the Creative Commons Attribution 4.0 License.
the Creative Commons Attribution 4.0 License.
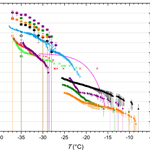
Coal fly ash: linking immersion freezing behavior and physicochemical particle properties
Stefanie Augustin-Bauditz
Hans-Christian Clemen
Martin Ebert
Stine Eriksen Hammer
Jasmin Lubitz
Naama Reicher
Yinon Rudich
Johannes Schneider
Robert Staacke
Frank Stratmann
André Welti
Heike Wex
To date, only a few studies have investigated the potential of coal fly ash particles to trigger heterogeneous ice nucleation in cloud droplets. The presented measurements aim at expanding the sparse dataset and improving process understanding of how physicochemical particle properties can influence the freezing behavior of coal fly ash particles immersed in water.
Firstly, immersion freezing measurements were performed with two single particle techniques, i.e., the Leipzig Aerosol Cloud Interaction Simulator (LACIS) and the SPectrometer for Ice Nuclei (SPIN). The effect of suspension time on the efficiency of the coal fly ash particles when immersed in a cloud droplet is analyzed based on the different residence times of the two instruments and employing both dry and wet particle generation. Secondly, two cold-stage setups, one using microliter sized droplets (Leipzig Ice Nucleation Array) and one using nanoliter sized droplets (WeIzmann Supercooled Droplets Observation on Microarray setup) were applied.
We found that coal fly ash particles are comparable to mineral dust in their immersion freezing behavior when being dry generated. However, a significant decrease in immersion freezing efficiency was observed during experiments with wet-generated particles in LACIS and SPIN. The efficiency of wet-generated particles is in agreement with the cold-stage measurements. In order to understand the reason behind the deactivation, a series of chemical composition, morphology, and crystallography analyses (single particle mass spectrometry, scanning electron microscopy coupled with energy dispersive X-ray microanalysis, X-ray diffraction analysis) were performed with dry- and wet-generated particles. From these investigations, we conclude that anhydrous CaSO4 and CaO – which, if investigated in pure form, show the same qualitative immersion freezing behavior as observed for dry-generated coal fly ash particles – contribute to triggering heterogeneous ice nucleation at the particle–water interface. The observed deactivation in contact with water is related to changes in the particle surface properties which are potentially caused by hydration of CaSO4 and CaO. The contribution of coal fly ash to the ambient population of ice-nucleating particles therefore depends on whether and for how long particles are immersed in cloud droplets.
- Article
(11258 KB) - Full-text XML
-
Supplement
(27732 KB) - BibTeX
- EndNote
It is known that naturally occurring aerosol such as biological particles (e.g., bacteria, pollen, spores) and mineral dust are acting as ice-nucleating particles (INPs; Hoose and Möhler, 2012 and references therein). In contrast, there is an ongoing discussion about the impact of anthropogenic aerosol emissions on the concentration of atmospheric INPs (e.g., Szyrmer and Zawadzki, 1997; Chen et al., 2018). The strongest source of anthropogenic aerosol is the combustion of fossil fuels, where primary particles such as carbonaceous aerosol and ash, as well as secondary particles from gaseous precursors, are generated.
Carbonaceous aerosol, such as soot, which is a product of incomplete combustion of organic material, has been shown to act as INPs (e.g., DeMott, 1990; Diehl and Mitra, 1998; Fornea et al., 2009). However, there are large discrepancies between studies investigating the ice nucleation ability of soot, which might be related to the source and/or mixing state of the particles (Kanji et al., 2017). Hoose and Möhler (2012) summarize that “soot is a generally worse ice nucleus than mineral dust”. In contrast to soot and other carbonaceous aerosol types, ash only contains a limited amount of carbon. Defined as the solid remains from the combustion of organic substances, e.g., wood or fossil fuels, it mostly consists of the noncombustible constituents in the fuel, i.e., mineral inclusions and atoms other than C and H, e.g., K, Ca, Mn, Fe, etc. (Flagan and Seinfeld, 1988a). A distinction is made between the fine ash fraction, i.e., fly ash, that is emitted during combustion together with flue gases, and the coarse ash fraction, i.e., bottom ash. The latter is defined as the fraction that remains in the power plant, fireplace, or on the ground after a wildfire and can be emitted due to wind erosion.
Coal is difficult to substitute in the energy mix of most industrial countries and hence it is only slowly replaced by renewable energy sources (U. S. Energy Information Administration, 2017). In total, 6711 coal-fired power plants (30 MW and larger; endcoal.org, 2017; status: July 2017) are in operation worldwide, producing 600 Mt a−1 of coal ash (Ahmaruzzaman, 2010). The vast majority of this mass is not emitted into the atmosphere, as coal-fired power plants are equipped with different types of particle removal technology to clean flue gases of coal fly ash (CFA). Estimating CFA emissions is not trivial, because filtering systems show varying efficiencies and part of the collected CFA is emitted during disposal (Mueller et al., 2013). A rough assessment was given by Smil (2008), estimating that 30 Mt a−1 of CFA are released into the atmosphere worldwide. Reff et al. (2009) state that coal combustion causes PM2.5 emissions of ∼0.5 Mt a−1 in the USA. In addition to a large uncertainty in these estimates, there is no detailed information about temporal and spatial variability in CFA emission and dispersion, which is important for assessing the effect of CFA particles on cloud formation and glaciation.
A lot of research has already been conducted in the field of CFA sample characterization for identifying CFA particles in the atmosphere. This was mainly driven by concerns about the negative effects of CFA particles on human health (e.g., Davison et al., 1974; Damle et al., 1982; Yi et al., 2006; and references therein). These studies show that CFA has a complex and highly variable composition. Except for some trace elements whose contents are heterogeneously distributed among different size fractions, CFA composition is comparable to mineral dust, making it difficult to identify via single particle mass spectrometry (Cziczo et al., 2004, 2006; Kamphus et al., 2010). CFA particles are, in contrast to irregularly shaped mineral dust particles, often spherical because of their generation process, where minerals melt and form spherical droplets that retain their shape upon solidification (Damle et al., 1982; Flagan and Seinfeld, 1988a). However, shape is not a perfect criterion for identifying CFA, as other high-temperature processes such as fuel-oil combustion or metal processing also emit spherical fly ash particles. In addition, there are various aerosol types which occur in spherical shapes, e.g., biological particles (Huffman et al., 2012), tar balls (Laskin et al., 2006; Sedlacek III et al., 2018), or deliquesced salt particles (Freney et al., 2009). In conclusion, a reliable identification of CFA particles is not trivial and requires a combination of chemical composition and morphology analyses (e.g., DeMott et al., 2003; Weinbruch et al., 2010, 2012).
Concerning the ice nucleation activity of ash particles, only few studies have been published so far. Early investigations of aerosol from coal-fired power plant plumes were contradictory as to whether the particles are able to act as INPs (Parungo et al., 1978) or not (Schnell et al., 1976). More recent studies (Havlíček et al., 1993; Umo et al., 2015; Garimella, 2016; Grawe et al., 2016) agreed that ash particles indeed trigger heterogeneous ice nucleation. Havlíček et al. (1993) investigated chemical composition and ice nucleation characteristics of CFA from nine different power plants in the former Czechoslovakia focusing on the effect of water-soluble material in the samples. The chemical composition analysis showed that the water-soluble fraction of the samples varied between 0.43 and 1.34 wt % and mainly consisted of anhydrite (anhydrous CaSO4). Ice nucleation experiments were carried out with two methods. Firstly, polydisperse CFA particles were aerosolized in a thermodiffusion chamber subsaturated with respect to liquid water at −15 ∘C, i.e., only deposition nucleation was investigated. Secondly, suspensions of CFA in distilled water were used to produce droplets onto a cooled plate (cold stage), i.e., immersion freezing was investigated. The water-soluble components were separated from all samples and ice nucleation experiments were carried out with the original samples, the water-insoluble components, and the water-soluble components. Immersion freezing was found to be less efficient than deposition nucleation in all cases. The water-insoluble components were up to 3 orders of magnitude less efficient in the deposition mode than the untreated samples. However, when the water-soluble components alone were investigated, they showed surprisingly low efficiency. This finding illustrates the complex interplay of physicochemical particle properties and freezing behavior, as the water-soluble components increased the ice nucleation efficiency only when associated with the CFA particles.
Four ash samples including CFA, coal bottom ash, wood bottom ash, and bottom ash from a domestic oven were investigated by Umo et al. (2015). The immersion freezing behavior was quantified using a cold-stage setup (Whale et al., 2015). In comparison to the bottom ashes, CFA was more efficient at nucleating ice between −17 and −27 ∘C, showing a strong increase starting at −16 ∘C and an apparent plateau below roughly −24 ∘C. The bottom ashes behaved similar to one another, with a slight trend of coal bottom ash being less efficient and wood bottom ash being more efficient.
Garimella (2016) investigated the freezing behavior of four different CFA samples from the USA using the SPectrometer for Ice Nuclei (SPIN; Droplet Measurement Technologies Inc., Boulder, CO, USA). In this study, particles were dry-generated and size selected. Activated fractions of 1 % were observed at T<−30 ∘C (1.25<Sice<1.4) for deposition nucleation and at T<−20 ∘C for immersion freezing. This is contradictory to the measurements by Havlíček et al. (1993) who found deposition nucleation to be more efficient than immersion freezing. When comparing measurements of CFA by Garimella (2016) and Umo et al. (2015), a discrepancy of more than 1 order of magnitude was found, with the cold-stage measurements being below the immersion freezing measurements with SPIN. In addition, Garimella (2016) showed that 300 nm particles are more efficient per unit surface area than 700 nm particles, possibly indicating that trace metals, which are enriched in smaller particles due to size-dependent cooling rates, could contribute to the immersion freezing efficiency. This could explain why the results by Umo et al. (2015), where the size distribution of immersed particles had a mode diameter of ∼10 µm, were much lower.
Previously (Grawe et al., 2016), we investigated the freezing behavior of wood bottom ash, coal bottom ash, and CFA. Experiments were performed with the Leipzig Aerosol Cloud Interaction Simulator (LACIS; Hartmann et al., 2011), a laminar flow tube in which single, size-selected particles are activated to droplets and cooled down to investigate immersion freezing (see Sect. 2.3.1). It was found that dry-generated CFA particles showed the highest immersion freezing efficiency of the examined samples, being only slightly less efficient below −27 ∘C than a K-feldspar sample (Augustin-Bauditz et al., 2014). Interestingly, a change in immersion freezing efficiency could be seen in the transition to wet particle generation, i.e., producing ash suspensions which were sprayed with an atomizer and sent through a dryer. In this case, a decrease towards the limit of detection was observed. As the size of dry- and wet-generated particles was identical, the deactivation contradicts the proposed hypothesis of Garimella (2016) that the size dependent enrichment of trace elements causes the discrepancy between measurements with single particle instruments and cold stages.
The presented study intends to function as a follow-up to our previous paper and aims at answering the following questions:
-
Do CFA samples from different power plants feature a similar immersion freezing behavior?
-
Is the deactivation of the ice nucleation properties in the transition from dry to wet particle generation observable for different CFA samples?
-
Is it possible to find a connection between physicochemical sample properties and the observed immersion freezing behavior?
-
Which particle generation technique (dry or wet particle generation) or measurement method (single particle or cold stage) is appropriate for representing atmospheric processes after CFA emission?
Four CFA samples from German power plants were investigated as immersion freezing INPs in an attempt to answer these questions. Additional sample characterization with respect to chemical composition, morphology, and crystallography, was performed and used for interpretation of the immersion freezing results.
The immersion freezing and particle characterization measurements of CFA particles were performed during a campaign at TROPOS in November 2016 together with collaborators from the Ice Nuclei research UnIT (INUIT). The main setup (see Fig. 1) consists of particle generation, size selection, and distribution of the size-selected aerosol to the following instruments: (1) LACIS, (2) SPIN, (3) the Aircraft-based Laser ABlation Aerosol MAss spectrometer (ALABAMA), and (4) the multi-Micro INertial Impactor (multi-MINI) sampling particles onto substrates for environmental scanning electron microscopy coupled with energy dispersive X-ray spectroscopy (ESEM/EDX). In addition to LACIS and SPIN, immersion freezing measurements were performed with two cold-stage setups: (1) the Leipzig Ice Nucleation Array (LINA) and (2) the WeIzmann Supercooled Droplets Observation on Microarray (WISDOM) setup. For this, suspensions of CFA in water were prepared using the bulk material, which is why further bulk analyses regarding chemical composition and crystallography were performed.
2.1 Origin of the samples
The CFA samples were taken from the electrostatic precipitators1 of four coal-fired power plants in Germany. It is unknown which flue gas desulfurization technique is applied in the power plants or whether the electrostatic precipitators are installed up- or downstream of the flue gas desulfurization systems. This technical information could either not be obtained, or is unknown to us because the sample origin must remain anonymous. CFA1 is identical to the CFA sample from Grawe et al. (2016) and originates from the Lippendorf power plant situated 15 km south of Leipzig, Germany. CFA1, CFA2, and CFA4 are from brown (sub-bituminous) coal combustion, CFA3 is from black (bituminous) coal combustion.
Quicklime (CaO), anhydrite (CaSO4), and gypsum (CaSO4•2H2O) from Merck KGaA (Darmstadt, Germany) were used for additional investigations.
2.2 Sample preparation and particle generation
Two different kinds of particle generation were used in connection with the LACIS and SPIN immersion freezing experiments: dry particle generation, i.e., aerosolization of particles from dry ash powder, and wet particle generation, i.e., atomization of a CFA–water suspension. Suspensions were also used for the experiments with LINA and WISDOM.
2.2.1 Dry particle generation
The dry CFA samples were placed into an aerosol generator operating via pressurized air and an electric imbalance motor (Grawe et al., 2016). The samples were not sieved prior to aerosol generation. The aerosol was sent through a mixing bottle and a cyclone (D50=500 nm) to reduce the amount of large particles in the flow. Further downstream, a neutralizer was passed, before a differential mobility analyzer (DMA, Vienna type, medium) was used for size selection. A mobility diameter of 300 nm was chosen for the immersion freezing experiments with LACIS and SPIN for two reasons. Firstly, electrostatic precipitators have a minimum collection efficiency for particle sizes between 0.1 and 1 µm (Flagan and Seinfeld, 1988b; Nóbrega et al., 2004; Kim et al., 2012), meaning that CFA particles in this size range are more likely to be emitted compared to smaller or larger particles. Secondly, 300 nm particles will experience relevant atmospheric residence times once emitted (Jaenicke, 1978).
Afterwards, the quasi-monodisperse aerosol was distributed to a condensation particle counter (CPC, model 3010, TSI Inc., St. Paul, MN, USA), LACIS, SPIN, multi-MINI, and ALABAMA. ALABAMA measurements of vacuum aerodynamic diameter were used for multiply charged fraction determination. The multiple charge correction was made using frozen fraction values (fice, number of frozen hydrometeors divided by total number of hydrometeors). The method and results are described in Sect. S7 in the Supplement. Unfortunately, only data acquired in parallel to ALABAMA measurements could be corrected. Data without multiple charge correction are indicated.
2.2.2 Preparation of CFA suspensions for cold stages and wet particle generation
The CFA–water suspensions for LACIS, SPIN, and LINA measurements were prepared following the description in Umo et al. (2015). Briefly, a certain amount of CFA was mixed with distilled water (LINA: 0.1 g CFA in 100 mL water, LACIS and SPIN: 0.5 g CFA in 100 mL water) and ultrasonicated (RK100H Sonorex Super, BANDELIN electronic GmbH & Co. KG, Berlin, Germany) for 10 min. Afterwards, the suspension was stirred with a magnetic stirrer for 24 h. This approach was chosen to allow comparability to results by Umo et al. (2015) and Grawe et al. (2016). The procedure helps with breaking up large aggregates and hence prevents fast sedimentation that would lead to an uneven distribution of material in the droplets on LINA. As sedimentation is not a limiting factor for wet particle generation (a flask shaker was used), LACIS measurements were performed with both the standard suspensions (ultrasonification and 24 h stirring) and suspensions that were prepared right before the experiment by simply mixing 0.5 g CFA with 100 mL distilled water. In this way, particles were in suspension for no more than 5 min before being used for LACIS measurements. Due to instrument availability, SPIN measurements could only be performed with the standard suspensions. The suspensions, either fresh or standard, were sprayed with an atomizer (similar to Model 3076, TSI Inc., St. Paul, MN, USA) and resulting droplets were sent through a diffusion dryer. Then, size selection of the particles by the DMA and distribution to LACIS, SPIN, CPC, multi-MINI, and ALABAMA took place.
In contrast to LINA measurements, size selection of the CFA samples was necessary for WISDOM because large particles that are present in the original sample would clog the microfluidic device which is used for droplet production (see Sect. 2.3.4). Size selection took place by running dry particle generation (aerosol generator, mixing bottle, cyclone) for several hours and collecting the accumulated material from the cyclone (D50=450 nm). During this procedure, coarse material was deposited inside the mixing bottle and a subfraction of the bulk, hereafter referred to as fine CFA, remained in the cyclone. Suspensions of 0.1 g fine CFA in 100 mL distilled water were mixed for three cycles of 30 s each with 10 s break in a small-volume sonicator (UP200St, Hielscher Ultrasonics GmbH, Teltow, Germany) and were used for droplet production and immersion freezing experiments within 2 min.
2.3 Immersion freezing instrumentation
2.3.1 LACIS
LACIS is a laminar flow tube consisting of seven 1 m long sections, each temperature controlled by individual thermostats. At the inlet, the aerosol flow is enclosed by a humidified sheath flow. As a result, a stable 2 mm wide particle beam is created along the LACIS centerline, ensuring that all particles experience identical thermodynamic conditions. Supersaturation is created by adjusting the dew point of the sheath air and the wall temperature. Like this, each particle is activated to a droplet before being cooled down for immersion freezing investigation. The ice nucleation time in LACIS is 1.6 s.
Supercooled liquid droplets and ice particles coexist at the outlet of the tube in a certain temperature range above the homogeneous freezing limit. The thermo-stabilized Optical Particle Spectrometer for the detection of Ice (TOPS-Ice; Clauß et al., 2013) is used to determine the phase state of the hydrometeors and from this fice. The measurement principle exploits the difference in scattering properties, i.e., depolarization, between nonspherical ice particles and spherical liquid droplets.
At least 2000 hydrometeors were classified for each LACIS data point presented in this study. The only exception to this is the measurement with the fresh CFA3 suspension where, due to low particle number concentrations, only ∼500 hydrometeors were considered (see Fig. 5). Occasionally, three or more data points of separate measurements under the same conditions were averaged. In these cases, the fice error is indicated by the standard deviation of the separate measurements. Otherwise, a Poisson error is given depending on the total number of classified hydrometeors in a single measurement. The temperature error of ±0.3 K is defined by the temperature stability of the thermostats. The ice nucleation active surface site density ns was calculated from Eq. (1) assuming the particle surface area Ap to be equal to the surface area of a sphere with a diameter of 300 nm.
2.3.2 SPIN
SPIN is a continuous flow diffusion chamber (CFDC) that has been described in detail by Garimella et al. (2016). In contrast to LACIS, the fraction of particles active as INPs (activated fraction, AF) is calculated by dividing the number of ice crystals detected with an optical particle counter (OPC) by the total number of aerosol particles measured with a CPC (model 3772, TSI Inc., St. Paul, MN, USA). A threshold size of 3 µm was used to identify ice crystals in the OPC signals. The uncertainty in AF is 14 % due to 10 % uncertainty in both CPC and OPC. The temperature uncertainties represent the highest and lowest deviations from the average lamina temperature in the chamber. When compared to LACIS measurements, SPIN data provide information on how immersion freezing results are affected by the different residence times in the two instruments. Ice nucleation times in SPIN depend on thermodynamic conditions and are between 8 and 12 s. In addition to the cyclone, an impactor (0.071 cm orifice, TSI Inc., St. Paul, MN, USA) with D50 = 500 nm was used upstream of the SPIN inlet to reduce the amount of multiply charged particles in the CFA aerosol. Hence, no multiple charge correction was applied to the SPIN data.
2.3.3 LINA
Based on the Bielefeld Ice Nucleation ARraY (BINARY; Budke and Koop, 2015), the newly developed LINA is a cold-stage setup for investigating immersion freezing. Ninety suspension droplets, each 1 µL in volume, were placed into separate compartments onto a hydrophobic glass slide. The compartments, formed by a perforated aluminum plate covered with a second glass slide, prevent interaction between the droplets, e.g., via the Wegener–Bergeron–Findeisen process or splintering while freezing. Also, the compartments suppress evaporation of the droplets. A cooling stage (LTS120, Linkam Scientific Instruments, Waterfield, UK) with a 40 mm×40 mm Peltier element is used for cooling the sample array at a rate of 1 K min−1. A thin layer of squalene oil on top of the Peltier element guarantees direct contact to the glass slide and improves heat transfer away from the droplets. The setup is situated in an aluminum housing that is purged with particle-free, dry air during the experiment. See Chen et al. (2018) for details on the temperature calibration routine.
The determination of fice is almost fully automated. A digital camera coupled with an LED dome light takes images every 6 s, which is equal to a temperature resolution of 0.1 K at a cooling rate of 1 K min−1. Parts of the LED light are shielded with a cardboard ring to cause ring-shaped structures to be reflected from the liquid droplets. As the reflective properties of a droplet change upon freezing, the reflection of the ring vanishes directly after the phase change. The images, each relating to a certain temperature, are later imported into a computer program that detects the number of rings. From this, fice(T) can be derived. See Appendix A for details on the correction of LINA data with respect to background INPs, calculation of ns, and error estimation.
2.3.4 WISDOM
The WISDOM setup (Reicher et al., 2018) was used to study the immersion freezing of the fine CFA fraction. WISDOM is a freezing array of monodisperse nanoliter droplets that are produced by a microfluidic device and subsequently arranged into an array of chambers based on Schmitz et al. (2009). Droplets are suspended in an oil mixture, consisting of mineral oil (Sigma Aldrich, St. Louis, MO, USA) stabilized with 2 wt % non-ionic surfactant (span80®, Sigma Aldrich, St. Louis, MO, USA). Pure water droplets within the device can be supercooled to below −35 ∘C, where first freezing occurs, i.e., above this temperature no correction regarding background INPs is necessary. The temperature accuracy of WISDOM is 0.34 ∘C. Freezing is observed by a microscope (BX51 with 10× objective and transmission mode, Olympus Optical, Tokyo, Japan) and detected for each droplet individually when the optical brightness of the droplet decreases due to the formation of ice crystals. The microfluidic devices are fabricated in the laboratory from polydimethylsiloxane and attached to a 1 mm microscope slide using oxygen plasma treatment. Freezing experiments are conducted in a commercial cryostage (THMS600, Linkam Scientific Instruments, Waterfield, UK) at a cooling rate of 1 K min−1.
ns was determined according to Eq. (2), with the droplet volume pL, the Brunauer–Emmett–Teller (BET; Brunauer et al., 1938) specific surface area of the fine CFA fraction ABET (see Sect. S8), and the concentration of CFA in suspension C. The ns error was estimated by propagating the uncertainties in the measurements of Vdrop and ABET, and the Poisson distribution of particles in suspension.
2.4 Sample characterization
In the following sections, we describe the instrumentation used for the analyses of chemical composition, morphology, and crystallography of the CFA samples. The most important findings, which will be referred to in the discussion of the immersion freezing results (see Sect. 3), are summarized. For more details concerning sample characterization, see the Supplement.
2.4.1 Size-selected CFA
ALABAMA
ALABAMA, which was originally developed for aircraft operation (Brands et al., 2011) but is also used in ground-based campaigns (Roth et al., 2016; Schmidt et al., 2017), is a single particle laser ablation instrument using a Z-shaped time-of-flight mass spectrometer. After entering the instrument, aerosol particles are focused to a narrow particle beam by an aerodynamic lens. In the following, the particles pass two subsequent detection lasers (wavelength of 405 nm). Information about the time-of-flight between the detection lasers is needed to trigger the ablation laser. In addition, the time-of-flight can be used to calculate the particle vacuum aerodynamic diameter for particles in a size range between ∼200 and 2500 nm. The ablation laser, a Nd:YAG operating at a wavelength of 266 nm, evaporates the particles and ionizes the molecule fragments. The resulting ions are analyzed in the bipolar mass spectrometer such that one anion and one cation spectrum is obtained for each single particle, yielding information about their chemical composition. Single particle mass spectra were averaged, resulting in a mean chemical composition of each CFA sample for both dry and wet particle generation.
The overall composition of the CFA samples is comparable to mineral dust, as elements like Al, Ca, K, Fe, Si, S, P, Na, and Mg frequently occur. Some trace elements seem to be characteristic for the sampled CFA particles. Especially Ti-, Sr-, and Ba-related mass-to-charge ratios occur in more than 50 % of all dry-sampled particles (see Figs. S1 and S2) and could potentially be used as indicators for CFA particles in the atmosphere, together with the overall fingerprint of their mass spectra. There are some features that could explain differences in immersion freezing behavior between the different samples (see Fig. S4). For example, CFA1 has the highest concentration of Ca and S in 300 nm particles and CFA3 has the lowest concentration of Ca and S in 300 nm particles, whereas CFA3 has the highest concentration of Si in 300 nm particles and CFA1 has the lowest concentration of Si in 300 nm particles. Furthermore, CFA1 is the sample with the highest concentration of Pb in 300 nm particles. The comparison of averaged mass spectra of dry- and wet-generated CFA particles indicates the hydration of oxides, e.g., CaO, SrO, and BaO, in suspension (see Fig. S3). S-containing substances are present to a lower extent in wet-generated CFA compared to dry-generated particles. A more detailed description of the ALABAMA results is given in Sect. S1.
Multi-MINI – ESEM/EDX
Particles were collected on boron substrates behind the DMA with the multi-MINI (Ebert et al., 2016), using one stage with D50=1 µm. Sampling durations ranged from 30 s to 6 min, depending on average particle number concentrations of the different samples (80 to 300 cm−3). Chemical composition, size, and morphology were investigated with a Quanta 400 FEG ESEM (FEI Company, Hillsboro, OR, USA). No coating was applied to the substrates prior to the ESEM/EDX investigations. Particles impacted on the substrate located in the impaction spot were randomly selected for analysis. Chemical elements with an atomic number larger than 5 were detected with an EDX detector and analyzed with the AZtec software (version 3.3 SP1, Oxford Instruments, Abingdon, UK). All measurements were carried out with 12.5 keV, 10 mm working distance, and 20 s acquisition time per particle.
The ESEM images (see Figs. S5 and S6) show that CFA1 is special in terms of particle morphology. Dry-generated CFA1 particles consist of irregularly shaped agglomerates of small spherules, which were not observed to this extent for the other samples. Wet-generated CFA1 particles appear to be an external mixture of spherules and needle-shaped particles. CFA1 is the only sample for which needle-shaped particles were observed in connection with wet particle generation, and also the only sample for which a clear difference in morphology was observed between the dry and wet particle generation methods. The major elements detected by EDX agree with the ones identified in the ALABAMA mass spectra (see Table S1). However, trace elements, e.g., Ti, Sr, and Ba, could not be found, presumably for statistical reasons. A more detailed description of the ESEM/EDX results is given in Sect. S2.
2.4.2 Bulk CFA
XRD
For the crystallographic characterization of the CFA samples, X-ray diffraction (XRD) analyses were performed with both dry particles and suspension particles. Dry particles were ground using a mortar and pestle before being pressed into a sample holder as densely as possible. CFA suspensions were prepared as for the LACIS measurements (see Sect. 2.2.2) and then left in a desiccator (steady flow of particle-free, dry air) until all water was evaporated. The remaining dry powder was pressed into a sample holder. Both procedures were applied to all four samples, resulting in eight measurements. A Bragg–Brentano diffractometer with a Cu anode (Philips X'Pert, PANalytical, Almelo, the Netherlands) was used to perform 2Theta-Omega scans from 10 to 70∘ with a step size of 0.03∘ and an integration time of 20 s. Quantitative phase identification was done by Rietveld refinement using reference patterns from the Crystallography Open Database (Gražulis et al., 2009).
The XRD patterns indicate quartz (SiO2) as the major crystalline phase in all CFA samples (see Figs. S7 to S10). Furthermore, anhydrite and quicklime occur in all samples, but to the largest extent in CFA1. CFA1 is also the only sample where a definite change can be seen between the original dry sample and the sample that was produced by evaporating all water from the suspension. Here, the conversion of anhydrite (CaSO4) to gypsum (CaSO4•2H2O, see R1) and the conversion of quicklime (CaO) to calcite (CaCO3, see R2 and R3) can be observed. CFA3 is the sample with the highest amorphous, i.e., noncrystalline, fraction in bulk, and likely also in 300 nm particles, as an increase in the amorphous fraction in CFA towards smaller particle sizes has been reported in a previous study (Matsunaga et al., 2002). A more detailed description of the XRD results is given in Sect. S3.
Chemical composition
The bulk chemical composition analysis was performed using inductively coupled plasma-sector field mass spectrometry (ICP-SFMS) at ALS Scandinavia AB (Luleå, Sweden). Measured mass fractions of major ions were recalculated into their most common oxide forms (see Fig. S11). Because of its high CaO content of 26 wt %, CFA1 is classified as class C CFA according to the American Society for Testing Materials (ASTM, 2015, standard C618). Class C CFA is cementitious, i.e., self-hardening in contact with water. The occurrence of needle-shaped particles in wet-generated CFA1 could cause this cementitious property. CFA2, CFA3, and CFA4 are class F CFA, meaning that additives are needed to induce hardening of a CFA–water mixture. Loss on ignition (LOI) values were determined by heating a defined amount of the CFA samples to 1000 ∘C and comparing pre- and post-ignition weights. LOI values are proportional to the amount of unburnt fuel resulting from incomplete combustion in the power plants. No weight change within measurement uncertainty was registered for CFA1, CFA2, or CFA3. CFA4 still contains a relevant amount of unburnt fuel (LOI = 8.1±5 %). A more detailed description of the bulk chemical composition results is given in Sect. S4.
In addition to the ICP-SFMS and LOI measurements, water activity and pH values of CFA suspensions were determined. The water activity of the CFA samples was ∼1, i.e., no difference to pure water could be detected. The CFA2, CFA3, and CFA4 suspensions were neutral to slightly alkaline (pH ∼7–8). The CFA1 suspension was strongly alkaline (pH ∼ 11), likely due to the high CaO content and the formation of portlandite (Ca(OH)2, see R2) which dissociates into Ca2+ and OH− ions.
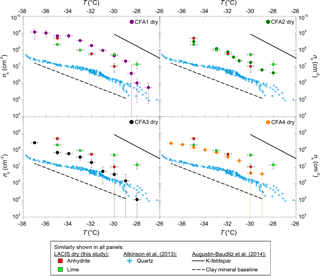
Figure 2ns from LACIS measurements with dry-generated CFA particles. Measurements with dry-generated anhydrite and quicklime are included for comparison in all panels but are (in contrast to CFA) not corrected with respect to multiple charges. Measurements with quartz shown in all panels are taken from Atkinson et al. (2013). Fit lines to LACIS measurements with a K-feldspar sample and different kinds of mineral dust coated with sulfuric acid (clay mineral baseline) are taken from Augustin-Bauditz et al. (2014).
In the following, refer to Fig. 2 for comparing LACIS measurements of individual CFA samples with measurements of different substances contained in CFA. Figure 3 shows SPIN results of measurements with our German CFA samples and four CFA samples from the USA (Garimella, 2016). Figure 4 shows the comparison of CFA results from LACIS, LINA, and WISDOM and the intercomparison between samples.
3.1 Dry particle generation
3.1.1 CFA
LACIS measurements with dry-generated CFA particles are reported between −26 ∘C, where the first signal above the limit of detection could be observed, and −37 ∘C, where homogeneous ice nucleation starts to contribute. Data showing measurements with dry-generated particles from CFA1 have previously been published in Grawe et al. (2016). Comparing the ns spectra of all four CFA samples (see full circles in Fig. 4) shows variation within a factor of 37 (difference between CFA2 and CFA3 at −28 ∘C). CFA1 has the highest ns, followed by CFA2, CFA4, and CFA3. This order is valid throughout the whole examined temperature range, except for T>−29 ∘C, where ns decreases rapidly in case of CFA1. The curve shape for T<−29 ∘C with the relatively shallow increase is comparable for all samples. The broad temperature range, in which the increase in ns is observed, hints at a variety of different types of ice nucleation active sites at the surface of the CFA particles. In case of uniform ice nucleation properties, a steep increase would be observed.
To put the efficiency of the CFA particles into perspective, Fig. 2 includes fits to LACIS measurements with a K-feldspar sample (76 % microcline, 24 % albite) and different kinds of mineral dust that featured a similar immersion freezing behavior after coating with sulfuric acid (clay mineral baseline) by Augustin-Bauditz et al. (2014). Dry-generated CFA particles are not as efficient as the K-feldspar sample, which is also the most efficient mineral dust sample investigated with LACIS so far, but CFA1 is only 1 order of magnitude below. All of our dry-generated CFA samples are at least 1 order of magnitude above the clay mineral baseline for T<−29 ∘C. In conclusion, the dry-generated CFA particles are comparable to mineral dust in their immersion freezing behavior.
Figure 3 shows a comparison of SPIN measurements with 300 nm CFA particles between this study and Garimella (2016), who performed immersion freezing measurements with four CFA samples from the USA, two class C and two class F samples. Note that horizontal error bars indicating the temperature uncertainty are only included for CFA2 and CFA4. This was done for greater clarity in the case of CFA1 and CFA3, for which more data are available and temperature uncertainties are comparable to the values shown in the CFA2 and CFA4 panels. Only towards the warmer end of the examined temperature range, ns of the samples from the USA is comparable to what we found for the German ones. At −36.5 ∘C, the lowest temperature at which both instruments have been operated, ns of the samples from the USA is up to 2 orders of magnitude lower than ns of the German samples. In general, the ns spectra of the samples from the USA have a much shallower slope than the German CFA ns spectra. As the same type of instrument was used for both investigations, we conclude that differences between the German samples and the samples from the USA originate from differences in physicochemical particle properties, and not from differences in methodology. Both SPIN experiments, ours and that of Garimella (2016), have in common that no large inter-sample variability was observed. This is in contrast to LACIS, where the class C CFA (CFA1) clearly has the highest efficiency. SPIN results have earlier been shown to differ from results obtained with instruments specially developed to measure immersion freezing (DeMott et al., 2015; Burkert-Kohn et al., 2017). See Sect. 4 for details on the intercomparison between SPIN and LACIS in the framework of the present study.
3.1.2 Comparison of CFA with anhydrite, quicklime, and quartz
From the comparison of ns to chemical information from ALABAMA measurements, it was concluded that components containing Ca and S could contribute to the observed differences in immersion freezing behavior between the CFA samples (see Fig. S4a). The occurrence of the Ca cation cluster series ((CaO)n, (CaO)nH, and partially Ca(CaO)n) together with the S anion cluster series (SOn, see Fig. S1) could be an indication for the presence of anhydrite, as suggested by Gallavardin et al. (2008). Therefore, anhydrite and also quicklime were chosen as test substances for additional LACIS measurements. To our knowledge, these are the first immersion freezing measurements using dry-generated anhydrite and quicklime particles. Both substances are known to occur in CFA and are enriched in submicron CFA particles (Enders, 1996; Querol et al., 1996). Anhydrite is of special interest because Havlíček et al. (1993) found that water-soluble material on the CFA particle surface is mainly anhydrite and suggested that it is responsible for initiating heterogeneous freezing on the particles.
Both anhydrite and quicklime are efficient INPs in the immersion mode when being dry generated (see Fig. 2). Note that multiple charge correction was not possible for LACIS measurements with anhydrite and quicklime (in contrast to CFA). The correction would shift the ns spectra of anhydrite and quicklime towards lower ns values but the slope would stay the same. Generally, multiple charge correction lowers ns values for the dry-generated CFA particles by less than a factor of 3.5 and we expect that it would be comparable for the anhydrite and quicklime particles. Anhydrite is more efficient than quicklime at ∘C (a factor of 2) and less efficient at ∘C (a factor of 5), i.e., there is a slightly steeper slope of the anhydrite ns spectrum. The shape and magnitude of the anhydrite ns spectrum are comparable within measurement uncertainty to what was found for CFA2 and CFA4. CFA3, which contains the lowest concentration of Ca and S, and presumably the lowest concentration of anhydrite in 300 nm particles, is less efficient than pure anhydrite. CFA1 is more efficient than pure anhydrite, indicating that other compounds might influence the immersion freezing efficiency of this sample. A possible component contributing to ns of CFA1 might be Pb, which occurs in 20 % of 300 nm particles from CFA1 (in ≤10 % of particles from CFA2, CFA3, and CFA4, see Fig. S2) and has been discussed previously as a potential INP, or as amplifying the ice nucleation efficiency of other compounds (Cziczo et al., 2009; Kamphus et al., 2010).
Quartz – which is the main crystalline phase of all our CFA samples according to XRD measurements and likely also occurs in 300 nm CFA particles (Si was identified by both ALABAMA and ESEM/EDX) – is at least 1 order of magnitude less efficient than CFA1, CFA2, and CFA4. We compared the CFA results to cold-stage measurements of quartz by Atkinson et al. (2013) here because this dataset spans the relevant temperature range and because there is a lack of immersion freezing results of dry-generated quartz in the literature. The ns spectrum of CFA3, which contains the highest concentration of Si (presumably quartz) in 300 nm particles is higher by a factor of 2 to 10 compared to the quartz ns spectrum. This indicates that quartz might contribute to some of the observed immersion freezing behavior, especially in the case of CFA3, but it is not the most active component in CFA1, CFA2, and CFA4; ALABAMA results showing that 300 nm particles in CFA1 contain the lowest concentration of Si compounds, followed by CFA2 and CFA4, support this hypothesis (see Fig. S4b).
The hypothesis that the amorphous material in CFA has a promoting effect on its immersion freezing efficiency (Umo et al., 2015) cannot be confirmed for our samples. XRD investigations show that CFA3, which was the least efficient of the four dry-dispersed samples, contains the highest amorphous fraction.
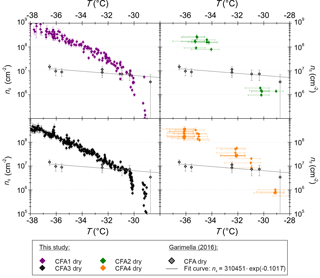
Figure 3ns from SPIN measurements with dry-generated 300 nm CFA particles. Horizontal error bars are omitted in the CFA1 and CFA3 panels for greater clarity but are comparable to the values shown in the CFA2 and CFA4 panels. Measurements with CFA samples from the USA (Garimella, 2016) are included for comparison.
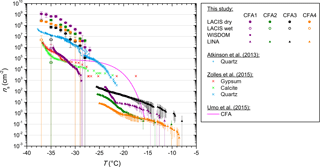
Figure 4ns from LACIS measurements with dry- and wet-generated 300 nm particles. ns from WISDOM measurements with the fine CFA fraction and ns from LINA measurements with bulk material are included for comparison. Measurements with gypsum, calcite, and quartz are taken from Zolles et al. (2015) and Atkinson et al. (2013), measurements with a CFA sample of different origin from Umo et al. (2015).
3.2 Suspension methods
3.2.1 CFA
Figure 4 summarizes ns derived from LACIS measurements with dry- and wet-generated CFA particles (full and open circles), ns from WISDOM measurements with the fine CFA fraction (squares), and ns from LINA measurements with the bulk CFA (triangles). Firstly, LACIS results will be described and then compared to those of the other two instruments. Secondly, we will compare these to measurements with the hydration products of anhydrite and quicklime by Zolles et al. (2015), and finally to measurements with a CFA sample of different origin by Umo et al. (2015). A comparison to measurements by Havlíček et al. (1993) is unfeasible because no specific surface area values of the samples are given in this publication.
LACIS
When comparing ns from LACIS measurements with dry-generated particles (full circles in Fig. 4) to measurements with wet-generated particles (open circles), a significant decrease can be seen. The ns values at −35 ∘C were lowered by between 1 (CFA3) and 4 (CFA2) orders of magnitude. The ns values of wet-generated particles vary by up to 2 orders of magnitude between the four CFA samples. This can possibly be attributed to low values of fice that are only slightly above values usually measured for homogeneous nucleation (see Fig. B1), i.e., close to the limit of detection. As a result, the error in fice and ns is larger than for the dry-generated particles at the same temperature.
Note that data for wet-generated CFA1 particles differ from those published in Grawe et al. (2016), which needed to be corrected due to the identification of a sample-specific artifact (see Appendix B). ESEM images from both Grawe et al. (2016) and the present study show two different particle types sampled after wet particle generation and size selection of CFA1, i.e., spheroidal particles and needle-shaped particles (see Figs. S5 and S6).
The occurrence of needle-shaped particles suggests that compounds are dissolved from the particles in suspension. During LACIS measurements, purely water-soluble particles, i.e., particles which do not contain water-insoluble material, would activate to droplets that then would only freeze homogeneously, causing an underestimation of fice. To make sure that no purely water-soluble particles with a size of 300 nm were produced when spraying the suspensions, size distributions of particles from all CFA suspensions were measured before LACIS experiments took place. From the size distribution measurements (see Sect. S5), we conclude that a negligibly small number of purely water-soluble particles with a size of 300 nm was produced from CFA2, CFA3, and CFA4, i.e., the decrease we observe in the transition from dry to wet particle generation is not caused by a measurement artifact. The evaluation of the CFA1 size distribution is not unambiguous because of the superimposition of size distributions of spheroidal and needle-shaped particles.
A decrease in immersion freezing efficiency from dry to wet particle generation was already reported for CFA and coal bottom ash in Grawe et al. (2016). A possible explanation for the observed discrepancy was presented following previous investigations of Hiranuma et al. (2015), who conducted immersion freezing measurements with both dry-dispersed mineral dust and mineral dust suspensions. There it was hypothesized that the increased time that the particles spend in contact with water leads to a change in chemical particle properties. For our previous study (Grawe et al., 2016), it was not possible to identify relevant processes because information on the chemical composition of 300 nm particles was missing. In the framework of the present study, differences in chemical composition of dry- and wet-generated CFA particles were identified (see Sects. 2.4 and S1), and will be discussed in relation with the immersion freezing results in Sect. 3.2.5.
Comparison of LACIS, LINA, and WISDOM results
LINA measurements (triangles in Fig. 4) were performed between 0 and −26 ∘C. In the temperature range from −8 to −23 ∘C, CFA3 has the highest, and CFA4 the lowest ns values of all samples, with those being 2 orders of magnitude apart. According to LOI measurements (see Sect. S4), CFA4 contains the highest amount of unburnt fuel, which is presumably made up of carbonaceous particles. The low immersion freezing efficiency of CFA4 in the investigated temperature range could hence be related to the occurrence of carbonaceous particles, which have previously been found to be inefficient at nucleating ice in the immersion mode (e.g., Chen et al., 2018). CFA1 shows a steep increase in ns between −21 and −24 ∘C, below which all droplets were frozen. In contrast, the last droplets of CFA2, CFA3, and CFA4 suspensions froze below −25 ∘C. Note that coagulation of particles in the suspensions, which was observed to some extent for CFA suspensions with higher concentrations (see Fig. S13), might have a reducing effect on the surface area available for ice nucleation in the cold-stage measurements (Emersic et al., 2015).
WISDOM measurements (squares in Fig. 4) were performed as an attempt to close the temperature gap between LACIS measurements with wet-generated particles ( ∘C) and LINA measurements ( ∘C). This could not be realized for two reasons. Firstly, WISDOM measurements with suspensions of 0.1 g CFA in 100 mL distilled water were only possible with CFA1, because the other samples showed no immersion freezing activity. Increasing the concentration to a level, for which signals above the homogeneous freezing limit could be expected, led to strong settling of particles in the CFA2, CFA3, and CFA4 suspensions. Secondly, freezing was only observed for ∘C for CFA1, i.e., there is no temperature overlap between LINA and WISDOM. However, extrapolation suggests that both instruments could yield similar results.
Good agreement can be observed for WISDOM and LACIS at ∘C with CFA1. At this temperature, the contribution of homogeneous nucleation is still minor in WISDOM measurements and hence we conclude that the major contribution to the observed freezing behavior is due to immersion freezing triggered by CFA particles. The good agreement between WISDOM and LACIS firstly implies that there is no pronounced effect of size-dependent composition on the immersion freezing behavior of CFA1. This finding could be specific to CFA1, as it is in contrast to Garimella (2016), who found that ns increases with decreasing particle size. Secondly, the successful instrument intercomparison indicates that drying of the CFA1 suspension droplets after atomization (which does not take place in WISDOM experiments) does not have a strong effect on the immersion freezing efficiency of the CFA1 particles.
Comparison to Umo et al. (2015)
Cold-stage measurements with a CFA sample of different origin by Umo et al. (2015, see Fig. 4) yielded results that differ substantially from what we measured in the framework of the present study. The efficiency of the sample investigated by Umo et al. (2015) increases strongly for −20 ∘C < T < −15 ∘C and levels off from T<−25 ∘C. This is in contrast to the gradual increase over a broad temperature range that we observed for our samples. Our suspensions were prepared in the same way as described by Umo et al. (2015), and LINA and the microliter Nucleation by Immersed Particles Instrument (µL-NIPI; Whale et al., 2015) used by Umo et al. (2015) have successfully been intercompared with a different ash sample (not shown). Therefore, we infer that the CFA samples are really different in their immersion freezing behavior and we do not observe artifacts related to methodology. The comparison to Umo et al. (2015), and the results by Garimella (2016) shown in Figs. 3 and 4, suggest that CFA samples from different geographical origin show a highly variable immersion freezing behavior.
3.2.2 Comparison of CFA with gypsum and calcite
A comparison of ALABAMA measurements of dry- and wet-generated CFA particles hints at hydration of several oxides (see Fig. S3). It is difficult to say which hydration reactions in the complex mixture cause the decrease in immersion freezing behavior in measurements with the suspension methods. However, for bulk CFA1 there is clear evidence from XRD measurements that anhydrite and quicklime, which were already identified as species potentially influencing immersion freezing of the dry-generated particles, are hydrated in suspension, resulting in the formation of gypsum and calcite (see Fig. S7). In the following, we compare immersion freezing results of CFA suspension particles to measurements presented in Zolles et al. (2015) of gypsum and calcite (see Fig. 4).
Both hydration products, i.e., gypsum and calcite, are lower in their immersion freezing efficiency by 3 orders of magnitude compared to anhydrite and quicklime, i.e., as for CFA there is a significant decrease in efficiency of the hydration products compared to their anhydrous precursors. In general, gypsum and calcite are similar in their immersion freezing efficiency. LACIS measurements with wet-generated CFA and WISDOM measurements agree with the gypsum and calcite results within 1 order of magnitude. The only exception to this is CFA3 which will be discussed below in relation to quartz.
The hydration of anhydrite inevitably takes place once CFA comes into contact with water, because anhydrite is present at the particle surface (Enders, 1996). Sievert et al. (2005) described the hydration of pure anhydrite particles in the following way. Firstly, anhydrite is dissolved from the particles and Ca2+ and ions are hydrated in the solution. The hydrated ions are then adsorbed to the surface of the anhydrite particles due to electrostatic attraction. From this point on, further dissolution and interaction of water molecules with the anhydrite surface is reduced because of the adsorbed layer of hydrated ions. Secondly, as the thickness of the adsorbed layer increases, cracks are formed through which water molecules migrate to the anhydrite surface. Only then, nuclei of gypsum are formed and crystallization takes place. The first process (formation of the adsorbed layer of hydrated ions) is thought to happen rather quickly; the second process (formation of gypsum) can take several hours up to days. See Sects. 3.3 and 4 for details on the duration of hydration.
The formation of calcite occurs via the hydration of quicklime to portlandite (Ca(OH)2) which is then carbonated (see R2 and R3). It is possible that this process causes the precipitation of needle-shaped particles in suspension, but only if the quicklime content is sufficiently high (as for CFA1). It cannot be ruled out that calcite is also formed in the other CFA suspensions; however, in contrast to CFA1, calcite could not be clearly identified in the other samples by XRD.
Possibly, both above-described mechanisms, and potentially even more hydration reactions, cause the observed decrease in immersion freezing efficiency in the transition from dry to wet particle generation. Additional LACIS measurements with different sample treatments were performed to verify this hypothesis (see Sect. 3.3).
3.2.3 Comparison of CFA with quartz
In addition to quartz measurements by Atkinson et al. (2013), we now include quartz measurements by Zolles et al. (2015) in our discussion because they cover T>−28 ∘C, which is the more relevant temperature range for our cold-stage measurements with CFA. We compare these to the most efficient of the quartz samples investigated by Zolles et al. (2015). The ns spectra of the quartz samples used by Zolles et al. (2015) and Atkinson et al. (2013) agree in the narrow temperature overlap (−26 ∘C < T<−28 ∘C). It is obvious that quartz is significantly more efficient in the immersion mode than suspended particles of CFA1, CFA2, and CFA4, with ns being at least 1 order of magnitude higher over the complete investigated temperature range. The deviation is smallest for CFA3 which contains the highest concentration of Si species (quartz) and lowest concentration of Ca and S species in 300 nm particles. For this sample, we assume the smallest effect of the hydration reactions and a larger influence of quartz on the immersion freezing behavior compared to the other samples. The fact that the other samples also contain significant amounts of quartz – both in 300 nm particles and in bulk, and, nevertheless, feature a much lower efficiency – supports the hypothesis of the particles being covered by a layer which suppresses the ice nucleation efficiency of the quartz. In the case of the dry particle generation method, the layer is more efficient at initiating immersion freezing than quartz. In the case of the suspension methods, the layer is less efficient than quartz, with this change brought on by the above-described hydration reactions.
3.3 Effect of sample treatment on the immersion freezing efficiency of CFA
Additional LACIS measurements with differently treated CFA and anhydrite samples, as well as pure gypsum, were performed in order to test the hypothesis that the hydration of anhydrite leads to a decrease in immersion freezing efficiency in suspension (see Fig. 5). All measurements were performed at −35 ∘C with 300 nm particles. Here, we forewent the multiple charge correction for better comparability to measurements that took place after the campaign, where no correction was possible. The corrected values for CFA (used for the ns calculations in Fig. 4) are shown as circles.
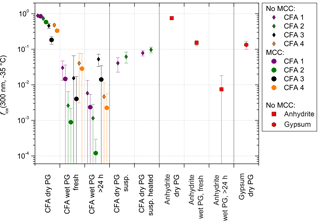
Figure 5fice (−35 ∘C) from LACIS measurements with 300 nm particles. Multiple charge correction (MCC) was not performed, except for the measurements indicated by the circles in the first three columns. “Dry PG susp.” means dry particle generation (PG) with a sample resulting from the evaporation of a suspension and “dry PG susp. heated” means additional heating of this sample to 250 ∘C prior to particle generation.
When comparing dry-generated CFA particles with wet-generated particles, either from a fresh suspension (i.e., measured within 5 min after preparation) or from the standard suspension (10 min of ultrasonification and 24 h stirring), a decrease in fice (−35 ∘C) can be observed. However, the particles from the freshly prepared suspension seem to be slightly more efficient than the ones from the standard suspension. The only exception is CFA3, where it was extremely difficult to generate a sufficiently high particle number concentration from the fresh suspension, resulting in a large error due to the small amount of classified hydrometeors (∼500). Dry- and wet-generated anhydrite particles show the same trend as observed for CFA, i.e., the wet-generated particles are significantly less efficient than the dry-generated particles, and the longer the particles stay in suspension, the stronger the decrease in fice.
Sullivan et al. (2010) described an increase in hygroscopicity of wet-generated anhydrite particles in comparison to dry particle generation. Also, the hygroscopicity of the wet-generated particles increased with the time that the particles spent in the suspension. Sullivan et al. (2010) attributed this behavior to the formation of hydrates and hypothesized that this process could have an effect not only on hygroscopicity but also on the ice nucleation efficiency of the particles. In our case, the dependency of immersion freezing efficiency on suspension time could result from the two stages of anhydrite hydration described in Sect. 3.2.5. Firstly, on the timescale of minutes, anhydrite is dissolved and hydrated ions form a layer on top of the CFA particles causing a sudden decrease in immersion freezing efficiency. It seems that the limited suspension time of 1.6 s in the case of dry particle generation is not sufficient to cause hydration. Secondly, on the timescale of several hours up to days, anhydrite is converted into gypsum which decreases fice further. Gypsum, like anhydrite, consists of molecules which are strong electrical dipoles (Klimchouk, 1996) and hence will also be surrounded by hydrated ions in suspension.
The ultrasonicated and stirred suspensions of CFA1 and CFA2 were left in a desiccator (steady flow of particle-free, dry air) until all water was evaporated. XRD measurements of the resulting powder show that the anhydrite–gypsum conversion had taken place and we assume that gypsum was already present in the stirred suspensions. The powder was then dry-dispersed and fice (−35 ∘C) of 300 nm particles was measured. An increase of almost 1 order of magnitude for CFA1 and almost 2 orders of magnitude for CFA2 in comparison to the wet-generated particles was registered. We attribute this increase to the difference in particle generation. For wet particle generation, possibly only the bulk water is removed in the diffusion dryer downstream of the atomizer, whereas the water molecules in the layer of hydrated ions remain. Drying in a desiccator, which takes several days, could lead to partial dehydration, i.e., removal of the hydrated layer surrounding the CFA particles. For dry particle generation, the limited suspension time of 1.6 s in LACIS is apparently not long enough for rehydration.
Additionally, the powder from the evaporated suspensions of CFA1 and CFA2 was heated to 250 ∘C for 15 min. According to Deer et al. (1992), this temperature is sufficient to dehydrate gypsum and form anhydrite. fice (−35 ∘C) of 300 nm particles slightly increased by a factor of 2 after the heat treatment, but it did not restore the immersion freezing efficiency of the original dry-dispersed particles. It is known that other hydrated species are present in the suspension particles that are only dehydrating at much higher temperatures (e.g., dehydration temperature of portlandite: 510 ∘C; Bai et al., 1994) and hence it is not surprising that only a small increase in fice could be achieved. In general, there is good agreement between measurements with dry-generated gypsum particles (see Fig. 5) and particles from the dried and heated CFA suspensions, indicating that gypsum is present in the investigated 300 nm CFA particles after they have spent a sufficiently long time in water.
It is beyond the scope of this paper to examine why hydration leads to a lower immersion freezing efficiency. Hence, we only offer some possible explanations here without further discussing their likelihood. A simple explanation would be that the adsorbed layer of hydrated ions on the particle surface blocks the interaction with the surrounding water molecules. Consequently, freezing would not be triggered as efficiently as for the dry-generated particles, where the contact with water is too short to dissolve a sufficient amount of anhydrite. Another hypothesis (Sihvonen et al., 2014) describes a change in lattice parameters upon forced hydration of mineral dust particles towards a greater mismatch with ice.
In view of the atmospheric relevance of the above-described findings, it is important to discuss whether the observed decrease in immersion freezing efficiency of CFA associated with switching from dry to wet particle generation would also occur in the atmosphere. From LACIS measurements with the freshly prepared CFA suspensions, we know that particles in the bulk suspension are deactivated within ∼5 min but it is not clear if this would also be observed when a single particle is immersed in a cloud droplet. As already mentioned, it seems that 1.6 s, which is the residence time of CFA particles in water for LACIS measurements with dry-generated CFA, is not enough to cause hydration of anhydrite and quicklime.
Unfortunately, an increase in nucleation time by more than a factor of 2 is not possible with LACIS. However, during the campaign, SPIN measurements with dry-generated CFA particles were performed above water saturation (see Appendix C). From the results, which agree with LACIS for CFA3 and CFA4 but are below LACIS for CFA1 and CFA2, it can be speculated that the longer residence time in SPIN (∼10 s) already leads to some deactivation by the formation of a hydrated layer on top of those particles which contain the highest concentration of water-soluble anhydrite. However, the effect is much more pronounced for longer hydration times, as can be seen in the results of SPIN measurements with wet-generated particles. It would be necessary to increase nucleation time further to evaluate the time needed to decrease the immersion freezing efficiency of single particles immersed in droplets to the efficiency of particles hydrated in the bulk suspension. Within the framework of the present study, it was not possible to keep cloud droplets with a single immersed CFA particle stable for longer than a few seconds before investigating immersion freezing. Hence, we can only give a range of how efficiently CFA induces immersion freezing in the atmosphere, because, for CFA containing a certain amount of anhydrite, this will depend on the time between activation to cloud droplets and triggering of freezing.
Figure 6 shows INP concentrations estimated from our CFA measurements in combination with size distributions measured ∼80 km downstream of a coal-fired power plant (Parungo et al., 1978). For this, the ambient size distribution was subtracted from the size distribution in the plume to only consider particles emitted from the power plant. The procedure is explained in detail in Grawe et al. (2016), where we already estimated the INP concentration due to the emission of CFA1 to be higher than typical atmospheric INP concentrations, assuming ns of dry-generated particles. This is equally true for the other dry-generated CFA samples, with estimated INP concentrations being roughly 2 orders of magnitude above the upper boundary given by Petters and Wright (2015) at −37 ∘C. In light of our new findings, and assuming that atmospheric processing will lead to a decrease in immersion freezing efficiency, we also estimated the INP concentrations using ns from LACIS measurements with wet-generated CFA and cold-stage measurements. Above −30 ∘C, INP concentrations derived from measurements with CFA suspensions are close to or below the lower boundary given by Petters and Wright (2015), except for CFA3, which is within the boundaries given by Petters and Wright (2015) for T>−23 ∘C. This indicates that the majority of our CFA samples only contribute very little to atmospheric INP concentrations above −30 ∘C, when we assume that the suspension results are representative for processes occurring in the atmosphere. Our estimate suggests that particles from CFA1, CFA2, and CFA4 only become relevant for atmospheric immersion freezing at temperatures below −30 ∘C. Note that in close proximity to the source, i.e., in an undiluted plume directly after emission, INP concentrations will be much higher than estimated above. At greater distances from the power plant, INP concentrations will be significantly lower due to dilution. Garimella (2016) estimated that CFA particles are present at cirrus level in concentrations of ∼0.1 to 1 L−1.
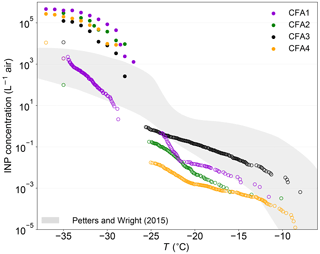
Figure 6Estimated INP concentrations ∼80 km downstream of a coal-fired power plant based on size distributions measured in a plume by Parungo et al. (1978). Full circles represent dry particle generation, open circles wet particle generation and cold-stage measurements. The shaded area indicates typical atmospheric INP concentrations derived from precipitation samples (Petters and Wright, 2015).
In the framework of this study, four CFA samples from German power plants were investigated concerning their immersion freezing behavior, chemical composition, morphology, and crystallography. In light of our new findings, we now revisit the questions from the introduction.
-
Do CFA samples from different power plants feature a similar immersion freezing behavior? and
-
Is the deactivation in the transition from dry to wet particle generation observable for different CFA samples?
All four samples were found to be efficient INPs in the immersion mode below −28 ∘C when dry particle generation was used. The ns spectra of dry-generated particles differed by approximately 1 order of magnitude, with the curve shapes being very similar. A decrease in immersion freezing efficiency was observed for all of our samples when particles were generated from a suspension. However, the dataset is still too small to make a conclusive statement about the variability in immersion freezing results caused by different samples and differences in methodology. Comparisons to samples of different geographical origin (Umo et al., 2015; Garimella, 2016) suggest that the spread is indeed larger than what we found for the German CFA samples. Further immersion freezing measurements with more CFA samples from different sources, which should also focus on the effect of hydration, would be needed to provide a suitable parameterization.
-
Is it possible to find a connection between physicochemical sample properties and the observed immersion freezing behavior?
From ALABAMA measurements, it was derived that the amount of molecular species containing Ca and S correlates with the immersion freezing efficiency of the dry-generated samples. Additional LACIS measurements with anhydrite and quicklime yielded similar results as for CFA, suggesting that both substances contribute to the observed freezing behavior. Both anhydrite and quicklime are hydrated (quicklime also carbonated) in contact with water which might cause a decrease in immersion freezing efficiency. Cold-stage measurements with the hydration products gypsum and calcite (Zolles et al., 2015) are comparable to LACIS measurements with wet-generated CFA particles and to WISDOM measurements. An exception is CFA3, which has the lowest concentration of Ca and the highest concentration of Si in both 300 nm particles and bulk. Here, the decrease in immersion freezing efficiency in the transition from dry to wet particle generation is smallest, and LACIS measurements are relatively close to cold-stage measurements with quartz (Atkinson et al., 2013). Quartz was detected as the major crystalline phase in all of the bulk samples. From this, we conclude that an influence of quartz on the immersion freezing behavior of CFA can only be seen in the case when the amount of anhydrite and quicklime is below a certain, not clearly definable, threshold.
-
Which particle generation technique (dry or wet particle generation) or measurement method (single particle or cold stage) is appropriate for representing atmospheric processes after CFA emission?
It is important to know that for CFA, it is necessary to consider dissolution effects in suspension and changes in immersion freezing behavior on short timescales. We observed that dry-generated particles, which were immersed in droplets in LACIS for 1.6 s before freezing, are efficient INPs and can potentially contribute to the atmospheric INP spectrum if concentrations are high. However, for two of the samples, a decrease in freezing efficiency could already be seen when particles were immersed for ∼10 s in SPIN, suggesting that the ability of CFA to act as INP can decrease quickly in contact with water. Estimating atmospheric INP concentrations due to CFA emission, and assuming atmospheric processing of the particles, indicates that CFA is relevant at T<−30 ∘C, i.e., an effect on cirrus formation could be possible. Concerning this, it could also be worthwhile to further investigate deposition nucleation on CFA particles.
An approach to improve process understanding of CFA ageing in the atmosphere is to either sample particles in a coal-fired power plant plume on filters or perform in situ INP measurements, preferably at several distances downstream of the stack. In the case that it should turn out that the lower limit given by LACIS measurements with wet-generated particles and cold-stage measurements is reproducible, one might be able to provide sample-specific parameterizations and, once more samples have been investigated, boundaries for the immersion freezing efficiency of CFA.
Future research should also focus on quantifying CFA emissions and temporal and spacial variability of CFA particle concentrations. Mass spectrometry measurements of CFA, as performed in the framework of this study, can help to identify CFA in the atmosphere. However, the classification of single particles still remains difficult because CFA particles are heterogeneous in their composition and not all of them contain a characteristic marker. More composition measurements of atmospheric aerosol and ice crystal residues are needed to better assess the effect of CFA emission on weather and climate.
The datasets are available upon request to the contact author (grawe@tropos.de) and will be stored in a publicly accessible database in the near future.
In consultation with the power plant operators, sample origin shall not be disclosed and distribution is not possible.
For each CFA sample, was determined from LINA measurements with the distilled water that was used to prepare the CFA suspension. Freezing caused by impurities in the distilled water and on the glass slide was accounted for in the following way. Firstly, the number of sites active at a given temperature T per droplet volume Vdrop, was calculated for distilled water (Eq. A1; Vali, 1971). Secondly, this value was subtracted from KCFA(T) (Eq. A2; Umo et al., 2015). Finally, the difference was used to calculate a corrected ns value (Eq. A3), with C the mass concentration of CFA in suspension and ABET the BET specific surface area (see Sect. S8).
The ns, corr values from four measurements were averaged for a mean ns value, i.e., a total number of 360 droplets was investigated for each sample. The uncertainty in the mean ns, given as vertical error bars in Fig. 4, is equal to the standard deviation of the four ns, corr values. The largest possible ns error of the LINA measurements is illustrated in Fig. A1. For this, the uncertainties in concentration from weighing of the CFA sample and pipetting distilled water, as well as BET specific surface area, and volume of the droplets were propagated. Here, the error in fice was assumed to be related to the standard deviation of the Poisson distribution of particles in the suspension.
Data shown in Fig. B1 is taken from Grawe et al. (2016, similar to Fig. 4d) and shows fice(T) for dry- and wet-generated CFA1 particles. Measurements with dry-generated particles are identical to those shown in Fig. 2. Measurements with wet-generated particles from a suspension, prepared as described by Umo et al. (2015), i.e., 10 min of ultrasonification and 24 h of stirring, suggest that CFA1 retains some activity even when being wet generated. fice was found to be around 5 % between −24 and −35 ∘C, indicating no strong temperature dependence.
At this point it was already known that needle-shaped particles are present among wet-generated CFA1 particles. However, it was assumed that the needle-shaped particles are composed of water-soluble material which will dissolve once a needle-shaped particle is immersed in a droplet. fice would be underestimated due to the occurrence of purely water-soluble particles, and according to this hypothesis, fice was multiplied by a scaling factor of 4.54 (, assuming that only 22 % of the droplets contained a water-insoluble particle).
Additional measurements were performed with modified suspensions. When the suspension was prepared without ultrasonification, just stirring, lower fice values around 1 % at −35 ∘C were observed. When filtering the suspension through a 200 nm syringe filter, fice was only slightly above values measured for highly diluted ammonium sulphate droplets, i.e., homogeneous nucleation.
ESEM images (see Figs. S5 and S6) show that CFA1 is indeed the only one of the four CFA samples for which needle-shaped particles form during wet particle generation. Optical microscope images of liquid suspension droplets (see Fig. S13a) show that the needle-shaped particles are even present in the aqueous environment, disproving the earlier hypothesis of water-soluble needle-shaped particles. Even though the substrates were loaded after size selection, needle-shaped particles which are much longer than the selected 300 nm can be seen on the ESEM images and could be introduced into LACIS. This is due to the fact that the dynamic shape factor of the needle-shaped particles differs significantly from unity. The ESEM images suggest that some of the needle-shaped particles are even longer than the usual droplet diameter at the LACIS outlet (which is 5 µm). This represents a challenge for the optical detection with TOPS-Ice, because the determination of fice is based on depolarization, and hence largely on the shape of the hydrometeors. In usual LACIS immersion freezing experiments with 300 nm particles, the supercooled liquid droplets are spherical because a sufficient amount of water vapor is provided to form a thick (with respect to the particle diameter) layer of water on top of the particle upon activation. However, if we imagine an experiment with particles from the CFA1 suspension, the long needle-shaped particles have a much larger surface area that will be covered by water molecules when exposed to same supersaturation with respect to liquid water. As a result, a much thinner water layer is formed which will not be able to “hide” the irregular particle shape. Due to this, there is a fraction of needle-shaped particles longer than 5 µm causing droplets being non-spherical, yet unfrozen. Consequently, depolarization signals are produced, which are associated with ice particles. This artifact can also be observed at T>0 ∘C and thus we falsely interpreted signals caused by long needle-shaped particles as frozen droplets in Grawe et al. (2016), overestimating the immersion freezing efficiency of wet-generated CFA1 particles.
To determine the realistic freezing potential of wet-generated CFA1 particles, the suspension was put through a filter (4–7 µm particle retention, grade 595, Whatman International, Ltd., Maidstone, UK) prior to wet particle generation to remove large needle-shaped particles. As a result, experiments could be conducted with 5 µm sized droplets, which were then spherical when unfrozen. fice was found to be below 0.1 % at −35 ∘C, i.e., the wet-generated CFA1 particles are roughly 3 orders of magnitude less efficient than the dry-generated ones.
Concerning the lower fice values for particles from the CFA1 suspension without ultrasonification from Grawe et al. (2016), it can be hypothesized that, due to the lack of agitation, less of the material responsible for the formation of needle-shaped particles was dissolved from the CFA particles. Consequently, less and/or shorter needle-shaped particles might have formed which would not disturb the spherical shape of the droplets.
SPIN measurements above water saturation (1.03≤Sw< droplet breakthrough) were performed with dry-generated 300 nm particles from all four CFA samples and wet-generated 300 nm particles from CFA1 (see Fig. C1). Measurements with wet-generated particles could only be done for CFA1 due to instrument availability. For comparison to LACIS results, SPIN AF data are shown as measured and additionally multiplied by a factor of 3, based on results from a previous intercomparison campaign including LACIS and SPIN (Burkert-Kohn et al., 2017) and a comparison between a different CFDC and a cloud chamber (DeMott et al., 2015). Corrected data were interpolated for better clarity and are represented by the dashed lines.
The decrease in the transition from dry to wet particle generation, which was observed in LACIS, was also measured with SPIN. Concerning dry-generated CFA particles, there is nearly perfect agreement between LACIS and SPIN for CFA3 and CFA4 after correction. In the case of CFA2, SPIN results are lower than LACIS results, especially for ∘C. The biggest difference is observed for dry-generated particles of CFA1, where SPIN data are significantly below LACIS for ∘C. A possible explanation could be that CFA1 is the sample with the highest amount of Ca, and presumably anhydrite, that will be dissolved once the particles are activated. The LACIS measurements indicate that an activation time of 1.6 s is too short to cause the formation of an adsorbed layer of hydrated ions (as described in Sect. 3.2.5). The residence time of the particles in SPIN is a factor of 6 higher and this could be enough time to dissolve a sufficient amount of anhydrite. The dependency of residence time in SPIN on the thermodynamic conditions in the chamber (9.2 s at ∘C and 8.2 s at ∘C) could explain why the discrepancy between SPIN and LACIS is higher at higher temperatures. An increase in residence time allows for more ions to dissolve from the CFA particle surface at higher temperatures, which consequently leads to a stronger decrease in AF at higher temperatures. This effect is also visible for CFA2. Following this hypothesis, CFA3 and CFA4 do not contain a sufficient amount of anhydrite to form a hydrated shell around the particles within ∼10 s.
The supplement related to this article is available online at: https://doi.org/10.5194/acp-18-13903-2018-supplement.
SG wrote the manuscript with contributions from HCC, SEH, NR, and HW. LACIS measurements and data evaluation were performed by SG, SAB, and JL. LINA measurements and data evaluation were performed by JL and SG. HCC performed ALABAMA measurements and data analysis with the support of JS. SEH sampled particles with the impactor and performed the ESEM/EDX particle analysis together with ME. NR measured with WISDOM and provided BET results. AW performed SPIN measurements and data evaluation. RS performed XRD measurements. SG, SAB, FS, and HW discussed the immersion freezing results and further experiments after the campaign. HW procured the CFA samples and coordinated the campaign. All co-authors proofread and commented on the manuscript.
The authors declare that they have no conflict of interests.
This article is part of the special issue “Results from the ice nucleation research unit (INUIT) (ACP/AMT inter-journal SI)”. It is not associated with a conference.
This research was conducted in the framework of the DFG funded Ice Nuclei
research UnIT (INUIT, FOR1525), WE 4722/1-2, SCHN1138/2-2. We thank the
anonymous suppliers of the CFA samples, the Lippendorf power plant, Mette
Sidelmann and Merete Bilde (Department of Chemistry, Aarhus University,
Denmark) for water-activity measurements, Michael Lorenz (Semiconductor
Physics Group, University of Leipzig, Germany) for providing access to the
XRD instrument, Anke Rödger, Khanneh W. Fomba, Anett Dietze, Susanne Fuchs,
and Dominik van Pinxteren (TROPOS, Leipzig, Germany) for bulk chemical
composition analysis, Xianda Gong (TROPOS, Leipzig, Germany) for helpful
discussions, Roswitha Heller (Leibniz Institute of Surface Modification,
Leipzig, Germany) and Jens Voigtländer (TROPOS, Leipzig, Germany) for
introduction to the optical microscopes, and Thomas Conrath (TROPOS, Leipzig,
Germany) for technical support.
Edited by:
Allan Bertram
Reviewed by: two anonymous referees
Ahmaruzzaman, M.: A review on the utilization of fly ash, Prog. Energ. Combust., 36, 327–363, 2010. a
ASTM: C618 - 17a. Standard specification for coal fly ash and raw or calcined natural pozzolan for use in concrete, Tech. rep., Am. Soc. Test. Mater., West Conshohocken, PA, USA, 2017. a
Atkinson, J. D., Murray, B. J., Woodhouse, M. T., Whale, T. F., Baustian, K. J., Carslaw, K. S., Dobbie, S., O'Sullivan, D., and Malkin, T. L.: The importance of feldspar for ice nucleation by mineral dust in mixed-phase clouds, Nature, 498, 355–358, 2013. a, b, c, d, e, f
Augustin-Bauditz, S., Wex, H., Kanter, S., Ebert, M., Niedermeier, D., Stolz, F., Prager, A., and Stratmann, F.: The immersion mode ice nucleation behavior of mineral dusts: A comparison of different pure and surface modified dusts, Geophys. Res. Lett., 41, 7375–7382, 2014. a, b, c
Bai, T. B., Koster van Groos, A. F., and Guggenheim, S.: Phase transition, dehydration, and melting relationships of portlandite, Am. Mineral., 79, 1223–1226, 1994. a
Brands, M., Kamphus, M., Böttger, T., Schneider, J., Drewnick, F., Roth, A., Curtius, J., Voigt, C., Borbon, A., Beekmann, M., Bourdon, A., Perrin, T., and Borrmann, S.: Characterization of a newly developed aircraft-based laser ablation aerosol mass spectrometer (ALABAMA) and first field deployment in urban pollution plumes over Paris during MEGAPOLI 2009, Aerosol Sci. Tech., 45, 46–64, 2011. a
Brunauer, S., Emmett, P. H., and Teller, E.: Adsorption of gases in multimolecular layers, J. Am. Chem. Soc., 60, 309–319, 1938. a
Budke, C. and Koop, T.: BINARY: an optical freezing array for assessing temperature and time dependence of heterogeneous ice nucleation, Atmos. Meas. Tech., 8, 689–703, https://doi.org/10.5194/amt-8-689-2015, 2015. a
Burkert-Kohn, M., Wex, H., Welti, A., Hartmann, S., Grawe, S., Hellner, L., Herenz, P., Atkinson, J. D., Stratmann, F., and Kanji, Z. A.: Leipzig Ice Nucleation chamber Comparison (LINC): intercomparison of four online ice nucleation counters, Atmos. Chem. Phys., 17, 11683–11705, https://doi.org/10.5194/acp-17-11683-2017, 2017. a, b
Chen, J., Wu, Z., Augustin-Bauditz, S., Grawe, S., Hartmann, M., Pei, X., Liu, Z., Ji, D., and Wex, H.: Ice-nucleating particle concentrations unaffected by urban air pollution in Beijing, China, Atmos. Chem. Phys., 18, 3523–3539, https://doi.org/10.5194/acp-18-3523-2018, 2018. a, b, c, d
Clauß, T., Kiselev, A., Hartmann, S., Augustin, S., Pfeifer, S., Niedermeier, D., Wex, H., and Stratmann, F.: Application of linear polarized light for the discrimination of frozen and liquid droplets in ice nucleation experiments, Atmos. Meas. Tech., 6, 1041–1052, https://doi.org/10.5194/amt-6-1041-2013, 2013. a
Cziczo, D., Stetzer, O., Worringen, A., Ebert, M., Weinbruch, S., Kamphus, M., Gallavardin, S. J., Curtius, J., Borrmann, S., Froyd, K. D., Mertes, S., Möhler, O., and Lohmann, U.: Inadvertent climate modification due to anthropogenic lead, Nat. Geosci., 2, 333–336, 2009. a
Cziczo, D. J., Murphy, D. M., Hudson, P. K., and Thomson, D. S.: Single particle measurements of the chemical composition of cirrus ice residue during CRYSTAL-FACE, J. Geophys. Res., 109, D4, https://doi.org/10.1029/2003JD004032, 2004. a
Cziczo, D. J., Thomson, D. S., Thompson, T. L., DeMott, P. J., and Murphy, D. M.: Particle analysis by laser mass spectrometry (PALMS) studies of ice nuclei and other low number density particles, Int. J. Mass Spectrom., 258, 21–29, 2006. a
Damle, A. S., Ensor, D. S., and Ranade, M. B.: Coal combustion mechanisms: A review, Aerosol Sci. Tech., 1, 119–133, 1982. a, b
Davison, R. L., Natusch, D. F. S., Wallace, J. R., and Evans Jr., C. A.: Trace elements in fly ash: dependence of concentration on particle size, Environ. Sci. Technol., 8, 1107–1113, https://doi.org/10.1021/es60098a003, 1974. a
Deer, W. A., Howie, R. A., and Zussman, J.: An Introduction to the Rock Forming Minerals, 2nd edn., Longman Scientific and Technical, Essex, UK, 1992. a
DeMott, P. J.: An exploratory study of ice nucleation by soot aerosols, J. Appl. Meteorol., 29, 1072–1079, 1990. a
DeMott, P. J., Cziczo, D. J., Prenni, A. J., Murphy, D. M., Kreidenweis, S. M., Thomson, D. S., Borys, R., and Rogers, D. C.: Measurements of the concentration and composition of nuclei for cirrus formation, P. Natl. Acad. Sci. USA, 100, 14 655–14 660, 2003. a
DeMott, P. J., Prenni, A. J., McMeeking, G. R., Sullivan, R. C., Petters, M. D., Tobo, Y., Niemand, M., Möhler, O., Snider, J. R., Wang, Z., and Kreidenweis, S. M.: Integrating laboratory and field data to quantify the immersion freezing ice nucleation activity of mineral dust particles, Atmos. Chem. Phys., 15, 393–409, https://doi.org/10.5194/acp-15-393-2015, 2015. a, b
Diehl, K. and Mitra, S. K.: A laboratory study of the effects of a kerosene-burner exhaust on ice nucleation and the evaporation rate of ice crystals, Atmos. Environ., 32, 3145–3151, 1998. a
Ebert, M., Weigel, R., Kandler, K., Günther, G., Molleker, S., Grooß, J.-U., Vogel, B., Weinbruch, S., and Borrmann, S.: Chemical analysis of refractory stratospheric aerosol particles collected within the arctic vortex and inside polar stratospheric clouds, Atmos. Chem. Phys., 16, 8405–8421, https://doi.org/10.5194/acp-16-8405-2016, 2016. a
Emersic, C., Connolly, P. J., Boult, S., Campana, M., and Li, Z.: Investigating the discrepancy between wet-suspension- and dry-dispersion-derived ice nucleation efficiency of mineral particles, Atmos. Chem. Phys., 15, 11311–11326, https://doi.org/10.5194/acp-15-11311-2015, 2015. a
endcoal.org: Coal plants by country (Units) – July 2017, available at: https://endcoal.org/wp-content/uploads/2017/07/PDFs-for-GCPT-July-2017-Countries-Units.pdf, last access: 25 October 2017. a
Enders, M.: The CaO distribution to mineral phases in a high calcium fly ash from Eastern Germany, Cement Concrete Res., 26, 243–251, 1996. a, b
Flagan, R. C. and Seinfeld, J. H.: Fundamentals of Air Pollution Engineering, chap. Particle formation in combustion, 358–390, Prentice-Hall, Inc., Englewood Cliffs, New Jersey, 1988a. a, b
Flagan, R. C. and Seinfeld, J. H.: Fundamentals of Air Pollution Engineering, chap. Removal of Particles from Gas Streams, 391–478, Prentice-Hall, Inc., Englewood Cliffs, New Jersey, 1988b. a, b
Fornea, A. P., Brooks, S. D., Dooley, J. B., and Saha, A.: Heterogeneous freezing of ice on atmospheric aerosols containing ash, soot and soil, J. Geophys. Res., 114, D13201, https://doi.org/10.1029/2009JD011958, 2009. a
Freney, E. J., Martin, S. T., and Buseck, P. R.: Deliquescence and efflorescence of potassium salts relevant to biomass-burning aerosol particles, Aerosol Sci. Tech., 43, 799–807, 2009. a
Gallavardin, S. J., Lohmann, U., and Cziczo, D. J.: Analysis and differentiation of mineral dust by single particle laser mass spectrometry, Int. J. Mass Spectrom., 274, 56–63, 2008. a
Garimella, S.: A vertically-integrated approach to climate science: From measurements and machine learning to models and policy, PhD thesis, Massachusetts Institute of Technology, Cambridge, MA, USA, 2016. a, b, c, d, e, f, g, h, i, j, k, l, m
Garimella, S., Kristensen, T. B., Ignatius, K., Welti, A., Voigtländer, J., Kulkarni, G. R., Sagan, F., Kok, G. L., Dorsey, J., Nichman, L., Rothenberg, D. A., Rösch, M., Kirchgäßner, A. C. R., Ladkin, R., Wex, H., Wilson, T. W., Ladino, L. A., Abbatt, J. P. D., Stetzer, O., Lohmann, U., Stratmann, F., and Cziczo, D. J.: The SPectrometer for Ice Nuclei (SPIN): an instrument to investigate ice nucleation, Atmos. Meas. Tech., 9, 2781–2795, https://doi.org/10.5194/amt-9-2781-2016, 2016. a
Grawe, S., Augustin-Bauditz, S., Hartmann, S., Hellner, L., Pettersson, J. B. C., Prager, A., Stratmann, F., and Wex, H.: The immersion freezing behavior of ash particles from wood and brown coal burning, Atmos. Chem. Phys., 16, 13911–13928, https://doi.org/10.5194/acp-16-13911-2016, 2016. a, b, c, d, e, f, g, h, i, j, k, l, m, n
Gražulis, S., Chateigner, D., Downs, R. T., Yokochi, A. F. T., Quirós, M., Lutterotti, L., Manakova, E., Butkus, J., Moeck, P., and Le Bail, A.: Crystallography Open Database – an open-access collection of crystal structures, J. Appl. Crystallogr., 42, 726–729, 2009. a
Hartmann, S., Niedermeier, D., Voigtländer, J., Clauss, T., Shaw, R. A., Wex, H., Kiselev, A., and Stratmann, F.: Homogeneous and heterogeneous ice nucleation at LACIS: operating principle and theoretical studies, Atmos. Chem. Phys., 11, 1753–1767, https://doi.org/10.5194/acp-11-1753-2011, 2011. a
Havlíček, D., Přibil, R., and Školoud, O.: The chemical and mineralogical composition of the water-soluble fraction of power-plant ash and its effect on the process of crystallization of water, Atmos. Environ., 27A, 655–660, 1993. a, b, c, d, e
Hiranuma, N., Augustin-Bauditz, S., Bingemer, H., Budke, C., Curtius, J., Danielczok, A., Diehl, K., Dreischmeier, K., Ebert, M., Frank, F., Hoffmann, N., Kandler, K., Kiselev, A., Koop, T., Leisner, T., Möhler, O., Nillius, B., Peckhaus, A., Rose, D., Weinbruch, S., Wex, H., Boose, Y., DeMott, P. J., Hader, J. D., Hill, T. C. J., Kanji, Z. A., Kulkarni, G., Levin, E. J. T., McCluskey, C. S., Murakami, M., Murray, B. J., Niedermeier, D., Petters, M. D., O'Sullivan, D., Saito, A., Schill, G. P., Tajiri, T., Tolbert, M. A., Welti, A., Whale, T. F., Wright, T. P., and Yamashita, K.: A comprehensive laboratory study on the immersion freezing behavior of illite NX particles: a comparison of 17 ice nucleation measurement techniques, Atmos. Chem. Phys., 15, 2489–2518, https://doi.org/10.5194/acp-15-2489-2015, 2015. a
Hoose, C. and Möhler, O.: Heterogeneous ice nucleation on atmospheric aerosols: a review of results from laboratory experiments, Atmos. Chem. Phys., 12, 9817–9854, https://doi.org/10.5194/acp-12-9817-2012, 2012. a, b
Huffman, J. A., Sinha, B., Garland, R. M., Snee-Pollmann, A., Gunthe, S. S., Artaxo, P., Martin, S. T., Andreae, M. O., and Pöschl, U.: Size distributions and temporal variations of biological aerosol particles in the Amazon rainforest characterized by microscopy and real-time UV-APS fluorescence techniques during AMAZE-08, Atmos. Chem. Phys., 12, 11997–12019, https://doi.org/10.5194/acp-12-11997-2012, 2012. a
Jaenicke, R.: Über die Dynamik atmosphärischer Aitkenteilchen, Berich. Bunsen Gesell., 82, 1198–1202, https://doi.org/10.1002/bbpc.19780821126, 1978. a
Kamphus, M., Ettner-Mahl, M., Klimach, T., Drewnick, F., Keller, L., Cziczo, D. J., Mertes, S., Borrmann, S., and Curtius, J.: Chemical composition of ambient aerosol, ice residues and cloud droplet residues in mixed-phase clouds: single particle analysis during the Cloud and Aerosol Characterization Experiment (CLACE 6), Atmos. Chem. Phys., 10, 8077–8095, https://doi.org/10.5194/acp-10-8077-2010, 2010. a, b
Kanji, Z. A., Ladino, L. A., Wex, H., Boose, Y., Burkert-Kohn, M., Cziczo, D. J., and Krämer, M.: Overview of Ice Nucleating Particles, Meteor. Mon., 58, 1.1–1.33, https://doi.org/10.1175/AMSMONOGRAPHS-D-16-0006.1, 2017. a
Kim, J.-H., Yoo, H.-J., Hwang, Y.-S., and Kim, H.-G.: Removal of particulate matter in a tubular wet electrostatic precipitator using a water collection electrode, Sci. World J., ID 532354, https://doi.org/10.1100/2012/532354, 2012. a
Klimchouk, A.: The dissolution and conversion of gypsum and anhydrite, Int. J. Speleol., 25, 21–36, 1996. a
Laskin, A., Cowin, J. P., and Iedema, M. J.: Analysis of individual environmental particles using modern methods of electron microscopy and X-ray microanalysis, J. Electron. Spectrosc., 150, 260–274, 2006. a
Matsunaga, T., Kim, J. K., Hardcastle, S., and Rohatgi, P. K.: Crystallinity and selected properties of fly ash particles, Adv. Mater Res.-Switz., 325, 333–343, https://doi.org/10.1016/S0921-5093(01)01466-6, 2002. a
Mueller, S. F., Mallard, J. W., Mao, Q., and Shaw, S. L.: Fugitive particulate emission factors for dry fly ash disposal, Japca J. Air Waste Ma., 63, 806–818, 2013. a
Nóbrega, S. W., Falaguasta, M. C. R., and Coury, J. R.: A study of a wire-plate electrostatic precipitator operating in the removal of polydispersed particles, Braz. J. Chem. Eng., 21, 275–284, 2004. a
Parungo, F. P., Ackerman, E., Proulx, H., and Pueschel, R. F.: Nucleation properties of fly ash in a coal-fired power-plant plume, Atmos. Environ., 12, 929–935, 1978. a, b, c, d
Petters, M. D. and Wright, T. P.: Revisiting ice nucleation from precipitation samples, Geophys. Res. Lett., 42, 8758–8766, 2015. a, b, c, d
Querol, X., Alastuey, A., Lopez-Soler, A., Mantilla, E., and Plana, F.: Mineral composition of atmospheric particulates around a large coal-fired power station, Atmos. Environ., 30, 3557–3572, 1996. a
Reff, A., Bhave, P. V., Simon, H., Pace, T. G., Pouliot, G. A., Mobley, J. D., and Houyoux, M.: Emissions inventory of PM2.5 trace elements across the United States, Environ. Sci. Technol., 43, 5790–5796, https://doi.org/10.1021/es802930x, 2009. a
Reicher, N., Segev, L., and Rudich, Y.: The WeIzmann Supercooled Droplets Observation on a Microarray (WISDOM) and application for ambient dust, Atmos. Meas. Tech., 11, 233–248, https://doi.org/10.5194/amt-11-233-2018, 2018. a
Roth, A., Schneider, J., Klimach, T., Mertes, S., van Pinxteren, D., Herrmann, H., and Borrmann, S.: Aerosol properties, source identification, and cloud processing in orographic clouds measured by single particle mass spectrometry on a central European mountain site during HCCT-2010, Atmos. Chem. Phys., 16, 505–524, https://doi.org/10.5194/acp-16-505-2016, 2016. a
Schmidt, S., Schneider, J., Klimach, T., Mertes, S., Schenk, L. P., Kupiszewski, P., Curtius, J., and Borrmann, S.: Online single particle analysis of ice particle residuals from mountain-top mixed-phase clouds using laboratory derived particle type assignment, Atmos. Chem. Phys., 17, 575–594, https://doi.org/10.5194/acp-17-575-2017, 2017. a
Schmitz, C. H. J., Rowat, A. C., Köster, S., and Weitz, D. A.: Dropspots: a picoliter array in a microfluidic device, Lab Chip, 9, 44–49, 2009. a
Schnell, R. C., Valin, C. C. V., and Pueschel, R. F.: Atmospheric ice nuclei: No detectable effect from a coal-fired powerpower plume, Geophys. Res. Lett., 3, 657–660, 1976. a
Sedlacek III, A. J., Buseck, P. R., Adachi, K., Onasch, T. B., Springston, S. R., and Kleinman, L.: Formation and evolution of tar balls from northwestern US wildfires, Atmos. Chem. Phys., 18, 11289–11301, https://doi.org/10.5194/acp-18-11289-2018, 2018. a
Sievert, T., Wolter, A., and Singh, N. B.: Hydration of anhydrite of gypsum (CaSO4. II) in a ball mill, Cement Concrete Res., 35, 623–630, 2005. a
Sihvonen, S. K., Schill, G. P., Lyktey, N. A., Veghte, D. P., Tolbert, M. A., and Freedman, M. A.: Chemical and physical transformations of aluminosilicate clay minerals due to acid treatment and consequences for heterogeneous ice nucleation, J. Phys. Chem. A, 118, 8787–8796, https://doi.org/10.1021/jp504846g, 2014. a
Smil, V.: Energy in Nature and Society: General Energetics of Complex Systems, MIT Press, Cambridge, MA, USA, 2008. a
Sullivan, R. C., Moore, M. J. K., Petters, M. D., Kreidenweis, S. M., Qafoku, O., Laskin, A., Roberts, G. C., and Prather, K. A.: Impact of particle generation method on the apparent hygroscopicity of insoluble mineral particles, Aerosol Sci. Tech., 44, 830–846, 2010. a, b
Szyrmer, W. and Zawadzki, I.: Biogenic and anthropogenic sources of ice-forming nuclei: A review, B. Am. Meteorol. Soc., 78, 209–228, 1997. a
U. S. Energy Information Administration: International Energy Outlook 2017, available at: https://www.eia.gov/outlooks/ieo/pdf/0484(2017).pdf, last access: 24 October 2017. a
Umo, N. S., Murray, B. J., Baeza-Romero, M. T., Jones, J. M., Lea-Langton, A. R., Malkin, T. L., O'Sullivan, D., Neve, L., Plane, J. M. C., and Williams, A.: Ice nucleation by combustion ash particles at conditions relevant to mixed-phase clouds, Atmos. Chem. Phys., 15, 5195–5210, https://doi.org/10.5194/acp-15-5195-2015, 2015. a, b, c, d, e, f, g, h, i, j, k, l, m, n, o, p, q
Vali, G.: Quantitative evaluation of experimental results on the heterogeneous freezing nucleation of supercooled liquids, J. Atmos. Sci., 28, 402–409, 1971. a
Weinbruch, S., Ebert, M., Gorzawski, H., Dirsch, T., Berg, T., and Steinnes, E.: Characterisation of individual aerosol particles on moss surfaces: implications for source apportionment, J. Environ. Monitor., 12, 1064–1071, 2010. a
Weinbruch, S., Wiesemann, D., Ebert, M., Schütze, K., Kallenborn, R., and Ström, J.: Chemical composition and sources of aerosol particles at Zeppelin Mountain (Ny Ålesund, Svalbard): An electron microscopy study, Atmos. Environ., 49, 142–150, 2012. a
Whale, T. F., Murray, B. J., O'Sullivan, D., Wilson, T. W., Umo, N. S., Baustian, K. J., Atkinson, J. D., Workneh, D. A., and Morris, G. J.: A technique for quantifying heterogeneous ice nucleation in microlitre supercooled water droplets, Atmos. Meas. Tech., 8, 2437–2447, https://doi.org/10.5194/amt-8-2437-2015, 2015. a, b
Yi, H., Guo, X., Hao, J., Duan, L., and Li, X.: Characteristics of inhalable particulate matter concentration and size distribution from power plants in China, Japca J. Air Waste Ma., 56, 1243–1251, 2006. a, b
Zolles, T., Burkart, J., Häusler, T., Pummer, B., Hitzenberger, R., and Grothe, H.: Identification of ice nucleation active sites on feldspar dust particles, J. Phys. Chem. A, 119, 2692–2700, 2015. a, b, c, d, e, f, g
Electrostatic precipitators work on the principle of charging the particles and subsequently sending the flow through an electric field. Particles then migrate to the oppositely charged electrode and hence particulate matter is removed from the flue gas (Flagan and Seinfeld, 1988b). The precipitator itself does not alter particle properties like morphology or chemical composition, only number and mass size distributions are changed (Yi et al., 2006). However, it has been argued that particles which are not captured potentially contain a larger amount of species condensing from the gas phase onto the CFA surface upon cooling (Parungo et al., 1978).
- Abstract
- Introduction
- Materials and methods
- Results and discussion
- Atmospheric implications
- Summary and conclusions
- Data availability
- Sample availability
- LINA water background correction, ns calculation, and error estimation
- Appendix B: Comment on misinterpreted LACIS measurements with wet-generated CFA1 particles published in Grawe et al. (2016) and correction of those
- Appendix C: Potential influence of residence time on immersion freezing efficiency of dry-generated CFA
- Author contributions
- Competing interests
- Special issue statement
- Acknowledgements
- References
- Supplement
- Abstract
- Introduction
- Materials and methods
- Results and discussion
- Atmospheric implications
- Summary and conclusions
- Data availability
- Sample availability
- LINA water background correction, ns calculation, and error estimation
- Appendix B: Comment on misinterpreted LACIS measurements with wet-generated CFA1 particles published in Grawe et al. (2016) and correction of those
- Appendix C: Potential influence of residence time on immersion freezing efficiency of dry-generated CFA
- Author contributions
- Competing interests
- Special issue statement
- Acknowledgements
- References
- Supplement