EPA (2016) reports a national anthropogenic emission total of 29.2 Tg CH4 a−1 for 2014, with no significant trend over the past decade and less than ±3 % interannual variability. Major contributors are livestock (32 %), the oil and gas industry (32 %), waste (22 %), and coal mining (8 %). The EPA (2016) inventory is consistent with Lyon et al. (2015) for oil and gas systems and Wolf et al. (2017) for livestock, and 8 % higher than the previous versions (EPA, 2013, 2014), largely due to updated oil and gas emissions. There is also a highly uncertain natural source from wetlands, estimated at 4.5–14 Tg a−1 for the contiguous US in the WETCHIMP compilation of inventories (Melton et al., 2013). Inverse analyses of atmospheric methane observations have suggested that the EPA bottom-up inventory (EPA, 2013, 2014) is too low by about 30 % (Miller et al., 2013; Turner et al., 2015; Alexe et al., 2015). However, Turner et al. (2015) and Alexe et al. (2015) relied on prior estimates from the global EDGAR v4.2 inventory (European Commission, 2011) that have large errors in source patterns particularly for oil and gas systems (Maasakkers et al., 2016; Sheng et al., 2017). Errors in source patterns used as prior estimates can greatly bias inversion results (Jacob et al., 2016), though this depends on the constraint from observations and on the uncertainty assigned to the prior estimates. Miller et al. (2013) used a geostatistical inversion that did not rely on any prior estimates, but had little constraints in the Southeast US.
The SEAC4RS aircraft campaign conducted in August–September 2013 offers an opportunity for better estimating methane emissions in the Southeast US, a region that accounts for about half of total anthropogenic methane emissions in the US, according to the gridded EPA inventory (Maasakkers et al., 2016), and also has extensive wetlands. The aircraft flights provided extensive boundary layer measurements of methane across the region. We conduct an inverse analysis of the SEAC4RS data with the GEOS-Chem chemical transport model (CTM) at resolution, using state-of-the-art prior estimates from the gridded EPA inventory of Maasakkers et al. (2016) and the WetCHARTs extended ensemble wetlands inventory of Bloom et al. (2017). This allows us to evaluate the EPA inventory with better accuracy and resolution than has been done before and also to gain better understanding of US wetland emissions.
We derive an optimized estimate of spatially resolved methane emissions in the Southeast US (domain of Fig. 1) through Bayesian inverse analysis of atmospheric methane observations from the SEAC4RS aircraft campaign. Let the vector x represent a gridded ensemble of methane emissions in the region (state vector for the inversion).The inversion minimizes the cost function J(x) by solving ∇xJ(x)=0 as follows:
Here the methane observations are assembled as a vector y, xA is the prior emission estimate, K is the Jacobian matrix describing the sensitivity of concentrations to emissions, and SA and SO are the prior and observational error covariance matrices, respectively. The observational error includes contributions from both the instrument error and the model transport error.
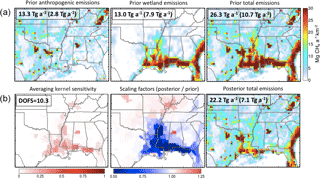
Figure 1Methane emissions in the Southeast US in August–September 2013. Panel (a) shows the prior anthropogenic and wetland methane emissions, and panel (b) shows the inversion results including posterior emissions, scaling factors (posterior ∕ prior emission ratios), and the diagonal elements of the averaging kernel matrix representing the sensitivity of the inversion results to the observations. The sum of these diagonal elements over the domain (trace of the averaging kernel matrix) quantifies the degrees of freedom for signal (DOFS) of the inversion. Numbers inset in the emission panels are the regional totals expressed as annual means for clarity (i.e., assuming that August–September emission rates hold for the rest of the year). Values in parentheses are the totals for the region with averaging kernel sensitivities larger than 0.05 (stippled areas in lower left panel).
Analytical solution of ∇xJ(x)=0 yields the optimal estimate , the posterior error covariance matrix , and the associated averaging kernel matrix A as follows (Rodgers, 2000; Brasseur and Jacob, 2017):
where In is the identity matrix with n being the dimension of the state vector x. Inversions of atmospheric methane observations may solve ∇xJ(x)=0 either analytically, or numerically using an adjoint method (Jacob et al., 2016). Unlike adjoint-based inversions, analytical solution provides direct error characterization of the optimal estimate through its error covariance matrix . The related averaging kernel matrix A describes the sensitivity of the optimal estimate to the true emissions x. The trace of A quantifies the degrees of freedom for signal (DOFS), i.e., the number of pieces of information in the observing system for constraining the methane emissions (DOFS ≤n).
The Jacobian matrix K for the inversion is constructed with the GEOS-Chem CTM (http://www.geos-chem.org, last access: 1 November 2017), which relates methane emissions to atmospheric concentrations through simulation of atmospheric transport. We use a nested version of GEOS-Chem as described by Kim et al. (2015) with horizontal resolution over the North America window and adjacent oceans (9.75–60∘ N, 130–60∘ W), driven by GEOS-FP assimilated meteorological data from the NASA Global Modeling and Assimilation Office (GMAO). The same version of the GEOS-Chem has been applied to simulation of other chemical observations from the SEAC4RS campaign (Kim et al., 2015; Fisher et al., 2016; Marais et al., 2016; Travis et al., 2016; Zhu et al., 2016; Yu et al., 2016; Chan Miller et al., 2017). The boundary conditions for the nested-grid simulation are from a global simulation by Turner et al. (2015) using methane emissions optimized with three years of GOSAT satellite data. The GOSAT-optimized emissions have been independently evaluated with atmospheric methane observations from the NOAA surface network (Turner et al., 2015). The GEOS-Chem uses a 3-D archive of monthly average OH concentrations from Park et al. (2004) to compute the methane sink, with a lifetime of 8.9 years in the troposphere consistent with observational constraints (Prather et al., 2012; Turner et al., 2017). The sink is irrelevant for our North American simulation since ventilation of the domain is much faster (Wecht et al., 2014). Since we treat OH concentrations as decoupled from methane in the inversion, the relationship between emissions and concentrations is strictly linear, so that K fully describes the GEOS-Chem model for the purpose of the inversion.
The prior emission estimates for the inversion are taken from the gridded version of the EPA anthropogenic greenhouse gas emission inventory for 2012 (Maasakkers et al., 2016) and the mean wetland emissions from the monthly WetCHARTs extended ensemble for 2013 (Bloom et al., 2017). Figure 1 (top panels) shows the distribution of these prior methane emissions over the inversion domain for August–September 2013. Emissions total 13.3 Tg a−1 for anthropogenic sources and 13.0 Tg a−1 for wetlands over these two months (expressed on an annual basis). Anthropogenic emissions in the EPA inventory have little seasonal or interannual variability (Turner et al., 2015; Maasakkers et al., 2016), while wetland emissions have a large seasonal variation.
The SEAC4RS DC-8 aircraft conducted 21 flights over the Southeast between 6 August and 21 September 2013. Methane was measured by gas chromatography from whole air flask samples and calibrated to the NOAA standard. Figure 2 (left panel) shows the SEAC4RS flight tracks and the spatial distribution of the methane flask measurements below 2 km altitude. The mean observed vertical profile is shown in the right panel of Fig. 2, and compared to the GEOS-Chem profile using the prior emissions. The model is unbiased in the free troposphere above 2 km, implying a successful representation of background methane by the boundary conditions. Model overestimation in the boundary layer below 2 km suggests that the prior US emissions are too high. For the inversion, we use the SEAC4RS observations below 2 km altitude averaged over the GEOS-Chem grid for individual flights. This represents 652 individual methane observations.
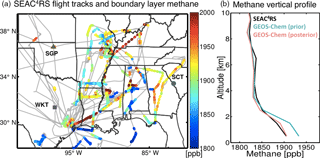
Figure 2Boundary layer methane concentrations over the Southeast US measured during the SEAC4RS aircraft campaign (6 August–21 September 2013). Panel (a) shows the flight tracks in grey and the methane measurements at 0–2 km altitude. The three NOAA∕ESRL sites at SGP (Southern Great Plains, Oklahoma; 36.6∘ N, 97.5∘ W), WKT (Moody, Texas; 31.3∘ N, 97.3∘ W), and SCT (Beech Island, South Carolina; 33.4∘, 81.8∘ W) are indicated. SGP is co-located with the TCCON site at Lamont, Oklahoma. Panel (b) shows the mean methane vertical profiles over the Southeast US domain measured from the aircraft and simulated by GEOS-Chem using the prior and posterior emissions.
We use the residual error method (Heald et al., 2004) to estimate the diagonal elements of the observational error covariance matrix SO. The method assumes that the mean model bias between the observations and the model is due to error in prior emissions to be corrected by the inversion. The residuals are the differences between observed and modeled values after removing the mean model bias. The residual error standard deviation (RSD) is taken to represent the observational error including contributions from the instrument and the transport model. Figure 3 shows the vertical profile of the RSD for the ensemble of the SEAC4RS data over the Southeast US. The RSD is about 60 ppb below 2 km and 20 ppb in the free troposphere above. Subsetting the data by latitudinal bands gives similar results. Thus we use 60 ppb for the standard deviation of the observational error (diagonal elements in SO). The instrument precision is better than 2 ppb (Simpson et al., 2002), therefore most of that observational error is from the transport model. We take SO to be diagonal since error correlations between boundary layer observations on the GEOS-Chem grid are not significant (Wecht et al., 2014).
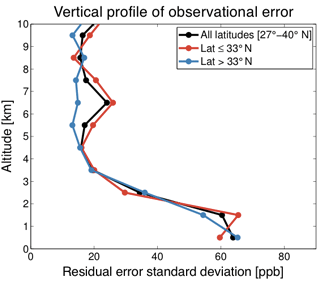
Figure 3Residual standard deviation (RSD) of the difference between SEAC4RS methane observations and the GEOS-Chem model with prior emissions, for 1 km altitude bins. The RSD defines the observational error standard deviations for the inversion as described in the text. Values are shown for two latitudinal bands.
The inversion can, in principle, optimize emissions at the grid resolution of the GEOS-Chem model, representing 3004 grid cells over the inversion domain. But the aircraft observations do not have sufficient information to constrain emissions at that resolution. In order to reduce the dimensionality of the state vector, we project the 3004 grid cells onto 216 elements of a Gaussian mixture model (GMM) with radial basis functions based on spatial proximity and source type patterns (Turner and Jacob, 2015). The use of the GMM allows us to retain high resolution of up to 25 km for major localized sources while degrading resolution in areas of weak or broadly distributed sources. Areas dominated by wetlands have resolution of 100–200 km in the GMM because they are broadly distributed.
The anthropogenic inventory of Maasakkers et al. (2016) and the wetlands inventory of Bloom et al. (2017) both include gridded error estimates that serve as the diagonal elements of the prior error covariance matrix SA. Maasakkers et al. (2016) found no significant spatial error correlation at resolution in their inventory while a variogram analysis across the elements of the WetCHARTS ensemble indicates a spatial error correlation length scale of 130 km. Here we ignore this correlation and take SA to be diagonal.
Figure 1b shows the results of the inversion including the optimized posterior emissions, the corrections to the prior emissions, and the DOFS as measured by the diagonal elements of the averaging kernel matrix. Figure 4 (top panels) compare the observed boundary layer methane concentrations to the values simulated by GEOS-Chem with prior and posterior emissions (Fig. 1). The simulation with prior emissions has a positive bias that is effectively corrected when using posterior emissions. The coefficient of determination (R2) between model and observations increases from 0.30 to 0.50 when using posterior emissions. Figure 4 also evaluates the SEAC4RS inversion results with independent surface air observations from the three NOAA∕ESRL surface network sites in the region (Andrews et al., 2014) and with methane column observations from the TCCON site in Lamont, Oklahoma (Wunch et al., 2011; Wennberg et al., 2017). The posterior emissions improve the simulation of these independent data sets. GOSAT satellite observations are another source of independent data but the 2 month period is too sparse for useful evaluation (Wecht et al., 2014).
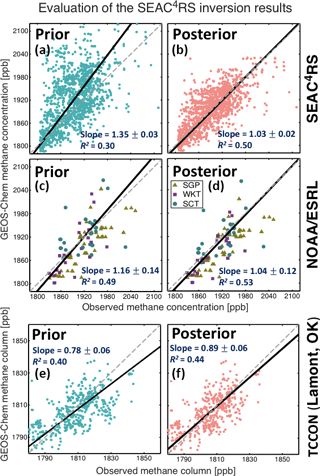
Figure 4Evaluation of the SEAC4RS inversion of methane emissions in the Southeast US for the 6 August–21 September 2013 period. Panels (a, b) compare GEOS-Chem methane concentrations with the SEAC4RS observations, using prior emissions (left) and posterior emissions (right). Panels (c, d) compare GEOS-Chem methane concentrations with independent observations from the three NOAA∕ESRL surface sites in the inversion domain (see Fig. 2 and caption). Panels (e, f) compare GEOS-Chem methane columns with TCCON hourly column observations at Lamont, Oklahoma (Wennberg et al., 2017), after correction for stratospheric bias in the model (Turner et al., 2015). The 1:1 lines (dashed) and the reduced-major-axis(black solid line) linear regressions are also shown, along with the coefficients of determination (R2) and the slopes (±1σ) derived from the bootstrap method.
Total posterior emissions over the SEAC4RS domain are 15 % (4 Tg a−1) lower than the prior estimate (Fig. 1). The inversion is able to constrain about 10 pieces of information in the spatial distribution of methane emissions as measured by the DOFS. It is strongly sensitive to the Gulf Coast and to large anthropogenic source areas such as the Floyd Shale in central Alabama. For the regions with averaging kernel sensitivity larger than 0.05, posterior emissions are 35 % lower than the prior estimate. The posterior errors are 18–30 % over these regions. The scaling factors show large downward corrections of prior emissions in Louisiana and Mississippi, and along the Gulf Coast, where wetlands are the dominant sources. There are also downward corrections in southern West Virginia, where coal mines are dominant, and in the Haynesville Shale gas production region of northern Louisiana and southern Arkansas. On the other hand, there are significant upward corrections for the coal mines of southern Illinois and for the Floyd Shale in central Alabama.
We can attribute the scaling factors from the inversion to specific methane source sectors by using the sector-resolved spatial patterns in the prior emission inventories, as described by Turner et al. (2015) but here with the improved anthropogenic source patterns from Maasakkers et al. (2016) and wetland emissions from (Bloom et al., 2017). Anthropogenic and wetland sources are well separated spatially in these inventories. Figure 5 compares our results with the prior emission totals for the different sectors in the Southeast US. We find a significant 27 % reduction in regional wetland emissions relative to the prior estimate (mean of the WetCHARTs extended ensemble). For the subdomain with averaging kernel sensitivity larger than 0.05 that reduction is 42 %. By contrast, we find no significant regional bias in the EPA anthropogenic inventory for any of the major source sectors for the period of August–September 2013. However, there are large local biases that tend to cancel each other on a regional scale (e.g., Haynesville Shale vs. Floyd Shale for natural gas). This suggests that methane emission factors for the oil and gas sector are more variable than assumed in the EPA (2016) inventory.
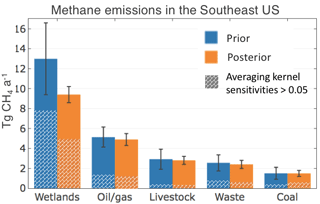
Figure 5Prior and posterior methane emissions in the Southeast US (domain of Fig. 1) for August–September 2013. The prior anthropogenic emissions are from the EPA national inventory for 2012 (EPA, 2016; Maasakkers et al., 2016) and the prior wetland emissions are the means of the WetCHARTs extended ensemble (Bloom et al., 2017). Error bars (one standard deviation) on sectoral emissions are from the prior and posterior error variances of our inversion. Methane emissions in the subdomain with averaging kernel sensitivities larger than 0.05 (Fig. 1) are also indicated.
The WetCHARTs extended ensemble includes 18 wetland methane emission models intended to encompass the uncertainties in estimating wetland emissions (Bloom et al., 2017). The different models (ensemble members) use different datasets for wetland extent fraction A [m2 wetlands m−2 surface area], heterotrophic respiration rate R [mg C day−1 m−2 of wetland area], temperature-dependent factor of C respired as CH4 [mg CH4 mg−1 C] where T is the surface skin temperature, and global scaling factors s. The wetland methane emission flux E [mg CH4 m−2 day−1] at a time t and location x for each of these members is given by the following equation:
The 18-member ensemble consists of three temperature dependence factors (), three global scale factors (), and two wetland extent maps (A) from the Global Lakes and Wetlands Database (GLWD; Lehner and Dölla, 2004) and GLOBCOVER (Bontemps et al., 2011). The heterotrophic respiration rate (R) is the median output from the carbon data model framework (CARDAMOM; Bloom et al., 2016), and is not varied across that ensemble.
Figure 6 shows the Southeast US wetland emissions for each WetCHARTs member, along with the root-mean-square error (RMSE) of its spatial distribution relative to our optimized posterior estimate on the grid. Consistency in spatial distribution with our optimized estimate is indicated by a low RMSE. We find that the specification of wetland extent is the most systematic source of error in wetland emission estimates; all GLOBCOVER-based models underestimate wetland emissions, while all GLWD-based models overestimate emissions. Estimates using q10=1 (no temperature dependence in the CH4 : C respiration ratio) exhibit the lowest RMSE values. The WetCHARTs ensemble mean used as prior for our inversion has the lowest RMSE, although this may reflect its influence on the posterior solution.
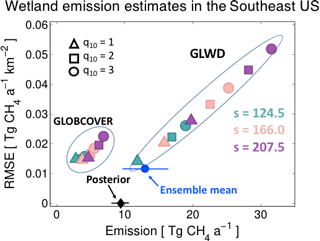
Figure 6Range of wetland emission estimates in the Southeast US (domain of Fig. 1) for August–September 2013. The figure shows the spread of the WetCHARTs extended ensemble and compares with the posterior emission estimate from our inversion in terms of emission total and root-mean-square error (RMSE) on the spatial grid. The RMSE of the posterior emission estimate with itself is zero by definition. WetCHARTs ensemble members use wetland areal extent data from either the GLOBCOVER (Bontemps et al., 2011) or GLWD (Lehner and Dölla, 2004) databases, as well as different estimates of temperature sensitivity q10 and global scaling factors s (see Eq. 5 and text). The posterior wetland emission estimate from our inversion is shown as black solid diamond with its associated error standard deviation. The mean of the WetCHARTs ensemble used as prior for our inversion is shown as blue solid circle with its associated error standard deviation.
We used extensive boundary layer methane observations from the SEAC4RS aircraft campaign over the Southeast US in August–September 2013 to optimize methane emissions in that region with up to spatial resolution and with detailed error characterization. The inversion used new state-of-the-art inventories as prior information, including the gridded version of the EPA (2016) national anthropogenic inventory from Maasakkers et al. (2016) and the WetCHARTs wetlands extended ensemble from Bloom et al. (2017). The inversion domain over the Southeast US accounts for 45 % of national methane emissions in the EPA inventory, and for 56 % of wetland emissions over the contiguous US in the mean WetCHARTs estimate.
Our inversion results suggest that the EPA emission inventory has no significant bias on the regional scale for the major anthropogenic source sectors (livestock, oil and gas, waste, coal), while the mean of the WetCHARTs wetland ensemble needs to be reduced by 27 % over the inversion domain. These results are supported by independent methane observations from the NOAA∕ESRL surface network and from the TCCON site in Lamont, Oklahoma. The specification of wetland areal extent is the dominant source of error in the WetCHARTs ensemble. Results also indicate that a low temperature dependence for the CH4 : C heterotrophic respiration ratio best explains the spatial variability of the posterior emissions. Despite regional agreement with the EPA anthropogenic inventory, we still find significant local discrepancies with the EPA inventory for the oil and gas sector, suggesting that methane emission factors are more variable than assumed in the inventory.
SEAC4RS aircraft methane observations are available at https://www-air.larc.nasa.gov/cgi-bin/ArcView/seac4rs\#BLAKE.DONALD/ (last access: 1 November 2017). TCCON data (Wunch et al., 2011; Wennberg et al., 2017) are available at http://tccondata.org (last access: 1 November 2017). The NOAA data (Andrews et al., 2014) are available at https://www.esrl.noaa.gov/gmd/ccgg/obspack/ (last access: 1 November 2017).
The authors declare that they have no conflict of interest.
This work was funded by the NASA Earth Science Division. Part of
this research was carried out at the Jet Propulsion Laboratory,
California Institute of Technology, under a contract with
NASA. Special thanks to Donald R. Blake for providing SEAC4RS aircraft
methane observations (available at
https://www-air.larc.nasa.gov/cgi-bin/ArcView/seac4rs#BLAKE.DONALD/, last access: 1 November 2017). TCCON
data were obtained from the TCCON Data Archive, hosted by
CaltechData (http://tccondata.org, last access: 1 November 2017). The NOAA data are
available from the ObsPack portal
(https://www.esrl.noaa.gov/gmd/ccgg/obspack/, last access: 1 November 2017).
Edited by: Christoph Gerbig
Reviewed by: two
anonymous referees
Alexe, M., Bergamaschi, P., Segers, A., Detmers, R., Butz, A., Hasekamp, O., Guerlet, S., Parker, R., Boesch, H., Frankenberg, C., Scheepmaker, R. A., Dlugokencky, E., Sweeney, C., Wofsy, S. C., and Kort, E. A.: Inverse modelling of CH4 emissions for 2010–2011 using different satellite retrieval products from GOSAT and SCIAMACHY, Atmos. Chem. Phys., 15, 113–133, https://doi.org/10.5194/acp-15-113-2015, 2015. a, b
Andrews, A. E., Kofler, J. D., Trudeau, M. E., Williams, J. C., Neff, D. H., Masarie, K. A., Chao, D. Y., Kitzis, D. R., Novelli, P. C., Zhao, C. L., Dlugokencky, E. J., Lang, P. M., Crotwell, M. J., Fischer, M. L., Parker, M. J., Lee, J. T., Baumann, D. D., Desai, A. R., Stanier, C. O., De Wekker, S. F. J., Wolfe, D. E., Munger, J. W., and Tans, P. P.: CO2, CO, and CH4 measurements from tall towers in the NOAA Earth System Research Laboratory's Global Greenhouse Gas Reference Network: instrumentation, uncertainty analysis, and recommendations for future high-accuracy greenhouse gas monitoring efforts, Atmos. Meas. Tech., 7, 647–687, https://doi.org/10.5194/amt-7-647-2014, 2014. a, b
Bloom, A. A., Exbrayat, J.-F., van der Velde, I. R., Feng, L., and Williams, M.: The decadal state of the terrestrial carbon cycle: Global retrievals of terrestrial carbon allocation, pools, and residence times, P. Natl. Acad. Sci. USA, 113, 1285–1290, https://doi.org/10.1073/pnas.1515160113, 2016. a
Bloom, A. A., Bowman, K. W., Lee, M., Turner, A. J., Schroeder, R., Worden, J. R., Weidner, R., McDonald, K. C., and Jacob, D. J.: A global wetland methane emissions and uncertainty dataset for atmospheric chemical transport models (WetCHARTs version 1.0), Geosci. Model Dev., 10, 2141–2156, https://doi.org/10.5194/gmd-10-2141-2017, 2017. a, b, c, d, e, f, g
Bontemps, S., Defourny, P., Bogaert, E. V., Arino, O., Kalogirou, V., and Perez, J R.: GLOBCOVER 2009: Products Description and Validation Report, Tech. rep., ESA, 2, 2011. a, b
Brandt, A. R., Heath, G. A., Kort, E. A., O'Sullivan, F., Pétron, G., Jordaan, S. M., Tans, P., Wilcox, J., Gopstein, A. M., Arent, D., Wofsy, S., Brown, N. J., Bradley, R., Stucky, G. D., Eardley, D., and Harriss, R.: Methane Leaks from North American Natural Gas Systems, Science, 343, 733–735, https://doi.org/10.1126/science.1247045, 2014. a
Brasseur, G. P. and Jacob, D. J.: Modeling of Atmospheric Chemistry, 1st Edn., Cambridge University Press, 2017. a
Chan Miller, C., Jacob, D. J., Marais, E. A., Yu, K., Travis, K. R., Kim, P. S., Fisher, J. A., Zhu, L., Wolfe, G. M., Hanisco, T. F., Keutsch, F. N., Kaiser, J., Min, K.-E., Brown, S. S., Washenfelder, R. A., González Abad, G., and Chance, K.: Glyoxal yield from isoprene oxidation and relation to formaldehyde: chemical mechanism, constraints from SENEX aircraft observations, and interpretation of OMI satellite data, Atmos. Chem. Phys., 17, 8725–8738, https://doi.org/10.5194/acp-17-8725-2017, 2017. a
EPA: Inventory of US Greenhouse Gas Emissions and Sinks 1990–2011, US Environmental Protection Agency, Washington DC, 2013. a, b
EPA: Inventory of US Greenhouse Gas Emissions and Sinks 1990–2014, US Environmental Protection Agency, Washington DC, 2014. a, b
EPA: Inventory of US Greenhouse Gas Emissions and Sinks 1990–2014,US Environmental Protection Agency, Washington DC, 2016. a, b, c, d, e
European Commission: Emission Database for Global Atmospheric Research (EDGAR), release version 4.2, Tech. rep., Joint Research Centre (JRC)/Netherlands Environmental Assessment Agency (PBL), available at: http://edgar.jrc.ec.europa.eu (last access: 1 November 2017), 2011 a
Fisher, J. A., Jacob, D. J., Travis, K. R., Kim, P. S., Marais, E. A., Chan Miller, C., Yu, K., Zhu, L., Yantosca, R. M., Sulprizio, M. P., Mao, J., Wennberg, P. O., Crounse, J. D., Teng, A. P., Nguyen, T. B., St. Clair, J. M., Cohen, R. C., Romer, P., Nault, B. A., Wooldridge, P. J., Jimenez, J. L., Campuzano-Jost, P., Day, D. A., Hu, W., Shepson, P. B., Xiong, F., Blake, D. R., Goldstein, A. H., Misztal, P. K., Hanisco, T. F., Wolfe, G. M., Ryerson, T. B., Wisthaler, A., and Mikoviny, T.: Organic nitrate chemistry and its implications for nitrogen budgets in an isoprene- and monoterpene-rich atmosphere: constraints from aircraft (SEAC4RS) and ground-based (SOAS) observations in the Southeast US, Atmos. Chem. Phys., 16, 5969–5991, https://doi.org/10.5194/acp-16-5969-2016, 2016. a
Heald, C. L., Jacob, D. J., Jones, D. B. A., Palmer, P. I., Logan, J. A., Streets, D. G., Sachse, G. W., Gille, J. C., Hoffman, R. N., and Nehrkorn, T.: Comparative inverse analysis of satellite (MOPITT) and aircraft (TRACE-P) observations to estimate Asian sources of carbon monoxide, J. Geophys. Res., 109, D23306, https://doi.org/10.1029/2004JD005185, 2004. a
Henze, D. K., Hakami, A., and Seinfeld, J. H.: Development of the adjoint of GEOS-Chem, Atmos. Chem. Phys., 7, 2413–2433, https://doi.org/10.5194/acp-7-2413-2007, 2007.
Jacob, D. J., Turner, A. J., Maasakkers, J. D., Sheng, J., Sun, K., Liu, X., Chance, K., Aben, I., McKeever, J., and Frankenberg, C.: Satellite observations of atmospheric methane and their value for quantifying methane emissions, Atmos. Chem. Phys., 16, 14371–14396, https://doi.org/10.5194/acp-16-14371-2016, 2016. a, b
Kim, P. S., Jacob, D. J., Fisher, J. A., Travis, K., Yu, K., Zhu, L., Yantosca, R. M., Sulprizio, M. P., Jimenez, J. L., Campuzano-Jost, P., Froyd, K. D., Liao, J., Hair, J. W., Fenn, M. A., Butler, C. F., Wagner, N. L., Gordon, T. D., Welti, A., Wennberg, P. O., Crounse, J. D., St. Clair, J. M., Teng, A. P., Millet, D. B., Schwarz, J. P., Markovic, M. Z., and Perring, A. E.: Sources, seasonality, and trends of southeast US aerosol: an integrated analysis of surface, aircraft, and satellite observations with the GEOS-Chem chemical transport model, Atmos. Chem. Phys., 15, 10411–10433, https://doi.org/10.5194/acp-15-10411-2015, 2015. a, b
Lehner, B. and Dölla, P.: Development and validation of a global database of lakes, reservoirs and wetlands, J. Hydrol., 296, 1–22, https://doi.org/10.1016/j.jhydrol.2004.03.028, 2004. a, b
Lyon, D. R., Zavala-Araiza, D., Alvarez, R. A., Harriss, R., Palacios, V., Lan, X., Talbot, R., Lavoie, T., Shepson, P., Yacovitch, T. I., Herndon, S. C., Marchese, A. J., Zimmerle, D., Robinson, A. L., and Hamburg, S. P.: Constructing a Spatially Resolved Methane Emission Inventory for the Barnett Shale Region, Environ. Sci. Technol., 49, 8147–8157, https://doi.org/10.1021/es506359c, 2015. a
Maasakkers, J. D., Jacob, D. J., Sulprizio, M. P., Turner, A. J., Weitz, M., Wirth, T., Hight, C., DeFigueiredo, M., Desai, M., Schmeltz, R., Hockstad, L., Bloom, A. A., Bowman, K. W., Jeong, S., and Fischer, M. L.: Gridded National Inventory of US Methane Emissions, Environ. Sci. Technol., 50, 13123–13133, https://doi.org/10.1021/acs.est.6b02878, 2016. a, b, c, d, e, f, g, h, i, j, k
Marais, E. A., Jacob, D. J., Jimenez, J. L., Campuzano-Jost, P., Day, D. A., Hu, W., Krechmer, J., Zhu, L., Kim, P. S., Miller, C. C., Fisher, J. A., Travis, K., Yu, K., Hanisco, T. F., Wolfe, G. M., Arkinson, H. L., Pye, H. O. T., Froyd, K. D., Liao, J., and McNeill, V. F.: Aqueous-phase mechanism for secondary organic aerosol formation from isoprene: application to the southeast United States and co-benefit of SO2 emission controls, Atmos. Chem. Phys., 16, 1603–1618, https://doi.org/10.5194/acp-16-1603-2016, 2016. a
Melton, J. R., Wania, R., Hodson, E. L., Poulter, B., Ringeval, B., Spahni, R., Bohn, T., Avis, C. A., Beerling, D. J., Chen, G., Eliseev, A. V., Denisov, S. N., Hopcroft, P. O., Lettenmaier, D. P., Riley, W. J., Singarayer, J. S., Subin, Z. M., Tian, H., Zürcher, S., Brovkin, V., van Bodegom, P. M., Kleinen, T., Yu, Z. C., and Kaplan, J. O.: Present state of global wetland extent and wetland methane modelling: conclusions from a model inter-comparison project (WETCHIMP), Biogeosciences, 10, 753–788, https://doi.org/10.5194/bg-10-753-2013, 2013. a
Miller, S. M., Wofsy, S. C., Michalak, A. M., Kort, E. A., Andrews, A. E., Biraud, S. C., Dlugokencky, E. J., Eluszkiewicz, J., Fischer, M. L., Janssens-Maenhout, G., Miller, B. R., Miller, J. B., Montzka, S. A., Nehrkorn, T., and Sweeney, C.: Anthropogenic emissions of methane in the United States, P. Natl. Acad. Sci. USA, 110, 20018–20022, https://doi.org/10.1073/pnas.1314392110, 2013. a, b
Myhre, G., Shindell, D., Bréon, F.-M., Collins, W., Fuglestvedt, J., Huang, J., Koch, D., Lamarque, J.-F., Lee, D., Mendoza, B., Nakajima, T., Robock, A., Stephens, G., Takemura, T., and Zhang, H.: Anthropogenic and Natural Radiative Forcing, in: Climate Change 2013: The Physical Science Basis. Contribution of Working Group I to the Fifth Assessment Report of the Intergovernmental Panel on Climate Change, edited by: Stocker, T. F., Qin, D., Plattner, G.-K., Tignor, M., Allen, S. K., Boschung, J., Nauels, A., Xia, Y., Bex, V. and Midgley, P. M., Cambridge University Press, Cambridge, UK and New York, NY, USA, 2013. a
Park, R. J., Jacob, D. J., Field, B. D., Yantosca, R. M., and Chin, M.: Natural and transboundary pollution influences on sulfate-nitrate-ammonium aerosols in the United States: Implications for policy, J. Geophys. Res., 109, D15204, https://doi.org/10.1029/2003JD004473, 2004. a
Prather, M. J., Holmes, C. D., and Hsu, J.: Reactive greenhouse gas scenarios: Systematic exploration of uncertainties and the role of atmospheric chemistry, Geophys. Res. Lett., 39, L09803, https://doi.org/10.1029/2012GL051440, 2012. a
Rodgers, C. D.: Inverse Methods for Atmospheric Sounding: Theory and Practice, World Scientific Publishing, Singapore, 2000. a
Sheng, J.-X., Jacob, D. J., Maasakkers, J. D., Sulprizio, M. P., Zavala-Araiza, D., and Hamburg, S. P.: A high-resolution () inventory of methane emissions from Canadian and Mexican oil and gas systems, Atmos. Environ., 158, 211–215, https://doi.org/10.1016/j.atmosenv.2017.02.036, 2017. a
Simpson, I. J., Chenm, T.-Y., Blake, D. R., and Rowland, F. S.: Implications of the recent fluctuations in the growth rate of tropospheric methane, Geophys. Res. Lett., 29, 1171–1174, https://doi.org/10.1029/2001GL014521, 2002. a
Toon, O. B., Maring, H., Dibb, J., Ferrare, R., Jacob, D. J., Jensen, E. J., Luo, Z. J., Mace, G. G., Pan, L. L., Pfister, L., Rosenlof, K. H., Redemann, J., Reid, J. S., Singh, H. B., Thompson, A. M., Yokelson, R., Minnis, P., Chen, G., Jucks, K. W., and Pszenny, A.: Planning, implementation, and scientific goals of the Studies of Emissions and Atmospheric Composition, Clouds and Climate Coupling by Regional Surveys (SEAC4rs) field mission, J. Geophys. Res.-Atmos., 121, 2015JD024297, https://doi.org/10.1002/2015JD024297, 2016. a
Travis, K. R., Jacob, D. J., Fisher, J. A., Kim, P. S., Marais, E. A., Zhu, L., Yu, K., Miller, C. C., Yantosca, R. M., Sulprizio, M. P., Thompson, A. M., Wennberg, P. O., Crounse, J. D., St. Clair, J. M., Cohen, R. C., Laughner, J. L., Dibb, J. E., Hall, S. R., Ullmann, K., Wolfe, G. M., Pollack, I. B., Peischl, J., Neuman, J. A., and Zhou, X.: Why do models overestimate surface ozone in the Southeast United States?, Atmos. Chem. Phys., 16, 13561–13577, https://doi.org/10.5194/acp-16-13561-2016, 2016. a
Turner, A. J. and Jacob, D. J.: Balancing aggregation and smoothing errors in inverse models, Atmos. Chem. Phys., 15, 7039–7048, https://doi.org/10.5194/acp-15-7039-2015, 2015. a
Turner, A. J., Jacob, D. J., Wecht, K. J., Maasakkers, J. D., Lundgren, E., Andrews, A. E., Biraud, S. C., Boesch, H., Bowman, K. W., Deutscher, N. M., Dubey, M. K., Griffith, D. W. T., Hase, F., Kuze, A., Notholt, J., Ohyama, H., Parker, R., Payne, V. H., Sussmann, R., Sweeney, C., Velazco, V. A., Warneke, T., Wennberg, P. O., and Wunch, D.: Estimating global and North American methane emissions with high spatial resolution using GOSAT satellite data, Atmos. Chem. Phys., 15, 7049–7069, https://doi.org/10.5194/acp-15-7049-2015, 2015. a, b, c, d, e, f, g
Turner, A. J., Frankenberg, C., Wennberg, P. O., and Jacob, D. J.: Ambiguity in the causes for decadal trends in atmospheric methane and hydroxyl, P. Natl. Acad. Sci. USA, 114, 5367–5372, https://doi.org/10.1073/pnas.1616020114, 2017. a
United Nation: United Nations Framework Convention on Climate Change, Article 4(1)(a), available at: https://unfccc.int/resource/docs/convkp/conveng.pdf (last access: November 2017), 1992. a
Wecht, K. J., Jacob, D. J., Sulprizio, M. P., Santoni, G. W., Wofsy, S. C., Parker, R., Bösch, H., and Worden, J.: Spatially resolving methane emissions in California: constraints from the CalNex aircraft campaign and from present (GOSAT, TES) and future (TROPOMI, geostationary) satellite observations, Atmos. Chem. Phys., 14, 8173–8184, https://doi.org/10.5194/acp-14-8173-2014, 2014. a, b, c
Wennberg, P. O., Wunch, D., Roehl, C., Blavier, J. F., Toon, G. C., Allen, N., Dowell, P., Teske, K., Martin, C., and Martin, J.: TCCON data from Lamont, Oklahoma, USA, Release GGG2014r1, TCCON data archive, hosted by CaltechDATA, California Institute of Technology, Pasadena, CA, USA, https://doi.org/10.14291/tccon.ggg2014.lamont01.R1/1255070, 2017. a, b, c
Wolf, J., Asrar, G. R., and West, T. O.: Revised methane emissions factors and spatially distributed annual carbon fluxes for global livestock, Carbon Balance and Management, 12, 16, https://doi.org/10.1186/s13021-017-0084-y, 2017. a
Wunch, D., Toon, G. C., Blavier, J.-F. L., Washenfelder, R. A., Notholt, J., Connor, B. J., Griffith, D. W. T., Sherlock, V., and Wennberg, P. O.: The Total Carbon Column Observing Network, Philos. T. R. Soc. A, 369, 2087–2112, https://doi.org/10.1098/rsta.2010.0240, 2011. a, b
Yu, K., Jacob, D. J., Fisher, J. A., Kim, P. S., Marais, E. A., Miller, C. C., Travis, K. R., Zhu, L., Yantosca, R. M., Sulprizio, M. P., Cohen, R. C., Dibb, J. E., Fried, A., Mikoviny, T., Ryerson, T. B., Wennberg, P. O., and Wisthaler, A.: Sensitivity to grid resolution in the ability of a chemical transport model to simulate observed oxidant chemistry under high-isoprene conditions, Atmos. Chem. Phys., 16, 4369–4378, https://doi.org/10.5194/acp-16-4369-2016, 2016. a
Zhu, L., Jacob, D. J., Kim, P. S., Fisher, J. A., Yu, K., Travis, K. R., Mickley, L. J., Yantosca, R. M., Sulprizio, M. P., De Smedt, I., González Abad, G., Chance, K., Li, C., Ferrare, R., Fried, A., Hair, J. W., Hanisco, T. F., Richter, D., Jo Scarino, A., Walega, J., Weibring, P., and Wolfe, G. M.: Observing atmospheric formaldehyde (HCHO) from space: validation and intercomparison of six retrievals from four satellites (OMI, GOME2A, GOME2B, OMPS) with SEAC4RS aircraft observations over the southeast US, Atmos. Chem. Phys., 16, 13477–13490, https://doi.org/10.5194/acp-16-13477-2016, 2016. a